- Key Laboratory of Geographical Processes and Ecological Security in Changbai Mountains, Ministry of Education, School of Geographical Science, Northeast Normal University, Changchun, China
Phenology is an important biological indicator for monitoring terrestrial ecosystems and global change. Solar-induced chlorophyll fluorescence (SIF) emitted by chlorophyll has been proven to characterize vegetation photosynthesis and phenology. In this study, we used monthly normalized difference vegetation index (NDVI), enhanced vegetation index (EVI), and SIF products to qualitatively compare the effectiveness at detecting the phenological characteristics (SOS (start-of-season), EOS (end-of-season), and LOS (length-of-season)) over China during 2007–2013. The phenological characteristics determined by gross primary productivity (GPP) were applied as the reference to validate the phenological characteristics derived from NDVI, EVI, and SIF. The results demonstrated that the phenological characteristics derived from SIF were more consistent with that of GPP than VIs (NDVI and EVI) when considering all latitude grades, different elevation grades, and different land cover types in China. In the middle- and high-latitude regions, SOS derived from the vegetation indices (SOSVIs) did not deviate from those from GPP (SOSGPP) and SIF (SOSSIF), while in low latitudes, SOSVIs were about 20 d later than SOSSIF and SOSGPP. The VIs (EOSVIs) had a severe lag behind those of SIF (EOSSIF) in estimating the EOS at all latitudes. The EOSSIF had a deviation of fewer than 5 d compared with EOS estimated by GPP (EOSGPP), whereas the deviation of EOSVIs from EOSGPP was about 10–31 d across low to high latitude regions. The biases of SIF and VIs were due to the inconsistency between vegetation photosynthesis and leaf greenness. Also, VIs overestimated the LOS at all latitudes, the difference of LOS between estimated by NDVI and estimated by GPP was as long as 39 d in the high-latitude region. Our study suggests that SIF is suitable for estimating the phenological characteristics of vegetation regardless of different latitudes, elevation grades, and land cover types in China, providing a basis for SIF to study the vegetation phenological characteristics in a regional scope.
Introduction
Vegetation phenology is a significant factor in measuring the dynamic change of vegetation (Zhao et al., 2015) and influences such things as the terrestrial energy exchange and the global carbon cycle (Keenan et al., 2014; Tang et al., 2015). Research on vegetation phenology can further our understanding of the change of seasonal phenomena (Vrieling et al., 2013), agricultural production (Tao et al., 2006), and global change (Cleland et al., 2007). Vegetation phenology is especially strongly correlated with climatic fluctuation (Richardson et al., 2013). Global climate change seriously affects the growth of terrestrial vegetation and changes the distributions, growth cycles, and species composition of vegetation (Zhao et al., 2015). Therefore, accurate monitoring of phenology is of great significance for understanding the exchange of material and energy between vegetation and climate as well as for the accurate assessment of vegetation productivity and the global carbon budget (Piao et al., 2006; Yu et al., 2017).
Vegetation gross primary productivity (GPP) is the total photosynthetic CO2 fixation of vegetation at the ecosystem level (Xia et al., 2015). Several studies reported that canopy phenology (using the camera) was consistent with the phenology from GPP observations in the field, such as Bartlett Experimental Forest and Howland Forest in the United States (Richardson et al., 2009), the Lägeren Forest in northern Switzerland, and the Hainich Forest in central Germany (Ahrends et al., 2009). Therefore, GPP has been widely used as a reference for land surface phenology derived from satellite data analysis (Gonsamo et al., 2012; Jeong et al., 2017; Noumonvi et al., 2021).
In the past three decades, phenological vegetation information was monitored through time-series vegetation index data, which were obtained from satellite sensors, because these data have advantages in high temporal resolution and wide spatial coverage (Yu et al., 2017). Examples include the National Oceanic and Atmospheric Administration’s (NOAA’s) Advanced Very High-Resolution Radiometer (AVHRR) (Justice et al., 1985; Reed et al., 1994), Landsat (Melaas et al., 2013), MODerate Resolution Imaging Spectroradiometer (MODIS) (Zhang et al., 2003; Keenan et al., 2014; Walther et al., 2016), and Satellite Pour l’Observation de la Terre (SPOT) VEGETATION (Myneni et al., 1997; Zhou et al., 2001; Zhao et al., 2016). The normalized difference vegetation index (NDVI), which is associated with the greenness, seasonal, and interannual variabilities in most vegetation growth and activity, has been widely applied in the seasonal dynamic characteristics research on vegetation from the regional scale to the global scale (Tucker, 1979; Piao et al., 2006; Melaas et al., 2013). Compared with NDVI, the enhanced vegetation index (EVI) is less prone to saturation, which is caused by the luxuriant vegetation, and thus there are also many studies using EVI to observe vegetation growth (Sims et al., 2006; Testa et al., 2018). Although satellite vegetation indices (VIs)-based phenology is widely used to monitor seasonal growth and productivity of vegetation except for evergreen forests (Keenan et al., 2014; Testa et al., 2018), inconsistencies have been found between reflectance-based phenology and true vegetation photosynthesis based on ground observation (Churkina et al., 2005; Joiner et al., 2014; Cui T. et al., 2017; Jeong et al., 2017; Sun et al., 2018). In addition, NDVI-based phenological characteristics have also led to a longer length-of-season (LOS) than that derived by gross primary productivity due to an earlier estimated start-of-season (SOS) and later estimated end-of-season (EOS) in Harvard Forest (Gonsamo et al., 2012; Jeong et al., 2017; Lu et al., 2018). These discrepancies could be explained by the differences in temporal and spatial scales and dissimilarity between vegetation greenness and functions (Kross et al., 2011; Zhang et al., 2017). It has been suggested that the reflectance-based phenological detection method may not be an accurate proxy for plant photosynthesis (Frankenberg et al., 2011; Yang et al., 2014), and therefore, additional observations to help detect photosynthetic activity are necessary.
More recently, satellite remote sensing of solar-induced chlorophyll fluorescence (SIF) observations provides a new method for monitoring plant photosynthetic activities (Frankenberg et al., 2011; Guanter et al., 2014). SIF is a by-product of the photosynthesis process of a plant, and approximately 1% of absorbed photosynthetically active radiation (APAR) is re-emitted at a longer wavelength (640–850 nm) by chlorophyll, which has two emission peaks: a red spectral region with a peak at approximately 685 nm and a near-infrared region (NIR) with a maximum at approximately 740 nm (Wagle et al., 2016; Lu et al., 2018). Using high spectral resolution spectrometers and the advanced retrieval methodology based on the exploitation of Fraunhofer lines, SIF can be retrieved from platforms such as the Greenhouse gases Observing SATellite (GOSAT) (Frankenberg et al., 2011; Guanter et al., 2012), Global Ozone Monitoring Instrument-2 (GOME-2) (Joiner et al., 2013; Zhang et al., 2016), Orbiting Carbon Observatory (OCO-2) (Frankenberg et al., 2014; Li and Xiao, 2019), and TROPOspheric Monitoring Instrument (TROPOMI) (Guanter et al., 2015). Considering the longer time series of GOME-2 data, a number of studies applied it for long time-series research (Zhang et al., 2014; Cui Y. et al., 2017; Hu and Mo, 2020).
SIF data showed a significant positive linear relationship with GPP across the growing season, which suggests that SIF has good predictability for GPP from the canopy to satellite measurements (Damm et al., 2015; Yang et al., 2017; Li et al., 2018). Furthermore, some studies have shown that SIF could estimate the onset of spring and decline of photosynthesis in autumn more accurately in different biomes and regions, and thus can effectively estimate vegetation phenology (Joiner et al., 2014; Huang et al., 2021). Moreover, SIF had been demonstrated to be more sensitive for tracking short-term changes in GPP than reflectance-based vegetation indices (Damm et al., 2010; Yang et al., 2015; Luus et al., 2017; Sinha et al., 2017). However, most of the previous research using SIF to assess the vegetation phenology focused on the regions in relatively high latitude, in which the phenology is easily detected by SIF, few studies have compared vegetation indices and SIF in estimating phenological characteristics over a lengthy period at various latitudes. Also, few works estimated the phenology in different types of land cover or elevations in China (Jiao et al., 2020; Cheng et al., 2021).
In this study, we looked to quantify the improvement in detecting phenology by using SIF compared to NDVI and EVI products in different land cover types (evergreen forests, deciduous forests, shrublands, grasslands, and croplands), elevation grades (divided into 10 levels, according to the equal number of pixels [Supplementary Table S1]), as well as latitudes (high- (>40°N), middle- (30°N–40°N), and low- (<30°N) latitude regions). The reason for latitude grades division is in Synchronization of seasonal fluctuations between GPP and SIF, as well as VIs in each grid cell over China in China (Figure 1). Specifically, we compared phenological characteristics derived from MODIS NDVI data with those derived from GOME-2 SIF data in deciduous forests, shrublands, grasslands, croplands, and evergreen forests using GPP data obtained from MODIS and the Max Planck Institute (MPI) as references. Considering that SIF data has been available since 2007, and GPP data accessed by MPI is only available until 2013, thus we limit the study year to 2007–2013. The main objectives of this research are twofold. One is to compare the performance of SIF-based and NDVI-based satellite remote sensing data in monitoring the satellite-GPP-based vegetation photosynthesis. The other is to study the phenological characteristics of SIF and NDVI in different regions (different latitudes, elevations, and land cover types), taking GPP as the reference.
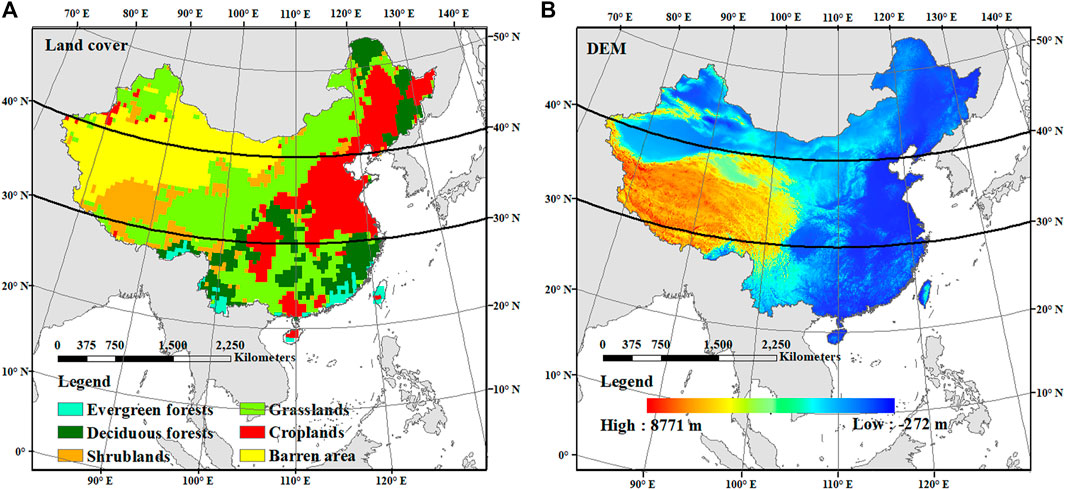
FIGURE 1. Land cover map (A) and DEM (B) of China. The land cover was reclassified into evergreen forests, deciduous forests, shrublands, grasslands, croplands, and barren areas. The spatial resolution of the land cover map is processed to 0.5° × 0.5°. The study area was divided into the high-, middle-, and low-latitude regions by the bold and solid lines in the two panels.
