- 1Key Laboratory of Ministry of Education on Virtual Geographic Environment, Nanjing Normal University, Nanjing, China
- 2Jiangsu Center for Collaborative Innovation in Geographical Information Resource Development and Application, Nanjing, China
Permafrost degradation triggered by climate warming can disturb alpine ecosystem stability and further influence net primary productivity (NPP). Known as the “water tower of China”, the Three-River Headwaters Region (TRHR) on the eastern Qinghai-Tibet plateau (QTP), is characterized by a fragile alpine meadow ecosystem underlain by large areas of unstable permafrost and has been subject to rapid climate change in recent decades. Despite some site-specific studies, the spatial and temporal changes in NPP in the different frozen ground zones across the TRHR associated with climate change remain poorly understood. In this study, a physically explicit Noah land surface model with multi-parameterization options (Noah-MP) was employed to simulate NPP changes on the TRHR during 1989–2018. The simulation was performed with a spatial resolution of 0.1° and a temporal resolution of 3h, and validated at two sites with meteorological and flux observations. The results show that the average NPP was estimated to be 299.7 g C m−2 yr−1 in the seasonally frozen ground (SFG) zone and 198.5 g C m−2 yr−1 in the permafrost zone. NPP in the TRHR increased at a rate of 1.09 g C m−2 yr−2 during 1989–2018, increasing in 1989–2003 and then decreasing in subsequent years. The NPP in permafrost area increased at a rate of 1.43 g C m−2 yr−2 during 1989–2018, which is much higher than the rate of change in NPP in the SFG area (0.67 g C m−2 yr−2). Permafrost degradation has complicated ecosystem implications. In areas where permafrost degradation has occurred, both increasing and decreasing changes in NPP have been observed.
Introduction
On the Qinghai-Tibetan Plateau (QTP), carbon cycles occur in a unique way owing to the special geographical features and ecological environment (Lin et al., 2019). From an ecological perspective, the ecosystems of the QTP are extremely vulnerable, and climate changes could pose a great challenge to them (Chen et al., 2014). Climatic warming has been observed across the world and on the QTP during recent decades (Liu and Chen, 2000; Zhao et al., 2004; Trenberth et al., 2013). As a critical ecological barrier for regional sustainable development in China, the Three-River Headwaters Region (TRHR) is one of the most fragile and sensitive terrestrial ecosystems on the QTP (Tong et al., 2014; Mao et al., 2015; Jiang and Zhang, 2016a). At some monitoring sites, ecosystem degradation has been observed in the TRHR in recent years (Guo et al., 2015).
Net primary productivity (NPP) refers to the amount of net organic matter that plants take up from the atmosphere through photosynthesis, and it plays an important role in regulating the carbon cycle of ecosystems (Melillo et al., 1993). Dynamic changes in NPP can be used to assess the Earth’s ability to sustain life and the sustainability of the ecosystem to maintain development in the face of climate change (Zhao and Running, 2010). The TRHR is known as the “Water Tower of China”, and the accurate estimation of NPP change in the TRHR is very important and has always been a hot topic in terrestrial carbon cycle and climate change research on the QTP (Zhao et al., 2013; Jiang and Zhang, 2015; Liang et al., 2016). Most existing studies of NPP changes, especially those related to frozen ground, are generally restricted to the site scale. However, there are few monitoring sites, making it difficult to gain complete insights into NPP changes across the region.
Frozen ground is an integral part of the plateau cryosphere and helps preserve a stable microclimate and ecosystem balance by supplying soil moisture and nutrients for vegetation growth. A significant portion of unstable permafrost underlies the TRHR, which is highly sensitive to climate change. Global warming has triggered permafrost degradation over the past several decades, not only in the circum-Arctic (Osterkamp, 2005; Smith et al., 2005) but also in the TRHR (Wu and Zhang, 2008). It has already been demonstrated that permafrost degradation disturbs alpine ecosystem stability through repeated freezing and thawing and further influences NPP accumulation (Qin and Ding, 2010; Schuur and Abbott, 2011; Burke et al., 2013). Previous modeling studies on NPP changes have primarily been conducted using semiempirical, analytical, or ecological models (Zhang et al., 2016; Lin et al., 2017) and different datasets, and reported widely ranging mean annual NPP values (e.g., Wang et al., 2009; Guo et al., 2013). Unfortunately, these models are generally unable to describe the physical processes occurring in frozen ground, so they lack the ability to represent the effects of frozen ground on changes in NPP in terms of sustaining vegetation growth.
Physically explicit numerical models such as the land surface model (LSM) are theoretically superior to empirical models in their ability to describe physical processes within the soil (Abramowitz et al., 2008). At present, most LSMs focus only on the energy and water balance on the surface without considering the carbon cycle (Chen and Dudhia, 2001; Ek et al., 2003). In addition, many LSMs do not simulate vegetation dynamically, but rely on prescribed vegetation indices (Ruddell et al., 2015). This limits the ability of these LSMs to monitor NPP changes and their responses to permafrost changes. Therefore, a general-purpose LSM that incorporates not only water and energy transport processes, but also carbon cycling and dynamic vegetation is usually required to better represent the physiological mechanisms of vegetation and the interplay between vegetation and frozen ground. Recently, the Noah LSM has been extended into a multiphysics simulation platform (Noah-MP) that includes a dynamic vegetation component (Niu et al., 2011). This model has the potential to facilitate the simulation of carbon assimilation processes and permafrost dynamics. Thus, it provides a solid foundation for this study to simulate NPP changes with full interaction with permafrost dynamics over the TRHR.
This study aims to: 1) investigate the spatiotemporal characteristics of NPP over the entire TRHR during 1989–2018; 2) identify the responses of NPP to climate change in different frozen ground zones in the TRHR; and 3) strengthen the understanding of NPP responses to permafrost degradation in the region. This work has the potential to provide a scientific reference for restoring, conserving and developing the local ecological environment in a changing climate in a sustainable way.
Materials and Methods
Study Area and Data
The TRHR is bounded by longitude 89°24′–102°27′E and latitude 31°39′–36°10′N (Figure 1), with an area of approximately 3.95 × 105 km2. The altitude gradually decreases from the northwest to the southeast, averaging 4,000 m above sea level (a.s.l.). As the source area of the Yellow River, Yangtze River, and Lancang River, the TRHR delivers approximately 40 billion m3 of water downstream every year (Feng et al., 2017). The main land cover types are grassland, desert, wetland, shrub, and forest, with alpine steppe and alpine meadow being the main grassland types (Liu et al., 2014). The TRHR has a typical high-altitude continental climate, with temperature and precipitation exhibiting considerable spatial variations across the TRHR. The mean annual air temperature over the TRHR ranges from −5.6 to −3.8°C and decreases from southeast to northwest (Yi et al., 2012). Annual precipitation generally falls from the southeast (772 mm) to the northwest (262 mm) and is primarily concentrated between June and September due to the influence of the warm and humid air currents emanating from the southern Bay of Bengal (Shi et al., 2017). The ecosystem in the TRHR is fragile and vulnerable, with low resilience to disturbance (Jiang and Zhang, 2016b). Permafrost is widespread in the upper reaches of the Yangtze River, while in the eastern part permafrost is sporadic and the seasonally frozen ground (SFG) is found at low elevations (Zou et al., 2017). Two flux sites are located in the north and southeast to the THRH: the Damxung flux site (DX; 30.49°N, 91.06°E, 4,286 m a.s.l; covered by alpine meadow; Figure 1) and the Haibei flux site (HB; 37.06°N, 101.32°E, 3,205 m a.s.l; covered by alpine shrub-meadow; Figure 1).
