- 1Complexity Science Group, Department of Physics and Astronomy, University of Calgary, Calgary, AB, Canada
- 2Department of Geoscience, University of Calgary, Calgary, AB, Canada
- 3Hotchkiss Brain Institute, University of Calgary, Calgary, AB, Canada
Induced seismicity has become a concern for industry and nearby residents. Fluid-induced earthquakes are a side effect of industrial operations such as hydraulic fracturing, where high-pressure fluids are pumped into the Earth’s crust to increase hydrocarbon flow to a well from petroleum-bearing rock formations. Previous studies on fluid-induced seismicity showed the existence of spatiotemporal clustering and aftershock activity. Here, we study seismic catalogs from the Kiskatinaw Seismic Monitoring and Mitigation Area (KSMMA), British Columbia, Canada to investigate spatiotemporal clustering and determine the aftershock activity due to secondary triggering mechanisms such as static and dynamic stress changes arising from preceding seismic events. Our analysis is based on modern tools of statistical seismology such as the Bi-test and triggering analysis. We find significant aftershock triggering and spatiotemporal clustering across the KSMMA but with large spatial variations, which might be indicative of pre-existing stresses and fault systems. In particular, aftershock triggering is characterized by rapidly decaying spatial distributions of aftershocks beyond the mainshock rupture area and by a strong dominance of small but frequent triggers, consistent with that observed for hydraulic fracturing and saltwater disposal elsewhere.
1 Introduction
Fluid invasion in stressed materials can initiate processes of mechanical failure due to changes in effective stress (Eaton, 2018; Goebel and Brodsky, 2018). This phenomenon can account for fluid-induced seismicity related to enhanced geothermal systems (EGSs) (Martínez-Garzón et al., 2014; Schoenball et al., 2012, 2015), hydraulic fracturing (HF) operations (Maghsoudi et al., 2016; Schultz et al., 2018), and underground saltwater disposal (SWD) (Ellsworth, 2013; Schoenball and Ellsworth, 2017). These injection activities have led to an increase in earthquake rates in parts of North America and Central Europe (Ellsworth, 2013; Yeck et al., 2017; Schoenball et al., 2018). An M4.6 earthquake in 2019 in Red Deer, Canada (Yu et al., 2019), induced by HF and an M5.5 earthquake in 2015, Pohang, South Korea (Ellsworth et al., 2019), induced by EGS are two examples of the potential seismic hazards associated with fluid injections. Reliable seismic hazard assessment of such industrial operations, especially in tectonically active areas and highly populated areas, is thus critical.
To improve our current ability to assess such seismic hazards, it is essential to establish the degree to which the underlying mechanisms are not only related to direct effects of injection such as pore pressure diffusion but also arise from secondary processes (Cattania et al., 2015; Maghsoudi et al., 2018). This includes interevent triggering that emerges from stress changes due to preceding events, leading to cascades of seismic activities such as aftershock sequences (Gu et al., 2013; Smirnov et al., 2019; Kothari et al., 2020). In general, earthquake catalogs can be decomposed into two populations: dependent and independent events (Baiesi and Paczuski, 2004; Zaliapin et al., 2008). Dependent events or aftershocks are thought to be triggered by mechanical processes that are at least partly controlled by previous earthquakes (Maghsoudi et al., 2016, 2018). Independent or background earthquakes are directly caused by aseismic mechanisms such as tectonic loading or fluid injections (Shapiro et al., 2005; Hainzl et al., 2013; Eyre et al., 2019; Eyre et al., 2022).
The presence and importance of aftershocks in the context of fluid-induced seismicity have been analyzed in several case studies: In Salton Sea EGS field in California (Martínez-Garzón et al., 2018), Hoadley HF field of Alberta, and HF operations in the Horn River area in British Columbia (Maghsoudi et al., 2018) and in Oklahoma SWD (Schoenball and Ellsworth, 2017; Schoenball et al., 2018; Karimi and Davidsen, 2021), aftershock triggering has been documented, while in some situations [e.g., Soultz, France (Langenbruch et al., 2011; Schoenball et al., 2012) and Geysers EGS fields in California (Martínez-Garzón et al., 2018)], this is not the case. Schoenball et al. (2015) showed that fluid-induced seismicity in the Coso EGS field in California appears primarily as independent events, but aftershock triggering is also present. In this study, we investigate the presence of spatiotemporal clustering and aftershock triggering in the Kiskatinaw Seismic Monitoring and Mitigation Area (KSMMA) in British Columbia, Canada, a region where regulations have been implemented to manage risks of induced seismicity and where large scale fluid injection operations are based. We first examine the Gutenberg–Richter relation and find the magnitude of completeness. Using the Bi-test, we show that one can reject the hypothesis of independent events in the form of a homogeneous or non-homogeneous Poisson process at high confidence levels. Finally, we present direct evidence for aftershock triggering using the triggering analysis method explained in Section 2.2.2. Based on this methodology, we identify the background and triggered events and study their respective properties, such as the aftershock triggering rates and the aftershock productivity relation, as well as spatial aftershock zones. The strong regional variations in the response to fluid injections highlight the importance of pre-existing stresses and fault systems.
2 Materials and Methods
2.1 Data
The data used in this study were obtained from the analysis of continuous data from a 15-station broadband seismograph array in the KSMMA together with nearby public stations (Eaton et al., 2021; Salvage et al., 2021). The KSMMA was designated in the greater Farmington area of BC, Canada, with the issuance of a special project in May 2018 by the BC Oil and Gas Commission (BCOGC). After seismic events ranging from 3.4 to 4.5 magnitude, hydraulic fracture operations at a well pad were suspended on 29 November 2018. There have been a few reports of felt events at the surface. The events were caused by fluid injection during hydraulic fracturing operations in the Montney formation (Fox et al., 2019; Eaton et al., 2021; Salvage and Eaton, 2021; Salvage et al., 2021) in addition to seismicity triggered by SWD (Salvage and Eaton, 2021, 2022). In early 2020, in addition to the 9 public sensors, 13 additional broadband seismic stations and two accelerometers were installed within the KSMMA as part of a joint project between the University of Calgary, Nanometrics, Geoscience BC, and several universities in South Korea to monitor seismicity associated with hydraulic fracturing operations (Salvage and Eaton, 2021).