Materials and Methods
Vegetation Indices Data
The monthly NDVI and EVI data from 2007 to 2013 were provided by the MODIS Terra Vegetation Indices dataset (MOD13C2) with a spatial resolution of 0.05°×0.05°. The MODIS NDVI dataset is based on specific spectral bands (red and infrared) designed to offer stable global vegetation leaves greenness monitoring. It has been widely used in land cover classification, carbon exchange modeling, and phenology studies (Shen et al., 2014; Aredehey et al., 2017; Biudes et al., 2021). In addition, EVI is more sensitive to high biomass areas and is minimally impacted by soil and atmosphere due to the increased blue band information (Huete et al., 2002; Jiang et al., 2008). Therefore, both NDVI and EVI were selected for phenological research in this study. In addition, NDVI and EVI were spatially resized to 0.5°×0.5° grid cells using the bilinear resampling method to make it consistent with the spatial resolution of SIF data.
Solar-Induced Chlorophyll Fluorescence Data
The SIF is acquired from the GOME-2 onboard the Eumetsat’s MetOp-A platform, a sun-synchronous orbit satellite whose nominal nadir footprint size is 40 × 80 km and spectral resolution is 0.5 nm with an equator crossing time of 9:30 a.m. (Joiner et al., 2014). The SIF signal is obtained primarily by the filling-in of solar Fraunhofer lines at wavelengths near 740 nm, which is the far-red fluorescence emission peak. A principal component analysis approach with a simplified radiative transfer model was used to separate the spectral signatures of atmospheric absorption and surface reflection from the SIF emission. The monthly SIF product had been cloud filtered and aggregated to 0.5°×0.5° spatial resolution by Joiner et al. (2013) and can be downloaded at http://avdc.gsfc.nasa.gov. In this study, we used version 2.7 Level 3 data from 2007 to 2013.
Gross Primary Productivity Data
The GPP data were used as the phenological reference from two different sources. One source of GPP product was data-driven derived from the MPI (GPPMPI). Specifically, it was estimated by a statistical model to obtain GPP estimates by upscaling global FLUXNET eddy covariance observations with the climate forcing data set CRUNCEPv6 using a machine learning method (Tramontana et al., 2016; Jung et al., 2017). GPPMPI has been widely used for GPP validation in many pieces of research (Frankenberg et al., 2011; Jung et al., 2011; Guanter et al., 2014; Jiang and Ryu, 2016). A more detailed description of GPPMPI is given in Tramontana et al. (2016). The monthly global GPP data set at the 0.5° resolution, covering 2007–2013, was downloaded from www.bgc-jena.mpg.de/geodb/projects/Data.php.
The second source of GPP data was the MODIS GPP product (GPPMOD), which is calculated by using the MOD15A2 fraction of photosynthetically active radiation (fPAR) product and meteorological data, and applying the semi-empirical method (Running et al., 2004). In this study, we downloaded the MOD17A2 monthly product with a spatial resolution of 0.05°, which was accessed from the Numerical Terra dynamic Simulation Group (NTSG), covering the years 2007–2013. The data was downloaded from http://files.ntsg.umt.edu/data/NTSG_Products/MOD17/GeoTIFF. Then, the bilinear resampling method was used to resize the spatial resolution of GPP to 0.5°×0.5° grid cell, which was consistent with the spatial resolution of SIF data.
Ancillary Data
The land cover data set was obtained from the MODIS dataset (MOD12Q1), the University of Maryland (UMD) land cover classification type was chosen and downloaded from http://files.ntsg.umt.edu/data/NTSG_Products/MOD17/GeoTIFF/MOD12Q1/.
Temperature data were from Earth System Research Laboratory (ESRL) Physical Sciences Division (PSD) (www.esrl.noaa.gov/psd/data/gridded/data.UDel_AirT_Precip.html). We chose the global long-term monthly means V4.01 data which covers 2007–2013, with the spatial resolution of 0.5°×0.5°.
Digital elevation data were generated from the CGIAR Consortium for Spatial Information (CGIAR-CSI) Shuttle Radar Topography Mission (SRTM) version 4.1 with a spatial resolution of 250 × 250 m. The elevation files have been mosaiced into a seamless near-global coverage (up to 60° north and south) and downloaded from https://cgiarcsi.community/.
Land cover data were spatially resampled to 0.5°×0.5° grid cells by the majority algorithm to be consistent with the spatial resolution of SIF and GPPMPI data, while elevation data were resampled by the mean resampling method.
Phenological Characteristics Extraction
To estimate vegetation phenological characteristics, the monthly SIF, VIs, GPPMPI, and GPPMOD data are smoothed and interpolated into a daily scale by the spline method (Chen et al., 2004), then normalized the daily scale data. In order to reduce noise and extract vegetation phenological characteristics from time-series data accurately, the Savitzky-Golay smoothing model (Chen et al., 2004; White et al., 2009; Brown et al., 2010; Zhao et al., 2016) was applied to the original SIF, VIs (NDVI and EVI), GPPMPI, and GPPMOD time series data. Smoothed and daily interpolated data have been applied to estimate fluctuation of vegetation growth or vegetation phenological characteristics with high accuracy (Jonsson and Eklundh, 2002; Piao et al., 2006; Zhang et al., 2013; Jeong et al., 2017; Chang et al., 2019; Wang et al., 2019). They have demonstrated that monthly data could provide a reliable result in phenological characterizing. The data used in this study were normalized by the annual maximum and minimum data to fill the magnitude gap among different data.
Then, the SOS, EOS, and LOS were estimated based on the daily SIF, VIs, GPPMPI, and GPPMOD. In this study, we defined SOS or EOS as a rapid continuous increase or decrease in remotely sensed time-series data after the long annual period of photosynthetic senescence (White et al., 2009). Thus, we adopted the threshold determined by White et al. (1997) which is the 50% point of annual normalized time series seasonal curve to calculate the phenology characteristics, because this threshold has been verified as being sensitive to the dynamics of underlying vegetation, canopy cover, and structure (Richardson et al., 2009; de Beurs and Henebry, 2010; Lu et al., 2018). We also calculated the LOS, which is the difference between the SOS and EOS. The schematic diagram of the phenological estimation method is shown in Figure 2, the intersection points of the 50% threshold line (the red horizontal dotted line) and the preprocessing seasonal curve (the black solid line) corresponded to SOS and EOS. Especially, the croplands in the middle-latitude region are planted twice a year, thus we estimate the first and last time to reach the 50% threshold as the SOS and EOS of these regions.
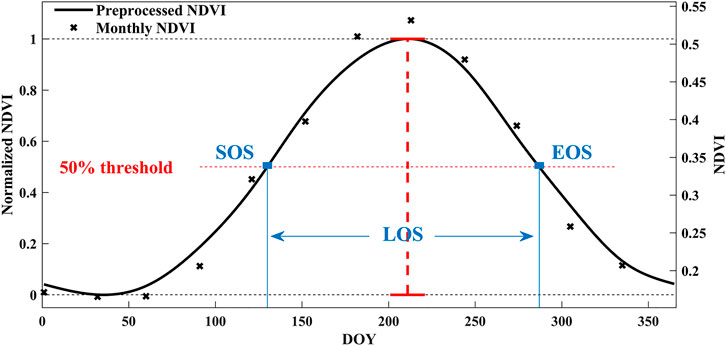
FIGURE 2. The method of estimating phenological characteristics. The “×” points are original data. The black solid line is a preprocessed seasonal curve that has been smoothed, interpolated, and normalized. The red horizontal dotted line is the 50% threshold of maximum and minimum of the preprocessed seasonal curve (the red vertical dotted line). The blue square dots are the SOS and EOS, and LOS is the difference between SOS and EOS.
Due to the data series being at the monthly temporal resolution, it may cause some deviation from the daily data in estimation phenology characteristics in the daily unit. The flux site GPP data, which has both monthly and daily data, were used to estimate the phenology characteristics, using Savitzky-Golay smoothing and spline interpolation, to determine the uncertainty of the data with different temporal resolutions, as Supplementary Figure S1 shows. It is found that after the preprocessing, the SOS and EOS derived from the daily data were 8 d different from the monthly data.
Resampling all the remotely sensed data to 0.5° spatial resolution may lead to some mixed pixels in the images, but the effect of the resampling was limited as Supplementary Figure S2 shows. The EOS from the GPPMOD data was calculated with 0.05° (Supplementary Figure S2A) and 0.5° (Supplementary Figure S2B) spatial resolutions. We can find that the EOS distributions were not changed significantly before and after resampling. Supplementary Figure S2(C) shows the histograms of the calculated EOS. The histograms from different resolutions data were also similar, which indicates that the uncertainty from resampling preprocessing did not affect the phenology characteristics significantly.
Quantifying Synchronicity Methods
To quantify the synchronization of seasonal fluctuations of GPPs (GPPMPI and GPPMOD), SIF, and VIs (NDVI and EVI), the mean absolute error (MAE, as Eq. 1) was conducted. In detail, we calculated MAE on monthly normalized SIF, NDVI, and EVI with GPPMPI and GPPMOD during spring and autumn (March, April, May, September, October, and November) in each grid cell from 2007 to 2013.
where
Results
Synchronization of seasonal fluctuations between GPP and SIF, as well as VIs in each grid cell over China
To explore the synchronization of the seasonal curves of GPPs and SIF, as well as GPPs and VIs in the period of vegetation leaf development and leaf senescence, the spatial distributions of MAE in spring and autumn are shown in Figure 3. The MAE values between GPPs and SIF (Figure 3A for GPPMPI and Figure 3D for GPPMOD), NDVI (Figure 3B for GPPMPI and Figure 3E for GPPMOD), as well as EVI (Figure 3C for GPPMPI and Figure 3F for GPPMOD) are shown. This figure shows lower MAE values obtained from SIF and GPPs than that from VIs and GPPs, which suggests that SIF curves can produce more similar phenological characteristics to GPP. Therefore, SIF could provide a basis for the application of SIF to indicate vegetation phenological characteristics.
