- 1Institute of Hydrobiology, Chinese Academy of Sciences, Wuhan, China
- 2China Institute of Water Resources and Hydropower Research, Beijing, China
Habitat degradation has caused reduction in fishery yields in many freshwater ecosystems, particularly recession of water levels in natural lakes. Poyang Lake, the largest freshwater lake and one of the most exploited regions in China, has exhibited a dramatic variation in the water level for decades, especially after the operation of the Three Gorges Dam. We evaluated the long-term dynamics of fishery yields and the relationship to hydrological variability of Poyang Lake from 1990 to 2016. There was a strong positive effect on the annual maximum water level (Hmax), the minimum water level in April (HMmin4), the maximum water level in August (HMmax8), the average water level in October (HMmean10), and the number of days when the water level was above the wet threshold (Wetdays) on fishery yields. The all-subsets regression model identified the best variable combination subset which contains eight hydrological variables (R2 = 0.9493), and the HMmin4, HMmax8, and HMmean10 variables were the most important variable predictor for fishery yields (contributing to 63.03% of the explained variability). The Mann–Kendall test showed that the time series of the fishery yield of Poyang Lake had significant decreasing trends over the past few decades. Moreover, Wetdays, HMmin4, and HMmean10 also showed significantly decreasing abrupt changes, and the abrupt changes’ time of HMmean10 was the same as that of the fishery yield in 2005. The mean fishery yield and HMmean10 dropped from 42,581 tonnes and 14.15 m during 1990–2005 to 27,464 tonnes and 11.78 m during 2006–2016, respectively. This study is critical for implementing effective strategies for the protection of fish resources and lake ecosystems.
Introduction
River–floodplain system integrity is strongly associated with natural flow regimes (Poff et al., 1997; Chovanec and Waringer, 2001), and periodic flooding plays an important role in large river ecosystems (Junk et al., 1989; Poff et al., 1997; Junk, 1999). River–floodplain systems have high biodiversity and high productivity, owing to diverse habitats driven by the changes in frequency, magnitude, and timing of the floods (Welcomme, 1979; Bayley, 1995), which can be crucial for aquatic fauna, especially for fish (Robinson et al., 2002; Górski, 2010).
Floodplains are known as important spawning, nursery, and refuge habitat for riverine fish during the flooding season, which could increase the availability of plant-based food resources and enhance growth and recruitment rates (Prance and Goulding, 1981; Agostinho et al., 2004; Nunn et al., 2007). Conversely, declining water levels trigger mortality processes by constraining fish to small water bodies, where increased fish densities intensify predation rates and poor water quality (Welcomme, 1979; Matthews and Marsh-Matthews, 2003; Castello et al., 2011; Castello et al., 2015). Fluctuation in water levels, thus, influences fishery yields. Extremely high water is considered to increase biomass by enhancing the growth and recruitment rates of fish, while extremely low water is considered to reduce biomass (Halls and Welcomme, 2004). Therefore, fishery yields are linked to natural flood pulse dynamics and are expected to respond to flood alterations (Castello et al., 2015).
However, river–floodplain systems are strongly influenced by global climate change and intensified human activities, while water level decline, frequent floods, and droughts are the main threats faced by lake ecosystems (Paerl et al., 2011; Jian et al., 2015; Li et al., 2020). These changes have an impact on biomass and the production of fishes (Welcomme, 2001).
Poyang Lake is the largest floodplain lake in China and supports high biodiversity and high productivity. Poyang Lake provides critical habitats for nearly half of the entire population of the endangered Yangtze finless porpoise (Neophocaena asiaeorientalis) and nearly 95% of the entire world population of the endangered Siberian crane (Leucogeranus leucogeranus) (Wu et al., 2009; Dronova et al., 2011) and many rare fish species such as seasonal shad (Tenualosa reevesii) (Liu et al., 2019b). Poyang Lake is also the highest in fish biodiversity and fishery productivity in the Yangtze River Basin (Liu et al., 2019b; Zhang et al., 2020).
Since the 2000s, the hydrologic regime of Poyang Lake has undergone significant modification, and the associated ecological impacts have drawn much attention (Mei et al., 2015; Ye et al., 2018). Climate change, operation of the Three Gorges Dam (TGD), and sand mining appear to be the leading causes of the water level decline in Poyang Lake (Li et al., 2020), particularly the operation of TGD (Zhang et al., 2015; Liu et al., 2016). The impacts of the dramatic recession of the water level in Poyang Lake mainly focused on different aspects of the environment, i.e., water quality (Li et al., 2018), landscape (Feng et al., 2016), migratory birds (Tang et al., 2016), and Yangtze finless porpoises (Li et al., 2020), but little focus has been given to its impact on fish resources.
