- 1School of Civil Engineering, Henan Polytechnic University, Jiaozuo, China
- 2School of Surveying and Land Information Engineering, Henan Polytechnic University, Jiaozuo, China
With the rapid development of high-speed railways in China, it is inevitable that some of the lines will have to traverse through the mine goaf ground, and there is little research on whether the “activation” of the foundation of the mine goaf ground occurs under the influence of train loads. In order to provide a safe and reliable basis for the construction of high-speed railway in mine goaf ground, a new classification method of mine goaf ground activation is proposed considering the stability and railway influence. First, the stability evaluation system of the mine goaf site is established with 3 primary indexes and 12 secondary indexes. The 47 groups’ data of the mine goaf ground site are collected as learning samples. Five machine learning methods including decision tree, discriminant analysis, support vector machine, and classifier ensemble are used to learn and test the data. The optimal algorithm is selected and the stability evaluation model is established to classify the stability of the mine goaf site. Second, influencing factors of railway are graded to establish an extension comprehensive evaluation model. Finally, based on the above two models, a new classification method of high-speed railway goaf ground activation considering the two factors and five sub-factors is proposed. Through the verification of two engineering examples, the prediction result of this method is “easily activation” and the need to treat the goaf area, and the actual construction is also taken to grouting treatment, proving that the method has certain guiding significance for the project.
1 Introduction
With the acceleration of China’s railway network construction, construction conditions faced by railway engineering are becoming more and more demanding. Taking Shanxi Province as an example, due to its rich coal resources, after years of intensive mining, it has formed nearly 2300 km2 of goaf areas, the size of which makes it inevitable that many planned high-speed railway lines will have to pass through these goaf areas (Figure 1A). When the high-speed railway passes through the mine goaf ground (Figure 1B), the influence of the train load may lead to the separation closure or the instability of the stable structure in the mine goaf ground (Du et al., 2020), which leads to the “activation” deformation of the foundation and the uneven settlement of the high-speed railway subgrade, thus affecting the safety of the train operation (Jiang and Wang, 2019).
Many scholars have conducted extensive research on the stability of underground spaces and disaster prevention (Liu et al., 2020, 2021). Helm et al. (2013) established 1,320 numerical simulation models to analyze the influence of shallow-buried room-and-pillar mine goaf ground on surface traffic facilities, and pointed out that seasonal water-level changes would have a great impact on the stability of overlying strata and surface in the mine goaf ground. Liu et al. (2018, 2019) monitored the rock burst of the tunnel using the acoustic emission technology and infrared monitoring equipment. Cui et al. (2014) and Bell et al. (2001) analyzed the collapse instability cases of shallow mining areas after partial mining, and revealed that the partial mining instability was caused by the decrease of residual coal pillar strength, which made the internal and external stress arches interrelated. Li et al. (2016) proposed a calculation method of release space based on the pore distribution characteristics of rock strata above mine goaf ground and applied it to engineering practice to prove the effectiveness of this method. Hu and Li (2012) selected four indicators as the risk identification factors of mine goaf ground stability and proposed a mine risk analysis method based on Bayesian discriminant analysis. Through the training of 40 samples, the model accuracy reached 0.025. Qin et al. (2019) proposed an improved tradabost algorithm and introduced the concept of dynamic factor to improve the generalization ability of the algorithm for different mine goaf ground samples. The algorithm can also maintain high accuracy with fewer samples. Guo et al. (2019), based on fuzzy theory, established the instability risk evaluation model of an expressway construction site in the mine goaf ground. The weight and membership degree were determined by the gray correlation method and the Delphi method. The reliability of the model was verified by subgrade settlement monitoring data.
Although there has been some research into the stability of the mine goaf ground and the effects of external loads on the mine goaf ground, there has been less research into the “activation” of mine goaf ground of high-speed railway. The foundation problem of the mine goaf ground is an extremely complex problem, which is not only affected by mining technology, burial depth, and mining height but also by hydrogeology, spatial location distribution, and external load. In order to establish a reasonable evaluation system of high-speed railway mine goaf ground activation, first, collect a large number of mine goaf ground data, select the evaluation index of mine goaf ground stability, adopt the classification algorithm of machine learning to learn the samples, and complete the classification of stability grade so as to determine the stability grade of the mine goaf ground. Then, the information entropy-extension comprehensive evaluation model is established (Xie et al., 2021), and the mine goaf ground activation grade is obtained by combining the stability of the mine goaf ground and the influence of train load.
Compared with traditional evaluation methods, it not only solves the problem of subjective selection of weights in the comprehensive evaluation method but also solves the problem of difficult selection of parameters and complicated calculation in the mechanical analysis method.