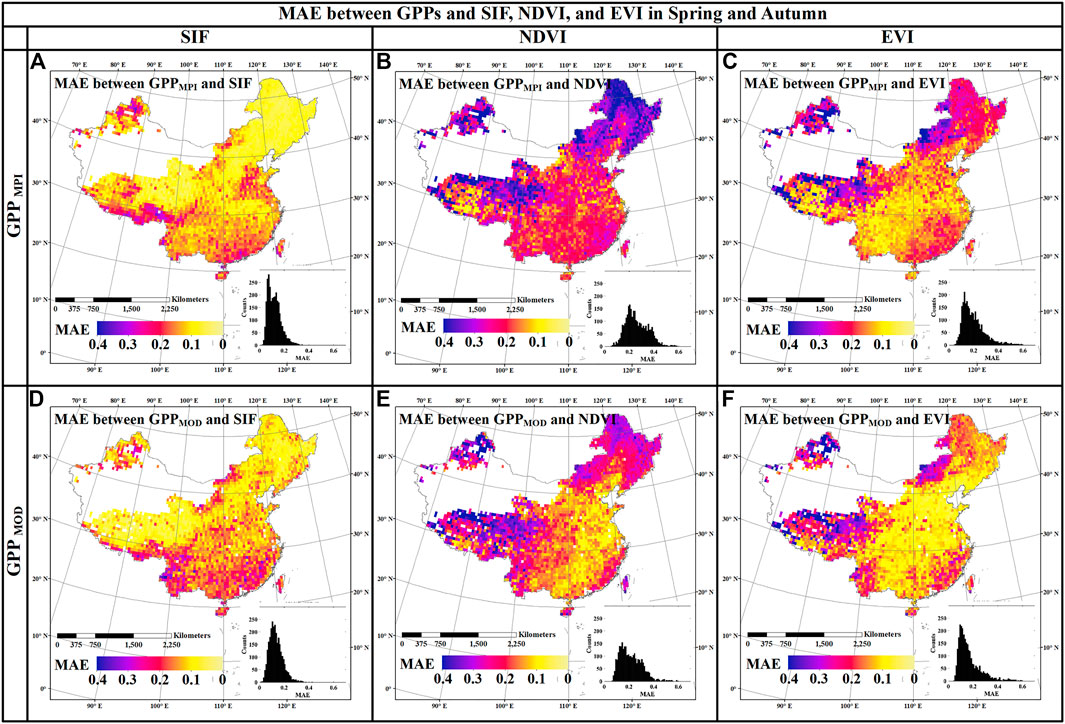
FIGURE 3. Spatial distributions of MAE between GPPs and SIF [(A) for GPPMPI and (D) for GPPMOD], GPPs and NDVI [(B) for GPPMPI and (E) for GPPMOD], as well as GPPs and EVI [(C) for GPPMPI and (F) for GPPMOD] in China during 2007–2013.
In addition, it was found that the synchronization between SIF and GPPs was differentiated in latitudes. SIF and GPPs showed high-level synchronicity in the high-latitude regions (>40°N), medium-level synchronicity in the middle-latitude regions (30°N–40°N), and relatively low-level synchronicity in the low-latitude regions (<30°N). The variation of the synchronizations provides a necessity to analyze the phenological characteristics by SIF at different latitudes. Therefore, the study area was divided into high-, middle-, and low-latitude regions, respectively. Meanwhile, the synchronicity between SIF and GPPs had some minor differences at the same latitude region, which might be caused by the influence of elevation and land cover types.
Seasonal Cycles of SIF and VIs at Different Latitudes
We analyzed the phenological seasonal cycles of different land cover types (deciduous forests, shrublands, grasslands, croplands, and evergreen forests) in different latitude regions (high-, middle-, and low-latitude regions) (Figures 4–6, and 9). The value of each point in these figures was the average monthly value of the corresponding land cover in the latitude region during the 2007–2013 period. We found that the seasonal dynamic curve of SIF was closer to that of GPPs, but the curve of VIs in autumn had a significant mismatch with the seasonal curves of two products of GPP in most cases. Figure 4 shows that the VIs curve ascended earlier than the other curves derived from GPPs in spring, while in autumn, it descended later than GPPs in the high-latitude region. Compared to GPPs, the SIF curve rose a little later than GPPs and fell together with GPPs. Although compared with NDVI and EVI, SIF had little advantage (±10 d) for estimating SOS, for autumn phenology, the difference between EOSSIF (EOS estimated by SIF) and EOSGPPs (EOS estimated by GPPMPI and GPPMOD) (about 3 d) was much smaller than that between EOSVIs (EOS estimated by NDVI and EVI) and EOSGPPs (about 31 d for NDVI and about 19 d for EVI). Almost all the phenological curves except croplands in the middle-latitude region rose together in spring (±10 d), while in autumn, the VIs curves fell behind SIF and GPPs (about 5 d for SIF, about 11 d for EVI, and about 22 d for NDVI), as shown in Figure 5. While in contrast to the SIF, GPPMOD, and GPPMPI, the seasonal cycle of VIs was delayed in both spring and autumn in the low-latitude region (Figure 6) on deciduous forests, shrublands, grasslands, and croplands. The difference between SOSSIF (SOS estimated by SIF) and SOSGPPs (SOS estimated by GPPMPI and GPPMOD) (about 5 d) was smaller than that between SOSVIs (SOS estimated by NDVI and EVI) and SOSGPPs (about 18 d for EVI and about 24 d for NDVI). Additionally, the difference between EOSSIF and EOSGPPs (about 4 d) was also smaller than that between EOSVIs and EOSGPPs (about 10 d for EVI and about 28 d for NDVI). This phenomenon can also be seen in Figure 9. Furthermore, the mismatch between SOS and EOS derived from VIs, GPPs, and SIF, especially the advance of SOSVIs in the high-latitude region, and the serious delay of EOSVIs in all latitudes would lead to an overestimation of LOSVIs (LOS estimated by NDVI and EVI), especially the clear difference between LOSNDVI (LOS estimated by NDVI) and LOSGPPs (LOS estimated by GPPMPI and GPPMOD) was about 39 d in high latitudes. In a word, considering all cases, the bias of SIF estimation of phenological characteristics was minimal.
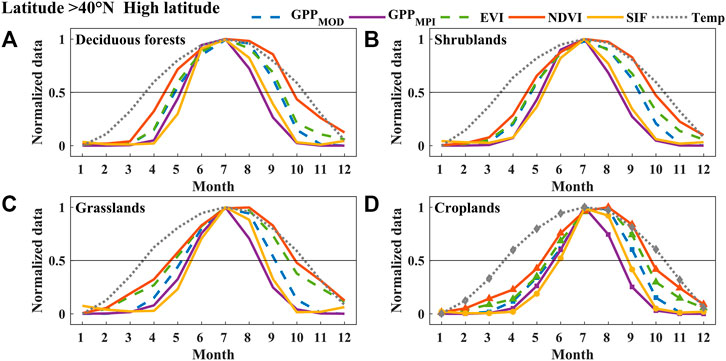
FIGURE 4. The normalized mean seasonal cycle of area-averaged GPPMOD, GPPMPI, NDVI, EVI, SIF, and Temperature (Temp) of deciduous forests (A), shrublands (B), grasslands (C), and croplands (D) in the high-latitude region (over 40°N) of China between 2007 and 2013. Error bars in all subplots represent the monthly standard deviations during 2007–2013.
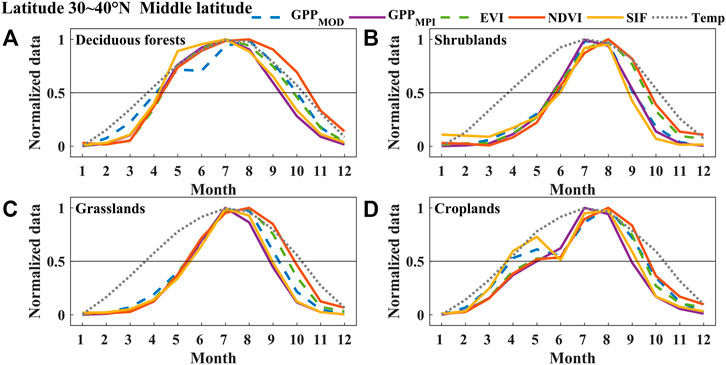
FIGURE 5. The normalized mean seasonal cycle of area-averaged GPPMOD, GPPMPI, NDVI, EVI, SIF, and Temperature (Temp) of deciduous forests (A), shrublands (B), grasslands (C), and croplands (D) in the middle-latitude region (31–40°N) of China between 2007 and 2013. Error bars in all subplots represent the monthly standard deviations during 2007–2013.
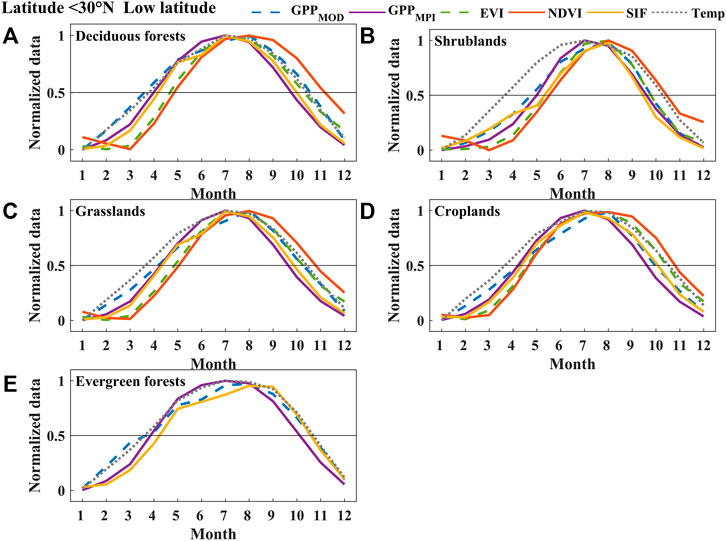
FIGURE 6. The normalized mean seasonal cycle of area-averaged GPPMOD, GPPMPI, NDVI, EVI, SIF, and Temperature (Temp) of deciduous forests (A), shrublands (B), grasslands (C), croplands (D), and evergreen forests (E) in the low-latitude region (below 31°N) of China between 2007 and 2013. Error bars in all subplots represent the monthly standard deviations during 2007–2013.
The annual changes of GPPMPI, GPPMOD, SIF, and NDVI as well as EVI of evergreen forests over 7 years (2007–2013) are shown in Supplementary Figure S3. It was found that the changes in NDVI and EVI throughout the year are very apparent. The difference between the maximum and minimum values of NDVI did not exceed 0.15, as well as that of EVI, was no more than 0.2, and the upward and downward trends were not very distinct. Thus, it is difficult to distinguish SOS and EOS through VIs in the evergreen region. However, SIF showed a clear upward trend from March to May and a significant downward trend from September to November, which was the same as the changing trend of the two sources of GPP, and the phenological change trend could be distinguished. Therefore, the phenological characteristics estimated by SIF (Figure 6E, Figure 7, Figure 8, Figure 9, and Figure 10; Supplementary Figures S4 and S5) of the evergreen forests are displayed, but would not be compared with VIs in the results.
