- 1Ecological Engineering Laboratory (ECOL), Institute of Environmental Engineering (IIE), School of Architecture, Civil and Environmental Engineering (ENAC), École Polytechnique Fédérale de Lausanne (EPFL), Lausanne, Switzerland
- 2Ecohydrology Laboratory (ECHO), Institute of Environmental Engineering (IIE), School of Architecture, Civil and Environmental Engineering (ENAC), École Polytechnique Fédérale de Lausanne (EPFL), Lausanne, Switzerland
- 3Dipartimento di Ingegneria Civile Edile e Ambientale, Universitá di Padova, Padua, Italy
- 4School of Architecture, Building and Civil Engineering, Loughborough University, Loughborough, United Kingdom
- 5Hydraulic Platform-LCH, Civil Engineering Institute (IIC), School of Architecture, Civil and Environmental Engineering (ENAC), École Polytechnique Fédérale de Lausanne (EPFL), Lausanne, Switzerland
- 6School of Engineering, Institute for Infrastructure and Environment, The University of Edinburgh, Edinburgh, United Kingdom
- 7Department of Aquatic Ecosystem Analysis and Management, Helmholtz Centre for Environmental Research—UFZ, Magdeburg, Germany
Despite numerous applications of physically-based models for incised landscapes, their applicability for overland flow on unchanneled surfaces is not known. This work challenges a widely used landscape evolution model for the case of non-uniform rainfall and absence of rills using laboratory flume experiment. Rainfall with an average intensity of 85 mm h−1 was applied for 16 h during which high resolution laser scans of the morphology were captured. The overland flow was modeled as a network that preserves the water flux for each cell in the discretized domain. This network represented the gravity-driven surface flow and determined the evolution direction. The model was calibrated using the first 8 h of the experiment and was then used to predict the second 8 h. The calibrated model predicted, as expected, a smoother surface morphology (and less detailed overland flow network) than that measured. This difference resulted from quenched randomness (e.g., small pebbles) within the experimental soil that emerged during erosion and that were captured by the laser scans. To investigate the quality of the prediction, a low-pass filter was applied to remove the small-scale variability of the surface morphology. This step confirmed that the model simulations captured the main characteristics of the measured morphology. The experimental results were found to satisfy a scaling relation for the exceedance probability of discharge even in absence of rills. However, the model did not reproduce the experimental scaling relation as the detailed surface micro-roughness was not accounted for by the model. A lower cutoff on the scale of applicability of the general landscape evolution equation is thus suggested, complementing other work on the upper cutoff underpinned by runoff-producing areas.
Introduction
The complexity of natural landscapes reflects the numerous factors involved in their formation such as climate (Francipane et al., 2015; Han et al., 2015; Hooshyar et al., 2019; van der Meij et al., 2020), chemical and physical properties of the sediments (Massong and Montgomery, 2000; Sklar and Dietrich, 2001; Park and Latrubesse, 2015), episodic gully erosion (Pazzaglia et al., 2015), different vegetation types (Istanbulluoglu and Bras, 2005; Jeffery et al., 2014; Corenblit et al., 2015), tectonic effects (Pedrazzini et al., 2016) and the rate of sediment production (Reusser et al., 2015; Rodriguez-Lloveras et al., 2015; Forte et al., 2016). Landscape formation and evolution are not directly measurable in the field due to the time scales involved. Consequently, suitably designed laboratory experiments are of considerable value in that they provide empirical data that are amenable to testing hypotheses of mechanisms underlying observed geomorphological changes (Paola et al., 2009).
Physically-based landscape evolution models (LEMs) (Willgoose, 1989; Willgoose et al., 1991, 1992; Howard, 1994; Whipple and Tucker, 1999, 2002) are useful tools to explain the surface geometry in landscapes and laboratory experiments (Mudd, 2016; Whipple et al., 2016, 2017; Sinclair, 2017). In LEMs, the complex fluid-particle interactions within landscapes are described by a governing equation for the surface elevation, with an additional model for surface flow (Chen et al., 2014). For catchments, the focus of surface flow is the stream/river drainage network, rather than the overland flow. Typically, flow is modeled in a simplified manner that conserves the volume flux at each cell in the landscape (O’Callaghan and Mark, 1984; Freeman, 1991; Quinn et al., 1991; Costa-Cabral and Burges, 1994; Tarboton, 1997). Broadly speaking, the relative importance of the advective and diffusive processes described by the LEM controls the landscape geometry produced. That is, considering an initially non-incised morphology, localized (channel-forming) landscape incision is favored when advection (or surface shear stress) dominates, whereas more gradually varying landscapes will result when (effective) diffusion dominates.
There are numerous applications of LEMs to understand different features of the surface morphology of natural landscapes (Yang et al., 2015; Mudd, 2016; Whipple et al., 2016, 2017; Sinclair, 2017; Bonetti et al., 2020; Hooshyar and Porporato, 2021; Hu et al., 2021; Kwang et al., 2021; Shelef and Goren, 2021; Litwin et al., 2022). For example, Perron et al. (2008) derived an expression for the distance between first-order valleys and validated the formula via measurements from five different natural landscapes (Perron et al., 2009). Willett et al. (2014) showed that in disequilibrium conditions, the drainage divides between basins and migrates towards a steady-state condition and thereby reorganize their structure. The criteria defining the reorganization direction were found by using the steady-state solution of an LEM (Perron and Royden, 2013). It is noteworthy that the LEM equation in its original formulation (where the local landscape-forming fluxes are surrogated by total contributing area thus postulating uniform precipitation) corresponds to the leading term of the small-gradient approximation of the general mass-balance equation under general reprametrization invariance (Banavar et al., 2001). This has consequences, because at steady state optimal channel networks (OCNs) are exact constructions and each local, dynamically accessible minimum proves to be a tree (Rodriguez-Iturbe and Rinaldo, 2001; Rinaldo et al., 2014).
Similar to their application to natural landscapes, LEMs were also used in the analyses of laboratory experiments. Such experiments permit exploration of different initial conditions, e.g., a land surface composed of uniform and non-cohesive sediment grains, with surface morphology changes induced by rainfall. Advection-dominated setups focus on the evolution of the network structure (i.e., surface incisions) (Hancock and Willgoose, 2002; Bonnet and Crave, 2003; Hasbargen and Paola, 2003; Lague et al., 2003; Bonnet and Crave, 2006; Bonnet, 2009; Paola et al., 2009; Graveleau et al., 2012; Rohais et al., 2012; Reinhardt and Ellis, 2015; Singh et al., 2015; Cheraghi et al., 2018). Small raindrop sizes minimize the kinetic energy of raindrop impact, leading to surface morphologies that evolve almost exclusively through shear stress of surface flows. For instance, Hasbargen and Paola (2000) set up an experiment in an elliptical plot (98 cm × 87 cm) subjected to a constant uplift rate. They pointed out that the oscillation of the erosion rate at steady-state was the result of knickpoint migration in the domain. The spatial patterns of landslides and knickpoints in a steady-state landscape were measured by Bigi et al. (2006), who found a power-law relation between the number of landslides and drainage area. The power-law relation was consistent with the gamma-distribution probability density function of landslides in the field (Malamud et al., 2004). Sweeney et al. (2015) analyzed landscapes subjected to rainfall events with different droplet sizes considering the same initial condition. For the different landscape patterns so-created, they showed that the drainage density decreases with increasing droplet size (i.e., relative increase in diffusion).
There are numerous similar studies that examined rill formation on hillslopes (Parker, 1977; Bryan and Poesen, 1989; Gomez and Mullen, 1992; Brunton and Bryan, 2000; Römkens et al., 2002; Pelletier, 2003; Raff et al., 2004; Rieke-Zapp and Nearing, 2005; Tatard et al., 2008; Yao et al., 2008; Oliveto et al., 2010; Gordon et al., 2011, 2012; Stefanon et al., 2012; Shit et al., 2013; He et al., 2014; Bennett et al., 2015; Bennett and Liu, 2016; Wu and Chen, 2020; Ren et al., 2021). In these experiments, droplet sizes are large enough to induce splash-impact erosion, which is manifested in changes in surface morphology. For instance, Gómez et al. (2003) and Berger et al. (2010) tested the minimal energy expenditure theory (Rinaldo et al., 1992) for rill networks considering different slopes, rainfall intensities and initial conditions. They found that, when the land was effectively incised, the total energy dissipation decreased as the rills evolved. McGuire et al. (2013) calibrated experimental data with the shallow water flow equations coupled with a process-based erosion model (Hairsine and Rose, 1991, 1992a,b; Simpson and Schlunegger, 2003; Simpson and Castelltort, 2006). They found that the entrainment term differences between the models have a greater effect on morphology predictions of the models than the diffusive term.