2 Stability Evaluation of Mine Goaf Ground
2.1 Evaluation Index System
The deformation of a mining site is an extremely complex process, and the most critical step in establishing a scientific index system for evaluating the stability of a mine goaf ground is to fully consider the impact of various factors on the mine goaf ground. Guo et al. (2019) and Zhao et al. (2021) established different evaluation index systems of mine goaf ground stability. In this study, the above three evaluation index systems are comprehensively considered, and the evaluation index system of mine goaf ground stability is established as shown in Figure 2. Compared with other evaluation systems, the two factors of final mining time and loose layer thickness are added to the consideration. The final mining time is related to the residual deformation of the mine goaf ground, which plays a relatively important role in the construction of the mine goaf ground site, and the thickness of the loose layer also has a certain influence. Many scholars have found that the loose layer will form a loose arch structure under a certain thickness, and bear part of the external load.
2.2 Stability Classification
To be better combined with the analysis of train load, this study combined with the code for investigation of geotechnical engineering in the coal mine goaf (GB51044-2014, 2014) and the research of some scholars (Gong et al., 2008; Song et al., 2020;Song D. et al., 2021; Song DQ. et al., 2021). The stability grade of the mine goaf ground is divided into four grades according to Table 1, and the qualitative index grade is divided and assigned according to Table 2.
2.3 Filtering Algorithms
Machine learning and deep learning are the hotspots of artificial intelligence research, and many related algorithms and theories have been applied in many geotechnical engineering (Cai et al., 2020, He et al., 2020; Lawal and Kwon, 2021; Tarawneh et al., 2018). In the era of big data, the ability of machine learning to mine effective information in the data has been greatly improved. In order to better evaluate the stability of the mine goaf ground and excavate the key factors, this study selects five machine learning methods, namely, decision tree, discriminant analysis, support vector machine, nearest neighbor classifier, and classifier ensemble, to process the data, and selects AUC value (area under curve) and accuracy as the criteria for the evaluation algorithm. AUC (Toh et al., 2008) is defined as the area under the ROC curve, which is usually used as the evaluation standard of the machine learning model. The abscissa of the ROC curve is the false positive rate and the ordinate is the true positive rate.
The index data of mine goaf ground site in reference and the evaluation grade of mine goaf ground site based on unascertained mathematics and actual working conditions are used (Gong et al., 2008).
This study selected five algorithms and twenty models for training. The accuracy and AUC of each model are shown in Figure 3 and Table 3.
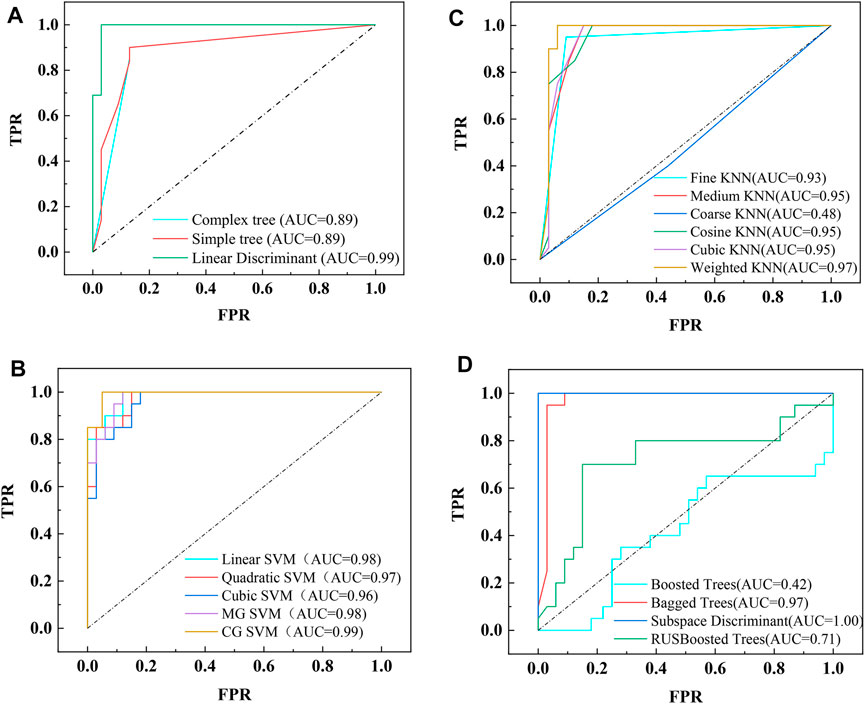
FIGURE 3. Performance of AUC values of each model. (A) Decision tree and discriminant analysis, (B) support vector machine, (C) K-nearest neighbor, and (D) classifier ensemble.
