- 1Zoological Institute, Technische Universität Braunschweig, Braunschweig, Germany
- 2Institute of Ecology and Earth Sciences, University of Tartu, Tartu, Estonia
- 3Institute of Geosystems and Bioindication, Technische Universität Braunschweig, Braunschweig, Germany
- 4Faculty of Geosciences and Environmental Engineering, Southwest Jiaotong University, Chengdu, China
- 5State Key Laboratory of Tibetan Plateau Earth System, Resources and Environment (TPESRE), Institute of Tibetan Plateau Research, Chinese Academy of Sciences, Beijing, China
- 6CAS Center for Excellence in Tibetan Plateau Earth Sciences, Chinese Academy of Sciences, Beijing, China
- 7Center for the Pan-Third Pole Environment, Lanzhou University, Lanzhou, China
- 8Department of Evolutionary Genetics, Max Planck Institute for Evolutionary Biology, Plön, Germany
- 9Institute of Technology, University of Tartu, Tartu, Estonia
- 10Key Laboratory of Cenozoic Geology & Environment, Institute of Geology and Geophysics, Chinese Academy of Sciences, Beijing, China
- 11CAS Center for Excellence in Life and Paleoenvironment, Beijing, China
- 12Key Laboratory of Tibetan Environment Changes and Land Surface Processes, Institute of Tibetan Plateau Research, Chinese Academy of Sciences, Beijing, China
Lake sediments represent valuable and widely used archives for tracking environmental and biotic changes over time. Past aquatic communities are traditionally studied via morphological identification of the remains of organisms. However, molecular identification tools, such as DNA metabarcoding, have revolutionized the field of biomonitoring by enabling high-throughput and fast identification of organisms from environmental samples (e.g., sediments and soil). Sedimentary ancient DNA (sedaDNA) metabarcoding, an approach to track the biodiversity of target organisms from sediment cores, spanning thousands of years, has been successfully applied in many studies. However, researchers seldom explore how well the signals from sedaDNA data correlate with the fossil records of target organisms. This information is essential to infer past environmental conditions and community changes of bioindicators when the increasingly popular molecular identification method, metabarcoding, is desired instead of a morphological identification approach. In this study, we explore the correlations of diatom valve records across the last ∼940 years with the diatom sedaDNA metabarcoding data from the same sediment core from lake Nam Co (Tibetan Plateau). Overall, the results from valve vs. sedaDNA data revealed concordant diatom richness as well as community patterns. However, several mismatches in the diatom taxonomic composition existed between the data sets. In general, sedaDNA data harbored much higher diatom diversity, but due to the lack of reference sequences in public databases, many molecular units (amplicon sequence variants) remained unclassified to lower taxonomic levels. As our study lake, Nam Co, is characterized by brackish water and alkaline pH, some likely cases for the observed taxonomic composition mismatches may be due to a valve dissolution issue. Nevertheless, significant drivers for the diatom richness and community structure largely corresponded between data sets. Both valve and sedaDNA data demonstrated similar breakpoints for historical diatom community shifts. A particularly strong shift in the diatom community structure occurred after ∼1950 CE, which may be associated with abrupt environmental changes on the Tibetan Plateau. Altogether, our study indicates that environmentally driven signals reflected by the diatom communities are successfully recovered via microfossil as well as molecular identification methods.
Introduction
Various pieces of information hidden in lake sediments are widely used to infer past environmental conditions over long time periods (Capo et al., 2021). Some of the most important clues about the past environment may be obtained through studying community structures of bioindicator organisms. These groups of organisms respond quickly to the changes in their environment, thus indicating the environmental condition of their habitat. Among the most widely used environmental indicators are diatoms (Bacillariophyta) because of their sensitivity to a variety of ecological conditions and their high abundance in aquatic ecosystems with usually well-preserved valves in the sediments (Reid et al., 1995; Smol and Douglas, 1996; Lobo et al., 2016).
The investigation of diatoms in the sediments has largely relied on morphological identifications of their remains. The integrity of diatom records in the sediments is a main concern if the fossil assemblages are used to infer paleo-environmental conditions (Flower and Ryves, 2009). After the completion of the diatom’s life cycle, the remaining valves are subject to a variety of taphonomic processes that may modify the fossil archive in the sediments. Some of the important factors affecting diatom preservation are pH and salinity of the environment, as well as sedimentation rate (Flower, 1993; Ryves et al., 2006). One of the hot spots for paleo-environmental studies—besides polar regions—is the Tibetan Plateau, which harbors many lakes that are characterized by saline, high pH waters (Mianping, 1997). Although the increased salinity and pH potentially favors diatom valve dissolution in the lake sediments (Flower, 1993; Ryves et al., 2001; Ryves et al., 2006), several studies have used diatom fossil assemblages from Tibetan lake sediment cores to infer paleo-environmental conditions (e.g., Wang et al., 2011; Kasper et al., 2013; Laug et al., 2021). Diatom dissolution indices (such as mentioned in Flower and Ryves, 2009) have rarely been calculated to evaluate the integrity of diatom fossil records from Tibetan lake sediment cores. However, the dissolution effect of diatom valves seems to be evident as, for example, from Taro Co, Laug et al. (2021) reported completely dissolved or very low abundance of diatom records beyond core depth 110 cm (>3,600 cal. year BP) with the increasing diatom concentrations towards the upper core layers. From more saline lakes, Chen Co and Nam Co, Wang et al. (2011) noted that the deeper sediment layers, already beyond ∼20 cm, were affected by diatom dissolution. Therefore, deeper core layers were discarded from the analyses and the temporal scale of the latter study could focus only on the last two centuries. Similarly, working with the sediment core from Nam Co, Kasper et al. (2013) reported poor preservation of diatom valves, already starting from the 5th cm of the core. Therefore, the preservation of diatom valves may largely shape the conclusions made based only on their fossil records (Flower and Ryves, 2009; Smol and Stoermer, 2010).
With the rapid development of high-throughput DNA sequencing technologies, since the beginning of the 21st century, there has been increasing interest in DNA-based methods in paleolimnology (Domaizon et al., 2017; Capo et al., 2021). These methods have made it possible to trace the historical changes of even non-fossilized organisms (Li et al., 2016; Stivrins et al., 2018; Liu et al., 2021; Talas et al., 2021). Studies comparing the recovered diatom community compositions from the top lake sediment layers have reported highly correlating results between morphological and DNA-based identification (i.e., metabarcoding) data sets (Dulias et al., 2017; Piredda et al., 2017; Stoof-Leichsenring et al., 2020; Kang et al., 2021). This suggests that sedimentary ancient DNA (sedaDNA) analyses could serve as a complementary tool to investigate the past diatom community structures from the sediment cores when the fossil preservation could be an issue. However, the molecular methods applied to modern sediments (top sediment layer) cannot be straightforwardly used for analyzing older sediment layers to capture informative DNA fragments from sedimentary ancient DNA (sedaDNA). The DNA in older sediment deposits is often too fragmented to amplify >300 bp regions of the rbcL gene, a standard genetic marker in diatom metabarcoding studies (Vasselon et al., 2017; Kelly et al., 2020). Therefore, researchers have developed DNA primers to amplify short (<100 bp), taxonomically informative fragments of the rbcL gene to track the diatom community changes in sediment cores (Stoof-Leichsenring et al., 2012). That first diatom sedaDNA study by Stoof-Leichsenring et al. (2012) also demonstrated that the results from morphological and DNA-based diatom identification methods were well correlated. Subsequent studies applied the same DNA markers for diatom sedaDNA analyses and reported results concordant with those from microscopic analyses of diatom valves (Epp et al., 2015; Stoof-Leichsenring et al., 2020), as well as the potential of DNA-based methods to reveal higher taxonomic resolution (Stoof-Leichsenring et al., 2014; Stoof-Leichsenring et al., 2020).
Previous studies comparing the morphology and DNA-based methods for diatom identifications have worked with sediment cores from freshwater systems where the preservation of valves as well as DNA is expected to be favorable. A recent study about plant DNA preservation in the sediments suggested that lakes with pH 7–9 and conductivities with 100–500 μS/cm may have better preservation of sedimentary DNA compared to lakes with higher or lower values of latter measures (Jia et al., 2021). However, sedimentary DNA studies to identify diatom (Kang et al., 2021) and ostracod (Echeverría-Galindo et al., 2021) communities indicated the potential of DNA metabarcoding in the environmental settings of the lake Nam Co with conductivity >1,400 μS/cm and pH ∼9. Although the overall diatom community patterns matched well between morphological and DNA-based identification methods in the study by Kang et al. (2021), it also indicated that the diatom species with fragile valves may be missed via microscopical analyses due to the dissolution effect. Here, we aim to further investigate the consistency of diatom communities identified via microscopy and DNA analyses by examining a sediment core from a brackish, high pH Tibetan lake, Nam Co.
Methods
Sampling
On September 1, 2019, a 107-cm-long sediment core was taken from the eastern part of lake Nam Co (30.812°N 90.992°E, altitude 4623 m asl; Figure 1) at a water depth of 27 m with a UWITEC piston corer. Nam Co is a brackish lake (2 g/L) with an average conductivity of 1447 μS/cm and pH of 9.1 (measured in September 2019). After retrieving, the sediment core was stored in the dark at ∼10°C for a few days. The core was cut lengthwise at the Lhasa campus of the Institute of Tibetan Plateau Research (ITP), Chinese Academy of Sciences (CAS). The split core was transported to the laboratory of the Beijing campus of the ITP and stored at 4°C for subsequent analyses (beginning of October 2019).