In this work, we report a laboratory experiment with two significant differences to those described above. First, by design rainfall was highly non-uniform in space; and second, the surface morphology did not become incised at all in the experiment (i.e., rills did not form), but still exhibiting self-organized aggregation. Cheraghi et al. (2018) showed that the morphology measured in the laboratory flume exhibits the same scale-invariant power laws during its evolution as found for catchments. In other words, the statistical features of catchment channel networks were found in the unchanneled area when the morphology was captured and analyzed at high resolution. That finding motivated this investigation based on a widely-used catchment-scale LEM (Howard, 1994; Perron et al., 2008) as a tool to predict the evolution of its unchanneled morphology, i.e., for the situation where diffusive processes dominate such that channels do not form. Previous applications of this LEM considered an incised landscape in which discharge occurs either as overland flow (to channels/rivers), or as flow within the channels. In our experiment, only overland flow occurs, and the surface is never incised. Nevertheless, we model the overland flow as a discharge network, as done in other applications of the LEM. Additionally, we test the ability of the model to simulate directly the measured surface morphology, a test that is not possible at the landscape scale, and was hitherto not attempted at the laboratory experiment scale.
Materials and methods
Experiment
The same experimental data set was used previously (Cheraghi et al., 2018), and only essential details are presented here. We applied a nonuniform rainfall with an average intensity of 85 mm h−1 on a 2-m × 1-m erosion flume with 5% slope (Figure 1). There was no infiltration at the flume’s bottom. Sandy sediment was added to the flume to a depth of 15 cm (Table 1). Digital Elevation Models (DEMs) at 0.25, 0.5, 1, 2, 4, 8 and 16 h were captured by a 3D laser scanner with about 4-mm resolution. The rainfall stopped while the morphology was measured and then restarted.
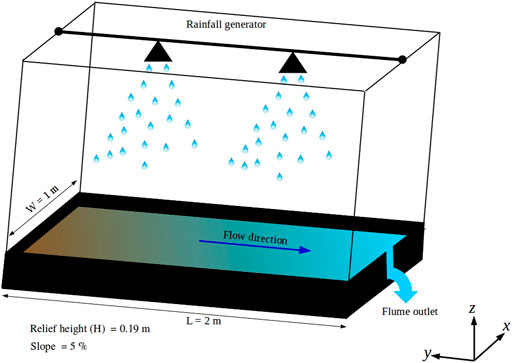
FIGURE 1. Schematic of the flume experiment. The sediment elevation was measured from the outlet (z = 0) and with respect to the horizon. The relief height (the maximum elevation) was 0.19 m. Details on the experimental setup are given in the Supplementary materials of Cheraghi et al. (2018).
Landscape evolution model
We critically assess the applicability of Fokker–Planck form of landscape evolution model at a scale which is less than a computational cell of catchments. Due to large spatial scales, the model is derived based on simplification of turbulent flows and erosion mechanisms. In this work, the idea is to consider the large-scale model at the plot scale and assess how well the simple LEM can represent the complex fluid-particle interactions during heterogeneous rainfall and in absence of incision. The LEM used here is a modification of Howard’s model (Howard, 1994) and is derived similarly to the approach of Perron et al. (2008) with two differences: 1) it is based on the critical stream power rather than critical shear stress, and 2) instead of the drainage area at large scales, the discharge (Q) is used in the nonlinear part of the advection term. In absence of tectonic effects the model is:
where Cartesian coordinates are used. In this equation, z(x, y, t), t, S, are elevation from the horizontal, time and slope, respectively, and f(ζ) = ζ H(ζ) where H(ζ) is the Heaviside step function. The parameter Ωcr is proportional to the critical stream power by which fluvial sediment transport is initiated. The two parameters D and K are functions of rainfall intensity, droplet size, sediment properties (e.g., density, particle size, cohesion) and surface roughness (Furbish et al., 2007; Dunne et al., 2010; Mahmoodabadi and Sajjadi, 2016; Sadeghi et al., 2017). The exponent m takes values in the range 0.41–0.857 (Willgoose, 1989; Rodriguez-Iturbe and Rinaldo, 2001). We took m = 1/2 as this value was also used in studies on optimal channel networks based on the theory of minimum energy expenditure for river network evolution (Rinaldo et al., 1992, 1993). At catchment scales, the first term on the right side of Eq. 1 is dominant for hillslopes and includes different processes such as weathering (Perron, 2017), soil creep and rain splash (Culling, 1960, 1963, 1965). The second term on the right is usually assumed to model sediment transport within the river network (since elsewhere this term vanishes). However, in our flume-scale experiment, there were no surface incisions, and the overland flow is at all times continuous across the entire flume. Thus, the overland flow in the experiment is modeled as a network, although there is no river network as found in previous applications of the LEM (Tucker and Hancock, 2010; Benaïchouche et al., 2016; Whipple et al., 2016; Hancock et al., 2017; Perron, 2017).
Numerical simulation and calibration
During the initial stage of the experiment, the surface morphology at the flume exit changed rapidly due to the location of the flume drain. Such behavior is not captured by the model. Therefore, the scanned morphology at t = 15 min was used as the initial condition for the numerical simulations. In applying the LEM, at each time step, the pit points were removed using the algorithm of Planchon and Darboux (2002). After determining the flow direction via the d8 algorithm (O’Callaghan and Mark, 1984), the discharge at the ith cell, Qi, was calculated using:
where the summation over j refers to the eight cells surrounding the ith cell. The value of the element of the connectivity matrix, wji (−) is unit if the cell j flows into cell i, and zero otherwise. Ri (mm h−1) is rainfall intensity on the cell i (Figure 2) and Δx (mm) and Δy (mm) are the grid sizes in x and y directions, respectively. Numerical results presented here used cell sizes of 8 mm × 8 mm.
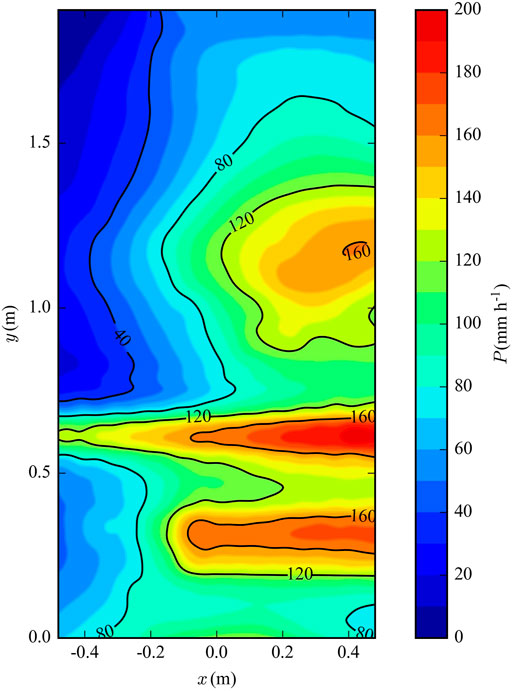
FIGURE 2. Rainfall distribution on the flume. The average precipitation and the uniformity coefficient were 85 mm h−1 and 26%, respectively.
The numerical solution was obtained by two fractional time steps (Press et al., 2007). The first step used the second-order Runge-Kutta scheme to solve the advection term (Eq. 1), while the second used the Alternating-Direction Implicit (ADI) method for the diffusion part. This approach was used previously by Perron et al. (2008), who explain it in detail. At the side wall, a conditional boundary condition was used as shown in Figure 3. At each time step, after the matrix operations (Runge-Kutta + ADI), the gradient normal to the boundary (
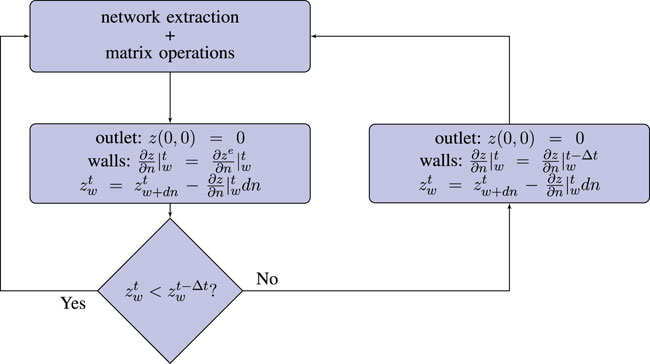
FIGURE 3. The wall boundary condition assuming
An evolutionary algorithm, Borg MOEA (Hadka and Reed, 2013), was used to find the optimal parameters. The initial condition of the numerical modeling was the experimental data at t = 0.25 h (results in Figure 4). The model was calibrated using the root-mean-square error of the morphology (z) at t = 8:
where zi,model and zi, exp are the modeled and measured elevations for cell i.