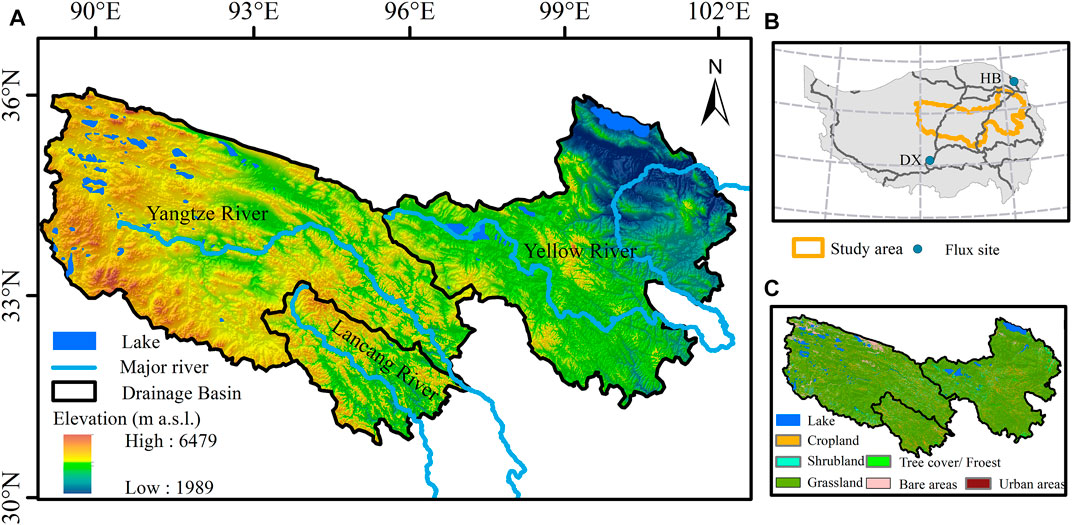
FIGURE 1. Topography of the Three-River Headwaters Region (TRHR) (A), its location on the Qinghai-Tibet Plateau (B), and the distribution of land cover within this region (C). DX: the Damxung flux site; HB: the Haibei flux site.
Vegetation types on the TRHR were extracted from the Vegetation Atlas of China at 1:1 million scale (Hou, 2001). A QTP soil dataset with a resolution of 1 km were used, covering the entire TRHR with 18 soil layers (Wu and Nan, 2016). The gridded Chinese Meteorological Forcing Dataset (CMFD) (0.1° in space and 3 h in time) (He et al., 2020) were used to drive the model. It includes seven meteorological elements such as 2 m air temperature, 10 m wind speed, air pressure, specific humidity, precipitation rate, downward shortwave radiation, and longwave radiation.
Model and Setting
The Noah-MP model is a new-generation land surface model based on Noah LSM, extends the physical processes and introduces the latest developments in related parameterization schemes (Niu et al., 2011). In this model, the states of terrestrial energy, water, carbon, and associated flux exchanges between the land surface and the atmosphere are described in detail. Surface temperatures are calculated through an iterative solution of the surface energy balance including solar radiation, longwave radiation, sensible heat, latent heat, and ground heat fluxes. The “semitile” method is employed to address the heterogeneity of the surface. Based on the Clapp-Hornberber water retention relationship, Noah-MP solves the one-dimensional Richards equation to determine vertical soil moisture distribution (Clapp and Hornberger, 1978).
Noah-MP describes the photosynthesis of C3 and C4 plants by a biochemical model (Collatz et al., 1991; Collatz et al., 1992). Specifically, the gross photosynthesis rate is determined by the minimum of three limiting factors: Rubisco limitation, light limitation and transport of photosynthate for C3 plants and PEP-carboxylase limitation for C4 plants. A short-term vegetation phenology model is coupled to Noah-MP, allowing the model to describe carbon assimilation (Dickinson et al., 1998). The carbon budgets are described for various plant parts, such as leaves, wood, and roots, as well as carbon pools (fast and slow). The assimilation of carbon through photosynthesis, the allocation of the assimilated carbon to various biological carbon pools (leaf, stem, wood, root, and soil), and the respiration from each pool are included in the model. The rate of change in leaf carbon mass,
where
Noah-MP offers a framework for providing multiple options for interpreting and parameterizing a given process (Table 1). Two to four different parameterization schemes are available for twelve physical subprocesses of the model. Each subprocess parameterization scheme can be combined within the model depending on the user’s needs to perform simulation research. The Noah-MP LSM with improved simulation capability for permafrost over the QTP was employed in this study. The physical options used in this study were basically similar to Li et al. (2020) as presented in Table 1. The strength of Noah-MP in simulating thermal and hydrological processes occurring with frozen ground on the QTP was well demonstrated in Li et al. (2020), where more details were also described.
The model was then forced for the period of January 1989 to December 2018, using the CMFD. Prior to the start of the model run, a 30-year spin-up using the repeat forcing in 1989 was conducted to mitigate the effects of the initial values. The total simulation depth was set to 8 m. The lower temperature boundary was set at 16 m using the annual mean air temperature at 2 m (Niu et al., 2011). The model resolution is 3 h in time and 0.1° in space.
Permafrost was determined from the simulation results following the definition of permafrost, the soil with temperatures below 0°C for more than two consecutive years (Muller, 1943). More specifically, a grid cell was classified as permafrost if the maximum soil temperature of any soil layers was below or equal to 0°C for two consecutive years. The remainder of grid cells was classified as SFG only if the minimum soil temperature of any layer was equal to or below 0°C for the same period. When calculating the areas, the simulation maps were projected into Albers’ equal-area projection system and the lakes were excluded from the processing.
Model Calibration and Validation
Restricted by data availability, two monitoring sites (Damxung and Haibei) from the China Flux Network nearby the TRHR with typical alpine vegetation types were selected for model calibration and validation in this study (Figure 1). Two nearby flux monitoring sites, i.e., the Golog site covered by alpine shrub meadow and the Maqu site covered by marsh meadow, were not used because no open-access data are currently available. According to previous studies on the sensitivity of Noah-MP parameters (e.g., Arsenault et al., 2018), we selected two most sensitive parameters related to the vegetation dynamics, i.e., max carboxylation rate and optimal growth temperature of vegetation. We followed an error and trial procedure to calibrate the parameters so that the simulated gross primary product (GPP) data best fit the observed GPP data at the two sites by referring to existing studies (Chen et al., 2021; Yang et al., 2021). At the Damxung site, simulation results were validated against the observed time series (2004–2006) of soil temperature and soil moisture content, and of GPP (2004–2005). At the Haibei site, the soil temperature and soil moisture records from 2004–2006 and GPP from 2003–2005 were used for validation. Metrics such as the widely-used Nash-Sutcliffe efficiency coefficient (NSE) and Pearson’s correlation coefficient (R) were used to quantify the agreement.
Trend Analysis
Theil-Sen trend analysis is a robust statistical trend analysis method widely used to identify both the trends and magnitudes of hydrometeorological variables (Sen, 1968). We computed the change rate (CR) of NPP and climatic factors using Sen’s slope following Eq. 2:
where
The M-K test statistic S is computed as follows:
where n is the number of data points, xi and xj are the NPP values at the time i and j (j > i), respectively, and sgn() is a symbolic function. In cases where the sample size n > 10, the standard normal test statistic (ZS) of the M-K test is given by:
The null hypothesis, H0, is rejected when
Results and Discussion
Model Validation at Two Flux Sites
Figure 2 shows the time series of simulated and observed daily mean soil temperature for differernt soil layers at the Damxung and Haibei sites. The simulated (red lines in Figure 2) and observed (dark green lines) soil temperature were in good agreement at both sites. The NSE and R values for soil temperature were above 0.88 and 0.81, respetively, for all depths at two sites. Soil temperature exhibted strong seasonality in these layers, and the amplitude of soil temperature variation decreased with soil depth, as expected. In spite of some discrepancies between simulation and observation, the Noah-MP LSM performed well overall and can capture well the temporal variation of observations at different depths at the two sites.
