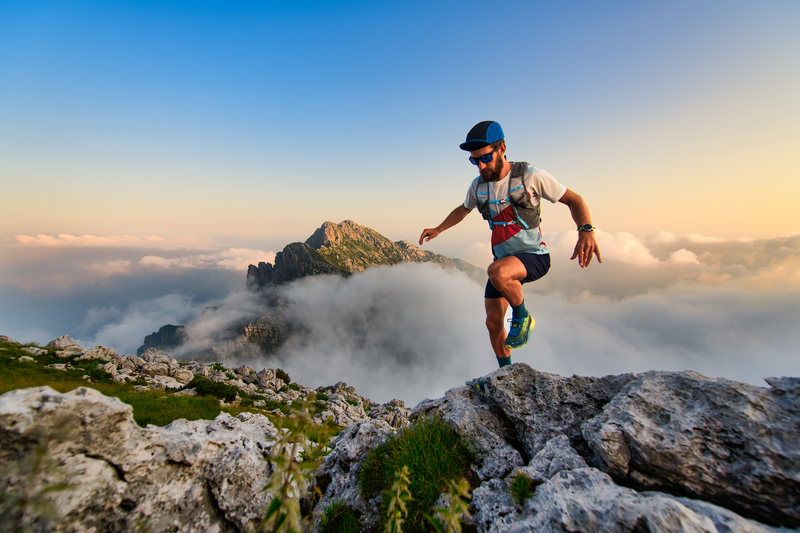
94% of researchers rate our articles as excellent or good
Learn more about the work of our research integrity team to safeguard the quality of each article we publish.
Find out more
ORIGINAL RESEARCH article
Front. Earth Sci. , 24 May 2022
Sec. Hydrosphere
Volume 10 - 2022 | https://doi.org/10.3389/feart.2022.876286
This article is part of the Research Topic The Impact of Three Gorges Dam on Downstream River-Lake System View all 9 articles
The Three Gorges Reservoir (TGR) operation has profoundly altered the hydrological regimes and ecosystem in the middle and lower Yangtze River basin. Its impacts on the river flow regime, sedimentation, lake level decline, and biodiversity have been extensively studied. However, the quantitative influence of the TGR on the downstream water exchange between the Yangtze River and Poyang Lake is lacking attention. In this study, we developed a machine learning model to investigate the river–lake water exchange variations under the TGR operation in typical hydrological years, compared with the natural scenario (i.e., no TGR). The results show that the machine learning model could provide a fast and accurate approach to identify the long-distance impact of reservoirs. The operation of the TGR has significant effects on the water exchange between the Yangtze River and Poyang Lake in the impounding period, with a 10,000 m³/s decrease in reservoir discharge approximately causing a 4,000 m³/s decrease in river–lake water exchange in the dry year and a decrease of 6,000 m³/s and 8,000 m³/s in reservoir discharge causing an increase of 4,000 m³/s and 6,000 m³/s in the normal year and the wet year, respectively. The TGR effect varies with different hydrological conditions of the river and lake, showing longer time range (from May to October) and greater degree (1.5 times the change rate in other years) in the wet year. The TGR operation is beneficial to the water maintenance of the lake in the dry year and flood control in the lake area in the wet year. This study provides a constructive approach and valuable information for decision making in water resource management and ecosystem protection in large river–lake systems.
With the development of society and economy, large numbers of dams and reservoirs have been constructed, and nearly two-thirds of the world’s large rivers are currently affected by damming (Nilsson et al., 2005; Taylor et al., 2020; Chen et al., 2021). Reservoirs not only play important roles in flood control, power generation, water supply, and shipping but also profoundly alter the downstream hydrological regimes and lead to severe consequences on the riverine ecological and morphological equilibrium (Ferrazzi and Botter, 2019; Hecht, et al., 2019; Dai et al., 2021). Reservoir regulation alters the magnitude, frequency, duration, timing, and rate of change of flow and, thus, modifies the channel scouring and siltation and sediment transportation (Poff et al., 2007; Destouni et al., 2012; Dai et al., 2013). Large lowland rivers often have extensive floodplains (for example, the Amazon, Mekong, Congo, and Yangtze rivers), and the river–floodplain environments exhibit some of the highest levels of biodiversity and productivity in the world, and the upstream reservoirs would also change the exchange of water, sediments, nutrients, and biota between the river channel and the floodplain (including river-connected lakes) (Winemiller et al., 2016; Timpe, and Kaplan, 2017).
The Three Gorges Reservoir (TGR), located in the middle reach of the Yangtze River in China, is the largest hydroelectric project in the world with a total storage capacity of 39.3 billion m3 at the normal water level of 175 m and a regulation storage capacity of 22.15 billion m3, having comprehensive benefits of power generation, flood control, and navigation (Zhao et al., 2015; Su et al., 2020; Guo et al., 2021). Its construction and operation also significantly change the hydrological regime, sedimentation, and ecosystem of the middle and lower reaches of the Yangtze River and, thus, alter the downstream river–lake interactions (Gao et al., 2013; Lai et al., 2014b; Han et al., 2021; Ren et al., 2021). Poyang Lake is one of the two larger river-connected lakes in the Yangtze River basin and the largest freshwater lake in China, which is not only the natural flood diversion area of the Yangtze River and water resources for millions of people but also the first batch of the Ramsar Convention List of Wetlands of International Importance and is on the list of globally important ecological areas designated by the World Wide Fund For Nature (Liu and Liu, 2012; Li et al., 2021). It has received great attention because of both its importance and dramatic hydrological alterations, and the impact of the TGR on it is one of the hot spots (Shankman et al., 2006; Lai et al., 2014a; Hou et al., 2018; Xu et al., 2020).