It can be seen from Figure 3 and Table 3 that the accuracy of Subspace Discriminant and Quadratic SVM models in the integrated classifier algorithm is the highest, but the AUC value of the former reaches 1.00, which belongs to the perfect classifier. Therefore, this algorithm model is used to evaluate and predict the stability of the mine goaf ground.
3 Classification of High-Speed Railway Influence
The main problem faced by the construction of high-speed railway in the mine goaf ground is whether the project will cause the activation deformation of the mine goaf ground, and when the activation deformation occurs, whether the deformation will affect the train operation. The influence of railway engineering on the foundation of mine goaf ground generally has four aspects, namely, the train axle load (Shi and Hou, 2017), train speed (Bratov et al., 2014; Lamas-Lopez et al., 2016), track type (Connolly et al., 2020) and the distance between the railway line and the surface center of the mine goaf ground. In this study, according to the relevant norms (GB 51044-2014, 2014), the impact of each factor is divided into four levels.
3.1 Train Axle Load
The axle loads of different types of trains are also different. The greater the axle load of the train, the greater the force on the foundation of the goaf, and the deeper the influence depth. When the influence depth of the force reaches the fault zone area, it will have a safety impact on the use of buildings on the foundation of the mine goaf ground. The influence of axle load is analyzed by FLAC3D software to simulate the disturbance depth of train load under different axle load conditions. As shown in Figure 4, the influence depth of train is linearly related to the change of axle load. At present, the range of axle load in China is between 140–300 kN, considering the full load and no-load running vehicles. The influence degree of this classification and the corresponding axle load range are shown in Table 4.
3.2 Railway Speed
Train speed is the most obvious and indigenous factor affecting the dynamic load inside the subgrade of high-speed trains, and the magnitude of the dynamic load inside the subgrade is an important factor to determine whether the foundation in the mine goaf ground activated deformation. Based on the field measurement data, Bian et al. (2014) found that when the vehicle speed was lower than 150 km/h or higher than 300 km/h, the dynamic stress of subgrade no longer changed with the speed. When the train speed was between 150 and 300 km/h, the dynamic stress of the subgrade changed linearly with the vehicle speed. The influence degree and speed range of this classification are shown in Table 5.
3.3 Track Type
The influence of track mainly lies in the selection of track type (Li et al., 2021) and the height of subgrade (Mosayebi et al., 2017). Li et al. (2018) proposed the attenuation formula of subgrade dynamic stress along depth as shown in Eq. 1:
where
Since there are many types of orbital structures, four representative orbital structures are selected and divided into four grades according to Table 6.
3.4 Railway Line Location
Through the study, Qian et al. (1996) found that after the mine goaf ground was mined, a region similar to the masonry beam structure appeared at both ends of the mine goaf ground. The length of this region was approximately 8 times the periodic pressure step distance of the working face cycle, and the stability of the masonry beam structure was mainly determined by the key block (Cao and Zhou, 2015). According to this study, the mine goaf ground is horizontally divided into four regions. As shown in Figure 5, the risk is small when the region far from the mine goaf ground boundary is constructed. It is most dangerous to build in the key block area of a masonry beam, which may make the masonry beam structure unstable. In the construction of the non-key block area of the masonry beam structure, although there is cushion support, the risk is still large. When the central area of the mine goaf ground is constructed, most of the area has been compacted, there are no large cracks and separate layers, and the risk is small.
To study the different impacts of different distances between the railway trunk line and the surface center of the mine goaf ground, the influence degree is divided according to Table 7.
4 Extension Evaluation Model
Extension theory is a method proposed to solve complex problems by combining matter-element theory with extension set theory (Smarandache, 2012). The extension comprehensive evaluation model has been widely used in various fields.
In this article, the influence factors of mine goaf ground are simplified by the machine learning method, and the influence of the mine goaf ground is evaluated by the stability grade of the mine goaf ground site. The extension evaluation system of foundation activation for mine goaf ground of high-speed railway is constructed as shown in Figure 6. Referring to the specifications and research results, combined with the classification of the stability grade of the mine goaf ground and the train influence grade, the goaf foundation activation grade is divided into four grades (Table 8):
4.1 Identification of Classic and Joint Domains
Let
where N0j represents level j, cn represents each evaluation index of the model, and V0ji represents the value range of each evaluation index at level j.
According to the division of Tables 4–7, the classical domain construction is carried out. Because the track type cannot be quantitatively assigned, this study takes (0, 2), (2, 4), (4, 6), and (6, 8) as characteristic value ranges. When the track type is I, II, III, and IV, then 1, 3, 5, and 7 are given as the evaluation values, and the mine goaf ground stability is also treated according to this method. The influence of the main line position is related to the factors of the mine goaf ground itself, which needs to be calculated according to the actual working conditions.