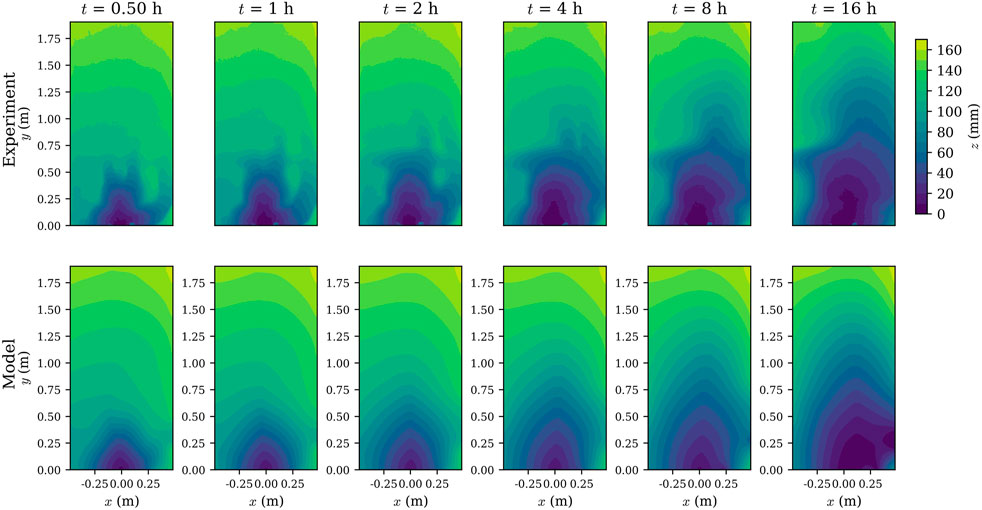
FIGURE 4. Numerical simulation of the LEM and the experimental data. In both experiment and simulation, the morphology evolves toward the high precipitation area.
Results and discussion
Here, we critically examine the LEM’s ability to reproduce the experimental observations of Cheraghi et al. (2018).
Morphological evolution and the corresponding discharge network
The calibrated parameters are presented in Table 2. The average diffusion coefficient (D) is also higher than those reported for the field scale (0.16–222.4 mm2 h−1) (Martin, 2000; Benaïchouche et al., 2016). This is consistent with the lack of rilling in our experiments, as rill formation is favored for lower diffusivities (Sweeney et al., 2015). In contrast to the present experimental setup, previous laboratory experiments analyzed with the LEM used fine particles, low rainfall intensities and small droplets, i.e., they were designed to produce rills (Hancock and Willgoose, 2002; Bonnet and Crave, 2003; Hasbargen and Paola, 2003; Lague et al., 2003; Bonnet and Crave, 2006; Bonnet, 2009; Paola et al., 2009; Graveleau et al., 2012; Rohais et al., 2012; Reinhardt and Ellis, 2015; Singh et al., 2015; Sweeney et al., 2015).
The numerical results are compared with the experimental data in Figure 4. The model is able to capture the main characteristics of the morphology, i.e., the downstream area (z < 60 mm) has a symmetric shape while the upstream (z ≥ 60 mm) area is being eroded. In agreement with the experiment, the model shows a noticeable erosion and growth of downstream region (z < 60 mm) at t = 4 and 8 h. Recall that the model was calibrated using measurements at 8 h, and then used to predict the morphology at t = 16 h, where the agreement is satisfactory. The evolution of the surface elevation change in both experiment and model is more evident in the high precipitation area (right hand side of the flume).
Despite simulating the main characteristics of the morphology evolution, some differences are seen between the model and the experiment. These differences are likely due to the local scale fluid-particle and particle-particle interactions that are not accounted for in the model. One of these processes is the armoring effect (Polyakov and Nearing, 2003; Wang et al., 2014; Cheraghi et al., 2016; Lisle et al., 2017). Due to shorter erosion time scales, the fine sediment particles are rapidly removed while the larger particles are deposited on the surface or are not moved at all, resulting in a surface covered by pebbles (Figure 5). Nonetheless, the calibrated LEM can reproduce the main features of the morphology.
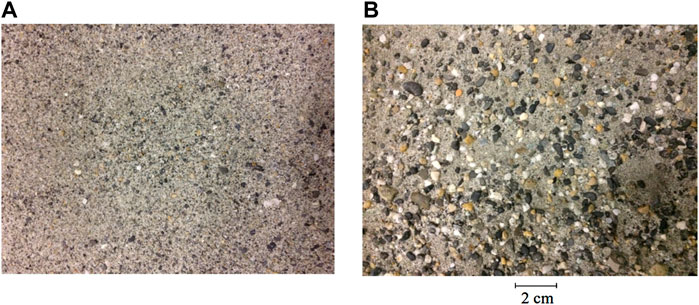
FIGURE 5. Flume surface at t = 0 (A) and t = 16 h (B). This figure is from Cheraghi et al. (2018).
In the LEM (Eq. 1), the advective term is involved in the computation when the value of Q1/2S exceeds the critical value of Ωcr. At the catchment scale, the advection-dominated area are observed as rivers networks (Howard, 1994; Perron et al., 2008). However, in this experiment, there is no visual geomorphological criterion to identify the advective regions as there is no incision.
Spectral analysis
The differences in the modeled and measured morphologies were quantified by taking 2D Fourier transforms of each. We present the resulting power spectral densities (PSD) for the morphologies at t = 8 h (plots for other times are similar) in Figure 6, where we show results as a function of the horizontal wave number (azimuthally averaged). The results show that measured and modeled PSD agree up to a wave number of 5–9 m−1. To check the effect of the differences in the spectra, we applied a low-pass filter (Blackman window, Blackman and Tukey (1958)) to both measurements and modeled morphologies, with a cutoff wave number of about 7 m−1. In Figure 7, the original morphologies of the model and experiment are compared with the filtered ones. Filtering removes the small scale roughness (the large deposited stones shown in Figure 5B) of the experiment while the model results are much less sensitive to the low-pass filtering. In Figure 8, the discharge was calculated via Eq. 2 using the original and filtered morphologies. For the experiment, the complex flow patterns of the measured surfaces (due to microtopography, small scale roughness and armoring) are removed by filtering. On the other hand, there is little change to the discharge network of the model. In short, after filtering, the flow networks for both the experimental measurements and model results are in good agreement. Note that the cutoff wave number and the difference between drainage network before and after filtering are a function of many factors such as sediment particle distribution, microtopography, grain size, cohesion (which does not exist in this experiment) and precipitation rate.
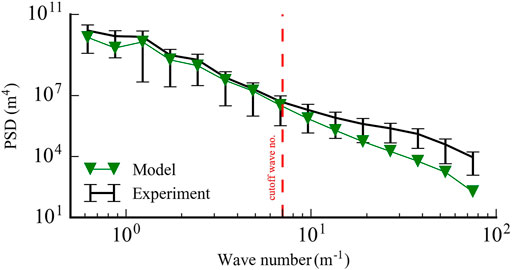
FIGURE 6. Power spectral density of the morphology at t = 8 h. The error bars of the experimental data are based on the standard deviation from the azimuthally averaged PSD in the wave number domain.