In this case study, we consider the seismic catalog from the KSMMA, as shown in Figure 1. Using the seismograph network (15 stations), 14,159 seismic events (from −0.93 to 3.72 ML) were detected and located during January 21, 2020 to September 30, 2021 (Figures 1, 2). As Figure 2A shows, this catalog exhibits periods with highly clustered seismic activity. Related to this, there are large variations in the seismicity rates, as can be seen in Figures 2A,B. All the injection data shown in Figure 2 are from HF wells, yet we need to bear in mind that SWD wells are also operational in the region (Salvage et al., 2021; Salvage and Eaton, 2022), and their injection data are not available. HF well operators have 1 year to report their injection data; therefore, there are a small number of HF wells with no reported injection data after February 02, 2021 (Figure 2). From March 30 to July 14 of 2020, there were no fluid injections occurring in KSMMA due to the COVID-19 lockdown (Salvage and Eaton, 2021; Salvage et al., 2021), yet there are still events being recorded (Figures 2A,B).
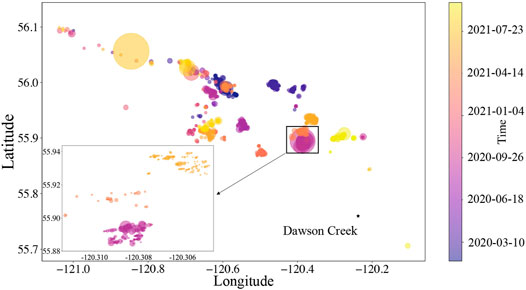
FIGURE 1. Spatial map of seismic events in KSMMA catalog; 14,159 seismic events (from −0.93 to 3.72 ML) were detected and located during January 21, 2020 to September 30, 2021. The color intensity shown is associated with the passing of time. The radius is associated with the size of the earthquakes. Only events above magnitude of completeness (mc = 1.4) are shown here.
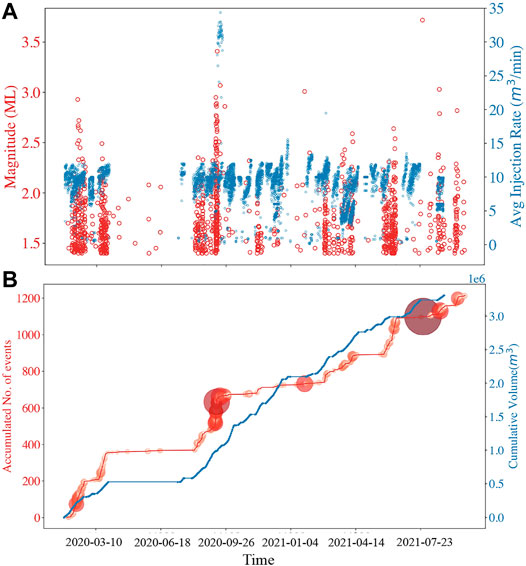
FIGURE 2. (A) Red circles show the magnitudes (ML) of the events in the full catalog from January 22, 2020 to September 30, 2021. Only events above magnitude of completeness are shown here. Blue circles show the average daily injection rate from January 21, 2020 to August 28, 2021 due to hydraulic fracturing. From March 30 to July 14 of 2020, there are no fluid injections due to the COVID-19 lockdown, yet there are still events being recorded. (B) Red line indicates the accumulated number of events above magnitude of completeness. The radius of the red circles is proportional to the magnitude of the induced events. The blue line shows the accumulated amount of injection volume to the KSMMA from January 21, 2020 to August 28, 2021 due to hydraulic fracturing. (Injection Data provided by geoLOGIC systems ltd.ⓒ.)
2.1.1 Frequency–Magnitude Distribution
As expected, the frequency–magnitude distribution follows the Gutenberg–Richter relation,
We alter mc and include events with m ≥ mc to estimate b-value as a function of mc using a maximum-likelihood estimator (Aki, 1965). Note that the magnitudes in our catalog correspond to local magnitudes. The magnitude of completeness in Figure 3 has been estimated as the lowest magnitude threshold, for which the estimate of the b-value in the frequency–magnitude distribution became constant (Bhattacharya et al., 2011; Davidsen et al., 2015, 2021). We estimate b = 1.4 ± 0.05 for magnitudes larger than mc = 1.4 (Figure 3). In the following, we only consider events with magnitude bigger than mc. Note that both b-value and mc are input parameters for the triggering analysis method explained in Section 2.2.2.
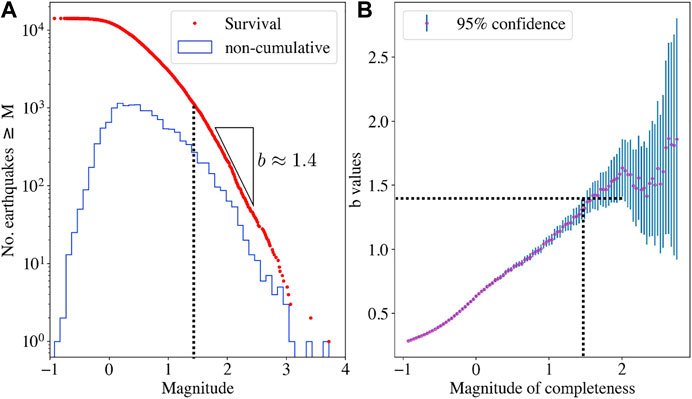
FIGURE 3. (A) Frequency–magnitude distributions for the KSMMA catalog. (B) b-values estimated by maximum likelihood within the interval of magnitudes mc < m < ∞ as a function of mc. Based on the stability analysis, mc ≈ 1.4.
2.2 Methods
2.2.1 Bi-Test
The Bi-test allows testing whether seismic activity follows a Poisson process or whether temporal clustering or regular behavior is present (Maghsoudi et al., 2018). Consider the temporal sequence of events (tj). The Bi-test consists of the joint evaluation of the interevent time from a given event at tj to the closest one in time: δtj = min(tj−tj−1, tj+1−tj) and the consecutive interevent time in the same temporal direction, that is, δτj = tj−1−tj−2 if δtj = tj−tj−1 and δτj = tj+2−tj+1 if δtj = tj+1−tj. From the data pairs (δtj, δτj), we can build the statistic variable:
with values between 0 and 1. If the data are indeed drawn from a random process that is locally Poisson, then the values δtj are randomly distributed and independent from the values δτj. In this case, it can be shown that the variable Hj is uniformly distributed between 0 and 1 and independent of the local Poisson rate (Bi et al., 1989). Deviations from the uniform distribution indicate the presence of correlations between events. If the interevent times exhibit a regular pattern, ⟨δtj⟩ ≈ ⟨δτj⟩ and thus H will be distributed around
Using the Bi-test, deviations from Poisson behavior can be systematically quantified using the performance of the Kolmogorov–Smirnov (K-S) test (Stephens, 1970) comparing the experimental cumulative distribution function Fn (H) to the uniform distribution F(H) = H expected for a Poisson process. Specifically, a p-value can be assigned to the process as the probability of obtaining an extreme difference max [Fn(H)−H] in a Poisson process. Figure 5 shows Fn(H)−H scaled by the factor
1) oscillate around 0 for a Poisson process;
2) display a rotated S-shaped (excess of H values around ⟨H⟩ = 2/3) whenever there exists regularity among the interevent times;
3) exhibit a rotated Z-shaped (excess of H-values around H ≈ 1 and H ≈ 0) whenever the process exhibits clustering (Figure 5).
