- 1Geography Department, Humboldt-Universität zu Berlin, Berlin, Germany
- 2Department of Atmospheric and Cryospheric Sciences, University of Innsbruck, Innsbruck, Austria
In High Mountain Asia (HMA), rising temperatures and retreating glaciers are leading to the formation of new glacial lakes and the expansion of existing ones. The sudden release of water from such lakes can lead to devastating glacial lake outburst floods (GLOF) threatening people and infrastructure for many kilometers downstream. Therefore, information on future glacial lakes, e.g., their location, area and volume as well as the timing of their development, is vital for sustainable development of settlements and infrastructures. In this study, we present comprehensive estimates for future glacial lake development in HMA with unprecedented temporal resolution. We rely on an ensemble of fifteen global climate models using the newest CMIP6 data and employ a set of four Shared Socioeconomic Pathway (SSP) scenarios. With the Open Global Glacier Model (OGGM), we use a modeling framework that explicitly simulates glacier dynamics in order to model glacier change until 2100 and estimate the formation period for each of the 2,700 largest future glacial lakes (>0.1 km2) in HMA. We estimate the glacial lake area in the entire region to grow by 474 ± 121 km2 for SSP126 and 833 ± 148 km2 for SSP585. Following recent estimates of currently existing glacial lakes (>0.1 km2), this would constitute an increase in lake area of ∼120–∼210% in 2100 compared to 2018. The lake volume is expected to increase by 22.8 ± 6.7 km3 for SSP126 and 39.7 ± 7.7 km3 for SSP585. This range includes a drastic tenfold increase in lake volume, from estimated 3.9 km3 in 2018 to 43.6 ± 7.7 km3 in 2100. However, there is a considerable spread between total and relative increase in glacial lake area and volume for different sub-regions of High Mountain Asia. As both, lake area and lake volume, could to lead to an increase in GLOF risk, the results emphasize the urgent need for more localized, in-depth studies at especially vulnerable locations in order to enable local communities to adapt to emerging challenges, to implement risk minimization measures, and to improve sustainable development in High Mountain Asia.
1 Introduction
As a result of climate change, temperatures are rising globally causing glacial melting in nearly all glaciated regions of the world (Hugonnet et al., 2021). The acceleration of this phenomenon is especially visible in High Mountain Asia (HMA) (Lee et al., 2021), where glaciers are estimated to lose 29%–67% of their ice mass until 2100 (Rounce et al., 2020). As glaciers recede, one of the most apparent and dynamic impacts is the further growth of existing glacial lakes (Zhang et al., 2019; Shugar et al., 2020; Wang et al., 2020) and the formation of new glacial lakes (Haeberli and Weingartner, 2020; Zheng et al., 2021). This, too, is particularly evident in HMA. In this region, research has shown a substantially more negative mass balance for lake-terminating glaciers compared to land-terminating glaciers (King et al., 2019; Watson et al., 2020) and the world’s fastest long-term ice loss acceleration (Lee et al., 2021). Moreover, if the initial lake growth decouples from climate, glacial lakes can cause even more rapid melting due to their development at the termini of downwasting glaciers (Bolch et al., 2012; Trüssel et al., 2013).
On the one hand, glacial lakes can offer opportunities for use with regard to tourism, hydropower production or water supply (Haeberli et al., 2016; Farinotti et al., 2019b). On the other hand, they can substantially increase the hazard and risk potential for people and infrastructures downstream. This is especially the case for lakes dammed by abandoned moraines that are particularly prone to outbursts caused by rock or ice fall, internal erosion by piping or overflowing waves (Veh et al., 2020). The sudden discharge of water following a dam failure can develop into a catastrophic debris flow with ranges of over 100 km (Allen et al., 2016). Therefore, glacial lake outburst floods (GLOF) are among the most dangerous natural hazards in high mountain areas where they pose an enormous threat to people and infrastructures (Mohanty and Maiti, 2021). Particularly in HMA, these floods have had the highest death toll worldwide (Carrivick and Tweed, 2016). Therefore, hydrological and glaciological information on glacial lakes, e.g., their location, area and volume, the stability of their moraine dams and the surrounding slopes as well as the timeframe of their development, is crucial for hazard and risk assessments. These in turn are necessary for the installment of early warning systems and the employment of risk reduction measures (Haeberli et al., 2016; Veh et al., 2020).
So far, research has been concentrated primarily on mapping currently existing glacial lakes and quantifying lake development over the last decades. While Shugar et al. (2020) provided information on glacial lake growth on a global scale, several studies investigated parts of HMA (Zhang et al., 2019; Li et al., 2020; Wang et al., 2020; Chen et al., 2021). Other research concentrated on assessing the hazard potential of existing glacial lakes at a local (Lala et al., 2018; Sattar et al., 2021) or regional scale (Rounce et al., 2017; Veh et al., 2020). Otto et al. (2021) have evaluated the performance of ice-thickness models for the estimation of future lakes in Austria. Their study shows that the amount of subglacial overdeepenings alone may lead to an overestimation of the potential for future glacial lakes because overdeepenings may fill up with sediment rather than water. Some research has been conducted on the locations and morphological characteristics of future glacial lakes and their hazard potentials in parts of HMA (Allen et al., 2016; Linsbauer et al., 2016) as well as the entire region (Furian et al., 2021). Zheng et al. (2021) have provided a GLOF-risk analysis and a two-step overview on the timeframe of potential glacial lake development in 2050 and 2100. They employed data from phase 5 of the Coupled Model Intercomparison Project (CMIP5) and three Representative Concentration Pathway (RCP) scenarios in their work.
With this study, we provide a detailed analysis of future glacial lake development in HMA until the year 2100 with unprecedented temporal resolution. In order to be able to determine the timeframe of exposure of subglacial overdeepenings, we use a high-resolution glacier-by-glacier modeling approach. We employ 15 global climate models (GCM), each belonging to phase 6 of the Coupled Model Intercomparison Project (CMIP6) (Eyring et al., 2016; Balaji et al., 2018). To estimate glacier development following different future climate projections, we apply the Open Global Glacier Model (OGGM; Maussion et al., 2019), an open-source numerical model framework able to explicitly simulate glacier mass balance and glacier dynamics. To our knowledge, this study is the first to use an ice dynamical flowline model to study future glacial lakes. This approach increases the computation time and adds complexity to the model. However, it improves the representation of the ice flow and enables the localization of the glacier front, which is necessary for studying lake evolution at such a high spatial resolution. To account for different future socio-economic and political environments, we rely on an ensemble of four pathways from the new set of Shared Socioeconomic Pathway (SSP) scenarios, the successors to the previous RCP scenarios (Meinshausen et al., 2020).
Based on these projections, OGGM models the glaciers’ mass balance and, most importantly, estimates the position of the glaciers’ termini at a given point in time. We combine this information with recently published data on the location and morphology of subglacial bedrock overdeepenings (Furian et al., 2021). These overdeepenings, after becoming ice free, could potentially fill up with precipitation and melt water forming future glacial lakes. With this data, we are able to assign a time of formation to each future lake and, thus, provide a comprehensive temporally resolved transient overview on potential changes to overall glacial lake area and volume in HMA until 2100. While an in-depth analysis of potential moraine features would be beneficial to more accurately determine the potential retention volume of future glacial lakes (Haritashya et al., 2018), it is beyond the scope of this study. Instead, we combine the results with information about each future lake’s predisposition for mass-movement impacts that could trigger a GLOF (provided by Furian et al., 2021) in a synoptic analysis to identify potentially dangerous glacial lakes that are to form in the near future. This study presents a comprehensive overview on spatial and temporal future lake developments in HMA until 2100. The results form the basis for more localized approaches to further investigate locations at which a timely formation of potentially hazardous glacial lakes is likely to occur.
2 Study Area
According to the Randolph Glacier Inventory, version 6.0 (RGI v6), there are 95,536 glaciers in HMA covering an area of 97,605 km2 (RGI Consortium, 2017) which makes HMA the most heavily glaciated region outside of the Arctic and Antarctica (Brun et al., 2017). This multitude of glaciers allows the formation of numerous new and possibly dangerous glacial lakes (Shugar et al., 2020). At the same time, settlement density in HMA is expected to increase following the continued expansion of infrastructure into higher mountain ranges for, e.g., tourism, agriculture or hydropower (GAPHAZ, 2017). The RGI divides the glaciers of HMA into three subregions: Central Asia (zone 13), Southwest Asia (zone 14) and Southeast Asia (zone 15) (Pfeffer et al., 2014). While these zones work very well for global analyses, we additionally employ a more detailed dataset by Loibl (2020) based on glacier regions by the Global Terrestrial Network for Glaciers (GTN-G, 2017). This updated dataset more closely follows the main valleys instead of national borders to better separate orographic units and, thus, facilitate regional glaciological and climatological analyses. Figure 1 shows the distribution of glaciers, current and future glacial lakes in the different regions of HMA.
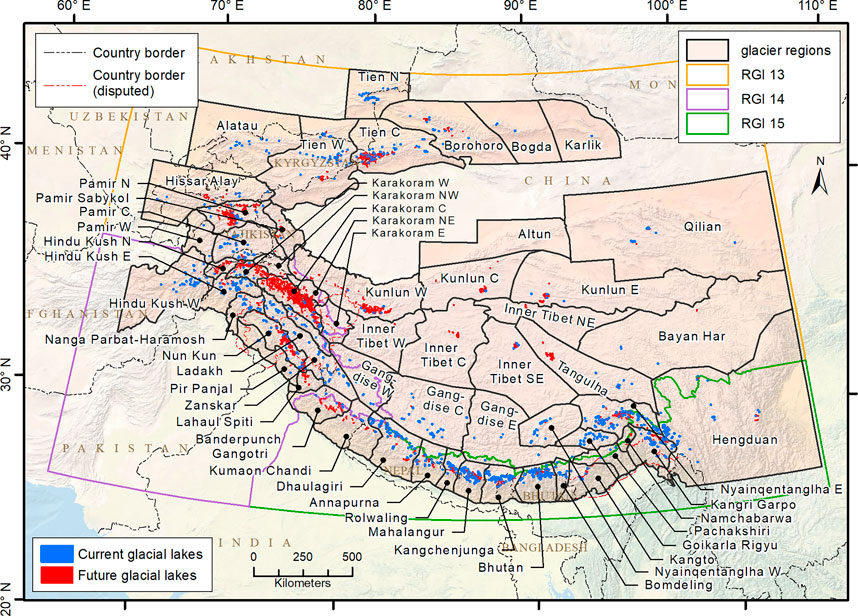
FIGURE 1. Overview of the study area with current glacial lakes (>0.05 km2, blue areas) by Shugar et al. (2020) and future glacial lakes (>0.1 km2, red areas) by Furian et al. (2021). Glacier regions (in orange) are taken from Loibl (2020) and international (disputed) borders are part of the World Bank Official Boundaries dataset (World Bank, 2020). For better visibility, glacial lakes are not depicted to scale.
Regarding its precipitation regime, HMA can be roughly separated into two regions. The northwestern part, including the western Himalaya, the Hindu Kush and Pamir, Karakoram and Tien Shan, receives about two thirds of its annual precipitation from mid-latitude cyclones of the westerlies. In the southern and eastern parts of the Himalaya, the Indian and East Asian summer monsoons provide approximately 80% of the annual precipitation (Bothe et al., 2012; Maussion et al., 2014). Depending on the chosen climate change scenario, annual mean precipitation over HMA is expected to increase by 4%–14% until 2100 with even stronger changes for the northern parts and especially in the Tien Shan (Huang et al., 2014; Jiang et al., 2020). Regarding HMA’s glaciers, the total annual glacier mass change was estimated to be –19.0 ± 2.5 Gt. yr−1 by Shean et al. (2020) for the period between 2000 and 2018. For the period from 2015 to 2019, the annual mass change rate is already estimated to be much higher at –30.2 ± 10.9 Gt. yr−1 (Hugonnet et al., 2021). Continued glacial wastage could lead to a loss in ice mass of up to 87% by 2100 (Kraaijenbrink et al., 2017; Hock et al., 2019a) which would expose many subglacial overdeepenings that could develop into lakes.
Regarding currently existing glacial lakes, a number of datasets are available covering the entire HMA region. In these datasets, estimates about the number of currently existing glacial lakes range from ∼15,000 lakes (Chen et al., 2021) to ∼26,600 lakes (Zheng et al., 2021), covering an area of 1,600 and 1,970 km2, respectively. While employing a minimum lake area threshold of 0.05 km2, Shugar et al. (2020) identify ∼2,200 lakes (1,123 of them larger than 0.1 km2). This underlines the fact that most glacial lakes are comparably small and, thus, more sensitive to climate change (Zhang et al., 2015). Regardless of the amount of identified lakes, all studies agree on the ongoing expansion of lake area, especially at the approximately 700 currently existing proglacial lakes, which have increased in area by 50% in the last 30 years (King et al., 2019; Tsutaki et al., 2019). Despite numerous challenges in modeling lake-glacier interactions (Carrivick et al., 2020), proglacial lakes play a significant role in assessing the formation of future glacial lakes because of their large influence on future glacier development (King et al., 2019). As previous studies have shown, a multitude of new proglacial lakes can be expected to develop in HMA over the next decades (Furian et al., 2021; Zheng et al., 2021). This expansion will further accelerate glacier retreat due to positive feedback loops between increased ablation at the terminus, lowered effective pressure, rising ice velocity and longitudinal strain (Carrivick and Tweed, 2013; King et al., 2018; King et al., 2019; Sutherland et al., 2020). As a result of a complete glacial retreat, a maximum of ∼25,000 new subglacial overdeepenings with a volume of ∼100 km3 could become exposed in HMA, covering an area of ∼2,700 km2 and potentially developing into glacial lakes (Furian et al., 2021).