Geochemical Analysis and Dating
One-half of the core was subjected to x-ray fluorescence (XRF) analyses with the ITRAX XRF core scanner at ITP, Beijing. The scanner was equipped with a molybdenum (Mo) tube as an energy source, with a voltage of 30 kV, a current of 50 mA, and an exposure time of 10 s. Elements, such as silicon (Si), sulfur (S), potassium (K), calcium (Ca), titanium (Ti), chromium (Cr), manganese (Mn), iron (Fe), nickel (Ni), zinc (Zn), rubidium (Rb), strontium (Sr), and zirconium (Zr) were analyzed as relative abundance at a 0.1-mm resolution. The scanning results are presented as counts per second (cps; Supplementary Table S1). Total organic carbon was determined by loss on ignition (LOI%) measured at the ISE-CNR Institute of Ecosystem Study, Italy (Heiri et al., 2001). After XRF scanning, the same core half was sub-sectioned at 0.5-cm intervals and subjected to freeze-drying. A total of nine sub-sections with bulk organic material (Supplementary Table S2) were dated by accelerator mass spectrometry radiocarbon dating (14C-AMS) at Beta Analytic Inc., Miami, United States. Samples from the upper 20 cm were subjected to 210Pb and 137Cs measurements with a gamma spectrometer (GCW3023, Canberra-Meriden, Meriden, Connecticut, United States) at ITP-CAS for detecting the year 1950—a representative year of standard radiocarbon activities before the influence of nuclear weapon tests (van der Plicht and Hogg, 2006). All ages were calibrated to 2 sigma weighted mean ages using the IntCal 20 (Northern Hemisphere) data set (Reimer et al., 2020). The calibrated age-depth model was built using the Bacon’s R algorithm (Blaauw and Christen, 2011). According to the age model (Supplementary Figure S1), the core depth of 9.5 cm corresponds to year 1950 CE. All ages are expressed in calendar years of the Common Era (CE), representing the weighted mean age of the core layer (Supplementary Table S2). The samples from this core half were also used for morphological identification of diatom valves.
Morphological Identification of Diatom Valves
Morphological identification of diatom valves followed the procedures specified in Kang et al. (2021). Specifically, 0.1 g of freeze-dried sediments per sample was treated with 10% hydrochloric acid (HCl) and 30% hydrogen peroxide (H2O2) in tubes placed in a water bath at 80°C for 2–3 h to remove carbonates and organic matter. After fixing the samples to microscope slides, at least 400 valves were identified using a light optical microscope (Leica DM6B, magnification ×1,000). Additionally, scanning electron microscope images were taken using a Zeiss MERLIN instrument to aid with the identification. The morphological identification of diatoms was mainly based on general floras, such as Lange-Bertalot et al. (2017), the Diatoms of North America website (https://diatoms.org/), and Morales and Edlund (2003) for fragilarioid species. A total of 49 samples (up to 51 cm core depth) were examined. The sample layers from the top of the core (0–13 cm depth) were examined with 0.5-cm resolution, and all others were examined with 1-cm resolution (Supplementary Table S3). A total of 135 diatom morphospecies were identified from those samples (Supplementary Table S3).
DNA Extraction
The second half of the core was dedicated to sedaDNA analyses. Samples for DNA extractions were taken after every centimeter along the core with a spatula (head size of 0.45 cm) by first removing the top ca. 5-mm layer with a separate spatula (head size of 1 cm). Spatulas were sterilized in 5% bleach, followed by washing in ethanol and removing the remaining ethanol over the flames of the spirit burner after each sample processing. Two replicate samples per 1-cm layer were taken for DNA extraction. Each sample consisted of ∼0.5 g of sediments (wet weight). Uncapped PowerBead Tubes under a clean bench, where the DNA extraction work was simultaneously performed, were used as negative controls for DNA extraction (total = 6 negative DNA extraction controls). DNA was extracted using the DNeasy PowerSoil Kit (Qiagen, Germany). The first step of DNA extraction included incubating samples in PowerBead Tubes with 60 μl of solution C1 (PowerSoil Kit solution), 4 μl of proteinase K, and 25 μl of dithiothreitol (DTT) for about 10 h at 56°C. After incubation, samples were processed using a FastPrep-24 homogenizer for 40 s. The final DNA elution step was performed twice by first adding 35 μl of elution buffer to the center of the spin filter membrane, incubating samples at room temperature for 3 min followed by centrifuging at 10,400 rpm for 30 s; then, this step was repeated (total volume of 70 μl of elution buffer passed through a filter membrane per sample). Other steps followed the manufacturer’s instructions. Two replicate DNA extractions per sample were pooled and DNA concentration was measured using Qubit dsDNA High-Sensitivity Assay Kit (Invitrogen). Samples were stored at −80°C until further processing. DNA extraction was performed under a dedicated clean bench that was cleaned with 5% bleach and UV sterilized before and after work.
Primers for Polymerase Chain Reaction
Primers to amplify short rbcL gene fragments for diatom identification purposes from sedaDNA samples have been designed in an earlier study by Stoof-Leichsenring et al. (2012). To check the potential suitability of these primers on our study system (lake Nam Co), the in silico amplification success of them against the diatom amplicon sequence variants (ASVs) data set from lake Nam Co (Kang et al., 2021) and against a specialized reference sequence database for diatoms [R-syst v7.2 (Rimet et al., 2016)] was tested. For that, PrimerMiner v0.21 (Elbrecht and Leese, 2017) with default primer scoring parameters was used. To generate a primer evaluation database for PrimerMiner, diatom ASVs from Kang et al. (2021) were clustered with a 97% sequence similarity threshold [vsearch cluster_fast (Rognes et al., 2016)]. Sequences from the R-syst database were dereplicated [uniqe.seqs using mothur (Schloss et al., 2009)] and reads lacking either forward or reverse primer binding sites (target ∼80 bp) were excluded (Supplementary Table S4). Both databases were aligned with MAFFT v7 (Katoh et al., 2019) using default settings. Primers from Stoof-Leichsenring et al. (2012) demonstrated in silico amplification success only up to 75.1% and 56.4% of reads from diatom ASVs (Kang et al., 2021) and R-syst database, respectively (Supplementary File S1); therefore, these primers were modified to potentially amplify a wider range of diatoms. Primer modifications were manually made based on the above specified database alignments, visualized in Seaview (Gouy et al., 2010). Best-performing modified primers were Diat_rbcL_709F (5′-GGT GAA RYT AAA GGT TCW TAC TTD AA-3′) and Diat_rbcL_808Rd (3′-GTR TAA CCY AWW ACT AAR TCR ATC AT-5′) that demonstrated in silico amplification success of 99.2% and 98.7% of reads from diatom ASVs (Kang et al., 2021) and R-syst database, respectively (details in Supplementary File S1). Bold characters in the primer sequences denote bases modified in comparison with Stoof-Leichsenring et al. (2012) primers Diat_rbcL_708F and Diat_rbcL-808R.
Amplification and Sequencing
Sample preparations for PCR were performed under a dedicated clean bench that was cleaned with 5% bleach and UV sterilized before and after work. Short, 73-bp rbcL fragments (excluding primers) from the sediment core samples were amplified using herein designed primers Diat_rbcL_709F (5′-GGT GAA RYT AAA GGT TCW TAC TTD AA-3′) and Diat_rbcL_808Rd (3′-GTR TAA CCY AWW ACT AAR TCR ATC AT-5’; Supplementary File S1). Primers were indexed with 8-bp unique identifiers and 0- to 4-bp heterogeneity spacers. Combinatorial indexing was used where several unused combinations served as tag-switching controls (Taberlet et al., 2018). Total volume of 50 µl of PCR mix per sample consisted of 10 µl of Hot Start FirePol Master Mix (Solis BioDyne, Estonia), 1 μl of forward and reverse primer (10 μM), and a template DNA concentration of 4 ng/ml. The rest of the volume was filled with nuclease-free water. Samples without any template DNA were used as negative controls for PCR (total = 7 negative PCR controls). PCR conditions included initial activation at 95°C for 15 min followed by 50 cycles at 98°C for 20 s, 54°C for 45 s, 72°C for 1 min, and final extension at 72°C for 5 min. Two uniquely indexed replicate PCRs were made per sample and their success checked during gel electrophoresis by pipetting 5 μl of PCR product on 1% agarose gel. All PCR products were purified using the MinElute PCR Purification Kit (Qiagen, Germany), following the manufacturer’s protocol. Samples were pooled in equimolar concentrations for sequencing. Sequencing was performed with an Illumina NextSeq 550 System, using the high-output kit to generate 2 × 150 bp paired-end reads. Raw sequencing data have been deposited in the Sequence Read Archive (SRA) under BioProject PRJNA781478.
Sequencing Data Processing
Raw paired-end sequences were processed using the PipeCraft (Anslan et al., 2017), version 2.0 (release 0.1.0; https://pipecraft2-manual.readthedocs.io/en/stable/), which incorporates the following tools in a graphical user interface platform. Since the sequence orientation in raw data is mixed (5′-3′ and 3′-5′ oriented reads), the paired-end reads were first reoriented to 5′-3′ based on PCR primer strings (by allowing 2 mismatches). Primers were then clipped from the reoriented amplicons using cutadapt v3.2 (Martin, 2011). Amplicon sequence variants (ASVs) were generated using the DADA2 pipeline (Callahan et al., 2016) with quality filtering options of maxN = 0, maxEE = 2, truncQ = 2 and minLen = 45. Putative chimeras were removed with the “consensus” option in the DADA2 pipeline. The blastn algorithm (Camacho et al., 2009) was used to assign taxonomy to the ASVs using diatom specific R-syst v7.2 (Rimet et al., 2016) and additionally general EMBL v143 (Kanz et al., 2005) as reference databases.