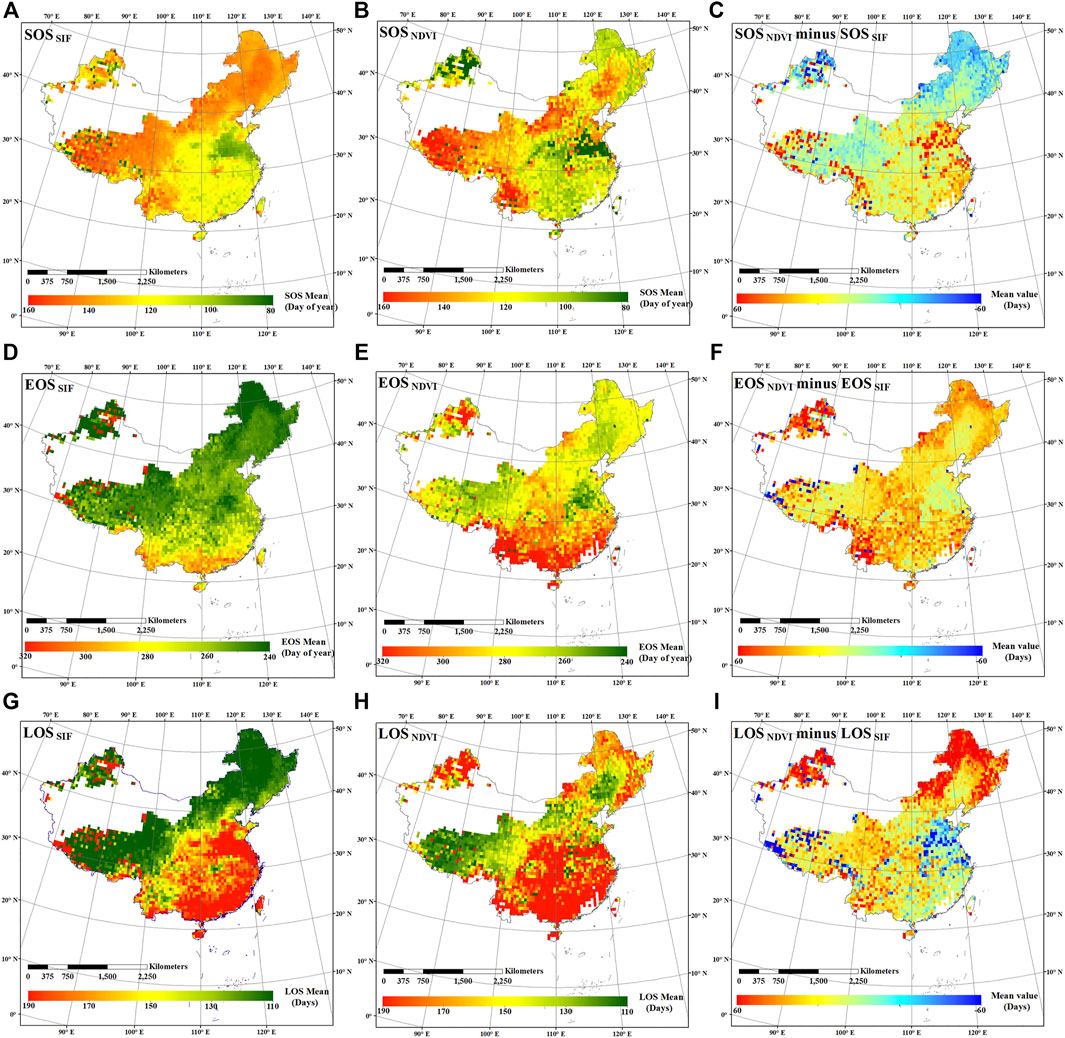
FIGURE 7. Spatial distributions of 7-years averaged SIF and NDVI-based SOS (A, B), EOS (D, E), and LOS (G, H) dates, the difference between NDVI and SIF-based SOS (C), EOS (F), and LOS (I) dates in China during 2007–2013.
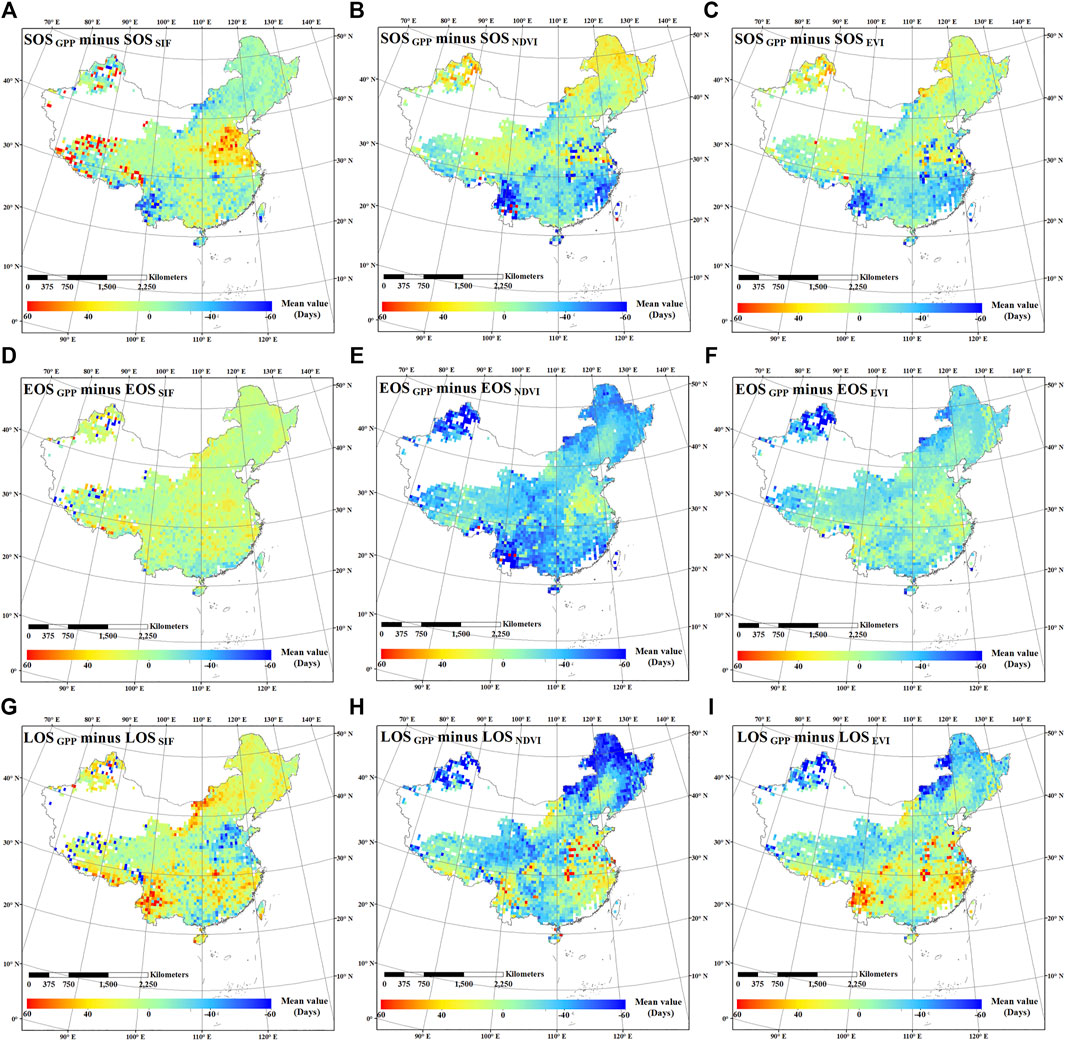
FIGURE 8. Spatial distributions of the difference between SOSGPP and SOSSIF (A), EOSGPP and EOSSIF (D), and LOSGPP and LOSSIF (G), the difference between SOSGPP and SOSNDVI (B), EOSGPP and EOSNDVI (E), and LOSGPP and LOSNDVI (H), and the difference between SOSGPP and SOSEVI (C), EOSGPP and EOSEVI (F), and LOSGPP and LOSEVI (I) in China.
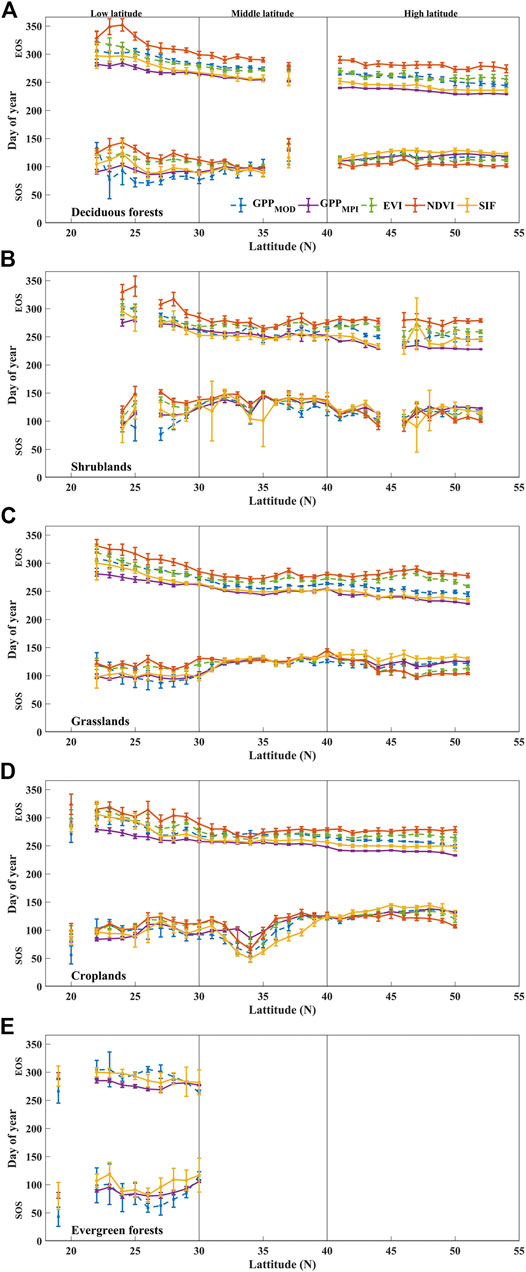
FIGURE 9. The area-averaged SOS (the lower lines) and EOS (the upper lines) of GPPMOD, GPPMPI, NDVI, EVI, and SIF over deciduous forests (A), shrublands (B), grasslands (C), croplands (D), and evergreen forests (E) during 2007–2013 in China. The points and error bars in all subplots represent the mean and standard deviations for 2007–2013, respectively.