3 Data and Methods
3.1 Input Data
To model the temporal evolution of potential future glacial lakes, we rely on several datasets. We use the RGI v6, a globally nearly complete inventory of glaciers containing information on, e.g., area, type, elevation, slope, length, and aspect of most glaciers for glacier outlines (RGI Consortium, 2017). The inventory of future glacial lakes in HMA by Furian et al. (2021) provides information on the morphology and impact hazard of subglacial overdeepenings, i.e., potential future lakes. For more information on the development of this dataset, we refer to the original publication (Furian et al., 2021). In short, this dataset was generated by subtracting glacier ice thickness (Farinotti et al., 2019a) from the ALOS World 3D 30 m digital surface model and analyzing the morphology of the resulting subglacial overdeepenings and the hazard potential of their surrounding slopes. The dataset contains information on the potential depth, surface area, and volume of potential lakes, inter alia. Due to its format, this dataset offers the possibility to use the morphological characteristics of each subglacial overdeepening to investigate when it will be partially or fully exposed. This way, we can determine not only when a lake may form completely, but also at what time partial lake formation may occur beforehand. This is especially important for large, elongated proglacial lakes, as their formation can extend over several decades.
Additionally, the dataset provides an assessment of the mass-movement impact predisposition for 2,700 potential future lakes with an individual lake area >0.1 km2, indicating possible GLOF hazard hotspots. Regarding the potential total future glacial lake area and volume in HMA, these lakes would account for ∼60% of the area (1,623 ± 515 km2) and ∼70% of the volume (72.6 ± 23 km3) (Furian et al., 2021). Lying beneath a total of 670 glaciers, these 2,700 potential future lakes comprise mainly the large, often proglacial, lakes in lower altitudes that could develop in the nearer future. Regionally, these lakes would then pose a major threat to infrastructure and settlements due to their large volume and close proximity.
We rely on an ensemble of 15 GCMs to model glacier evolution through 2100. The ensemble is obtained from the latest generation of climate models (CMIP6, Eyring et al., 2016) Klicken oder tippen Sie hier, um Text einzugeben. and was the largest ensemble we could retrieve at the date of acquisition (2021). Supplementary Table S1 comprises a full list of the GCMs including their availability for each of the four SSPs used in this study. We use for every GCM different climate scenarios, each following a different development path of human society on the basis of the SSP scenarios (Meinshausen et al., 2020). With our selection of scenarios, we follow the ScenarioMIP-agreement for CMIP6 (O’Neill et al., 2016) and employ four individual scenarios: SSP126, SSP245, SSP370 and SSP585. Each denotation indicates the basic pathway (e.g., SSP1, SSP2) as well as the additional radiative forcing that would be achieved in 2100. For example, SSP126 indicates the development into an increasingly sustainable world (SSP1) with an additional radiative forcing of 2.6 W/m2 in the year 2100. SSP126 is an improved version of the previously used CMIP5 scenario RCP2.6 and was designed to simulate a development in accordance with the 2°C target. On the other hand, SSP585 signifies the upper boundary of scenarios and describes a fossil-fueled world with high technological progress (SSP5) and an additional radiative forcing of 8.5 W/m2 in 2100. It can be described as an update of the CMIP5 scenario RCP8.5, combined with economic and social reasoning for its emission pathway and land use changes (Kriegler et al., 2014; Riahi et al., 2017).
3.2 Glacier Modeling
Based on the projected climate forcing, OGGM v1.4.0 (Maussion et al., 2021) was used to simulate the future mass balances and geometries of 670 individual glaciers in HMA with at least one large subglacial overdeepening according to data by Furian et al. (2021). OGGM is a state-of-the-art large-scale glacier modeling framework able to simulate glacier ice dynamics. For a detailed description of OGGM, we refer to Maussion et al. (2019) and the extensive documentation available online. In short, OGGM uses RGI v6 glacier outlines and topographical data and employs a geometrical routing algorithm to calculate glacier centerlines (Kienholz et al., 2014). Glacier “flowlines” are obtained from the centerlines using a previously specified grid spacing. In our case, we set the grid resolution to 100 m as a compromise between a preferably high resolution needed for the location of the glacier front and the large computational costs and uncertainties inherent to high resolution modeling. The geometrical widths at each grid point are then corrected with the catchment area along each flowline resulting in a close agreement between the flowlines and the actual altitude-area distribution of the glacier.
The mass balance model of OGGM is based on a modified version of the temperature index model by Marzeion et al. (2012). It simulates the historical glacier mass balance using monthly climate data interpolated to the location of the glacier, altitude-corrected at each grid point. In an updated version to Maussion et al. (2019), the mass-balance model now relies on the ERA5 atmospheric reanalysis as the reference climate (Hersbach et al., 2020) instead of CRU (Harris and Jones, 2013). The GCMs are bias-corrected for each glacier using a “delta method” (e.g., Zekollari et al., 2019) applied to the reference period 1979–2014 which signifies the largest overlap between the historical GCM simulations and ERA5. The mass-balance model is tuned to match the regional mass-balance estimates from Hugonnet et al. (2021). Similarly, OGGM’s ice thickness inversion module is tuned to match the regional ice volume estimates of Farinotti et al. (2019a).
In this study, OGGM is first used to simulate the historical mass balance and glacier geometry evolution starting at the last valid date of the RGI v6 glacier outline (ranging from 1998 to 2013) up to the year 2019 using ERA5 as forcing. Following previously selected projection scenarios, OGGM’s dynamical flowline model then simulates annual advances and retreats of glaciers until the year 2100 with a depth-integrated shallow ice approximation model (SIA) (e.g., Hutter, 1981, 1983; Oerlemans, 1997) using the physics of ice flow (Cuffey and Paterson, 2010; Jarosch et al., 2013). In order to avoid small numerical fluctuations at the terminus, OGGM computes the main flowline length using a minimum ice thickness threshold of 1 m. Model outputs include files for the different main and contributory flowlines of every glacier in HMA as well as data files providing information on, e.g., glacier volume, area, ice thickness near the terminus, and main flowline length (see Data Availability Statement).
3.3 Lake Formation
Of the annual OGGM data, we selected a decadal resolution for the lake formation analyses, therefore our time series starts in 2030 and ends 7 decades later in 2100. The decadal resolution is a considerable improvement compared to the temporal resolution of the study by Zheng et al. (2021), who modeled glacial lake development at only two time steps: 2050 and 2100. With the output of OGGM, we can identify the position of the terminus for each glacier every decade. While this resolution does not allow us to determine the exact timing of the detachment of the retreating glaciers from the proglacial lakes, the information about the terminus location enables us to spatially relate glacier fronts to the subglacial depressions. Thus, we can identify partially or fully exposed overdeepenings in the bedrock that could fill up with precipitation and meltwater. Using GIS software (ESRI, 2019), we intersect the glacier flowlines produced by OGGM with the spatial data on potential future glacial lakes larger than 0.1 km2 (Furian et al., 2021). The threshold of 0.1 km2 was chosen because 1) larger lakes are more likely to actually form and are subject to lower uncertainties (Haeberli et al., 2013) and 2) these 2,700 lakes represent about two-thirds of the area and volume of all 25,000 potential glacial lakes (Furian et al., 2021). This means that the remaining thousands of smaller lakes would have a lower probability of developing, but at the same time would increase the computational cost several fold. Therefore, this threshold value serves as a compromise between the highest possible resolution and a manageable computation time.
At decadal intervals and using the glacier’s respective main flowline length, we investigate glacier retreat and subsequent exposure of bedrock overdeepenings. We approximate the shape of the terminus by creating a line perpendicular to the smoothed last 250 m of flowline (Gabrisch, 2011). Due to this generalization, we avoid artifacts in the terminus orientation due to small changes in direction of the main flowline. Finally, we investigate whether a subglacial overdeepening is intersected by the terminus line and calculate the exposed part of the overdeepening as a proxy for a partially formed proglacial lake. To account for increased frontal ablation from such a proglacial lake, we take into consideration the ice thickness of the glacier tongue. If the ice thickness at the terminus is smaller than the depth of the underlying overdeepening, i.e., if the glacier tongue lies completely inside the bedrock overdeepening, it can be assumed that water stored in the overdeepening would greatly increase glacial retreat (King et al., 2019). In this case, we create a new terminus line at the next grid point up the glacier’s main flowline and repeat the previous steps. If the elevation of the glacier terminus is greater than the surface elevation of any of the remaining overdeepenings in the glacier bed, those overdeepenings are considered to be fully exposed. With the terminus retreating to higher elevations over time, more and more overdeepenings will become partially or fully exposed and can fill up with precipitation and meltwater.
3.4 Uncertainty Assessment and Data Quality
In general, research into future glacial lakes is subject to considerable uncertainties, which in this case are mainly determined by four factors: 1) the CMIP6 models and the SSP scenario data, 2) the OGGM model runs, 3) the dataset of future glacial lakes by Furian et al. (2021), and 4) the glacier front approximation. Consistent with current large-scale glacier modeling and climate-impact studies (e.g., Marzeion et al., 2020; Rounce et al., 2020), the uncertainty range quantified in this study is based on the GCM and SSP scenarios. GCM uncertainty is expected to play a large role in the coming decades, while scenario uncertainty, which is comparatively small at the beginning, increases over time and accounts for the larger share of the overall uncertainty towards the end of the 21st century (Marzeion et al., 2020). To account for other effects, which are difficult to quantify and are described further below, we have taken the pragmatic approach to inflate the uncertainty range by 10%. This number is arbitrarily chosen, but in our opinion provides a reasonable approximation of the total uncertainty.
CMIP6 performance varies over the different parts of HMA (Guo et al., 2021). In general, CMIP6 models show a systematic cold bias over the Tibetan Plateau, underestimating observed temperatures (You et al., 2021). However, since several of our models (e.g., EC-Earth3-Veg, MPI-ESM1-2-HR, CAMS-CSM1-0) simulate temperature over the entire HMA very well (Zhu and Yang, 2020), and due to the bias correction performed by OGGM, this is not expected to have a significant impact on the results. Among our ensemble are some of the best rated models for simulating precipitation over HMA: CESM and CESM-WACCM proved to be some of the best models for the entire region, while EC-Earth3, EC-Earth3-Veg, GFDL-ESM4 and FGOALS-f3-L were ranked among the best suited models for the southern and southwestern parts of the Himalayan arc (Guo et al., 2021). EC-Earth3 and EC-Earth3-Veg also outperform other models in simulating precipitation extremes (Dong and Dong, 2021). Following these model performance estimates, we expect our model ensemble to fairly well represent temperature and precipitation over HMA. As can be seen in Supplementary Figures S1, S2, model runs using EC-Earth3 and EC-Earth3-Veg data result in comparably high values for glacial lake area and volume. However, due to the overall comparably high-performance rating for both the EC-Earth3 and the EC-Earth3-Veg model, we refrain from excluding their results.
Uncertainties in glacier models are expected to be largest in the first half of the century with respect to uncertainties related to GCMs and climate scenarios (Maussion et al., 2021). For OGGM, the mass balance estimates can be assumed to be reasonable due to the large-scale application over HMA (Farinotti et al., 2017; Maussion et al., 2019). However, processes affecting ice thickness introduce uncertainties. Although the employed parameters are a good compromise for large-scale applications (Maussion et al., 2019), they may lead to overestimated ice volume (Farinotti et al., 2019a). Debris cover on glaciers, which considerably influences glacier mass balance (Rounce et al., 2021), is often investigated at a local scale (Anderson and Anderson, 2018; Rowan et al., 2021) and has only recently been incorporated into a global glacier model (GloGEM, Compagno et al., 2021). Because debris cover can both accelerate and reduce ice loss, we cannot comment at this point on the extent to which debris cover will influence glacial lake formation in HMA in the 21st century. While the comparatively high grid resolution of the flowlines allows for a better estimation of the terminus position, it may in some cases lead to numerical errors during the glacier modeling. In our case, 5% of all glaciers could not be modeled, resulting in an underestimation of glacial lake area and volume increases. For some glaciers, spin-up problems complicate the first few years of the modeling process which can lead to initial glacier growth that is not to be expected in reality. Thus, the OGGM results up to the year 2030 are, in some cases, to be viewed as conservative. In our study, we only consider glacier length changes relative to the RGI outlines, which are assumed to be well constrained. Length changes are a result of past climate and ice-dynamical processes, the latter being very poorly constrained due to the lack of observational data, and the high level of parameterization in OGGM.
Some additional uncertainty regarding the extent of the glacier retreat originates from proglacial lakes, which play a significant role for glacier mass loss in HMA (Brun et al., 2017; King et al., 2019). Studies (ours included) predict the development of more proglacial lakes during the 21st century (Furian et al., 2021; Zheng et al., 2021) and show that nearly all of the larger glaciers in HMA will either have new proglacial lakes formed in the future or have the already existing proglacial lakes enlarged. However, the feedback loop between a proglacial lake and glacier melt is not currently represented by OGGM (or any large-scale model). This suggests that ablation at the glacier terminus may be underestimated for many of the larger glaciers, which in turn may indicate that lake development may be happening at an even faster pace than presented here.
The future glacial lakes dataset by Furian et al. (2021) is based on ice thickness estimates, which themselves are subject to considerable uncertainties (Farinotti et al., 2017). However, ice thickness models generally are robust in detecting the location and estimating the shape of subglacial overdeepenings (Linsbauer et al., 2012; Linsbauer et al., 2016). Drawing conclusions about future lakes actually forming from overdeepenings is difficult, but appears to be more consistent for valley glaciers (Otto et al., 2021). For our study, valley glaciers are indeed more important, as we concentrate on large future lakes that develop mostly beneath those glaciers. Nevertheless, future lake area and volume depend on dam composition and geometry as well as the existence of an outlet channel—all of which can hardly be determined for future glacial lakes (Furian et al., 2021; Zheng et al., 2021). For this reason, it is unclear how many of the potential lakes studied here will actually develop. Possibly, the results of this study represent an upper limit with respect to the number, area and volume of glacial lakes. The applied method to estimate glacier terminus morphology is very basic as it represents the glacier front as a straight line perpendicular to the general orientation of the main flowline. Therefore, the morphologies are a rough approximation of the potential future reality. However, they enable identifying partially developed glacial lakes and assessing their area and volume as well. In calculating the glacier front, we use the main flowline and follow Zheng et al. (2021) in assuming that all other parts of the glacier lower than the glacier front have completely melted. While this is presumably a valid assumption for many glaciers, there could also be glaciers where tributary glacier branches or dead-ice remnants at the same altitude melt less rapidly due to local valley morphology and debris cover. For example, this could imply that, on the one hand, some of the smaller lakes in side valleys could possibly form later than modeled. On the other hand, the remaining ice from tributary valleys could also dam up new lakes made up of meltwater from the main glacier.