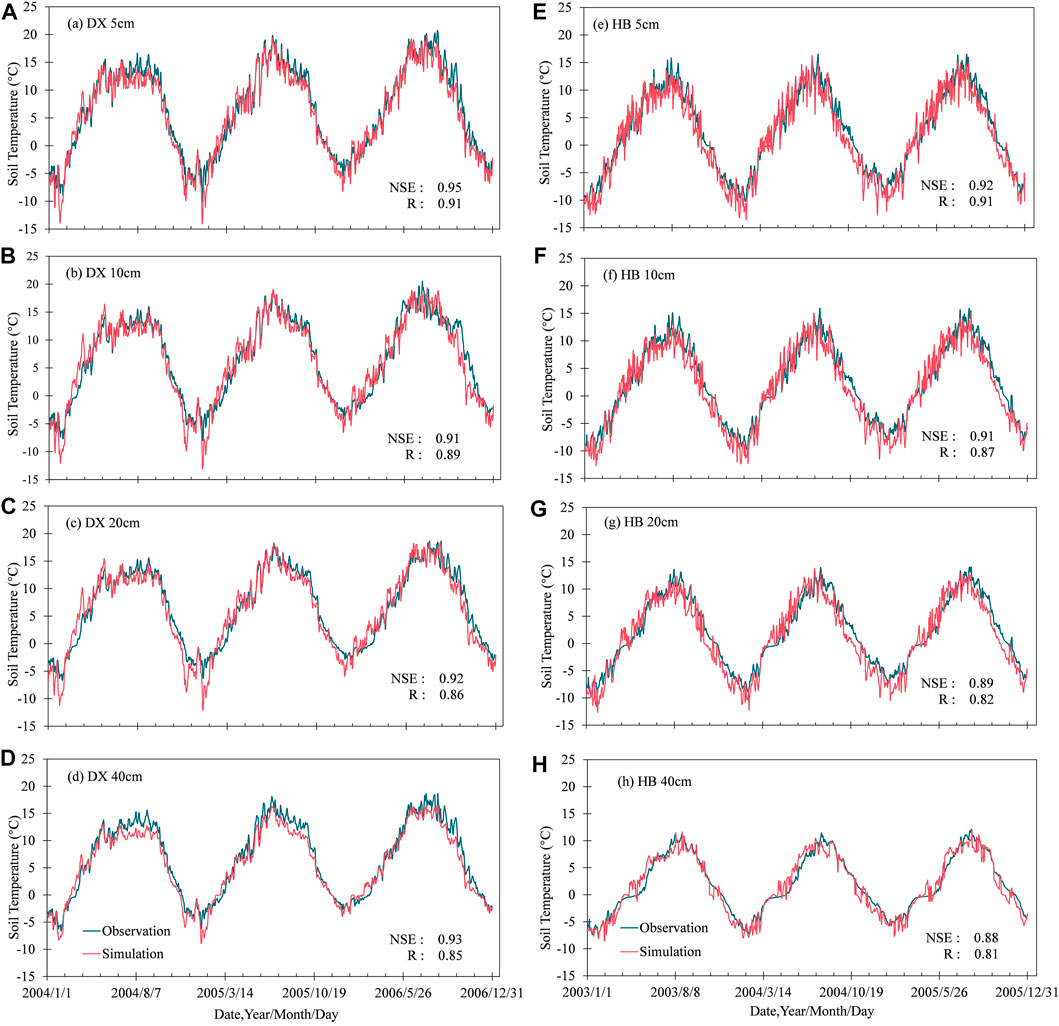
FIGURE 2. Simulated and observed soil temperatures at different depths at Damxung [DX, (A–D)] and Haibei [HB, (E–H)] sites. NSE: Nash-Sutcliffe efficiency coefficient; R: Pearson’s correlation coefficient.
There was also a good agreement between the simulated (red lines in Figure 3) and measured (dark green lines) unfrozen water content. Since no measurements of unfrozen water content were made in deep layers, the comparison was limited to the upper layers. The NSE values for unfrozen water content ranged from 0.80 to 0.83 in all layers at both sites, and the R values exceeded 0.8 in all layers. The unfrozen water content cyclically fluctuated with the seasons. During the transition periods from the frozen to the thawed state, the simulated unfrozen water content changes rapidly in response to the phase change. At the Haibei site, there was a slight overestimation during the cold seasons (December to March). This could be caused by insufficient consideration of the ice process in the model. Overall, the Noah-MP LSM captured well the dynamics of soil mositure at different depths at the two sites.
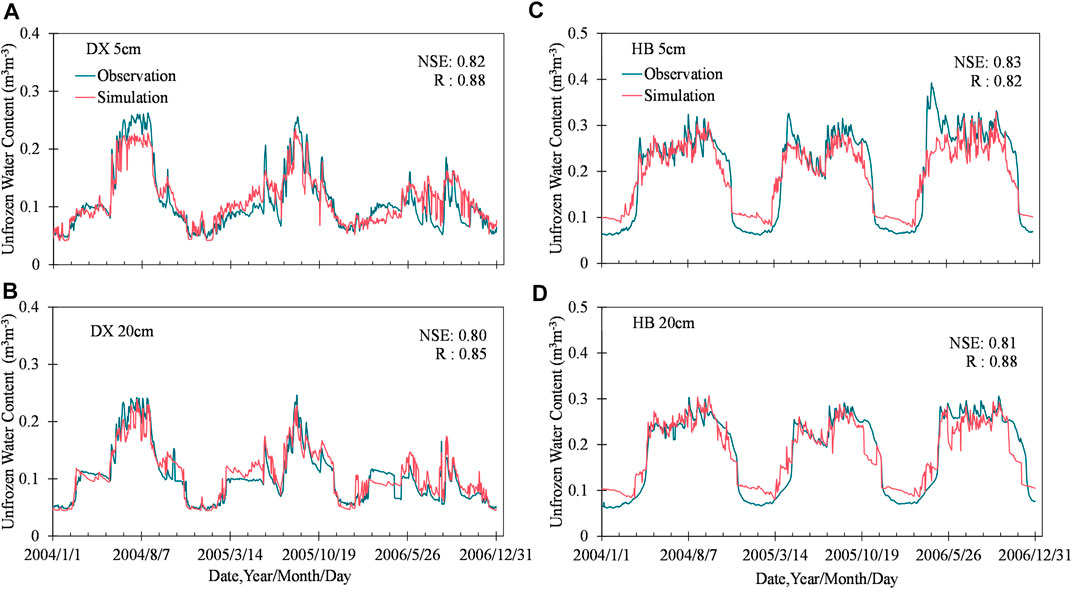
FIGURE 3. Simulated and observed unfrozen water content at different depths at Damxung [DX, (A,B)] and Haibei [HB, (C,D)] sites. NSE: Nash-Sutcliffe efficiency coefficient; R: Pearson’s correlation coefficient.
The comparisons between the simulations and the corresponding observations in terms of GPP are shown in Figure 4. The simulated (orange lines in Figure 4) and observed (dark green lines) GPP agreed well at both sites, as indicated by favorable values in NSE (0.79 at Damxung and 0.82 at Haibei) and R (0.75 at Damxung and 0.78 at Haibe). Some discrepancies between the simulation and observation can be observed at the Damxung site. For example, the GPP observations recorded a short-term spike in June 2004, while the GPP simulations showed a trend toward a gradual increase during the same period. According to our knowledge, the growing season at the Damxung site usually occurred in the warm months from May to September each year and vegetation growth generally peaked amidst the growing season (Shen et al., 2015). Since that flux observations are directly measured by the eddy correlation method based on the micrometeorology theory, some error maybe be present in the observations. Despite some discrepancies, the favorable NSE and R scores for both sites are suggestive that the Noah-MP LSM is capable of tracking daily GPP changes over time.