The objective of our study was to quantitatively analyze variations in fishery yields of Poyang Lake and to assess how they respond to fluctuations in the annual water level. For this purpose, we first analyzed the characteristics of the fishery yields and the annual water level in Poyang Lake (1990–2016). Then, we analyzed the relationship between the fishery yield and annual water level. Finally, we examined the influence of hydrological changes on the fishery yield.
Materials and Methods
Study Area
Poyang Lake is located in the middle reaches of the Yangtze River (Figure 1), which is one of the most exploited regions in the Yangtze River Basin (Zhang et al., 2020). Poyang Lake has a length that fluctuates from 74 to 173 km (Feng et al., 2011). In the flood season (April–August), the lake is a large floodplain, which has an area of >4000 km2 and an average depth of about 11.6 m as the water level is approximately 22 m. In the dry season (September–March), the lake transforms into different landscape types, which have an area of<1000 km2 and an average depth of about 3.7 m as the water level is approximately 8 m (Shankman et al., 2006; Wang et al., 2011; Qi et al., 2016).
Data Collection
The data on fishery yields in Poyang Lake were mainly obtained from the Bulletin on the Ecological and Environmental Monitoring Results of the Three Gorges Project from 1997 to 2016. During 1990–1996, the fishery yield data were obtained from Cui and Li (2005). All hydrological data were obtained from the Hydrology Bureau of Jiangxi Province.
For the detailed analyses of water level characteristics, we identified 42 potentially relevant hydrological variables from the daily data records from 1990 to 2016. As a general rule, it is customary for the lake level at the Xingzi station to drop below 12 m as a sign that Poyang Lake has entered the dry period, and the lake level below 10 was extreme droughts, while rising above 16 m as a sign of entering the flood period (Huang et al., 2021). These variables included the annual or monthly water level, the number of days/year ≥16 m was used as an indicator of flood duration, the number of days/year ≥12 m was used as an indicator of wet duration, and the number of days/year ≤10 m was used as an indicator of extreme dry duration. These variables were used as predictor variables for the purpose of modeling the influence of hydrological variables on metrics of fishery production (Table 1, Table 2).
Data Analysis
We performed an all-subsets method of model selection, as implemented using the leaps in R (Lumley and Miller, 2009). The all-subsets regression analysis was conducted using data from 1990 to 2016; the fishery yield was used as the response variable, with 42 hydrological variables used as explanatory variables. This method selects the single best combination of variables for each subset of variables (e.g., 2 variables, 3 variables…42 variables), using a branch and bound search algorithm. The best variable combination for each subset was then compared using BIC, with the combination with the lowest BIC score selected. Correlations between all pairs of fishery yields and hydrological variables were estimated for the best variable combination subsets using a Pearson correlation coefficient.
To determine whether or not a trend exists in time series data of fishery yields and hydrological variables in Poyang Lake, a Mann–Kendall (MK) trend test was conducted. The MK test (Mann, 1945; Kendall, 1975; Gilbert, 1987) is a nonparametric test used to statistically assess if there is a monotonic upward or downward trend of the variable of interest over time. A monotonic upward (downward) trend means that the variable consistently increases (decreases) through time, but the trend may or may not be linear. The MK test can be used in place of a parametric linear regression analysis, which can be used to test if the slope of the estimated linear regression line is different from zero. Significance was assessed at 0.05.
The sequential MK test is useful for finding the year in which a trend starts, abrupt changes in direction, and fluctuations in direction (Bari et al., 2016). This test consists of two sets, progressive series u(t) and backward or retrograde series u′(t). When a progressive series cross ±1.96 (95% confidence limit) and does not return, it indicates that there is existence of a significant increasing or decreasing trend. The abrupt change can be statistically significant when u(t) and u′(t) cross each other beyond straight lines at ± 1.96 (95% confidence limit). The intersection of u(t) with u′(t) in between the straight lines is considered insignificant. The u(t) and u′(t) values can be calculated for the time series (x1, x2, …, xn), according to Partal and Kahya (2006).
Results
Fishery yields in Poyang Lake showed declining trends during 1990–2016. The average decadal yields from the 1990s to 2010s were 46,400, 31,750, and 25,916 tonnes, respectively. The maximum yield of 71,900 tonnes was reported in 1998, and the minimum yield of 22,300 tonnes was reported in 2011.
The multivariate fishery yield predictive equations were generated using all-subsets model methods. Based on the corresponding BIC values, the best variable combination subsets contain Hmax, Flooddays, Wetdays, HMmin4, HMmax6, HMmax8, HMmean10, and HMmin11 (Figure 2). The relative importance of predictor variables of HMmax8, HMmean10, and HMmin4 was relatively higher. There is a positive correlation relationship between fishery yields and Wetdays (r = 0.627, p < 0.001), HMmin4 (r = 0.537, p < 0.01), HMmax8 (r = 0.705, p < 0.001), and HMmean10 (r = 0.6, p < 0.01) (Figure 3).