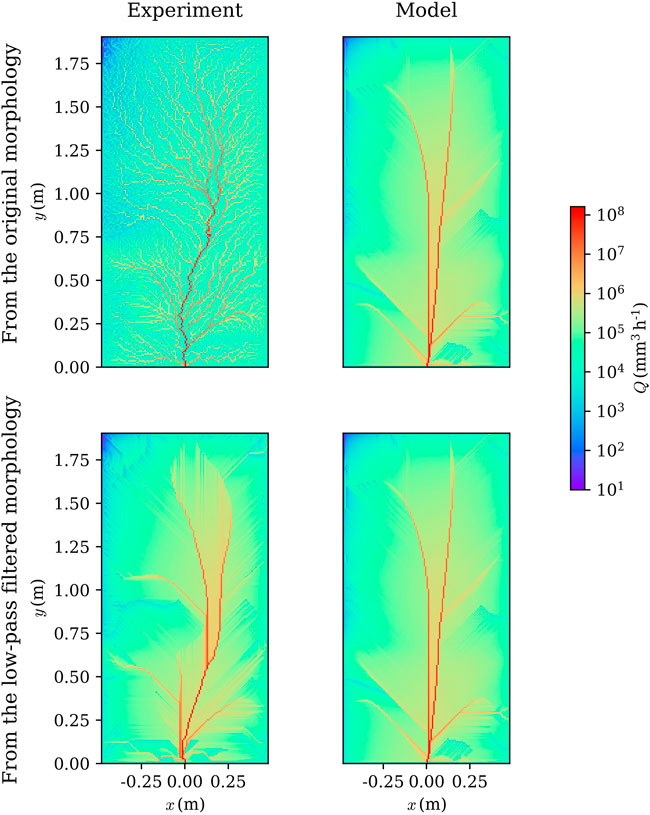
FIGURE 8. The original discharge network and the network extracted from the low-pass filtered morphology at t = 8 h.
The discharge network of the filtered experimental results are compared with the model network in Figure 9. In both experiment and model, the network has a dendritic form at t = 0.5 h. Afterwards, the network becomes more concentrated and the flow from the upstream is directed to two main streams. The two streams migrate from the left to the right of the flume as the precipitation proceeds. This migration is responsible for the dynamic change in the erosion pattern of the upstream (Figure 4). The model is able to reproduce the migration of the concentrated flow to the right hand side, which induces the downstream morphological evolution.
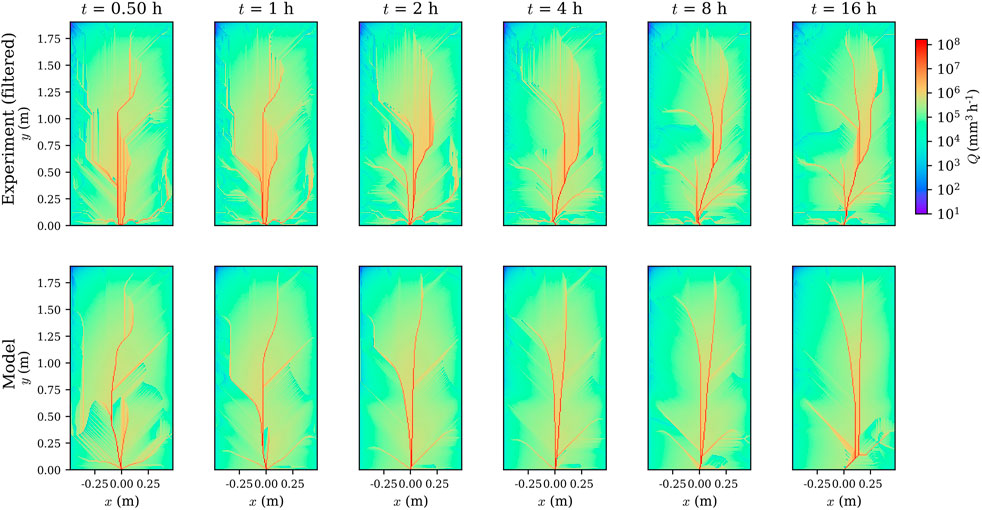
FIGURE 9. Discharge distribution at different times (logarithmic scale). The experimental network was calculated based on the filtered morphology.
An important factor to be investigated in future research would be the scanning resolution. At the catchment-scale, the effect of resolution on the hydrological features such as landslide susceptibility mapping (Meena and Gudiyangada Nachappa, 2019), stream network position (McMaster, 2002), and flood modeling (Muthusamy et al., 2021) is studied. Muthusamy et al. (2021) concluded that the resolution has to be finer than the considered river width for modeling. In the absence of rills, it is highly possible to have similar limitation for the LEM simulation based on the grain sizes and the inter-particle distance after armoring.
Drainage area network statistical analysis
Here we examine the ability of the model to reproduce experimental scaling laws derived from the drainage area network. The drainage area at the ith cell, Ai, was calculated using:
where the summation over j and wji has the same definition as in Eq. 2.
In the analysis of Cheraghi et al. (2018), the experimental data followed a time-invariant power-law relation (Hack’s law, A = lh) between upstream length (l) and drainage area (A) with the scaling exponent h in the range [0.54–0.6] during the network evolution. Furthermore, even though no rills were observed, the exceedance probability of drainage area (P(A > a) = a−β) and the exceedance probability of length (P(L > l) = l−ψ) had time-invariant exponent values of β = 0.47 and ψ = 0.75. These findings show that, at the flume-scale and in absence of incision, the surface morphology is statistically similar to catchments (Rinaldo et al., 1992; Rodriguez-Iturbe and Rinaldo, 2001) in that the same scalings are observed in each case.
The relation between the upstream length (l) and drainage area (A) and the exceedance probabilities for the LEM, original experiment and its low-pass filtered morphology are presented in Figure 10, Figure 11, Figure 12. The results show that the LEM deviates from the original experimental data for the metrics shown in each plot. However, the results each plot show that the model agrees with the low-pass filtered experimental data. These comparisons further confirm the ability of the model to capture the low-pass filtered experimental morphology, but not the micro roughness. On the other hand, the results for the unfiltered experimental data produce scaling laws that agree with previous results for catchments (Rinaldo et al., 1992; Rodriguez-Iturbe and Rinaldo, 2001). Higher order streams of the network (higher drainage area, A > 2 × 104 mm2) are not affected by the low-pass filtering. As a result, both model and filtered experimental results are in agreement with the original experiment in this range.
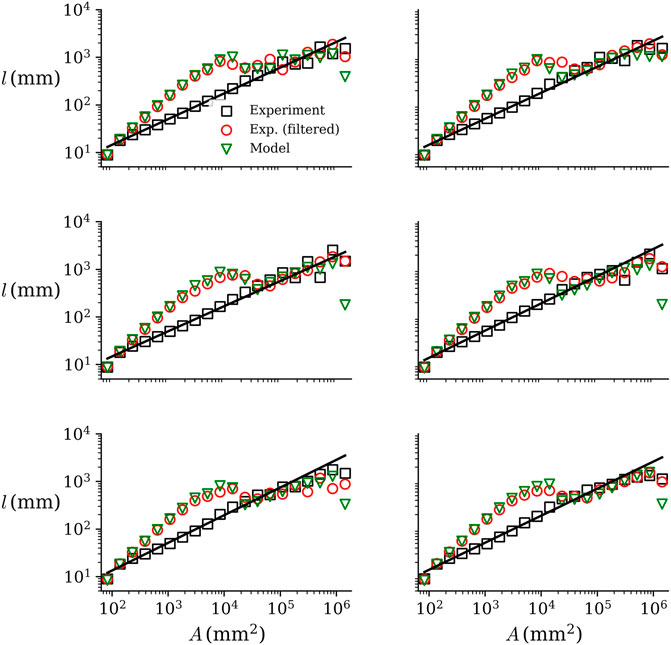
FIGURE 10. Relation between the upstream length and drainage area (Hack’s law) for the model and experiment. The model results differ from the (unfiltered) experimental data, but agrees with the experimental data subjected to the low-pass filter.
