- 1Jiangsu Key Laboratory of Agricultural Meteorology, School of Applied Meteorology, Nanjing University of Information Science and Technology, Nanjing, China
- 2State Key Laboratory of Water Resources and Hydropower Engineering Science, Wuhan University, Wuhan, China
Because of their relatively simple structures, plastic greenhouses in southern China have poor resistance to adverse external weather conditions. Adverse meteorological condition inside the greenhouse is one of the main limiting factors for facility crop production in southern China. Among them, high temperature and high humidity (HTHH) often co-occurred in greenhouses, inducing great losses. Tomatoes (Lycopersicon esculentum Mill.) are grown under protected environment worldwide. Here, characteristics of HTHH inside plastic greenhouses in southern China were analyzed and tomato was chosen as the sample facility crop to study the effects of HTHH. Daily maximum temperature and average relative humidity (RH) inside plastic greenhouses were simulated using the extreme learning machine (ELM) method to identify HTHH events. The results showed that the plastic greenhouse HTHH events mainly occurred from June to September in southern China, especially in the southernmost region. During 1990 and 2019, the occurrence times and accumulative days of the HTHH events showed a downward trend at 0.3 times/decade and 2.6 days/decade, respectively, which is mainly due to their reduction in July. HTHH affected the growth of tomato, in which high temperature plays a more important role than high RH. Days of flower bud differentiation was more sensitive to HTHH stress than other physiological indexes of tomato. With the increase of the return period of HTHH events, the corresponding losses of physiological indexes of tomato increased, except for the western region, where HTHH events rarely occurred. The results in this study could provide guidance for production and layout of greenhouse-grown tomato, and the research approach can also be applied to other greenhouse-grown crops and meteorological disasters.
Introduction
Tomatoes (Lycopersicon esculentum Mill.), originated in South America, are widely grown worldwide (Viuda-Martos et al., 2014). The optimal air temperature for tomato is between 18.5 and 26.5°C, and relative humidity (RH) is between 50% and 70% (Jones, 2013; Harel et al., 2014; Shamshiri et al., 2018; Zheng et al., 2020). China is one of the main producers of tomato, which produced 125,739,004 t in 2019, ranking first worldwide (FAOSTAT, 2021). In China, ∼38% of vegetables are produced under protected cultivation. Among them, solar greenhouses and plastic greenhouses are the two basic types, accounting for 30% and 67% of the total area of protected cultivation, respectively, and the remaining 3% are mainly multi-span greenhouses (Wang et al., 2020). The main planting areas are located in northern China for solar greenhouses and southern China for plastic greenhouses. Southern China is the hottest and most humid area in the entire country; RH commonly exceeds 70% in summer, and extreme heat increased during the past decades (Wang et al., 2014; Fan et al., 2016; Li and Zha, 2018). High temperature combined with high humidity (HTHH) put more stress on human health (Guo et al., 2019), crop growth, and developing seeds (Wang et al., 2012; Zhao, 2020; Zheng et al., 2020) than a univariate perspective. However, studies on HTHH events at the regional scale and their impacts on protected agriculture are not sufficient.
Temperature is one of the most important environmental factors affecting the growth of tomatoes (Shamshiri et al., 2018). As a result of global warming, high temperature outside the greenhouse frequently occurs (Wang et al., 2014). For protected cultivation, it can be 20–30°C hotter inside greenhouses than outside if there is no microclimate controller (Kittas et al., 2005; Shamshiri et al., 2018). In China, over two-thirds of the protected cultivation were under low-tech facilities. Hence, their ability to regulate and resist adverse meteorological conditions is poor (Li et al., 2017; Li et al., 2019). High temperature can induce low seed setting rate of tomato, and seriously affect yield and quality (Sato et al., 2000; Adams et al., 2001; Mulholland et al., 2003). In many studies, 32°C was taken as the threshold of high temperature for tomato. For instance, Pressman et al. (2002) found that the number and viability of pollen grains of tomato significantly decreased as temperature exceeded 32°C. Sato et al. (2004) indicated that the proportion of aborted flowers significantly increased and the fruit setting rate decreased when temperature was higher than 32°C. Excessive temperature in plastic greenhouses can also cause additional risks and production costs (Shamshiri et al., 2018). These facts indicate that the high temperature-related disasters are a concern in the production of greenhouse-grown tomatoes.
A certain range of RH can alleviate the adverse effects of high temperature on tomato. Plants may also need higher RH if they are suffering from high temperature (Kittas et al., 2005; Shamshiri et al., 2018). For instance, Harel et al. (2014) found that tomato pollination was enhanced as RH reaches 70%. However, excessive RH can enhance high-temperature stress by affecting the transpiration, causing plants to wilt due to the inability of the roots of the plants to acquire sufficient water (Shamshiri et al., 2018), affecting plant photosynthesis (Yang et al., 2018). At present, studies on HTHH stress on greenhouse-grown plants are rare. Weng et al. (2021) used plant height, stem diameter, chlorophyll (Chl), and photosynthetic parameters to analyze the physiological response of melon to HTHH stress at the seedling stage. Zhao (2020) found that tomato fruit size and quality significantly decreased when exposed to HTHH during the fruit expansion period. Flower bud differentiation is a critical indication of final fruit production and quality (Wan et al., 2018), which is the key period from vegetative growth to reproductive growth, and environmental stress at the seedling stage can greatly affect flower bud differentiation (Li et al., 2019). However, after suffering from HTHH stress at the seedling stage, response characteristics for the flower bud differentiation of tomato remain unclear, which is of great significance for the early prediction of tomato fruit production and quality.