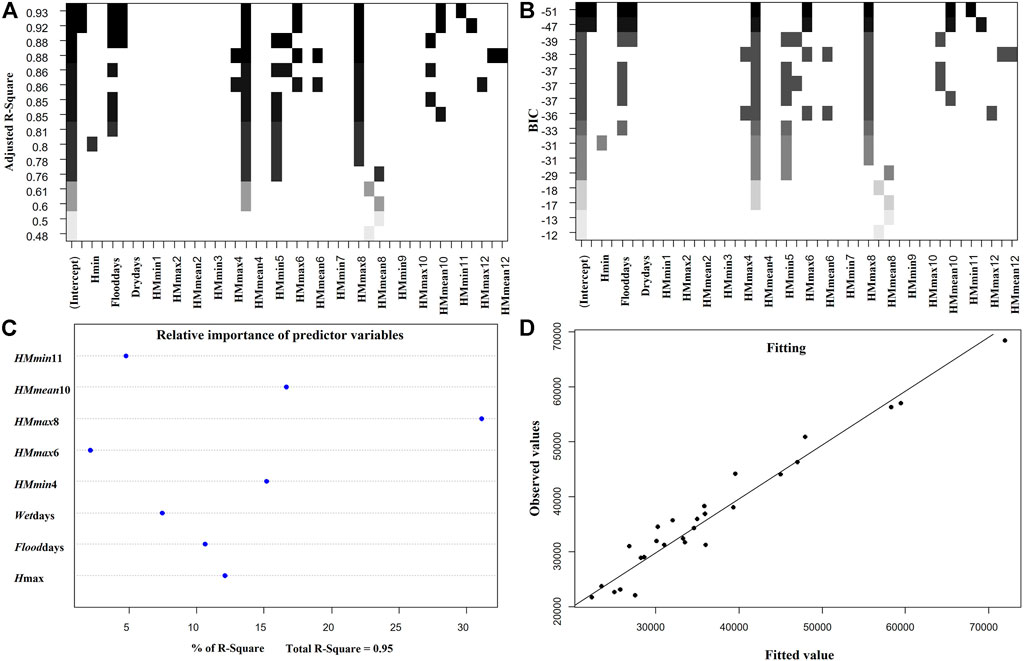
FIGURE 2. Plots of adjusted R2 (A), BIC (B), the relative importance of predictor variables (C), and the fitted value of fishery yields (D) for all-subset regression analyses.
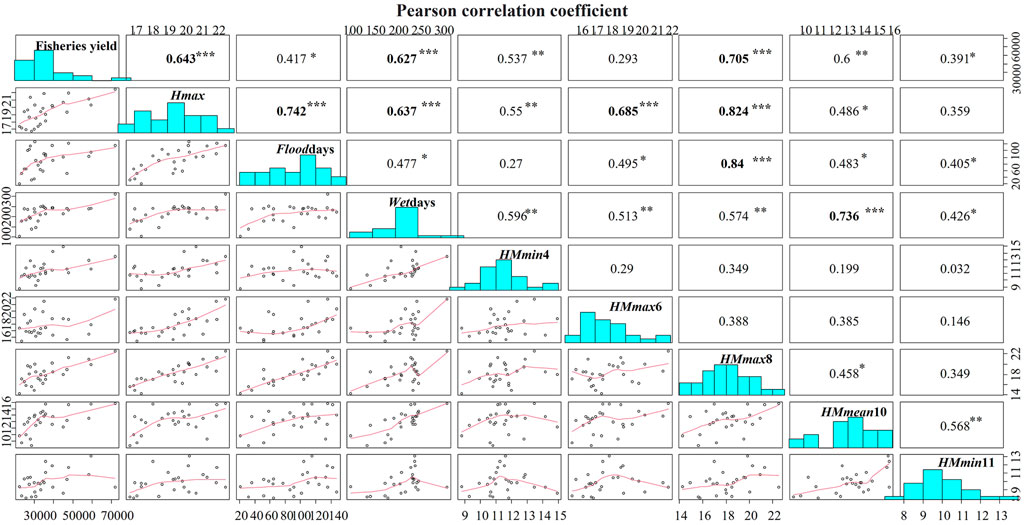
FIGURE 3. Pearson correlation coefficient matrix of best variable combination subsets in all-subsets regression models (Signif. codes: < 0.001∗∗∗, <0.01∗∗, and <0.05∗).
The MK trend analysis and change point detection show that fishery yields, Wetdays, HMmin4, and HMmean10 have significant decreasing trends (p < 0.05) (Table 3). An insignificant decreasing trend was observed for other hydrological variables in the best variable combination subsets.
The results for the annual visibility time series suggested that the fishery yields, Wetdays, HMmin4, and HMmean10 have one significant change point (Figure 4). For instance, fishery yields and HMmean10 have one significant change point (abrupt change) in 2005. This significant change point is observed based on the intersection between u(t) and u′(t) under the dotted line, which is a threshold of −1.96 (95% confidence limit). The Wetdays and HMmin4 variables have a significant abrupt change in 2000 and 1998, respectively.
