- 1Key Laboratory of Ecology of Rare and Endangered Species and Environmental Protection (Guangxi Normal University), Ministry of Education, Guilin, China
- 2Guangxi Key Laboratory of Landscape Resources Conservation and Sustainable Utilization in Lijiang River Basin, Guangxi Normal University, Guilin, China
- 3College of Life Sciences, Guangxi Normal University, Guilin, China
- 4College of Geomatics and Geoinformation, Guilin University of Technology, Guilin, China
Vegetation in karst areas is crucial for maintaining fragile local ecosystems, driven by climate change and human activities. Southwest China contains the largest continuous karst zone in the world and its vegetation dynamics are more sensitive to climate changes and human activities. However, previous studies rarely studied the driving roles of vegetation dynamics in karst areas during the last 20 years, and whether climate change or human disturbance factors have dominated the vegetation dynamics are still uncertain. The objective of this work is to study vegetation dynamics and its responses to climate change and human activities from 2001 to 2019 using the normalized difference vegetation index (NDVI). Taking the three karst provinces of southwest China as study area. Vegetation variation characteristics under the influence of climate variations and human activities were distinguished through a residual analysis. The results indicated general greening trends with about 90.31% of the study area experiencing an increase in NDVI and about 9.69% of the area showing a decrease. Within the combination of climate change and human activities, human activities became the dominant factor in the process of vegetation cover improvement and degradation in the study area during 2001–2019, with average relative roles of 62 and 59%, respectively. Temperature made the greatest positive contribution among the climatic factors, followed by precipitation and relative humidity. In contrast, sunshine duration had a negative effect on NDVI in the study area. Human activities have had different effects on the vegetation dynamics of the three karst provinces in southwest China, including destruction of vegetation around some cities.
Introduction
Vegetation links natural elements such as soil, atmosphere and water, and can represent the overall ecological environment of a region to a great extent (Breil et al., 2017). Vegetation dynamics are an essential indicator of global climate change (Zhao et al., 2020). Unlike other types of data, remote sensing data have spatio-temporal continuity, so they are often used to monitor the spatial and temporal distribution characteristics and variation trends of global and regional vegetation, providing a solid foundation for studying the spatial distribution pattern of vegetation and predicting future development trends (Jong et al., 2012). Vegetation indexes reflect the characteristics of surface vegetation and can be used to effectively characterize the details of surface vegetation coverage. The NDVI is very sensitive to the biophysical characteristics of vegetation, and as an important measurement parameter of vegetation growth, it is effectively used to monitor the dynamic changes of vegetation. Many scholars (Eckert et al., 2015; Hou et al., 2015; Chen et al., 2018) have applied NDVI data widely in vegetation status monitoring, ecological environment assessment, crop growth detection and natural disaster monitoring in the world and large areas. Eckert et al. (2015) used Moderate Resolution Imaging Spectroradiometer (MODIS) NDVI to reverse the changes of vegetation cover in Mongolia, and found that the vegetation degradation areas and vegetation restoration areas are closely related to the change of land use types and regional precipitation. Chen et al. (2018) used MODIS NDVI data to distinguish the driving factors of land degradation in Songnen grasslands and quantify the influence of climate change on NDVI dynamics. The impact of climate change on vegetation cover is complex due to the spatio-temporal variation in climate effects and the ecological environment conditions in different regions (Hou et al., 2015).
The influence of climate factors on vegetation dynamics is still unclear, and the spatial difference of the climate-vegetation relationship is also complicated by different vegetation species that have different response sensitivities to climate variables (Nanzad et al., 2019). Therefore, many researchers (Levin 2016; Chu et al., 2018; He et al., 2020) have studied the driving mechanism of vegetation change. Relevant research shows that changes in vegetation cover are affected by climate change and human activities, and the rapid development of remote sensing technology has greatly promoted the research process of large-scale vegetation dynamics change. Baniya et al. (2018) found that NDVI variation is more sensitive to temperature than precipitation in Nepal. Nanzad et al. (2019) proposed that anomalous variation in growing season NDVI was correlated with precipitation and temperature anomalies over Mongolia from 2000 to 2016. Previous researches (Xu et al., 2017; Zhao et al., 2018) have reported general greening trends in mid–high latitudes of the northern hemisphere, which have primarily been related to the climate change based on a long-term time series of land surface observations and on model simulations (Meng et al., 2019). Nevertheless, vegetation variations and the controlling effect of climate vary geographically and temporally. Further, with the continuous strengthening of agricultural activities, ecological construction, urban expansion and other human activities, the influence of human factors on vegetation coverage has become more and more significant on the global scale and over long periods (Ostwald & Chen 2006). Wang Hao et al. (2020) found that the vegetation dynamics of the Otindag Sandy Land in China showed a downward trend during 2001–2017 and that human-driven disturbances had a more profound impact on the vegetation dynamics than climate factors in such a short period, with a general negative influence. Xiu et al. (2019) found a reduction in wetland areas of the Hongjian Nur region was mainly caused by climatic and human factors. They concluded that the contribution of temperature and precipitation to vegetation growth were small and the impacts of human activities on the wetland areas were mainly caused by agricultural irrigation, artificial surface expansion and ecological water use. Gemitzi et al. (Gemitzi et al., 2019) suggested the economic crisis in Greece during the last decade, which caused a dramatic drop in urban expansion, positively impacted vegetation productivity in urban areas as well as sites protected by environmental legislation. Therefore, human disturbance complicates the influence of climate change on vegetation dynamics.