The impacts of the TGR on the water regime and ecological environment of Poyang Lake have been studied by means of data analysis methods, hydrodynamic models, and remote sensing. Based on the extensive hydrological data, statistical analysis has been conducted to demonstrate the TGR operation effects on the downstream river discharge and water levels and surface areas of Poyang Lake. The results showed that the water level of Poyang Lake reduced over the dry period, and the lake water storage also decreased after the activation of the TGR. (Zhang et al., 2012; Wang et al., 2017; Zhou et al., 2019; Dai, et al., 2021). Some researches established the hydrodynamic models to investigate the effects of the TGR on water level and lake outflow, and found that the lake stage was lowered and the water level of lake outlet would fall 0.4–1.6 m and that inside Poyang Lake wound fall 0.3–1.2 m due to the water storing of the reservoir (Lai, et al., 2012; Chen and Xu, 2013; Yang, et al., 2020). Moreover, models based on the time-series satellite data have been developed to simulate the lake storage variation and wetland vegetation pattern responding to water level changes in Poyang lake before and after the TGR impoundment (Hu et al., 2015; Li, et al., 2020; Xu et al., 2020).
Most existing research achievements are currently focused on the change of water level, surface water area, and water storage of Poyang Lake under the TGR operation (Li et al., 2016; Fan et al., 2019; Dai et al., 2021). However, the river–lake water exchange is also influenced by the TGR. Variation of the water exchange will modify the dynamic equilibrium of the river and lake, and affects the hydrological regime of both Poyang Lake and the downstream Yangtze River. Considering from a system perspective, the water exchange between the Yangtze River and Poyang Lake can be seen as a vital indicator for the system element association. As the TGR is located at the upstream of the Yangtze River and the direct impact of the reservoir operation is on the downstream river, its impact on the river-connected lake (e.g., Poyang Lake) is through the river–lake interaction at the junction. Water exchange is the representative of the river–lake interactions and helps to explain the mechanism how the TGR on the Yangtze River affects its connected lakes. Even though the influence of the TGR on the water regime variation of Poyang Lake has been studied extensively, its impact on the river–lake water exchange still needs to be investigated. Fang et al. (2012) calculated the Poyang Lake discharge variation caused by the change of the discharge of Jiujiang station on the Yangtze River based on the relationship between the water level of the lake outlet and the discharge of the Yangtze mainstream and the discharge capacity of the outlet section at Poyang Lake. Fan et al. (2019) proposed an empirical formula for Poyang Lake discharge calculation and gave the result that if the water level at the Poyang Lake outlet remains, the lake outflow will decrease about 723 m3/s when the discharge of Jiujiang station increases by 1,000 m3/s. Zhang et al. (2019) examined the hydrological changes in the other river–lake system in the Yangtze River basin (the Yangtze River and Dongting Lake system) based on a coupled hydrodynamic model and the results showed that the diverted flow from the Yangtze River into Dongting Lake and outflow from the lake back to the river drastically decreased during the impounding periods. However, the empirical formula could not be completely applicable to different river–lake situations and the hydrodynamic models for the large river–lake systems are usually accompanied with heavy computation burdens. There is still a lack of necessary quantitative analysis of the TGR under different operational schemes, and a fast and accurate model to identify the long-distance impact of reservoirs is also needed.
In view of this, a machine learning model is established in this study to provide a constructive approach to simulate the river–lake water exchange affected by the far upstream reservoir. The river–lake water exchange variations between the Yangtze River and Poyang Lake under the impact of the TGR are investigated in different operational schemes of typical hydrological years. Then, the change of water exchange is quantitatively analyzed compared to the natural scenario (i.e., no TGR), and its relationship with the change of river discharge altered when the TGR is explored.
Located in the middle and lower reaches of the Yangtze River, Poyang Lake plays a vital role in the protection and development of the Yangtze River and the global ecological environment (Guo et al., 2012). Poyang Lake is a representative of river-connected lakes that are freely connected to the mainstream of the Yangtze River. Apart from supplying water to a population of 11.13 million in the surrounding areas, the lake is a vital spawning habitat for fish in the Yangtze River. As one of the most important wetlands in the world, Poyang Lake ranges from 500 km2 in the dry season to 4,125 km2 in the wet season (Lai et al., 2014b), sustaining approximately 3,000 km2 of grassland around, and provides a winter habitat for more than 95% of the white cranes in the world (Cui, 2004; Li et al., 2019). Poyang Lake receives water from five rivers including the Ganjiang River, Fuhe River, Xinjiang River, Raohe River, and Xiushui River, and then flows into the Yangtze River through Hukou. The water level of Poyang Lake is affected by all the five rivers and the Yangtze River, resulting in a complex relationship between the rivers and lakes with a unique hydrological rhythm in the wet and dry seasons. In recent years, the evolution of hydrological interactions of the Yangtze River and Poyang Lake is increasingly affected by global climate change and human activities (Yang et al., 2016). Poyang Lake has faced many water problems, such as early-occurring and prolonged dry seasons, low water level, shortage of water resources, and ecological degradation of wetland, which affect the water use and ecological security of the lake and draw widespread attention (Ouyang and Liu, 2014; Li et al., 2021).