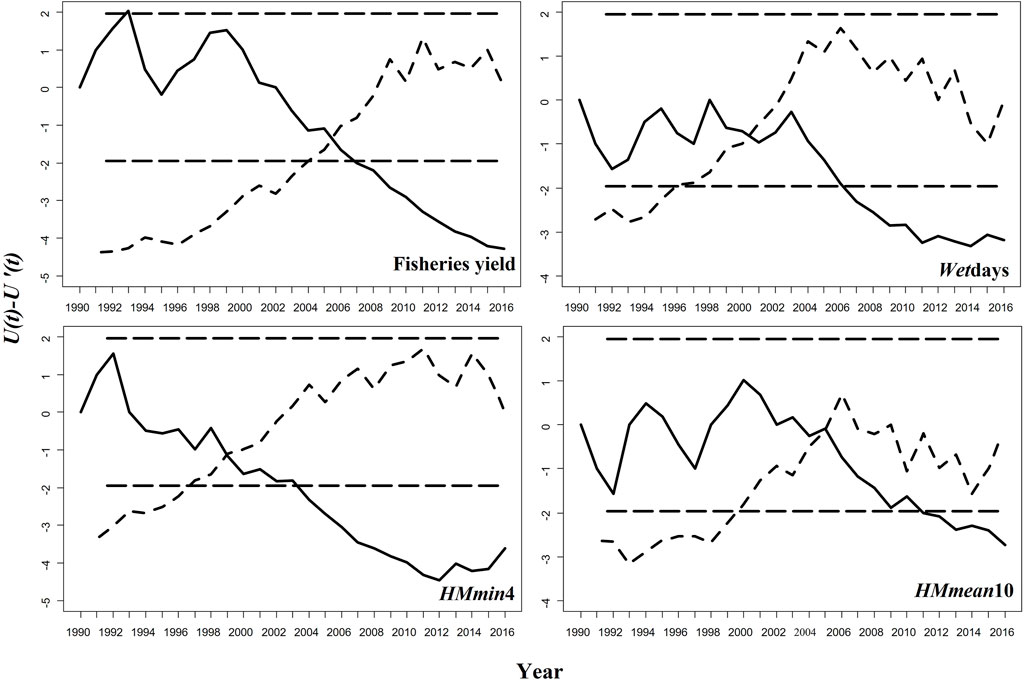
FIGURE 4. MK test statistics for fishery yields and hydrological visibility. The dotted horizontal straight lines represent the upper and lower limits of the 95% confidence interval.
Discussion
Fishery Yields Responding to Hydrological Alterations
Water level fluctuations are among the major driving forces for shallow lake ecosystems and play an important role in the structure and function of these ecosystems (Coops et al., 2003). This study contributes to understanding the complex interannual dynamics of floodplain lake fisheries. Based on the all-sub regression model, the best variable combination subsets contain eight hydrological variables, and the fitting effect is good (R2 = 0.9493).
HMmin4, HMmax8, and HMmean10 were the most important variable predictors for fishery yields, which contributed 63.03% of the explained variability in yield. The start of the flooding time of Poyang Lake is in April. When the water level rises, terrestrial vegetation is submerged, resulting in better spawning conditions for many fish species in Poyang Lake (Ding, 2017). Moreover, plankton and fish production would be increased because of nutrients from inflowing water and leaching from decomposing organic matter (dung, terrestrial grass, and shrubs) (Liu et al., 2019a). In August, flooding of the marginal areas results in excellent conditions for the growth and survival of juveniles of both lake-residence fish and river–lake migratory fish (Tan et al., 2015; Zhang et al., 2021). In October, the drainage from Poyang Lake determines the feeding time of fish (Zeng, 1990). This strong relationship between fish productivity and water level fluctuation is also proved in many floodplain lakes (Karenge and Kolding, 1995; Talling, 2001; Kolding and van Zwieten, 2012).
Anthropogenic Disturbances Result in the Lake Water Decline Affect the Fishery Yields
This study shows that the recession of the water level is becoming more frequent and intense in Poyang Lake, strongly affecting the freshwater fisheries. The mean fishery yields and HMmean10 dropped from 42,581 tonnes and 14.15 m during 1990–2005 to 27,464 tonnes and 11.78 m during 2006–2016, respectively. The same phenomenon has been discovered in the Kafue River in Africa, where the low waters of an average hydrological year decreased by 40% the fish biomass found in the preceding high waters (Caraballo et al., 2014).
It was well documented that the lake area was shrinking, and the water level was continuously declining in Poyang Lake (Feng et al., 2012; Lai et al., 2014b; Yao et al., 2018). Since 2000, the dry season has advanced and been prolonged, and the water decline has accelerated in Poyang Lake (Dronova et al., 2011; Lai et al., 2014a; Cheng et al., 2019). The establishment of the TGD and the extensive sand mining were considered the two major factors that have led to hydrological issues in Poyang Lake (Wu et al., 2007; Guo et al., 2012).