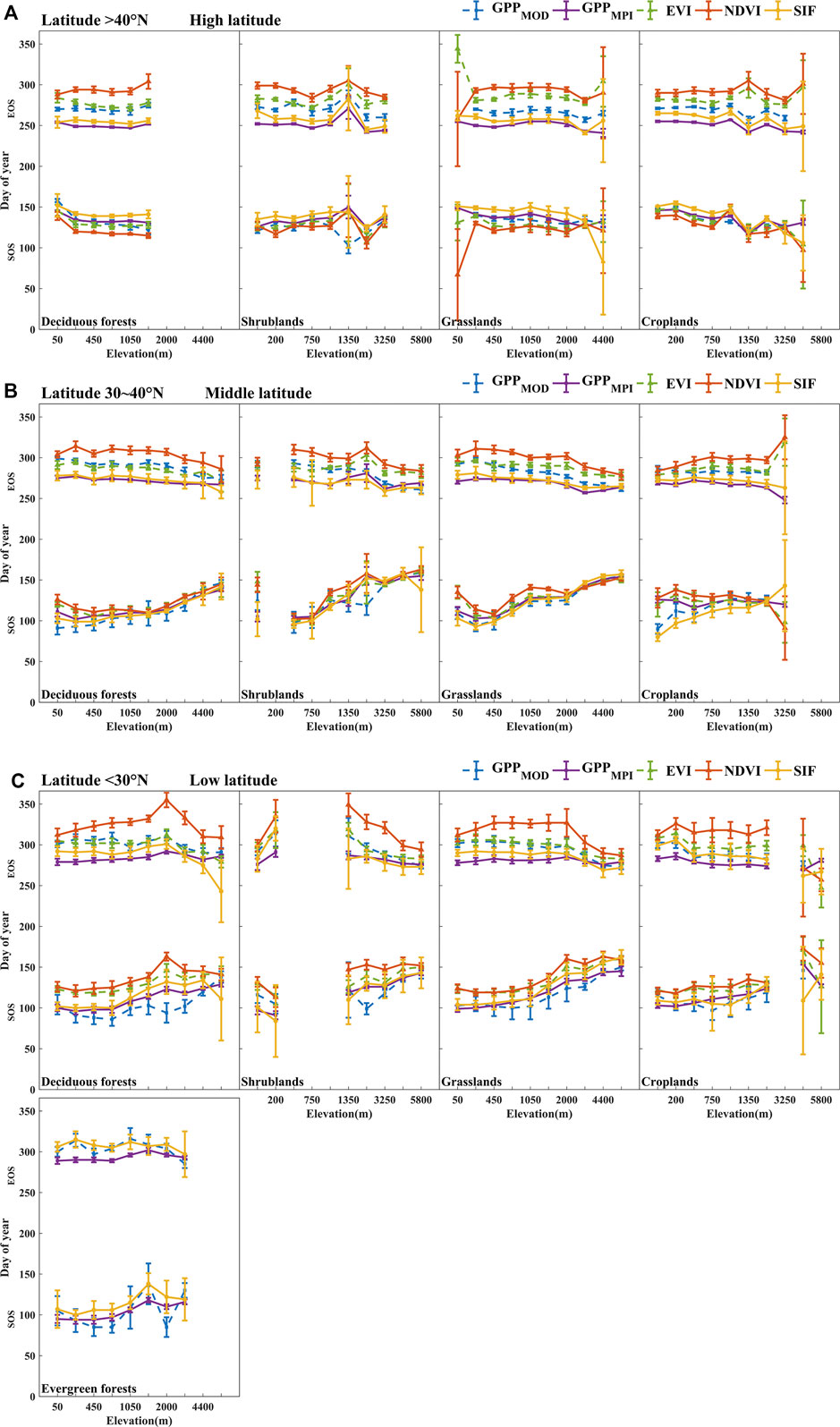
FIGURE 10. The area-averaged SOS (the lower lines) and EOS (the upper lines) of GPPMOD, GPPMPI, NDVI, EVI, and SIF over deciduous forests, shrublands, grasslands, croplands, and evergreen forests during 2007–2013 in high- (A), middle- (B), and low- (C) latitude regions. The points and error bars in all figures represent the mean and standard deviations for 2007–2013, respectively.
Spatial Distribution of Phenological Characteristics Estimated From SIF, VIs, and GPP
To analyze the spatial distribution of the phenological characteristics in China, the average values of SOS, EOS, and LOS based on SIF and NDVI and the difference between their estimations in deciduous forests, shrublands, grasslands, and croplands in China over 7 years (2007–2013) were mapped (Figure 7). In the high latitude areas, SOSSIF was later than SOSNDVI (SOS estimated by NDVI); in the low latitude areas, SOSSIF was earlier than SOSNDVI; while in the middle latitude areas, SOSSIF was later than SOSNDVI in the west and earlier in the east, which may be due to elevation effect. Except for a few anomalies, the EOSSIF data was ahead of that of the EOSNDVI (EOS estimated by NDVI) data for all of China. The advance of SOS and the delay of EOS has led to an overestimation of LOSNDVI in most areas of China, except for croplands in the middle latitudes and a small area of Tibetan shrublands and grasslands. The spatial distribution of phenological characteristics estimated from EVI and the difference between SIF and EVI estimations were shown in Supplementary Figure S4. Compared with NDVI, the phenological characteristics estimated by EVI were closer to the phenological characteristics estimated by SIF. Overall, these results indicated that SIF and VIs are vastly different in estimating phenological characteristics.
The spatial distribution of the difference between the phenological characteristics estimated by GPP and those estimated by SIF, NDVI, and EVI are shown in Figure 8. The phenological characteristics of GPP were the average value of the two sources of GPP (GPPMOD and GPPMPI) estimated phenological characteristics. It was found that the phenological characteristics estimated by SIF were more similar to those estimated by GPP, and the difference was within ±40 d. However, the difference between the phenological characteristics estimated by NDVI and those estimated by GPP was the largest. Most of the differences were delayed (that is, the difference was negative), with a difference of more than 40 d in many regions. The difference of the phenological characteristics estimated by EVI and GPP is a little smaller than that between NDVI and GPP. However, the estimated date was still delayed in many areas. Compared with VIs, SIF had the advantage to estimate EOS in that the difference between EOSSIF and EOSGPP was the smallest. EOSVIs had delayed estimation in almost the entire study area, distinguishing between −40 and −60 d in many areas. This also leads to an overestimation of LOSVIs, especially in high latitudes, and where the difference is around −50 d. Supplementary Figure S5 shows the frequency statistics of Figure 8, and it could also be found that SIF is more suitable than VIs in estimating vegetation phenological characteristics.
Phenological Characteristics Estimated From SIF and VIs With GPP as a Reference in Different Land Covers
Figure 9 represents the phenological characteristics derived from different datasets (SIF, NDVI, EVI, and GPP) in different land covers along latitudes. Different land cover types showed distinct SOS and EOS changes along with latitudes. For example, the SOS shifted to an earlier date and EOS delayed gradually for deciduous forests and grasslands from high to low latitudes and resulted in a longer LOS in lower latitudes. Nevertheless, for shrublands and croplands, the curves undulated intensively with the latitude change. For instance, the estimated SOS for croplands, especially in the middle-latitude region, showed a “concave”. The reason may be intensive planting twice a year in central China, the phenology trend represents a two-peak pattern (Figure 5D). In addition, since evergreen forest only appears in low latitudes, this study did not show the variation in middle and high latitudes.
However, the land cover types did not influence the difference in the estimation of phenological characteristics between SIF and VIs compared with GPP. Specifically, the difference between SIF and GPP is smaller than the difference between VIs and GPP in different land cover types. The differences of 7-years averaged SOS, EOS, and LOS between the NDVI and GPP, EVI, and GPP, as well as SIF and GPP are shown in Supplementary Figure S5. The phenological characteristics of GPP were the average value of the GPPMOD and GPPMPI estimated phenological characteristics. The frequency of difference values above the total and different land cover types by pixel were counted in this figure. The distribution curve conformed to an approximately normal distribution, and frequency distributions of different land cover types were similar to total frequency distribution. The differences between SIF and GPP phenological characteristics were more concentrated and closer to 0, while there was systematic bias between VIs and GPP phenological characteristics, and this caused the differences to be far from 0, especially in EOS. It manifested that SIF-estimated SOS, EOS, and LOS were all consistent with that estimated from GPP regardless of the land cover type. Conversely, the VIs-estimated SOS, EOS, and LOS were further away from those derived from GPP. The above results further proved that SIF is more available for estimating phenological characteristics in most land covers in China.
Phenological Characteristics Estimated From SIF and VIs With GPP as a Reference in Different Elevations
Figure 10 shows the variation of SOS and EOS with elevation in different land cover types and different latitude regions. Overall, the phenological characteristics have the same tendency with the elevation rising, the SOS of the vegetation becomes later, the EOS is earlier, and the vegetation growth period is shortened. This phenomenon is particularly pronounced in the middle- and low-latitude regions, possibly because the temperature at these latitudes is strongly influenced by the elevation. In the middle- and low-latitude regions, the average elevation of the Qinghai-Tibet Plateau in the west of the study area can reach about 6000 m, while the elevation in the eastern coastal area is only about 0 m. The great variation of elevation would result in significant temperature differences, and the vegetation phenology will also change. In the high-latitude region, the variation of elevation is not clear, which may be due to the relatively small difference in elevation, and the lower temperature in high latitudes. However, the differences between SOSVIs and SOSSIF (or SOSGPPs) and between EOSVIs and EOSSIF (or EOSGPPs) have always existed. That is, the SOS and EOS derived from VIs deviated more from SIF and GPPs in most elevations.
Discussion
The phenological changes of GPPs and SIF were consistent among the high-, middle-, and low-latitude regions, while the seasonal fluctuations of VIs were largely different from those of GPPs (Figures 4–6). In the high-latitude region, SOSVIs and SOSSIF were both close to SOSGPPs, but SOSVIs was always earlier than SOSSIF, whereas in the middle-latitude region, the seasonal curves of VIs rose synchronously with SIF and GPPs in spring phenology. However, in the low-latitude region, the VIs-based spring phenology has a lag to the SOS estimated by SIF and GPP. Meanwhile, VIs largely lagged behind SIF or GPPs in the estimation of autumn phenological characteristics at high to low latitudes, especially EOSNDVI. The estimated phenological characteristics by SIF in the high-latitude region were consistent with the results of previous research, in which NDVI showed an advance in spring and a distinct lag to GPP and SIF in autumn were found over northern forests (Jeong et al., 2017) or a specific forest such as Harvard Forest (Lu et al., 2018). Walther et al. (2016) also verified a similar seasonal change over deciduous broadleaf forests in the middle latitude along the east coast of North America, that is, NDVI lagged behind GPP, while SIF was synchronized with GPP. The distinct bias of VIs, compared with SIF, in estimating phenological characteristics might be due to the time inconsistency between photosynthesis and greenness. VIs generally indicated the greenness of vegetation, while SIF is closely related to vegetation photosynthesis. In spring, vegetation in the high- and middle-latitude regions might require time to photosynthesize and increase primary productivity by assimilating carbon after leaf emergence (Kikuzawa, 2003; Jeong et al., 2017). In addition, it caused a rapid increase in VIs values, but a slow increase in SIF and GPP values in the sprouting period. However, the result that SIF had an absolute advantage to estimate the spring phenological characteristics based on GPP in the low-latitude region is different from that in the high- and middle-latitude region and is still to be explained. On the other hand, EOSVIs have a lag at high to low latitudes, which may be due to the fact that VIs estimate vegetation phenology by tracking vegetation characteristics such as leaf area index and chlorophyll content and could not reflect slight phenological changes in time in autumn (Lu et al., 2018). In the case of autumn, carbon assimilation and SIF are mainly limited by sunlight or suitable temperature availability (Suni et al., 2003; Medvigy et al., 2013; Jeong and Medvigy, 2014; Wang et al., 2020), while vegetation indices are different from SIF, which are primarily controlled by the air temperature (Wang et al., 2020; Yang et al., 2020). Thus, the leaves may drop after the photosynthesis has begun to decrease, which results in distinct lags in VIs, but a synchronized change with GPP in SIF (Daumard et al., 2010). Especially, EOSNDVI significantly lags behind other sources of data (GPPs, SIF, and EVI), possibly because NDVI was reported to have saturation effects in high biomass canopies (Walther et al., 2016) and it starts decreasing after a large number of leaves become senescent and drop.