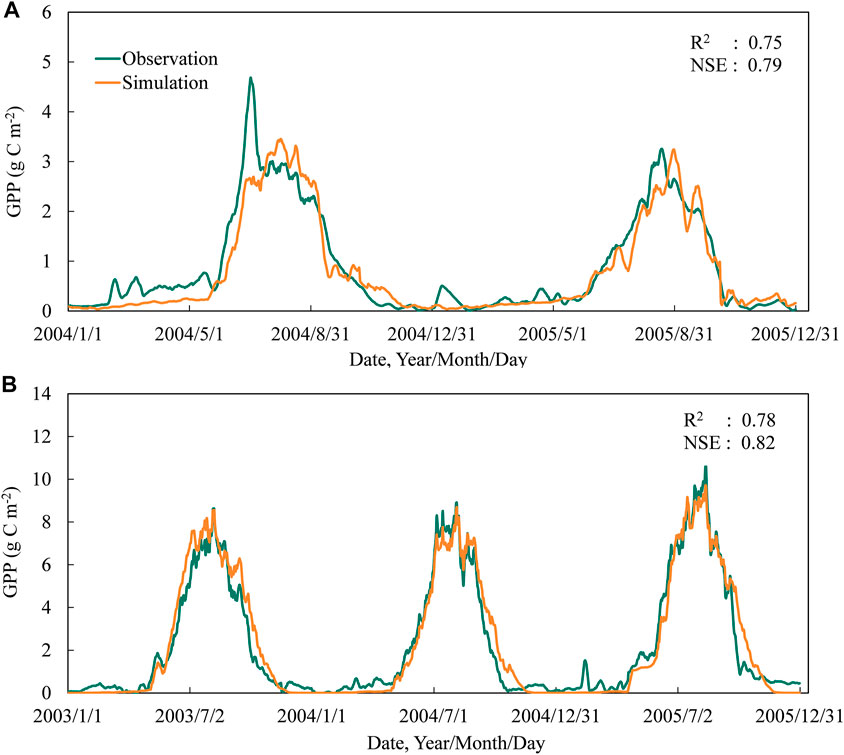
FIGURE 4. Simulated and observed gross primary product (GPP) values at Damxung (A) and Haibei (B) sites.
Spatiotemporal Patterns of NPP Over the TRHR
Figure 5A displays NPP distribution averaged over the period 1989 to 2018. The regionally averaged NPP during this study period was 237.86 g C m−2 yr−1 and ranged from 0 to 750 g C m−2 yr−1. The NPP decreased gradually from the southeast to the northwest, which is largely consistent with the temperature and precipitation distributions in the TRHR (Figures 5B,C). Similar results have been reported in previous research in the same region based on remote sensing data and the Carnegie–Ames–Stanford model (Zhang et al., 2016).
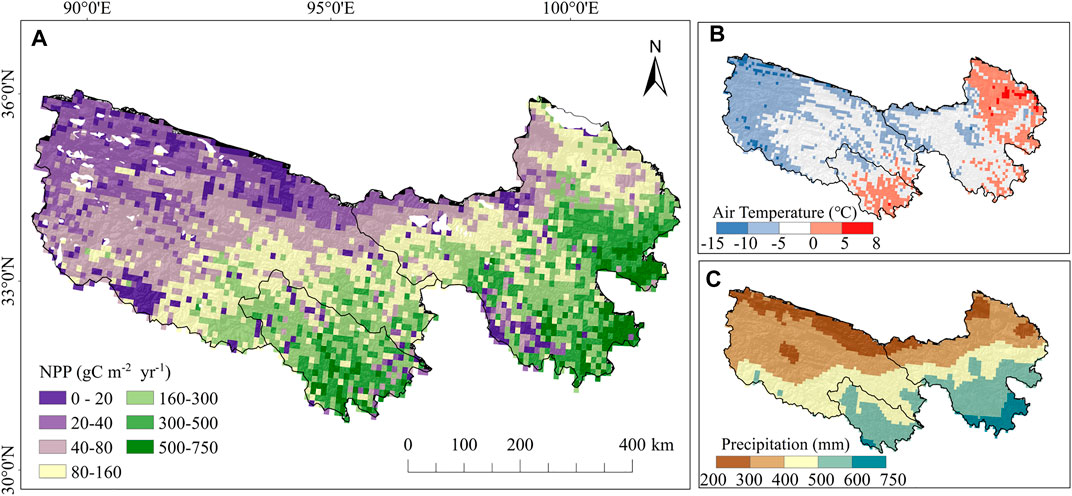
FIGURE 5. Distribution of net primary product (NPP) (A) averaged over the 1989–2018 period in the TRHR, along with spatial distributions of mean annual air temperature (B) and mean annual precipitation (C) over the same period. Lakes are excluded in (A).
Large snow mountains and deserts stretch over the Yangtze River headwaters area, and rivers and lakes are scattered throughout the region, which is characterized by a dry and cold climate with less than 300 mm in annual precipitation and a mean air temperature below −5°C. The average annual NPP in the Yangtze River headwaters area was relatively low, mostly ranging from 0–80 g C m−2 yr−1, and only in the southeast parts of its headwaters area, where meadows are widespread, reached 200 g C m−2 yr−1. In contrast, NPP values in the Lancang River headwaters area and the southeastern parts of the Yellow River headwaters area were much higher due to the favorable climate, abundant rainfall, and diverse vegetation types, and most of them exceeded 300 g C m−2 yr−1.
The simulation showed highly variable growth of NPP in the TRHR during the study period (Figure 6A), increasing from about 210 g C m−2 yr−1 in 1989 to 269 g C m−2 yr−1 in 2018 with a total growth of 59 g C m−2 yr−1. A significant increase in NPP was observed during the study period with an estimated rate of change of 1.09 g C m−2 yr−2 in response to increasing air temperature (0.06°C yr−1) and precipitation (4.08 mm yr−1).
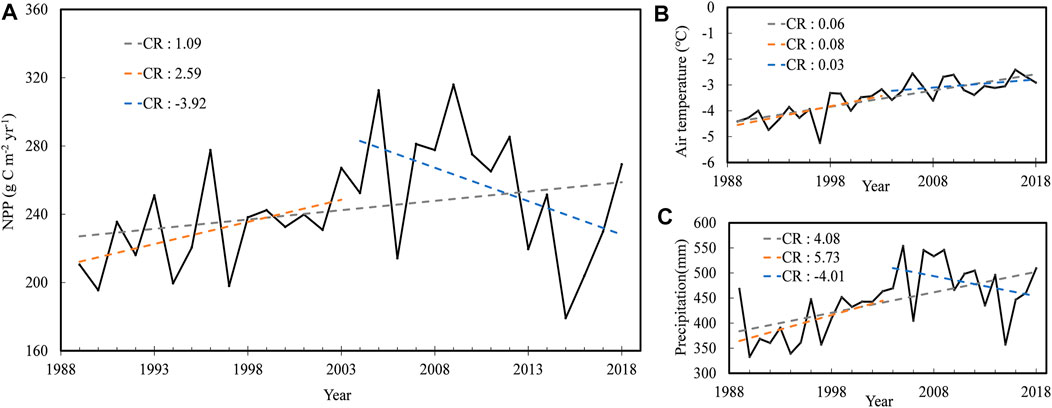
FIGURE 6. Inter-annual variations of simulated NPP (A), air temperature (B) and precipitation (C) in the TRHR from 1989 to 2018. Gray, orange and blue dashed lines represent the trend line from 1989 to 2018, 1989 to 2003 and 2004 to 2018, respectively. CR: the change rate of NPP, air temperature and precipitation in a unit of g C m−2 yr−2 (A) °C yr−1 (B) and mm yr−1 (C), respectively.
As shown in Figure 6A, the interannual variation in NPP across the region from 1981 to 2018 exhibited obvious inter-phase changes. NPP increased rapidly in the first 15 years (1989–2003), but decreased significantly in the next 15 years after 2004. The TRHR has experienced increases in temperature and precipitation over the past 30 years, reflecting a “warming and wetting” trend from 1989 to 2003 (Figures 6B,C), with an annual increase in air temperature and precipitation of 0.08°C per year and 5.73 mm per year, respectively. Increased precipitation and temperature provide water and energy availability for vegetation growth and survival. The green-up period of vegetation was advanced and the wilting period was delayed, which facilitated the accumulation of NPP. Over the period of 2004–2018, a steady increase in temperature and a concomitant decrease in precipitation were observed in the TRHR, at rates of change of 0.03°C yr−1 and −4.01 mm yr−1, respectively. Due to the continued decline in precipitation, plants do not receive enough water, which has adversely affected their photosynthesis and accumulation of NPP.