The classical domain matter elements of mine goaf ground influencing each level established in this study are
R01 = [N01 Stability grade of mine goaf ground (0,2)]
R02 = [N02 Stability grade of mine goaf ground (2,4)]
R03 = [N03 Stability grade of mine goaf ground (4,6)]
R04 = [N04 Stability grade of mine goaf ground (6,8)]
The joint domain matter element is
RD = [D Stability grade of mine goaf ground (0,8)]
The classical domain matter elements of the train influencing each level are
The joint domain matter element is
4.2 Matter Element
The data of the evaluation object ai are represented by matter elements, and the matter element Ri to be evaluated is obtained.
where pi is the ith evaluation object and vij is the value of pi with respect to characteristic index cj.
4.3 Index Weight
Evaluation of x requires I1, I2, ..., Im, m indicators, xj is the measured value of x on Ij. For xij, there are p evaluation grades c1, c2, … , cn, and let μjk = μ (xj ∈ ck) denote the degree to which xj belongs to the kth evaluation class ck.
satisfies that μ of Eqs 2–4 is an unascertained measure, and the matrix
is called a single index measure evaluation matrix.
According to the influence degree division of 3.1–3.4 subsections, the single index measure function diagram of each factor can be obtained. As shown in Figure 7, the single index measure evaluation matrix of the instance can be obtained by substituting the instance data.
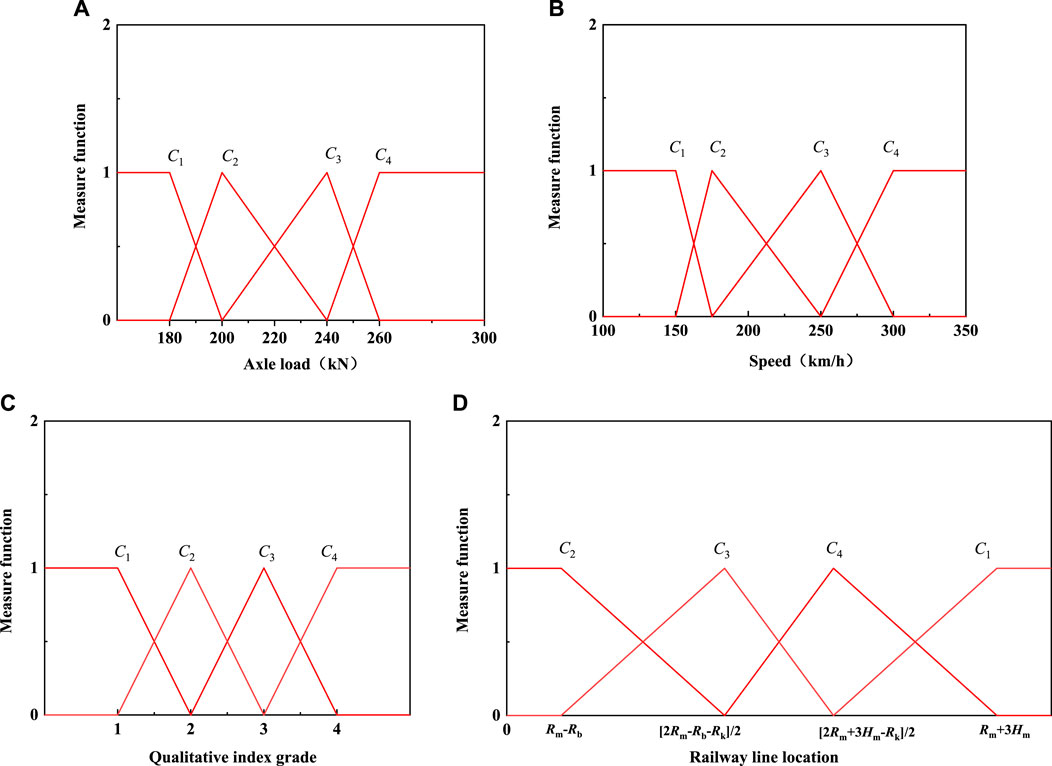
FIGURE 7. Uncertainty measurement function of different factors: (A) axle load, (B) train speed, (C) qualitative index grade, and (D) railway line location.
After getting the single index measurement matrix, it is necessary to determine the weight of each index. To make wj a weight of xj, wj needs to satisfy two conditions:
① 0 ≤ wj ≤ 1
② w1+ w2+…+ wm = 1.
At this time, the information entropy theory is used to calculate the weight, and the calculation steps are shown in Eqs 5, 6.
The determination of the index weight coefficient in the first layer of the extension system considers the value of other scholars in the evaluation system of goaf foundation stability. It is known that the weight of building load or traffic load is mostly between 0.116 (Zhang, 2009) and 0.232 (Wang, 2016). In this study, 0.2 and 0.8 are selected as the weight coefficients of train load and goaf stability, respectively.