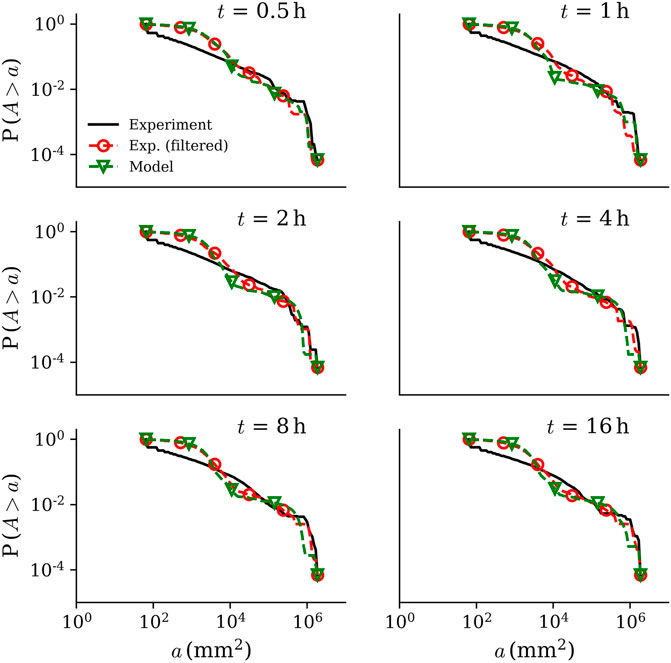
FIGURE 11. Exceedance probability of drainage area for the model, original experiment and its low-pass filtered morphology. The model results and filtered experimental results agree at all times.
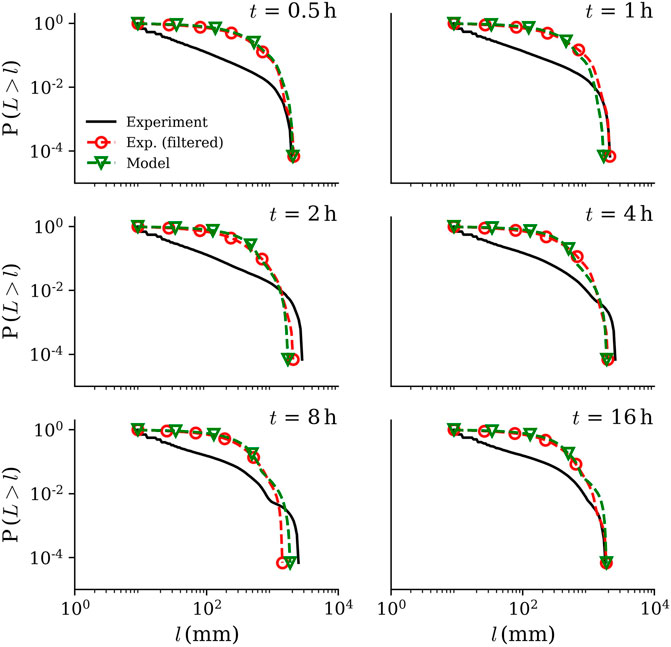
FIGURE 12. Exceedance probability of upstream length the model, original experiment and its low-pass filtered morphology. There is good agreement between the filtered data and the model.
Conclusion
This study introduced the large scale landscape evolution model (LEM), coupled with the d8 algorithm for shallow overland flow, as a robust simulation tool for describing land surface morphology changes in the absence of rills. By calibrating only three model parameters, the LEM was able to capture morphological changes that evolved under a heterogeneous rainfall. This is a manifestation of a simple and efficient model as highlighted by Paola and Leeder (2011). The erosive processes captured by the LEM are broadly characterized as raindrop impact-induced diffusion and surface flow-induced shear stress. Unlike previous investigations, here the surface is continuously covered by overland flow, which is modeled as a flow network.
As described by the LEM, shear stress-driven erosion does not occur until a soil-specific flow rate is exceeded. The LEM-based analysis revealed that even for the considered situation where the morphological evolution is dominated by diffusive processes (and so the surface is not incised), the advective term in the LEM is still necessary to predict the surface evolution. Similarly to natural river networks at catchment scale and incised surfaces in previously reported laboratory experiments, a power-law relation was observed in the discharge exceedance probability for the experimental data. The intricate surface flow details captured by the detailed surface scans of the experimental flume were, however, not present in the modeled surface. Low-pass filtering of the measured and modeled surfaces markedly improved the agreement between the calculated flow networks. However, in that case, the discharge exceedance scaling relationships were not maintained. In general, the LEM could reproduce the low-pass filtered experimental results, i.e., small scale variability due surface roughness that increased over the course of the experiment is not present in the LEM, and had to be removed to obtain reasonable agreement between the experimental measurements and the model results.
Data availability statement
The original contributions presented in the study are included in the article/Supplementary Material, further inquiries can be directed to the corresponding author.
Author contributions
Conceptualization, writing the original draft, investigation and modeling were done by MC. DB, AR and GS supervised the work and edited the manuscript. PP provided the laser scanner and supervised the scanning calibration. AC contributed to the spectral analysis. SJ guided the initial phases of experiment and further contributed to analyzing the rainfall patterns.
Funding
Financial support was provided by the Swiss National Science Foundation (200021-144320).
Acknowledgments
Jacques Roland Golay, Antoine Wiedmer, Pierre-Alain Hildenbrand, Htet Kyi Wynn and Julian Barry provided essential technical support for the execution of the experiment. We also appreciate the collaboration of the SAGRAVE company, Lausanne, Switzerland.
Conflict of interest
The authors declare that the research was conducted in the absence of any commercial or financial relationships that could be construed as a potential conflict of interest.
Publisher’s note
All claims expressed in this article are solely those of the authors and do not necessarily represent those of their affiliated organizations, or those of the publisher, the editors and the reviewers. Any product that may be evaluated in this article, or claim that may be made by its manufacturer, is not guaranteed or endorsed by the publisher.
References
Banavar, J. R., Colaiori, F., Flammini, A., Maritan, A., and Rinaldo, A. (2001). Scaling, optimality, and landscape evolution. J. Stat. Phys. 104, 1–48. doi:10.1023/A:1010397325029
Benaïchouche, A., Stab, O., Tessier, B., and Cojan, I. (2016). Evaluation of a landscape evolution model to simulate stream piracies: Insights from multivariable numerical tests using the example of the Meuse basin, France. Geomorphology 253, 168–180. doi:10.1016/j.geomorph.2015.10.001
Bennett, S. J., Gordon, L. M., Neroni, V., and Wells, R. R. (2015). Emergence, persistence, and organization of rill networks on a soil-mantled experimental landscape. Nat. Hazards 79, 7–24. doi:10.1007/s11069-015-1599-8
Bennett, S. J., and Liu, R. (2016). Basin self-similarity, Hack's law, and the evolution of experimental rill networks. Geology 44, 35–38. doi:10.1130/G37214.1
Berger, C., Schulze, M., Rieke-Zapp, D., and Schlunegger, F. (2010). Rill development and soil erosion: A laboratory study of slope and rainfall intensity. Earth Surf. Process. Landforms 35, 1456–1467. doi:10.1002/esp.1989
Bigi, A., Hasbargen, L. E., Montanari, A., and Paola, C. (2006). Knickpoints and hillslope failures: Interactions in a steady-state experimental landscape. Geol. Soc. Am. Special Pap. 398, 295–307. doi:10.1130/2006.2398(1810.1130/2006.2398(18)
Blackman, R. B., and Tukey, J. W. (1958). The measurement of power spectra from the point of view of communications engineering - Part I. Bell Syst. Tech. J. 37, 185–282. doi:10.1002/j.1538-7305.1958.tb03874.x
Bonetti, S., Hooshyar, M., Camporeale, C., and Porporato, A. (2020). Channelization cascade in landscape evolution. Proc. Natl. Acad. Sci. U.S.A. 117, 1375–1382. doi:10.1073/pnas.1911817117
Bonnet, S., and Crave, A. (2003). Landscape response to climate change: Insights from experimental modeling and implications for tectonic versus climatic uplift of topography. Geol 31, 123–126. doi:10.1130/0091-7613(2003)031<0123:lrtcci>2.0.co;2
Bonnet, S., and Crave, A. (2006). Macroscale dynamics of experimental landscapes. Geol. Soc. Lond. Spec. Publ. 253, 327–339. doi:10.1144/GSL.SP.2006.253.01.17
Bonnet, S. (2009). Erratum: Shrinking and splitting of drainage basins in orogenic landscapes from the migration of the main drainage divide. Nat. Geosci. 2, 897. doi:10.1038/ngeo700
Brunton, D. A., and Bryan, R. B. (2000). Rill network development and sediment budgets. Earth Surf. Process. Landforms 25, 783–800. doi:10.1002/1096-9837(200007)25:7<783::aid-esp106>3.0.co;2-w
Bryan, R. B., and Poesen, J. (1989). Laboratory experiments on the influence of slope length on runoff, percolation and rill development. Earth Surf. Process. Landforms 14, 211–231. doi:10.1002/esp.3290140304
Chen, A., Darbon, J., Buttazzo, G., Santambrogio, F., and Morel, J.-M. (2014). On the equations of landscape formation. Interfaces Free Bound. 16, 105–136. doi:10.4171/IFB/315
Cheraghi, M., Jomaa, S., Sander, G. C., and Barry, D. A. (2016). Hysteretic sediment fluxes in rainfall-driven soil erosion: Particle size effects. Water Resour. Res. 52, 8613–8629. doi:10.1002/2016WR019314