Thus, for low p-values, one can identify the type of deviation from the Poisson hypothesis. Clustering (rotated Z-shaped) is given by
2.2.2 Triggering Analysis
The robust classification of earthquakes into independent and dependent events and understanding how one earthquake triggers another one are important challenges. Addressing them can help us better understand earthquake occurrence physics, distinguish between earthquakes directly caused by external dynamics (e.g., fluid injection driven and aseismic slip) and those triggered by other earthquakes, and reflect the internal dynamics. To directly show the presence of interevent triggering and aftershocks, we perform a more detailed statistical analysis of interevent triggering using a method first proposed by Baiesi and Paczuski (2004), which has been used for the recognition of statistically significant interevent triggering (Zaliapin et al., 2008; Gu et al., 2013; Moradpour et al., 2014; Schoenball et al., 2015; Shebalin and Narteau, 2017; Kothari et al., 2020; Shebalin et al., 2020; Davidsen et al., 2021).
In the absence of interevent triggering, events are expected to follow a Poisson process with a spatiotemporal magnitude rate given by the Gutenberg–Richter relation (Maghsoudi et al., 2016, 2018). Therefore, we look for violations of this null hypothesis to identify potential triggering relations between events. Consider a time-ordered earthquake catalog given as
where df is the fractal dimension of hypocenters, tij = tj−ti, and c is a constant that depends on the specific region under consideration. This equation is based on the Gutenberg–Richter relation.
Based on Equation (2), we can designate the most plausible candidate i that triggered event j as the one that minimizes the expected number of earthquakes nij, since it is the least likely event pair to have happened at random. In the following, we denote
This follows from Figure 4 which shows density plots of the set
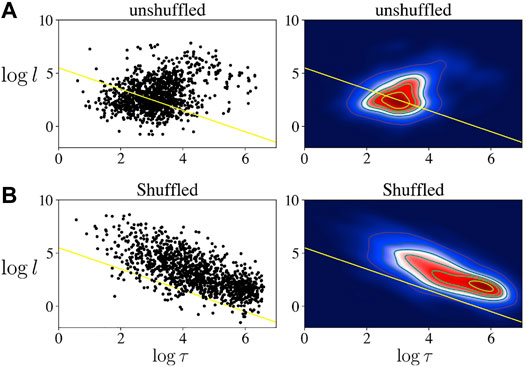
FIGURE 4. Density plots of the set n* represented in log τ-log l space. (A) Result for the original KSMMA catalog. (B) Result for the shuffled KSMMA catalog. Here, we used the shuffled catalog to estimate n*, shown as a yellow line that separates the two populations of triggered events below the line and background events above the line.
3 Results
3.1 Temporal Correlations
Without clustering and any causal relation between events, one would expect a Poisson process. The time intervals between successive events would follow an exponential distribution with a characteristic rate. For fluid-induced seismicity, this is the hypothesis proposed in Hajati et al. (2015). Nevertheless, Maghsoudi et al. (2016, 2018) showed that for several fluid-induced seismic catalogs, the distribution does not follow exponential behavior. In principle, such behavior can arise from a Poisson process with a time-varying rate, which might emerge due to time-varying injection rates in fluid-induced seismicity. To establish explicitly whether this is the case, we use the Bi-test (described in Section 2.2.1) to test the hypothesis.
The curve shown in Figure 5 corresponds to the rescaled K-S distances for events with magnitude above the respective magnitude of completeness mc = 1.4. The curve in Figure 5 resembles a rotated “S” shape, which is a signature of regularity, as described in Section 2.2.1. The shaded areas in Figure 5 represent different probabilities that a given value of H is not consistent with the Poisson hypothesis. For mc = 1.4, the p-value associated with the K-S test is below 0.05%. Therefore, the Bi-test provides strong evidence that the seismic activity here does not follow a (time-dependent or time-independent) Poisson process. This indicates that a simple proportionality between injection rates and seismic activity is inadequate to describe the observations.
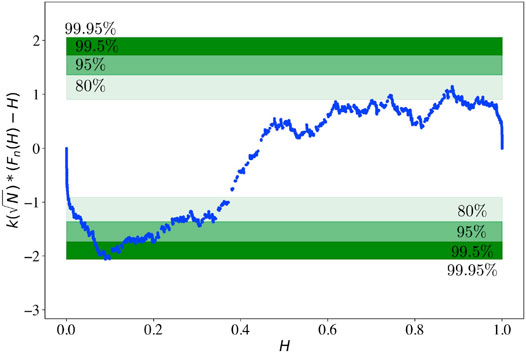
FIGURE 5. Results of the Bi-test. Deviations from Poisson behavior in the cumulative distribution function for KSMMA catalog. The corresponding 80%, 95, 99.5, and 99.95% probability that a given value is not consistent with the Poisson hypothesis is highlighted by the shaded areas.
3.2 Interevent Triggering
To use the triggering analysis discussed in Section 2.2.2, we need the b-value and df for the KSMMA catalog. As discussed in Section 3.1, we find b = 1.4 and mc = 1.4. The parameter df is harder to estimate reliably, yet the overall results of the methodology are typically robust over a range of realistic df-values (Gu et al., 2013). Note that we focus on hypocenters here and the 3D distances between them. Thus, in the following, we present our findings only for df = 2.2.
The top panels of Figure 4 provide clear evidence in favor of interevent triggering in the KSMMA catalog. The denser regions in Figure 4 correspond to the most probable occurrences of weighted relative time and distances of all pairs, and an almost bimodal structure is visible in the top panels of Figure 4. Such a structure indicates that the triggering relations of event pairs with
Figure 6 shows the classification of events into the background and triggered ones. As expected, the number of triggered events is exceptionally high during periods of high activity. After the division of events into background and triggered ones, one can investigate the ratio of triggered to background events over time (Figure 6A). As a specific example, during September 9–13, 2020, we observe that the ratio of triggered to background events is at a maximum (Figure 6A), and the temporal clustering of events during this period is significant. Additionally, the spatial map of events during this period shows clustering in space near Farmington, BC (Figure 6C), a region with significant number of faults (Wozniakowska et al., 2021; Hayes et al., 2020). During September 9–13, 2020, hydraulic fracturing took place in this region along seven horizontal wells with multiple stages each; the surface location of the wells is represented by a black cross in Figure 6C. The overall seismic activity closely aligns with the location of the wells orientated along the east/northeast direction. This suggest that the high rate of seismic activity and the dominance of triggered events in this region are related to pre-existing high stress levels and fault systems (Hayes et al., 2020; Wozniakowska et al., 2021).