The lake exchanges water with the Yangtze River through a long and narrow reach in the northern lake area. The outflow of the Yangtze River and Poyang Lake meets near Hukou County, and the junction is an important control area to connect and realize the material exchange between the river and lake (Tang et al., 2020). The controlling station at the outlet of Poyang Lake is the Hukou hydrology station (Huang et al., 2014), whose water level shows strong positive correlation with the mainstream of the Yangtze River, and the correlation has no significant change after the operation of the TGR (Fang et al., 2012; Mei et al., 2015). From April to June, the water level in Poyang Lake rises with the flood of five rivers, and the water level remains high in July to September due to the jacking or backflow of the flood of the Yangtze River (Liu, 2007). In general, the flood season is from April to September and the dry season is from October to March of the next year. Poyang Lake exchanges water with the Yangtze River at Hukou station, which plays a significant role in regulating and storing water. Water is replenished from the lake to the river most of the time of the year, with the average annual outflow to the Yangtze River being 14.6 billion m3, and flows backward from the Yangtze river into the lake for several days in the wet season with an annual average of 2.29 billion m³ (Chen and Qian, 2019; Li, et al., 2021). The lake outflow exhibits obvious seasonal variation. It increases from January to June, reaches the peak in June to August, and then decreases from August to December. In recent years, the influence of the watershed-controlled water conservancy projects on the river–lake interaction has become more obvious (Guo et al., 2011; Ou et al., 2011; Bing et al., 2017).
The daily observed data of the TGR discharge, water discharge at Gaobazhou station on the Qingjiang River, water discharge at Xiantao station on the Hanjiang River, water flow at Chenglingji station, and water discharge at Jiujiang station (Figure 1A) are used for the Yangtze River flood routing model to simulate the water discharge evolution from the TGR to Jiujiang station. The daily observed data of water level at Xingzi station, river discharge at Jiujiang station, and water flow at Hukou station (Figure 1B) are used for the water exchange simulating model. The daily inflows and outflows of the TGR in the typical years (2006, 2007, and 2010) are used to mimic the reservoir discharge in the dry, normal, and wet years, respectively.
River–lake water exchange is usually a two-way exchange, i.e., the lake water into the river and the river water into the lake. However, water exchange between the Yangtze River and Poyang Lake is mostly in the way of lake water into the river water. The reason is that Poyang Lake collects the runoffs from the upstream five tributaries in the lake basin, and then drains into the Yangtze River from the northern channel. In some cases, usually when the Yangtze River is flooding, the river water can flow into Poyang Lake through the river–lake junction. As Hukou station is located at the junction of the Yangtze River and Poyang Lake, the discharge of the station can be used as a representation of the river–lake water exchange. Often, positive values indicate that water flows from the lake to the river, and negative values indicate the opposite. A machine learning model is established to simulate the water exchange between the Yangtze River and Poyang Lake under different river and lake situations, with the water flow at Jiujiang station representing the Yangtze River situation and the water level at Xingzi station representing the Poyang Lake situation. To analyze the effects of the TGR operation, the Yangtze River mainstream rooting model is established to simulate the water flow of Jiujiang station affected by the TGR. Then, the abovementioned two models are integrated to simulate the water exchange between the Yangtze River and Poyang Lake affected by the TGR. The concept map is shown as Figure 2.
Machine learning is a branch of artificial intelligence and computer science which focuses on the use of data and algorithms to imitate the way humans learn, gradually improving its accuracy. Support Vector Machines (SVMs) are learning machines that implement the structural risk minimization inductive principle to obtain a good generalization using a limited number of learning patterns. The SVM model is established by the support vectors instead of all the samples, resulting in good performance at few-shot learning (Kambalimath and Deka, 2021; Liu et al., 2021). Support Vector Regression (SVR), proposed by Vapnik (1998), is the most common application form of SVMs. This method was developed to solve the nonlinear regression estimation problems (Gunn, 1998) and has been a widely used data-driven method for modeling environmental and water resource variables (Maier, et al., 2010; Hu et al., 2018). The basic theory of SVR and the river–lake water exchange can be found in our previous study (Zhang et al., 2020). Three parameters, the regularization parameter C, the error threshold ε, and the kernel parameter γ, are needed to be defined in the SVR model. To obtain an ideal combination of the three parameter values, a metaheuristic genetic algorithm (GA) is coupled into the SVR model for the water exchange model in this river–lake water exchange model.
Focused on water exchange at the river–lake junction, the model boundary is set as the water flow at Jiujiang station and water level at Xingzi station (Figure 3), as Jiujiang station is the nearest hydrological station to the river–lake junction on the Yangtze River and thus is regarded as the representative station to indicate the condition of the river reach near the lake, and Xingzi station is the nearest lake level gauging station to the junction and represents the lake condition. The output variable of the model is the Hukou station discharge, which represent the water exchange between the Yangtze River and Poyang Lake. The model input variables are the discharge at Jiujiang station discharge and the water level at Xingzi station. This approach is reasonable because the river–lake water exchange is directly determined by the hydrological conditions of the nearest river and lake area, and the discharge of the five tributaries impacts the water exchange through changing the water level of the lake. We use the observed daily data from 2010-2013 as the training data (with 1,461 daily data groups), and the daily data from 2014-2015 (with a sample size of 730) as the testing data. The statistical indicator for the model performance evaluation is the coefficient of determination R2, which is calculated as follows:.
where
FIGURE 3. River–lake water system (A), and Scope and input variables of the river–lake water exchange model (B).
The river–lake water exchange varies with the change of the conditions of the Yangtze River and Poyang Lake, and the hydrological conditions of the Yangtze River are mainly regulated by the upstream TGR on the upper Yangtze River, the world’s largest water resource project. The effects of the TGR are directly reflected in the river discharge variation, here, represented by the water flow of Jiujiang station. As the distance from the TGR to Jiujiang station is as long as 900 km, a mainstream rooting model based on the Muskingum flood rooting method is built to simulate the effect of the TGR on the water flow at Jiujiang station.