Southwest China, which has tropical and subtropical monsoon climates, contains the largest continuous karst landforms in the world. Guizhou, Guangxi and Yunnan Provinces have received a lot of attention since 82% of China’s karst landforms are located here, which are all characterized by fragile environment, broken high topographical complexity (including steep mountain topography) and poor soil conditions (Peng et al., 2020). Owing to high population stress together with the extremely fragile ecosystem, more severe rocky desertification occurred in this region. More than 20% of the karst regions have deteriorated greatly and are suffering rocky desertification (Cai et al., 2014), which further increases the poverty of the population. Karst, which develops on soluble rock, is a fragile ecosystem that is vulnerable to rocky desertification affected by large population stress (Peng et al., 2020). The three karst provinces in southwest China was selected for study because of its ecological environment is highly sensitive to external disturbances, including climate change and human-driven disturbance (Cai et al., 2014; Hou et al., 2015; Peng et al., 2020). In karst areas, forests are generally the main types of land cover (Cai et al., 2014). Once forests in a karst area are destroyed, they have difficultly recovering through natural succession because the rocky desertification is usually followed by unique geological and hydrogeological conditions. Since most previous studies have concentrated on climate variables (Hou et al., 2015; Deng et al., 2018), a systematic investigation of vegetation sensitivities to different environmental factors, including both climate variables and human disturbance, is needed. To protect and promote the ecological environment of west China, the Chinese government conducted the “Grain to Green Program” (GTGP) in 2000. Some researches of vegetation greenness in the karst area demonstrated general greening trends from 2000 to 2011 (Xu et al., 2014), so whether climate factors or human-driven disturbances have developed the ecological restoration in recent years is uncertain (Cai et al., 2014).
An accurate quantitative analysis of the contributions of climate change and human activities to vegetation NDVI is crucial for elucidating the relevant driving mechanisms in the three karst provinces of southwest China. Two decades (2001–2019) of climate variables and vegetation dynamics in the three karst provinces of southwest China from the GTGP in 2000 were examined 1) to investigate the characteristics of climate change and vegetation dynamics over these two decades by the coefficient of variation and the Theil-Sen median trend analysis and explore the significance of NDVI change trend through use of the Mann-Kendall test, 2) to analyze the vegetation sensitivities to individual climate variables (temperature, precipitation, sunshine duration and relative humidity), and 3) to explore the influence of human disturbance on the climate-vegetation relationship. The findings of this study contribute to our understanding of vegetation response processes to climate change and provide scientific bases and technical support for the ecological restoration in the three karst provinces of southwest China.
Materials and Methods
Study Area
The study area in southwest China encompasses three provinces: Guizhou, Guangxi, and Yunnan (approx. 793,984 km2) (Figure 1). This area has the most serious rocky desertification of fragile subtropical karst environment caused by the climate change and disturbance of unreasonable social and economic activities (Cai et al., 2014). The karst landform occupies approximately 40% of the study area (approx. 321,000 km2). It is the most aggregated and typical karst landform region of China. The three provinces contain up to 82% of the rocky desertification areas (Jiang et al., 2014). Additionally, it is also the main area of the GTGP project, and ecological afforestation plots have been implemented in each county around this area. Thus, the three provinces were chosen as study area.
Data Collection and Quality
The NDVI dataset used to analyse the vegetation dynamics in this study are from the Moderate Resolution Imaging Spectroradiometer (MODIS). The Terra MODIS NDVI Monthly L3 Global 1 km SIN Grid V006 dataset (MOD13Q1.A2020001, tile h26v05\h26v06\h27v05\h27v06) was obtained from NASA’s Earth Observing System (http://reverb.echo.nasa.gov/reverb/) for the period of January 2001 to December 2019 with a spatial resolution of 1 × 1 km2. The annual average NDVI time series data of the study area were obtained with the Maximum Value Composite method, which minimizes atmospheric effects, scan angle effects, cloud contamination and solar zenith angle effects.
The climate dataset used in the study was from the Meteorology Information Center of the Chinese National Bureau of Meteorology (http://data.cma.cn), which has strict precision control and good quality. For missing data, this study used SPSS 22.0 (IBM SPSS Statistics, New York, United States) to interpolate the missing data. Geographical location will have a certain impact on the interpolation accuracy of meteorological data. In order to improve the accuracy of interpolation results, ANUSPLIN interpolation software package (Hutchinson 1998) was selected to introduce a digital elevation model (DEM) as covariant to interpolate meteorological data. A high-precision meteorological spatial dataset was obtained with the same spatial and temporal resolution and consistent projection as NDVI. Data was verified based on meteorological stations in the three karst provinces by interpolating some data and then verifying the remaining data. The simulation test showed that the proposed method has high accuracy (see the results in Supplementary Material). The dataset included the monthly mean temperature, the monthly precipitation, the monthly average sunshine duration, and the relative humidity of 299 national standard weather stations during 2001–2019 (Figure 1).