Cheraghi, M., Rinaldo, A., Sander, G. C., Perona, P., and Barry, D. A. (2018). Catchment drainage network scaling laws found experimentally in overland flow morphologies. Geophys. Res. Lett. 45, 9614–9622. doi:10.1029/2018GL078351
Corenblit, D., Davies, N. S., Steiger, J., Gibling, M. R., and Bornette, G. (2015). Considering river structure and stability in the light of evolution: Feedbacks between riparian vegetation and hydrogeomorphology. Earth Surf. Process. Landforms 40, 189–207. doi:10.1002/esp.3643
Costa-Cabral, M. C., and Burges, S. J. (1994). Digital Elevation Model Networks (DEMON): A model of flow over hillslopes for computation of contributing and dispersal areas. Water Resour. Res. 30, 1681–1692. doi:10.1029/93WR03512
Culling, W. E. H. (1963). Soil creep and the development of hillside slopes. J. Geol. 71, 127–161. doi:10.1086/626891
Culling, W. E. H. (1965). Theory of erosion on soil-covered slopes. J. Geol. 73, 230–254. doi:10.1086/627060
Yao, C., Lei, T., Elliot, W. J., McCool, D. K., J. Zhao, J., and S. Chen, S. (2008). Critical conditions for rill initiation. Trans. Am. Soc. Agric. Biol. Eng. 51, 107–114. doi:10.13031/2013.24231
Dunne, T., Malmon, D. V., and Mudd, S. M. (2010). A rain splash transport equation assimilating field and laboratory measurements. J. Geophys. Res. 115, F01001. doi:10.1029/2009JF001302
Forte, A. M., Yanites, B. J., and Whipple, K. X. (2016). Complexities of landscape evolution during incision through layered stratigraphy with contrasts in rock strength. Earth Surf. Process. Landforms 41, 1736–1757. doi:10.1002/esp.3947
Francipane, A., Fatichi, S., Ivanov, V. Y., and Noto, L. V. (2015). Stochastic assessment of climate impacts on hydrology and geomorphology of semiarid headwater basins using a physically based model. J. Geophys. Res. Earth Surf. 120, 507–533. doi:10.1002/2014JF003232
Freeman, T. G. (1991). Calculating catchment area with divergent flow based on a regular grid. Comput. Geosciences 17, 413–422. doi:10.1016/0098-3004(91)90048-I
Furbish, D. J., Hamner, K. K., Schmeeckle, M., Borosund, M. N., Mudd, S. M., Hamner, K. K., et al. (2007). Rain splash of dry sand revealed by high-speed imaging and sticky paper splash targets. J. Geophys. Res. 112, F01001. doi:10.1029/2006JF000498
Gomez, B., and Mullen, V. T. (1992). An experimental study of sapped drainage network development. Earth Surf. Process. Landforms 17, 465–476. doi:10.1002/esp.3290170506
Gómez, J. A., Darboux, F., and Nearing, M. A. (2003). Development and evolution of rill networks under simulated rainfall. Water Resour. Res. 39, 1148. doi:10.1029/2002WR001437
Gordon, L. M., Bennett, S. J., and Wells, R. R. (2011). Evolution of rill networks on soil-mantled experimental landscapes driven by rainfall and baselevel adjustments. Landf. Anal. 17, 57–63.
Gordon, L. M., Bennett, S. J., and Wells, R. R. (2012). Response of a soil‐mantled experimental landscape to exogenic forcing. Water Resour. Res. 48, 1–15. doi:10.1029/2012WR012283
Graveleau, F., Malavieille, J., and Dominguez, S. (2012). Experimental modelling of orogenic wedges: A review. Tectonophysics 538-540, 1–66. doi:10.1016/j.tecto.2012.01.027
Hadka, D., and Reed, P. (2013). Borg: An auto-adaptive many-objective evolutionary computing framework. Evol. Comput. 21, 231–259. doi:10.1162/EVCO-a-0007510.1162/evco_a_00075
Hairsine, P. B., and Rose, C. W. (1992a). Modeling water erosion due to overland flow using physical principles: 1. Sheet flow. Water Resour. Res. 28, 237–243. doi:10.1029/91WR02380
Hairsine, P. B., and Rose, C. W. (1992b). Modeling water erosion due to overland flow using physical principles: 2. Rill flow. Water Resour. Res. 28, 245–250. doi:10.1029/91WR02381
Hairsine, P. B., and Rose, C. W. (1991). Rainfall detachment and deposition: sediment transport in the absence of flow-driven processes. Soil Sci. Soc. Am. J. 55, 320. doi:10.2136/sssaj1991.03615995005500020003x
Han, J., Gasparini, N. M., and Johnson, J. P. L. (2015). Measuring the imprint of orographic rainfall gradients on the morphology of steady-state numerical fluvial landscapes. Earth Surf. Process. Landforms 40, 1334–1350. doi:10.1002/esp.3723
Hancock, G. R., Verdon-Kidd, D., and Lowry, J. B. C. (2017). Soil erosion predictions from a landscape evolution model - An assessment of a post-mining landform using spatial climate change analogues. Sci. Total Environ. 601-602, 109–121. doi:10.1016/j.scitotenv.2017.04.038
Hancock, G. R., and Willgoose, G. R. (2002). The use of a landscape simulator in the validation of the Siberia landscape evolution model: Transient landforms. Earth Surf. Process. Landforms 27, 1321–1334. doi:10.1002/esp.414
Hasbargen, L. E., and Paola, C. (2003). How predictable is local erosion rate in eroding landscapes? Predict. Geomorphol. 231, 231–240. doi:10.1029/135GM16
Hasbargen, L. E., and Paola, C. (2000). Landscape instability in an experimental drainage basin. Geology 28, 1067–1070. doi:10.1130/0091-7613(2000)028<1067:liiaed>2.3.co;2
He, J., Li, X., Jia, L., Gong, H., and Cai, Q. (2014). Experimental study of rill evolution processes and relationships between runoff and erosion on clay loam and loess. Soil Sci. Soc. Am. J. 78, 1716–1725. doi:10.2136/sssaj2014.02.0063
Hooshyar, M., and Porporato, A. (2021). Mean dynamics and elevation-contributing area covariance in landscape evolution models. Water Resour. Res. 57, e2021WR029727. doi:10.1029/2021WR029727
Hooshyar, M., Singh, A., Wang, D., and Foufoula‐Georgiou, E. (2019). Climatic controls on landscape dissection and network structure in the absence of vegetation. Geophys. Res. Lett. 46, 3216–3224. doi:10.1029/2019GL082043
Howard, A. D. (1994). A detachment-limited model of drainage basin evolution. Water Resour. Res. 30, 2261–2285. doi:10.1029/94WR00757
Hu, K., Fang, X., Ferrier, K. L., Granger, D. E., Zhao, Z., and Ruetenik, G. A. (2021). Covariation of cross-divide differences in denudation rate and χ: Implications for drainage basin reorganization in the qilian shan, northeast Tibet. Earth Planet. Sci. Lett. 562, 116812. doi:10.1016/j.epsl.2021.116812
Istanbulluoglu, E., and Bras, R. L. (2005). Vegetation-modulated landscape evolution: Effects of vegetation on landscape processes, drainage density, and topography. J. Geophys. Res. 110, F02012. doi:10.1029/2004JF000249
Jeffery, M. L., Yanites, B. J., Poulsen, C. J., and Ehlers, T. A. (2014). Vegetation-precipitation controls on Central Andean topography. J. Geophys. Res. Earth Surf. 119, 1354–1375. doi:10.1002/2013JF002919
Kwang, J. S., Langston, A. L., and Parker, G. (2021). The role of lateral erosion in the evolution of nondendritic drainage networks to dendricity and the persistence of dynamic networks. Proc. Natl. Acad. Sci. U.S.A. 118, e2015770118. doi:10.1073/pnas.2015770118
Lague, D., Crave, A., and Davy, P. (2003). Laboratory experiments simulating the geomorphic response to tectonic uplift. J. Geophys. Res. 108, 3–1. ETG 3–1–ETG 3–20. doi:10.1029/2002JB001785