The Muskingum method was proposed by MaCarthy in 1938, first applied to the Muskingum River in the United States. This method uses the river channel storage equation to replace the complex hydrodynamic equation, simplifying the calculation process and achieving the practical calculation accuracy and thus is widely used in the world. The basic theory and process of the Muskingum method can be found in the related references (Chow, 1959). In the Yangtze River mainstream rooting model, as the river reach is very long, and there are three tributaries (the Qingjiang River, the Hanjiang River, and Dong Lake) considered in this reach. The mainstream in the study area is divided into several segments and the Muskingum method is used in every river segment. The first Muskingum parameter in each river segment Kl is equal to the calculated time interval △t. The number of river segments n, the length of river segments Ll, and the second Muskingum parameter of each river segment xl are calculated according to Eq. 2–Eq. 4. K and x are the two Muskingum parameters of the whole river reach, which are needed to be calibrated. The K parameter is equivalent to the travel time through the calculated river reach, and x is a weighting factor for volume distribution between the wedge and prism.
The routing equation of the ith river segment in the jth time interval is as follows:
where Iij is the inflow of the ith river segment at the beginning of the jth time interval, and is equal to the sum of Qi-1,j and the tributary discharge at the upstream node of the ith river segment. C0, C1, and C2 are the parameters calculated from △t, Kl, and xl, respectively.
For the initial condition, as the river section is divided into segments and there is no hydrological station at the nodes, we apply the linear interpolation method to calculate the initial inflow of each river segment. If one river segment has tributaries, the discharge from the tributaries will be added to the nearest node.
The operation principle of the TGR is to control flood in the wet season and to supply water in the dry season. From late May to early June, the water level of the TGR dropped to the 145 m flood limit level and the reservoir generally operates at this low water level in the flood season (June to September). In October (usually from late September), the reservoir gradually impounds water to 175 m, and the impoundment process may continue till November in dry years. Therefore, the TGR operation causes obvious declination of the river discharge in October. From December to next April, the reservoir water level is maintained as high as possible at 175 m. From January to April, the reservoir water level gradually decreases to release the flood control capacity, but the water level should be higher than 155 m before the end of April to ensure power generation and navigation. Its specific scheduling scheme is depended on the real-time reservoir inflow. Here, we analyze the effect of the TGR utilizing the reservoir operation schemes in three typical hydrological years as examples, i.e., the typical dry, normal, and wet years, and probe the alteration characteristic of the water exchange between the Yangtze River and Poyang Lake under different reservoir regulation schemes. The reservoir inflow is treated as the natural Yangtze River discharge (without TGR on the river) and the river–lake-lake water exchange under this scenario is compared with that under the reservoir operation to quantify the effect of the TGR. In this approach, the impact of the TGD is considered by comparing the river–lake water exchange under different reservoir discharges, which will directly change the downstream river discharge. This approach focuses on the change of the river condition and ignored the complex interaction between the lake level and river–lake water exchange. Therefore, the impact of the TGD on the river–lake water exchange in this study is basically represented by the change of river-effect caused by the TGD. To focus on the effect of the TGR, the discharges of the Yangtze River’s tributaries (measured at Gaobazhou station, Xiantao station, and Chenglingji station) remain the same as in the normal year, and only the reservoir discharge and Poyang Lake level change in different typical years.
Since the runoff sequence of hydrological stations after the TGR operation (from 2003) is not long enough to analyze the runoff frequency, the typical years after the TGR operation are defined according to the classification standard of the wet and dry runoff conditions from the Information Center of the Ministry of Water Resources of China. The runoff departure (%) is calculated as follows:
where Ri is the annual runoff of the ith year and
FIGURE 4. Operation schemes of the TGR in the typical dry year (A), the typical normal year (B), and the typical wet year (C).
The training and testing results of the river–lake water exchange model are shown in Figure 5. The final values of the three SVR parameters C, ε, and γ computed by GA are 5000, 31.4, and 100, respectively. As mentioned previously, negative values of the Hukou discharge imply that the river water flows into the lake. The model shows good performance in general and the simulated water exchange is in good agreement with the observed data. Though the model testing result is not as accurate as the training portion, it still achieves a satisfactory accuracy. The training and testing coefficients of determination R2 are 0.9562 and 0.8734, respectively. To summarize, the training and testing results indicate that the model is capable of simulating the variations in water exchange between Poyang Lake and the Yangtze River.
FIGURE 5. Training (A) and testing (B) results of the machine learning model simulating water exchange between the Yangtze River and Poyang Lake.
Based on the analysis of the historical discharge data of the TGR and Jiujiang station, it is found that the time of the flood rooting from the TGR to Jiujiang station is about 5–10 days. According to the physical meaning of the channel storage constant K, the value of K is preliminarily determined in the range of 120–240. As the corresponding flow data are all daily data, the calculation time interval △t = 24 h is assigned. The relationship between the discharge specific gravity coefficient x and the characteristic river length l can be written as 1/2-L/2L; thus, the value range of x is
The output variable of the Yangtze River mainstream rooting model, the water discharge at Jiujiang station, is computed and the input to the river–lake exchange model, and thus forms the integrated model simulating water exchange between the Yangtze River and Poyang Lake affected by the TGR. As the model coupling usually brings to the amplification of the simulating errors, the integrated model was also validated and the result is shown in Figure 7. Compared to the high accuracy of the water exchange model and the mainstream rooting model, the accuracy of the integrated model is reduced, with R2 equal to 0.6708, but the simulation result is satisfactory, and the variation trend of the observed river–lake water exchange is well traced.
FIGURE 7. Model performance of the integrated model simulating water exchange between the Yangtze River and Poyang Lake affected by the TGR.