In addition, the phenological characteristics estimation of croplands in middle-latitude regions was only identified on the first and last date with the values of 50% thresholds as SOS and EOS. This may not be appropriate because the crops are always harvested two times per year. To detect the double peaks in mid-latitude croplands is our task in future studies.
SIF has also been proven reliable to track GPP phenological fluctuations in evergreen forests, as shown in Supplementary Figure S3. This finding supports the viewpoint of Magney et al. (2019) that a good correlation between SIF and GPP in Colorado subalpine evergreen coniferous forests enables SIF and GPP to track each other consistently. The large seasonal variations in SIF yield highlight its unique ability to accurately track the seasonality of photosynthesis in different land covers, even in evergreen forests, which was not detected successfully by VIs.
Furthermore, since the temperature is one of the important climatic factors affecting vegetation phenology (Cong et al., 2013; Liang and Zhang, 2016; Lang et al., 2019; Han et al., 2020), the seasonal curve of temperature in the high-, middle-, and low-latitude regions in different land cover types is shown in Figures 4–6. We can find that the fluctuations of temperature were similar in the same latitude region in different land cover types, but the fluctuations of vegetation curves (GPPs, SIF, and VIs) were different, which indicated that the phenological characteristics of different vegetation have different sensitivities to temperature. For example, in the middle-latitude region, the seasonal fluctuations of deciduous forests were similar to the temporal change of temperature, whereas in shrublands and grasslands, the curves of GPPs, SIF, and VIs began to rise after about 2 months of temperature rise in spring. Moreover, by comparing the seasonal fluctuation of temperature and the curves of GPPs and SIF in various latitude regions, we found that the lower the latitude region, the higher the sensitivity of GPPs and SIF to temperature. These results are consistent with some previous studies (Doi and Takahashi, 2008; Gao et al., 2020). Therefore, the phenological characteristics calculated from the remotely sensed temperature have limitations in terms of the different responses of vegetation types to temperature, especially in the middle to high latitudes.
Overall, our research showed the fact that SIF tracked the variation in vegetation phenology more accurately than VIs in different latitudes, elevations, and land cover areas based on monthly data in China.
Conclusion
In this study, we compared and analyzed the discrepancies of phenological characteristics derived from GOME-2 SIF product and MODIS VIs products in China from 2007 to 2013. SIF showed more effectiveness in estimating the phenological characteristics than VIs in different latitudes, elevations, and land cover areas in China. The phenological curves of VIs and SIF in the high- and middle-latitude regions rose together in spring, while the VIs curve lagged far behind SIF in autumn. In the low-latitude region, in contrast to the SIF and GPP, the seasonal cycles of VIs were delayed in both spring and autumn. Thus, SIF appears to be a better option to accurately estimate the characteristics of vegetation phenology and the phenological changes. This provides a basis for future research on the application scenarios of using SIF data to describe vegetation growth conditions and analyze vegetation phenological characteristics. Moreover, the obviously different phenological changes between VIs and SIF in the high-latitude region, middle-latitude region, and low-latitude region provided evidence for future research on the relationship between vegetation photosynthesis and vegetation greenness across latitudes.
Data Availability Statement
The original contributions presented in the study are included in the article/Supplementary Material, further inquiries can be directed to the corresponding authors.
Author Contributions
XW, SL, and ZS designed the study; XW performed the analysis; XW and SL interpreted the data results; XW and ZZ drafted the original manuscript; SL, ZZ, and SZ acquired funding; and all authors contributed to the results and reviewed and edited the manuscript.
Funding
This research was funded by the National Natural Science Foundation of China (41971290, 41977407, 41671347, and 41001258) and the Fundamental Research Funds for the Central Universities (130014925).
Conflict of Interest
The authors declare that the research was conducted in the absence of any commercial or financial relationships that could be construed as a potential conflict of interest.
Publisher’s Note
All claims expressed in this article are solely those of the authors and do not necessarily represent those of their affiliated organizations, or those of the publisher, the editors, and the reviewers. Any product that may be evaluated in this article, or claim that may be made by its manufacturer, is not guaranteed or endorsed by the publisher.
Acknowledgments
The authors would like to thank the editor and all anonymous reviewers for their comments toward improving the technical quality of the manuscript.
Supplementary Material
The Supplementary Material for this article can be found online at: https://www.frontiersin.org/articles/10.3389/feart.2022.802763/full#supplementary-material
References
Ahrends, H., Etzold, S., Kutsch, W., Stoeckli, R., Bruegger, R., Jeanneret, F., et al. (2009). Tree Phenology and Carbon Dioxide Fluxes: Use of Digital Photography for Process-Based Interpretation at the Ecosystem Scale. Clim. Res. 39, 261–274. doi:10.3354/cr00811
Aredehey, G., Mezgebu, A., and Girma, A. (2017). Land-use Land-Cover Classification Analysis of Giba Catchment Using Hyper Temporal MODIS NDVI Satellite Images. Int. J. Remote Sensing. 39 (3), 810–821. doi:10.1080/01431161.2017.1392639
Biudes, M. S., Vourlitis, G. L., Velasque, M. C. S., Machado, N. G., Danelichen, V. H. d. M., Pavão, V. M., et al. (2021). Gross Primary Productivity of Brazilian Savanna (Cerrado) Estimated by Different Remote Sensing-Based Models. Agric. For. Meteorology. 307, 108456. doi:10.1016/j.agrformet.2021.108456
Brown, M. E., de Beurs, K., and Vrieling, A. (2010). The Response of African Land Surface Phenology to Large Scale Climate Oscillations. Remote Sensing Environ. 114 (10), 2286–2296. doi:10.1016/j.rse.2010.05.005
Chang, Q., Xiao, X., Jiao, W., Wu, X., Doughty, R., Wang, J., et al. (2019). Assessing Consistency of spring Phenology of Snow-Covered Forests as Estimated by Vegetation Indices, Gross Primary Production, and Solar-Induced Chlorophyll Fluorescence. Agric. For. Meteorology. 275, 305–316. doi:10.1016/j.agrformet.2019.06.002
Chen, J., Jönsson, P., Tamura, M., Gu, Z., Matsushita, B., and Eklundh, L. (2004). A Simple Method for Reconstructing a High-Quality NDVI Time-Series Data Set Based on the Savitzky-Golay Filter. Remote Sensing Environ. 91 (3-4), 332–344. doi:10.1016/j.rse.2004.03.014
Cheng, W., Li, Z., and Yan, L. (2021). Uniforming spring Phenology under Non-uniform Climate Warming across Latitude in China. Sci. Total Environ. 762, 143177. doi:10.1016/j.scitotenv.2020.143177
Churkina, G., Schimel, D., Braswell, B. H., and Xiao, X. (2005). Spatial Analysis of Growing Season Length Control over Net Ecosystem Exchange. Glob. Change Biol .11, 1777–1787. doi:10.1111/j.1365-2486.2005.01012.x
Cleland, E., Chuine, I., Menzel, A., Mooney, H., and Schwartz, M. (2007). Shifting Plant Phenology in Response to Global Change. Trends Ecol. Evol. 22 (7), 357–365. doi:10.1016/j.tree.2007.04.003
Cong, N., Wang, T., Nan, H., Ma, Y., Wang, X., Myneni, R. B., et al. (2013). Changes in Satellite-Derived spring Vegetation green-up Date and its Linkage to Climate in China from 1982 to 2010: a Multimethod Analysis. Glob. Change Biol. 19 (3), 881–891. doi:10.1111/gcb.12077
Cui, T., Sun, R., Qiao, C., Zhang, Q., Yu, T., Liu, G., et al. (2017). Estimating Diurnal Courses of Gross Primary Production for Maize: A Comparison of Sun-Induced Chlorophyll Fluorescence, Light-Use Efficiency and Process-Based Models. Remote Sensing. 9 (12), 1267. doi:10.3390/rs9121267
Cui, Y., Xiao, X., Zhang, Y., Dong, J., Qin, Y., Doughty, R. B., et al. (2017). Temporal Consistency between Gross Primary Production and Solar-Induced Chlorophyll Fluorescence in the Ten Most Populous Megacity Areas over Years. Sci. Rep. 7 (1), 14963. doi:10.1038/s41598-017-13783-5
Damm, A., Elbers, J. A. N., Erler, A., Gioli, B., Hamdi, K., Hutjes, R., et al. (2010). Remote Sensing of Sun-Induced Fluorescence to Improve Modeling of Diurnal Courses of Gross Primary Production (GPP). Global Change Biology 16 (1), 171–186. doi:10.1111/j.1365-2486.2009.01908.x
Damm, A., Guanter, L., Paul-Limoges, E., van der Tol, C., Hueni, A., Buchmann, N., et al. (2015). Far-red Sun-Induced Chlorophyll Fluorescence Shows Ecosystem-Specific Relationships to Gross Primary Production: An Assessment Based on Observational and Modeling Approaches. Remote Sensing Environ. 166, 91–105. doi:10.1016/j.rse.2015.06.004
Daumard, F., Champagne, S., Fournier, A., Goulas, Y., Ounis, A., Hanocq, J.-F., et al. (2010). A Field Platform for Continuous Measurement of Canopy Fluorescence. IEEE Trans. Geosci. Remote Sensing. 48 (9), 3358–3368. doi:10.1109/tgrs.2010.2046420
de Beurs, K. M., and Henebry, G. M. (2010). Spatio-Temporal Statistical Methods for Modelling Land Surface Phenology. Dordrecht: Springer.