4.4 Correlation Function
The calculation formula of the distance between point and interval is
When vki ∉ V0ji, the correlation function is
When vki ∈V0ji, the correlation function is
4.5 Correlation Degree
To facilitate analysis and comparison, the correlation degree is standardized by Eq. 10.
4.6 Activation Classification
The weight coefficient of each eigenvalue and the normalized correlation degree are calculated according to Eq. 11, and the comprehensive correlation degree of the evaluation object is obtained.
If
then the activation level of evaluation object p is k.
5 Case Study
5.1 Case 1
Case 1 selects a section of the Tai-Jiao high-speed railway crossing the mine goaf ground site project to verify the established classification method of mine goaf ground activation. The length, width, and mining thickness of the mine goaf ground under the section of Tai-Jiao higher railway are about 110 m, 18, and 1.8 m, respectively. The overall buried depth of the mine goaf ground is 56 m. The wall rock is flooded for a long time, the rock mass structure is relatively loose, and the joint fissures are developed. There is an adjacent mine goaf ground near the section. The dip angle of the ore layer is 12°, the area ratio of the ore pillar is 15%, the stop mining time is 20 a, and the thickness of the loose layer is 15 m. The axle load of a high-speed railway is 17 t, and the designed speed is 250 km/h. The low subgrade of the ballasted track is used in the track, and the railway line is about 20 m away from the center of the mine goaf ground.
5.1.1 Stability Evaluation
According to the comparison test of the machine learning algorithm model, the subspace discriminant algorithm model is selected as the classifier for evaluating the stability of mine goaf ground. The input matrix of the engineering example to be verified is compiled in Matlab. According to the engineering situation, the input matrix is
The input matrix is imported into the ensemble classification learner, and the stability evaluation level of the mine goaf ground is grade III.
5.1.2 Activation Evaluation of Mine Goaf Ground
5.1.2.1 Extension Evaluation of Mine Goaf Ground Stability
The matter element to be evaluated is
R1 = [p1 Stability grade of mine goaf ground 5].
The correlation matrix K1 is obtained by calculating with Eqs 7–9.
According to Eq. 10, correlation matrix K`1 is obtained.
According to Eq. 11, the comprehensive correlation degree is calculated, and matrix Kp1 is obtained. Since this evaluation layer has only one index, the weight coefficient is 1.
5.1.2.2 Extension Evaluation of Train Impact
According to the general situation of the project, the matter element to be evaluated of Tai-Jiao high-speed railway is
The correlation matrix K2 is obtained by calculating with Eqs 7–9.
According to Eq. 10, correlation matrix K`2 is obtained.
Through the measure function of Figure 6, the single index evaluation measure matrix of train influencing factors is determined:
It can be seen from Eqs 5, 6 that
According to Eq. 11, comprehensive correlation matrix Kp1 of the Tai-Jiao high-speed railway is calculated as follows:
5.1.2.3 Extensible Pre-Evaluation of Ground Activation Grade in Mine Goaf Ground
According to Eq. 11, the comprehensive evaluation of the first level of Tai-Jiao high-speed railway is as follows:
According to Eq. 12, the activation grade of mine goaf ground in the Tai-Jiao high railway section is “Easily activated.” In practical engineering, through a large number of exploration and tests in the early stage, the grouting treatment of goaf is decided. At present, the operation condition of this section is well, which proves that the method in this article has a certain reference value.
5.2 Case 2
In the second engineering case, a section of the Nan-Qin railway crossing the mine goaf ground along the coast of Guangxi is selected. The buried depth of the mine goaf ground under the Nan-Qin railway is 142 m, and the mining thickness is 5 m. The buried rock structure is loose, and the mine goaf ground is rich in water and high in water pressure. The dip angle of the coal seam is 20°, and the area ratio of the pillar is 15%. The stop mining time is 10 a, and the thickness of the loose layer is 10 m.
The axle load of the railway is 20 t, and the designed speed is 250 km/h. The low subgrade of the ballasted track is used in the track, and the Nan-Qin line is about 70 m according to the center of mine goaf ground.
5.2.1 Site Stability Evaluation
The input matrix of Nan-Qin railway is
The input matrix is imported into the ensemble classification learner, and the stability evaluation level of the mine goaf ground is grade III.
5.2.2 Activation Evaluation of Mine Goaf Ground
5.2.2.1 Extension Evaluation of Mine Goaf Ground Stability
According to the evaluation results of machine learning, the evaluation results of two engineering examples are the same, and the extension evaluation results should be the same. So, the correlation matrix is the same as that of instance one.
5.2.2.2 Extension Evaluation of Train Impact
According to the general situation of the project, the matter element to be evaluated of Nan-Qin high-speed railway is
Correlation matrix K2 is obtained by calculating with Eqs 7–9.