Figure 8 shows the variations of water exchange between the Yangtze River and Poyang Lake in the typical dry, normal, and wet year. Water exchanges with and without the TGR operation are compared in the left panels, and the water exchange differential (water exchange with the TGR minus that without the TGR) is drawn in the right panels. As seen from the figures, the river–lake water exchange under the influence of the TGR operation is approximate to that without the TGR, under which scenario the reservoir discharge is equal to the reservoir inflow, in most time of the year. The TGR operation caused obvious alteration of the river–lake water exchange in October in all the typical years, with a dramatic decline in the dry year and a significant increase in both the normal and wet years.
FIGURE 8. Variation of water exchange between the Yangtze River and Poyang Lake affected by the TGR in the dry year (A), normal year (B), and wet year (C).
For the typical dry year, the river–lake water exchange increases with fluctuations from January to June, and dramatically decreases in July. The effect of the TGR on the river–lake water exchange is mainly shown in late May to early June and in October. For the typical normal year, the river–lake water exchange increases from January to March, fluctuates drastically to early July, and then drops precipitously to negative values, meaning that water flows reversely (from the river to the lake). The effect of the TGR is not obvious during this time period, with the water exchange differential less than 1,000 m³/s. Unlike in the dry year, the TGR operation in the normal year causes a big increase of water exchange, indicating that more water flows from Poyang Lake to the Yangtze River in October in the normal year. For the typical wet year, the river–lake water exchange fluctuates with an increase from January to late July, and also has a decline that is moderate compared to that in the other years. The effect of the TGR on water exchange is significant from June to October, increasing the water exchange in July, September, and October and decreasing it in June and August. The backward flow in late September under the scenario without the TGR is even eliminated by the TGR operation. The increase of river–lake water exchange generated by the TGR impoundment in October leads to a reduction of the lake water storage, and thus would lower the lake level and might bring forward the dry period of the river-connected lakes. However, in the very dry year (e.g. 2006 in this study) the reservoir impoundment might decrease the water exchange, with the change in lake level ignored. Moreover, the reservoir operation in the flood season of the wet year is beneficial for flood prevention of the Poyang Lake basin.
To analyze the relationship between the river–lake water exchange variation and the TGR regulation, we compared the water flow differential through the TGR,
where
FIGURE 9. Comparison of the water flow differential through the TGR (reservoir outflow minus reservoir inflow) and of the water exchange differential with and without the TGR in the dry year (A), normal year (B), and wet year (C).
As can be seen from Figure 9, the waves of the river–lake exchange differential appear several days later than the significant change of the reservoir water flow differential, which means that the effect of the TGR on the water exchange has a delay of several days. It is noticeable that the water flow differential through the TGR is negative (
To explore the underlying reasons of why the river–lake water exchange changes in a different way in the dry year compared to the normal and wet year, we examined the data characteristics of the input variables and intermediate results of the water exchange model. Figure 10 shows that the reservoir outflow with the TGR (B), reservoir flow differential with and without the TGR schemes (C), and the differential of simulated water flow at Jiujiang station with and without the TGR schemes (D) in October of the three typical years are similar, but the lake level (A) in the typical dry year is dramatically lower than the other 2 years. The river discharge at Jiujiang station is also declined in the dry year as the upstream reservoir discharge decreased in October, which is the same as the normal and wet year. Therefore, the very low lake level is most likely the cause of water exchange decreasing under the impoundment of the TGR in October. It is possible that when the water level of Poyang Lake is very low, the decline of the Yangtze River discharge will cause the decrease of the lake outflow, because Poyang Lake is more like a river at this condition and its discharge might have a positive relationship with the Yangtze River discharge.
FIGURE 10. Comparison of the input variables, lake level (A), reservoir outflow (B), reservoir flow differential (C), and the intermediate results, differential of simulated water flow at Jiujiang station between with and without the TGR schemes (D), of the water exchange model in different typical years.
To examine whether the low lake level plays a decisive role on the special results in October of the dry year, we increased the lake level gradually while the other model conditions remained and computed the river–lake water exchange differential under different lake levels in October of the typical dry year (Figure 11). The results show that the river–lake water exchange differential with and without the TGR increases as the lake level rises. When the lake level is increased by 2 m, values of the water exchange differential turn positive, which means that water exchange increases under the impoundment of the TGR (similar to the results in the typical normal and wet years). When the lake level is increased by 3–5 m, the water exchange keeps positive, implying that the impoundment of the TGR also causes the water exchange to increase when the lake level is not so low.
FIGURE 11. Water exchange differential with the lake level increasing gradually in October of the typical dry year.
However, lake level is not the only factor that modifies the impact of the TGR operation on the river–lake -lake water exchange (represented by the water exchange differential). Other factors such as the amount of the reservoir discharge, the water flow differential caused by the TGR, and the interaction between water exchange and lake level also lead to the TGR affecting the water exchange in different ways and different intensities. The model errors in this study might also be a potential factor. The influence mechanism and extent of these factors need to be further studied in the future.
To investigate the impact of the TGR operation on water exchange between the Yangtze River and Poyang Lake, this study developed a machine learning model to simulate the river–lake water exchange variations affected by the TGR and to analyze the variation laws of the river–lake water exchange under different operational schemes in three typical years. It is demonstrated that the TGR has significant effects on the water exchange between the Yangtze River and Poyang Lake, especially in the impounding period. The effect has a delay of several days and varies with different hydrological conditions of the river and the lake. Reservoir impoundment causes a decrease of the river–lake water exchange in the dry year (with the maximum decrease of 4,000 m³/s in this study case), but causes an increase in the normal and wet year (with the maximum increase of 4,000 m³/s and 6,000 m³/s in this study case, respectively). And the influence of the TGR in the wet year is larger than that in the other years, and the impact period lasts from May to October. The variation results of the water exchange indicate that the TGR operation is beneficial to the water maintenance of the lake in the dry year and the flood control in the lake area in the wet year. This study provides valuable reference for water resource management and reservoir operation in the Yangtze–Poyang water system and other large river–lake systems.