ASV Table Curation
Potential tag-switching errors were corrected using the unused index combination controls (at PCR step) based on the relative abundances of sequences in these control samples (Taberlet et al., 2018). Specifically, if a relative sequence abundance in the ASV table was lower than 8E-05, then this low occurrence ASV record in a sample was considered as an artifact and therefore discarded. This procedure removed a total of 0.035% of sequences. After this correction, no sequences remained in the negative controls (DNA extraction and PCR negative controls), indicating no obvious contamination. Furthermore, to exclude potentially non-target taxa (non-diatom ASVs), only ASVs that demonstrated a blastn match coverage and identity of ≥90% against a reference diatom sequence were considered as diatom ASVs and retained in the data set. This procedure removed 86 ASVs and kept 360 diatom ASVs. The total proportion of sequences from non-diatom ASVs accounted for 10.1%. Three most abundant non-diatom ASVs were identified as unicellular eukaryotic algae from Eustigmatales (likely Nannochloropsis sp.) that accounted for 94.4% of reads among all non-diatom ASVs. PCR and sequencing replicates per sample were pooled (because replicates demonstrated significantly high correlations, Mantel R = 0.776, p < 0.001) and the data set was rarified to an equal depth of 30,979 sequences per sample using vegan v2.5.7 (Oksanen et al., 2020) package in R (R-Core-Team, 2021). Rarefaction curves are presented in Supplementary Figure S1. The rarefied sedaDNA data set corresponding to the sediment core layers (samples) where diatom valves were morphologically identified (36 samples from the sediment core up to 51 cm depth) consisted of 357 diatom ASVs.
Taxonomy curation of the diatom ASVs for genus and family levels followed the blastn identity percentage thresholds of ≥98% and 96%–98% against a reference sequence in the databases, respectively. Other diatom ASVs with identity percentage thresholds of 90%–94% and 94%–96% remained to phylum and class level, respectively. Higher-level taxonomic (above genus) classifications of the diatom genera followed the classifications in AlgaeBase (Guiry et al., 2014). Final sedaDNA diatom ASV table used for the analyses is presented in Supplementary Table S5.
Statistics
SedaDNA samples were taken by sampling the core layers in 1-cm intervals (1 cm resolution), but for the morphological identification, the top 13 cm of the core was examined with 0.5 cm resolution (i.e., 2 samples examined, and 800 valves identified per 1-cm layer). From the following layers, 400 valves were identified per sample (Supplementary Table S3). To form a 1-cm-resolution diatom valve data set, 0.5-cm-resolution samples were merged and then subjected to repeated sampling procedure (rarefaction) to a depth of 400 valve counts per sample using the “phyloseq” v1.34.0 (McMurdie and Holmes, 2013) package in R (rarefaction curves in Supplementary Figure S2). The rarefied valve data set [400 valves per 1-cm core layer (i.e., sample); 103 diatom morphospecies; Supplementary Table S3] and sedaDNA data set (30,979 reads per sample; 357 diatom ASVs; Supplementary Table S5) were subjected to the following statistical analyses (36 corresponding samples from both data sets).
Distance-based redundancy analysis (dbRDA) was conducted in R using the “vegan” v2.5.7 package. Environmental variables included log-transformed data from XRF scanning, Ti, Ca, Ni, Ca, and Zr/Rb ratio as grain size proxy and Sr/Ca ratio as salinity proxy. The latter variables represent a set of pre-selected non-highly correlating variables as based on the Spearman correlation test. Variable correlation plot was conducted using “corrplot” v0.88 (method = “spear”; Wei et al., 2017; Supplementary Figure S2). Additionally, LOI% (log-transformed) and reconstructed temperature data were used as predictor variables in the analyses. The temperature data between 1000 and 1980 CE included the reconstructed Northern Hemisphere (NH) mean surface temperature anomaly from Mann and Jones (2003). Because of the relatively short time regional temperature data recordings, the global mean temperature change after 1980 was integrated with NH mean surface temperature anomaly using linear interpolation in order to extend the time series for the whole time period of the sediment core (Collins et al., 2013). Temperature anomaly data per sediment sample correspond to the top of the layer age (Supplementary Table S1).
The correlation between diatom valves and sedaDNA dbRDA ordinations was assessed with Procrustes analysis (with 9999 permutations) using the “vegan” package in R. Environmental vectors were fitted onto ordinations using envfit function (with 9,999 permutations) as implemented in the “vegan” package. Distance-based linear models (DistLM; 9,999 permutations, AICc selection criterion) with backward selection procedure were used (in PRIMER v6; Clarke and Gorley, 2006) to detect environmental variables that best explain the variations in diatom community structures. Constrained hierarchical clustering was performed using the rioja v0.9-26 package in R (method = “coniss”; Juggins, 2020). Additionally, multivariate regression tree (MRT) analysis was used [mvpart v1.6-2 package (De'ath, 2014) in R] to identify major breakpoints in the diatom community time series [1,000 cross-validated trees, selection of tree with one standard error (1se) cross-validation]. Analysis of Similarities (ANOSIM as implemented in the “vegan” package; 9,999 permutations) was used to test if diatom communities are significantly different between samples grouped by MRT breaking points. SIMPER analysis (in PRIMER v6) was used to detect which morphospecies/ASVs have the greatest impact on the dissimilarity between groups detected by MRT. Bray–Curtis distance matrices of Hellinger-transformed data served as inputs for all community analyses.
Multiple linear regressions (MLR) with forward stepwise model building were performed for detecting the drivers in diatom richness in both data sets, valves and sedaDNA, using STATISTICA software v7 (StatSoft I, 2004). Dependent variables for the diatom valve data set included log-transformed effective number of morphospecies (valve data) and ASVs (sedaDNA data). Effective numbers of morphospecies/ASVs (Hill numbers) were calculated following guidelines by Alberdi and Gilbert (2019) setting parameter q to 1 (morphospecies/ASVs are weighted by their frequency, without disproportionately favoring either rare or abundant ones). Hill numbers may limit the impact of potentially erroneous ASVs and allow adjusting the weight of common and rare molecular units; thus, they are suggested to be used as biodiversity measures for environmental DNA data (Chen and Ficetola, 2020). Environmental variables for MLR were the same as noted above.
Results
Diatom Community Correlations Between Valves and SedaDNA Data
The comparison of consistency in recovered diatom communities with valves and sedaDNA data using Procrustes test revealed a significant correlation between the community structures (dbRDA ordinations; Procrustes correlation = 0.717, p < 0.001; Figure 2). For the valve data set, the model chosen by the DistLM analyses identified temperature, grain size proxy (Zr/Rb), Ti, and LOI (order of importance; Supplementary Table S6) as the most important variables explaining the variation in diatom community composition (model R2 = 0.480). For the corresponding samples in sedaDNA data, the DistLM model included temperature, grain size proxy (Zr/Rb), and Ni (R2 = 0.276). Constrained hierarchical clustering (CONISS) revealed highly correlating discontinuities of diatom communities between valves and sedaDNA data sets (Figure 3). Multivariate regression trees (MRT) separated diatom communities into two groups (based on temperature data) by indicating abrupt diatom community changes after ∼1950 CE in both data sets (cross-validation error = 0.817 and 1.02 for valves and sedaDNA, respectively). ANOSIM analyses based on the MRT grouping revealed significant differences between those two groups in both data sets (ANOSIM R = 0.823, p < 0.001 and R = 0.463, p < 0.001 for valve and sedaDNA data sets, respectively). Samples from the upper core layers (∼1955–2019 CE) demonstrated an eminent increase of Pseudostaurosira polonica and decrease of Amphora pediculus + indistincta (represents the merged morphospecies of A. pediculus and A. indistincta) in the valve data set (Figure 4A). Correspondingly, for the same sample sets in the sedaDNA data, a notable increase of Pseudostaurosira and decrease of Amphora was observed (Figure 4B). These taxa were the most dominant ones in both data sets (in terms of number of valves and sequences). The counted number of valves and number of sequences per sample of these dominant taxa demonstrated significant correlations (n = 36, Spearman R = 0.612, p < 0.001 and Spearman R = 0.404, p = 0.015 for Amphora and Pseudostaurosira, respectively; Supplementary Figure S4). SIMPER analysis revealed that species of Pseudostaurosira and Amphora posed the greatest contribution to the dissimilarity between both diatom community groups established by MRT (Supplementary Table S6).
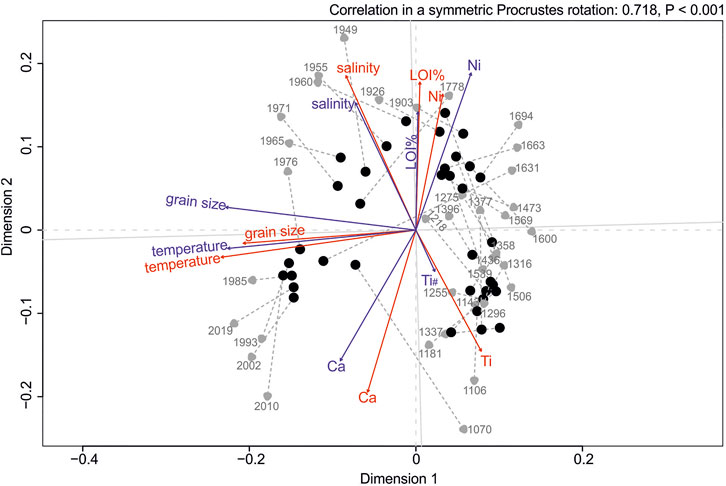
FIGURE 2. Procrustes test plot demonstrating the dbRDA ordination correlations between diatom communities recovered in valves (gray circles) and sedaDNA (black circles) data. The gray dashed lines point to the configuration in valve data ordination. Dark gray numbers (from 1070 to 2019) denote sample names, which correspond to the sediment layer date [year CE, weighted mean age of the core layer (Supplementary Table S2)]. Vectors on the plot denote environmental variables fitted on the graph with enfit function (red: vectors for valve data, blue: vectors for sedaDNA data; # in the vector name denotes non-significance as based on envfit results).