According to Eq. 10, correlation matrix K`2 is obtained.
Through the measure function of Figure 6, the single index evaluation measure matrix of the train influencing factors is determined:
It can be seen from Eqs 5, 6 that
According to Eq. 11, comprehensive correlation matrix Kp1 of Tai-Jiao high-speed railway is calculated as follows:
5.2.2.3 Extensible Pre-Evaluation of Ground Activation Grade in Mine Goaf Ground
According to Eq. 11, the comprehensive evaluation of the first level of Nan-Qin high-speed railway is as follows:
According to Eq. 12, the activation grade of the mine goaf ground in the Nan-Qin high railway section is “Easily activated.” In the engineering construction, through the investigation of the site and the verification of the geological data, the constructor adopted the grouting method to reinforce the goaf. In the years of train operation, there was no activation deformation in the site, which proved that the research method in this article was effective.
6 Conclusion
Coal is the main energy in China, and the main coal base formed a large area of mine goaf ground due to high strength mining; at the same time, with the rapid layout of China’s high-speed railway network, some lines will inevitably cross the mine goaf ground, and whether the mine goaf ground is activated under the influence of the train load is an important research direction. Considering the stability of the mine goaf ground and the influence of train, a new classification method of ground activation in the mine goaf ground is proposed. The main conclusions are as follows:
1) The evaluation of the mine goaf ground activation is divided into the evaluation of the stability level of the mine goaf ground and the evaluation of the train impact level, and the overall evaluation is completed by combining the two evaluation results through the extension theory and the unascertained measure theory, which greatly simplifies the evaluation process of the activation level of the mine goaf ground.
2) Through numerical simulation, theoretical derivation, and reference to other scholars’ research, the factors influencing the construction of high-speed railways at mined sites are categorized into four main categories: axle weight, vehicle speed, trunk line location, and roadbed structure, and their influence levels are classified and described qualitatively and quantitatively.
3) The field engineering examples of the mine goaf grounds passed by the Tai-Jiao high-speed railway and the Nan-Qin high-speed railway are selected to verify the model established in this article. The analysis results of engineering examples show that the model established in this article is suitable for the ground activity analysis and discrimination of high-speed railway mine goaf ground.
Data Availability Statement
The raw data supporting the conclusion of this article will be made available by the authors, without undue reservation.
Author Contributions
L-wR is responsible for the investigation and data sorting of the cases, P-fH is responsible for the selection of machine learning algorithms and the writing of articles, Y-FZ is responsible for the work related to extension theory, Z-LD is responsible for the establishment of unascertained measure function, Z-sZ and S-rW are responsible for reviewing the quality, format, and logic of the article.
Funding
This work was supported by the National Natural Science Foundation of China (No. U1810203).
Conflict of Interest
The authors declare that the research was conducted in the absence of any commercial or financial relationships that could be construed as a potential conflict of interest.
Publisher’s Note
All claims expressed in this article are solely those of the authors and do not necessarily represent those of their affiliated organizations, or those of the publisher, the editors, and the reviewers. Any product that may be evaluated in this article, or claim that may be made by its manufacturer, is not guaranteed or endorsed by the publisher.
References
Bell, F. G., Bullock, S. E. T., Halbich, T. F. J., and Lindsay, P. (2001). Environmental Impacts Associated with an Abandoned Mine in the Withbank Coalfield, South Africa. Int. J. Coal Geol. 45 (2-3), 195–216. doi:10.1016/s0166-5162(00)00033-1
Bian, X., Jiang, H., Cheng, C., Chen, Y., Chen, R., and Jiang, J. (2014). Full-scale Model Testing on a Ballastless High-Speed Railway under Simulated Train Moving Loads. Soil Dyn. Earthq. Eng. 66, 368–384. doi:10.1016/j.soildyn.2014.08.003
Bratov, V., Petrov, Y., Semenov, B., and Darienko, I. (2014). Modelling the High-Speed Train Induced Dynamic Response of Railway Embankment. Mater. Phys. Mech. 22 (1), 69.