Lisle, I. G., Sander, G. C., Parlange, J.-Y., Rose, C. W., Hogarth, W. L., Braddock, R. D., et al. (2017). Transport time scales in soil erosion modeling. Vadose Zone J. 16, vzj2017.06.0121. doi:10.2136/vzj2017.06.0121
Litwin, D. G., Tucker, G. E., Barnhart, K. R., and Harman, C. J. (2022). Groundwater affects the geomorphic and hydrologic properties of coevolved landscapes. J. Geophys. Res. Earth Surf. 127, e2021JF006239. doi:10.1029/2021JF006239
Mahmoodabadi, M., and Sajjadi, S. A. (2016). Effects of rain intensity, slope gradient and particle size distribution on the relative contributions of splash and wash loads to rain-induced erosion. Geomorphology 253, 159–167. doi:10.1016/j.geomorph.2015.10.010
Malamud, B. D., Turcotte, D. L., Guzzetti, F., and Reichenbach, P. (2004). Landslide inventories and their statistical properties. Earth Surf. Process. Landforms 29, 687–711. doi:10.1002/esp.1064
Martin, Y. (2000). Modelling hillslope evolution: Linear and nonlinear transport relations. Geomorphology 34, 1–21. doi:10.1016/S0169-555X(99)00127-0
Massong, T. M., and Montgomery, D. R. (2000). Influence of sediment supply, lithology, and wood debris on the distribution of bedrock and alluvial channels. Geol. Soc. Am. Bull. 112, 591–599. doi:10.1130/0016-7606(2000)112<591:IOSSLA>2.0.CO;2
McGuire, L. A., Pelletier, J. D., Gómez, J. A., and Nearing, M. A. (2013). Controls on the spacing and geometry of rill networks on hillslopes: Rain splash detachment, initial hillslope roughness, and the competition between fluvial and colluvial transport. J. Geophys. Res. Earth Surf. 118, 241–256. doi:10.1002/jgrf.20028
McMaster, K. J. (2002). Effects of digital elevation model resolution on derived stream network positions. Water Resour. Res. 38, 13-1–13-8. doi:10.1029/2000WR000150
Mudd, S. M. (2016). Detection of transience in eroding landscapes. Earth Surf. Process. Landforms 42, 24–41. doi:10.1002/esp.3923
Muthusamy, M., Casado, M. R., Butler, D., and Leinster, P. (2021). Understanding the effects of digital elevation model resolution in urban fluvial flood modelling. J. Hydrology 596, 126088. doi:10.1016/j.jhydrol.2021.126088
O’Callaghan, J. F., and Mark, D. M. (1984). The extraction of drainage networks from digital elevation data. Comput. Vis. Graph. Image Process. 28, 323–344. doi:10.1016/S0734-189X(84)80011-0
Oliveto, G., Palma, D., and Domenico, A. D. (2010). “Scaling properties of laboratory-generated river networks,” in Proceedings of the International Conference on Fluvial Hydraulics (Braunschweig, Germany. Available at: http://vzb.baw.de/e-medien/river-flow-2010/PDF/B2/B2_14.pdf.
Paola, C., and Leeder, M. (2011). Simplicity versus complexity. Nature 469, 38–39. doi:10.1038/469038a
Paola, C., Straub, K., Mohrig, D., and Reinhardt, L. (2009). The “unreasonable effectiveness” of stratigraphic and geomorphic experiments. Earth-Science Rev. 97, 1–43. doi:10.1016/j.earscirev.2009.05.003
Park, E., and Latrubesse, E. M. (2015). Surface water types and sediment distribution patterns at the confluence of mega rivers: The solimões-amazon and negro rivers junction. Water Resour. Res. 51, 6197–6213. doi:10.1002/2014WR016757
Parker, R. A. (1977). Experimental study of basin evolution and its hydrologic implications. Fort Collins: Colorado State University. Phd thesis. Available at: http://www.dtic.mil/cgi-bin/GetTRDoc?Location=U2&doc=GetTRDoc.pdf&AD=ADA051839.
Pazzaglia, F. J., Carter, M., Berti, C., Counts, R., Hancock, G., Harbor, D., et al. (2015). Geomorphology, active tectonics and landscape evolution in the mid-atlantic region. Geol. Soc. Amer., Field Guides 40, 109–169. doi:10.1130/2015.0040(06)
Pedrazzini, A., Humair, F., Jaboyedoff, M., and Tonini, M. (2016). Characterisation and spatial distribution of gravitational slope deformation in the upper rhone catchment (western swiss alps). Landslides 13, 259–277. doi:10.1007/s10346-015-0562-9
Pelletier, J. D. (2003). Drainage basin evolution in the rainfall erosion facility: Dependence on initial conditions. Geomorphology 53, 183–196. doi:10.1016/S0169-555X(02)00353-7
Perron, J. T. (2017). Climate and the pace of erosional landscape evolution. Annu. Rev. Earth Planet. Sci. 45, 561–591. doi:10.1146/annurev-earth-060614-105405
Perron, J. T., Dietrich, W. E., and Kirchner, J. W. (2008). Controls on the spacing of first-order valleys. J. Geophys. Res. 113, 1–21. doi:10.1029/2007JF000977
Perron, J. T., Kirchner, J. W., and Dietrich, W. E. (2009). Formation of evenly spaced ridges and valleys. Nature 460, 502–505. doi:10.1038/nature08174
Perron, J. T., and Royden, L. (2013). An integral approach to bedrock river profile analysis. Earth Surf. Process. Landforms 38, 570–576. doi:10.1002/esp.3302
Planchon, O., and Darboux, F. (2002). A fast, simple and versatile algorithm to fill the depressions of digital elevation models. Catena 46, 159–176. doi:10.1016/S0341-8162(01)00164-3
Polyakov, V. O., and Nearing, M. A. (2003). Sediment transport in rill flow under deposition and detachment conditions. Catena 51, 33–43. doi:10.1016/S0341-8162(02)00090-5
Press, W. H., Teukolsky, S. A., Vettering, W. T., and Flannery, B. P. (2007). Numerical recipes: The art of scientific computing. Cambridge, UK: Cambridge University Press.
Quinn, P., Beven, K., Chevallier, P., and Planchon, O. (1991). The prediction of hillslope flow paths for distributed hydrological modelling using digital terrain models. Hydrol. Process. 5, 59–79. doi:10.1002/hyp.3360050106
Raff, D. A., Ramírez, J. A., Smith, J. L., and Ramı, J. A. (2004). Hillslope drainage development with time: A physical experiment. Geomorphology 62, 169–180. doi:10.1016/j.geomorph.2004.02.011
Reinhardt, L., and Ellis, M. A. (2015). The emergence of topographic steady state in a perpetually dynamic self‐organized critical landscape. Water Resour. Res. 51, 4986–5003. doi:10.1002/2014WR016223
Ren, Z., Zhang, X., Zhang, X.-c., Li, Z., Li, P., and Zhou, Z. (2021). Sand cover enhances rill formation under laboratory rainfall simulation. Catena 205, 105472. doi:10.1016/j.catena.2021.105472
Reusser, L., Bierman, P., and Rood, D. (2015). Quantifying human impacts on rates of erosion and sediment transport at a landscape scale. Geology 43, 171–174. doi:10.1130/G36272.1
Rieke-Zapp, D. H., and Nearing, M. A. (2005). Slope shape effects on erosion. Soil Sci. Soc. Am. J. 69, 1463–1471. doi:10.2136/sssaj2005.0015
Rinaldo, A., Rigon, R., Banavar, J. R., Maritan, A., and Rodriguez-Iturbe, I. (2014). Evolution and selection of river networks: Statics, dynamics, and complexity. Proc. Natl. Acad. Sci. U.S.A. 111, 2417–2424. doi:10.1073/pnas.1322700111
Rinaldo, A., Rodriguez-Iturbe, I., Rigon, R., Bras, R. L., Ijjasz-Vasquez, E., and Marani, A. (1992). Minimum energy and fractal structures of drainage networks. Water Resour. Res. 28, 2183–2195. doi:10.1029/92WR00801
Rinaldo, A., Rodriguez-Iturbe, I., Rigon, R., Ijjasz-Vasquez, E., and Bras, R. L. (1993). Self-organized fractal river networks. Phys. Rev. Lett. 70, 822–825. doi:10.1103/PhysRevLett.70.822
Rodriguez-Iturbe, I., and Rinaldo, A. (2001). Fractal river basins: chance and self-organization. UK: Cambridge University Press.