A number of previous studies have also reported the mean annual NPP in the TRHR based on different methods and data. Han et al. (2018) estimated mean annual NPP in the TRHR based on a statistical model to be 283.97 g C m−2 yr−1 from 1988 to 2012, which is slightly higher than our estimate (247.26 g C m−2 yr−1) for 1989–2012. Guo et al. (2013) even reported an extremely high estimate for 1960–2011 in the same region using a climate productivity model (Thornthwaite Memorial model), which is 570.35 g C gm−2 yr−1. In contrast, Wang et al. (2009) reported a mean annual NPP (143.17 g C m−2 yr−1) for the period of 1988–2004 using an ecological model fed with satellite-based GPP data, which is much lower than our estimate (231.72 g C m−2 yr−1 for 1989–2004). Our simulation reveals an increase-then-decrease trend of NPP in the TRHR, as also found in Zhang et al. (2016). However, their study gave an overall increasing rate of 1.31 g C m−2 yr−2 using the process-based CASA model driven by remote sensing and climate data, which was a little higher than our result (1.09 g C m−2 yr−2). The reasons for these discrepancies could be multifaceted, including the special treatment of freeze-thaw dynamics in Noah-MP that is not present in other methods.
Changes of Net Primary Productivity in Different Frozen Ground Zones
The simulation indicates a considerable reduction in permafrost extent in the TRHR during the study period, declining from about 296 × 103km2 in 1989 to about 221 × 103km2 in 2018 with a reduced total area of 75 × 103km2. The trend of decreasing permafrost area was clearly observed throughout the period, with a change rate estimated at 2.87 × 103 km2 yr−1, in parallel to the increasing in air temperature (0.06°C yr−1). Most of the thawed permafrost was converted to SFG, leading to an increase in SFG areas in the TRHR. The rate of change in SFG area was estimated to be 2.17 × 103km2 per year, which is slightly lower than the rate at which permafrost disappeared.
Spatially, permafrost has degraded extensively in the TRHR. In the central portion of the Yangtze River headwaters area and south of the Yellow River headwaters area, permafrost declined sharply during 1989–2018 (Figure 7A). Permafrost also disappeared during the period in the central portion of the Lancang River headwaters. Those areas where permafrost disappeared consistently show the highest increase in air temperature, resulting in attenuated thickness of permafrost and disappearance of some of those that were already thermally unstable. The loss of permafrost in the TRHR occurred mostly in areas along major rivers.
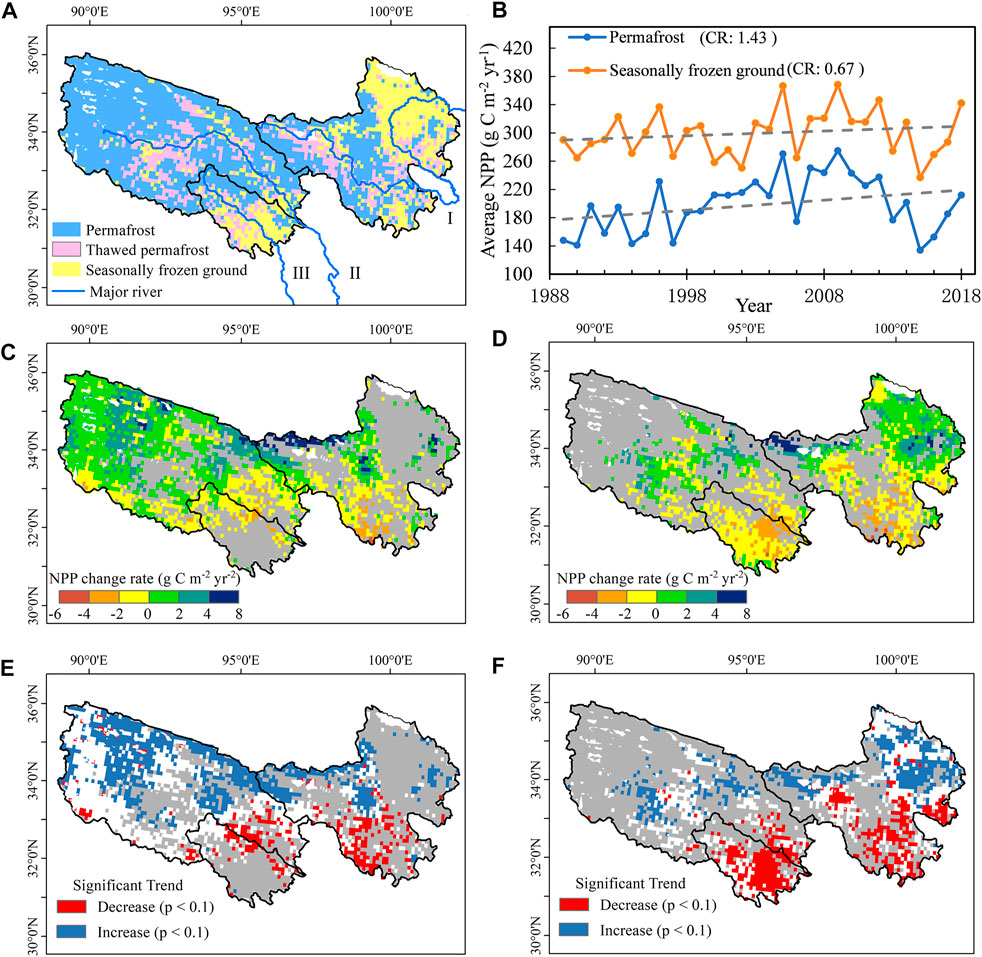
FIGURE 7. Distribution of frozen ground type in the TRHR in 2018 (A), inter-annual variability of NPP in the TRHR stratified by frozen ground type (B), and the rates of change in NPP in the permafrost zone (C) and the seasonally frozen ground (SFG) zone (D) in the TRHR from 1989 to 2018 based on a 30-year Noah-MP simulation, as well as significance tests for the trends in the permafrost zone (E) and SFG zone (F). The thawed permafrost in (A) was identified relative to the beginning of the simulation. I, II, III represent the Yellow River, the Yangtze River and Langcang River, respectively. The gray dashed lines in (B) represent the trend from 1989 to 2018. Lakes are excluded from the maps. CR: the rate of change in a unit of g C m−2 yr−2. The blank areas in (E,F) indicate a p value >0.1.
Based on the distribution of frozen ground (Figure 7A) obtained from the simulation, we estimated the inter-annual variability of NPP by stratified frozen ground type, i.e., for the permafrost zone and the SFG zone, respectively. Thawed permafrost areas were specifically identified by contrasting the occurrence of permafrost in 2018 to that in 1989. The mean annual NPP in the SFG zone was estimated as 299.7 g C m−2 yr−1, significantly higher than in the permafrost zone (198.5 g C m−2 yr−1). We found that the mean annual NPP in both the permafrost zone and the SFG zone showed substantially increasing trends during the study period in relation to climate changes (Figures 6B,C). Unlike the magnitude of the mean annual NPP, the rate of change in NPP in the permafrost zone was estimated to be 1.43 g C m−2 yr−2, which is significantly greater than the rate of change of NPP in the SFG zone (0.67 g C m−2 yr−2). A consistently remarkable reduction in NPP was observed in both the permafrost and SFG zones in 2006, echoing the largest fall in precipitation that year (Figure 6C).
The spatial patterns of NPP change rate in the permafrost zone and SFG zone were different, as shown in Figures 7B,C. In the permafrost zone, NPP decreased primarily in the southern and central TRHR and increased in the extensive northwestern TRHR. The highest growth in NPP in the permafrost zone was observed in the northwest parts of the Yellow River headwaters area and west of the Yangtze River headwaters area. The growth of NPP occurred primarily in regions that permafrost extensively distributes, while NPP decreased in the areas that permafrost sporadically distributes. In the SFG zone, the NPP decreased in the majority of the Lancang River headwaters area and the southern parts of the Yellow River headwaters area, while it mainly increased in the northeast of the Yellow River headwaters area. The sharpest decreases in NPP in the SFG area occurred in the northeast portion of the Lancang River headwaters area. In the regions where permafrost degraded to SFG, both increases and decreases in NPP were observed. Figures 7D,E shows the significance of changes in NPP in the permafrost zone and SFG zone based on the M-K trend test. It indicates that the rates of change greater than 2 g C m−2 yr−2 and most negative rates of change are significant regardless of frozen ground zone, except for rates of change with modest increases (0–2 g C m−2 yr−2), which are insignificant.