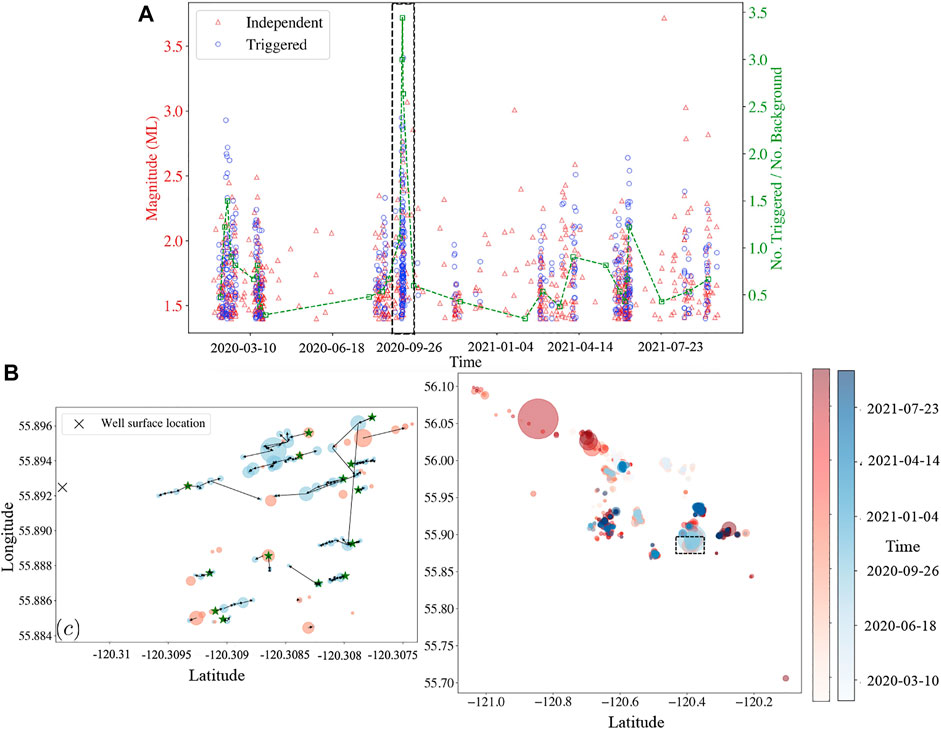
FIGURE 6. Interevent triggering in the KSMMA catalog, where we have separated events into triggered (blue) and independent (red) events. Only events above magnitude of completeness (mc = 1.4) are shown here. (A) Magnitude time series and the ratio of triggered to independent events over a window size of 50 events. The dashed black rectangle represents a period (September 9–13, 2020) with maximum triggered to independent ratio. (B) Spatial map of events. The color intensity and radius are associated with the passing of time and the size of the earthquakes, respectively. (C) Spatial map of triggering cascades associated with the period represented by the dashed black rectangle in (A) and (B). The green stars indicate the root events of each triggering cascade where more than two events have been triggered in that cascade.
3.3 Interevent Triggering Rates and Productivity
To characterize the behavior of the interevent triggering in more detail, we now focus on the occurrence rate of triggered events as a function of the time after the trigger. One specific property is that the decay rate of aftershocks above a certain magnitude is typically inversely proportional to the time since the mainshock as captured by the Omori–Utsu relation:
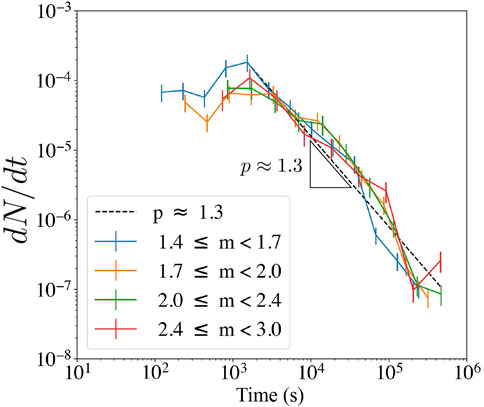
FIGURE 7. Average triggering or aftershock rates above mc of mainshocks within different magnitude ranges for KSMMA catalog. The black dashed line represents the Omori exponent (p ≈ 1.3).
To study the triggering productivity, we analyze the total number of triggered events or aftershocks (Naftershock) triggered by a mainshock. The average number of aftershocks of a tectonic earthquake of magnitude m typically scales with m as Naftershock(m) ≈ 10αm (Helmstetter and Sornette, 2003; Hainzl et al., 2013; Davidsen and Baiesi, 2016; Shebalin et al., 2020). Figure 8 shows the average number of aftershocks triggered by earthquakes within magnitude ranges of size δm ≥ 0.15. We find α ≈ 0.35 for the KSMMA catalog, which indicates that the aftershock productivity increases relatively slowly with the magnitude of the trigger.
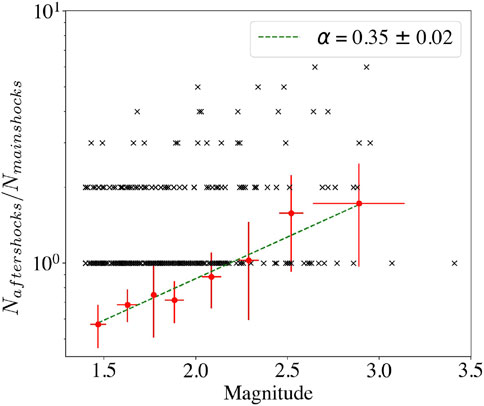
FIGURE 8. Average number of triggered events as a function of the magnitude of the trigger over magnitude bins of size δm ≥ 0.15, which increases as
3.4 Spatial Aftershock Zones
We assess the normalized linear aftershock density P(r) associated with triggers (or mainshocks) of magnitude m. As shown in Figure 9A, aftershock densities show an initial increase up to a maximum, followed by a power-law decay. The location of the peak is closely aligned with the rupture length of the trigger, which scales as rrup ∝ 10σm (Gu et al., 2013; Karimi and Davidsen, 2021). To test this explicitly, we rescale the distance r with 10σm, and we indeed obtain a robust data collapse of all aftershock densities for KSMMA in Figure 9B. The value of σ = 0.4 for KSMMA is consistent with previously reported estimates for tectonic earthquakes (Gu et al., 2013; Moradpour et al., 2014), and fluid-induced and natural swarms catalogs (Karimi and Davidsen, 2021). The data collapse also indicates that beyond the rupture length, the aftershock density decays as r−ν. Aftershock zones in the KSMMA show a decay with ν = 2.4 ± 0.1 (Figure 9B), where this range of decay is consistent with the seismic catalogs related to SWD in Oklahoma and southern Kansas as well as those of natural swarms from the Long Valley Caldera, Fillmore, and Mogul (Karimi and Davidsen, 2021).