The flow regimes in the mid-lower reaches of the Yangtze River after the TGD began operating were completely different from those during the pre-dam period (Chai et al., 2019), especially during the impoundment periods of TGD from September to November (Lai et al., 2014a). Poyang Lake is naturally connected with the middle-lower Yangtze River. Thus, the TGD has a remarkable effect on the decrease in the water level of Poyang Lake (Huang et al., 2021) and is thought to intensify drought during the dry season of Poyang Lake (Zhang et al., 2012; Li et al., 2020), which would negatively affect the feeding grounds and wintering grounds of fish in Poyang Lake. The abrupt changes in time of HMmean10 and fishery yields of Poyang Lake were in 2005,just the year before the second impoundment of TGD (raised the water level to 156 m) (Zhang et al., 2016). The contribution of water storage of the TGD to the decline in the water level of Poyang Lake from late September to mid-October is approximately 60% (Wang et al., 2019).
Conclusion
This study shows that water level fluctuations are the major driving forces for the fishery yields of Poyang Lake, and the recession of the water level affects strongly on freshwater fisheries, especially the water level in October. Previous research has detailed the establishment of the TGD as one of the important events that led to extreme changes in the hydrology conditions in Poyang Lake. Moreover, the recent water decline in Poyang Lake should not be viewed as a long-term natural trend but as a regime shift (Liu et al., 2013; Li et al., 2020). It will be practical for future studies to focus on the impacts of the decreasing water level to better understand the different ecosystem processes of Poyang Lake to the water decline. These efforts will serve to better protect and ensure the continued health of the ecosystem.
Data Availability Statement
The raw data supporting the conclusions of this article will be made available by the authors, without undue reservation.
Author Contributions
ML: conceptualization, methodology, formal analysis, writing—original draft, writing—review and editing, and supervision. CL: data curation and formal analysis. FL: methodology and funding acquisition. JW: conceptualization, methodology, and funding acquisition. HL: supervision and project administration.
Funding
This work was financially supported by the National Key Research & Development Program of China (2018YFD0900801), the National Natural Science Foundation of China (31801982), the Biodiversity Survey and Assessment Project of the Ministry of Ecology and Environment, China (2019HJ2096001006), and Chinese Three Gorges Corporation (No: 202003229).
Conflict of Interest
The authors declare that the research was conducted in the absence of any commercial or financial relationships that could be construed as a potential conflict of interest.
Publisher’s Note
All claims expressed in this article are solely those of the authors and do not necessarily represent those of their affiliated organizations, or those of the publisher, the editors, and the reviewers. Any product that may be evaluated in this article, or claim that may be made by its manufacturer, is not guaranteed or endorsed by the publisher.
References
Agostinho, A. A., Gomes, L. C., Veríssimo, S., and Okada, E. (2004). Flood Regime, Dam Regulation and Fish in the Upper Paraná River: Effects on Assemblage Attributes, Reproduction and Recruitment. Rev. Fish. Biol. Fish. 14 (1), 11–19. doi:10.1007/s11160-004-3551-y
Bari, S. H., Rahman, M. T. U., Hoque, M. A., and Hussain, M. M. (2016). Analysis of Seasonal and Annual Rainfall Trends in the Northern Region of Bangladesh. Atmos. Res. 176-177, 148–158. doi:10.1016/j.atmosres.2016.02.008
Bayley, P. B. (1995). Understanding Large River: Floodplain Ecosystems. Bioscience 45 (3), 153–158. doi:10.2307/1312554
Caraballo, P., Forsberg, B. R., Almeida, F. F. d., and Leite, R. G. (2014). Diel Patterns of Temperature, Conductivity and Dissolved Oxygen in an Amazon Floodplain Lake: Description of a Friagem Phenomenon. Acta Limnol. Bras. 26 (3), 318–331. doi:10.1590/s2179-975x2014000300011
Castello, L., Stewart, D. J., and Arantes, C. C. (2011). Modeling Population Dynamics and Conservation of arapaima in the Amazon. Rev. Fish. Biol. Fish. 21 (3), 623–640. doi:10.1007/s11160-010-9197-z
Castello, L., Isaac, V. J., and Thapa, R. (2015). Flood Pulse Effects on Multispecies Fishery Yields in the Lower Amazon. R. Soc. open Sci. 2 (11), 150299. doi:10.1098/rsos.150299
Chai, Y., Li, Y., Yang, Y., Zhu, B., Li, S., Xu, C., et al. (2019). Influence of Climate Variability and Reservoir Operation on Streamflow in the Yangtze River. Sci. Rep. 9 (1), 5060. doi:10.1038/s41598-019-41583-6
Cheng, J., Xu, L., Feng, W., Fan, H., and Jiang, J. (2019). Changes in Water Level Regimes in China's Two Largest Freshwater Lakes: Characterization and Implication. Water 11 (5), 917. doi:10.3390/w11050917
Chovanec, A., and Waringer, J. (2001). Ecological Integrity of River-Floodplain Systems?assessment by Dragonfly Surveys (Insecta: Odonata). Regul. Rivers Res. Mgmt. 17, 493–507. doi:10.1002/rrr.664
Coops, H., Beklioglu, M., and Crisman, T. L. (2003). The Role of Water-Level Fluctuations in Shallow Lake Ecosystems - Workshop Conclusions. Hydrobiologia 506-509 (1), 23–27. doi:10.1023/B:HYDR.0000008595.14393.77
Cui, Y. B., and Li, Z. J. (2005). Fishery Resources and Conservation of Environment in Lakes of the Changjiang River Basin. Beijing, China: Science Press.