4 Results
4.1 General Findings
Using a 15-GCM ensemble and four different SSP scenarios, we simulate the development of 670 glaciers in HMA during the 21st century. In the bedrock of these glaciers, a total of 2,700 subglacial overdeepenings could potentially develop into glacial lakes with an individual surface area larger than 0.1 km2. Figure 2 provides an overview of the results. Our results show an increase in the number of glacial lakes by the end of the 21st century for the entire HMA region. This is accompanied by an increase in both glacial lake area and glacial lake volume in the coming decades which confirms the results of previous studies (e.g., Shugar et al., 2020; Zheng et al., 2021). For the sake of clarity, individual model runs are not shown. Instead, each bold colored line represents the mean of the results from the 15-GCM ensemble. The standard deviation of the GCMs is shown as the 1-sigma uncertainty range by the shaded areas. For more information on the individual results of all GCMs under each SSP scenario, see Supplementary Figures S1, S2.
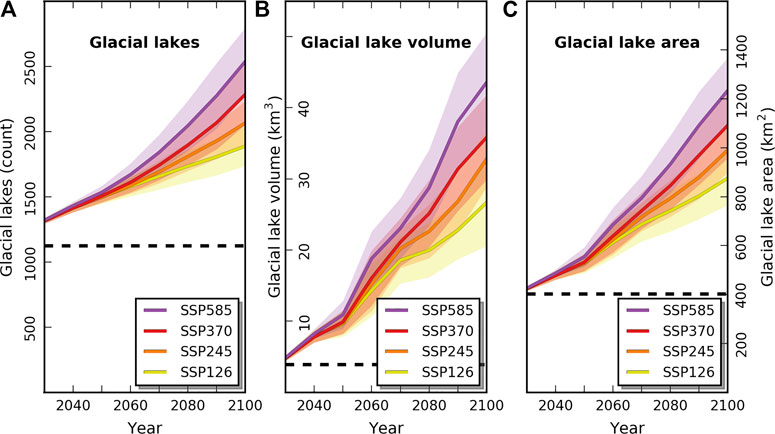
FIGURE 2. Overview of the development of (A) the number of glacial lakes (>0.1 km2) in HMA, (B) their volume, and (C) their area under four SSP scenarios until 2100. Main colored lines represent the mean of an ensemble run of 15 CMIP6 GCMs, while the shaded areas indicate the increasing ensemble standard deviation over time. The dashed black lines indicate the state of glacial lakes (>0.1 km2) in 2018, using estimates by Shugar et al. (2020): 1,123 glacial lakes with 3.9 km3 of volume and 402 km2 of area.
In Figure 2, we include results of Shugar et al. (2020) as a benchmark, representing the state of glacial lakes in HMA in 2018 (indicated by the dashed black lines). In their study, they use a threshold of 0.05 km2 and identify ∼2,200 existing lakes (476 km2, 4.6 km3). To allow meaningful comparisons, we filtered their results using our threshold of 0.1 km2, which leaves 1,123 glacial lakes with an area of 402 km2. Since in this dataset lake volume is given only as an HMA-wide value and does not appear on a glacier-by-glacier basis, we must resort to estimates in this regard. Calculations using empirical relationships between lake area and volume (Evans, 1986; Huggel et al., 2002) yield unrealistically high values for lake volume. Therefore, and although we are aware that it is a simplistic approach, the rule of three seems a feasible way to estimate the volume of the remaining 1,123 lakes at 3.9 km3. To the filtered results of Shugar et al. (2020), we add the increases in number, area, and volume of glacial lakes modeled in this study. Thus, Figure 2 displays our results for the future evolution of glacial lakes in context with the current situation in HMA. For each SSP scenario, Figure 2 shows a continuous increase of the number of exposed overdeepenings, i.e., potential glacial lakes and, related to this, an increase in glacial lake volume and area. Glacial lakes (>0.1 km2) show an increase of between 68% (SSP126) and 126% (SSP585) in 2100, considering the 1,123 glacial lakes (>0.1 km2) existing in 2018 modeled by Shugar et al. (2020). Differences between individual scenarios only become apparent after 2050 due to the consistent increase of ∼33% by 2050 under all SSPs (Table 1).
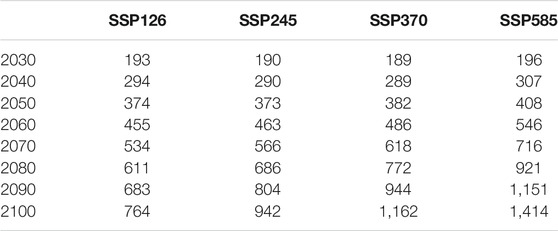
TABLE 1. Increase in the number of glacial lakes (>0.1 km2) in HMA under four SSP scenarios, represented by a 15-GCM ensemble mean.
When comparing lake area to lake volume in Figure 2, the difference in the magnitude of the increase is most noticeable. Following the SSP585 scenario, glacial lake area in HMA could almost triple by the year 2100. In the same period, however, glacial lake volume could show a tenfold increase. This difference between area and volume is also evident in the other scenarios, albeit in a somewhat weakened form. As for the spread inherent in the model ensemble, an increasing standard deviation is evident over the decades. This is more pronounced for the lake volume, which also shows a less linear increase over the decades compared to lake area. In Table 2, the absolute and relative increases in lake area and volume are listed for each SSP scenario. Until the mid-21st century, most GCMs agree in simulating the increase in glacial lake area and volume (for more details see also Supplementary Figures S1, S2). Further, up to this point, the results for the first three SSP scenarios (SSP126, SSP245 and SSP370) are nearly identical, and the values for SSP585 are also only slightly higher.
In the second half of the 21st century, however, the differences become more pronounced. According to the SSP126 scenario, HMA could gain 474 ± 121 km2 of additional glacial lake area and 22.8 ± 6.7 km3 of additional lake volume by 2100 from lakes >0.1 km2. This means that even with stringent climate policies, HMA could experience an increase of ∼120% in glacial lake area and up to almost 600% in glacial lake volume in 2100 compared to 2018. Extrapolating the past and current global development into the future using the “Middle of the Road” scenario SSP245, glacial lake area could increase by ∼150% and glacial lake volume by ∼750% until 2100. In the SSP370 scenario of increasing global competition and decreasing environmental awareness, glacial lake area could increase by ∼170% and glacial lake volume by ∼820% until 2100. Under a continuously fossil-fueled environment in the SSP585 scenario, HMA could see an increase of more than 200% in glacial lake area (833 ± 148 km2) and of ∼1,000% in glacial lake volume (39.7 ± 7.7 km3) until 2100.
4.2 Regional Differences
4.2.1 Glacial Lake Volume
When considering the distribution of future glacial lakes in HMA, the heterogeneity of this region becomes apparent. Figure 3 illustrates these spatial contrasts in terms of glacial lake volume. In this figure, the increase in lake volume as a function of the four SSP scenarios is shown as a bar graph for selected regions in HMA. The different coloring and shading of the bar diagrams highlights the spatial and temporal heterogeneity of the glacial lake development in HMA. In order to represent the temporal development of the future glacial lakes during the 21st century as accurately as possible while maintaining clarity, three points in time were chosen: 2040, 2070, and 2100. A numerical representation with higher temporal resolution for all sub-regions of HMA can be found in Supplementary Tables S2A–H.
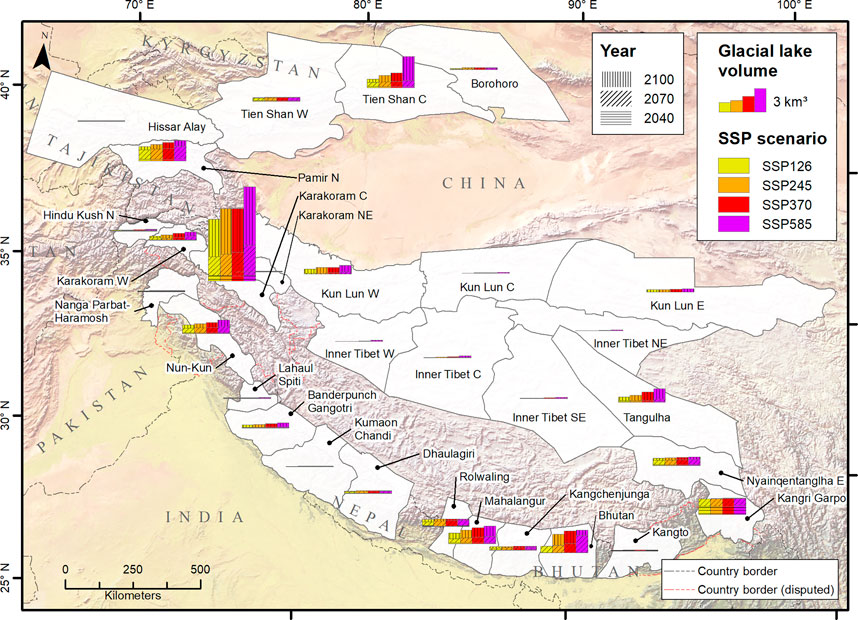
FIGURE 3. Glacial lake volume increase in HMA under four SSP scenarios up to three points in time during the 21st century. SSP scenarios are color-coded, years are indicated by different dashes. Regions with <0.1 km3 of additional lake volume in 2100 (SSP585) are excluded for reasons of clarity.
For most regions, the increase in glacial lake volume shows only minor differences when comparing the four SSP scenarios. In the Central Karakoram and the Tien Shan, however, the difference between the lower scenarios and the SSP585 scenario is more striking. In the southeastern parts of HMA, the increase in lake volume is considerable (1 km3 in Nyainqentanglha East and 2 km3 in Kangri Garpo in 2100), but there is almost no difference between the individual SSP scenarios. Further, the development of future glacial lakes in these regions appears to be occurring primarily in the first half of the 21st century. Moving west, the regions of Bhutan (2.9 km3) and Mahalangur (2.2 km3), as well as the Nun-Kun (1.7 km3) and the western parts of the Kun Lun (1.1 km3) show an increase in glacial lake volume of roughly the same order of magnitude. Here, however, the differences between the SSP scenarios are more clearly visible and the increase in volume is centered on the second half of the century. For large parts of the Tibetan Plateau, only a negligible increase in glacial lake volume is expected in the 21st century. In the northern and northwestern parts of HMA, the Tien Shan (3.9 km3) and the Pamir (2.6 km3) show a substantial increase in glacial lake volume, with visible increments from SSP126 to SSP585. Finally, the Central Karakoram region shows a disproportionately larger increase in glacial lake volume. Even under the moderate SSP126 scenario, lake volume in the Central Karakoram region increases by 0.7 km3 until 2040, to almost double in the following 10 years to 1.3 km3 in 2050. According to our modeling, an increase of 3.1 km3 can be expected in 2070 and by 2100, the increase could have reached 7.7 km3. With higher SSP scenarios, the modeled increases in glacial lake volume are also higher, and may reach 11.8 km3 for the SSP585 in 2100.
4.2.2 Glacial Lake Area
The close connection between glacial lake area and volume has been previously studied (Cook and Quincey, 2015; Veh et al., 2020). Therefore, it is not surprising that the spatial pattern of glacial lake area increases during the 21st century strongly resembles that of glacial lake volume. Figure 4 shows the spatial distribution of the modeled lake area increases in HMA for the four SSP scenarios. The previously described pattern prevails: in the southeastern Himalaya (especially in Nyainqentanglha East and Kangri Garpo), the increase in glacial lake area mostly happens in the next 50 years. Further, in these regions, it seems to make little difference whether a low- or high-emission scenario is used as the basis for the modeling. Moving westward along the Himalayan arc, in most regions the increase in glacial lake area in the 21st century is less than 25 km2, even under the SSP585 scenario. On the Tibetan Plateau, the increase is very small under all scenarios. Similar to lake volume, the Central Karakoram region is also a hotspot in the growth of glacial lake area. Our results indicate a potential increase in lake area of 16 km2 in the next 20 years, regardless of the SSP scenario. For later decades, the changes are increasingly linked to the chosen scenario. In 2070, glacial lake area in this area could grow between 58 km2 (SSP126) and 86 km2 (SSP585). Towards the end of the century, the increase in glacial lake area then ranges between 120 km2 (SSP126) and 200 km2 (SSP585).
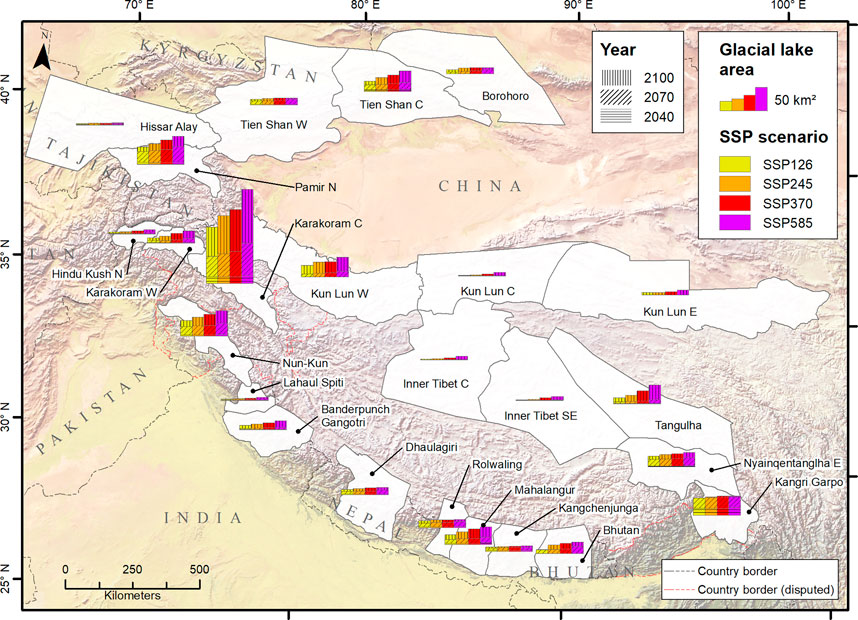
FIGURE 4. Increase of glacial lake area in HMA under four SSP scenarios up to three points in time during the 21st century. SSP scenarios are color-coded, years are indicated by different dashes. Regions with <5 km2 of lake area increase in 2100 (SSP585) are excluded for reasons of clarity.