The original contributions presented in the study are included in the article/Supplementary Material. Further inquiries can be directed to the corresponding author.
Conceptualization, methodology, review, and editing: PZ JM; data and resources, investigation, and validation: LD TH; original draft preparation and visualization: PZ and MT; and supervision: JM. All authors have read and agreed to the submitted version of the manuscript.
The research was supported by the National Natural Science Foundation of China (U2040205, 52109013, and 51909168), China Postdoctoral Science Foundation (2021M701049), and Science and technology program of Chongqing Transportation Bureau (2020–07).
Author LD is employed by China Three Gorges Corporation.
The remaining authors declare that the research was conducted in the absence of any commercial or financial relationships that could be construed as a potential conflict of interest.
All claims expressed in this article are solely those of the authors and do not necessarily represent those of their affiliated organizations, or those of the publisher, the editors, and the reviewers. Any product that may be evaluated in this article, or claim that may be made by its manufacturer, is not guaranteed or endorsed by the publisher.
Bing, J. P., Deng, P. X., Lv, S. Y., and Zhang, X. (2017). The Analysis of Water Exchange Regime Research on Poyang Lake and Yangtze River and Driving Factors. Sci. Sin. Technol. 47 (8), 856–870.
Chen, J., and Qian, X. (2019). Variation Characteristics of Water Recharge from Changjiang River into Poyang Lake. J. Yangtze River Sci. Res. Inst. 36 (5), 18–22+27. (In Chinese).
Chen, J., and Xu, J. J. (2013). Study on the Impact of the Three Gorges Reservoir on Poyang Lake and Some Proposals. J. Hydraul. Eng. 44 (7), 757–763.
Chen, J., Wang, P., Wang, C., Wang, X., Gao, H., Cui, G., et al. (2022). How Dam Construction Affects the Activity of Alkaline Phosphatases in Reservoir Sediments: A Study of Two Highly Regulated Rivers. Environ. Res. 207, 112236. doi:10.1016/j.envres.2021.112236
Cui, L. (2004). A Study on the Value Assessment of Poyang Lake Wetland Ecosystem Services. Chin. J. Ecol. 4, 47–51. (In Chinese).
Dai, Z., Liu, J. T., Yu, Z., Yang, G., and Xu, C. Y. (2013). Impacts of Large Dams on Downstream Fluvial Sedimentation: An Example of the Three Gorges Dam (TGD) on the Changjiang (Yangtze River). J. Hydrology 48035 (45), 10–18. doi:10.1016/j.jhydrol.2012.12.003
Dai, X., Yu, Z., Yang, G., and Xu, C.-Y. (2021). Investigation of Inner-Basin Variation: Impact of Large Reservoirs on Water Regimes of Downstream Water Bodies
Destouni, G., Jaramillo, F., and Prieto, C. (2012). Hydroclimatic Shifts Driven by Human Water Use for Food and Energy Production. Nat. Clim. Change 3 (3), 213–217. doi:10.1038/nclimate1719
Fan, S., Deng, J., Wang, X., and Zhang, R. (2019). Impact of the Three Gorges Reservoir on the Storage Capacity of Poyang Lake. Adv. water Sci. 30 (6), 537–545.
Fang, C. M., Cao, W. H., Mao, J. X., and Li, H. J. (2012). Relationship between Poyang Lake and Yangtze River and Influence of Three Georges Reservoir. J. Hydraul. Eng. 43 (2), 175–181.
Ferrazzi, M., and Botter, G. (2019). Contrasting Signatures of Distinct Human Water Uses in Regulated Flow Regimes. Environ. Res. Commun. 1, 071003. doi:10.1088/2515-7620/ab3324
Gao, B., Yang, D., and Yang, H. (2013). Impact of the Three Gorges Dam on Flow Regime in the Middle and Lower Yangtze River. Quat. Int. 304, 43–50. doi:10.1016/j.quaint.2012.11.023
Gunn, S. R. (1998). Support Vector Machines for Classification and Regression. UK: Technical ReportUniversity of Southampton.
Guo, H., Qi, H., and Zhang, Q. (2011). Changes in Hydrological Interactions of the Yangtze River and the Poyang Lake in China during 1957-2008. Acta Geogr. Sin. 66 (5), 609–618.
Guo, H., Hu, Q., Zhang, Q., and Feng, S. (2012). Effects of the Three Gorges Dam on Yangtze River Flow and River Interaction with Poyang Lake, China: 2003-2008. J. Hydrology 416-417, 19–27. doi:10.1016/j.jhydrol.2011.11.027
Guo, Z. T., Huang, F., Guo, L. D., and Wu, Y. (2021). Study on the Causes and Countermeasures of Hydrological Regime Evolution in Poyang Lake. J. Yangtze River Sci. Res. Inst. 38 (6), 27–31.