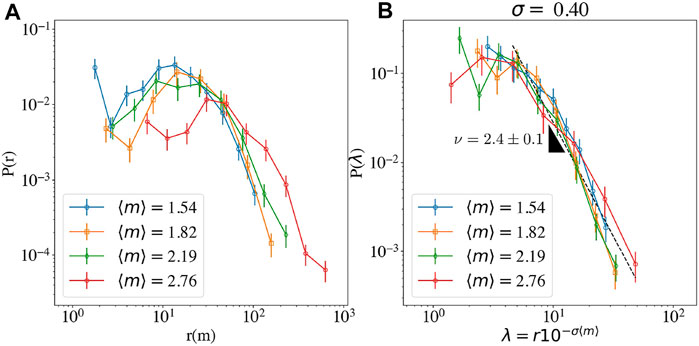
FIGURE 9. (A) Probability density function of spatial distances in meters between directly triggered aftershocks and their mainshocks for different ranges of mainshock magnitudes. (B) Probability density function of spatial distances between directly triggered aftershocks and their mainshocks (for r > 10 m), where a factor of 10−σ⟨m⟩ rescales the distances (in meters) with σ = 0.4.
4 Discussion and Conclusion
This case study provides evidence of seismic clustering in space and time in KSMMA. Our analysis shows that seismic activity does not follow a simple Poisson process, and aftershock triggering plays an essential role for fluid-induced seismicity here. Previous studies (Maghsoudi et al., 2016; Schoenball and Ellsworth, 2017; Maghsoudi et al., 2018; Schoenball et al., 2018; Karimi and Davidsen, 2021) observed aftershock triggering in other fluid-induced seismicity catalogs, similar to the findings of our study.
When directly comparing α and b-value across catalogs, different magnitude scales—for the KSMMA catalog, all magnitudes correspond to local magnitudes—need to be taken into account. Yet, b−α should be invariant under changes in the definition of magnitude (Karimi and Davidsen, 2021), and we focus on this difference here. Similar to the observation by Gu et al. (2013) and Karimi and Davidsen (2021), we find b > α implying that the overall interevent triggering is dominated by the more frequent smaller triggers, instead of the fewer larger triggers (Gu et al., 2013). Moreover, the larger b−α, the larger the contribution of the smallest triggers to the overall aftershock production. Karimi and Davidsen (2021) showed that b−α allows one to differentiate between natural swarms and fluid-induced seismicity, with b −α < 0.6 for natural swarms, and 0.8 < b−α for fluid-induced seismicity. For KSMMA, b−α ≈ 1.05, consistent with the previous results. In particular, this very limited role of large events for aftershock triggering is confirmed by the absence of significant aftershocks after a 3.74-ML earthquake in our case study. All these confirm that the overall seismic aftershock hazards associated with fluid-induced seismicity are quite different from natural swarms.
As one of the common properties of all fluid-driven seismicity (natural swarms and seismicity induced by humans), we identify a relatively steep decay of the spatial density of aftershocks P(r) beyond the rupture length of the mainshocks with ν ≈ 2.4, similar to the observations in Oklahoma and southern Kansas, as well as natural swarms in the Long Valley Caldera, Fillmore, and Mogul (Karimi and Davidsen, 2021). This relatively rapid decay of the aftershock zone is indicative of the dominant role of fluid migration compared to that observed for tectonic seismicity.
Investigating the event–event triggering clusters in KSMMA, our findings also show that the clustering is relatively localized in space and time. In the periods where seismic activity is somewhat higher, the interevent triggering is also higher (Figure 6A), and vice versa. The highly temporal clustered periods are also spatially localized (e.g., Figure 6C), which is further confirmed by the consistent rapid spatial decay of aftershocks (with a robust ν ≈ 2.4, see Supplementary Figure S1). In contrast, a series of events in the northwest of the KSMMA, including the aforementioned 3.74-ML event (Figure 6B), do not show any significant interevent triggering behavior as manifested by relatively high time difference and spatial distance between these events (Figure 1). The absence of aftershocks following relatively large earthquakes such as a 3.74-ML earthquake or a 4.1-Mw earthquake in Alberta, Canada, could be related to aseismic processes. Eyre et al. (2019) discussed the role of aseismic slip in fluid-induced seismicity, where pore pressure-driven aseismic slips have been the leading cause of 4.1-Mw earthquake. To directly establish that indeed aseismic slips caused by fluid injection plays a role in northwest of KSMMA, one would need to study ground-deformation observations measured by satellite radar (Eyre et al., 2022).
The more narrow spatial reach of fluid-induced seismicity and the relatively low contribution of significant seismic events to interevent triggering are essential cornerstones of assessing the seismic hazard associated with fluid injections related to HF in KSMMA and beyond. Indeed, our results indicate that the fluid-induced seismicity in KSMMA follows non-Poissonian statistics while being conditioned spatiotemporally by anthropogenic activities. This implies that the simple assumption of Poissonian behavior—sometimes used for short-term probabilistic seismic hazard assessment (Atkinson et al., 2015; Mulargia et al., 2017; Canales et al., 2022)—is not adequate here. Consequently, one must consider non-Poissonian approaches for time-dependent seismic hazard assessment and forecasting of seismicity (Beauval et al., 2006; Bachmann et al., 2011; Convertito et al., 2021). This is in addition to the correlation of induced seismicity with fluid injection (Broccardo et al., 2017; Convertito et al., 2021), since otherwise the probability of earthquake occurrence would be underestimated or misleading (Atkinson et al., 2015; Convertito et al., 2021). Additionally, as we showed here, investigating the seismic interevent triggering processes arising in fluid-induced seismicity can also provide indications of high stress or weakened regions (including faults), which is another crucial step toward improved seismic hazard assessments.
Data Availability Statement
Publicly available datasets were analyzed in this study. These data can be found at: https://www.geosciencebc.com/projects/2019-005/.
Author Contributions
OK performed the data analysis guided by JD. OK, DE, and JD contributed to the interpretation of the findings and writing of the manuscript.
Funding
JD was supported by the Natural Sciences and Engineering Research Council of Canada.
Conflict of Interest
The authors declare that the research was conducted in the absence of any commercial or financial relationships that could be construed as a potential conflict of interest.
Publisher’s Note
All claims expressed in this article are solely those of the authors and do not necessarily represent those of their affiliated organizations, or those of the publisher, the editors, and the reviewers. Any product that may be evaluated in this article, or claim that may be made by its manufacturer, is not guaranteed or endorsed by the publisher.