DEM data comes from SRTM (Shuttle Radar Topography Mission) of American Space Shuttle Endeavour and was used as the source for elevation. This dataset used a nearest neighbor resampling algorithm based on the latest SRTM V4.1 data, with an accuracy of 1 km. The DEM of the study area was obtained by clipping, which is mainly used to assist in generating time series of meteorological factors.
Methods
Theil-Sen Median Analysis and Mann-Kendall Significance Test
The Theil-Sen Median analysis is a non-parameter estimation method and was applied to analyze the dynamic variation of NDVI. The Theil-Sen Median analysis uses median values to estimate the variation trend of NDVI in each pixel, which can reduce the errors resulting from abnormal values of the time series NDVI and enhance the precision of estimation. The calculation of the trends in NDVI time series was as follows (Peng et al., 2015):
Where and are the NDVI value in the years and, respectively, and, with as the length of the time series. is the magnitude of the NDVI variation trend with, indicating an upward trend of vegetation cover during the study period and, indicating a downward trend.
The significance of the NDVI variation trend was tested using the M-K Significance test. The M-K Significance test is a non-parametric significance test. It is widely used to investigate variation trends of environmental, geographic, climatic, and hydrological time-series data because it accepts data in either a random order or a particular order, and the results are less affected by abnormal values (Li et al., 2019). In this study, the non-parametric M-K test was applied to test and assess the significance of linear trends in NDVI sequences. The M-K test statistic was computed as follows:
Where and are the NDVI values in the and years, respectively, and indicates the length of time series, and
To calculate the variance,
Where m is the number of the tied groups in the time-series data and is the number of data points in the tied group. When n ≥ 10, the test statistic, S, presents a normal distribution and the Z-value can be applied to detect the variation trends:
Under a given significance level, there is a threshold of the normal distribution Z1–a/2. When |Z| ≤ Z1–a/2, the null hypothesis is accepted, and when |Z| > Z1–a/2, the alternative hypothesis is accepted, indicating a significantly and monotonically increasing or decreasing trend in a time-series data. For instance, when |Z| > 1.96, it suggests that the linear variation trend is statistically significant at the p < 0.05 level. When |Z| > 2.58, it suggests that the linear variation trend is statistically significant at the p < 0.01 level.
Multiple Regression and Residual Analysis
Due to the lack of long-term observation data about the effects of anthropogenic effects on vegetation NDVI, it is difficult to directly quantified the effects. Therefore, the multiple regression and residual analysis were selected to distinguish the relative roles of climate variations and human activities on vegetation dynamics in this study. The methodology originated from that NDVI is only influenced by climate change under natural conditions. So it is easy to be understood for this method that the NDVI variation characteristics should be aligned with those of climate factors without the impacts of anthropogenic effects (Evans & Geerken 2004; Liu et al., 2013; Burrell et al., 2018). The multiple regression and residual methods were used to assess the impacts of climate factors on vegetation variation (Li et al., 2012). Four climate factors that mainly affect vegetation (temperature, precipitation, sunshine duration and relative humidity) (Sun et al., 2015), were used as independent variables for regression with NDVI values as responding variables. The variance inflation factor (VIF) was also employed to assess the multi-collinearity among four climate variables. It is calculated by taking the ratio of the variance of all given model’s betas divide by the variane of a single beta if it were fit alone. Normally, if the VIF is greater than 10, multicolinearity is likely present and we should consider dropping the variable. The calculation of VIFi was as follows (Roman et al., 2016):
Where
The four climate factors were screened by VIF analysis, which showed that all the VIF values were less than 5 (see Supplementary Material). Therefore, the multiple regression model was conducted for the four climate factors. The Eq. 8 characterizing the correlations among vegetation NDVI and the four climate factors were calculated and obtained through regression analysis. Then, the mean annual temperature, the accumulated annual precipitation, the accumulated annual sunshine duration, and the mean annual relative humidity dataset from 2001 to 2019 were respectively bought into the Eq. 8 to gain the vegetation NDVIC. The predicted values of NDVIC were considered as the impact of climate factors on vegetation NDVI under the absence of anthropogenic effects. Finally, the method of residual analysis was chosen to distinguish the relative contribution of anthropogenic effects for vegetation coverage (Li et al., 2017). The formula is as follows:
Where NDVIC(i,t) is the variable affected by climate factors for the ith predictive NDVI in the tth year, A is the regression coefficient for the mean annual temperature X1(i, t), B is the regression coefficient for the accumulated annual precipitation X2(i, t), C is the regression coefficient for the accumulated annual sunshine duration X3(i, t), D is the regression coefficient for the mean annual relative humidity, and c is a constant.