Ding, F. (2017). Study On Spawning Field of Fish Spawning on Plants in Dahuchi Lake Using Remote Sensing Technique Master. Shanghai: Shanghai Ocean University.
Dronova, I., Gong, P., and Wang, L. (2011). Object-based Analysis and Change Detection of Major Wetland Cover Types and Their Classification Uncertainty during the Low Water Period at Poyang Lake, China. Remote Sens. Environ. 115 (12), 3220–3236. doi:10.1016/j.rse.2011.07.006
Feng, L., Hu, C., Chen, X., and Li, R. (2011). Satellite Observations Make it Possible to Estimate Poyang Lake's Water Budget. Environ. Res. Lett. 6 (4), 044023. doi:10.1088/1748-9326/6/4/044023
Feng, L., Hu, C., Chen, X., Cai, X., Tian, L., and Gan, W. (2012). Assessment of Inundation Changes of Poyang Lake Using MODIS Observations between 2000 and 2010. Remote Sens. Environ. 121, 80–92. doi:10.1016/j.rse.2012.01.014
Feng, W., Xu, L., Wang, X., Li, H., and Jiang, J. (2016). Response of Carex Cinerascens Populations to Groundwater Level Gradients in the Poyang Lake Wetland. Acta Ecol. Sin. 36 (16), 5109–5115. doi:10.5846/stxb201501220180
Górski, K. (2010). Floods and Fish : Recruitment and Distribution of Fish in the Volga River Floodplain. Wageningen: WUR Wageningen.
Guo, H., Hu, Q., Zhang, Q., and Feng, S. (2012). Effects of the Three Gorges Dam on Yangtze River Flow and River Interaction with Poyang Lake, China: 2003-2008. J. Hydrology 416-417, 19–27. doi:10.1016/j.jhydrol.2011.11.027
Halls, A. S., and Welcomme, R. L. (2004). Dynamics of River Fish Populations in Response to Hydrological Conditions: a Simulation Study. River Res. applic. 20 (8), 985–1000. doi:10.1002/rra.804
Huang, S., Xia, J., Zeng, S., Wang, Y., and She, D. (2021). Effect of Three Gorges Dam on Poyang Lake Water Level at Daily Scale Based on Machine Learning. J. Geogr. Sci. 31 (11), 1598–1614. doi:10.1007/s11442-021-1913-1
Jian, W., Yingchang, C., Huimin, L., and Yongjin, G. (2015). Formation Conditions and Sedimentary Model of Over-flooding Lake Deltas within Continental Lake Basins: An Example from the Paleogene in the Jiyang Subbasin, Bohai Bay Basin. Acta Geol. Sin. - Engl. Ed. 89 (1), 270–284. doi:10.1111/1755-6724.12410
Junk, W., Bayley, P., and Sparks, R. (1989). The Flood Pulse Concept in River-Floodplain Systems. Ottawa: Canadian Special Publication of Fisheries and Aquatic Sciences, 110–127.