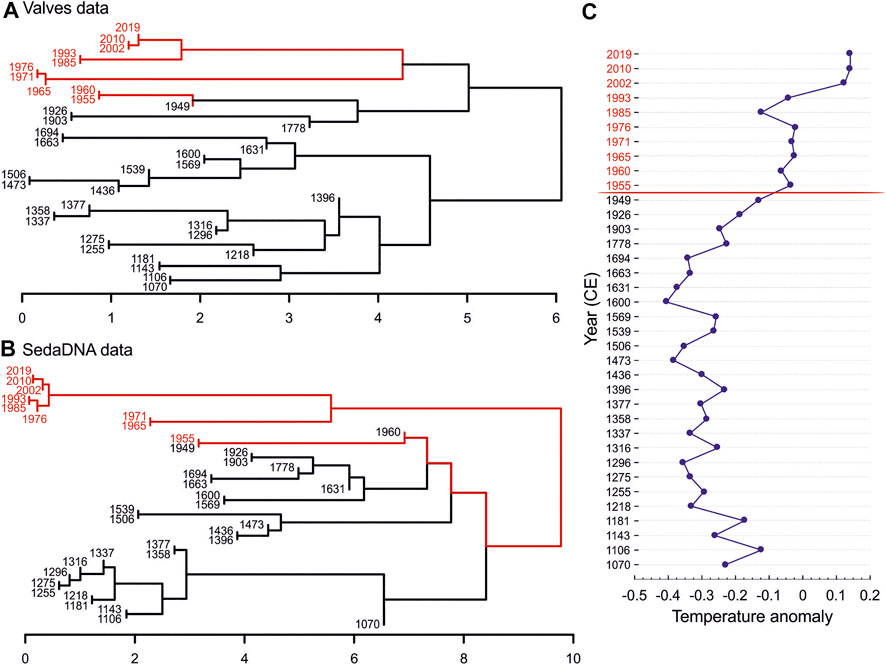
FIGURE 3. Constrained hierarchical clustering for valves (A) and sedaDNA (B) data. Numbers at the branch tips represent years (CE), weighted mean ages of the analyzed core layers (Supplementary Table S2). Branches in red and black denote the two groups identified by the multivariate regression tree (MRT) analyses. Panel (C) represents a scatter plot for the reconstructed temperature records (Mann and Jones, 2003; Collins et al., 2013; see Methods) per sample (i.e., year CE) where the red line between 1949 and 1955 denotes the breaking point detected by MRT analysis.
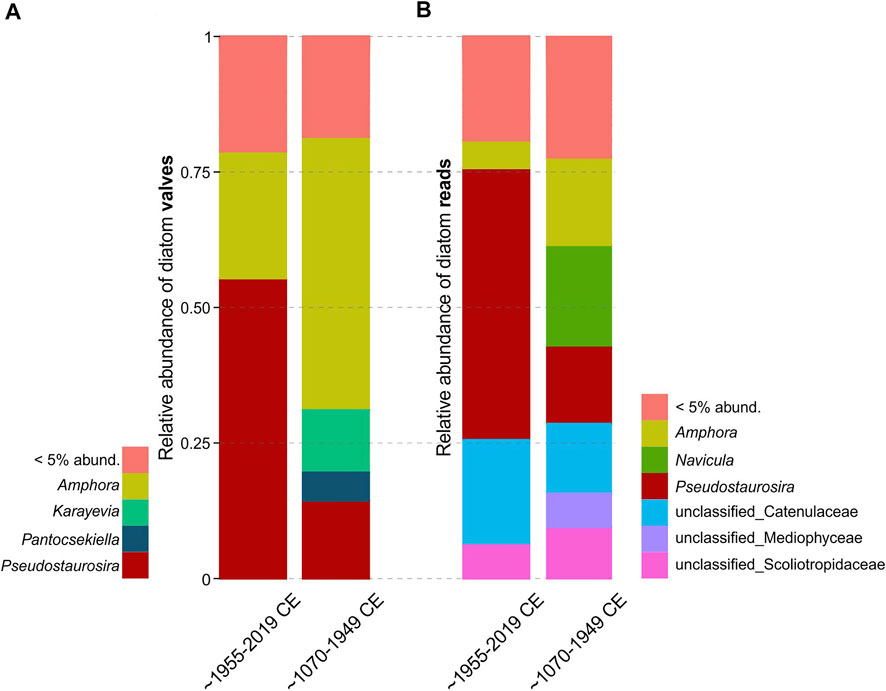
FIGURE 4. Diatom genus level bar plots for relative abundance of valves ((A), valve data) and reads ((B), sedaDNA data). The first column includes samples from the upper 10 cm of the core (n = 10; ∼1955–2019 CE) and the second column includes samples from the following layers until 51 cm depth (n = 26; ∼1070–1949 CE). This separation represents the breaking point detected by multivariate regression trees from both data sets. Most notable changes are in relative abundances of Amphora and Pseudostaurosira.
Diatom Richness in Valves vs. SedaDNA Data
The rarefaction curves demonstrated that the number of sequences per sample in relation to the detected number of ASVs reached a plateau, thus indicating a sufficient sequencing depth (Supplementary Figure S2). However, the number of identified morphospecies at the valves count cutoff level of 400 still exhibited continuous increase of the rarefaction curve (Supplementary Figure S2). This suggests that increasing the number of diatom valves per sample potentially results in a higher number of identified morphospecies. Nevertheless, there was a significant (but moderate) correlation between the effective number (q1) of diatom morphospecies detected and ASVs per sample (Spearman R = 0.495, p = 0.002). Interestingly, the effective number of both the morphospecies and ASVs showed a decline towards the upper core layers (n = 36, Spearman R = −0.394, p = 0.017 and Spearman R = −0.689, p < 0.001, respectively; Figure 5). The drop in diatom richness was especially notable from the breaking point detected by MRT (after ∼1950 CE; Figure 5). The most significant variable affecting the diatom richness as based on multiple linear regression (MLR) analyses was the grain size proxy (Zr/Rb) in both data sets (model F1,34 = 21.687, R2adj = 0.371, p < 0.001 and F1,34 = 37.746, R2adj = 0.512, p < 0.001, for valves and sedaDNA data, respectively). The grain size proxy moderately (but significantly; Spearman R = 0.629, p < 0.001) correlates with the temperature data. Therefore, simple regression analyses also demonstrated a relatively strong and significant effect of temperature (F1,34 = 5.865, R2adj = 0.147, p = 0.021 and F1,34 = 23.192, R2adj = 0.388, p < 0.001; for morphospecies and ASV richness, respectively). The salinity proxy was a marginally “non-significant” predictor for ASV richness (F1,34 = 4.005, R2adj = 0.105, p = 0.053), whereas it had a negligible effect on richness in the valve data (F1,34 = 0.434, R2adj = 0.013, p = 0.515). However, simple regression analysis detected the significant effect of nickel (Ni) in the morphospecies richness data (F1,34 = 6.721, R2adj = 0.140, p = 0.014).
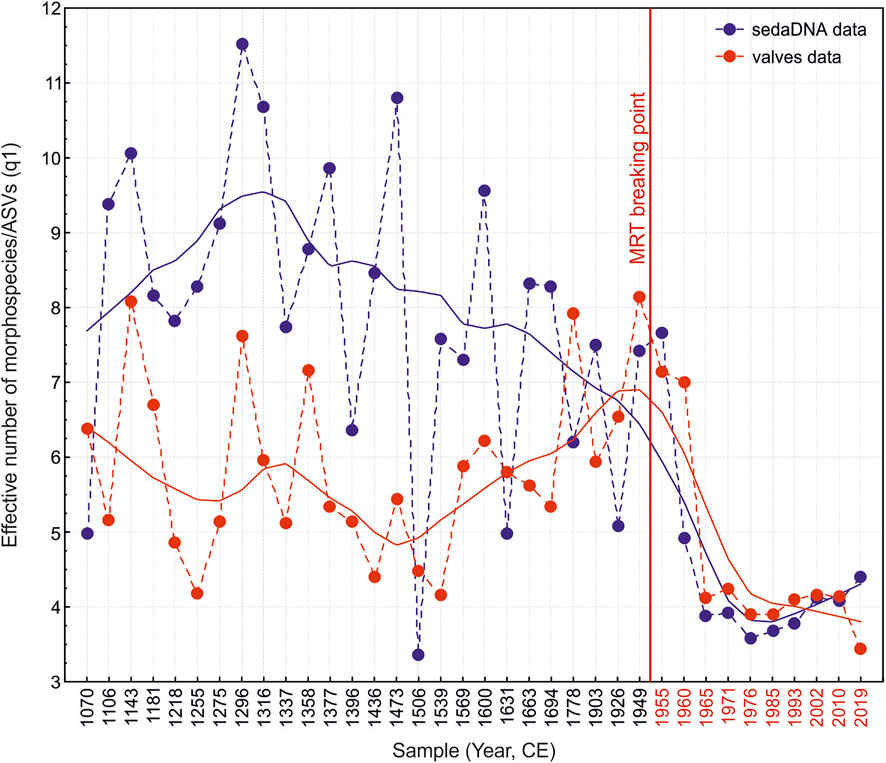
FIGURE 5. Effective number of morphospecies/ASVs per sample across the sediment core [represented as year (CE), weighted mean age of the core layer (Supplementary Table S2)]. The red vertical line denotes the breaking point detected by multivariate regression trees (MRT; for community data). Curves across the data points represent LOWESS fit.
Taxonomic Composition
The taxonomic composition between data sets was compared merging the morphospecies and ASVs to the genus level (merging taxa from the same genus using the phyloseq package in R). Via microscopy, 48 diatom genera were identified, while sedaDNA data harbored ASVs from 37 genera (Figure 6). However, the classification of 50.3% of ASVs remained at higher taxonomic levels due to low thresholds (see Methods, ASV table curation) against reference sequences. Clustering those 180 ASVs (unclassified to genus level) at a relaxed threshold of 94% sequence similarity (using vsearch --cluster_size, --iddef 2) resulted in 54 clusters. This suggests that sedaDNA captured a much higher diversity of diatoms, of which many taxa, however, remained unclassified to lower taxonomic levels because of the missing reference sequences.