Physiological indexes, such as Chl content, stem diameter, stem height, dry mass of seedlings, endogenous hormones, and days of flower bud differentiation, among others, were usually measured to show the response of plants to environmental stress (Zheng et al., 2020; Weng et al., 2021). Chlorophyll is a green pigment in chloroplast thylakoids used for photosynthesis (Fiedor et al., 2008), and the decrease of Chl content in leaves is the main feature of leaf senescence (Rossi et al., 2017). Indexes of stem diameter, stem height, and dry mass of seedlings are used to evaluate the health of plant. In previous studies, these indexes are integrated to construct a composite index to describe the plant health comprehensively (Liu et al., 2015; Li et al., 2020). Abscisic acid (ABA) is an endogenous hormone in plants, which has risen significantly under abiotic stress to regulate its physiological activities (Ng et al., 2014). However, when the ABA content in plant is at a high level, it will cause some negative effects, such as male sterility, resulting in a decline in plant final yield and quality (Singh and Sawhney, 1998). With the increase of abiotic stress, the rate of flower bud differentiation decreased and the days of flower bud differentiation prolonged. In addition, the number of flower buds is lower and they have a smaller size under abiotic stress, leading to the reduction of fruit yield and quality (Tsuchida and Jomura, 2020). Overall, monitoring these indexes is useful to show plant responses to abiotic stresses, an indication of the final fruit yield and quality, especially at the flower bud differentiation stage.
It should be noted that HTHH is one of the main restricting factors in the production of greenhouse-grown tomato in China. However, the distribution characteristics of greenhouse HTHH events at the regional scale are rarely reported, as well as the response characteristics of tomatoes after suffering from HTHH stress in the critical growth period. Hence, the objectives of this study were (1) to simulate the daily maximum temperature and daily average RH in typical plastic greenhouses by using outdoor meteorological observations; (2) to analyze the temporal and spatial variation of HTHH events inside plastic greenhouses; (3) to construct a comprehensive intensity index of HTHH events using the analytic hierarchy process (AHP) method so as to detect the response of tomato suffering from HTHH in seedling stage; and (4) to study the spatial distribution of greenhouse-grown tomato potential losses caused by HTHH. The results can provide essential information in guiding the layout of plastic greenhouses and early prediction of tomato losses when suffering from HTHH stress.
Materials and Methods
Study Area and Meteorological Data Source
Southern China (18°10′–35°07′N; 97°21′–122°50′E) is selected as the study region in this research. The geographic location and terrains are shown in Figure 1. Most of southern China has a subtropical monsoon climate, with a mean annual precipitation and an annual pan evaporation of 1,320 mm and 1,545 mm, respectively, and an annual average maximum and minimum air temperature of approximately 23 and 14°C, respectively (Fan et al., 2016). Owing to these climate characteristics, this region is prone to HHTH, especially in the summer. Greenhouse-grown tomatoes in southern China are mainly grown in the winter half year; seedlings are raised in July to August and fruits can ripen in November. Therefore, HTHH mainly occurred in the seedling stage of greenhouse-grown tomatoes. Data from 305 meteorological stations located in the study region during 1990–2019 are collected. The collected parameters included daily outdoor maximum air temperature, minimum air temperature, average RH, minimum RH, sunshine duration, and average wind speed at 1.5 m above ground surface. These meteorological data are maintained and rigorously quality controlled by the China Meteorological Administration (http://data.cma.cn/).
Definition of Plastic Greenhouse HTHH Events
For tomato production, the optimal range of RH is between 50% and 70%, and temperature exceeding 32°C will bring losses. Hence, in this study, we defined a daily maximum temperature of >32°C inside the plastic greenhouse as high temperature, and an average RH of >70% inside the plastic greenhouse is considered as high humidity. When high temperature and high humidity co-occurred and lasted for 3 days or more, it can be defined as a plastic greenhouse HTHH event.
Simulation of Daily Maximum Temperature and Average RH in Plastic Greenhouses
There are no long-term historical microclimate data inside plastic greenhouses in southern China. It is practical and valuable to simulate microclimate inside greenhouses by using observations from outdoor meteorological stations for studying spatiotemporal characteristics of plastic greenhouse HTHH events at the regional scale. Machine learning methods are employed to simulate long-term daily maximum temperature and average RH in typical plastic greenhouses. Based on the existing literatures, we select six machine learning methods that are most commonly used in the field of meteorology. The selected models include multiple linear regression (MLR) (Lee et al., 2019); support vector machine (SVM) (Bayat et al., 2020; Fan et al., 2021), which is a kernel-based algorithm; two neural networks algorithms, back-propagation neural network (BP) (Sun, 2017) and extreme learning machine (ELM) (Feng et al., 2020); and two tree-based ensemble models, random forest (RF) (Jin et al., 2020) and extreme gradient boosting models (XGBoost) (Fan et al., 2021).
In order to build the simulation models, daily maximum temperature and average RH in three typical plastic greenhouses were observed. The locations of the greenhouses and the observation periods are shown in Table 1. After abandoning the abnormal observations, which accounted for 1.06% of the total observation, there are a total of 2,230 daily maximum temperature records and 1,502 daily average RH records (the observations from Liancheng were not used to build the RH simulation model, because of RH inside this greenhouse is mainly controlled artificially). The daily observations of nearby meteorological stations are used as input independent variables to simulate daily maximum temperature and average RH inside greenhouses. The sample data are divided into two subsets, the odd days are used to train model and the remaining are used to evaluate the model.
After several variable combination attempts, eight variables are selected to simulate daily maximum temperature inside the plastic greenhouse: outdoor maximum temperature of the day, outdoor average RH of the day, outdoor minimum RH of the day, outdoor sunshine duration of the day, outdoor maximum temperature of the previous day, outdoor maximum temperature of the next day, outdoor average RH of the next day, and outdoor minimum RH of the next day. Seven variables are selected to simulate the daily average RH inside the plastic greenhouse: day sequence, outdoor average RH of the day, outdoor minimum RH of the day, outdoor sunshine duration of the day, outdoor minimum RH of the previous day, outdoor average wind speed of the previous day, and outdoor minimum RH of the next day.