Implications for NPP Responses to Permafrost Degradation
Permafrost forms and exists in a low temperature climate. These climate conditions are mainly characterized by long, cold winters and short, relatively dry, cool summer. Plant communities in the alpine permafrost region are dominated by alpine vegetation types that includes meadow, steppe, cold desert and subnival cushion plant. Biomass in permafrost regions is low due to the combined influence of rigid climatic conditions, and NPP accumulation is also impaired. In the SFG zone, climate conditions are relatively better, there is relatively abundant precipitation and moderate temperatures, and vegetation is more diverse and abundant compared to the permafrost zone. The biomass in the SFG zone is relatively high, and NPP can increase more quickly. Therefore, the mean value of NPP in the SFG zone is much higher than that in the permafrost zone. This is consistent with the result of our simulation.
However, contrary to our general understanding, the simulation shows that the rate of change in NPP in the present permafrost zone is significantly greater than that in the SFG zone. Li et al. (2022) calculated the temporal and spatial changes of NPP on the QTP using the random forest and the Biome-BGC model and also found that the NPP change rate in permafrost regions was higher than non-permafrost regions, confirming the same finding from our simulation. Since permafrost is very sensitive to climate change, a small change in air temperature may result in considerable heat disturbance in the ground. A rapid response of permafrost to climate change has contributed to rapid growth of NPP in the permafrost zone. Once the magnitude of climate change exceeds the threshold that permafrost can withstand, permafrost will degrade. Permafrost degradation can lead to changes in vegetation distribution as well as ecosystem productivity.
Permafrost degradation exerts complicated impacts on ecosystems. In areas where permafrost degradation has occurred, both increasing and decreasing changes in NPP have been observed (Figures 7A,D). It is generally understood that permafrost degradation causes the conversion of permafrost to SFG, i.e., the conversion from lower NPP to higher NPP. An increase in NPP was observed in central parts of the Yangtze River headwaters area where permafrost has degraded (Figure 7C). Climate warming directly affects permafrost by increasing soil temperature. The increased ground temperature creates better thermal conditions for vegetation growth. The growth of plant root is no longer restricted by the low temperature of permafrost, which promotes the decomposition of organic matter and prolongs the growing season of plants, which in turn increases the productivity of the ecosystem (Euskirchen et al., 2006; Chen et al., 2012).
However, in many cases, the direction of NPP changes due to permafrost degradation is the opposite (Figure 7D). For example, in the southern parts of the Yellow River headwaters area, NPP showed a decreasing trend in the areas where permafrost degradation occurred (corresponding to the thawed permafrost areas in Figure 7A). During the degradation, seasonal melt depth increases and ground ice begins to melt. Soil moisture in the root zone is no longer retained by the frozen soil, but instead percolates and migrates downwards, ultimately leading to a drop in both the soil moisture conditions and ground water levels. Plant growth is inhibited by this reduced water availability, leading to stunted roots and subsequently reduced ecosystem productivity (Torre Jorgenson et al., 2013; Streletskiy et al., 2015).
In contrast to the Arctic and Antarctic regions, strong interactions exist between the atmosphere, cryosphere, hydrosphere, and biosphere in the TRHR (Bibi et al., 2018). Given limited knowledge of biosphere’s response to climate change, further modeling studies and long-term field observations are required to understand phenological dynamics and response to climate change. Although the Noah-MP model includes the basic carbon cycle processes, biological community succession and human activities are not included in the model. The simulation results were only a manifestation of the fundamental changes in the natural cycle. Further research needs to incorporate a range of external processes into the model to more realistically simulate NPP changes in this region.
Conclusion
Changes in NPP in the TRHR during 1989–2018, stratified by frozen ground type, were simulated using the Noah-MP LSM operating at a spatial resolution of 0.1° and a temporal resolution of 3 h. Results were validated against available meteorological and flux observations at two nearby sites. The following conclusions can be drawn:
1) The validation demonstrated that Noah-MP is able to effectively simulate the spatiotemporal variations of NPP in the TRHR. There was good agreement between the simulation and observations at two sites, with NSE and R values above 0.80 for both soil temperature and soil moisture and above 0.75 for GPP.
2) During 1989–2018, NPP in the TRHR increased at a rate of 1.09 g C m−2 yr−2. NPP increased rapidly in the first 15 years (1989–2003), but decreased significantly in the next 15 years after 2004.
3) The mean annual NPP averaged in the SFG zone (299.7 g C m−2 yr−1) was much higher than that in the permafrost zone (198.5 g C m−2 yr−1) during 1989–2018, but it was estimated that NPP in the permafrost area increase at a rate of 1.43 g C m−2 yr−2, which was much higher than the rate of change in NPP in the SFG zone (0.67 g C m−2 yr−2). In areas where permafrost degradation has occurred, both increasing and decreasing changes in NPP have been observed.
Although modeling and validation efforts have been made, long-term in-situ monitoring and comprehensive field investigations in the TRHR are still required to validate the simulation results. This study lays the foundation for further research on NPP response to permafrost changes and its feedback in the future.
Data Availability Statement
The original contributions presented in the study are included in the article/Supplementary Material, further inquiries can be directed to the corresponding author.
Author Contributions
Conceptualization, ZN and JH; Methodology, JH and ZN; Software, JH and HJ; data collection, JH and HJ; Funding acquisition, ZN; Supervision, ZN; Writing—Original Draft Preparation, JH; Writing—Review and Editing, ZN and JH.
Funding
This work was supported by the Second Tibetan Plateau Scientific Expedition and Research (STEP) program (No. 2019QZKK0905-08) and the grants of National Natural Science Foundation of China (Nos 41971074 and 42171125).
Conflict of Interest
The authors declare that the research was conducted in the absence of any commercial or financial relationships that could be construed as a potential conflict of interest.
Publisher’s Note
All claims expressed in this article are solely those of the authors and do not necessarily represent those of their affiliated organizations, or those of the publisher, the editors and the reviewers. Any product that may be evaluated in this article, or claim that may be made by its manufacturer, is not guaranteed or endorsed by the publisher.
Acknowledgments
The authors would like to thank the China FLUX network (http://www.cnern.org.cn, accessed 15 December 2021) and the National Ecosystem Science Data Center, National Science and Technology Infrastructure of China (http://www.nesdc.org.cn) for providing the data.