Han, J., Wang, Y., and Sun, Z. (2021). Changes of Water Stage in the Middle Yangtze River Influenced by Human Activities in the Past 70 Years. Front. Earth Sci. 15 (1), 121–132. doi:10.1007/s11707-020-0855-8
Hecht, J. S., Lacombe, G., Arias, M. E., Dang, T. D., and Piman, T. (2019). Hydropower Dams of the Mekong River Basin: A Review of Their Hydrological Impacts. J. Hydrology 568, 285–300. doi:10.1016/j.jhydrol.2018.10.045
Hou, X., Feng, L., Chen, X., and Zhang, Y. (2018). Dynamics of the Wetland Vegetation in Large Lakes of the Yangtze Plain in Response to Both Fertilizer Consumption and Climatic Changes. ISPRS J. Photogrammetry Remote Sens. 141, 148–160. doi:10.1016/j.isprsjprs.2018.04.015
Hu, Y.-x., Huang, J.-l., Du, Y., Han, P.-p., Wang, J.-l., and Huang, W. (2015). Monitoring Wetland Vegetation Pattern Response to Water-Level Change Resulting from the Three Gorges Project in the Two Largest Freshwater Lakes of China. Ecol. Eng. 74, 274–285. doi:10.1016/j.ecoleng.2014.10.002
Hu, T., Mao, J., Pan, S., Dai, L., Zhang, P., Xu, D., et al. (2018). Water Level Management of Lakes Connected to Regulated Rivers: an Integrated Modeling and Analytical Methodology. J. Hydrology 562, 796–808. doi:10.1016/j.jhydrol.2018.05.038
Huang, Y., Lv, S. Y., Guo, H. J., and Xu, G. H. (2014). Analysis of Water Balance in Poyang Lake Area. J. Water Resour. Res. 3, 458–464.
Kambalimath S, S., and Deka, P. C. (2021). Performance Enhancement of SVM Model Using Discrete Wavelet Transform for Daily Streamflow Forecasting. Environ. Earth Sci. 80, 101. doi:10.1007/s12665-021-09394-z
Lai, X., Jiang, J., and Huang, Q. (2012). Water Storage Effects of Three Gorges Project on Water Regime of Poyang Lake. J. Hydroelectr. Eng. 31 (6), 132–136+148.
Lai, X., Jiang, J., Yang, G., and Lu, X. X. (2014a). Should the Three Gorges Dam Be Blamed for the Extremely Low Water Levels in the Middle-Lower Yangtze River? Hydrol. Process. 28 (1), 150–160. doi:10.1002/hyp.10077
Lai, X., Liang, Q., Jiang, J., and Huang, Q. (2014b). Impoundment Effects of the Three-Gorges-Dam on Flow Regimes in Two China's Largest Freshwater Lakes. Water Resour. Manage 28 (14), 5111–5124. doi:10.1007/s11269-014-0797-6
Li, X., Yao, J., Li, Y., Zhang, Q., and Xu, C.-Y. (2016). A Modeling Study of the Influences of Yangtze River and Local Catchment on the Development of Floods in Poyang Lake, China. Hydrol. Res. 47 (S1), 102–119. doi:10.2166/nh.2016.198
Li, Y., Zhang, Q., Liu, X., Tan, Z., and Yao, J. (2019). The Role of a Seasonal Lake Groups in the Complex Poyang Lake-floodplain System (China): Insights into Hydrological Behaviors. J. Hydrology 578, 124055. doi:10.1016/j.jhydrol.2019.124055
Li, P., Li, H., Chen, F., and Cai, X. (2020). Monitoring Long-Term Lake Level Variations in Middle and Lower Yangtze Basin over 2002-2017 through Integration of Multiple Satellite Altimetry Datasets. Remote Sens. 12 (9), 1448. doi:10.3390/rs12091448
Li, Q., Lai, G., and Devlin, A. T. (2021). A Review on the Driving Forces of Water Decline and its Impacts on the Environment in Poyang Lake, China. J. Water Clim. Change. 12 (5), 1370–1391. doi:10.2166/wcc.2020.216
Liu, C., and Liu, X. (2012). Research on Poyang Lake Wetland Information Extraction and Change Monitoring Based on Spatial Data Mining. Phys. Procedia 33, 1412–1419. doi:10.1016/j.phpro.2012.05.231
Liu, X., Lu, M., Chai, Y., Tang, J., and Gao, J. (2021). A Comprehensive Framework for HSPF Hydrological Parameter Sensitivity, Optimization and Uncertainty Evaluation Based on SVM Surrogate Model-A Case Study in Qinglong River Watershed, China. Environ. Modell. Softw. 143, 105126.doi:10.1016/j.envsoft.2021.105126
Liu, W. B. (2007). Study on the Impacts of the Three Gorges Reservoir Running in Initial Stages on the Trend of High Water Level in the Poyang Lake. Nanchang: Nanchang University. [master’s thesis]. [Nanchang].
Maier, H. R., Jain, A., Dandy, G. C., and Sudheer, K. P. (2010). Methods Used for the Development of Neural Networks for the Prediction of Water Resource Variables in River Systems: Current Status and Future Directions. Environ. Model. Softw. 25, 891–909. doi:10.1016/j.envsoft.2010.02.003
Mei, X., Dai, Z., Gelder, P. H. A. J. M., and Gao, J. (2015). Linking Three Gorges Dam and Downstream Hydrological Regimes along the Yangtze River, China. Earth Space Sci. 2 (4), 94–106. doi:10.1002/2014ea000052
Nilsson, C., Reidy, C. A., Dynesius, M., and Revenga, C. (2005). Fragmentation and Flow Regulation of the World's Large River Systems. Science 308, 405–408. doi:10.1126/science.1107887
Ou, M. L., Zhou, W. B., and Hu, C. H. (2011). Variation Characteristics of Water Environmental Capacity in Poyang Lake under the Scheduling of Three Gorges Reservoir. Meteorological Environ. Res. 3, 46–48+52.
Ouyang, Q. L., and Liu, W. L. (2014). Variation Characteristics of Water Level in Poyang Lake over 50 Years. Resour. Environ. Yangtze Basin 11, 1545–1550.