4.3 Relation to Current Glacial Lakes
Compared to the spatial pattern of absolute increase in glacial lake area and volume, the spatial distribution in relative terms stands out even more. To illustrate this pattern, we again use the results of Shugar et al. (2020) as a baseline, which provide a good representation of the current status of major glacial lakes in Central Asia. Given the amount of current large glacial lakes in the southern and southeastern regions of HMA, the relative increase in glacial lake area in these regions is comparatively small. Nevertheless, it is substantial, with an increase of, for example, ∼50% in Bhutan, ∼70% in Nyainqentanglha or 110% in Mahalangur (following the SSP585). On the other hand, the Central Karakoram could see an increase in glacial lake area of >5,000%, even under a comparably sustainable scenario (SSP126). With a more fossil-fueled scenario (SSP585), the increase could rise to ∼8,500% in 2100. In some of the other regions, while the relative increase may still be significant, this is mostly due to the relatively small lake area in 2018 (e.g., in the Northern Hindu Kush, the Central Kun Lun or Inner Tibet). For a more detailed view at the absolute and relative increase in lake area per region, see Supplementary Table S3.
Our results indicate a potential for the emergence of about 400 additional future proglacial lakes in 2100 (Figure 5). About 16% (69 lakes) of the potential future proglacial lakes found by our model ensemble were already either fully or partially developed in 2018, according to glacial lake data from Shugar et al. (2020). In most cases, these lakes represent the area increase of already existing proglacial lakes due to glacial retreat. In some cases, this indicates a considerable glacier retreat since the date of recording of the RGI glacier outlines. In Supplementary Figure 3, we chose four lakes to represent (A) realistic estimates as well as (B) overestimations and (C, D) underestimations of potential lake area. The majority of the already (partially) developed proglacial lakes are located in the southern and southeastern part of the Himalaya (Mahalangur, Nyainqentanglha E and Kangri Garpo), while there are much fewer in the still heavily glaciated areas of Karakoram, Pamir and Tien Shan.
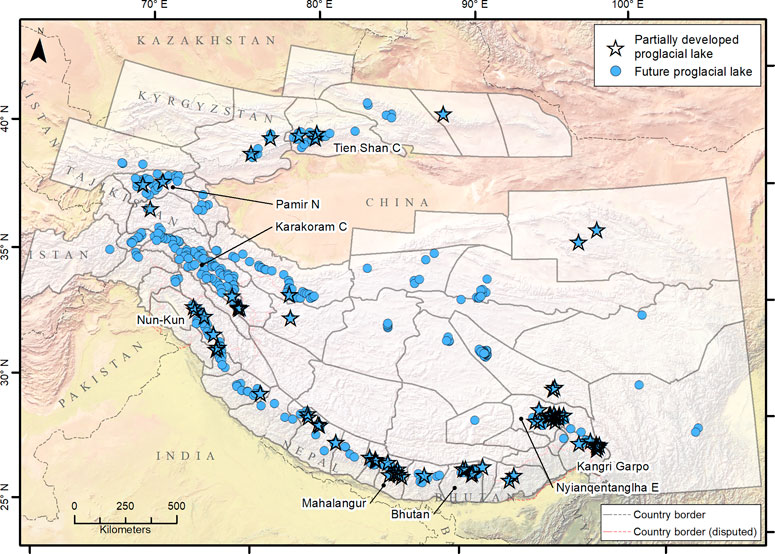
FIGURE 5. Future proglacial lakes (n = 415) in 2100. Stars (n = 69) indicate locations where modeled proglacial lakes are already forming, according to the comparison of our data with Shugar et al. (2020).
5 Discussion
5.1 Development of Glacial Lake Area and Volume
The modeled increase in glacial lake area and volume may indicate an increasing potential for the touristic or infrastructural use of glacial lakes on the one hand, but it also shows the possibility of an increase in the risk of GLOF events (Farinotti et al., 2019b; Zheng et al., 2021). Under all SSP scenarios, although with increasing intensity under the higher scenarios, communities in many regions of HMA will have to face the ongoing expansion of existing glacial lakes as well as the formation of numerous new and unmonitored ones during the next decades. Increasing precipitation over Central Asia (Jiang et al., 2020) will provide additional surface runoff to fill exposed subglacial overdeepenings, while increasingly frequent extreme precipitation events (Zhang et al., 2020) could damage moraine walls and lead to an increase in rock slides or rock falls triggering GLOFs. As glacial melt continues, in most cases the first lakes to enlarge or form will be the proglacial lakes. Some of those lakes could coalesce with already existing lakes forming even bigger and potentially more hazardous glacial lakes. Based on previous assessments of future glacial lakes (Allen et al., 2016; Furian et al., 2021), the hazard potential of these lakes can be estimated as the predisposition of the lakes to mass-movement impacts that could trigger a displacement wave capable of producing a GLOF event. Unfortunately, although the large proglacial lakes are often located at lower altitudes and thus surrounded by less steep slopes, their size makes them highly susceptible to mass-movement impacts from rock or ice falls. At the same time, their often-large volume and their relative proximity to infrastructure and settlements can lead to devastating floods in case of a GLOF. Thus, they were generally ranked as the most hazardous future glacial lakes in a previous study by Furian et al. (2021), Klicken oder tippen Sie hier, um Text einzugeben. By combining their hazard classification with the modeled timeframe of lake development, we can draw conclusions about the potential impact predisposition of those lakes that will emerge in the coming decades. The results suggest that the proglacial lakes that will form first are simultaneously assigned a high hazard category in terms of impact predisposition. The defining spatial feature appears to be the gradient between the current GLOF hotspots in the southeastern Himalaya mountains and the modeled potential future GLOF hotspot of the Central Karakoram (Furian et al., 2021; Zheng et al., 2021). While the southwestern regions already have a high number of glacial lakes, large glacial lakes in the Karakoram are still relatively sparse due to the comparatively high glaciation (Veh et al., 2020).
5.1.1 Southeastern Regions
The current hotspot of glacial lake development and, relatedly, the hotspot of GLOF events mostly comprises the regions of Mahalangur and Bhutan in the central Himalayas and Nyainqentanglha E and Kangri Garpo in the east (Veh et al., 2019). In these parts of HMA, due to high ablation rates over the last decades, most glacial lakes have developed at the terminus of glaciers, accelerating the melting processes even further (Gardelle et al., 2011). As can be seen in Figure 6, the influence of the chosen pathway scenario on the lake development varies from region to region. In Mahalangur and Bhutan, while there will be substantial increases in glacial lake volume and area even when following the lower SSP126 scenario, a higher SSP scenario also means larger lakes and a higher lake volume. This indicates that the high potential for deglaciation and subsequent lake formation is not exploited with lower SSP scenarios. Following SSP126, in 2100, Mahalangur will see an increase in glacial lake area by 21 km2 and in volume by 1.3 km3. In Bhutan, lake area could increase by 9 km2 and lake volume by 0.5 km3. Under higher scenarios, more and larger lakes form until these numbers would approximately double in Mahalangur (38 km2 and 2.2 km3) and increase even more in Bhutan (25 km2 and 2.9 km3), if the global climate changes more in line with SSP585.
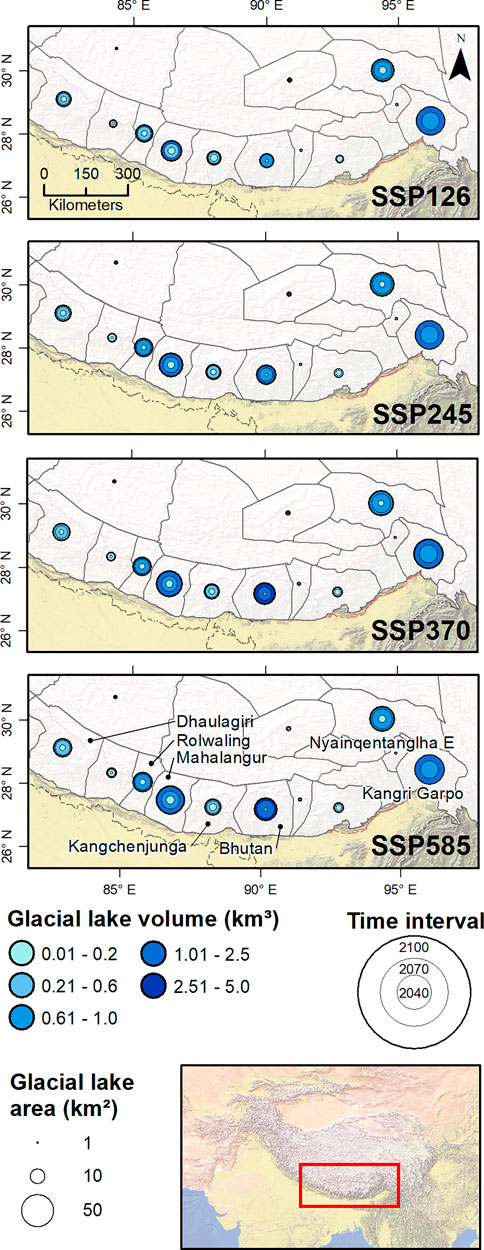
FIGURE 6. Glacial lake formation under four SSP scenarios in the southwestern part of HMA. The three timesteps (2040, 2070, and 2100) indicating the development throughout the 21st century are indicated by three overlying circles, and with circle size and blue color scale, lake size and lake volume are described.
In the results, we have shown that the ratio of future lake area to future lake volume is different in these two regions than in most of HMA. The relatively high volume with a comparatively small area indicates that mainly deeper lakes could form here. On the one hand, smaller lake areas could indicate a decreased predisposition to mass-movement impacts. On the other hand, it can be assumed that these lakes silt up less quickly and their large retention volume could pose a greater threat in the event of a GLOF. Thus, these results indicate a significant challenge to the rural communities of these regions. While those communities contribute to climate change only to a very small extent, they will nevertheless suffer disproportionately from its impacts (Reyer et al., 2017). Our results show that it makes quite a difference to these regions which (and how serious) global efforts to mitigate climate change are. In many regions of the southeastern HMA, adaptive strategies to meet future challenges in agriculture and hydropower are implemented (Ahmed et al., 2019; Chhogyel et al., 2020). However, such measures will be complicated by two factors: 1) Uncertainties about the SSP-dependent future lake development will further fragment and complicate the already incoherent adaptation efforts in these regions (Ahmed et al., 2019). 2) The comparatively small changes in lake area before 2040, especially in Bhutan, could reduce the urgency of adaptation measures. However, the large increase in lake area and volume after 2040 will make a delayed implementation of these measures more difficult.
As can be seen in Figures 3, 4, in other parts of the southeastern HMA (most noticeably in Nyainqentanglha E and Kangri Garpo, and somewhat less pronounced in Dhaulagiri, Rolwaling, and Kangchenjunga), the differences between the respective SSP scenarios regarding the increase in glacial lake area and volume are marginal. Because of the very negative glacier mass balance in these regions (Brun et al., 2017), it appears that the SSP126 scenario would be enough to melt the remaining glaciers sufficiently to exhaust the potential for further glacial lake development. Thus, under higher SSP scenarios, there would be very little further lake growth possible. This means that—at least in this respect—it would be irrelevant for the population of these regions which pathway the global climate would follow in the 21st century. This may provide some much-needed certainty about future lake development. Without the need to compare the respective current climatic situation with different SSP scenarios in order to then derive potential lake development, local communities can plan and strategize for the future with greater certainty. Consequently, upcoming more regionally or locally oriented studies may instead concentrate on detailed hazard assessment of the future lakes and support communities in this way.
While in most regions the development of glacial lakes will be more or less continuous throughout the 21st century, the Tibetan region of Kangri Garpo stands out in terms of the temporal aspect of lake development. Here, according to our modeling, almost half of the future lake development in the 21st century will be already completed by 2040. This would represent an increase in glacial lake area of nearly 20 km2 and glacial lake volume of ∼1 km3 over the next 20 years. The second half of the increase is likely to be completed before 2070. Thus, communities in Kangri Garpo could see an increase in glacial lake area of up to 42 km2 and in lake volume of 2 km3 over the next 50 years, regardless of the SSP scenario. According to the region’s glacial lake area in the Hi-MAG database by Chen et al. (2021) in 2017, this would indicate an increase of 20% in lake area until 2040, and of almost 50% in the next 50 years. Especially in this region, there is an imminent need for regional studies into future glacial lakes and the associated potentials and risks, as such a prompt change in the periglacial landscape would pose significant challenges for this remote region with its few rural communities.
5.1.2 Northern and Northwestern Regions
The northern and northwestern regions of HMA (i.e., the Pamir, Tien Shan, and the Karakoram) are currently still heavily glaciated, at least compared to the southeastern part of HMA (Brun et al., 2017). Over the last decades, melt water and precipitation has mostly accumulated in the form of numerous small supraglacial lakes, although larger proglacial lakes are starting to develop (Gardelle et al., 2011; King et al., 2019). However, because the period of glacier ice increase has passed here as well and the mass balances of most glaciers are becoming increasingly negative (Brun et al., 2017), lake formation can potentially occur on a much larger scale. Our results show that mountain ranges in this region have an enormous potential for the formation of large glacial lakes, even under a comparably mitigated global warming scenario (Figure 7). This means that due to the later formation of lakes, there are no areas where the lake formation is decoupled from the SSP scenarios, like it is the case in Bhutan and Mahalangur. In all of this part of HMA, the chosen pathway scenario plays a prominent role for the future development of glacial lakes.