Without considering other non-deterministic factors, NDVI residual is the influence of human factors on vegetation cover change (Li et al., 2015; Jiang et al., 2017). Therefore, the anthropogenic effects on vegetation coverage were identified as the whole impacts under the absence of climate change effects for this study. The formula is as follows:
Where NDVIH is the value of residual error, that is, the NDVI value is only affected by anthropogenic effects. If the result is positive, human activities promote vegetation NDVI; a negative trend indicates an inhibition of vegetation NDVI. NDVIobs is the remote-sensing data value, and NDVIC is the vegetation NDVI value under the influence of climate change alone. Furthermore, the results of NDVIH and NDVIC were analyzed for this study from 2001 to 2019.
Analysis of Relative Roles
Considering data availability and comparability, we selected three anthropogenic factors, i.e., the population density (PD), nighttime light (NTL) data and the land use/cover change (LUCC) information, which were often used as the main proxies for analyzing human activities on vegetation change (Geldmann et al., 2015; Qiu et al., 2018). The PD data were collected from 2001 to 2019 originated from WorldPop Population Counts (https://www.worldpop.org/geodata/listing?id=76). The Operational Linescan System (OLS) onboard the U.S. Defense Meteorological Satellite Program (DMSP) was most commonly selected for nighttime light sensor, which was employed to demonstrate economic activities. The DMSP-OLS derived the version 3 nighttime light (NTL) dataset is a freely available product obtained from the annual NPP-VIIRS-like nighttime light data, Harvard Dataverse (https://dataverse.harvard.edu/dataset.xhtml?persistentId=doi:10.7910/DVN/YGIVCD&version=3.1).
PD data and NTL data are available annually since 2001 at a resolution of 1 km2. LUCC is a signal that directly indicates the effects of human activity on environmental change on the Earth’s land surface. LUCC data is provided by the Data Center for Resources and Environmental Sciences of the Chinese Academy of Sciences (https://www.resdc.cn/Default.aspx). LUCC data of study areas derived from China’s current land use datasets for the period of 2000–2020, which is mainly based on the Landsat TM/ETM’s remote sensing images. Spatial resolution is 30 m based upon the national standard of the Classification of Land Use Status (GB/T21010-2017). A human-computer interaction interpretation method was conducted by the first-level standard classification system. The study area was divided into seven categories: cultivated land, forest land, grass land, water area, construction land, unused land and (Wang et al., 2021). Land use transfer matrix was employed to analyze a comprehensive land use dynamics degree (Liu et al., 2019). All the data of anthropogenic factors should be processed using Extract by Mask, Projections, Transformations and Resample in ArcGIS.
The ideas and methods for calculating the relative effect proposed by Xu et al. (2019) were used to determine the relative effect of climate variations and human activities in the process of vegetation change, as shown in Figure 2. First, through a residual analysis, we derived the NDVI value induced by each of climate variations and human activities for each pixel. The impact of climate variations was measured by predictive NDVI, whereas the impact of human activities was measured by NDVI residuals. Then, the trends in predicted NDVI and residual NDVI were combined with the trend in observed NDVI during 2001–2019, and three evaluation methods were built according to different scenarios to assess the relative roles of climate variations and human activities in vegetation change, as shown in Table 1. Comparison of NDVI between 2001 and 2019 are shown in the Figure 3.
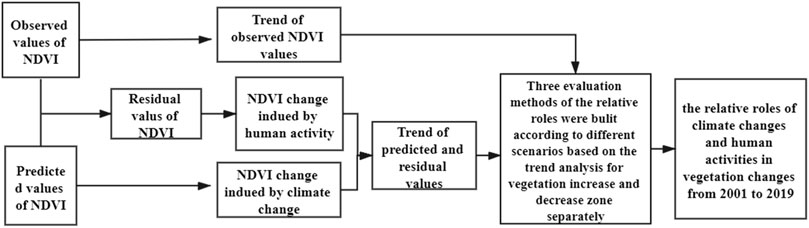
FIGURE 2. Flow chart of the process for assessing the relative roles of climate variations and human activities in the process of vegetation cover change.
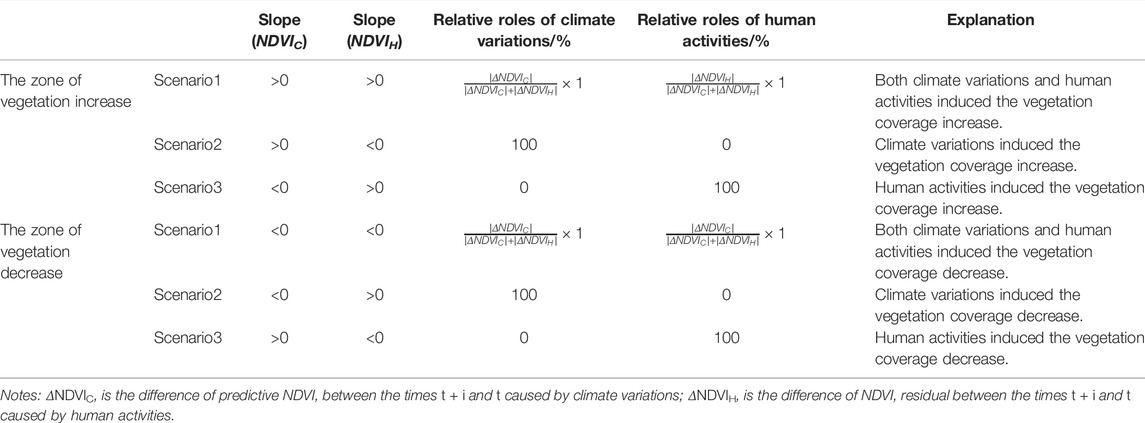
TABLE 1. Methods for assessing the relative roles of climate change and human activities on vegetation variation.