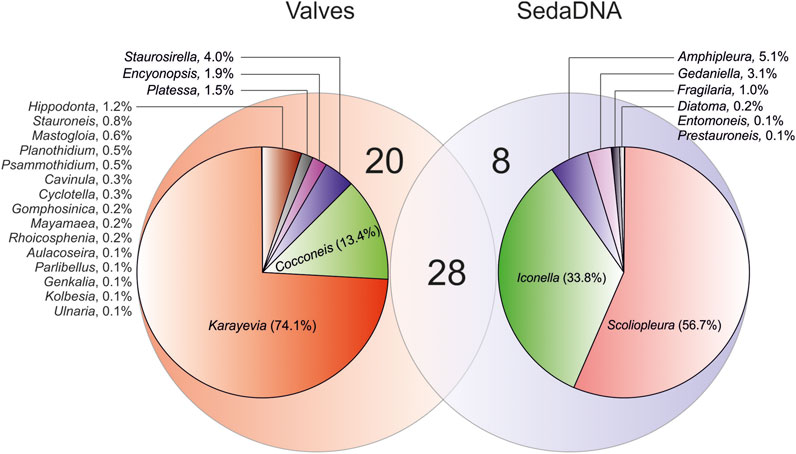
FIGURE 6. Venn diagram for shared and unique diatom genera identified in valves and sedaDNA data sets. There was a total of 28 shared genera between data sets, but 20 and 8 unique genera for valves and sedaDNA data set, respectively. Pie charts represent the proportion of valves/sequences of the genera that are unique per data set (i.e., only identified in valves or sedaDNA data set).
The most abundant morphologically identified genera that were missing from the sedaDNA data set were Karayevia (total of 1263 counted valves) and Cocconeis (228 valves). Other 18 genera in valve data that were not represented in sedaDNA data were much less abundant, with an average of ≤3 valves counted per sample (Figure 6). Most abundant genus level taxa detected with sedaDNA that were absent from the valve data set were Scoliopleura (total of 7,813 reads) and Iconella (4,658 reads). These taxa had an average read count of >245 per sample. Amphipleura (in 3 samples), Gedaniella (in 9 samples), and Fragilaria (in 3 samples) in the sedaDNA data, but missing from the valve data, were represented with an average of >46 reads per sample.
Discussion
Here, we compared the diatom community structures from sediment deposits, ranging over the last millennia, recovered via morphological and sedaDNA analyses from a brackish, high-pH Tibetan lake. Most dominant taxa (Amphora and Pseudostaurosira) were well represented in both data sets and demonstrated similar changes across the samples. The diatom assemblages as well as richness of morphospecies and ASVs demonstrated correlating patterns with largely concordant responses to the predictor variables.
Like in many alpine ecosystems across the globe, increases in air temperature have been registered on the Tibetan Plateau, especially after the 1960s (Wang et al., 2008; Guo and Wang, 2012). Consequently, the water temperature of the lakes has also increased (Wan et al., 2018), including the water temperature in our study lake, Nam Co (Huang et al., 2017). Along with Nam Co, the warming-induced changes have led to the surface area expansion of many lakes on the Tibetan Plateau (Liao et al., 2013; Anslan et al., 2020), with potential changes in other lake properties. The temperature reconstruction data used herein suggests a trend of a relatively strong temperature increase from the beginning of the 20th century (Figure 3C). This shift in the temperature may be the cause of past and ongoing changes in lake properties that affect the diatom communities (Rühland et al., 2015). Accordingly, the most important predictor variable in our data set was temperature, which explained the largest amount of variance in diatom community composition (Supplementary Table S6). From both data sets (valves and sedaDNA), a significant breaking point for diatom community composition was identified after ∼1950 CE (Figure 3). Subsequent to this breaking point, a notable drop in richness was observed, for both effective number of morphospecies and ASVs (Figure 5). Although the most significant variable predicting diatom richness was the grain size proxy (Zr/Rb), it is important to highlight that this variable significantly correlates with temperature. Coarser grain size with an increase in silt and sand are reflected by higher Zr/Rb ratios (Dypvik and Harris, 2001; Chen et al., 2006; Wang et al., 2015), suggesting an increase in precipitation and meltwater (due to higher temperatures). This enhances erosion from the catchment of Nam Co and, consequently, the transport of this material into the lake (Schütt et al., 2010). Thus, one of the factors possibly contributing to the decreases in diatom richness is the increased water turbidity (Vorobyeva et al., 2015). The decline in diatom species richness towards the recent century has also been found in other studies (Sorvari et al., 2002; Vorobyeva et al., 2015), with shifts in community composition around the decade of the 1960s (Yan et al., 2018). That this same pattern is reproducibly encountered in our valves and sedaDNA data sets demonstrates that both methods were able to recover major signals in the temporal dynamics of the diatom communities.
Although the general community and richness patterns matched well, there were substantial taxonomic mismatches between our valves and sedaDNA data sets (i.e., some taxa were found only via microscopy, but not in sedaDNA data, and vice versa; Figure 6). This was expected as the reference sequence libraries are far from being complete for diatoms (Stoof-Leichsenring et al., 2014; Rivera et al., 2018; Kang et al., 2021). About 50% of ASVs in the sedaDNA data set remained unclassified to lower taxonomic levels, such as genus or species. Therefore, several genera were present in the valve data but were missing from the sedaDNA data (such as Karayevia, Cocconeis, and Platessa; Figure 6). Due to the lack of reference sequences for Karayevia, ASVs could not be assigned to the latter genus; thus, they are possibly represented in the sedaDNA data as unclassified genus. Several reference sequences are available for Cocconeis, but some ASVs demonstrated a sequence identity match of 95.9%–97.3%. According to the taxonomy annotation criterion used herein (≥98% for genus level), these ASVs were assigned to only family level (Cocconeidaceae). However, the boundaries between inter- and intrageneric divergence of the short rbcL marker for diatoms are not clear. Therefore, these ASVs demonstrating <98% similarity against available Cocconeis reference sequences could possibly represent the latter genus. An additional factor affecting the potential absence of Cocconeis from sedaDNA data is the presence of two mismatches within the used forward primer. Mismatches at the positions 9 (T-A) and 21 (C-T) of the primer sequence are likely not too critical, but we cannot exclude the possibility that these have hampered the amplification success of DNA fragments from Cocconeis. Other “missing” genera from the sedaDNA data set were represented in very few numbers of counted valves in the latter data set. Rare taxa may be often missed with DNA metabarcoding (Kermarrec et al., 2013; Kang et al., 2021; possible reasons explained within). However, the potential taxonomic mismatches between data sets may also result from inter-investigator variation depending on changes in diatom taxonomy and the use of synonymous names (Kang et al., 2021). For example, the basionyms of Cavinula lapidosa and C. pseudoscutiformis identified via microscopy in our study correspond to Navicula species. Currently, there are no reference rbcL sequences for Cavinula; thus, none of the ASVs could be annotated to the latter genus, whereas many ASVs corresponded to Navicula sp. Therefore, it is possible that some ASVs assigned to Navicula correspond to Cavinula in the valve data set, because the use of synonyms (and misidentified taxa) is not uncommon in the public databases (Fort et al., 2021). Despite the gaps in the reference databases, the sedaDNA data set contained a much higher diversity of diatoms. Clustering the ASVs that remained unclassified to the genus level (at 94% sequence similarity threshold) formed more than 50 diatom genetic lineages, which already exceeds the total number of genera identified via microscopy. Although 94% sequence similarity threshold might not always correspond to intergeneric divergence of diatoms in this short rbcL fragment, based on the examination of the 10 best blastn hits for the ASVs, this threshold indeed often separated diatom ASV from different genera. Notably, the valve data set completely lacked species from the family Fragilariaceae, whereas five ASVs with a total of more than 30,000 reads were present in the sedaDNA data set. Since delicate taxa are more strongly subjected to dissolution compared to heavily silicified ones (Smol and Stoermer, 2010), we hypothesize that species from Fragilariaceae may be missed by microscopy, rather than represent artificial ASVs in the sedaDNA data. This hypothesis is somewhat supported by the fact that the valves of Fragilaria are especially subject to dissolution (Barker et al., 1994). Likewise, the genera Amphipleura and Entomoneis have weakly silicified valves, thus also likely missing from the valve data set because of the dissolution effect. It is noteworthy that genus Gedaniella (Fragilariaceae; found only in the sedaDNA data set) was created based on molecular information (Li et al., 2018; Morales et al., 2019). Because it was poorly defined in the original study (Li et al., 2018), it is not considered as a valid genus by diatomists working with morphological data, and species attributed to Gedaniella are rather identified as Pseudostaurosira or Sarcophagodes (Morales et al., 2019). Interestingly, the most abundant genera (in terms of sequences) that were absent in valve data, but detected via sedaDNA, were Scoliopleura (Neidiaceae) and Iconella (Surirellaceae; Figure 5). Species from these genera usually have relatively heavily silicified and large valves. The biovolume of the Iconella cell may be up to 1,000 times higher compared with, for example, Karayevia or Cocconeis species. Because the copy numbers of the rbcL gene can be highly correlated with the biovolume of a diatom (Vasselon et al., 2018), a relatively strong DNA “signal” in relation to the abundance proportion may have been recorded. Therefore, it is likely that Iconella and Scoliopleura are over-represented in the sedaDNA data set but missed from the valve data set because their valves had rare occurrences (as potential undersampling was indicated by the rarefaction curves, Supplementary Figure S2B). Here, compared with the conclusions based on sedaDNA data, the absence of potentially dissolved or rare species in the valve data set did not considerably alter the results. Nevertheless, it is important to consider the integrity of fossil records when applying the microscopic approach (Smol and Stoermer 2010).