Acknowledgments
We thank Geoscience BC, ARC Resources, Canadian Natural Resources Ltd., and the Natural Sciences and Engineering Research Council (NSERC) of Canada for funding the KSMMA network installation. Sponsors of the Microseismic Industry Consortium are sincerely thanked for their support of this initiative. We further wish to thank industry partners who enabled the structure of the structure KSMMA network. We also thank Nanometrics for installing and maintaining the seismic stations, near real-time analysis of incoming seismicity, and the Incorporated Research Institutions for Seismology (IRIS) for hosting the data. The authors would like to thank geoLOGIC systems Ltd. for their contribution of data and software used for this study. All geoLOGIC systems ltd. is provided under a restricted use license © 2022. We also thank Dr. Rebecca Salvage for her help and kind support and stimulating discussions. The authors would like to sincerely thank the sponsors of the Microseismic Industry Consortium for their ongoing support of this initiative. OK acknowledges Alberta Innovates for the financial support they provided.
Supplementary Material
The Supplementary Material for this article can be found online at: https://www.frontiersin.org/articles/10.3389/feart.2022.894549/full#supplementary-material
References
Aki, K. (1965). Maximum Likelihood Estimate of B in the Formula Log N= A-Bm and its Confidence Limits. Bull. Earthq. Res. Inst. Tokyo Univ. 43, 237–239.
Atkinson, G. M., Ghofrani, H., and Assatourians, K. (2015). Impact of Induced Seismicity on the Evaluation of Seismic hazard: Some Preliminary Considerations. Seismological Res. Lett. 86, 1009–1021. doi:10.1785/0220140204
Bachmann, C. E., Wiemer, S., Woessner, J., and Hainzl, S. (2011). Statistical Analysis of the Induced Basel 2006 Earthquake Sequence: Introducing a Probability-Based Monitoring Approach for Enhanced Geothermal Systems. Geophys. J. Int. 186, 793–807. doi:10.1111/j.1365-246x.2011.05068.x
Baiesi, M., and Paczuski, M. (2004). Scale-free Networks of Earthquakes and Aftershocks. Phys. Rev. E Stat. Nonlin Soft Matter Phys. 69, 066106. doi:10.1103/PhysRevE.69.066106
Beauval, C., Hainzl, S., and Scherbaum, F. (2006). Probabilistic Seismic hazard Estimation in Low-Seismicity Regions Considering Non-poissonian Seismic Occurrence. Geophys. J. Int. 164, 543–550. doi:10.1111/j.1365-246x.2006.02863.x
Bhattacharya, P., Phan, M., and Shcherbakov, R. (2011). Statistical Analysis of the 2002 Mw 7.9 Denali Earthquake Aftershock Sequence. Bull. Seismological Soc. America 101, 2662–2674. doi:10.1785/0120100336
Bi, H., Börner, G., and Chu, Y. (1989). Correlations in the Absorption Lines of the Quasar Q0420-388. Astron. Astrophysics 218, 19–23.
Broccardo, M., Mignan, A., Wiemer, S., Stojadinovic, B., and Giardini, D. (2017). Hierarchical Bayesian Modeling of Fluid-Induced Seismicity. Geophys. Res. Lett. 44, 11–357. doi:10.1002/2017gl075251
Canales, M. R., Yusifbayov, J., and van der Baan, M. (2022). Evolution of Short-Term Seismic hazard in Alberta, Canada, from Induced and Natural Earthquakes: 2011-2020. J. Geophys. Res. Solid Earth 2022, e2021JB022822.
Cattania, C., Hainzl, S., Wang, L., Enescu, B., and Roth, F. (2015). Aftershock Triggering by Postseismic Stresses: A Study Based on Coulomb Rate-And-State Models. J. Geophys. Res. Solid Earth 120, 2388–2407. doi:10.1002/2014jb011500
Convertito, V., Ebrahimian, H., Amoroso, O., Jalayer, F., De Matteis, R., and Capuano, P. (2021). Time-dependent Seismic hazard Analysis for Induced Seismicity: The Case of St Gallen (Switzerland), Geothermal Field. Energies 14, 2747. doi:10.3390/en14102747
Davidsen, J., and Baiesi, M. (2016). Self-similar Aftershock Rates. Phys. Rev. E 94, 022314. doi:10.1103/PhysRevE.94.022314
Davidsen, J., Goebel, T., Kwiatek, G., Stanchits, S., Baró, J., and Dresen, G. (2021). What Controls the Presence and Characteristics of Aftershocks in Rock Fracture in the Lab? J. Geophys. Res. Solid Earth 126, e2021JB022539. doi:10.1029/2021jb022539
Davidsen, J., Gu, C., and Baiesi, M. (2015). Generalized Omori-Utsu Law for Aftershock Sequences in Southern California. Geophys. J. Int. 201, 965–978. doi:10.1093/gji/ggv061
Dinske, C., and Shapiro, S. A. (2013). Seismotectonic State of Reservoirs Inferred from Magnitude Distributions of Fluid-Induced Seismicity. J. Seismol 17, 13–25. doi:10.1007/s10950-012-9292-9
Eaton, D. W., Davidsen, J., Pedersen, P. K., and Boroumand, N. (2014). Breakdown of the Gutenberg-Richter Relation for Microearthquakes Induced by Hydraulic Fracturing: Influence of Stratabound Fractures. Geophys. Prospecting 62, 806–818. doi:10.1111/1365-2478.12128
Eaton, D. W. (2018). Passive Seismic Monitoring of Induced Seismicity. Cambridge: Cambridge University Press.
Eaton, D. W., Salvage, R. O., MacDougall, K., Swinscoe, T., Dettmer, J., Esmaeilzadeh, Z., et al. (2021). Real-time Monitoring of Seismic Activity in the Kiskatinaw Area, Northeastern British Columbia (NTS 093P, 094A), Final Report. Geosci. BC Rep. 2021, 62.
Ellsworth, W. L., Giardini, D., Townend, J., Ge, S., and Shimamoto, T. (2019). Triggering of the Pohang, Korea, Earthquake (Mw 5.5) by Enhanced Geothermal System Stimulation. Seismological Res. Lett. 90, 1844–1858. doi:10.1785/0220190102
Ellsworth, W. L. (2013). Injection-induced Earthquakes. Science 341, 1225942. doi:10.1126/science.1225942
Eyre, T. S., Eaton, D. W., Garagash, D. I., Zecevic, M., Venieri, M., Weir, R., et al. (2019). The Role of Aseismic Slip in Hydraulic Fracturing-Induced Seismicity. Sci. Adv. 5, eaav7172. doi:10.1126/sciadv.aav7172
Eyre, T. S., Samsonov, S., Feng, W., Kao, H., and Eaton, D. W. (2022). InSAR Data Reveal that the Largest Hydraulic Fracturing-Induced Earthquake in Canada, to Date, Is a Slow-Slip Event. Sci. Rep. 12, 2043. doi:10.1038/s41598-022-06129-3
Fox, A. D., Geo, P., Watson, N. D., and Geol, P. (2019). Induced Seismicity Study in the Kiskatinaw Seismic Monitoring and Mitigation Area. British Columbia: BC Oil and Gas Commission.