Correlation Analysis
The correlation coefficient is widely selected to detect the internal relationship from multiple variables. Partial correlation coefficients report the linear relationship between two variables when regarding other variables as controls. Therefore, in this study, the partial correlation coefficient was applied to dissociate the impacts of temperature, precipitation, sunshine duration, and relative humidity on vegetation cover change. The calculation of the partial correlation coefficient is as follows:
Where
Results
Temporal Vegetation Dynamic Changes
The interannual variation of NDVI vegetation in southwest karst provinces of China are shown in Figure 4. Since the implementation of the GTGP in 2000, NDVI vegetation of the study area has been progressively improved, with a linear tendency of 0.003/a from 2001 to 2019 (R2 = 0.9274, p < 0.05). Over the past 20 years, the mean values of NDVI vegetation range from 0.771 in 2001 to 0.822 in 2017, implying that the overall variations of vegetation coverage in the study region proposed a trend of gradually increasing.
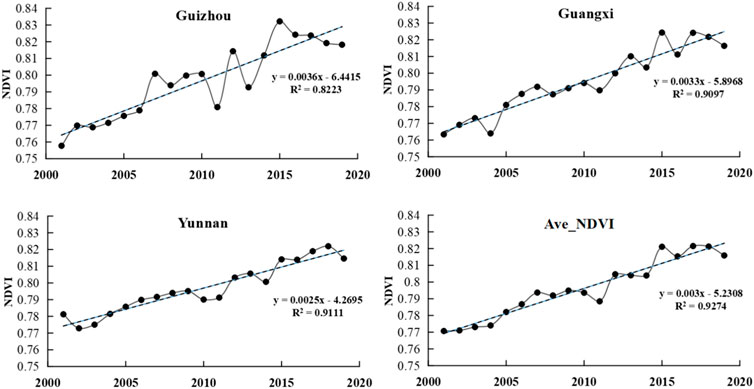
FIGURE 4. Variation of annual NDVI in the three karst provinces of southwest China during 2001–2019.
From different time periods, before 2008, NDVI of vegetation in the three southwest karst provinces increased significantly, and vegetation restoration improved continuously. However, from 2008 to 2019, it showed a fluctuating upward trend. During the four periods of 2009–2011, 2012–2014, 2015–2016 and 2017–2019, there was a continuous downward trend, and the NDVI of vegetation decreased by 0.0081/a, 0.0009/a, 0.0070/a and 0.0068/a, respectively. According to the changes in different provinces, the NDVI of vegetation in Guizhou has changed the most over the past 20 years, whereas that in Yunnan has changed the least, with the annual change rates of 0.0036/a and 0.0025/a respectively. Since 2009, the climate in southwest China has been warm and dry, and the sustained abnormal high temperature has been observed and recorded in summer over four consecutive years, showing obvious drought. The occurrence of natural disasters such as extreme weather, has an impact on NDVI, which may be the main reason for the reduction of vegetation coverage in some years in the three southwest karst provinces.
Although the vegetation coverage in the southwest karst landform tended to improve and the annual difference of NDVI was small, there was obvious spatial heterogeneity in the vegetation coverage change. In order to further describe the spatial change characteristics, the vegetation variation trend was divided into six grades (extremely significant decline, significant decline, slight decline, extremely significant increase, significant increase and slight increase) according to the Slope and significance level of NDVIobs, as shown in Table 2. The NDVI of vegetation in the study area showed an upward trend, which was larger than that of a downward trend from 2001 to 2019 (Figure 5). This demonstrates that over the 20 years since the GTGP began, the eco-environment in the study area has significantly improved. The characteristics of the spatial pattern evolution are shown in Figure 5: about 90.31% of the study area experienced an upward trend of vegetation coverage, whereas about 9.69% of the area showed a decreasing trend. NDVI with significantly decreased values was primarily concentrated in major cities, such as Kunming, Nanning, Guiyang, Baoshan, Dali, Qujing and Yulin.
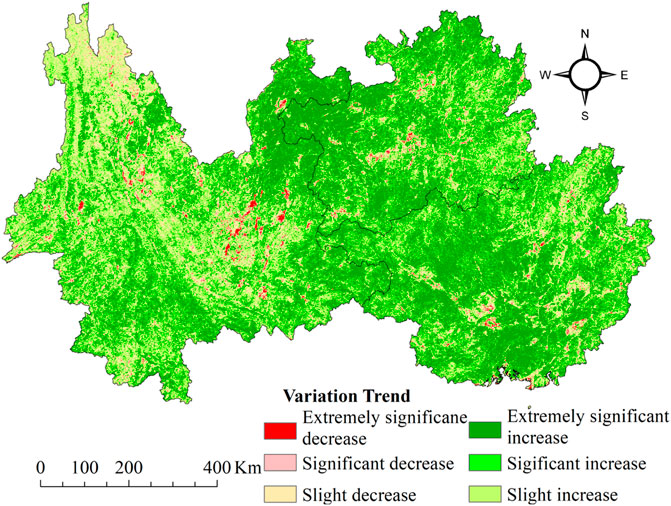
FIGURE 5. The spatial distribution of NDVI variation trends in the three karst provinces of southwest China from 2001 to 2019.