The performances of the six machine learning methods were evaluated using the coefficient of determination (
Comprehensive Intensity Index of HTHH
Response of tomato to HTHH stress is related to the temperature, humidity, and duration of the event. A comprehensive intensity index (
where
where
The AHP method was first introduced by Saaty (1977; Saaty 1994). It is an effective decision-making tool to solve multiple-criteria problem, and can provide the weights of the parameters (Halil et al., 2013; Pramanik, 2016; Ren et al., 2019). In this study, the AHP method is used to determine the weights of the three elements describing the intensity of HTHH. The basic step of AHP is to establish the judgment matrices by a pairwise comparison method. The weights can be obtained by solving the judgement matrix. The fundamental scale for the pairwise comparison was suggested by Saaty (1994), as shown in Table 2. The pairwise matrix and weights of the three elements of HTHH are shown in Effects of HTHH Stress on the Physiological Indexes of Tomato Based on the Experiment section.
Experimental Design and the Measured Physiological Indexes
Seedlings of tomato variety, Lycopersicon esculentum Mill. Shouhe Fenguan, were planted in a Venlo-type greenhouse in Nanjing University of Information Science and Technology from April to July 2020. At the stage of fourth true leaf, seedlings were transferred to an artificial climate box (TPG1260, Australia) and exposed to the HTHH stress. A three-factor orthogonal experimental design was conducted. The factor of high temperature (daily maximum/minimum temperature) was set as four levels: 32/22°C (
Days of flower bud differentiation (DD), Chl content of leaves, ABA content of terminal buds, and seedling quality index (SQI) were measured for each treatment to show the responses of plants to HTHH stress. The date when the apical meristem became slightly visible and small flat bumps began to form is recorded as the beginning of flower bud differentiation, and the date when flower organs became fully developed is regarded as the end of the flower bud differentiation. An Olympus electron microscope was used to observe the flower bud differentiation process. SQI, Chl, and ABA content were measured when flower bud differentiation ended. SQI was calculated as: (diameter of the main stem/plant height) × dry mass of whole plant (Li et al., 2020). Duncan’s test (p ≤ 0.05) was used to assess differences in physiological indices under different treatments.
To make the four physiological indexes comparable, change rates were calculated as Eqs. 3, 4. The physiological indexes that increased under abiotic stress were calculated with Eq. 3, such as ABA and DD. The physiological indexes that decreased under abiotic stress were calculated with Eq. 4, such as SQI and Chl.
where
Results
Performance of the Selected Machine Learning Methods
Six machine learning methods were used to simulate daily maximum temperature and average RH in typical plastic greenhouses. The performances of the machine learning methods are shown in Table 4. The results showed that for both daily average RH and maximum temperature, the ELM model had the greatest
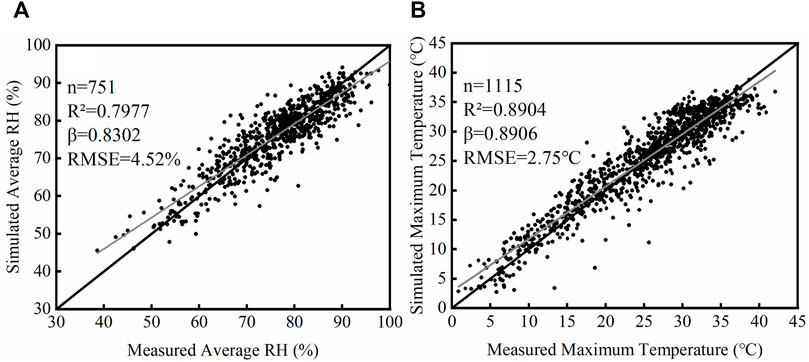
FIGURE 2. | Observation and simulated daily average RH (A) and maximum temperature (B) in plastic greenhouses by the ELM method.
The Spatiotemporal Variation of Plastic Greenhouse HTHH Events
HTHH events in plastic greenhouses were extracted in southern China during 1990 and 2019 according to the definition in the Definition of Plastic Greenhouse HTHH Events. The spatial distribution of multi-year average occurrence times, average duration days, and annual accumulative days of HTHH events were analyzed. As shown in Figure 3A, the annual occurrence times of HTHH events showed the largest value in the southernmost areas of the study region (often annually >10 times). Average duration days of HTHH events was high along the eastern and southern coasts with the events often lasting over 6 days (Figure 3B). Annual accumulative HTHH days were highest in the southern area (Figure 3C), where the HTHH events occurred frequently and lasted for longer days. However, in the western area of the study region, HTHH events seldom happened.
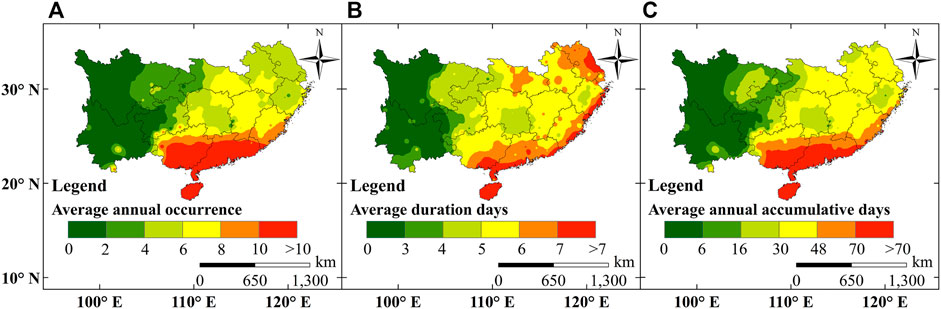
FIGURE 3. Spatial distribution of multi-year average occurrence times (A), average duration days (B), and annual accumulative days (C) of HTHH events in southern China.