References
Abramowitz, G., Leuning, R., Clark, M., and Pitman, A. (2008). Evaluating the Performance of Land Surface Models. J. Clim. 21 (21), 5468–5481. doi:10.1175/2008jcli2378.1
Arsenault, K. R., Nearing, G. S., Wang, S., Yatheendradas, S., and Peters-Lidard, C. D. (2018). Parameter Sensitivity of the Noah-MP Land Surface Model with Dynamic Vegetation. J. Hydrometeorology 19 (5), 815–830. doi:10.1175/jhm-d-17-0205.1
Bibi, S., Wang, L., Li, X., Zhou, J., Chen, D., and Yao, T. (2018). Climatic and Associated Cryospheric, Biospheric, and Hydrological Changes on the Tibetan Plateau: A Review. Int. J. Climatol 38, e1–e17. doi:10.1002/joc.5411
Burke, E. J., Jones, C. D., and Koven, C. D. (2013). Estimating the Permafrost-Carbon Climate Response in the CMIP5 Climate Models Using a Simplified Approach. J. Clim. 26 (14), 4897–4909. doi:10.1175/jcli-d-12-00550.1
Chen, B., Zhang, X., Tao, J., Wu, J., Wang, J., Shi, P., et al. (2014). The Impact of Climate Change and Anthropogenic Activities on alpine Grassland over the Qinghai-Tibet Plateau. Agric. For. Meteorology 189-190, 11–18. doi:10.1016/j.agrformet.2014.01.002
Chen, F., and Dudhia, J. (2001). Coupling an Advanced Land Surface-Hydrology Model with the Penn State-NCAR MM5 Modeling System. Part I: Model Implementation and Sensitivity. Mon. Wea. Rev. 129, 569–585. doi:10.1175/1520-0493(2001)129<0569:caalsh>2.0.co;2
Chen, S., Liu, W., Qin, X., Liu, Y., Zhang, T., Chen, K., et al. (2012). Response Characteristics of Vegetation and Soil Environment to Permafrost Degradation in the Upstream Regions of the Shule River Basin. Environ. Res. Lett. 7 (4), 045406. doi:10.1088/1748-9326/7/4/045406
Chen, Y., Feng, X., Fu, B., Wu, X., and Gao, Z. (2021). Improved Global Maps of the Optimum Growth Temperature, Maximum Light Use Efficiency, and Gross Primary Production for Vegetation. J. Geophys. Res. Biogeosci 126 (4), e2020JG005651. doi:10.1029/2020jg005651
Clapp, R. B., and Hornberger, G. M. (1978). Empirical Equations for Some Soil Hydraulic Properties. Water Resour. Res. 14 (4), 601–604. doi:10.1029/WR014i004p00601
Collatz, G. J., Ball, J. T., Grivet, C., and Berry, J. A. (1991). Physiological and Environmental Regulation of Stomatal Conductance, Photosynthesisand Transpiration: A Model that Includes a Laminar Boundary Layer. Agric. For. Meteorology 52 (2-4), 107–136. doi:10.1016/0168-1923(91)90002-8
Collatz, G., Ribas-Carbo, M., and Berry, J. (1992). Coupled Photosynthesis-Stomatal Conductance Model for Leaves of C4 Plants. Funct. Plant Biol. 19 (5), 519–538. doi:10.1071/PP9920519
Dickinson, R. E., Shaikh, M., Bryant, R., and Graumlich, L. (1998). Interactive Canopies for a Climate Model. J. Clim. 11 (11), 2832–2836. doi:10.1175/1520-0442(1998)011<2823:icfacm>2.0.co;2
Ek, M. B., Mitchell, K. E., Lin, Y., Rogers, E., Grunmann, P., Koren, V., et al. (2003). Implementation of Noah Land Surface Model Advances in the National Centers for Environmental Prediction Operational Mesoscale Eta Model. J. Geophys. Res. 108 (D22), 8851–8862. doi:10.1029/2002jd003296
Euskirchen, E. S., McGuire, A. D., Kicklighter, D. W., Zhuang, Q., Clein, J. S., Dargaville, R. J., et al. (2006). Importance of Recent Shifts in Soil thermal Dynamics on Growing Season Length, Productivity, and Carbon Sequestration in Terrestrial High-Latitude Ecosystems. Glob. Change Biol. 12 (4), 731–750. doi:10.1111/j.1365-2486.2006.01113.x
Feng, A., Li, Y., Gao, J., Wu, S., and Feng, A. (2017). The Determinants of Streamflow Variability and Variation in Three-River Source of China: Climate Change or Ecological Restoration? Environ. Earth Sci. 76 (20), 696. doi:10.1007/s12665-017-7026-6
Guo, B., Zhou, Y., Zhu, J., Liu, W., Wang, F., Wang, L., et al. (2015). Spatial Patterns of Ecosystem Vulnerability Changes during 2001-2011 in the Three-River Source Region of the Qinghai-Tibetan Plateau, China. J. Arid Land 8 (1), 23–35. doi:10.1007/s40333-015-0055-7
Guo, P., Yang, D., Wang, H., and Cheng, J.-Q. (2013). Climate Change and its Effects on Climatic Productivity in the Three-River Headwaters Region in 1960–2011. Chin. J. Ecol. 32 (10), 2806–2814. (in Chinese). doi:10.132992/j.1000-4890.2013.0302
Han, Z., Song, W., Deng, X., and Xu, X. (2018). Grassland Ecosystem Responses to Climate Change and Human Activities within the Three-River Headwaters Region of China. Sci. Rep. 8 (1), 9079. doi:10.1038/s41598-018-27150-5
He, J., Yang, K., Tang, W., Lu, H., Qin, J., Chen, Y., et al. (2020). The First High-Resolution Meteorological Forcing Dataset for Land Process Studies over China. Sci. Data 7 (1), 25. doi:10.1038/s41597-020-0369-y
Jiang, C., and Zhang, L. (2015). Climate Change and its Impact on the Eco-Environment of the Three-Rivers Headwater Region on the Tibetan Plateau, China. Int. J. Environ. Res. Public Health 12 (10), 12057–12081. doi:10.3390/ijerph121012057
Jiang, C., and Zhang, L. (2016a). Ecosystem Change Assessment in the Three-River Headwater Region, China: Patterns, Causes, and Implications. Ecol. Eng. 93, 24–36. doi:10.1016/j.ecoleng.2016.05.011
Jiang, C., and Zhang, L. (2016b). Effect of Ecological Restoration and Climate Change on Ecosystems: A Case Study in the Three-Rivers Headwater Region, China. Environ. Monit. Assess. 188 (6), 382. doi:10.1007/s10661-016-5368-2
Li, C., Sun, H., Liu, L., Dou, T., Zhou, M., Li, W., et al. (2022). The Importance of Permafrost in the Steady and Fast Increase in Net Primary Production of the Grassland on the Qinghai-Tibet Plateau. Catena 211, 105964. doi:10.1016/j.catena.2021.105964
Li, X., Wu, T., Zhu, X., Jiang, Y., Hu, G., Hao, J., et al. (2020). Improving the Noah‐MP Model for Simulating Hydrothermal Regime of the Active Layer in the Permafrost Regions of the Qinghai‐Tibet Plateau. J. Geophys. Res. Atmos. 125 (16). doi:10.1029/2020jd032588
Liang, T., Yang, S., Feng, Q., Liu, B., Zhang, R., Huang, X., et al. (2016). Multi-Factor Modeling of Above-Ground Biomass in alpine Grassland: A Case Study in the Three-River Headwaters Region, China. Remote Sensing Environ. 186, 164–172. doi:10.1016/j.rse.2016.08.014
Lin, S., Wang, G., Feng, J., Dan, L., Sun, X., Hu, Z., et al. (2019). A Carbon Flux Assessment Driven by Environmental Factors over the Tibetan Plateau and Various Permafrost Regions. J. Geophys. Res. Biogeosci. 124 (5), 1132–1147. doi:10.1029/2018jg004789
Lin, X., Han, P., Zhang, W., and Wang, G. (2017). Sensitivity of alpine Grassland Carbon Balance to Interannual Variability in Climate and Atmospheric CO 2 on the Tibetan Plateau during the Last century. Glob. Planet. Change 154, 23–32. doi:10.1016/j.gloplacha.2017.05.008
Liu, X., and Chen, B. (2000). Climatic Warming in the Tibetan Plateau during Recent Decades. Int. J. Climatol. 20, 1729–1742. doi:10.1002/1097-0088(20001130)20:14<1729:aid-joc556>3.0.co;2-y
Liu, X., Zhang, J., Zhu, X., Pan, Y., Liu, Y., Zhang, D., et al. (2014). Spatiotemporal Changes in Vegetation Coverage and its Driving Factors in the Three-River Headwaters Region during 2000-2011. J. Geogr. Sci. 24 (2), 288–302. doi:10.1007/s11442-014-1088-0
Mao, T., Wang, G., and Zhang, T. (2015). Impacts of Climatic Change on Hydrological Regime in the Three-River Headwaters Region, China, 1960-2009. Water Resour. Manage. 30 (1), 115–131. doi:10.1007/s11269-015-1149-x
Melillo, J. M., McGuire, A. D., Kicklighter, D. W., Moore, B., Vorosmarty, C. J., and Schloss, A. L. (1993). Global Climate Change and Terrestrial Net Primary Production. Nature 363 (6426), 234–240. doi:10.1038/363234a0
Muller, S. W. (1943). Permafrost: or, Permanently Frozen Ground and Related Engineering Problems. Michigan: U.S. Geological Survey Representative Strategic Engineering Study.