Relative Roles of Climate Change and Human Activities in the Increased Area of Vegetation Cover
Under the joint action of climate change and human activities, the entire vegetation coverage in the study area has been improved. The vegetation improvement area mentioned in this paper refers to the area with Slope>0, accounting for 90.31% of the total study area (Table 2). According to the three possible scenarios of the relative effects of climate change and human activities on vegetation improvement, the relative effects of climate change and human activities in the study area from 2001 to 2019 were quantitatively distinguished. The relative effect of human activities on vegetation improvement in the southwest karst provinces was slightly stronger than that of climate change (Figure 6A). The average relative effect of human activities was 62%, and that of climate change was 38%. Thus, human activities over the past 19 years were the leading factors for vegetation improvement in the three karst provinces of southwest China.
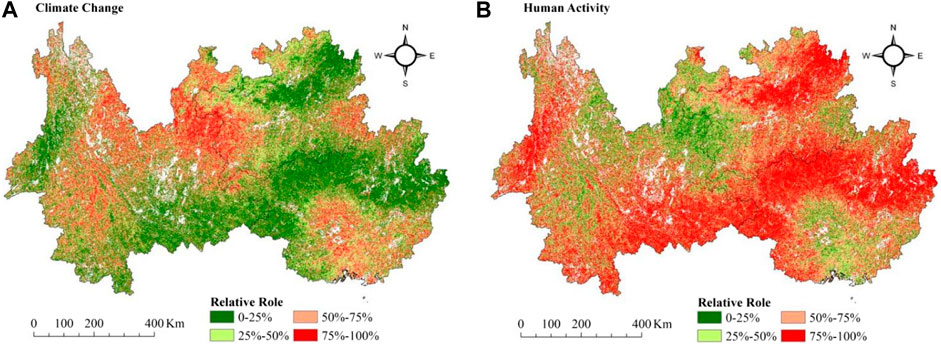
FIGURE 6. The relative role of climate change and human activities on vegetation improvement in the three karst provinces of southwest China from 2001 to 2019.
The relative effects of climate change and human activities show obvious spatial heterogeneity. As shown in Figure 6B, the area where vegetation improvement was mainly caused by human activities (the relative role>50%) accounts for 66.02% of the total improved area, mainly distributed in the southeast of Yunnan, the northwest and southwest of Guangxi, and the northeast of Guizhou. However, the vegetation improvement areas mainly caused by climate change accounted for 33.95% of the total improvement areas, mainly distributed at the junction of Yunnan and Guizhou and the central part of Guangxi. From the perspective of spatial distribution, the effects of climate change and human activities on vegetation improvement areas are intertwined, and human activities exert a major influence on vegetation variations in study areas where vegetation coverage has increased significantly.
Relative Roles of Climate Change and Human Activities in the Decreased Area of Vegetation Cover
Climate change and human activities not only promote the improvement of vegetation coverage in the southwest karst provinces of China, but also cause the vegetation vitality in some areas to decline, resulting in different degrees of vegetation degradation, deterioration of ecological environment and aggravation of rocky desertification. The vegetation degradation area refers to the area with Slope<0, accounting for 9.69% of the total study area (Table 2). Similar to the improvement areas, we found that the average relative effect of human activities on the vegetation degradation area in the southwest karst provinces of China from 2001 to 2019 was 59%, and the average relative effect of climate change was 41%. Therefore, human activities are still the main factor causing vegetation degradation in the three southwest karst provinces of China.
Climate change and human activities have jointly led to vegetation degradation in some areas of the three southwest karst provinces, but there is a big spatial difference in their relative effects. As shown in Figure 7, the area where vegetation degradation was mainly caused by climate change (relative role>50%) accounted for 37.24% of the total degraded area, and was scattered in central Yunnan and central Guangxi. The area of vegetation degradation mainly caused by human activities (relative role>50%) accounted for 62.76% of the total degraded area, mainly distributed in northeastern Guangxi, central Guizhou, and western and southern Yunnan. In the extremely significant decline and significant decline areas, the vegetation degradation areas mainly caused by human activities accounted for more than 50% of the total area.