Cao, Z., and Zhou, Y. (2015). Research on Coal Pillar Width in Roadway Driving along Goaf Based on the Stability of Key Block. Comput. Mater. Continua 48 (2), 77. doi:10.3970/cmc.2015.048.077
Connolly, D. P., Dong, K., Alves Costa, P., Soares, P., and Woodward, P. K. (2020). High Speed Railway Ground Dynamics: a Multi-Model Analysis. Int. J. Rail Transp. 8 (4), 324–346. doi:10.1080/23248378.2020.1712267
Cui, X., Gao, Y., and Yuan, D. (2014). Sudden Surface Collapse Disasters Caused by Shallow Partial Mining in Datong Coalfield, China. Nat. Hazards 74 (2), 911–929. doi:10.1007/s11069-014-1221-5
Du, H., Song, D., Chen, Z., and Guo, Z. (2020). Experimental Study of the Influence of Structural Planes on the Mechanical Properties of Sandstone Specimens under Cyclic Dynamic Disturbance. Energy Sci. Eng. 8, 4043–4063. doi:10.1002/ese3.794
Gong, F., Li, X., Dong, L., and Liu, X. (2008). Underground Goaf Risk Evaluation Based on Uncertainty Mensurement Theory. Chin. J. Rock Mech. Eng. (02), 323. doi:10.3321/j.issn:1000-6915.2008.02.014
Guo, Q., Li, Y., Meng, X., Guo, G., and Lv, X. (2019). Instability Risk Assessment of Expressway Construction Site above an Abandoned Goaf: a Case Study in China. Environ. Earth Sci. 78 (20), 8599. doi:10.1007/s12665-019-8599-z
He, X., Xu, H., Sabetamal, H., and Sheng, D. (2020). Machine Learning Aided Stochastic Reliability Analysis of Spatially Variable Slopes. Comput. Geotechnics 126, 103711. doi:10.1016/j.compgeo.2020.103711
Helm, P. R., Davie, C. T., and Glendinning, S. (2013). Numerical Modelling of Shallow Abandoned Mine Working Subsidence Affecting Transport Infrastructure. Eng. Geol. 154, 6–19. doi:10.1016/j.enggeo.2012.12.003
Hu, Y.-x., and Li, X.-b. (2012). Bayes Discriminant Analysis Method to Identify Risky of Complicated Goaf in Mines and its Application. Trans. Nonferrous Metals Soc. China 22 (2), 425–431. doi:10.1016/s1003-6326(11)61194-1
Jiang, S., and Wang, Y. (2019). Long-Term Ground Settlements over Mined-Out Region Induced by Railway Construction and Operation. Sustainability 11 (3), 30875. doi:10.3390/su11030875
Lamas-Lopez, F., Cui, Y.-J., Calon, N., Costa D’Aguiar, S., Peixoto De Oliveira, M., and Zhang, T. (2016). Track-bed Mechanical Behaviour under the Impact of Train at Different Speeds. Soils Found. 56 (4), 627–639. doi:10.1016/j.sandf.2016.07.004
Lawal, A. I., and Kwon, S. (2021). Application of Artificial Intelligence to Rock Mechanics: An Overview. J. Rock Mech. Geotechnical Eng. 13 (1), 248–266. doi:10.1016/j.jrmge.2020.05.010
Li, L., Wu, K., and Zhou, D. (2016). Evaluation Theory and Application of Foundation Stability of New Buildings over an Old Goaf Using Longwall Mining Technology. Environ. Earth Sci. 75 (9), 5574. doi:10.1007/s12665-016-5574-9
Li, S., Ling, X., Tian, S., Ye, Y., Tang, L., Cai, D., et al. (2021). In-situ Test and Analysis of Subgrade Vibration with Ballasted Track in Deep Seasonally Frozen Regions. Transp. Geotech. 31, 100658. doi:10.1016/j.trgeo.2021.100658
Li, W., Bian, X., Duan, X., Tutumluer, E., and Erol, (2018). Full-Scale Model Testing on Ballasted High-Speed Railway: Dynamic Responses and Accumulated Settlements. Transp. Res. Rec. 2672 (10), 125–135. doi:10.1177/0361198118784379
Liu, X., Liang, Z., Zhang, Y., Liang, P., and Tian, B. (2018). Experimental Study on the Monitoring of Rockburst in Tunnels under Dry and Saturated Conditions Using AE and Infrared Monitoring. Tunn. Undergr. Space Technol. 82 (12), 517–528. doi:10.1016/j.tust.2018.08.011
Liu, X., Wu, L., Zhang, Y., Liang, Z., Yao, X., and Liang, P. (2019). Frequency Properties of Acoustic Emissions from the Dry and Saturated Rock. Environ. Earth Sci. 78 (3), 67. doi:10.1007/s12665-019-8058-x
Liu, X., Wu, L., Zhang, Y., and Mao, W. (2021). Localized Enhancement of Infrared Radiation Temperature of Rock Compressively Sheared to Fracturing Sliding: Features and Significance. Front. Earth Sci. 9, 756369. doi:10.3389/feart.2021.756369
Liu, X., Wu, L., Zhang, Y., Wang, S., Yao, X., and Wu, X. (2020). The Characteristics of Crack Existence and Development during Rock Shear Fracturing Evolution. Bull. Eng. Geol. Environ. 80 (2), 1671–1682. doi:10.1007/s10064-020-01997-3
Mosayebi, S.-A., Zakeri, J.-A., and Esmaeili, M. (2017). Vehicle/track Dynamic Interaction Considering Developed Railway Substructure Models. Struct. Eng. Mech. 61 (6), 775–784. doi:10.12989/sem.2017.61.6.775
Qian, M., Miu, X., and Xu, J. (1996). Theoretical Study of Key Stratum in Ground Control. J. China Coal Soc. 21 (03), 2–7.