The variation of plastic greenhouse HTHH events in different months is shown in Figure 4A. The HTHH events mainly occurred from June to September in southern China, accounting for 86.93% of the total occurrence times of HTHH events, and 89.27% of the total accumulative days. From June to September, the average monthly occurrence times was ∼1.2 and the average duration days was ∼6 days. The HTHH events rarely occurred in October to May of the next year. The interannual variation of the HTHH events during 1990 and 2019 is shown in Figure 4B. The annual occurrence times and the annual accumulative days of the HTHH events showed a significant downward trend with 0.37 times/decade (p < 0.01) and 2.63 days/decade (p < 0.05), respectively, during this period. In order to find out which months caused this decreasing trend, the interannual changes of HTHH events in different months were analyzed. We found that the occurrence times and accumulative days of the HTHH events in July had a significant downward trend, while there was no obvious interannual trend in other months. As shown in Figure 4B, the occurrence times and the accumulative days of the HTHH events in July decreased significantly (p < 0.05) by 0.1 times/decade and 1.3 days/decade, respectively.
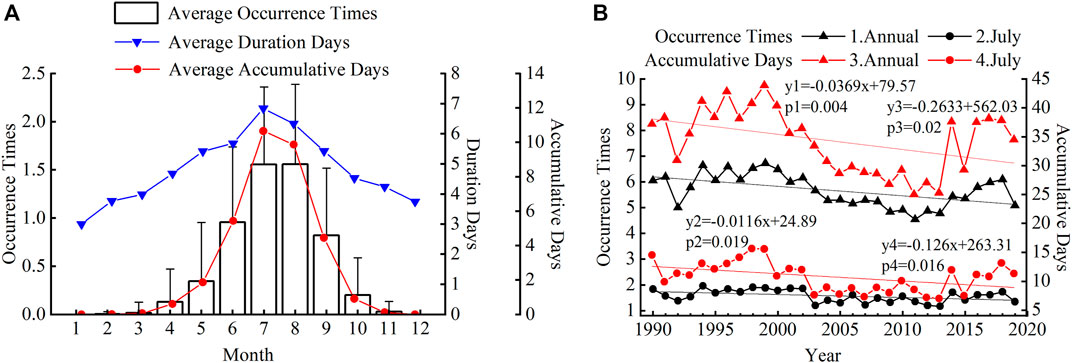
FIGURE 4. The temporal variations of HTHH events. (A) Variations from month to month. (B) Interannual variations of the HTHH events. Black lines show the variations of occurrence times of the HTHH events; red lines show the accumulative days of the HTHH events; triangles are for annual average HTHH events; and dots show the HTHH events in July.
Effects of HTHH Stress on the Physiological Indexes of Tomato Based on the Experiment
The physiological indexes of tomatoes after flower bud differentiation were measured for each treatment, as shown in Figure 5. The physiological indexes had significant (p < 0.05) differences under different high-temperature treatments. High temperature significantly increased the values of
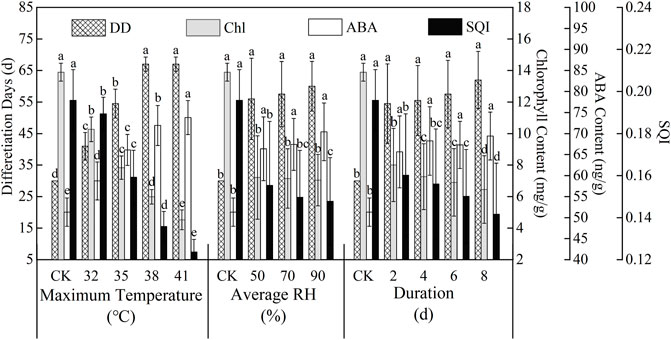
FIGURE 5. Values of physiological indexes of tomato under different HTHH stress treatments. In the same group, data having the same letters atop bars are not significantly different determined by Duncan’s test (p ≤ 0.05). The error bars represent standard deviation.
According to the responses of physiological indexes of tomato under different HTHH stress treatments and after consulting some experts, we build the pairwise comparison matrix. Then, the weight of each element of HTHH event based on the AHP method can be calculated. The results are shown in Table 5. The weight of high temperature, average RH, and duration days are 0.579, 0.234, and 0.187, respectively. Based on Eq. 1, the
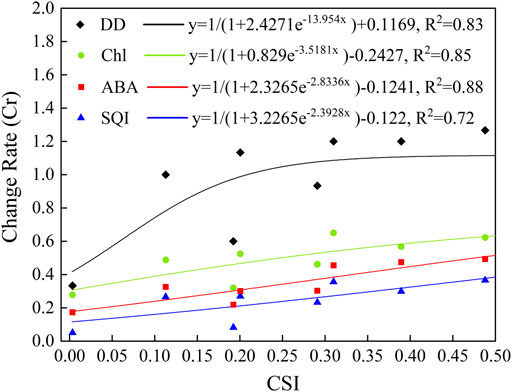
FIGURE 6. Relationships between comprehensive intensity of HTHH (
Change Rates of Tomato Physiological Indices Caused by the HTHH Events at Different Return Periods
HTHH events are recognized at every station from 1990 to 2019, and
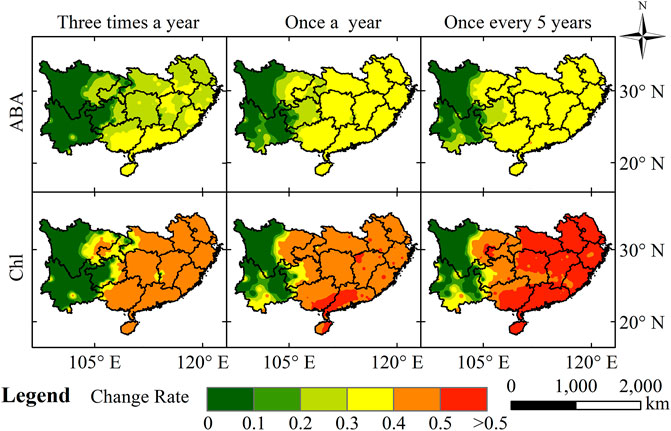
FIGURE 7. The spatial distribution of the potential loss rates of ABA and Chl caused by HTHH events in southern China with return periods of three times a year, once a year, and once every 5 years.