Goebel, T. H. W., and Brodsky, E. E. (2018). The Spatial Footprint of Injection wells in a Global Compilation of Induced Earthquake Sequences. Science 361, 899–904. doi:10.1126/science.aat5449
Gu, C., Schumann, A. Y., Baiesi, M., and Davidsen, J. (2013). Triggering Cascades and Statistical Properties of Aftershocks. J. Geophys. Res. Solid Earth 118, 4278–4295. doi:10.1002/jgrb.50306
Gutenberg, B., and Richter, C. F. (1944). Frequency of Earthquakes in California*. Bull. Seismological Soc. America 34, 185–188. doi:10.1785/bssa0340040185
Hainzl, S., Zakharova, O., and Marsan, D. (2013). Impact of Aseismic Transients on the Estimation of Aftershock Productivity Parameters. Bull. Seismological Soc. America 103, 1723–1732. doi:10.1785/0120120247
Hajati, T., Langenbruch, C., and Shapiro, S. (2015). A Statistical Model for Seismic hazard Assessment of Hydraulic-Fracturing-Induced Seismicity. Geophys. Res. Lett. 42, 10–601. doi:10.1002/2015gl066652
Hayes, B., Anderson, J., Cooper, M., McLellan, P., Rostron, B., and Clarke, J. (2020). Wastewater Disposal in the Maturing Montney Play Fairway, Northeastern British Columbia (Nts 093p, 094a, B, G, H). Geosci. BC Summ. Activities 2020, 2021.
Helmstetter, A., and Sornette, D. (2003). Båth’s Law Derived from the Gutenberg-Richter Law and from Aftershock Properties. Geophys. Res. Lett. 30. doi:10.1029/2003gl018186
Holschneider, M., Narteau, C., Shebalin, P., Peng, Z., and Schorlemmer, D. (2012). Bayesian Analysis of the Modified Omori Law. J. Geophys. Res. Solid Earth 117. doi:10.1029/2011jb009054
Karimi, K., and Davidsen, J. (2021). Aftershock Triggering and Spatial Aftershock Zones in Fluid-Driven Settings: Discriminating Induced Seismicity from Natural Swarms. Geophys. Res. Lett. 48, e2020GL092267. doi:10.1029/2020gl092267
Khajehdehi, O., Karimi, K., and Davidsen, J. (2022). The Effect of Correlated Permeability on Fluid-Induced Seismicity. Geophys. Res. Lett. 49, e2021GL095199. doi:10.1029/2021gl095199
Kothari, S., Shcherbakov, R., and Atkinson, G. (2020). Statistical Modeling and Characterization of Induced Seismicity within the Western Canada Sedimentary Basin. J. Geophys. Res. Solid Earth 125, e2020JB020606. doi:10.1029/2020jb020606
Langenbruch, C., Dinske, C., and Shapiro, S. A. (2011). Inter Event Times of Fluid Induced Earthquakes Suggest Their Poisson Nature. Geophys. Res. Lett. 38. doi:10.1029/2011gl049474
Lord-May, C., Baró, J., Eaton, D. W., and Davidsen, J. (2020). Seismic hazard Due to Fluid Injections. Phys. Rev. Res. 2, 043324. doi:10.1103/PhysRevResearch.2.043324
Maghsoudi, S., Baró, J., Kent, A., Eaton, D., and Davidsen, J. (2018). Interevent Triggering in Microseismicity Induced by Hydraulic Fracturing. Bull. Seismological Soc. America 108, 1133–1146. doi:10.1785/0120170368
Maghsoudi, S., Eaton, D. W., and Davidsen, J. (2016). Nontrivial Clustering of Microseismicity Induced by Hydraulic Fracturing. Geophys. Res. Lett. 43, 10–672. doi:10.1002/2016gl070983
Martínez-Garzón, P., Kwiatek, G., Sone, H., Bohnhoff, M., Dresen, G., and Hartline, C. (2014). Spatiotemporal Changes, Faulting Regimes, and Source Parameters of Induced Seismicity: A Case Study from the Geysers Geothermal Field. J. Geophys. Res. Solid Earth 119, 8378–8396. doi:10.1002/2014jb011385
Martínez-Garzón, P., Zaliapin, I., Ben-Zion, Y., Kwiatek, G., and Bohnhoff, M. (2018). Comparative Study of Earthquake Clustering in Relation to Hydraulic Activities at Geothermal fields in California. J. Geophys. Res. Solid Earth 123, 4041–4062.
Moradpour, J., Hainzl, S., and Davidsen, J. (2014). Nontrivial Decay of Aftershock Density with Distance in Southern California. J. Geophys. Res. Solid Earth 119, 5518–5535. doi:10.1002/2014jb010940
Mousavi, S. M., Ogwari, P. O., Horton, S. P., and Langston, C. A. (2017). Spatio-temporal Evolution of Frequency-Magnitude Distribution and Seismogenic index during Initiation of Induced Seismicity at Guy-Greenbrier, Arkansas. Phys. Earth Planet. Interiors 267, 53–66. doi:10.1016/j.pepi.2017.04.005
Mulargia, F., Stark, P. B., and Geller, R. J. (2017). Why Is Probabilistic Seismic hazard Analysis (PSHA) Still Used? Phys. Earth Planet. Interiors 264, 63–75. doi:10.1016/j.pepi.2016.12.002
Ommi, S., Zafarani, H., and Smirnov, V. B. (2016). Bayesian Estimation of the Modified Omori Law Parameters for the Iranian Plateau. J. Seismol 20, 953–970. doi:10.1007/s10950-016-9574-8
Pisarenko, V. F., and Rodkin, M. V. (2019). Declustering of Seismicity Flow: Statistical Analysis. Izv. Phys. Solid Earth 55, 733–745.
Salvage, R. O., Dettmer, J., Swinscoe, T., MacDougall, K., Eaton, D. W., Stacey, M., et al. (2021). Real-time Monitoring of Seismic Activity in the Kiskatinaw Area, Northeastern British Columbia (NTS 093P, 094A). Geosci. BC Summ. Activities 2020: Energ. Water 2021, 17–30.