Qin, Y., Luo, Z., Wang, J., Ma, S., and Feng, C. (2019). Evaluation of Goaf Stability Based on Transfer Learning Theory of Artificial Intelligence. Ieee Access 7, 96912–96925. doi:10.1109/access.2019.2929533
Shi, J., and Hou, D. (2017). Failure Analysis for Railroad Embankment under Heavy Haul Wagon Loads. Mechanika 23 (2), 197–203. doi:10.5755/j01.mech.23.2.13911
Smarandache, F. (2012). Generalizations of the Distance and Dependent Function in Extenics to 2D, 3D, and N - D. Prog. Phys. 3, 54. doi:10.1136/ard.2003.014274
Song, D., Liu, X., Huang, J., and Zhang, J. (2020). Energy-based Analysis of Seismic Failure Mechanism of a Rock Slope with Discontinuities Using Hilbert-Huang Transform and Marginal Spectrum in the Time-Frequency Domain. Landslides 18, 105–123. doi:10.1007/s10346-020-01491-7
Song, D., Liu, X., Li, B., Zhang, J., and Volcan, J. (2021a). Assessing the Influence of a Rapid Water Drawdown on the Seismic Response Characteristics of a Reservoir Rock Slope Using Time-Frequency Analysis. Acta Geotech. 16, 1281–1302. doi:10.1007/s11440-020-01094-5
Song, D. Q., Liu, X., and Huang, J. (2021b). Seismic Cumulative Failure Effects on a Reservoir Bank Slope with a Complex Geological Structure Considering Plastic Deformation Characteristics Using Shaking Table Tests. Eng. Geol. 286 (3). 106085. doi:10.1016/j.enggeo.2021.106085
Tarawneh, B., Nusairat, J., and Hakam, Y. (2018). Load Testing and Settlement of Shallow Foundation on Desert Sands. Proc. Institution Civ. Eng. - Geotechnical Eng. 171 (1), 52–63. doi:10.1680/jgeen.16.00109
The Professional Standards Compilation Group of People's Republic of China (2014). Code for Investigation of Geotechnical Engineering in the Coal Mine Goaf (GB 51044-2014) Beijing: China Planning Press.
Toh, K.-A., Kim, J., and Lee, S. (2008). Maximizing Area under ROC Curve for Biometric Scores Fusion. Pattern Recognit. 41 (11), 3373–3392. doi:10.1016/j.patcog.2008.04.002
Wang, J. (2016). Activation Law and Stability Evaluation of Goaf under Railway Load: Qingdao. China: Qingdao University of technology, 86.
Xie, X., Shi, S., Bu, L., Li, L., Sun, K., and Liu, H. (2021). Dynamic Optimization Risk Assessment Model and Control Measures for Tunnel Collapse and its Engineering Application. Himal. Geol. 42 (1), 117.
Zhang, J. (2009). Fuzzy Comprehensive Evaluation Method of the Foundation Stability of New-Buildings above Worked-Out Area. J. Univ. Sci. Technol. Beijing 31 (11), 1368–1372. doi:10.13374/j.issn1001-053x.2009.11.026
Keywords: mine goaf ground, activation classification, high-speed railway, machine learning, extension theory
Citation: Ren L-w, He P-f, Zou Y-F, Dun Z-L, Zou Z-s and Wang S-r (2022) A New Classification Method of Mine Goaf Ground Activation Considering High-Speed Railway Influence. Front. Earth Sci. 10:896459. doi: 10.3389/feart.2022.896459
Received: 15 March 2022; Accepted: 09 May 2022;
Published: 27 June 2022.
Edited by:
Shibing Huang, Wuhan University of Science and Technology, ChinaReviewed by:
Danqing Song, Tsinghua University, ChinaXiangxin Liu, North China University of Science and Technology, China
Copyright © 2022 Ren, He, Zou, Dun, Zou and Wang. This is an open-access article distributed under the terms of the Creative Commons Attribution License (CC BY). The use, distribution or reproduction in other forums is permitted, provided the original author(s) and the copyright owner(s) are credited and that the original publication in this journal is cited, in accordance with accepted academic practice. No use, distribution or reproduction is permitted which does not comply with these terms.
*Correspondence: Peng-fei He, MjEyMDA4MDEwMDI4QGhvbWUuaHB1LmVkdS5jbg==