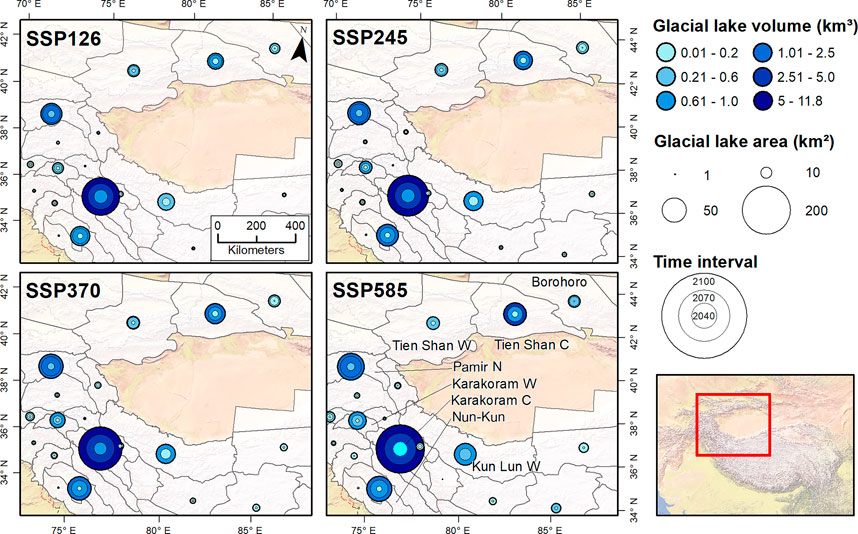
FIGURE 7. Glacial lake formation under four SSP scenarios in northwestern HMA. The three timesteps (2040, 2070 and 2100) indicating the development throughout the 21st century are indicated by three overlying circles.
In the central Tien Shan, glacial lake area would increase by ∼22 km2 under the SSP126 scenario or by 44 km2 under the SSP585 scenario, and lake volume would increase by ∼1 km3 (SSP126) or almost 4 km3 (SSP585), respectively. The conditions in the northern Pamir are similar ranging from 37 km2 to 1.8 km3 (SSP126) up to 60 km2 and 2.6 km3 (SSP585). In both regions, changes in temperature and precipitation patterns under higher SSP scenarios would exacerbate the lake formation processes. In both regions, lake formation can be expected to occur mainly between 2040 and 2070. The communities of these mountain ranges are already disadvantaged due to their remoteness, the poor infrastructure, and the difficult access (Xenarios et al., 2019). Thus, added environmental pressure would only aggravate the situation, if adaptation initiatives and policies are not implemented in a timely manner. Our results can serve as a basis for further research that should investigate potentially threatening future lakes and subsequently develop strategies to improve the vulnerability status of the related communities. In the Kun Lun region, especially in its western parts, a majority of glaciers are still maintaining a positive mass balance (Brun et al., 2017). This is reflected by our results, as under all SSP scenarios, substantial lake formation does not start before 2040. After that, accelerated glacial retreat can be expected which could lead to the formation of a number of glacial lakes due to the comparatively high glaciation. Thus, in this region, communities have the rare opportunity to develop adaptation concepts and implement strategies before the problem starts to fully evolve.
The region of the central Karakoram is transitioning from a positive to a negative overall mass balance as the so-called “Karakoram Anomaly” is shifting eastward towards the Kun Lun (Brun et al., 2017). Nevertheless, due to the high remaining glacier ice extent, lake formation could increase drastically even under the SSP126 scenario with additional 120 km2 of glacial lake area and 7.7 km3 of glacial lake volume or 202 km2 and 11.8 km3 under the SSP585 scenario. Thus, our modeling supports the result of Zheng et al. (2021) that the Karakoram is the hotspot of future glacial lake development in HMA and, thus, also a potential future GLOF hotspot. The current glacier response to global warming in this area is a combination of ice thinning and rapid ice advance, leading to an increase in glacier surges (Bazai et al., 2021). As surge events lead to a higher probability of glacial lakes forming behind unstable ice dams (Hewitt and Liu, 2010), GLOF are among the predominant natural hazards in this region (Ashraf et al., 2021). The high number of future proglacial lakes (see also Figure 5) underscores this as a progressive trend. The large amount of surging glaciers would imply that future glacial lakes in the Karakoram are at a higher risk of creating GLOFs than in other parts of HMA, especially if lakes are located at the terminus (Bazai et al., 2022). Many studies report GLOFs produced by glacier surges (e.g., Round et al., 2017; Hock et al., 2019b; Yin et al., 2019; Khan et al., 2021). Both surge events and GLOFs are closely coupled with the climate evolution over a time-span of several decades. Studies indicate that, while there is currently no clear increasing trend of the number of GLOFs, both glacier surges and GLOFs are predicted to become more frequent in the next decades (Harrison et al., 2018; Bazai et al., 2021).
Due to the sparse population density of the Karakoram, is it possible that a significant part of the increase in lake area and volume does not impact any community, infrastructure or agricultural land. However, the few and sparse communities that are under threat are highly vulnerable when they are affected. Our results show that lake formation is occurring increasingly rapidly during the 21st century, making the next years until 2040 crucial for the development and implementation of adaptation strategies. So far, climate change adaptation measures have had hardly any effect on this region (Ahmed et al., 2019), in part due to the ongoing Siachen conflict (Baghel and Nüsser, 2015). Detailed information on potentially threatening glacial lakes is key to assess and realize the adaptive capacity of local communities. Therefore, we underline the urgent need for more in-depth regional studies at sites of future lake formation close to populated areas in the central Karakoram.
5.2 Debris Cover Analyses
The decrease in mountain glaciers in the coming decades will be accompanied by a proportional increase in debris-covered glaciers (Herreid and Pellicciotti, 2020). Debris on the surface of glaciers can accelerate or reduce surface ablation, influence the development of glacial lakes and increase the probability of GLOFs (Shugar et al., 2020; Lee et al., 2021; Rounce et al., 2021). Nevertheless, the exact implications of debris cover have not yet been fully explored. A broader understanding of the interplay of debris cover and lake development as well as glacier-related hazards is needed (Harrison et al., 2018; Racoviteanu et al., 2021). Still, with recent information on the distribution of supraglacial debris cover (Rounce et al., 2021), we can provide preliminary indications of a possible interplay between debris cover and future lake development. In Figure 8, the distribution of supraglacial debris cover at locations of potential future glacial lakes is depicted exemplary for the region of Mahalangur (A) as well as in an overview map for entire HMA (B). Following Rounce et al. (2021), we classify supraglacial debris as “thin” (orange areas) and “thick” (red areas). Thin covers of debris (<5 cm) enhance melting processes beneath the layer, while thicker covers can reduce sub-debris melt by up to 90% (Rounce et al., 2021). Figure 8A shows that as expected, most glacier tongues are heavily covered in debris while only small patches further up the glacier are covered with thin layers of debris (Anderson and Anderson, 2018). Besides Mahalangur, this relatively thick cover of debris can also be found in Rolwaling and Kangchenjunga as well as certain parts of the Pamir and Karakoram ranges (Figure 8B). Since glacier melt is likely to be altered accordingly, this will also have an impact on the formation of lakes. On the one hand, the reduced melt under thick debris covers may also mean a delay in lake formation at the glacier terminus. On the other hand, due to the inverted ablation gradient close to the glacier front, this phenomenon may cause melt water from stronger melt further up on the glacier to accumulate behind the developing ice walls near the terminus. In this way, particularly unstable proglacial lakes could form, which would pose a particular flood hazard for settlements and infrastructure downstream.
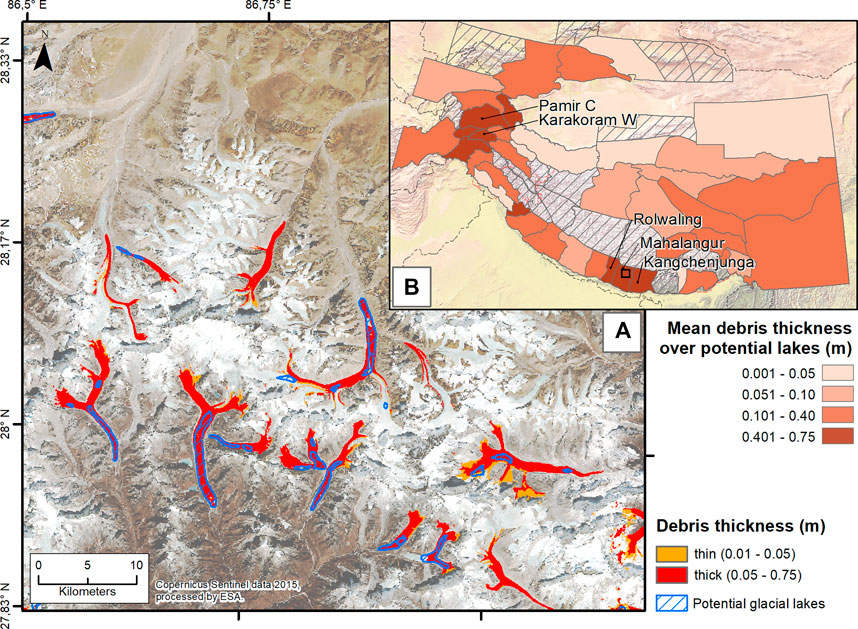
FIGURE 8. Glacier debris cover in Mahalangur at the potential locations of future lakes (A) and the mean debris thickness over lake locations in entire HMA (B). The small black rectangle in B indicates the extent of (A). The debris cover dataset is provided by Rounce et al. (2021), although some regions in (B) are excluded due to insufficient debris cover data (hatched areas).
On a large scale, the results show that especially in the aforementioned regions, a majority of large future lakes have the potential to develop in environments with an abundance of supraglacial debris which could increase the GLOF risk at these locations. This is all the more problematic because a large number of proglacial lakes have already started to form in Mahalangur and adjacent regions in recent years, which were not expected to develop until the near future (see Figure 5). This suggests that reduced sub-debris melt does not necessarily inhibit proglacial lake development, but, on the contrary, increased melt in higher parts of the glacier may facilitate accelerated proglacial lake formation. Thick layers of debris can also be found on many glaciers in other regions of the Himalayan arc as well as in the Central Karakoram and the Tien Shan. Clearly, the uncertainty associated with increased debris cover on glacier tongues complicates the interpretation of the results for local communities. Therefore, we emphasize the need to consider debris cover in upcoming studies on glacial lake development in HMA. Generally, without regionally or even locally focused and contextualized studies in such regions, it will be difficult to identify potentially threatening proglacial lakes and develop potential mitigation measures (Racoviteanu et al., 2021). We provide examples of such an approach in the following section.
5.3 Case Examples
Although the exact current morphological and hydrological situation for most of the glacial lakes in HMA is unknown, a large number of studies exist that focus on the currently most dangerous lakes in this region, for example Merzbacher Lake (Shangguan et al., 2017), Lower Barun Lake (Sattar et al., 2021), Imja Tsho (Lala et al., 2018) as well as Tsho Rolpa or Thulagi (Bajracharya et al., 2020). With our results, it is possible to provide an outlook into a potential future for these dangerous lakes depending on different SSP scenarios. This allows us to demonstrate how different climate trends would differently affect glacial lakes that are already classified as hazardous. Indeed, this may serve as a first indication toward possible adaptation strategies. In all cases, we use lake volume and size as a first-order metric for assessing hazard potential. Larger volumes directly influence the potential magnitude of a GLOF event, while larger areas can indicate a higher mass-movement impact predisposition of the lake. For the visualization of lake evolution over time, we use the results of the OGGM run based on the CESM2-WACCM model since this GCM well reproduces the mean of the model ensemble. However, since the simulated exposed lake area is highly dependent on the chosen GCM, picking a single specific model run implies that the results are initial approximations only.
Figure 9 shows the development of a very large proglacial lake directly behind today’s Lake Merzbacher, a regularly outbursting glacial lake in the central Tien Shan mountains. Under the SSP126 scenario, this lake could slowly develop throughout the entire 21st century. It would form over a length of ∼7 km having a surface area of ∼6 km2 and a volume of about 0.5 km3 in 2100. This could increase the hazard potential of the regularly occurring GLOF events at this site (Wortmann et al., 2014). With higher SSP scenarios, the proglacial lake would start to grow even faster and extend further up the valley. Under scenario SSP585, the lake formation accelerates towards the end of the century. The lake would be fully formed already in 2090, extending over more than 20 km with an area of 16 km2 and a volume of 1.5 km3. According to the results of Furian et al. (2021), rock or ice avalanches from over 50 km2 of surrounding slopes could then hit the lake directly and trigger displacement waves, which could significantly increase both the frequency and magnitude of GLOF events. On the one hand, the mostly thick supraglacial debris cover suggests that the timing of lake formation may tend to be shifted toward the end of the century. On the other hand, numerous supraglacial lakes, especially in the first kilometers of the glacier, already indicate the formation of such lakes (Benn et al., 2012).
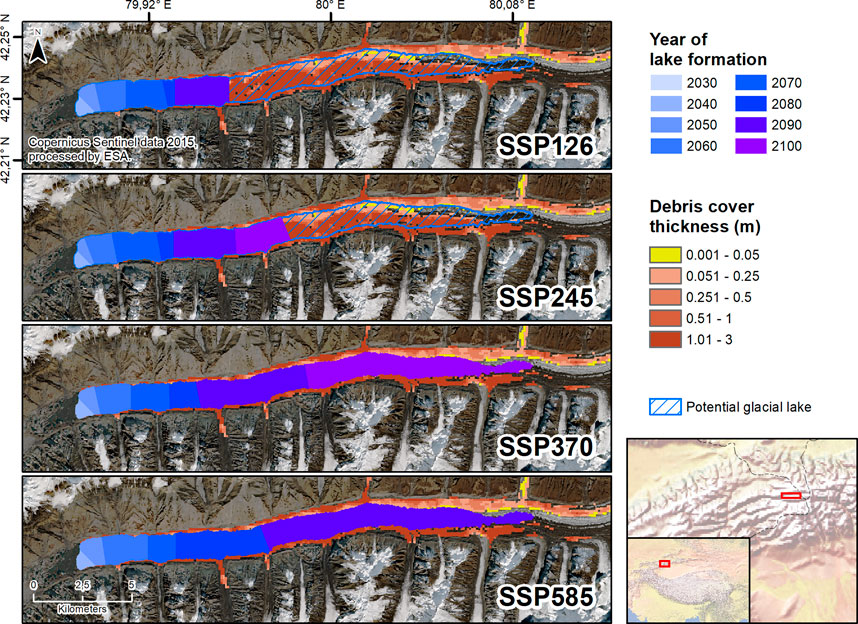
FIGURE 9. Development of a future proglacial extension of Merzbacher Lake under different SSP scenarios until 2100. Lake extent based on CESM2-WACCM modeling, debris cover data by Rounce et al. (2021).