Doi, H., and Takahashi, M. (2008). Latitudinal Patterns in the Phenological Responses of Leaf Colouring and Leaf Fall to Climate Change in Japan. Glob. Ecol Biogeogr. 17 (4), 556–561. doi:10.1111/j.1466-8238.2008.00398.x
Frankenberg, C., Fisher, J. B., Worden, J., Badgley, G., Saatchi, S. S., Lee, J.-E., et al. (2011). New Global Observations of the Terrestrial Carbon Cycle from GOSAT: Patterns of Plant Fluorescence with Gross Primary Productivity. Geophys. Res. Lett. 38 (17), n. doi:10.1029/2011gl048738
Frankenberg, C., O'Dell, C., Berry, J., Guanter, L., Joiner, J., Köhler, P., et al. (2014). Prospects for Chlorophyll Fluorescence Remote Sensing from the Orbiting Carbon Observatory-2. Remote Sensing Environ. 147, 1–12. doi:10.1016/j.rse.2014.02.007
Gao, M., Wang, X., Meng, F., Liu, Q., Li, X., Zhang, Y., et al. (2020). Three‐Dimensional Change in Temperature Sensitivity of Northern Vegetation Phenology. Glob. Change Biol. 26 (9), 5189–5201. doi:10.1111/gcb.15200
Gonsamo, A., Chen, J. M., Price, D. T., Kurz, W. A., and Wu, C. (2012). Land Surface Phenology From Optical Satellite Measurement and CO2 eddy Covariance Technique. J. Geophys. Res. Biogeosciences. 117, G03032. doi:10.1029/2012JG002070
Guanter, L., Aben, I., Tol, P., Krijger, J. M., Hollstein, A., Köhler, P., et al. (2015). Potential of the TROPOspheric Monitoring Instrument (TROPOMI) Onboard the Sentinel-5 Precursor for the Monitoring of Terrestrial Chlorophyll Fluorescence. Atmos. Meas. Tech. 8 (3), 1337–1352. doi:10.5194/amt-8-1337-2015
Guanter, L., Frankenberg, C., Dudhia, A., Lewis, P. E., Gómez-Dans, J., Kuze, A., et al. (2012). Retrieval and Global Assessment of Terrestrial Chlorophyll Fluorescence from GOSAT Space Measurements. Remote Sensing Environ. 121, 236–251. doi:10.1016/j.rse.2012.02.006
Guanter, L., Zhang, Y., Jung, M., Joiner, J., Voigt, M., Berry, J. A., et al. (2014). Global and Time-Resolved Monitoring of Crop Photosynthesis with Chlorophyll Fluorescence. Proc. Natl. Acad. Sci. 111 (14), E1327–E1333. doi:10.1073/pnas.1320008111
Han, H., Bai, J., Ma, G., and Yan, J. (2020). Vegetation Phenological Changes in Multiple Landforms and Responses to Climate Change. ISPRS Int. J. Geo Inf. 9 (2), 111. doi:10.3390/ijgi9020111
Hu, S., and Mo, X. (2020). Detecting Regional GPP Variations with Statistically Downscaled Solar-Induced Chlorophyll Fluorescence (SIF) Based on GOME-2 and MODIS Data. Int. J. Remote Sensing. 41 (23), 9206–9228. doi:10.1080/01431161.2020.1798549
Huang, K., Zhang, Y., Tagesson, T., Brandt, M., Wang, L., Chen, N., et al. (2021). The Confounding Effect of Snow Cover on Assessing spring Phenology from Space: A New Look at Trends on the Tibetan Plateau. Sci. Total Environ. 756, 144011. doi:10.1016/j.scitotenv.2020.144011
Huete, A., Didan, K., Miura, T., Rodriguez, E. P., Gao, X., and Ferreira, L. G. (2002). Overview of the Radiometric and Biophysical Performance of the MODIS Vegetation Indices. Remote Sensing Environ. 83 (1-2), 195–213. doi:10.1016/S0034-4257(02)00096-2
Jeong, S.-J., and Medvigy, D. (2014). Macroscale Prediction of Autumn Leaf Coloration Throughout the continental United States. Glob. Ecol. Biogeogr. 23 (11), 1245–1254. doi:10.1111/geb.12206
Jeong, S.-J., Schimel, D., Frankenberg, C., Drewry, D. T., Fisher, J. B., Verma, M., et al. (2017). Application of Satellite Solar-Induced Chlorophyll Fluorescence to Understanding Large-Scale Variations in Vegetation Phenology and Function over Northern High Latitude Forests. Remote Sensing Environ. 190, 178–187. doi:10.1016/j.rse.2016.11.021
Jiang, C., and Ryu, Y. (2016). Multi-scale Evaluation of Global Gross Primary Productivity and Evapotranspiration Products Derived from Breathing Earth System Simulator (BESS). Remote Sensing Environ. 186, 528–547. doi:10.1016/j.rse.2016.08.030
Jiang, Z., Huete, A., Didan, K., and Miura, T. (2008). Development of a Two-Band Enhanced Vegetation index without a Blue Band. Remote Sensing Environ. 112 (10), 3833–3845. doi:10.1016/j.rse.2008.06.006
Jiao, F., Liu, H., Xu, X., Gong, H., and Lin, Z. (2020). Trend Evolution of Vegetation Phenology in China during the Period of 1981-2016. Remote Sensing. 12 (3), 572. doi:10.3390/rs12030572
Joiner, J., Guanter, L., Lindstrot, R., Voigt, M., Vasilkov, A. P., Middleton, E. M., et al. (2013). Global Monitoring of Terrestrial Chlorophyll Fluorescence from Moderate Spectral Resolution Near-Infrared Satellite Measurements: Methodology, Simulations, and Application to GOME-2. Atmos. Meas. Tech. 6 (2), 3883–3930. doi:10.5194/amtd-6-3883-2013
Joiner, J., Yoshida, Y., Vasilkov, A. P., Schaefer, K., Jung, M., Guanter, L., et al. (2014). The Seasonal Cycle of Satellite Chlorophyll Fluorescence Observations and its Relationship to Vegetation Phenology and Ecosystem Atmosphere Carbon Exchange. Remote Sensing Environ. 152, 375–391. doi:10.1016/j.rse.2014.06.022
Jonsson, P., and Eklundh, L. (2002). Seasonality Extraction by Function Fitting to Time-Series of Satellite Sensor Data. IEEE Trans. Geosci. Remote Sensing. 40 (8), 1824–1832. doi:10.1109/Tgrs.2002.802519
Jung, M., Reichstein, M., Margolis, H. A., Cescatti, A., Richardson, A. D., Arain, M. A., et al. (2011). Global Patterns of Land-Atmosphere Fluxes of Carbon Dioxide, Latent Heat, and Sensible Heat Derived from Eddy Covariance, Satellite, and Meteorological Observations. J. Geophys. Res. 116. doi:10.1029/2010jg001566
Jung, M., Reichstein, M., Schwalm, C. R., Huntingford, C., Sitch, S., Ahlström, A., et al. (2017). Compensatory Water Effects Link Yearly Global Land CO2 Sink Changes to Temperature. Nature. 541 (7638), 516–520. doi:10.1038/nature20780
Justice, C. O., Townshend, J. R. G., Holben, B. N., and Tucker, C. J. (1985). Analysis of the Phenology of Global Vegetation Using Meteorological Satellite Data. Int. J. Remote Sensing. 6, 1271–1318. doi:10.1080/01431168508948281
Keenan, T. F., Gray, J., Friedl, M. A., Toomey, M., Bohrer, G., Hollinger, D. Y., et al. (2014). Net Carbon Uptake Has Increased through Warming-Induced Changes in Temperate forest Phenology. Nat. Clim Change. 4 (7), 598–604. doi:10.1038/nclimate2253
Kikuzawa, K. (2003). Phenological and Morphological Adaptations to the Light Environment in Two Woody and Two Herbaceous Plant Species. Funct. Ecol. 17, 29–38. doi:10.1046/j.1365-2435.2003.00707.x
Kross, A., Fernandes, R., Seaquist, J., and Beaubien, E. (2011). The Effect of the Temporal Resolution of NDVI Data on Season Onset Dates and Trends across Canadian Broadleaf Forests. Remote Sensing Environ. 115 (6), 1564–1575. doi:10.1016/j.rse.2011.02.015
Lang, C., Chen, R., and Qian, R. (2019). Geographic and Climatic Attributions of Autumn Land Surface Phenology Spatial Patterns in the Temperate Deciduous Broadleaf Forest of China. Remote Sensing. 11 (13), 1546. doi:10.3390/rs11131546
Li, X., and Xiao, J. (2019). A Global, 0.05-Degree Product of Solar-Induced Chlorophyll Fluorescence Derived from OCO-2, MODIS, and Reanalysis Data. Remote Sensing. 11 (5), 517. doi:10.3390/rs11050517
Li, X., Xiao, J., He, B., Altaf Arain, M., Beringer, J., Desai, A. R., et al. (2018). Solar‐induced Chlorophyll Fluorescence Is Strongly Correlated with Terrestrial Photosynthesis for a Wide Variety of Biomes: First Global Analysis Based on OCO‐2 and Flux tower Observations. Glob. Change Biol. 24 (9), 3990–4008. doi:10.1111/gcb.14297
Liang, L., and Zhang, X. (2016). Coupled Spatiotemporal Variability of Temperature and spring Phenology in the Eastern United States. Int. J. Climatol. 36 (4), 1744–1754. doi:10.1002/joc.4456
Luus, K. A., Commane, R., Parazoo, N. C., Benmergui, J., Euskirchen, E. S., Frankenberg, C., et al. (2017). Tundra Photosynthesis Captured by Satellite‐Observed Solar‐Induced Chlorophyll Fluorescence. Geophysical Research Letters 44 (3), 1564–1573. doi:10.1002/2016gl070842
Lu, X., Liu, Z., Zhou, Y., Liu, Y., An, S., and Tang, J. (2018). Comparison of Phenology Estimated from Reflectance-Based Indices and Solar-Induced Chlorophyll Fluorescence (SIF) Observations in a Temperate Forest Using GPP-Based Phenology as the Standard. Remote Sensing. 10 (6), 932. doi:10.3390/rs10060932
Magney, T. S., Bowling, D. R., Logan, B. A., Grossmann, K., Stutz, J., Blanken, P. D., et al. (2019). Mechanistic Evidence for Tracking the Seasonality of Photosynthesis with Solar-Induced Fluorescence. Proc. Natl. Acad. Sci. USA. 116 (24), 201900278–201911645. doi:10.1073/pnas.1900278116
Medvigy, D., Jeong, S.-J., Clark, K. L., Skowronski, N. S., and Schäfer, K. V. R. (2013). Effects of Seasonal Variation of Photosynthetic Capacity on the Carbon Fluxes of a Temperate Deciduous forest. J. Geophys. Res. Biogeosci. 118 (4), 1703–1714. doi:10.1002/2013jg002421
Melaas, E. K., Friedl, M. A., and Zhu, Z. (2013). Detecting Interannual Variation in Deciduous Broadleaf forest Phenology Using Landsat TM/ETM+ Data. Remote Sensing Environ. 132, 176–185. doi:10.1016/j.rse.2013.01.011
Myneni, R. B., Keeling, C. D., Tucker, C. J., Asrar, G., and Nemani, R. R. (1997). Increased Plant Growth in the Northern High Latitudes from 1981 to 1991. Nature. 386 (6626), 698–702. doi:10.1038/386698a0
Noumonvi, K. D., Oblišar, G., Žust, A., and Vilhar, U. (2021). Empirical Approach for Modelling Tree Phenology in Mixed Forests Using Remote Sensing. Remote Sensing. 13 (15), 3015. doi:10.3390/rs13153015
Piao, S., Fang, J., Zhou, L., Ciais, P., and Zhu, B. (2006). Variations in Satellite-Derived Phenology in China's Temperate Vegetation. Glob. Change Biol. 12 (4), 672–685. doi:10.1111/j.1365-2486.2006.01123.x
Reed, B. C., Brown, J. F., Vanderzee, D., Loveland, T. R., Merchant, J. W., and Ohlen, D. O. (1994). Measuring Phenological Variability from Satellite Imagery. J. Vegetation Sci. 5(5), 703–714. doi:10.2307/3235884