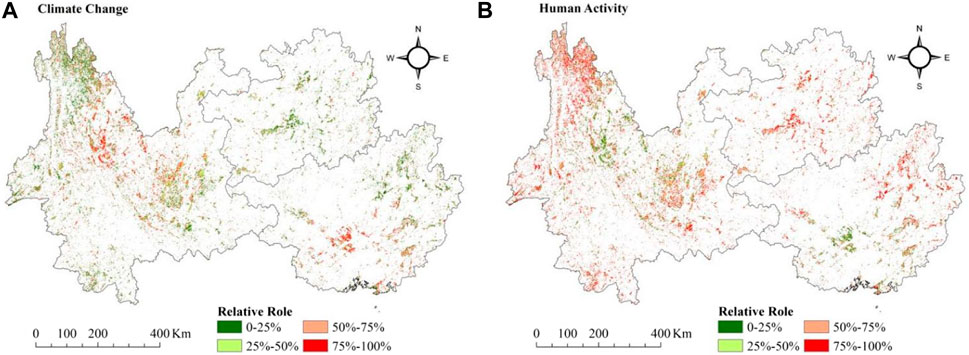
FIGURE 7. The relative roles of climate change and human activities on vegetation degradation in the three karst provinces of southwest China from 2001 to 2019.
Discussion
Effect of Climate Change on Vegetation Cover in the Three Karst Provinces
A number of findings have suggested that climate-driven variability is major contribution for trends in vegetation productivity of China (Piao et al., 2006; Deng et al., 2018). In this paper, the influence of climate change in the process of vegetation cover change was isolated through the residual method. According to the principle of the residual method, the impact of climate change on NDVI can be better isolated only when NDVI is significantly correlated with climate indicators. At present, the correlation analysis among NDVI and climate indicators mainly focuses on two climate indicators, temperature and precipitation, while the relationship among other climate indicators and NDVI is seldom studied. Considering the four indicators of precipitation, temperature, relative humidity and sunshine duration together to access the relationship among NDVI and climate indicators will improve comprehensive interpretation of climate changes on vegetation cover changes.
From Figure 8, the area with improved vegetation is obviously larger than the area with degraded vegetation, and the area with increased vegetation accounts for 82.08% of the total area, of which 20.78% of the total area had extremely significant rise or significant rise, mainly distributed in the central and southern parts of Guangxi and the junction of Yunnan, Guangxi and Guizhou provinces. One area with significant vegetation improvement is located in the ecological protection priority area from southeast Yunnan to west Guangxi, one of the three major biodiversity centers in China. It is rich in precipitation and sufficient in heat, which is suitable for plant growth. At the same time, the continuous promotion of ecological environment restoration projects in the area by the three provincial governments is also conducive to the continuous improvement of the ecological environment. In contrast, since the impacts of human activities on vegetation away from human settlements, especially primary forests, were non-significant (Nesha et al., 2021). Furthermore, the extremely significant decline and the significantly decreased areas only account for 1.18% of the total area and are scattered in Guangxi and Yunnan, especially around cities. The areas with a significant downward trend (Slope<0) are related to man-made scrubland, field crop and well-developed urban building. Those areas are usually in proximity to roads or around county seats, indicated that numerous engineering projects and rapid urbanization play a leading role for the degradation of vegetation, especially in major cities, such as Nanning, Liuzhou, Dali and Kunming. This indicates that human-driven urban expansion may be another factor that leads to the decline of NDVI.
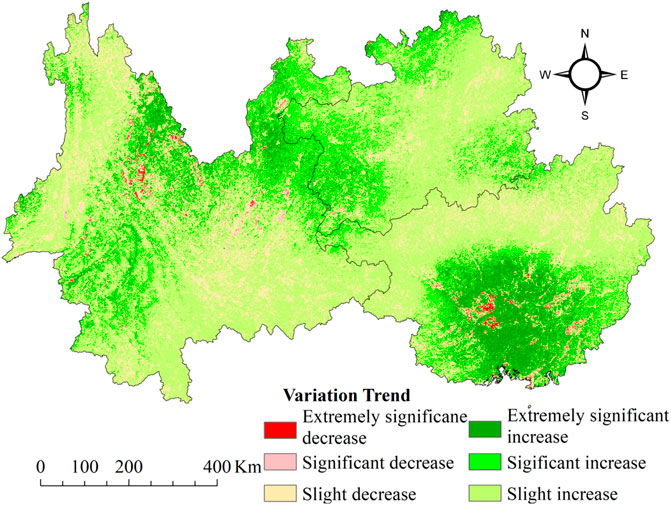
FIGURE 8. The spatial distribution of the predicted NDVI variation trend in the three karst provinces of southwest China from 2001 to 2019.
The feedback of vegetation coverage on climate variations (0.003/a) in the three karst provinces were more sensitive during 2001–2019 compared with results from other areas. For example, the NDVI increased at rates of 0.0005/a in northwest China and -0.0009/a on the Mongolian Plateau (Dai et al., 2011; Bao et al., 2014). Due to the large storage capacity and high infiltration rate of karst carbonate fissures and fractures, the soil-epikarst system plays an important role in runoff generation and may further weaken the effect of human activities (e.g., land use change) on water discharge. Therefore, this particular soil-epikarst architecture is responsible for the difference in the effects of climate and human activities on water discharge between karst and nonkarst areas.
From Figure 9A, 72.07% of the study area is positively correlated with precipitation, with 4.09% of the area having a significant positive correlation (p < 0.05). From Figure 9B, 70.91% of the total study area has a positive correlation with temperature, slightly less than precipitation. About 6.74% of the total area has a significant positive correlation (p < 0.05). As shown in Figure 9C, about 64.85% of the total study area is positively correlated with relative humidity, of which about 10.32% of the area has significant positive correlation (p < 0.05). From Figure 9D, 74.36% of the study area is negatively correlated with sunshine duration, with 8.01% of the area having a significant positive correlation (p < 0.05).