Here, we report that the overall diatom richness and community composition from the sediment core of the brackish lake Nam Co, covering the last millennium, were well correlated between valves and sedaDNA data sets. Therefore, interpretations about the paleo-environmental conditions (although not the scope of this study) would likely produce concordant conclusions. Importantly, our sediment core was taken from a relatively shallow part of the lake (water depth 27 m) where we did not observe highly critical diatom valve dissolution effects in our samples. On the contrary, studies examining sediment cores from much deeper parts of lake Nam Co (78 and 93 m; Wang et al., 2011; Kasper et al., 2013) noted an issue about diatom valve dissolution. Valve dissolution seems to be a greater concern in sediments from deeper water depths (Flower, 1993); thus, it is likely that microscopy and sedaDNA identification methods for diatoms would produce different results as observed herein when examining a core from a deeper part of the lake. The sedaDNA-based work on deep saline or brackish lakes on the Tibetan Plateau are currently scarce, but working with the sediment core, taken from 38 m water depth at brackish Tibetan lake, Dagze Co (pH 10.1), Liu et al. (2021) were able to amplify and sequence multiple groups of aquatic eukaryotes. Although it yet remains to be clarified, we therefore hypothesize that the sedaDNA analyses would capture more complete community structures of diatoms from the lake sediments where the valve dissolution effect is severe.
As suggested by our results, sedaDNA metabarcoding recovers a much higher diversity of diatoms, but many ASVs could not be identified to lower taxonomic levels—a limiting factor in cases when the identity of diatom species is essential for interpreting the results. On the other hand, classical microscopy may miss diatom taxa playing crucial roles in the community, as the data may be affected by the low integrity of the diatom fossil record. Although the salinity and high pH of our study lake could promote the dissolution of diatom valves, we found that this was not the major issue for the sediment core taken from a relatively shallow part of the lake (water depth 27 m). Clearly, there are trade-offs between the identification methods; thus, choosing among them (when both approaches are not feasible) largely depends on the study aims.
Data Availability Statement
The data sets presented in this study can be found in online repositories. The names of the repository/repositories and accession number(s) can be found below: https://www.ncbi.nlm.nih.gov/bioproject/PRJNA781478.
Author Contributions
SA and MV contributed to conception and design of the study. SA, WK, BW, PE-G, NB, AAS, and PP performed sampling. BW, WK, and PE-G contributed to the core dating processes. WK performed morphological analyses of the diatom fossils. SA and SK performed the molecular analysis with support from KL and YL. SA performed statistical analysis. ANS developed the program (DFG-GRK 2309) that funded this research. SA wrote the first draft of the manuscript. All authors contributed to manuscript revision and approved the submitted version.
Funding
Funding was provided by the Deutsche Forschungsgemeinschaft (DFG) via the International Research Training Group “Geo-ecosystems in transition on the Tibetan Plateau (TransTiP)”, DFG grant 317513741/GRK 2309.
Conflict of Interest
The authors declare that the research was conducted in the absence of any commercial or financial relationships that could be construed as a potential conflict of interest.
The handling editor declared a shared affiliation with one of the authors PR at the time of review.
Publisher’s Note
All claims expressed in this article are solely those of the authors and do not necessarily represent those of their affiliated organizations, or those of the publisher, the editors, and the reviewers. Any product that may be evaluated in this article, or claim that may be made by its manufacturer, is not guaranteed or endorsed by the publisher.
Acknowledgments
We thank the Institute of Tibetan Plateau Research, Chinese Academy of Sciences for supplying the research permission obtained from the Tibet Autonomous Region Government. We are grateful to the team of the International Research Training Group “Geoecosystems in transition on the Tibetan Plateau” (TransTiP) for logistic support and fruitful discussions. We thank Andrea Lami from the ISE-CNR Institute of Ecosystem Study for his measurement of the LOI. We thank Dada Yan from the Institute of Geographical Science, Freie Universität Berlin, for the discussions considering the age model construction and interpretation and Eike Reinosch for providing Nam Co catchment graphics.
Supplementary Material
The Supplementary Material for this article can be found online at: https://www.frontiersin.org/articles/10.3389/feart.2022.824656/full#supplementary-material
References
Alberdi, A., and Gilbert, M. T. P. (2019). A Guide to the Application of Hill Numbers to DNA‐based Diversity Analyses. Mol. Ecol. Resour. 19 (4), 804–817. doi:10.1111/1755-0998.13014
Anslan, S., Azizi Rad, M., Buckel, J., Echeverria Galindo, P., Kai, J., Kang, W., et al. (2020). Reviews and Syntheses: How Do Abiotic and Biotic Processes Respond to Climatic Variations in the Nam Co Catchment (Tibetan Plateau)? Biogeosciences 17 (5), 1261–1279. doi:10.5194/bg-17-1261-2020
Anslan, S., Bahram, M., Hiiesalu, I., and Tedersoo, L. (2017). PipeCraft: Flexible Open-Source Toolkit for Bioinformatics Analysis of Custom High-Throughput Amplicon Sequencing Data. Mol. Ecol. Resour. 17 (6), e234–e240. doi:10.1111/1755-0998.12692
Barker, P., Fontes, J.-C., Gasse, F., and Druart, J. C. (1994). Experimental Dissolution of Diatom Silica in Concentrated Salt Solutions and Implications for Paleoenvironmental Reconstruction. Limnol. Oceanogr. 39 (1), 99–110. doi:10.4319/lo.1994.39.1.0099
Blaauw, M., and Christen, J. A. (2011). Flexible Paleoclimate Age-Depth Models Using an Autoregressive Gamma Process. Bayesian Anal. 6 (3), 457–474. doi:10.1214/ba/1339616472
Callahan, B. J., McMurdie, P. J., Rosen, M. J., Han, A. W., Johnson, A. J. A., and Holmes, S. P. (2016). DADA2: High-Resolution Sample Inference from Illumina Amplicon Data. Nat. Methods 13 (7), 581–583. doi:10.1038/nmeth.3869
Camacho, C., Coulouris, G., Avagyan, V., Ma, N., Papadopoulos, J., Bealer, K., et al. (2009). BLAST+: Architecture and Applications. BMC Bioinformatics 10 (1), 421. doi:10.1186/1471-2105-10-421
Capo, E., Giguet-Covex, C., Rouillard, A., Nota, K., Heintzman, P. D., Vuillemin, A., et al. (2021). Lake Sedimentary DNA Research on Past Terrestrial and Aquatic Biodiversity: Overview and Recommendations. Quaternary 4 (1), 6. doi:10.3390/quat4010006
Chen, J., Chen, Y., Liu, L., Ji, J., Balsam, W., Sun, Y., et al. (2006). Zr/Rb Ratio in the Chinese Loess Sequences and its Implication for Changes in the East Asian winter Monsoon Strength. Geochimica et Cosmochimica Acta 70 (6), 1471–1482. doi:10.1016/j.gca.2005.11.029
Chen, W., and Ficetola, G. F. (2020). Numerical Methods for sedimentary‐ancient‐DNA‐based Study on Past Biodiversity and Ecosystem Functioning. Environ. DNA 2 (2), 115–129. doi:10.1002/edn3.79
Collins, M., Knutti, R., Arblaster, J., Dufresne, J.-L., Fichefet, T., Friedlingstein, P., et al. (2013). “Long-term Climate Change: Projections, Commitments and Irreversibility,” in Climate Change 2013-The Physical Science Basis: Contribution of Working Group I to the Fifth Assessment Report of the Intergovernmental Panel on Climate Change (Cambridge University Press), 1029–1136.
De'ath, G. (2014). Mvpart: Multivariate Partitioning. R Package Version 1.6-2. https://CRAN.R-project.org/package=mvpart.
Domaizon, I., Winegardner, A., Capo, E., Gauthier, J., and Gregory-Eaves, I. (2017). DNA-based Methods in Paleolimnology: New Opportunities for Investigating Long-Term Dynamics of Lacustrine Biodiversity. J. Paleolimnol. 58 (1), 1–21. doi:10.1007/s10933-017-9958-y
Dulias, K., Stoof-Leichsenring, K. R., Pestryakova, L. A., and Herzschuh, U. (2017). Sedimentary DNA versus Morphology in the Analysis of Diatom-Environment Relationships. J. Paleolimnol. 57 (1), 51–66. doi:10.1007/s10933-016-9926-y
Dypvik, H., and Harris, N. B. (2001). Geochemical Facies Analysis of fine-grained Siliciclastics Using Th/U, Zr/Rb and (Zr+ Rb)/Sr Ratios. Chem. Geology. 181 (1-4), 131–146. doi:10.1016/s0009-2541(01)00278-9
Echeverría‐Galindo, P., Anslan, S., Frenzel, P., Künzel, S., Vences, M., Pérez, L., et al. (2021). High‐throughput Identification of Non‐marine Ostracoda from the Tibetan Plateau: Evaluating the success of Various Primers on Sedimentary DNA Samples. Environ. DNA 3 (5), 982–996. doi:10.1002/edn3.222
Elbrecht, V., and Leese, F. (2017). PrimerMiner : an R Package for Development and In Silico Validation of DNA Metabarcoding Primers. Methods Ecol. Evol. 8 (5), 622–626. doi:10.1111/2041-210x.12687
Epp, L. S., Gussarova, G., Boessenkool, S., Olsen, J., Haile, J., Schrøder-Nielsen, A., et al. (2015). Lake Sediment Multi-Taxon DNA from North Greenland Records Early post-glacial Appearance of Vascular Plants and Accurately Tracks Environmental Changes. Quat. Sci. Rev. 117, 152–163. doi:10.1016/j.quascirev.2015.03.027
Flower, R. J. (1993). “Diatom Preservation: Experiments and Observations on Dissolution and Breakage in Modern and Fossil Material,” in Twelfth International Diatom Symposium (Springer), 473–484.