Richardson, A. D., Braswell, B. H., Hollinger, D. Y., Jenkins, J. P., and Ollinger, S. V. (2009). Near-Surface Remote Sensing of Spatial and Temporal Variation in Canopy Phenology. Ecol. Appl. 19 (6), 1417–1428. doi:10.1890/08-2022.1
Richardson, A. D., Keenan, T. F., Migliavacca, M., Ryu, Y., Sonnentag, O., and Toomey, M. (2013). Climate Change, Phenology, and Phenological Control of Vegetation Feedbacks to the Climate System. Agric. For. Meteorology. 169, 156–173. doi:10.1016/j.agrformet.2012.09.012
Running, S. W., Nemani, R. R., Heinsch, F. A., Zhao, M., Reeves, M., and Hashimoto, H. (2004). Measure of Global Terrestrial Primary Production. BioScience. 54–6. doi:10.1641/0006-3568(2004)054[0547:acsmog]2.0.co;2
Shen, M., Zhang, G., Cong, N., Wang, S., Kong, W., and Piao, S. (2014). Increasing Altitudinal Gradient of spring Vegetation Phenology during the Last Decade on the Qinghai-Tibetan Plateau. Agric. For. Meteorology. 189-190, 71–80. doi:10.1016/j.agrformet.2014.01.003
Sinha, S.K., Padalia, H., and Senthil Kumar, A. (2017). Space-Borne Sun-Induced Fluorescence: An Advanced Probe to Monitor Seasonality of Dry and Moist Tropical Forest Sites. Current Science 113(11), 2180. doi:10.18520/cs/v113/i11/2180-2183
Sims, D. A., Rahman, A. F., Cordova, V. D., El-Masri, B. Z., Baldocchi, D. D., Flanagan, L. B., et al. (2006). On the Use of MODIS EVI to Assess Gross Primary Productivity of North American Ecosystems. J. Geophys. Res. 111 (G4), G04015. doi:10.1029/2006jg000162
Sun, Y., Frankenberg, C., Jung, M., Joiner, J., Guanter, L., Köhler, P., et al. (2018). Overview of Solar-Induced Chlorophyll Fluorescence (SIF) from the Orbiting Carbon Observatory-2: Retrieval, Cross-mission Comparison, and Global Monitoring for GPP. Remote Sensing Environ. 209, 808–823. doi:10.1016/j.rse.2018.02.016
Suni, T., Berninger, F., Markkanen, T., Keronen, P., Rannik, Ü., and Vesala, T. (2003). Interannual Variability and Timing of Growing-Season CO2exchange in a Boreal forest. J. Geophys. Res. Atmospheres. 108 (D9), n. doi:10.1029/2002jd002381
Tang, H., Li, Z., Zhu, Z., Chen, B., Zhang, B., and Xin, X. (2015). Variability and Climate Change Trend in Vegetation Phenology of Recent Decades in the Greater Khingan Mountain Area, Northeastern China. Remote Sensing. 7 (9), 11914–11932. doi:10.3390/rs70911914
Tao, F., Yokozawa, M., Xu, Y., Hayashi, Y., and Zhang, Z. (2006). Climate Changes and Trends in Phenology and Yields of Field Crops in China, 1981-2000. Agric. For. Meteorology. 138 (1-4), 82–92. doi:10.1016/j.agrformet.2006.03.014
Testa, S., Soudani, K., Boschetti, L., and Borgogno Mondino, E. (2018). MODIS-derived EVI, NDVI and WDRVI Time Series to Estimate Phenological Metrics in French Deciduous Forests. Int. J. Appl. Earth Observation Geoinformation. 64, 132–144. doi:10.1016/j.jag.2017.08.006
Tramontana, G., Jung, M., Schwalm, C. R., Ichii, K., Camps-Valls, G., Ráduly, B., et al. (2016). Predicting Carbon Dioxide and Energy Fluxes Across Global FLUXNET Sites With Regression Algorithms. Biogeosciences. 13 (14), 4291–4313. doi:10.5194/bg-13-4291-2016
Tucker, C. J. (1979). Red and Photographic Infrared Linear Combinations for Monitoring Vegetation. Remote Sensing Environ. 8, 127–150. doi:10.1016/0034-4257(79)90013-0
Vrieling, A., de Leeuw, J., and Said, M. (2013). Length of Growing Period over Africa: Variability and Trends from 30 Years of NDVI Time Series. Remote Sensing. 5 (2), 982–1000. doi:10.3390/rs5020982
Wagle, P., Zhang, Y., JIn, c., and XIao, X. (2016). Comparison of Solar‐induced Chlorophyll Fluorescence, Light‐use Efficiency, and Process‐based GPP Models in maize. Ecol. Appl. 26 (4), 1211–1222. doi:10.1890/15-1434
Walther, S., Voigt, M., Thum, T., Gonsamo, A., Zhang, Y., Köhler, P., et al. (2016). Satellite Chlorophyll Fluorescence Measurements Reveal Large-Scale Decoupling of Photosynthesis and Greenness Dynamics in Boreal evergreen Forests. Glob. Change Biol. 22 (9), 2979–2996. doi:10.1111/gcb.13200
Wang, F., Chen, B., Lin, X., and Zhang, H. (2020). Solar-induced Chlorophyll Fluorescence as an Indicator for Determining the End Date of the Vegetation Growing Season. Ecol. Indicators. 109, 105755. doi:10.1016/j.ecolind.2019.105755
Wang, X., Xiao, J., Li, X., Cheng, G., Ma, M., Zhu, G., et al. (2019). No Trends in spring and Autumn Phenology during the Global Warming Hiatus. Nat. Commun. 10 (1), 2389. doi:10.1038/s41467-019-10235-8
White, M. A., de Beurs, K. M., Didan, K., Inouye, D. W., Richardson, A. D., Jensen, O. P., et al. (2009). Intercomparison, Interpretation, and Assessment of spring Phenology in North America Estimated from Remote Sensing for 1982-2006. Glob. Change Biol. 15 (10), 2335–2359. doi:10.1111/j.1365-2486.2009.01910.x
White, M. A., Thornton, P. E., and Running, S. W. (1997). A continental Phenology Model for Monitoring Vegetation Responses to Interannual Climatic Variability. Glob. Biogeochem. Cycles. 11 (2), 217–234. doi:10.1029/97gb00330
Xia, J., Niu, S., Ciais, P., Janssens, I. A., Chen, J., Ammann, C., et al. (2015). Joint Control of Terrestrial Gross Primary Productivity by Plant Phenology and Physiology. Proc. Natl. Acad. Sci. USA. 112 (9), 2788–2793. doi:10.1073/pnas.1413090112
Yang, H., Yang, X., Zhang, Y., Heskel, M. A., Lu, X., Munger, J. W., et al. (2017). Chlorophyll Fluorescence Tracks Seasonal Variations of Photosynthesis from Leaf to Canopy in a Temperate forest. Glob. Change Biol. 23 (7), 2874–2886. doi:10.1111/gcb.13590
Yang, P., van der Tol, C., Campbell, P. K. E., and Middleton, E. M. (2020). Fluorescence Correction Vegetation Index (FCVI): A Physically Based Reflectance index to Separate Physiological and Non-physiological Information in Far-Red Sun-Induced Chlorophyll Fluorescence. Remote Sensing Environ. 240, 111676. doi:10.1016/j.rse.2020.111676
Yang, X., Tang, J., and Mustard, J. F. (2014). Beyond Leaf Color: Comparing Camera-Based Phenological Metrics with Leaf Biochemical, Biophysical, and Spectral Properties throughout the Growing Season of a Temperate Deciduous forest. J. Geophys. Res. Biogeosci. 119, 181–191. doi:10.1002/10.1002/2013jg002460
Yang, X., Tang, J., Mustard, J. F., Lee, J.-E., Rossini, M., Joiner, J., et al. (2015). Solar-Induced Chlorophyll Fluorescence That Correlates With Canopy Photosynthesis on Diurnal and Seasonal Scales in a Temperate Deciduous Forest. Geophysical Research Letters 42, 2977–2987. doi:10.1002/2015GL063201
Yu, L., Liu, T., Bu, K., Yan, F., Yang, J., Chang, L., et al. (2017). Monitoring the Long Term Vegetation Phenology Change in Northeast China from 1982 to 2015. Sci. Rep. 7 (1), 14770. doi:10.1038/s41598-017-14918-4
Zhang, G., Zhang, Y., Dong, J., and Xiao, X. (2013). Green-up Dates in the Tibetan Plateau Have Continuously Advanced from 1982 to 2011. Proc. Natl. Acad. Sci. USA. 110 (11), 4309–4314. doi:10.1073/pnas.1210423110
Zhang, X., Friedl, M. A., Schaaf, C. B., Strahler, A. H., Hodges, J. C. F., Gao, F., et al. (2003). Monitoring Vegetation Phenology Using MODIS. Remote Sensing Environ. 84, 471–475. doi:10.1016/s0034-4257(02)00135-9
Zhang, X., Wang, J., Gao, F., Liu, Y., Schaaf, C., Friedl, M., et al. (2017). Exploration of Scaling Effects on Coarse Resolution Land Surface Phenology. Remote Sensing Environ. 190, 318–330. doi:10.1016/j.rse.2017.01.001
Zhang, Y., Guanter, L., Berry, J. A., Joiner, J., van der Tol, C., Huete, A., et al. (2014). Estimation of Vegetation Photosynthetic Capacity from Space-Based Measurements of Chlorophyll Fluorescence for Terrestrial Biosphere Models. Glob. Change Biol. 20 (12), 3727–3742. doi:10.1111/gcb.12664
Zhang, Y., Xiao, X., Jin, C., Dong, J., Zhou, S., Wagle, P., et al. (2016). Consistency between Sun-Induced Chlorophyll Fluorescence and Gross Primary Production of Vegetation in North America. Remote Sensing Environ. 183, 154–169. doi:10.1016/j.rse.2016.05.015
Zhao, J., Wang, Y., Zhang, Z., Zhang, H., Guo, X., Yu, S., et al. (2016). The Variations of Land Surface Phenology in Northeast China and its Responses to Climate Change from 1982 to 2013. Remote Sensing. 8 (5), 400. doi:10.3390/rs8050400
Zhao, J., Zhang, H., Zhang, Z., Guo, X., Li, X., and Chen, C. (2015). Spatial and Temporal Changes in Vegetation Phenology at Middle and High Latitudes of the Northern Hemisphere over the Past Three Decades. Remote Sensing. 7 (8), 10973–10995. doi:10.3390/rs70810973
Keywords: sun-induced chlorophyll fluorescence, vegetation indices, vegetation phenology, gross primary productivity, latitude
Citation: Wang X, Sun Z, Lu S and Zhang Z (2022) Comparison of Phenology Estimated From Monthly Vegetation Indices and Solar-Induced Chlorophyll Fluorescence in China. Front. Earth Sci. 10:802763. doi: 10.3389/feart.2022.802763
Received: 27 October 2021; Accepted: 12 January 2022;
Published: 15 February 2022.
Edited by:
Yeqiao Wang, University of Rhode Island, United StatesReviewed by:
Yuyu Zhou, Iowa State University, United StatesYi Liu, University of New South Wales, Australia
Copyright © 2022 Wang, Sun, Lu and Zhang. This is an open-access article distributed under the terms of the Creative Commons Attribution License (CC BY). The use, distribution or reproduction in other forums is permitted, provided the original author(s) and the copyright owner(s) are credited and that the original publication in this journal is cited, in accordance with accepted academic practice. No use, distribution or reproduction is permitted which does not comply with these terms.
*Correspondence: Shan Lu, lus123@nenu.edu.cn; Zhengxiang Zhang, zhangzx040@nenu.edu.cn