Poff, N. L., Olden, J. D., Merritt, D. M., and Pepin, D. M. (2007). Homogenization of Regional River Dynamics by Dams and Global Biodiversity Implications. Proc. Natl. Acad. Sci. U.S.A. 104 (14), 5732–5737. doi:10.1073/pnas.0609812104
Ren, S., Zhang, B., Wang, W.-J., Yuan, Y., and Guo, C. (2021). Sedimentation and its Response to Management Strategies of the Three Gorges Reservoir, Yangtze River, China. Catena 199, 105096. doi:10.1016/j.catena.2020.105096
Shankman, D., Keim, B. D., and Song, J. (2006). Flood Frequency in China's Poyang Lake Region: Trends and Teleconnections. Int. J. Climatol. 26 (9), 1255–1266. doi:10.1002/joc.1307
Su, Z., Ho, M., Hao, Z., Lall, U., Sun, X., Chen, X., et al. (2020). The Impact of the Three Gorges Dam on Summer Streamflow in the Yangtze River Basin. Hydrol. Process. 34 (3), 705–717. doi:10.1002/hyp.13619
Tang, H. W., Huang, S. J., Yuan, S. Y., Li, K., Xiao, Y., and Xiao, Z. K. (2020). Three-dimensional Flow Structure at the Confluence Zone of Yangtze River and Poyang Lake. J. Hohai Univ. Nat. Sci. 48 (2), 128–135.
Taylor, M., Chen, Q. W., Meter, V. K., Brown, E. L., Zhang, J. Y., Ni, J. R., et al. (2020). River Dam Impacts on Biogeochemical Cycling. Nat. Rev. 1, 103–116.
Timpe, K., and Kaplan, D. (2017). The Changing Hydrology of a Dammed Amazon. Sci. Adv. 3, e1700611. doi:10.1126/sciadv.1700611
Wang, J., Sheng, Y., and Wada, Y. (2017). Little Impact of the T Hree G Orges D Am on Recent Decadal Lake Decline across C Hina's Y Angtze P Lain. Water Resour. Res. 53 (5), 3854–3877. doi:10.1002/2016wr019817
Winemiller, K. O., McIntyre, P. B., Castello, L., Fluet-Chouinard, E., Giarrizzo, T., Nam, S., et al. (2016). Balancing Hydropower and Biodiversity in the Amazon, Congo, and Mekong. Science 351, 128–129. doi:10.1126/science.aac7082
Xu, Y., Li, J., Wang, J., Chen, J., Liu, Y., Ni, S., et al. (2020). Assessing Water Storage Changes of Lake Poyang from Multi-Mission Satellite Data and Hydrological Models. J. Hydrology 590, 125229. doi:10.1016/j.jhydrol.2020.125229
Yang, G., Zhang, Q., Wan, R., Lai, X., Jiang, X., Li, L., et al. (2016). Lake Hydrology, Water Quality and Ecology Impacts of Altered River-Lake Interactions: Advances in Research on the Middle Yangtze River. Hydrology Res. 47 (S), 1–7. doi:10.2166/nh.2016.003
Yang, X., Zhang, M., He, X., Ren, L., Pan, M., Yu, X., et al. (2020). Contrasting Influences of Human Activities on Hydrological Drought Regimes over China Based on High-Resolution Simulations. Water Resour. Res. 56 (6), 1–17. doi:10.1029/2019wr025843
Zhang, Q., Li, L., Wang, Y. G., Werner, A. D., Xin, P. T., and Jiang, D. A. (2012). Has the Three Gorges Dam Made the Poyang Lake Wetlands Wetter and Drier? Geophys. Res. Lett. 39 (20), L20402. doi:10.1029/2012gl053431
Zhang, J., Huang, T., Chen, L., Liu, X., Zhu, L., Feng, L., et al. (2019). Water-Exchange Response of Downstream River-Lake System to the Flow Regulation of the Three Gorges Reservoir, China. Water 11 (11), 2394. doi:10.3390/w11112394
Zhang, P., Mao, J., Hu, T., Dai, L., Xu, D., and Dai, H. (2020). Water Exchange in a Large River-Lake System: Modeling, Characteristics and Causes. River Res. Applic 36 (5), 697–708. doi:10.1002/rra.3569
Zhao, T., Zhao, J., Liu, P., and Lei, X. (2015). Evaluating the Marginal Utility Principle for Long-Term Hydropower Scheduling. Energy Convers. Manag. 106, 213–223. doi:10.1016/j.enconman.2015.09.032
Keywords: Three Gorges Reservoir, operation scheme, Poyang Lake, machine learning model, water exchange
Citation: Zhang P, Mao J, Tian M, Dai L and Hu T (2022) The Impact of the Three Gorges Reservoir on Water Exchange Between the Yangtze River and Poyang Lake. Front. Earth Sci. 10:876286. doi: 10.3389/feart.2022.876286
Received: 15 February 2022; Accepted: 20 April 2022;
Published: 24 May 2022.
Edited by:
Xijun Lai, Nanjing Institute of Geography and Limnology (CAS), ChinaReviewed by:
Binquan Li, Hohai University, ChinaCopyright © 2022 Zhang, Mao, Tian, Dai and Hu. This is an open-access article distributed under the terms of the Creative Commons Attribution License (CC BY). The use, distribution or reproduction in other forums is permitted, provided the original author(s) and the copyright owner(s) are credited and that the original publication in this journal is cited, in accordance with accepted academic practice. No use, distribution or reproduction is permitted which does not comply with these terms.
*Correspondence: Jingqiao Mao, bWFvanFAaGh1LmVkdS5jbg==
Disclaimer: All claims expressed in this article are solely those of the authors and do not necessarily represent those of their affiliated organizations, or those of the publisher, the editors and the reviewers. Any product that may be evaluated in this article or claim that may be made by its manufacturer is not guaranteed or endorsed by the publisher.
Research integrity at Frontiers
Learn more about the work of our research integrity team to safeguard the quality of each article we publish.