Discussion and Conclusion
Discussion
Outdoor daily maximum and minimum temperature, RH, sunshine duration, and wind speed are the essential elements to simulate the maximum temperature and RH inside plastic greenhouses. Against the backdrop of global warming, there were significant upward trends for the daily maximum temperature and daily minimum temperature across various climatic zones of China (Fan et al., 2016). A downward trend for daily RH was found during 1956–2015 in China; this downward trend reached significance at −0.124%/year during 1986–2015, and southern China showed the greatest decline magnitude (Fan et al., 2016). However, Yan et al. (2019) found that the high-temperature and high-humidity events have increased since the mid-1980s in the middle and lower reaches of Yangtze River, in the northern part of southern China. Wind speed decreased in the 1990s and increased after 2000 in southern China (Fan et al., 2016). Since the 1990s, the sunshine duration have increased in southern China (Fan et al., 2016; He et al., 2018). Previous studies were more concerned about univariate meteorological elements; high-temperature and high-humidity events were less researched. Western north Pacific subtropical high westward extension and intensification during the past decades plays an important role in regulating China’s climate in summer (He et al., 2015; Wang et al., 2014). In this study, it was found that there was a decreasing trend of the interannual variation of the HTHH events in plastic greenhouses, especially in summer. This is different from the result of increased outdoor HTHH events obtained by Yan et al. (2019). This may have something to do with increased sunshine duration and wind speeds, which prevents the RH in plastic greenhouse from being too high.
Previous studies on the environmental constraints for facility crops mainly focus on a single factor, such as high temperature, low temperature, scant light, and high humidity. However, these environmental constraints often co-occurred in actual production, such as low temperature and scant light, high temperature, and high humidity. However, these composite stresses are seldom studied. Heat stress research trials were carried out in many previous studies, and showed that high temperature had seriously adverse impacts on the growth, fruit yield, and quality of tomatoes (Mulholland et al., 2003; Shamshiri et al., 2018; Ro et al., 2021). Hence, identification of tomato genotypes with higher resistance at high temperatures continues to draw the attention of researchers (Xu et al., 2017; Akhoundnejad et al., 2020; Vijayakumar et al., 2021). These studies found that the effects of high temperature on heat-resistant tomato genotypes were commonly alleviated by more than 10% compared to that on heat-sensitive genotypes. Therefore, selection of heat-resistant tomato varieties can be an effective method to mitigate the adverse effect of high temperature. Compared with high temperature, studies on high humidity are relatively less reported. RH affects plants mainly though regulating stomata. Arve and Torre (2015) reported that high RH slightly decreased ABA, which is consistent with the results in this study. In addition, with regard to the effect of HTHH stress on tomato growth, we found that high temperature plays a more important role than high RH and duration days. Since high temperature and high humidity often occur simultaneously and will cause more serious effects, the performance of high-temperature-resistant genotypes under high-humidity conditions should be further assessed in a future study.
Here, we found that the law of physiological indexes of tomato changed with comprehensive intensity of HTHH, and the goodness of fit of all the curves was at the 0.01 significance level (Figure 6). In many previous studies, this kind of relationship was commonly called vulnerability curve, which can be used to assess the sensitivity and adaptability of a hazard-bearing body to adverse effects (Yue et al., 2015; Wang et al., 2016; Cui et al., 2019; Li et al., 2021). It is also an important part of disaster risk assessment. Most of these vulnerability curve studies were for field crops, such as soybean, wheat, and maize. However, facility agriculture was rarely involved. Hence, the curves obtained in this study can be potentially used for disaster risk assessment in the facility agriculture, and provide essential information for guiding the layout of plastic greenhouses and early prediction of tomato losses when suffering from HTHH stress. However, due to the differences in variety, irrigation, and fertilizer usage, among others, the response of tomatoes to HTHH stress in actual greenhouse production may differ, to a certain extent, from the estimated vulnerability curves derived in this study. In addition, plastic greenhouses in practical production have different qualities and structures, so the relationship between the microclimate inside plastic greenhouses and external meteorological conditions may be different for different plastic greenhouses. This increased the uncertainty of our results. In future research, collecting microclimate observations from a larger number of and more dispersed plastic greenhouses can reduce the uncertainty.
Conclusion
Adverse microclimate conditions in greenhouses can lead to crop production losses and increase regulation costs. In this study, southern China was chosen as the case study area to investigate the spatiotemporal pattern of HTHH events in plastic greenhouses. In addition, physiological responses of tomato to HTHH stress were explored, so as to quantitatively identify the spatial distribution of tomato potential losses under HTHH stress in southern China. Major conclusions are as follows:
1) Among the machine learning methods, the ELM method performed best to simulate the daily average RH and maximum temperature in typical plastic greenhouses by using outdoor meteorological observations.
2) The HTHH events in plastic greenhouses mainly occurred in the southernmost areas of the study region (often annually >10 times). About 86.93% of the HTHH events occurred from June to September. Both the occurrence times and the accumulative days showed a decreasing trend from 1990 to 2019, especially in July.
3) High temperature plays a more important role than RH and duration days with regard to the effect of HTHH stress on tomato growth. Among physiological indexes, DD was the most sensitive parameter to HTHH stress.
4) As the return period of HTHH event increased, the corresponding losses of physiological indexes of tomato increased, except for the western region, where the HTHH events rarely happened.
The results of this study are significant in guiding the layout of plastic greenhouses based on local climate resources. Although this research focused on greenhouse-grown tomato and HTHH, the approaches and technical means in this study can also be further applied to other greenhouse meteorological disasters and crops.