The Tsho Rolpa in the Tama River basin in Nepal is one of the most dangerous glacial lakes in HMA, since, as of 2020, over 140,000 people would be affected in the event of a GLOF (Bajracharya et al., 2020). Although the water level was lowered in 2000, the hazard potential remains high due to a steep and thin moraine and rapid glacier retreat. At this location, the lake expansion has the potential to take place much more rapidly than at Lake Merzbacher (Figure 10). Under SSP126, SSP245 and SSP370, the current lake could be grown about 1 km by 2030, thus enlarging the existing lake by about 50%. Ten years later, in all three scenarios, the lake may be fully formed with a length of about 2 km and an additional volume of 0.01 km3. Under the SSP585, this could already have happened before 2030. It is also worth mentioning a second larger lake further up on the glacier, which may begin to develop in the second half of the century and could grow to about 3 km2, with a volume of 0.08 km3. There, the thin debris (<5 cm) could enhance ablation and lead to an inverted ablation gradient. This would decrease the time needed for the second lake to develop while simultaneously increasing the probability for a GLOF event at the lower lake. These results can serve as a basis for evaluating whether the measures taken to lower the GLOF risk will be sufficient for the future or whether research into the development of further strategies is needed.
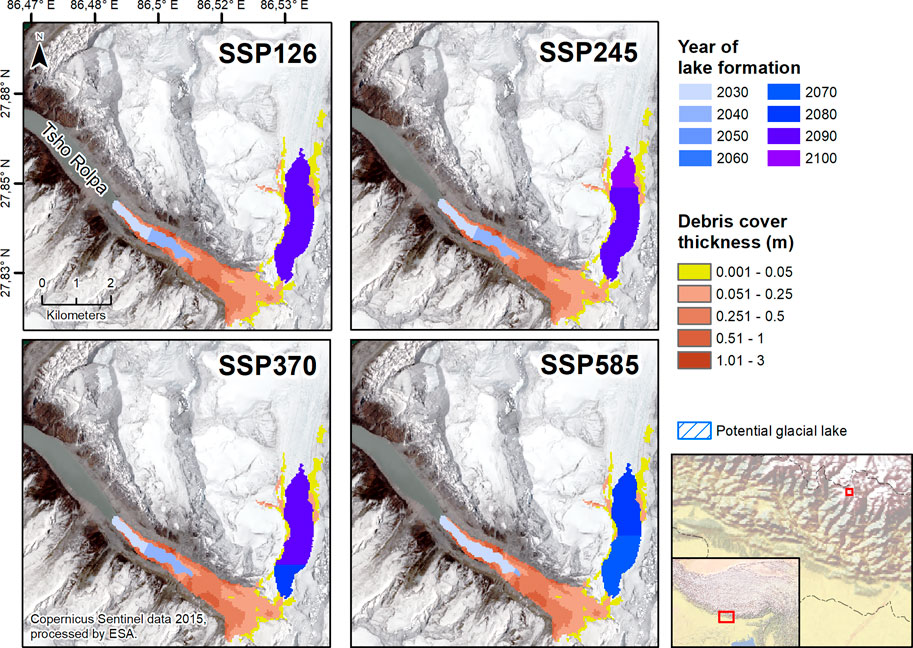
FIGURE 10. Potential extension of Tsho Rolpa and formation of a second lake further up the glacier under different SSP scenarios until 2100. Lake extent based on CESM2-WACCM modeling, debris cover data by Rounce et al. (2021).
At the Lower Barun glacial lake in Nepal, risk mitigation measures are planned following the examples at Tsho Rolpa and Imja Lake (Bajracharya et al., 2020). For this fast-growing and very deep lake, our results show that even under SSP126 the glacial lake could grow by about 50% by mid-century, increasing its area by 1 km2 and its volume by 0.1 km3 (Figure 11). For all other scenarios, the lake could be fully formed (2 km2, 0.15 km3) by 2090 (SSP245), 2070 (SSP370) or even by 2060 (SSP585), respectively. The thin debris cover on the glacier could further accelerate this process. Since several settlements and both existing and planned hydropower plants would be exposed to a GLOF at this location, regular monitoring at Lower Barun Lake is recommended to survey the continuing lake formation. Our results add the necessary temporal component to previous studies assessing the GLOF hazard at Lower Barun Lake (Maskey et al., 2020; Sattar et al., 2021) and can help identify a timeframe for the necessary development and implementation of mitigation measures.
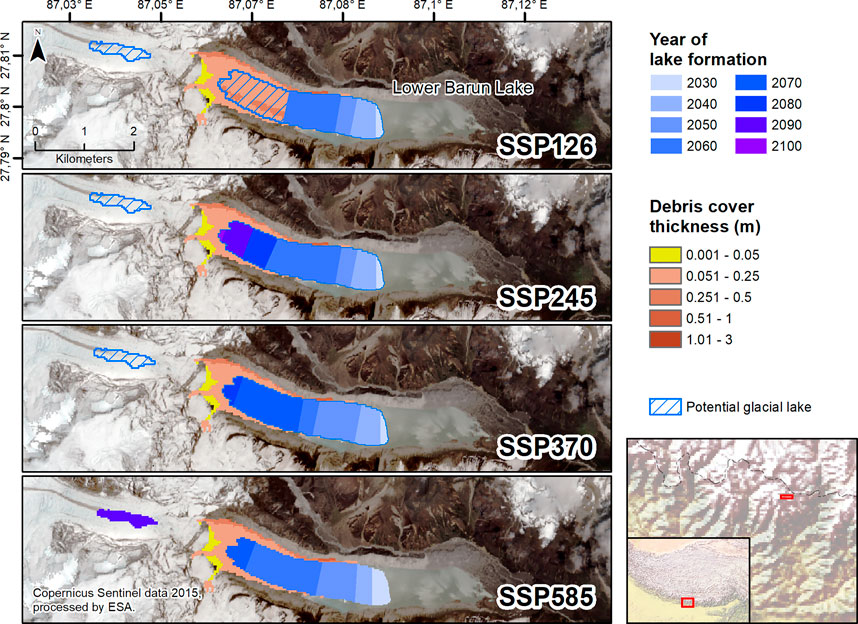
FIGURE 11. Potential extension of Lower Barun glacial lake under different SSP scenarios until 2100. Lake extent based on CESM2-WACCM modeling, debris cover data by Rounce et al. (2021).
6 Conclusion
In this paper, we use the current generation of CMIP6 climate model outputs under the new SSP scenarios to simulate the development of glacial lakes in HMA until 2100 using the glacier evolution model OGGM. We investigate 2,700 of the largest potential future lakes (>0.1 km2) and estimate the increase in glacial lake area in HMA to be 474 ± 121 km2 for SSP126 or 833 ± 148 km2 for SSP585. This would imply an increase in lake area of ∼120–∼210% in 2100 compared to 2018. Glacial lake volume is expected to increase by 22.8 ± 6.1 km3 (SSP126) or 39.7 ± 7.7 km3 (SSP585), which indicates a potential increase between ∼585 and ∼1,000%. For both parameters, there is a considerable spread between total and relative increase among the different regions of HMA. We identify a northwest-southeast gradient of lake development in HMA. In southeastern regions, lake formation or lake expansion is imminent for several dangerous glacial lakes which will pose major challenges for the local communities. Only in Bhutan and the Mahalangur region, different SSP scenarios have a significant influence on future lake development. There, values for lake area and volume nearly double between SSP126 and SSP585. In most other regions in the southern and southeastern HMA, lake development has decoupled from climate, so that the different SSP scenarios have hardly any influence on the rate of future lake development. In northern and northeastern regions (i.e., the Pamir, Karakoram and Tien Shan), lake formation may occur on a much larger scale, albeit later in the 21st century and with significant differences among the individual SSP scenarios.
Using the results of this study, follow-up research could increase the temporal resolution to investigate the detachment of glacial lakes from the terminus. Further, a hindcast simulation (e.g., 1980–2020) could be performed, which would be an interesting method to discuss uncertainties in modeled glacial lake development. However, the increase in the number of glacial lakes alone is not a sufficient predictor for GLOF risk. Therefore, we emphasize the urgent need for more localized approaches that use our results as a starting point for identifying potentially hazardous lakes and that consider the multiple parameters that influence lake formation and GLOF hazard (e.g., debris cover, stability of surrounding slopes). For local communities to develop mitigation strategies, the research focus of those studies needs to shift from the subcontinental scale to a mesoscale or even localized approach to model individual sites in greater detail also considering societal and economical predispositions.
Data Availability Statement
The glacier model can be accessed via the OGGM website [oggm.org] and the permanent DOI at Maussion et al. (2021) [10.5281/zenodo.4546676]. The main analysis code (in Python 2.7) is available on GitHub along with an aggregated version of the lake datasets generated in this study. Both are accessible via the permanent DOI at Furian (2022) [10.5281/zenodo.6024450].
Author Contributions
WF and CS developed the idea for this study. WF created the outline and designed the final structure together with CS and FM. FM and WF determined the model parameters, and FM performed the glacier modeling using OGGM. WF wrote the code for the subsequent analyses, performed them, evaluated and interpreted the results. CS and FM contributed to the geoscientific interpretation of the results. WF wrote the first version of the manuscript. All authors participated in structuring and finalizing the manuscript.
Funding
WF is funded by a personal grant of the Studienstiftung des Deutschen Volkes. We acknowledge support by the German Research Foundation (DFG) and the Open Access Publication Fund of Humboldt-Universität.
Conflict of Interest
The authors declare that the research was conducted in the absence of any commercial or financial relationships that could be construed as a potential conflict of interest.
Publisher’s Note
All claims expressed in this article are solely those of the authors and do not necessarily represent those of their affiliated organizations, or those of the publisher, the editors and the reviewers. Any product that may be evaluated in this article, or claim that may be made by its manufacturer, is not guaranteed or endorsed by the publisher.
Acknowledgments
We thank the OGGM team for the quick and thorough assistance in working with the model. The research conducted in this study was made possible by several open source software tools, such as PyCharm and RStudio, whose development teams we hereby thank. We also thank all researchers and research groups who made data freely available that has been used in this study, especially Shugar et al. for the use of their data on glacial lakes, Rounce et al. for their debris cover dataset as well as the RGI Consortium for their continuous work. Tobias Sauter is thanked for his valuable comments on an earlier version of the manuscript. We also thank our editor Qiao Liu and the three reviewers Jonathan L. Carrivick, Andreas Kääb, and Xin Wang, whose constructive comments and advice helped improve the manuscript.
Supplementary Material
The Supplementary Material for this article can be found online at: https://www.frontiersin.org/articles/10.3389/feart.2022.821798/full#supplementary-material
Supplementary Figure 1 |Individual results of 15 CMIP6 GCMs on the development of glacial lake area in HMA under different SSP scenarios. The dashed lines represent the state of HMA’s glacier lakes (>1 km2) in 2018, following Shugar et al. (2020).
Supplementary Figure 2 |Individual results of 15 CMIP6 GCMs on the development of glacial lake volume in HMA under different SSP scenarios. The dashed lines represent the state of HMA’s glacier lakes (>1 km2) in 2018, following Shugar et al. (2020).
Supplementary Figure 3 |Examples of the coherence between modeled glacial lakes and lakes existing in 2018 according to Shugar et al. (2020). Depicted are (A) a potentially realistic estimate of the future lake development in 2030 and 2050, (B) a potential overestimation of lake area in 2030, as well as a small (C) and a large (D) underestimation of potential lake area.