Flower, R. J., and Ryves, D. B. (2009). Diatom Preservation: Differential Preservation of Sedimentary Diatoms in Two saline Lakes. Acta Bot. Croat. 68 (2), 381–399.
Fort, A., McHale, M., Cascella, K., Potin, P., Perrineau, M. M., Kerrison, P. D., et al. (2021). Exhaustive Reanalysis of Barcode Sequences from Public Repositories Highlights Ongoing Misidentifications and Impacts Taxa Diversity and Distribution. Mol. Ecol. Resour. 22, 86–101. doi:10.1111/1755-0998.13453
Gouy, M., Guindon, S., and Gascuel, O. (2010). SeaView Version 4: A Multiplatform Graphical User Interface for Sequence Alignment and Phylogenetic Tree Building. Mol. Biol. Evol. 27 (2), 221–224. doi:10.1093/molbev/msp259
Guiry, M. D., Guiry, G. M., Morrison, L., Rindi, F., Miranda, S. V., Mathieson, A. C., et al. (2014). AlgaeBase: an On-Line Resource for Algae. Cryptogamie, Algologie 35 (2), 105–115. doi:10.7872/crya.v35.iss2.2014.105
Guo, D., and Wang, H. (2012). The Significant Climate Warming in the Northern Tibetan Plateau and its Possible Causes. Int. J. Climatol. 32 (12), 1775–1781. doi:10.1002/joc.2388
Heiri, O., Lotter, A. F., and Lemcke, G. (2001). Loss on Ignition as a Method for Estimating Organic and Carbonate Content in Sediments: Reproducibility and Comparability of Results. J. Paleolimnol. 25(1), 101–110. doi:10.1023/A:1008119611481
Huang, L., Wang, J., Zhu, L., Ju, J., and Daut, G. (2017). The Warming of Large Lakes on the Tibetan Plateau: Evidence from a Lake Model Simulation of Nam Co, China, during 1979-2012. J. Geophys. Res. Atmos. 122 (24), 095–113. doi:10.1002/2017jd027379
Jia, W., Liu, X., Stoof‐Leichsenring, K. R., Liu, S., Li, K., and Herzschuh, U. (2021). Preservation of Sedimentary Plant DNA Is Related to lake Water Chemistry. Environ. DNA 00, 1–15. doi:10.1002/edn3.259
Juggins, S. (2020). Rioja: Analysis of Quaternary Science Data. R Package Version 0.9-26. Available at: https://cran.r-project.org/web/packages/rioja/index.html.
Kang, W., Anslan, S., Börner, N., Schwarz, A., Schmidt, R., Künzel, S., et al. (2021). Diatom Metabarcoding and Microscopic Analyses from Sediment Samples at Lake Nam Co, Tibet: The Effect of Sample-Size and Bioinformatics on the Identified Communities. Ecol. Indicators 121, 107070. doi:10.1016/j.ecolind.2020.107070
Kanz, C., Aldebert, P., Althorpe, N., Baker, W., Baldwin, A., Bates, K., et al. (2004). The EMBL Nucleotide Sequence Database. Nucleic Acids Res. 33 (Database issue), D29–D33. doi:10.1093/nar/gki098
Kasper, T., Frenzel, P., Haberzettl, T., Schwarz, A., Daut, G., Meschner, S., et al. (2013). Interplay between Redox Conditions and Hydrological Changes in Sediments from Lake Nam Co (Tibetan Plateau) during the Past 4000cal BP Inferred from Geochemical and Micropaleontological Analyses. Palaeogeogr. Palaeoclimatol. Palaeoecol. 392, 261–271. doi:10.1016/j.palaeo.2013.09.027
Katoh, K., Rozewicki, J., and Yamada, K. D. (2019). MAFFT Online Service: Multiple Sequence Alignment, Interactive Sequence Choice and Visualization. Brief Bioinform 20 (4), 1160–1166. doi:10.1093/bib/bbx108
Kelly, M. G., Juggins, S., Mann, D. G., Sato, S., Glover, R., Boonham, N., et al. (2020). Development of a Novel Metric for Evaluating Diatom Assemblages in Rivers Using DNA Metabarcoding. Ecol. Indicators 118, 106725. doi:10.1016/j.ecolind.2020.106725
Kermarrec, L., Franc, A., Rimet, F., Chaumeil, P., Humbert, J. F., and Bouchez, A. (2013). Next‐generation Sequencing to Inventory Taxonomic Diversity in Eukaryotic Communities: a Test for Freshwater Diatoms. Mol. Ecol. Resour. 13 (4), 607–619. doi:10.1111/1755-0998.12105
Lange-Bertalot, H., Hofmann, G., Werum, M., Cantonati, M., and Kelly, M. G. (2017). Freshwater Benthic Diatoms of Central Europe: Over 800 Common Species Used in Ecological Assessment. Oberreifenberg, Germany: Koeltz Botanical Books Schmitten-Oberreifenberg.
Laug, A., Haberzettl, T., Pannes, A., Schwarz, A., Turner, F., Wang, J., et al. (2021). Holocene Paleoenvironmental Change Inferred from Two Sediment Cores Collected in the Tibetan lake Taro Co. J. Paleolimnol. 66 (3), 171–186. doi:10.1007/s10933-021-00198-6
Li, C. L., Witkowski, A., Ashworth, M. P., Dąbek, P., Sato, S., Zgłobicka, I., et al. (2018). The Morphology and Molecular Phylogenetics of Some marine Diatom Taxa within the Fragilariaceae, Including Twenty Undescribed Species and Their Relationship to Nanofrustulum, Opephora and Pseudostaurosira. Phytotaxa 355 (1), 1–104. doi:10.11646/phytotaxa.355.1.1
Li, G., Dong, H., Hou, W., Wang, S., Jiang, H., Yang, J., et al. (2016). Temporal Succession of Ancient Phytoplankton Community in Qinghai Lake and Implication for Paleo-Environmental Change. Sci. Rep. 6, 19769. doi:10.1038/srep19769
Liao, J., Shen, G., and Li, Y. (2013). Lake Variations in Response to Climate Change in the Tibetan Plateau in the Past 40 Years. Int. J. Digital Earth 6 (6), 534–549. doi:10.1080/17538947.2012.656290
Liu, K., Liu, Y., Hu, A., Wang, F., Liang, J., Zhang, Z., et al. (2021). Temporal Variability of Microbial Communities during the Past 600 Years in a Tibetan lake Sediment Core. Palaeogeogr. Palaeoclimatol. Palaeoecol. 584, 110678. doi:10.1016/j.palaeo.2021.110678
Lobo, E. A., Heinrich, C. G., Schuch, M., Wetzel, C. E., and Ector, L. (2016). “Diatoms as Bioindicators in Rivers,” in Diatoms as Bioindicators in Rivers. Editor O. NecchiJr. (Cham: Springer International Publishing), 245–271. doi:10.1007/978-3-319-31984-1_11
Mann, M. E., and Jones, P. D. (2003). Global Surface Temperatures over the Past Two Millennia. Geophys. Res. Lett. 30 (15), 1820. doi:10.1029/2003gl017814
Martin, M. (2011). Cutadapt Removes Adapter Sequences from High-Throughput Sequencing Reads. EMBnet j. 17 (1), 10–12. doi:10.14806/ej.17.1.200
McMurdie, P. J., and Holmes, S. (2013). Phyloseq: an R Package for Reproducible Interactive Analysis and Graphics of Microbiome Census Data. PLoS ONE 8 (4), e61217. doi:10.1371/journal.pone.0061217
Mianping, Z. (1997). “Saline Lakes and Lake Districts,” in An Introduction to Saline Lakes on the Qinghai—Tibet Plateau (Dordrecht: Springer Netherlands), 18–22. doi:10.1007/978-94-011-5458-1_2
Morales, E. A., and Edlund, M. B. (2003). Studies in Selected Fragilarioid Diatoms (Bacillariophyceae) from Lake Hovsgol, Mongolia. Phycological Res. 51 (4), 225–239. doi:10.1111/j.1440-1835.2003.x
Morales, E. A., Wetzel, C. E., Novais, M. H., Buczkó, K., Morais, M. M., and Ector, L. (2019). Morphological Reconsideration of the Araphid Genus Pseudostaurosira (Bacillariophyceae), a Revision of Gedaniella, Popovskayella and Serratifera, and a Description of a New Nanofrustulum Species. Pl. Ecol. Evol. 152 (2), 262–284. doi:10.5091/plecevo.2019.1604
Oksanen, J., Blanchet, F. G., Kindt, R., Legendre, P., Minchin, P. R., O’hara, R., et al. (2015). vegan: Community Ecology Package. R Package Version 2.5-7. https://CRAN.R-project.org/package=vegan
Piredda, R., Sarno, D., Lange, C. B., Tomasino, M. P., Zingone, A., and Montresor, M. (2017). Diatom Resting Stages in Surface Sediments: a Pilot Study Comparing Next Generation Sequencing and Serial Dilution Cultures. Cryptogamie, Algologie 38 (1), 31–46. doi:10.7872/crya/v38.iss1.2017.31
R-Core-Team (2021). R: A language and environment for statistical computing. Vienna, Austria: R Foundation for Statistical Computing. Available online: https://www.R-project.org/.