Data Availability Statement
Publicly available datasets were analyzed in this study. These data can be found here: http://data.cma.cn/.
Author Contributions
All authors contributed to the study conception and design. Data collection and analysis were performed by XZ and QH. The first draft of the manuscript was written by QZ and XZ. RQ and ZY approved the manuscript.
Funding
This work was supported by the National Key Research and Development Program of China under Grant No. 2019YFD1002202.
Conflict of Interest
The authors declare that the research was conducted in the absence of any commercial or financial relationships that could be construed as a potential conflict of interest.
Publisher’s Note
All claims expressed in this article are solely those of the authors and do not necessarily represent those of their affiliated organizations, or those of the publisher, the editors, and the reviewers. Any product that may be evaluated in this article, or claim that may be made by its manufacturer, is not guaranteed or endorsed by the publisher.
References
Adams, S., Cockshull, K. E., and Cave, C. R. J. (2001). Effect of Temperature on the Growth and Development of Tomato Fruits. Ann. Bot. 88 (5), 869–877. doi:10.1006/anbo.2001.1524
Akhoundnejad, Y., Dasgan, Y. H., and Karabiyik, S. (2020). Pollen Quality, Pollen Production and Yield of Some Tomato (Solanum lycopersicum) Genotypes under High Temperature Stress in Eastern Mediterranean. Not Bot. Horti Agrobo 48 (2), 893–905. doi:10.15835/nbha48211896
Armstrong, B., Sera, F., and Vicedo-Cabrera, A. M., 2019. The Role of Humidity in Associations of High Temperature with Mortality: a Multicountry, Multicity Study. Environmental Health Perspectives, 127, 097007.doi:10.1289/ehp5430
Arve, L. E., and Torre, S. (2015). Ethylene Is Involved in High Air Humidity Promoted Stomatal Opening of Tomato (Lycopersicon esculentum) Leaves. Funct. Plant Biol. 42, 376–386. doi:10.1071/fp14247
Bayat, H., Asghari, S., Rastgou, M., and Sheykhzadeh, G. R. (2020). Estimating Proctor Parameters in Agricultural Soils in the Ardabil plain of Iran Using Support Vector Machines, Artificial Neural Networks and Regression Methods. Catena 189, 104467. doi:10.1016/j.catena.2020.104467
Cui, Y., Jiang, S., Jin, J., Ning, S., and Feng, P. (2019). Quantitative Assessment of Soybean Drought Loss Sensitivity at Different Growth Stages Based on S-Shaped Damage Curve. Agric. Water Manage. 213, 821–832. doi:10.1016/j.agwat.2018.11.020
Dou, X., and Yang, Y. (2018). Estimating forest Carbon Fluxes Using Four Different Data-Driven Techniques Based on Long-Term Eddy Covariance Measurements: Model Comparison and Evaluation. Sci. Total Environ. 627, 78–94. doi:10.1016/j.scitotenv.2018.01.202
Fan, J., Wu, L., Zhang, F., Xiang, Y., and Zheng, J. (2016). Climate Change Effects on Reference Crop Evapotranspiration across Different Climatic Zones of China during 1956-2015. J. Hydrol. 542, 923–937. doi:10.1016/j.jhydrol.2016.09.060
Fan, J., Zheng, J., Wu, L., and Zhang, F. (2021). Estimation of Daily maize Transpiration Using Support Vector Machines, Extreme Gradient Boosting, Artificial and Deep Neural Networks Models. Agric. Water Manage. 245, 106547. doi:10.1016/j.agwat.2020.106547
Faostat, (2021). Food and Agriculture Organization Corporate Statistical Database. Available online: http://www.fao.org/faostat/es/#data/QC.
Feng, Z., Huang, G., and Chi, D. (2020). Classification of the Complex Agricultural Planting Structure with a Semi-supervised Extreme Learning Machine Framework. Remote Sensing 12, 3708. doi:10.3390/rs12223708
Fiedor, L., Kania, A., Myśliwa-Kurdziel, B., Orzeł, Ł., and Stochel, G. (2008). Understanding Chlorophylls: Central Magnesium Ion and Phytyl as Structural Determinants. Biochim. Biophys. Acta (Bba) - Bioenerg. 1777, 1491–1500. doi:10.1016/j.bbabio.2008.09.005
Halil, A., Ayse, Y. O., and Bülent, T. (2013). Agricultural Land Use Suitability Analysis Using GIS and AHP Technique. Comput. Electro. Agric. 97, 71–82.