References
Ahmed, A. U., Appadurai, A. N., and Neelormi, S. (2019). “Status of Climate Change Adaptation in South Asia Region,” in Status of Climate Change Adaptation in Asia and the Pacific. Editors M. Alam, J. Lee, and P. Sawhney (Cham: Springer International Publishing), 125–152. doi:10.1007/978-3-319-99347-8_7
Allen, S. K., Linsbauer, A., Randhawa, S. S., Huggel, C., Rana, P., and Kumari, A. (2016). Glacial lake Outburst Flood Risk in Himachal Pradesh, India: an Integrative and Anticipatory Approach Considering Current and Future Threats. Nat. Hazards 84, 1741–1763. doi:10.1007/s11069-016-2511-x
Anderson, L. S., and Anderson, R. S. (2018). Debris Thickness Patterns on Debris-Covered Glaciers. Geomorphology 311, 1–12. doi:10.1016/j.geomorph.2018.03.014
Ashraf, A., Iqbal, M. B., Mustafa, N., Naz, R., and Ahmad, B. (2021). Prevalent Risk of Glacial lake Outburst Flood hazard in the Hindu Kush-Karakoram-Himalaya Region of Pakistan. Environ. Earth Sci. 80. doi:10.1007/s12665-021-09740-1
Baghel, R., and Nüsser, M. (2015). Securing the Heights: The Vertical Dimension of the Siachen Conflict between India and Pakistan in the Eastern Karakoram. Polit. Geogr. 48, 24–36. doi:10.1016/j.polgeo.2015.05.001
Bajracharya, S., Maharjan, S. B., Shrestha, F., Sherpa, T. C., Wagle, N., and Shrestha, A. B. (2020). Inventory of Glacial Lakes and Identification of Potentially Dangerous Glacial Lakes in the Koshi, Gandaki, and Karnali River Basins of Nepal, the Tibet Autonomous Region of China, and India. Research Report. ICIMOD and UNDP. doi:10.53055/icimod.773
Balaji, V., Taylor, K. E., Juckes, M., Lawrence, B. N., Durack, P. J., Lautenschlager, M., et al. (2018). Requirements for a Global Data Infrastructure in Support of CMIP6. Geosci. Model. Dev. 11, 3659–3680. doi:10.5194/gmd-11-3659-2018
Bazai, N. A., Cui, P., Carling, P. A., Wang, H., Hassan, J., Liu, D., et al. (2021). Increasing Glacial lake Outburst Flood hazard in Response to Surge Glaciers in the Karakoram. Earth-Science Rev. 212, 103432. doi:10.1016/j.earscirev.2020.103432
Bazai, N. A., Cui, P., Liu, D., Carling, P. A., Wang, H., Zhang, G., et al. (2022). Glacier Surging Controls Glacier lake Formation and Outburst Floods: The Example of the Khurdopin Glacier, Karakoram. Glob. Planet. Change 208, 103710. doi:10.1016/j.gloplacha.2021.103710
Benn, D. I., Bolch, T., Hands, K., Gulley, J., Luckman, A., Nicholson, L. I., et al. (2012). Response of Debris-Covered Glaciers in the Mount Everest Region to Recent Warming, and Implications for Outburst Flood Hazards. Earth-Science Rev. 114, 156–174. doi:10.1016/j.earscirev.2012.03.008
Bolch, T., Kulkarni, A., Kääb, A., Huggel, C., Paul, F., Cogley, J. G., et al. (2012). The State and Fate of Himalayan Glaciers. Science 336, 310–314. doi:10.1126/science.1215828
Bothe, O., Fraedrich, K., and Zhu, X. (2012). Precipitation Climate of Central Asia and the Large-Scale Atmospheric Circulation. Theor. Appl. Climatol 108, 345–354. doi:10.1007/s00704-011-0537-2
Brun, F., Berthier, E., Wagnon, P., Kääb, A., and Treichler, D. (2017). A Spatially Resolved Estimate of High Mountain Asia Glacier Mass Balances from 2000 to 2016. Nat. Geosci 10, 668–673. doi:10.1038/ngeo2999
Carrivick, J. L., and Tweed, F. S. (2016). A Global Assessment of the Societal Impacts of Glacier Outburst Floods. Glob. Planet. Change 144, 1–16. doi:10.1016/j.gloplacha.2016.07.001
Carrivick, J. L., and Tweed, F. S. (2013). Proglacial Lakes: Character, Behaviour and Geological Importance. Quat. Sci. Rev. 78, 34–52. doi:10.1016/j.quascirev.2013.07.028
Carrivick, J. L., Tweed, F. S., Sutherland, J. L., and Mallalieu, J. (2020). Toward Numerical Modeling of Interactions between Ice-Marginal Proglacial Lakes and Glaciers. Front. Earth Sci. 8. doi:10.3389/feart.2020.577068
Chen, F., Zhang, M., Guo, H., Allen, S., Kargel, J. S., Haritashya, U. K., et al. (2021). Annual 30 M Dataset for Glacial Lakes in High Mountain Asia from 2008 to 2017. Earth Syst. Sci. Data 13, 741–766. doi:10.5194/essd-13-741-2021
Chhogyel, N., Kumar, L., Bajgai, Y., and Hasan, M. K. (2020). Perception of Farmers on Climate Change and its Impacts on Agriculture across Various Altitudinal Zones of Bhutan Himalayas. Int. J. Environ. Sci. Technol. 17, 3607–3620. doi:10.1007/s13762-020-02662-8
Compagno, L., Huss, M., Miles, E. S., McCarthy, M. J., Zekollari, H., Pellicciotti, F., et al. (2021). Modelling Supraglacial Debris-Cover Evolution from the Single Glacier to the Regional Scale: An Application to High Mountain Asia. The Cryosphere Discuss. [Preprint]. doi:10.5194/tc-2021-233
Cook, S. J., and Quincey, D. J. (2015). Estimating the Volume of Alpine Glacial Lakes. Earth Surf. Dynam. 3, 559–575. doi:10.5194/esurf-3-559-2015
Cuffey, K. M., and Paterson, W. S. B. (2010). The Physics of Glaciers. Amsterdam, Boston, Heidelberg: Butterworth-Heinemann.
Dong, T., and Dong, W. (2021). Evaluation of Extreme Precipitation over Asia in CMIP6 Models. Clim. Dyn. 57, 1751–1769. doi:10.1007/s00382-021-05773-1
Evans, S. (1986). “Landslide Damming in the Cordillera of Western Canada,” in Landslide Dams: Processes, Risk and Mitigation. Geotechnical Special Publication No. 3. Editor R. Schuster (New York: American Society of Civil Engineers), 111–130.
Eyring, V., Bony, S., Meehl, G. A., Senior, C. A., Stevens, B., Stouffer, R. J., et al. (2016). Overview of the Coupled Model Intercomparison Project Phase 6 (CMIP6) Experimental Design and Organization. Geosci. Model. Dev. 9, 1937–1958. doi:10.5194/gmd-9-1937-2016
Farinotti, D., Brinkerhoff, D. J., Clarke, G. K. C., Fürst, J. J., Frey, H., Gantayat, P., et al. (2017). How Accurate Are Estimates of Glacier Ice Thickness? Results from ITMIX, the Ice Thickness Models Intercomparison eXperiment. The Cryosphere 11, 949–970. doi:10.5194/tc-11-949-2017
Farinotti, D., Huss, M., Fürst, J. J., Landmann, J., Machguth, H., Maussion, F., et al. (2019a). A Consensus Estimate for the Ice Thickness Distribution of All Glaciers on Earth. Nat. Geosci. 12, 168–173. doi:10.1038/s41561-019-0300-3
Farinotti, D., Round, V., Huss, M., Compagno, L., and Zekollari, H. (2019b). Large Hydropower and Water-Storage Potential in Future Glacier-free Basins. Nature 575, 341–344. doi:10.1038/s41586-019-1740-z
Furian, W., Loibl, D., and Schneider, C. (2021). Future Glacial Lakes in High Mountain Asia: an Inventory and Assessment of hazard Potential from Surrounding Slopes. J. Glaciol. 67, 1–18. doi:10.1017/jog.2021.18
Furian, W. (2022). Projected 21st Century Glacial Lake Evolution in High Mountain Asia: A Dataset in Shapefile Format. v1.01. Zenodo doi:10.5281/zenodo.6024450
Gaphaz, (2017). Assessment of Glacier and Permafrost Hazards in Mountain Regions: Technical Guidance Document. Zurich, Switzerland/Lima, Peru: Standing Group on Glacier and Permafrost Hazards in Mountains (GAPHAZ) of the International Association of Cryospheric Sciences (IACS) and the International Permafrost Association (IPA).
Gardelle, J., Arnaud, Y., and Berthier, E. (2011). Contrasted Evolution of Glacial Lakes along the Hindu Kush Himalaya Mountain Range between 1990 and 2009. Glob. Planet. Change 75, 47–55. doi:10.1016/j.gloplacha.2010.10.003
GTN-G (2017). Global Terrestrial Network for Glaciers. doi:10.5904/gtng-glacreg-2017-07GTN-G Glacier Regions (GlacReg)
Guo, H., Bao, A., Chen, T., Zheng, G., Wang, Y., Jiang, L., et al. (2021). Assessment of CMIP6 in Simulating Precipitation over Arid Central Asia. Atmos. Res. 252, 105451. doi:10.1016/j.atmosres.2021.105451
Haeberli, W., Bütler, M., Huggel, C., Müller, H., and Schleiss, A. J. (2013). Neue Seen als Folge des Gletscherschwundes im Hochgebirge: Chancen und Risiken. Zürich: vdf Hochschulverlag AG an der ETH Zürich.
Haeberli, W., Buetler, M., Huggel, C., Friedli, T. L., Schaub, Y., and Schleiss, A. J. (2016). New Lakes in Deglaciating High-Mountain Regions - Opportunities and Risks. Climatic Change 139, 201–214. doi:10.1007/s10584-016-1771-5
Haeberli, W., and Weingartner, R. (2020). In Full Transition: Key Impacts of Vanishing Mountain Ice on Water-Security at Local to Global Scales. Water Security 11, 100074. doi:10.1016/j.wasec.2020.100074
Haritashya, U., Kargel, J., Shugar, D., Leonard, G., Strattman, K., Watson, C., et al. (2018). Evolution and Controls of Large Glacial Lakes in the Nepal Himalaya. Remote Sensing 10, 798. doi:10.3390/rs10050798
Harris, I., and Jones, P. (2013). CRU TS3.21: Climatic Research Unit (CRU) Time-Series (TS) Version 3.21 of High Resolution Gridded Data of Month-By-Month Variation in Climate. Leeds, United Kingdom: NCAS British Atmospheric Data Centre. doi:10.5285/D0E1585D-3417-485F-87AE-4FCECF10A992
Harrison, S., Kargel, J. S., Huggel, C., Reynolds, J., Shugar, D. H., Betts, R. A., et al. (2018). Climate Change and the Global Pattern of Moraine-Dammed Glacial lake Outburst Floods. The Cryosphere 12, 1195–1209. doi:10.5194/tc-12-1195-2018
Herreid, S., and Pellicciotti, F. (2020). The State of Rock Debris Covering Earth's Glaciers. Nat. Geosci. 13, 621–627. doi:10.1038/s41561-020-0615-0
Hersbach, H., Bell, B., Berrisford, P., Hirahara, S., Horányi, A., Muñoz‐Sabater, J., et al. (2020). The ERA5 Global Reanalysis. Q.J.R. Meteorol. Soc. 146, 1999–2049. doi:10.1002/qj.3803
Hewitt, K., and Liu, J. (2010). Ice-Dammed Lakes and Outburst Floods, Karakoram Himalaya: Historical Perspectives on Emerging Threats. Phys. Geogr. 31, 528–551. doi:10.2747/0272-3646.31.6.528
Hock, R., Bliss, A., Marzeion, B., Giesen, R. H., Hirabayashi, Y., Huss, M., et al. (2019a). GlacierMIP - A Model Intercomparison of Global-Scale Glacier Mass-Balance Models and Projections. J. Glaciol. 65, 453–467. doi:10.1017/jog.2019.22
Hock, R., Rasul, G., Adler, C., Cáceres, B., Gruber, S., Hirabayashi, Y., et al. (2019b). “High Mountain Areas,” in IPCC Special Report on the Ocean and Cryosphere in a Changing Climate (In Press).
Huang, A., Zhou, Y., Zhang, Y., Huang, D., Zhao, Y., and Wu, H. (2014). Changes of the Annual Precipitation over Central Asia in the Twenty-First Century Projected by Multimodels of CMIP5. J. Clim. 27, 6627–6646. doi:10.1175/JCLI-D-14-00070.1
Huggel, C., Kääb, A., Haeberli, W., Teysseire, P., and Paul, F. (2002). Remote Sensing Based Assessment of Hazards from Glacier lake Outbursts: a Case Study in the Swiss Alps. Can. Geotech. J. 39, 316–330. doi:10.1139/t01-099
Hugonnet, R., McNabb, R., Berthier, E., Menounos, B., Nuth, C., Girod, L., et al. (2021). Accelerated Global Glacier Mass Loss in the Early Twenty-First century. Nature 592, 726–731. doi:10.1038/s41586-021-03436-z
Hutter, K. (1981). The Effect of Longitudinal Strain on the Shear Stress of an Ice Sheet: In Defence of Using Stretched Coordinates. J. Glaciol. 27, 39–56. doi:10.3189/S0022143000011217
Hutter, K. (1983). Theoretical Glaciology: Material Science of Ice and the Mechanics of Glaciers and Ice Sheets. Dordrecht: Springer Netherlands.