Junk, W. J. (1999). The Flood Pulse Concept of Large Rivers: Learning from the Tropics. River Syst. 11 (3), 261–280. doi:10.1127/lr/11/1999/261
Karenge, L., and Kolding, J. (1995). On the Relationship between Hydrology and Fisheries in Man-Made Lake Kariba, Central Africa. Fish. Res. 22 (3), 205–226. doi:10.1016/0165-7836(94)00325-Q
Kolding, J., and van Zwieten, P. A. M. (2012). Relative Lake Level Fluctuations and Their Influence on Productivity and Resilience in Tropical Lakes and Reservoirs. Fish. Res. 115-116, 99–109. doi:10.1016/j.fishres.2011.11.008
Lai, X., Jiang, J., Yang, G., and Lu, X. X. (2014a). Should the Three Gorges Dam Be Blamed for the Extremely Low Water Levels in the Middle-Lower Yangtze River? Hydrol. Process. 28 (1), 150–160. doi:10.1002/hyp.10077
Lai, X., Shankman, D., Huber, C., Yesou, H., Huang, Q., and Jiang, J. (2014b). Sand Mining and Increasing Poyang Lake's Discharge Ability: A Reassessment of Causes for Lake Decline in China. J. Hydrology 519, 1698–1706. doi:10.1016/j.jhydrol.2014.09.058
Li, B., Yang, G., Wan, R., and Li, H. (2018). Hydrodynamic and Water Quality Modeling of a Large Floodplain Lake (Poyang Lake) in China. Environ. Sci. Pollut. Res. 25 (35), 35084–35098. doi:10.1007/s11356-018-3387-y
Li, Q., Lai, G., and Devlin, A. T. (2020). A Review on the Driving Forces of Water Decline and its Impacts on the Environment in Poyang Lake, China. J. Water Clim. Change 12 (5), 1370–1391. doi:10.2166/wcc.2020.216
Liu, Y., Wu, G., and Zhao, X. (2013). Recent Declines in China's Largest Freshwater Lake: Trend or Regime Shift? Environ. Res. Lett. 8 (1), 014010. doi:10.1088/1748-9326/8/1/014010
Liu, Y., Wu, G., Guo, R., and Wan, R. (2016). Changing Landscapes by Damming: the Three Gorges Dam Causes Downstream Lake Shrinkage and Severe Droughts. Landsc. Ecol. 31 (8), 1883–1890. doi:10.1007/s10980-016-0391-9
Liu, J., Chen, Y., Li, M., Liu, B., Liu, X., Wu, Z., et al. (2019a). Water-level Fluctuations Are Key for Phytoplankton Taxonomic Communities and Functional Groups in Poyang Lake. Ecol. Indic. 104, 470–478. doi:10.1016/j.ecolind.2019.05.021
Liu, X., Qin, J., Xu, Y., Zhou, M., Wu, X., and Ouyang, S. (2019b). Biodiversity Pattern of Fish Assemblages in Poyang Lake Basin: Threat and Conservation. Ecol. Evol. 9 (20), 11672–11683. doi:10.1002/ece3.5661
Mann, H. B. (1945). Non-parametric Tests against Trend. Econometrica 13, 163–171. doi:10.2307/1907187
Matthews, W. J., and Marsh-Matthews, E. (2003). Effects of Drought on Fish across Axes of Space, Time and Ecological Complexity. Freshw. Biol. 48 (7), 1232–1253. doi:10.1046/j.1365-2427.2003.01087.x
Mei, X., Dai, Z., Du, J., and Chen, J. (2015). Linkage between Three Gorges Dam Impacts and the Dramatic Recessions in China's Largest Freshwater Lake, Poyang Lake. Sci. Rep. 5, 9. doi:10.1038/srep18197
Nunn, A. D., Harvey, J. P., and Cowx, I. G. (2007). Benefits to 0+ Fishes of Connecting Man-Made Waterbodies to the Lower River Trent, England. River Res. applic. 23 (4), 361–376. doi:10.1002/rra.993
Paerl, H. W., Hall, N. S., and Calandrino, E. S. (2011). Controlling Harmful Cyanobacterial Blooms in a World Experiencing Anthropogenic and Climatic-Induced Change. Sci. Total Environ. 409 (10), 1739–1745. doi:10.1016/j.scitotenv.2011.02.001
Partal, T., and Kahya, E. (2006). Trend Analysis in Turkish Precipitation Data. Hydrol. Process. 20 (9), 2011–2026. doi:10.1002/hyp.5993
Poff, N. L., Allan, J. D., Bain, M. B., Karr, J. R., Prestegaard, K. L., Richter, B. D., et al. (1997). The Natural Flow Regime. BioScience 47 (11), 769–784. doi:10.2307/1313099
Prance, G. T., and Goulding, M. (1981). The Fishes and the Forest: Explorations in Amazonian Natural History. Brittonia 33, 257. doi:10.2307/2806336
Qi, H., Lu, J., Chen, X., Sauvage, S., and Sanchez-Pérez, J.-M. (2016). Water Age Prediction and its Potential Impacts on Water Quality Using a Hydrodynamic Model for Poyang Lake, China. Environ. Sci. Pollut. Res. 23 (13), 13327–13341. doi:10.1007/s11356-016-6516-5
Robinson, C. T., Tockner, K., and Ward, J. V. (2002). The Fauna of Dynamic Riverine Landscapes. Freshw. Biol. 47 (4), 661–677. doi:10.1046/j.1365-2427.2002.00921.x