Niu, G.-Y., Yang, Z.-L., Mitchell, K. E., Chen, F., Ek, M. B., Barlage, M., et al. (2011). The Community Noah Land Surface Model with Multiparameterization Options (Noah-MP): 1. Model Description and Evaluation with Local-Scale Measurements. J. Geophys. Res. 116 (D12), D12109. doi:10.1029/2010jd015139
Osterkamp, T. (2005). The Recent Warming of Permafrost in Alaska. Glob. Planet. Change 49 (3-4), 187–202. doi:10.1016/j.gloplacha.2005.09.001
Qin, D., and Ding, Y. (2010). Key Issues on Cryospheric Changes, Trends and Their Impacts. Adv. Clim. Change Res. 1 (1), 1–10. doi:10.3724/sp.J.1248.2010.00001
Ruddell, B. L., Yu, R., Kang, M., and Childers, D. L. (2015). Seasonally Varied Controls of Climate and Phenophase on Terrestrial Carbon Dynamics: Modeling Eco-Climate System State Using Dynamical Process Networks. Landscape Ecol. 31 (1), 165–180. doi:10.1007/s10980-015-0253-x
Schuur, E. A. G., and Abbott, B. (2011). High Risk of Permafrost Thaw. Nature 480, 32–33. doi:10.1038/480032a
Sen, P. K. (1968). Estimates of the Regression Coefficient Based on Kendall's Tau. J. Am. Stat. Assoc. 63, 1379–1389. doi:10.1080/01621459.1968.10480934
Shen, Z.-X., Li, Y.-L., and Fu, G. (2015). Response of Soil Respiration to Short-Term Experimental Warming and Precipitation Pulses over the Growing Season in an alpine Meadow on the Northern Tibet. Appl. Soil Ecol. 90, 35–40. doi:10.1016/j.apsoil.2015.01.015
Shi, H., Li, T., and Wei, J. (2017). Evaluation of the Gridded CRU TS Precipitation Dataset with the point Raingauge Records over the Three-River Headwaters Region. J. Hydrol. 548, 322–332. doi:10.1016/j.jhydrol.2017.03.017
Smith, S. L., Burgess, M. M., Riseborough, D., and Mark Nixon, F. (2005). Recent Trends from Canadian Permafrost thermal Monitoring Network Sites. Permafrost Periglac. Process. 16 (1), 19–30. doi:10.1002/ppp.511
Streletskiy, D. A., Tananaev, N. I., Opel, T., Shiklomanov, N. I., Nyland, K. E., Streletskaya, I. D., et al. (2015). Permafrost Hydrology in Changing Climatic Conditions: Seasonal Variability of Stable Isotope Composition in Rivers in Discontinuous Permafrost. Environ. Res. Lett. 10 (9), 095003. doi:10.1088/1748-9326/10/9/095003
Tong, L., Xu, X., Fu, Y., and Li, S. (2014). Wetland Changes and Their Responses to Climate Change in the "Three-River Headwaters" Region of China since the 1990s. Energies 7 (4), 2515–2534. doi:10.3390/en7042515
Torre Jorgenson, M., Harden, J., Kanevskiy, M., O’Donnell, J., Wickland, K., Ewing, S., et al. (2013). Reorganization of Vegetation, Hydrology and Soil Carbon after Permafrost Degradation across Heterogeneous Boreal Landscapes. Environ. Res. Lett. 8 (3), 035017. doi:10.1088/1748-9326/8/3/035017
Trenberth, K. E., Dai, A., van der Schrier, G., Jones, P. D., Barichivich, J., Briffa, K. R., et al. (2013). Global Warming and Changes in Drought. Nat. Clim Change 4 (1), 17–22. doi:10.1038/nclimate2067
Wang, J., Liu, J., Shao, Q., Liu, R-G., Fan, J-W., and Chen, Z-Q. (2009). Spatial-temporal Patterns of Net Primary Productivity for 1988–2004 Based on GLOPEM-CEVSA Model in the “Three-River Headwaters” Region of Qinghai Province, China. Chin. J. Plant Ecol. 33 (2), 254–269. (in Chinese). doi:10.3773/j.issn.1005-264x.2009.02.003
Wu, Q., and Zhang, T. (2008). Recent Permafrost Warming on the Qinghai-Tibetan Plateau. J. Geophys. Res. 113, D13108. doi:10.1029/2007jd009539
Wu, X., and Nan, Z. (2016). “A Multilayer Soil Texture Dataset for Permafrost Modeling over Qinghai– Tibetan Plateau,” in IEEE International Geoscience and Remote Sensing Symposium, Beijing, China, 10-15 July 2016, 4917–4920.
Yang, Q., Dan, L., Lv, M., Wu, J., Li, W., and Dong, W. (2021). Quantitative Assessment of the Parameterization Sensitivity of the Noah-MP Land Surface Model with Dynamic Vegetation Using ChinaFLUX Data. Agric. For. Meteorology 307, 108542. doi:10.1016/j.agrformet.2021.108542
Yi, X., Li, G., and Yin, Y. (2012). Temperature Variation and Abrupt Change Analysis in the Three-River Headwaters Region during 1961-2010. J. Geogr. Sci. 22 (3), 451–469. doi:10.1007/s11442-012-0939-9
Zhang, Y., Zhang, C., Wang, Z., Chen, Y., Gang, C., An, R., et al. (2016). Vegetation Dynamics and its Driving Forces from Climate Change and Human Activities in the Three-River Source Region, China from 1982 to 2012. Sci. Total Environ. 563-564, 210–220. doi:10.1016/j.scitotenv.2016.03.223
Zhao, D., Wu, S., and Yin, Y. (2013). Dynamic Responses of Soil Organic Carbon to Climate Change in the Three-River Headwater Region of the Tibetan Plateau. Clim. Res. 56 (1), 21–32. doi:10.3354/cr01141
Zhao, L., Ping, C.-L., Yang, D., Cheng, G., Ding, Y., and Liu, S. (2004). Changes of Climate and Seasonally Frozen Ground over the Past 30 Years in Qinghai-Xizang (Tibetan) Plateau, China. Glob. Planet. Change 43 (1-2), 19–31. doi:10.1016/j.gloplacha.2004.02.003
Zhao, M., and Running, S. W. (2010). Drought-Induced Reduction in Global Terrestrial Net Primary Production from 2000 through 2009. Science 329 (5994), 940–943. doi:10.1126/science.1192666
Keywords: net primary productivity (NPP), NOAH-MP, permafrost, seasonally frozen ground, three-river headwaters region
Citation: Hu J, Nan Z and Ji H (2022) Spatiotemporal Characteristics of NPP Changes in Frozen Ground Areas of the Three-River Headwaters Region, China: A Regional Modeling Perspective. Front. Earth Sci. 10:838558. doi: 10.3389/feart.2022.838558
Received: 17 December 2021; Accepted: 27 January 2022;
Published: 14 February 2022.
Edited by:
Dongliang Luo, Northwest Institute of Eco-Environment and Resources (CAS), ChinaReviewed by:
Lihui Luo, Northwest Institute of Eco-Environment and Resources (CAS), ChinaDehua Mao, Northeast Institute of Geography and Agroecology (CAS), China
Copyright © 2022 Hu, Nan and Ji. This is an open-access article distributed under the terms of the Creative Commons Attribution License (CC BY). The use, distribution or reproduction in other forums is permitted, provided the original author(s) and the copyright owner(s) are credited and that the original publication in this journal is cited, in accordance with accepted academic practice. No use, distribution or reproduction is permitted which does not comply with these terms.
*Correspondence: Zhuotong Nan, bmFuenRAbmpudS5lZHUuY24=