Jarosch, A. H., Schoof, C. G., and Anslow, F. S. (2013). Restoring Mass Conservation to Shallow Ice Flow Models over Complex Terrain. The Cryosphere 7, 229–240. doi:10.5194/tc-7-229-2013
Jiang, J., Zhou, T., Chen, X., and Zhang, L. (2020). Future Changes in Precipitation over Central Asia Based on CMIP6 Projections. Environ. Res. Lett. 15, 054009. doi:10.1088/1748-9326/ab7d03
Khan, G., Ali, S., Xiangke, X., Qureshi, J. A., Ali, M., and Karim, I. (2021). Expansion of Shishper Glacier lake and Recent Glacier lake Outburst Flood (GLOF), Gilgit-Baltistan, Pakistan. Environ. Sci. Pollut. Res. 28, 20290–20298. doi:10.1007/s11356-020-11929-z
Kienholz, C., Rich, J. L., Arendt, A. A., and Hock, R. (2014). A New Method for Deriving Glacier Centerlines Applied to Glaciers in Alaska and Northwest Canada. The Cryosphere 8, 503–519. doi:10.5194/tc-8-503-2014
King, O., Bhattacharya, A., Bhambri, R., and Bolch, T. (2019). Glacial Lakes Exacerbate Himalayan Glacier Mass Loss. Sci. Rep. 9, 18145. doi:10.1038/s41598-019-53733-x
King, O., Dehecq, A., Quincey, D., and Carrivick, J. (2018). Contrasting Geometric and Dynamic Evolution of lake and Land-Terminating Glaciers in the central Himalaya. Glob. Planet. Change 167, 46–60. doi:10.1016/J.GLOPLACHA.2018.05.006
Kraaijenbrink, P. D. A., Bierkens, M. F. P., Lutz, A. F., and Immerzeel, W. W. (2017). Impact of a Global Temperature Rise of 1.5 Degrees Celsius on Asia's Glaciers. Nature 549, 257–260. doi:10.1038/nature23878
Kriegler, E., Edmonds, J., Hallegatte, S., Ebi, K. L., Kram, T., Riahi, K., et al. (2014). A New Scenario Framework for Climate Change Research: the Concept of Shared Climate Policy Assumptions. Climatic Change 122, 401–414. doi:10.1007/s10584-013-0971-5
Lala, J. M., Rounce, D. R., and McKinney, D. C. (2018). Modeling the Glacial lake Outburst Flood Process Chain in the Nepal Himalaya: Reassessing Imja Tsho's hazard. Hydrol. Earth Syst. Sci. 22, 3721–3737. doi:10.5194/hess-22-3721-2018
Lee, E., Carrivick, J. L., Quincey, D. J., Cook, S. J., James, W. H. M., and Brown, L. E. (2021). Accelerated Mass Loss of Himalayan Glaciers since the Little Ice Age. Sci. Rep. 11, 24284. doi:10.1038/s41598-021-03805-8
Li, D., Shangguan, D., and Anjum, M. N. (2020). Glacial Lake Inventory Derived from Landsat 8 OLI in 2016-2018 in China-Pakistan Economic Corridor. IJGI 9, 294. doi:10.3390/ijgi9050294
Linsbauer, A., Frey, H., Haeberli, W., Machguth, H., Azam, M. F., and Allen, S. (2016). Modelling Glacier-Bed Overdeepenings and Possible Future Lakes for the Glaciers in the Himalaya-Karakoram Region. Ann. Glaciol. 57, 119–130. doi:10.3189/2016AoG71A627
Linsbauer, A., Paul, F., and Haeberli, W. (2012). Modeling Glacier Thickness Distribution and Bed Topography over Entire Mountain Ranges with GlabTop: Application of a Fast and Robust Approach. J. Geophys. Res. 117, n/a. doi:10.1029/2011jf002313
Marzeion, B., Hock, R., Anderson, B., Bliss, A., Champollion, N., Fujita, K., et al. (2020). Partitioning the Uncertainty of Ensemble Projections of Global Glacier Mass Change. Earth's Future 8. doi:10.1029/2019EF001470
Marzeion, B., Jarosch, A. H., and Hofer, M. (2012). Past and Future Sea-Level Change from the Surface Mass Balance of Glaciers. The Cryosphere 6, 1295–1322. doi:10.5194/tc-6-1295-2012
Maskey, S., Kayastha, R. B., and Kayastha, R. (2020). Glacial Lakes Outburst Floods (GLOFs) Modelling of Thulagi and Lower Barun Glacial Lakes of Nepalese Himalaya. Prog. Disaster Sci. 7, 100106. doi:10.1016/j.pdisas.2020.100106
Maussion, F., Butenko, A., Champollion, N., Dusch, M., Eis, J., Fourteau, K., et al. (2019). The Open Global Glacier Model (OGGM) v1.1. Geosci. Model. Dev. 12, 909–931. doi:10.5194/gmd-12-909-2019
Maussion, F., Roth, T., Dusch, M., Recinos, B., Vlug, A., Marzeion, B., et al. (2021). OGGM/oggm: v1.4.0. doi:10.5281/zenodo.4546676Zenodo
Maussion, F., Scherer, D., Mölg, T., Collier, E., Curio, J., and Finkelnburg, R. (2014). Precipitation Seasonality and Variability over the Tibetan Plateau as Resolved by the High Asia Reanalysis*. J. Clim. 27, 1910–1927. doi:10.1175/JCLI-D-13-00282.1
Meinshausen, M., Nicholls, Z. R. J., Lewis, J., Gidden, M. J., Vogel, E., Freund, M., et al. (2020). The Shared Socio-Economic Pathway (SSP) Greenhouse Gas Concentrations and Their Extensions to 2500. Geosci. Model. Dev. 13, 3571–3605. doi:10.5194/gmd-13-3571-2020
Mohanty, L., and Maiti, S. (2021). Probability of Glacial lake Outburst Flooding in the Himalaya. Resour. Environ. Sustainability 5, 100031. doi:10.1016/j.resenv.2021.100031
O'Neill, B. C., Tebaldi, C., van Vuuren, D. P., Eyring, V., Friedlingstein, P., Hurtt, G., et al. (2016). The Scenario Model Intercomparison Project (ScenarioMIP) for CMIP6. Geosci. Model. Dev. 9, 3461–3482. doi:10.5194/gmd-9-3461-2016
Oerlemans, J. (1997). A Flowline Model for Nigardsbreen, Norway: Projection of Future Glacier Length Based on Dynamic Calibration with the Historic Record. Ann. Glaciol. 24, 382–389. doi:10.3189/S0260305500012489
Otto, J. C., Helfricht, K., Prasicek, G., Binder, D., and Keuschnig, M. (2021). Testing the Performance of Ice Thickness Models to Estimate the Formation of Potential Future Glacial Lakes in Austria. Earth Surf. Process. Landforms. doi:10.1002/esp.5266
Pfeffer, W. T., Arendt, A. A., Bliss, A., Bolch, T., Cogley, J. G., Gardner, A. S., et al. (2014). The Randolph Glacier Inventory: a Globally Complete Inventory of Glaciers. J. Glaciol. 60, 537–552. doi:10.3189/2014JoG13J176
Racoviteanu, A. E., Nicholson, L., Glasser, N. F., Miles, E., Harrison, S., and Reynolds, J. M. (2021). Debris-covered Glacier Systems and Associated Glacial lake Outburst Flood Hazards: Challenges and Prospects. J. Geol. Soc., jgs2021–084. accepted manuscript. doi:10.1144/jgs2021-084
Reyer, C. P. O., Otto, I. M., Adams, S., Albrecht, T., Baarsch, F., Cartsburg, M., et al. (2017). Climate Change Impacts in Central Asia and Their Implications for Development. Reg. Environ. Change 17, 1639–1650. doi:10.1007/s10113-015-0893-z
RGI Consortium (2017). Randolph Glacier Inventory - A Dataset Of Global Glacier Outlines: Version 6.0: Technical Report. Colorado, USA: Global Land Ice Measurements from Space Initiative. Boulder, CO: Digital Media. doi:10.7265/N5-RGI-60
Riahi, K., van Vuuren, D. P., Kriegler, E., Edmonds, J., O’Neill, B. C., Fujimori, S., et al. (2017). The Shared Socioeconomic Pathways and Their Energy, Land Use, and Greenhouse Gas Emissions Implications: An Overview. Glob. Environ. Change 42, 153–168. doi:10.1016/j.gloenvcha.2016.05.009
Rounce, D. R., Hock, R., McNabb, R. W., Millan, R., Sommer, C., Braun, M. H., et al. (2021). Distributed Global Debris Thickness Estimates Reveal Debris Significantly Impacts Glacier Mass Balance. Geophys. Res. Lett. 48. doi:10.1029/2020GL091311
Rounce, D. R., Hock, R., and Shean, D. E. (2020). Glacier Mass Change in High Mountain Asia through 2100 Using the Open-Source Python Glacier Evolution Model (PyGEM). Front. Earth Sci. 7. doi:10.3389/feart.2019.00331
Rounce, D., Watson, C., and McKinney, D. (2017). Identification of Hazard and Risk for Glacial Lakes in the Nepal Himalaya Using Satellite Imagery from 2000-2015. Remote Sensing 9, 654. doi:10.3390/rs9070654
Round, V., Leinss, S., Huss, M., Haemmig, C., and Hajnsek, I. (2017). Surge Dynamics and lake Outbursts of Kyagar Glacier, Karakoram. The Cryosphere 11, 723–739. doi:10.5194/tc-11-723-2017
Rowan, A. V., Egholm, D. L., Quincey, D. J., Hubbard, B., King, O., Miles, E. S., et al. (2021). The Role of Differential Ablation and Dynamic Detachment in Driving Accelerating Mass Loss from a Debris‐Covered Himalayan Glacier. J. Geophys. Res. Earth Surf. 126. doi:10.1029/2020JF005761
Sattar, A., Haritashya, U. K., Kargel, J. S., Leonard, G. J., Shugar, D. H., and Chase, D. V. (2021). Modeling lake Outburst and Downstream hazard Assessment of the Lower Barun Glacial Lake, Nepal Himalaya. J. Hydrol. 598, 126208. doi:10.1016/j.jhydrol.2021.126208
Shangguan, D., Ding, Y., Liu, S., Xie, Z., Pieczonka, T., Xu, J., et al. (2017). Quick Release of Internal Water Storage in a Glacier Leads to Underestimation of the Hazard Potential of Glacial Lake Outburst Floods from Lake Merzbacher in Central Tian Shan Mountains. Geophys. Res. Lett. 44, 9786–9795. doi:10.1002/2017GL074443
Shean, D. E., Bhushan, S., Montesano, P., Rounce, D. R., Arendt, A., and Osmanoglu, B. (2020). A Systematic, Regional Assessment of High Mountain Asia Glacier Mass Balance. Front. Earth Sci. 7. doi:10.3389/feart.2019.00363
Shugar, D. H., Burr, A., Haritashya, U. K., Kargel, J. S., Watson, C. S., Kennedy, M. C., et al. (2020). Rapid Worldwide Growth of Glacial Lakes since 1990. Nat. Clim. Chang. 10, 939–945. doi:10.1038/s41558-020-0855-4
Sutherland, J. L., Carrivick, J. L., Gandy, N., Shulmeister, J., Quincey, D. J., and Cornford, S. L. (2020). Proglacial Lakes Control Glacier Geometry and Behavior during Recession. Geophys. Res. Lett. 47. doi:10.1029/2020GL088865
Trüssel, B. L., Motyka, R. J., Truffer, M., and Larsen, C. F. (2013). Rapid Thinning of lake-calving Yakutat Glacier and the Collapse of the Yakutat Icefield, Southeast Alaska, USA. J. Glaciol. 59, 149–161. doi:10.3189/2013J0G12J081
Tsutaki, S., Fujita, K., Nuimura, T., Sakai, A., Sugiyama, S., Komori, J., et al. (2019). Contrasting Thinning Patterns between lake- and Land-Terminating Glaciers in the Bhutanese Himalaya. The Cryosphere 13, 2733–2750. doi:10.5194/tc-13-2733-2019
Veh, G., Korup, O., von Specht, S., Roessner, S., and Walz, A. (2019). Unchanged Frequency of Moraine-Dammed Glacial lake Outburst Floods in the Himalaya. Nat. Clim. Chang. 9, 379–383. doi:10.1038/s41558-019-0437-5
Veh, G., Korup, O., and Walz, A. (2020). Hazard from Himalayan Glacier lake Outburst Floods. Proc. Natl. Acad. Sci. USA 117, 907–912. doi:10.1073/pnas.1914898117
Wang, X., Guo, X., Yang, C., Liu, Q., Wei, J., Zhang, Y., et al. (2020). Glacial lake Inventory of High-Mountain Asia in 1990 and 2018 Derived from Landsat Images. Earth Syst. Sci. Data 12, 2169–2182. doi:10.5194/essd-12-2169-2020
Watson, C. S., Kargel, J. S., Shugar, D. H., Haritashya, U. K., Schiassi, E., and Furfaro, R. (2020). Mass Loss from Calving in Himalayan Proglacial Lakes. Front. Earth Sci. 7. doi:10.3389/feart.2019.00342
World Bank (2020). World Bank Official Boundaries: International Borders and Disputed Borders. Washington, D.C.: World Bank Basemap Collection.
Wortmann, M., Krysanova, V., Kundzewicz, Z. W., Su, B., and Li, X. (2014). Assessing the Influence of the Merzbacher Lake Outburst Floods on Discharge Using the Hydrological Model SWIM in the Aksu Headwaters, Kyrgyzstan/NW China. Hydrol. Process. 28, 6337–6350. doi:10.1002/hyp.10118
Xenarios, S., Gafurov, A., Schmidt-Vogt, D., Sehring, J., Manandhar, S., Hergarten, C., et al. (2019). Climate Change and Adaptation of Mountain Societies in Central Asia: Uncertainties, Knowledge Gaps, and Data Constraints. Reg. Environ. Change 19, 1339–1352. doi:10.1007/s10113-018-1384-9
Yin, B., Zeng, J., Zhang, Y., Huai, B., and Wang, Y. (2019). Recent Kyagar Glacier lake Outburst Flood Frequency in Chinese Karakoram Unprecedented over the Last Two Centuries. Nat. Hazards 95, 877–881. doi:10.1007/s11069-018-3505-7
You, Q., Cai, Z., Wu, F., Jiang, Z., Pepin, N., and Shen, S. S. P. (2021). Temperature Dataset of CMIP6 Models over China: Evaluation, Trend and Uncertainty. Clim. Dyn. 57, 17–35. doi:10.1007/s00382-021-05691-2
Zekollari, H., Huss, M., and Farinotti, D. (2019). Modelling the Future Evolution of Glaciers in the European Alps under the EURO-CORDEX RCM Ensemble. The Cryosphere 13, 1125–1146. doi:10.5194/tc-13-1125-2019
Zhang, G., Bolch, T., Allen, S., Linsbauer, A., Chen, W., and Wang, W. (2019). Glacial lake Evolution and Glacier-lake Interactions in the Poiqu River basin, central Himalaya, 1964-2017. J. Glaciol. 65, 347–365. doi:10.1017/jog.2019.13
Zhang, G., Yao, T., Xie, H., Wang, W., and Yang, W. (2015). An Inventory of Glacial Lakes in the Third Pole Region and Their Changes in Response to Global Warming. Glob. Planet. Change 131, 148–157. doi:10.1016/j.gloplacha.2015.05.013
Zhang, M., Yu, H., King, A. D., Wei, Y., Huang, J., and Ren, Y. (2020). Greater Probability of Extreme Precipitation under 1.5 °C and 2 °C Warming Limits over East-Central Asia. Climatic Change 162, 603–619. doi:10.1007/s10584-020-02725-2
Zheng, G., Allen, S. K., Bao, A., Ballesteros-Cánovas, J. A., Huss, M., Zhang, G., et al. (2021). Increasing Risk of Glacial lake Outburst Floods from Future Third Pole Deglaciation. Nat. Clim. Chang. 11, 411–417. doi:10.1038/s41558-021-01028-3
Keywords: glacial lake, High Mountain Asia (HMA), glacier modeling, shared social economic pathways (SSPs), GLOF hazard, lake area and water volume
Citation: Furian W, Maussion F and Schneider C (2022) Projected 21st-Century Glacial Lake Evolution in High Mountain Asia. Front. Earth Sci. 10:821798. doi: 10.3389/feart.2022.821798
Received: 24 November 2021; Accepted: 07 February 2022;
Published: 03 March 2022.
Edited by:
Qiao Liu, Institute of Mountain Hazards and Environment (CAS), ChinaReviewed by:
Jonathan Lee Carrivick, University of Leeds, United KingdomAndreas Kääb, University of Oslo, Norway
Xin Wang, Hunan University of Science and Technology, China
Copyright © 2022 Furian, Maussion and Schneider. This is an open-access article distributed under the terms of the Creative Commons Attribution License (CC BY). The use, distribution or reproduction in other forums is permitted, provided the original author(s) and the copyright owner(s) are credited and that the original publication in this journal is cited, in accordance with accepted academic practice. No use, distribution or reproduction is permitted which does not comply with these terms.
*Correspondence: Wilhelm Furian, furiawil@geo.hu-berlin.de