Harel, D., Fadida, H., Slepoy, A., Gantz, S., and Shilo, K. (2014). The Effect of Mean Daily Temperature and Relative Humidity on Pollen, Fruit Set and Yield of Tomato Grown in Commercial Protected Cultivation. Agronomy 4, 167–177. doi:10.3390/agronomy4010167
He, C., Zhou, T., Lin, A., Wu, B., Gu, D., Li, C., et al. (2015). Enhanced or Weakened Western North Pacific Subtropical High under Global Warming? Sci. Rep. 5, 16771. doi:10.1038/srep16771
He, Y., Wang, K., Zhou, C., and Wild, M. (2018). A Revisit of Global Dimming and Brightening Based on the sunshine Duration. Geophys. Res. Lett. 45 (9), 4281–4289. doi:10.1029/2018gl077424
Jin, Y., Chen, B., Lampinen, B. D., and Brown, P. H. (2020). Advancing Agricultural Production with Machine Learning Analytics: Yield Determinants for California's Almond Orchards. Front. Plant Sci. 11, 290. doi:10.3389/fpls.2020.00290
Jones, J. B. (2013). Instructions for Growing Tomatoes in the Garden and green-house GroSystems. SC, USA: Anderson,
Kittas, C., Karamanis, M., and Katsoulas, N. (2005). Air Temperature Regime in a Forced Ventilated Greenhouse with Rose Crop. Energy and Buildings 37 (8), 807–812. doi:10.1016/j.enbuild.2004.10.009
Lee, Y., Jung, C., and Kim, S. (2019). Spatial Distribution of Soil Moisture Estimates Using a Multiple Linear Regression Model and Korean Geostationary Satellite (COMS) Data. Agric. Water Manage. 213, 580–593. doi:10.1016/j.agwat.2018.09.004
Li, L., and Zha, Y. (2018). Mapping Relative Humidity, Average and Extreme Temperature in Hot Summer over China. Sci. Total Environ. 615, 875–881. doi:10.1016/j.scitotenv.2017.10.022
Li, M., Chen, S., Liu, F., Zhao, L., Xue, Q., Wang, H., et al. (2017). A Risk Management System for Meteorological Disasters of Solar Greenhouse Vegetables. Precision Agric. 18 (6), 997–1010. doi:10.1007/s11119-017-9514-9
Li, T., Xu, Y., and Zhang, J. (2019). Present Situation and Trend of the Development of Vegetable, Melon and Edible Fungus Industries in China. China Vege 11, 6–9. doi:10.3390/su11195171
Li, Y., Li, J., Wu, G., Li, Y., Shen, A., Ma, D., et al. (2020). Design of an Air Blowing Device above Seedbed: The Effect of Air Disturbance on the Microenvironment and Growth of Tomato Seedlings. horts 55 (8), 1308–1314. doi:10.21273/hortsci15136-20
Li, Z., Zhang, Z., Zhang, J., Luo, Y., and Zhang, L. (2021). A New Framework to Quantify maize Production Risk from Chilling Injury in Northeast China. Clim. Risk Manage. 32, 100299. doi:10.1016/j.crm.2021.100299
Liu, J., Wang, W., Wang, L., and Sun, Y. (2015). Exogenous Melatonin Improves Seedling Health index and Drought Tolerance in Tomato. Plant Growth Regul. 77 (3), 317–326. doi:10.1007/s10725-015-0066-6
Mulholland, B. J., Edmondson, R. N., Fussell, M., Basham, J., and Ho, L. C. (2003). Effects of High Temperature on Tomato Summer Fruit Quality. J. Hortic. Sci. Biotechnol. 78, 365–374. doi:10.1080/14620316.2003.11511633
Ng, L. M., Melcher, K., Teh, B. T., and Xu, H. E. (2014). Abscisic Acid Perception and Signaling: Structural Mechanisms and Applications. Acta Pharmacol. Sin 35, 567–584. doi:10.1038/aps.2014.5
Pramanik, M. K. (2016). Site Suitability Analysis for Agricultural Land Use of Darjeeling District Using AHP and GIS Techniques. Model. Earth Syst. Environ. 2 (2), 56–78. doi:10.1007/s40808-016-0116-8
Pressman, E., Peet, M. M., and Pharr, D. M. (2002). The Effect of Heat Stress on Tomato Pollen Characteristics Is Associated with Changes in Carbohydrate Concentration in the Developing Anthers. Ann. Bot. 90, 631–636. doi:10.1093/aob/mcf240
Ren, C., Li, Z., and Zhang, H. (2019). Integrated Multi-Objective Stochastic Fuzzy Programming and AHP Method for Agricultural Water and Land Optimization Allocation under Multiple Uncertainties. J. Clean. Prod. 210, 12–24. doi:10.1016/j.jclepro.2018.10.348
Ro, S., Chea, L., Ngoun, S., Stewart, Z. P., Roeurn, S., Theam, P., et al. (2021). Response of Tomato Genotypes under Different High Temperatures in Field and Greenhouse Conditions. Plants 10, 449. doi:10.3390/plants10030449
Rossi, S., Burgess, P., Jespersen, D., and Huang, B. (2017). Heat-induced Leaf Senescence Associated with Chlorophyll Metabolism in Bentgrass Lines Differing Heat Tolerance. Crop Sci. 57, 169–178. doi:10.2135/cropsci2016.06.0542
Saaty, T. L. (1977). A Scaling Method for Priorities in Hierarchical Structures. J. Math. Psychol. 15, 57–68. doi:10.1016/0022-2496(77)90033-5
Saaty, T. L. (1994). How to Make a Decision: the Analytic Hierarchy Process. Eur. J. Oper. Res. 48 (1), 9–26. doi:10.1287/inte.24.6.19
Sato, S., Peet, M. M., and Gardner, R. G. (2004). Altered Flower Retention and Developmental Patterns in Nine Tomato Cultivars under Elevated Temperature. Scientia Horticulturae 101, 95–101. doi:10.1016/j.scienta.2003.10.008
Sato, S., Peet, M. M., and Thomas, J. F. (2000). Physiological Factors Limit Fruit Set of Tomato (Lycopersicon esculentum Mill.) under Chronic, Mild Heat Stress. Plant Cel Environ 23 (7), 719–726. doi:10.1046/j.1365-3040.2000.00589.x
Shamshiri, R. R., Jones, J. W., Thorp, K. R., Ahmad, D., Man, H. C., and Taheri, S. (2018). Review of Optimum Temperature, Humidity, and Vapour Pressure Deficit for Microclimate Evaluation and Control in Greenhouse Cultivation of Tomato: a Review. Int. Agrophys 32, 287–302. doi:10.1515/intag-2017-0005
Singh, S., and Sawhney, V. K. (1998). Abscisic Acid in a Male Sterile Tomato Mutant and its Regulation by Low Temperature. J. Exp. Bot. 49 (319), 199–203. doi:10.1093/jxb/49.319.199
Sun, Y.-J. (2017). Evaluation of Online purchase Frequency of Fresh Agricultural Products from the Perspective of BP Neural Network. J. Discrete Math. Sci. Cryptography 20, 1339–1344. doi:10.1080/09720529.2017.1392443
Tsuchida, Y., and Jomura, N. (2020). Effect of Irrigation Amount on Flower Bud Growth and Fruit Set in Japanese Apricot 'Nanko'. Hortic. J. 89 (5), 525–529. doi:10.2503/hortj.utd-213
Vijayakumar, A., Shaji, S., Beena, R., Sarada, S., Rani, S., Stephen, R., et al. 2021. High temperature induced changes in quality and yield parameters of tomato (Solanum lycopersicum L.) and similarity coefficients among genotypes using SSR markers.