Rodriguez-Lloveras, X., Bussi, G., Francés, F., Rodriguez-Caballero, E., Solé-Benet, A., Calle, M., et al. (2015). Patterns of runoff and sediment production in response to land-use changes in an ungauged mediterranean catchment. J. Hydrology 531, 1054–1066. doi:10.1016/j.jhydrol.2015.11.014
Rohais, S., Bonnet, S., and Eschard, R. (2012). Sedimentary record of tectonic and climatic erosional perturbations in an experimental coupled catchment-fan system. Basin Res. 24, 198–212. doi:10.1111/j.1365-2117.2011.00520.x
Römkens, M. J. M., Helming, K., and Prasad, S. N. (2002). Soil erosion under different rainfall intensities, surface roughness, and soil water regimes. Catena 46, 103–123. doi:10.1016/S0341-8162(01)00161-8
Sadeghi, S. H., Kiani Harchegani, M., and Asadi, H. (2017). Variability of particle size distributions of upward/downward splashed materials in different rainfall intensities and slopes. Geoderma 290, 100–106. doi:10.1016/j.geoderma.2016.12.007
Sansar Raj, S. R., and Thimmaiah, T. (2019). Impact of spatial resolution of digital elevation model on landslide susceptibility mapping: A case study in kullu valley, himalayas. Geosciences 9, 360. doi:10.3390/geosciences9080360
Shelef, E., and Goren, L. (2021). The rate and extent of wind-gap migration regulated by tributary confluences and avulsions. Earth Surf. Dynam. 9, 687–700. doi:10.5194/esurf-9-687-2021
Shit, P. K., Bhunia, G. S., and Maiti, R. (2013). Developments of rill networks: An experimental plot scale study. Jwarp 05, 133–141. doi:10.4236/jwarp.2013.52015
Simpson, G., and Castelltort, S. (2006). Coupled model of surface water flow, sediment transport and morphological evolution. Comput. Geosciences 32, 1600–1614. doi:10.1016/j.cageo.2006.02.020
Simpson, G., and Schlunegger, F. (2003). Topographic evolution and morphology of surfaces evolving in response to coupled fluvial and hillslope sediment transport. J. Geophys. Res. 108, 1–16. doi:10.1029/2002JB002162
Singh, A., Reinhardt, L., and Foufoula-Georgiou, E. (2015). Landscape reorganization under changing climatic forcing: Results from an experimental landscape. Water Resour. Res. 51, 4320–4337. doi:10.1002/2015WR017161
Sklar, L. S., and Dietrich, W. E. (2001). Sediment and rock strength controls on river incision into bedrock. Geol 29, 1087. doi:10.1130/0091-7613(2001)029<1087:SARSCO>2.0.CO;2
Stefanon, L., Carniello, L., D'Alpaos, A., and Rinaldo, A. (2012). Signatures of sea level changes on tidal geomorphology: Experiments on network incision and retreat. Geophys. Res. Lett. 39, a–n. doi:10.1029/2012GL051953
Sweeney, K. E., Roering, J. J., and Ellis, C. (2015). Experimental evidence for hillslope control of landscape scale. Science 349, 51–53. doi:10.1126/science.aab0017
Tarboton, D. G. (1997). A new method for the determination of flow directions and upslope areas in grid digital elevation models. Water Resour. Res. 33, 309–319. doi:10.1029/96WR03137
Tatard, L., Planchon, O., Wainwright, J., Nord, G., Favis-Mortlock, D., Silvera, N., et al. (2008). Measurement and modelling of high-resolution flow-velocity data under simulated rainfall on a low-slope sandy soil. J. Hydrology 348, 1–12. doi:10.1016/j.jhydrol.2007.07.016
Tucker, G. E., and Hancock, G. R. (2010). Modelling landscape evolution. Earth Surf. Process. Landforms 35, 28–50. doi:10.1002/esp.1952
van der Meij, W. M., Temme, A. J. A. M., Wallinga, J., and Sommer, M. (2020). Modeling soil and landscape evolution - the effect of rainfall and land-use change on soil and landscape patterns. SOIL 6, 337–358. doi:10.5194/soil-6-337-2020
Wang, L., Shi, Z. H., Wang, J., Fang, N. F., Wu, G. L., and Zhang, H. Y. (2014). Rainfall kinetic energy controlling erosion processes and sediment sorting on steep hillslopes: A case study of clay loam soil from the loess plateau, china. J. Hydrology 512, 168–176. doi:10.1016/j.jhydrol.2014.02.066
Whipple, K. X., DiBiase, R. A., Ouimet, W. B., and Forte, A. M. (2017). Preservation or piracy: Diagnosing low-relief, high-elevation surface formation mechanisms. Geology 45, 91–94. doi:10.1130/G38490.1
Whipple, K. X., Forte, A. M., DiBiase, R. A., Gasparini, N. M., and Ouimet, W. B. (2017). Timescales of landscape response to divide migration and drainage capture: Implications for the role of divide mobility in landscape evolution. J. Geophys. Res. Earth Surf. 122, 248–273. doi:10.1002/2016JF003973
Whipple, K. X., and Tucker, G. E. (1999). Dynamics of the stream-power river incision model: Implications for height limits of mountain ranges, landscape response timescales, and research needs. J. Geophys. Res. 104, 17661–17674. doi:10.1029/1999JB900120
Whipple, K. X., and Tucker, G. E. (2002). Implications of sediment-flux-dependent river incision models for landscape evolution. J. Geophys. Res. 107. ETG 3–1–ETG 3–20. doi:10.1029/2000JB000044
Willett, S. D., McCoy, S. W., Perron, J. T., Goren, L., and Chen, C.-Y. (2014). Dynamic reorganization of river basins. Science 343, 1248765. doi:10.1126/science.1248765
Willgoose, G., Bras, R. L., and Rodriguez-Iturbe, I. (1991). A coupled channel network growth and hillslope evolution model: 2. nondimensionalization and applications. Water Resour. Res. 27, 1685–1696. doi:10.1029/91WR00936
Willgoose, G., Bras, R. L., and Rodriguez-Iturbe, I. (1992). The relationship between catchment and hillslope properties: Implications of a catchment evolution model. Geomorphology 5, 21–37. doi:10.1016/0169-555X(92)90056-T
Willgoose, G. R. (1989). A physically based channel network and catchment evolution model. Massachusetts Institute of Technology. Ph.D. thesis. Available at: https://dspace.mit.edu/handle/1721.1/14316.
Wu, S., and Chen, L. (2020). Modeling soil erosion with evolving rills on hillslopes. Water Resour. Res. 56, e2020WR027768. doi:10.1029/2020WR027768
Keywords: landscape evolution model, flume experiment, absence of rills, non-uniform rainfall, high resolution morphology, spectral analysis, drainage network, scaling laws
Citation: Cheraghi M, Rinaldo A, Sander GC, Perona P, Cimatoribus A, Jomaa S and Barry DA (2022) Applicability of the landscape evolution model in the absence of rills. Front. Earth Sci. 10:872711. doi: 10.3389/feart.2022.872711
Received: 09 February 2022; Accepted: 15 June 2022;
Published: 07 July 2022.
Edited by:
Luca Mao, University of Lincoln, United KingdomReviewed by:
Daniel Caviedes-Voullième, Brandenburg University of Technology Cottbus-Senftenberg, GermanyErkan Istanbulluoglu, University of Washington, United States
Copyright © 2022 Cheraghi, Rinaldo, Sander, Perona, Cimatoribus, Jomaa and Barry. This is an open-access article distributed under the terms of the Creative Commons Attribution License (CC BY). The use, distribution or reproduction in other forums is permitted, provided the original author(s) and the copyright owner(s) are credited and that the original publication in this journal is cited, in accordance with accepted academic practice. No use, distribution or reproduction is permitted which does not comply with these terms.
*Correspondence: Mohsen Cheraghi, bXNuLmNoZXJhZ2hpQGdtYWlsLmNvbQ==