Reid, M. A., Tibby, J. C., Penny, D., and Gell, P. A. (1995). The Use of Diatoms to Assess Past and Present Water Quality. Austral Ecol. 20 (1), 57–64. doi:10.1111/j.1442-9993.1995.tb00522.x
Reimer, P. J., Austin, W. E. N., Bard, E., Bayliss, A., Blackwell, P. G., Bronk Ramsey, C., et al. (2020). The Intcal20 Northern Hemisphere Radiocarbon Age Calibration Curve (0-55 Cal Kbp). Radiocarbon 62 (4), 725–757. doi:10.1017/Rdc.2020.41
Rimet, F., Chaumeil, P., Keck, F., Kermarrec, L., Vasselon, V., Kahlert, M., et al. (2016). R-Syst: diatom: an Open-Access and Curated Barcode Database for Diatoms and Freshwater Monitoring. Database 2016, baw016. doi:10.1093/database/baw016
Rivera, S. F., Vasselon, V., Jacquet, S., Bouchez, A., Ariztegui, D., and Rimet, F. (2018). Metabarcoding of lake Benthic Diatoms: from Structure Assemblages to Ecological Assessment. Hydrobiologia 807 (1), 37–51. doi:10.1007/s10750-017-3381-2
Rognes, T., Flouri, T., Nichols, B., Quince, C., and Mahé, F. (2016). VSEARCH: a Versatile Open Source Tool for Metagenomics. PeerJ 4, e2584. doi:10.7717/peerj.2584
Rühland, K. M., Paterson, A. M., and Smol, J. P. (2015). Lake Diatom Responses to Warming: Reviewing the Evidence. J. Paleolimnol. 54 (1), 1–35. doi:10.1007/s10933-015-9837-3
Ryves, D. B., Juggins, S., Fritz, S. C., and Battarbee, R. W. (2001). Experimental Diatom Dissolution and the Quantification of Microfossil Preservation in Sediments. Palaeogeogr. Palaeoclimatol. Palaeoecol. 172 (1-2), 99–113. doi:10.1016/S0031-0182(01)00273-5
Ryves, D. B., Battarbee, R. W., Juggins, S., Fritz, S. C., and Anderson, N. J. (2006). Physical and Chemical Predictors of Diatom Dissolution in Freshwater and saline lake Sediments in North America and West Greenland. Limnol. Oceanogr. 51 (3), 1355–1368. doi:10.4319/lo.2006.51.3.1355
Schloss, P. D., Westcott, S. L., Ryabin, T., Hall, J. R., Hartmann, M., Hollister, E. B., et al. (2009). Introducing Mothur: Open-Source, Platform-independent, Community-Supported Software for Describing and Comparing Microbial Communities. Appl. Environ. Microbiol. 75 (23), 7537–7541. doi:10.1128/AEM.01541-09
Schütt, B., Berking, J., Frechen, M., Frenzel, P., Schwalb, A., and Wrozyna, C. (2010). Late Quaternary Transition from Lacustrine to a Fluvio-Lacustrine Environment in the north-western Nam Co, Tibetan Plateau, China. Quat. Int. 218 (1-2), 104–117. doi:10.1016/j.quaint.2009.05.009
Smol, J. P., and Douglas, M. S. V. (1996). Long-term Environmental Monitoring in Arctic Lakes and Ponds Using Diatoms and Other Biological Indicators. Geosci. Can. 23 (4), 225–230.
Smol, J. P., and Stoermer, E. F. (2010). The Diatoms: Applications for the Environmental and Earth Sciences. Cambridge University Press.
Sorvari, S., Korhola, A., and Thompson, R. (2002). Lake Diatom Response to Recent Arctic Warming in Finnish Lapland. Glob. Change Biol 8 (2), 171–181. doi:10.1046/j.1365-2486.2002.00463.x
StatSoft Inc., Tulsa, OK. (2004). STATISTICA (Data Analysis Software System), Version 7d. Available at: www.statsoft.com.
Stivrins, N., Soininen, J., Tõnno, I., Freiberg, R., Veski, S., and Kisand, V. (2018). Towards Understanding the Abundance of Non-pollen Palynomorphs: A Comparison of Fossil Algae, Algal Pigments and sedaDNA from Temperate lake Sediments. Rev. Palaeobotany Palynology 249, 9–15. doi:10.1016/j.revpalbo.2017.11.001
Stoof-Leichsenring, K. R., Bernhardt, N., Pestryakova, L. A., Epp, L. S., Herzschuh, U., and Tiedemann, R. (2014). A Combined Paleolimnological/genetic Analysis of Diatoms Reveals Divergent Evolutionary Lineages of Staurosira and Staurosirella (Bacillariophyta) in Siberian lake Sediments along a Latitudinal Transect. J. Paleolimnol. 52 (1-2), 77–93. doi:10.1007/s10933-014-9779-1
Stoof-Leichsenring, K. R., Dulias, K., Biskaborn, B. K., Pestryakova, L. A., and Herzschuh, U. (2020). Lake-depth Related Pattern of Genetic and Morphological Diatom Diversity in Boreal Lake Bolshoe Toko, Eastern Siberia. PLoS ONE 15 (4), e0230284. doi:10.1371/journal.pone.0230284
Stoof-Leichsenring, K. R., Epp, L. S., Trauth, M. H., and Tiedemann, R. (2012). Hidden Diversity in Diatoms of Kenyan Lake Naivasha: a Genetic Approach Detects Temporal Variation. Mol. Ecol. 21 (8), 1918–1930. doi:10.1111/j.1365-294X.2011.05412.x
Taberlet, P., Bonin, A., Coissac, E., and Zinger, L. (2018). Environmental DNA: For Biodiversity Research and Monitoring. Oxford: Oxford University Press.
Talas, L., Stivrins, N., Veski, S., Tedersoo, L., and Kisand, V. (2021). Sedimentary Ancient DNA (sedaDNA) Reveals Fungal Diversity and Environmental Drivers of Community Changes throughout the Holocene in the Present Boreal Lake Lielais Svētiņu (Eastern Latvia). Microorganisms 9 (4), 719. doi:10.3390/microorganisms9040719
Vanderplicht, J., and Hogg, A. (2006). A Note on Reporting Radiocarbon. Quat. Geochronol. 1 (4), 237–240. doi:10.1016/j.quageo.2006.07.001
Vasselon, V., Bouchez, A., Rimet, F., Jacquet, S., Trobajo, R., Corniquel, M., et al. (2018). Avoiding Quantification Bias in Metabarcoding: Application of a Cell Biovolume Correction Factor in Diatom Molecular Biomonitoring. Methods Ecol. Evol. 9 (4), 1060–1069. doi:10.1111/2041-210x.12960
Vasselon, V., Rimet, F., Tapolczai, K., and Bouchez, A. (2017). Assessing Ecological Status with Diatoms DNA Metabarcoding: Scaling-Up on a WFD Monitoring Network (Mayotte Island, France). Ecol. Indicators 82, 1–12. doi:10.1016/j.ecolind.2017.06.024
Vorobyeva, S. S., Trunova, V. A., Stepanova, O. G., Zvereva, V. V., Petrovskii, S. K., Melgunov, M. S., et al. (2015). Impact of Glacier Changes on Ecosystem of Proglacial Lakes in High Mountain Regions of East Siberia (Russia). Environ. Earth Sci. 74 (3), 2055–2063. doi:10.1007/s12665-015-4164-6
Wan, W., Zhao, L., Xie, H., Liu, B., Li, H., Cui, Y., et al. (2018). Lake Surface Water Temperature Change over the Tibetan Plateau from 2001 to 2015: A Sensitive Indicator of the Warming Climate. Geophys. Res. Lett. 45 (20), 11177–11186. doi:10.1029/2018gl078601
Wang, B., Bao, Q., Hoskins, B., Wu, G., and Liu, Y. (2008). Tibetan Plateau Warming and Precipitation Changes in East Asia. Geophys. Res. Lett. 35 (14). doi:10.1029/2008gl034330
Wang, J., Zhu, L., Wang, Y., Ju, J., Daut, G., and Li, M. (2015). Spatial Variability and the Controlling Mechanisms of Surface Sediments from Nam Co, central Tibetan Plateau, China. Sediment. Geology. 319, 69–77. doi:10.1016/j.sedgeo.2015.01.011
Wang, R., Yang, X., Langdon, P., and Zhang, E. (2011). Limnological Responses to Warming on the Xizang Plateau, Tibet, over the Past 200 Years. J. Paleolimnol. 45 (2), 257–271. doi:10.1007/s10933-011-9496-y
Wei, T., Simko, V., Levy, M., Xie, Y., Jin, Y., and Zemla, J. (2017). Package Corrplot. Statistician 56, 316–324.
Keywords: SedaDNA, Tibetan Plateau, Lake Nam Co, metabarcoding, microscopy, sediment core
Citation: Anslan S, Kang W, Dulias K, Wünnemann B, Echeverría-Galindo P, Börner N, Schwarz A, Liu Y, Liu K, Künzel S, Kisand V, Rioual P, Peng P, Wang J, Zhu L, Vences M and Schwalb A (2022) Compatibility of Diatom Valve Records With Sedimentary Ancient DNA Amplicon Data: A Case Study in a Brackish, Alkaline Tibetan Lake. Front. Earth Sci. 10:824656. doi: 10.3389/feart.2022.824656
Received: 29 November 2021; Accepted: 28 January 2022;
Published: 07 March 2022.
Edited by:
Yongli Wang, Institute of Geology and Geophysics (CAS), ChinaReviewed by:
Charles K. Lee, University of Waikato, New ZealandAlberto Saez, University of Barcelona, Spain
Copyright © 2022 Anslan, Kang, Dulias, Wünnemann, Echeverría-Galindo, Börner, Schwarz, Liu, Liu, Künzel, Kisand, Rioual, Peng, Wang, Zhu, Vences and Schwalb. This is an open-access article distributed under the terms of the Creative Commons Attribution License (CC BY). The use, distribution or reproduction in other forums is permitted, provided the original author(s) and the copyright owner(s) are credited and that the original publication in this journal is cited, in accordance with accepted academic practice. No use, distribution or reproduction is permitted which does not comply with these terms.
*Correspondence: Sten Anslan, c3Rlbi5hbnNsYW5AdXQuZWU=; Wengang Kang, d2VuZ2FuZy5rYW5nQHR1LWJyYXVuc2Nod2VpZy5kZQ==