Salvage, R. O., and Eaton, D. W. (2022). The Influence of a Transitional Stress Regime on the Source Characteristics of Induced Seismicity and Fault Activation: Evidence from the 30 November 2018 Fort St. John ML 4.5 Induced Earthquake Sequence. Bull. Seismological Soc. America. doi:10.1785/0120210210
Salvage, R. O., and Eaton, D. W. (2021). Unprecedented Quiescence in Resource Development Area Allows Detection of Long-Lived Latent Seismicity. Solid Earth 12, 765–783. doi:10.5194/se-12-765-2021
Schoenball, M., Baujard, C., Kohl, T., and Dorbath, L. (2012). The Role of Triggering by Static Stress Transfer during Geothermal Reservoir Stimulation. J. Geophys. Res. Solid Earth 117. doi:10.1029/2012jb009304
Schoenball, M., Davatzes, N. C., and Glen, J. M. G. (2015). Differentiating Induced and Natural Seismicity Using Space-Time-Magnitude Statistics Applied to the Coso Geothermal Field. Geophys. Res. Lett. 42, 6221–6228. doi:10.1002/2015gl064772
Schoenball, M., and Ellsworth, W. L. (2017). A Systematic Assessment of the Spatiotemporal Evolution of Fault Activation through Induced Seismicity in Oklahoma and Southern Kansas. J. Geophys. Res. Solid Earth 122, 10–189. doi:10.1002/2017jb014850
Schoenball, M., Walsh, F. R., Weingarten, M., and Ellsworth, W. L. (2018). How Faults Wake up: the Guthrie-Langston, Oklahoma Earthquakes. The Leading Edge 37, 100–106. doi:10.1190/tle37020100.1
Schultz, R., Atkinson, G., Eaton, D. W., Gu, Y. J., and Kao, H. (2018). Hydraulic Fracturing Volume Is Associated with Induced Earthquake Productivity in the Duvernay Play. Science 359, 304–308. doi:10.1126/science.aao0159
Shapiro, S. A., Rentsch, S., and Rothert, E. (2005). Fluid-induced Seismicity: Theory, Modeling, and Applications. J. Eng. Mech. 131, 947–952. doi:10.1061/(asce)0733-9399(2005)131:9(947)
Shebalin, P., and Narteau, C. (2017). Depth Dependent Stress Revealed by Aftershocks. Nat. Commun. 8, 1317–1318. doi:10.1038/s41467-017-01446-y
Shebalin, P. N., Narteau, C., and Baranov, S. V. (2020). Earthquake Productivity Law. Geophys. J. Int. 222, 1264–1269. doi:10.1093/gji/ggaa252
Smirnov, V. B., Ponomarev, A. V., Kartseva, T. I., Mikhailov, V. O., Chadha, R. K., and Aidarov, F. (2018). Dynamics of Induced Seismicity during the Filling of the Nurek Reservoir. Izv., Phys. Solid Earth 54, 641–651. doi:10.1134/s1069351318040110
Smirnov, V. B., Ponomarev, A. V., Stanchits, S. A., Potanina, M. G., Patonin, A. V., Dresen, G., et al. (2019). Laboratory Modeling of Aftershock Sequences: Stress Dependences of the Omori and Gutenberg-Richter Parameters. Izv., Phys. Solid Earth 55, 124–137. doi:10.1134/s1069351319010105
Stephens, M. A. (1970). Use of the Kolmogorov-Smirnov, Cramér-Von Mises and Related Statistics without Extensive Tables. J. R. Stat. Soc. Ser. B (Methodological) 32, 115–122. doi:10.1111/j.2517-6161.1970.tb00821.x
Utsu, T., Ogata, Y., and Matsu’ura, R. S. (1995). The Centenary of the Omori Formula for a Decay Law of Aftershock Activity. J. Phys. Earth 43, 1–33. doi:10.4294/jpe1952.43.1
Van der Elst, N. J., Page, M. T., Weiser, D. A., Goebel, T. H. W., and Hosseini, S. M. (2016). Induced Earthquake Magnitudes Are as Large as (Statistically) Expected. J. Geophys. Res. Solid Earth 121, 4575–4590. doi:10.1002/2016JB012818
Vives, E., Baró, J., and Planes, A. (2017). “From Labquakes in Porous Materials to Earthquakes,” in Avalanches in Functional Materials and Geophysics (Berlin, Germany: Springer), 31–58. doi:10.1007/978-3-319-45612-6_3
Wozniakowska, P., Eaton, D. W., Deblonde, C., Mort, A., and Ardakani, O. H. (2021). Identification of Regional Structural Corridors in the Montney Play Using Trend Surface Analysis Combined with Geophysical Imaging, British Columbia and Alberta. Geol. Surv. Can. 8814. doi:10.4095/328850
Yeck, W. L., Hayes, G. P., McNamara, D. E., Rubinstein, J. L., Barnhart, W. D., Earle, P. S., et al. (2017). Oklahoma Experiences Largest Earthquake during Ongoing Regional Wastewater Injection hazard Mitigation Efforts. Geophys. Res. Lett. 44, 711–717. doi:10.1002/2016gl071685
Yu, H., Harrington, R. M., Liu, Y., and Wang, B. (2019). Induced Seismicity Driven by Fluid Diffusion Revealed by a Near‐Field Hydraulic Stimulation Monitoring Array in the Montney Basin, British Columbia. J. Geophys. Res. Solid Earth 124, 4694–4709. doi:10.1029/2018jb017039
Keywords: induced seismicity, clustering, triggering, aftershocks, seismic hazard assessment
Citation: Khajehdehi O, Eaton DW and Davidsen J (2022) Spatiotemporal Clustering of Seismicity in the Kiskatinaw Seismic Monitoring and Mitigation Area. Front. Earth Sci. 10:894549. doi: 10.3389/feart.2022.894549
Received: 11 March 2022; Accepted: 30 March 2022;
Published: 03 May 2022.
Edited by:
Peter Shebalin, Institute of Earthquake Prediction Theory and Mathematical Geophysics (RAS), RussiaReviewed by:
Alexey Lyubushin, Institute of Physics of the Earth (RAS), RussiaMikhail Rodkin, Institute of Earthquake Prediction Theory and Mathematical Geophysics (RAS), Russia
Copyright © 2022 Khajehdehi, Eaton and Davidsen. This is an open-access article distributed under the terms of the Creative Commons Attribution License (CC BY). The use, distribution or reproduction in other forums is permitted, provided the original author(s) and the copyright owner(s) are credited and that the original publication in this journal is cited, in accordance with accepted academic practice. No use, distribution or reproduction is permitted which does not comply with these terms.
*Correspondence: Omid Khajehdehi, b21pZC5raGFqZWhkZWhpMUB1Y2FsZ2FyeS5jYQ==