Viude-Martos, M., Sanchez-Zapata, E., Sayas-Barberá, E., Sendra, E., Perez-Alvarez, J. A., and Fernández-López, J. (2014). Tomato and Tomato Byproducts. Human Health Benefits of Lycopene and its Application to Meat Products: a Review. Crit. Rev. Food Sci. Nutr. 54 (8), 1032–1049.
Wan, C., Mi, L., Chen, B., Li, J., Huo, H., Xu, J., et al. (2018). Effects of Nitrogen during nursery Stage on Flower Bud Differentiation and Early Harvest after Transplanting in Strawberry. Braz. J. Bot. 41 (1), 1–10. doi:10.1007/s40415-017-0417-9
Wang, L., Ma, H., Song, L., Shu, Y., and Gu, W. (2012). Comparative Proteomics Analysis Reveals the Mechanism of Pre-harvest Seed Deterioration of Soybean under High Temperature and Humidity Stress. J. Proteomics 75 (7), 2109–2127. doi:10.1016/j.jprot.2012.01.007
Wang, M. Y., Li, J. P., and Li, J. J. (2020). Historical Evolution, Scale Distribution and Regional Layout of Facility Vegetables in China. China Cucurbits and Vegetables 33 (7), 86
Wang, Z., Jiang, J., and Ma, Q. (2016). The Drought Risk of maize in the Farming-Pastoral Ecotone in Northern China Based on Physical Vulnerability Assessment. Nat. Hazards Earth Syst. Sci. 16, 2697–2711. doi:10.5194/nhess-16-2697-2016
Wang, W, W., Zhou, W., and Chen, D. (2014). Summer High Temperature Extremes in Southeast China: Bonding with the El Niño-Southern Oscillation and East Asian Summer Monsoon Coupled System. J. Clim. 27, 4122–4138. doi:10.1175/jcli-d-13-00545.1
Wang, Y, Y., Ren, F., and Zhang, X. (2014). Spatial and Temporal Variations of Regional High Temperature Events in China. Int. J. Climatol. 34 (10), 3054–3065. doi:10.1002/joc.3893
Weng, J., Li, P., Rehman, A., Wang, L., Gao, X., and Niu, Q. (2021). Physiological Response and Evaluation of Melon (Cucumis Melo L.) Germplasm Resources under High Temperature and Humidity Stress at Seedling Stage. Scientia Horticulturae 288, 110317. doi:10.1016/j.scienta.2021.110317
Xu, J., Wolters-Arts, M., Mariani, C., Huber, H., and Rieu, I. (2017). Heat Stress Affects Vegetative and Reproductive Performance and Trait Correlations in Tomato (Solanum lycopersicum). Euphytica 213, 156. doi:10.1007/s10681-017-1949-6
Yan, D. L., Yan, J. P., and Li, S. S. (2019). Spatiotemporal Evolution of High Temperature and Humidity in the north and South of the Qinling-Huaihe River. Resour. Environ. Yangtze Basin 28 (9), 2197
Yang, S. Q., Yang, Z. Q., Wang, L., Li, J., Zhang, M. Y., and Li, K. W. (2018). Effect of High Humidity and High Temperature Interaction on Photosynthetic Characteristics of Greenhouse Tomato Crops. Chin. J. Ecol. 37 (1), 57
Yue, Y., Li, J., Ye, X., Wang, Z., Zhu, A.-X., and Wang, J.-a. (2015). An EPIC Model-Based Vulnerability Assessment of Wheat Subject to Drought. Nat. Hazards 78, 1629–1652. doi:10.1007/s11069-015-1793-8
Zhao, L. (2020). Effects of High Temperature and High Humidity on Tomato Fruit Growth, Sugar and Nitrogen Metabolism in Greenhouse. MS. Thesis in Chinese (Jiangsu Province: Nanjing University of Information Science and Technology).
Keywords: high temperature and high humidity, tomato seedling, flower bud differentiation, southern China, machine learning, return period
Citation: Zhang Q, Zhang X, Yang Z, Huang Q and Qiu R (2022) Characteristics of Plastic Greenhouse High-Temperature and High-Humidity Events and Their Impacts on Facility Tomatoes Growth. Front. Earth Sci. 10:848924. doi: 10.3389/feart.2022.848924
Received: 05 January 2022; Accepted: 14 February 2022;
Published: 23 March 2022.
Edited by:
Shibo Fang, Chinese Academy of Meteorological Sciences, ChinaReviewed by:
Ahmed Kenawy, Mansoura University, EgyptEnliang Guo, Inner Mongolia Normal University, China
Zhongyi Sun, Hainan University, China
Copyright © 2022 Zhang, Zhang, Yang, Huang and Qiu. This is an open-access article distributed under the terms of the Creative Commons Attribution License (CC BY). The use, distribution or reproduction in other forums is permitted, provided the original author(s) and the copyright owner(s) are credited and that the original publication in this journal is cited, in accordance with accepted academic practice. No use, distribution or reproduction is permitted which does not comply with these terms.
*Correspondence: Qi Zhang, emhhbmdxODYxMjA2QDEyNi5jb20=; Rangjian Qiu, cWl1cmFuZ2ppYW5AdG9tLmNvbQ==
†These authors have contributed equally to this work and share first authorship