Shankman, D., Keim, B. D., and Song, J. (2006). Flood Frequency in China's Poyang Lake Region: Trends and Teleconnections. Int. J. Climatol. 26 (9), 1255–1266. doi:10.1002/joc.1307
Talling, J. F. (2001). Environmental Controls on the Functioning of Shallow Tropical Lakes. Hydrobiologia 458 (1), 1–8. doi:10.1023/A:1013121522321
Tan, Y., Yu, Y., Ding, J., and Tan, H. (2015). Control Effects of Lake Poyang Hydrological Process on the Wetland Biology. J. Lake Sci. 27 (6), 997–1003. (in Chniese). doi:10.18307/2015.0602
Tang, X., Li, H., Xu, X., Yang, G., Liu, G., Li, X., et al. (2016). Changing Land Use and its Impact on the Habitat Suitability for Wintering Anseriformes in China's Poyang Lake Region. Sci. Total Environ. 557-558, 296–306. doi:10.1016/j.scitotenv.2016.03.108
Wang, Y., Yu, X., Li, W., Xu, J., Chen, Y., and Fan, N. (2011). Potential Influence of Water Level Changes on Energy Flows in a Lake Food Web. Chin. Sci. Bull. 56 (26), 2794–2802. doi:10.1007/s11434-011-4649-y
Wang, D., Zhang, S., Wang, G., Han, Q., Huang, G., Wang, H., et al. (2019). Quantitative Assessment of the Influences of Three Gorges Dam on the Water Level of Poyang Lake, China. Water 11 (7), 1519. doi:10.3390/w11071519
Wu, G., de Leeuw, J., Skidmore, A. K., Prins, H. H. T., and Liu, Y. (2007). Concurrent Monitoring of Vessels and Water Turbidity Enhances the Strength of Evidence in Remotely Sensed Dredging Impact Assessment. Water Res. 41 (15), 3271–3280. doi:10.1016/j.watres.2007.05.018
Wu, G., de Leeuw, J., Skidmore, A. K., Prins, H. H. T., Best, E. P. H., and Liu, Y. (2009). Will the Three Gorges Dam Affect the Underwater Light Climate of Vallisneria Spiralis L. And Food Habitat of Siberian Crane in Poyang Lake? Hydrobiologia 623 (1), 213–222. doi:10.1007/s10750-008-9659-7
Yao, J., Zhang, Q., Ye, X., Zhang, D., and Bai, P. (2018). Quantifying the Impact of Bathymetric Changes on the Hydrological Regimes in a Large Floodplain Lake: Poyang Lake. J. Hydrology 561, 711–723. doi:10.1016/j.jhydrol.2018.04.035
Ye, X., Xu, C.-Y., Zhang, Q., Yao, J., and Li, X. (2018). Quantifying the Human Induced Water Level Decline of China's Largest Freshwater Lake from the Changing Underlying Surface in the Lake Region. Water Resour. Manage 32 (4), 1467–1482. doi:10.1007/s11269-017-1881-5
Zhang, Q., Li, L., Wang, Y. G., Werner, A. D., Xin, P., Jiang, T., et al. (2012). Has the Three‐Gorges Dam Made the Poyang Lake Wetlands Wetter and Drier? Geophys. Res. Lett. 39 (20). doi:10.1029/2012GL053431
Zhang, Z., Chen, X., Xu, C.-Y., Hong, Y., Hardy, J., and Sun, Z. (2015). Examining the Influence of River-Lake Interaction on the Drought and Water Resources in the Poyang Lake Basin. J. Hydrology 522, 510–521. doi:10.1016/j.jhydrol.2015.01.008
Zhang, X., Dong, Z., Gupta, H., Wu, G., and Li, D. (2016). Impact of the Three Gorges Dam on the Hydrology and Ecology of the Yangtze River. Water 8 (12), 590. doi:10.3390/w8120590
Zhang, H., Kang, M., Shen, L., Wu, J., Li, J., Du, H., et al. (2020). Rapid Change in Yangtze Fisheries and its Implications for Global Freshwater Ecosystem Management. Fish. Fish. 21 (3), 601–620. doi:10.1111/faf.12449
Keywords: fishery yield, recession of water levels, Three Gorges Dam, Mann-Kendall test, all-subsets regression
Citation: Li M, Liu C, Liu F, Wang J and Liu H (2022) Decrease in Fishery Yields in Response to Hydrological Alterations in the Largest Floodplain Lake (Poyang Lake) in China. Front. Earth Sci. 10:878439. doi: 10.3389/feart.2022.878439
Received: 18 February 2022; Accepted: 21 April 2022;
Published: 26 May 2022.
Edited by:
Xijun Lai, Nanjing Institute of Geography and Limnology (CAS), ChinaReviewed by:
Wu Xiaoping, Nanchang University, ChinaHui Zhang, Chinese Academy of Fishery Sciences (CAFS), China
Copyright © 2022 Li, Liu, Liu, Wang and Liu. This is an open-access article distributed under the terms of the Creative Commons Attribution License (CC BY). The use, distribution or reproduction in other forums is permitted, provided the original author(s) and the copyright owner(s) are credited and that the original publication in this journal is cited, in accordance with accepted academic practice. No use, distribution or reproduction is permitted which does not comply with these terms.
*Correspondence: Huanzhang Liu, hzliu@ihb.ac.cn