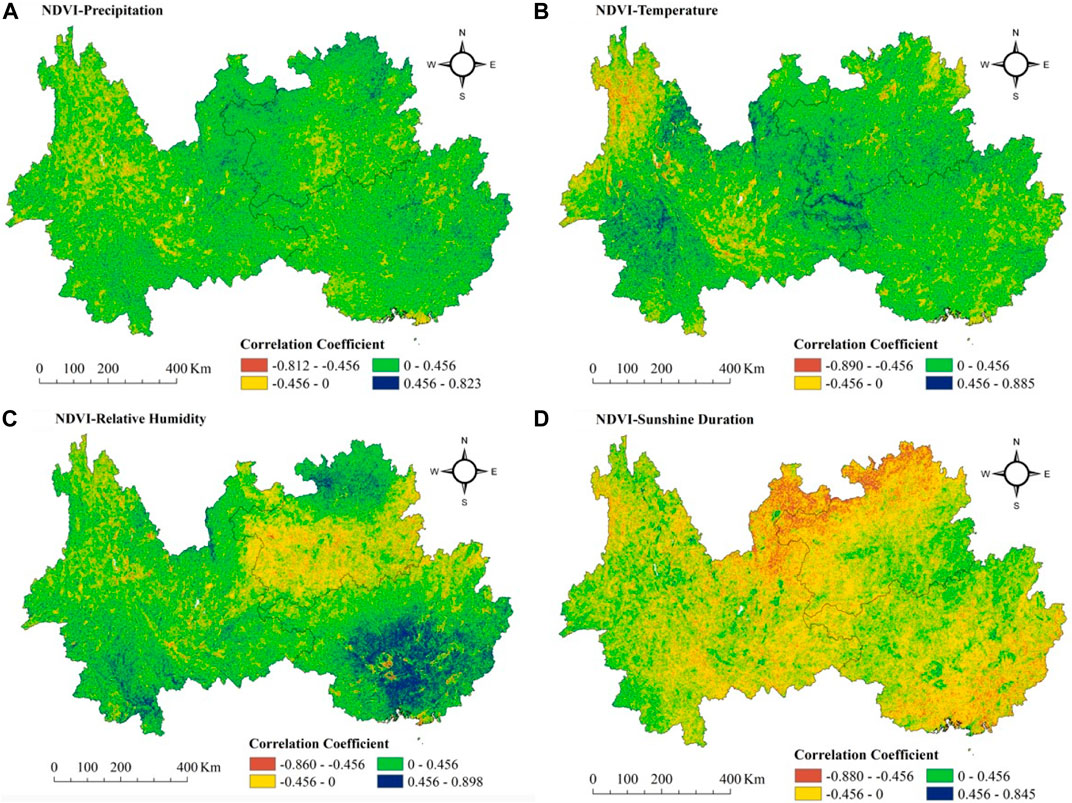
FIGURE 9. The correlation among NDVI and climate factors in the three karst provinces of southwest China.
According to the correlation analysis results, the NDVI of vegetation in the southwest karst provinces from 2001 to 2019 was positively correlated with air temperature, precipitation and relative humidity, with partial correlation coefficients of 0.120, 0.116 and 0.107, respectively, listed from most to least influence. In contrast, the NDVI was negatively correlated with sunshine duration, with a partial correlation coefficient of -0.15. Thus, vegetation growth in the southwest karst provinces is affected by climate change, which positively promotes and negatively inhibits growth, and the degree of influence is spatial heterogeneous.
The systematic research on the NDVI variations and their relationship from climate indicators implies the complex spatial-temporal heterogeneity in climate change for the study area and even for the karst ecosystem, which influences the obtain of more representative conclusions and the ability to surmise general rules regarding this region. Moreover, Our results do not only present a research framework for climate change adaption or ecosystem restoration in a degraded region but also contribute knowledge to the origin and mechanism of vegetation variations. Taking climate effects for instance, more efforts or measures should be emphasized for future restoration and reconstruction processes based on the important scientific and practical significance, including several high temperature resistance of engineering measures (NDVI is closely related to temperature).
Effect of Human Activities on Vegetation Coverage in the Three Karst Provinces
Human activities have always been one of the critical factors for vegetation changes, especially since the full implementation of the GTGP in China in 2000 (Erb et al., 2018). Hence, it is also essential to accurately quantify the contributions of human activities to changes in vegetation. As explained above, human activities exhibited spatially heterogeneous effects on vegetation growth. Human activities displayed significant positive effects on vegetation growth in farming areas and GTGP areas (Figure 10), except around the cities and roads, indicating that farming and afforestation were beneficial to vegetation growth in the three karst provinces (Peng et al., 2020). The areas with positive and negative NDVIH trends accounted for 88.43 and 11.58% of the total study area, respectively. The above results show that human activities had dual effects on regional vegetation growth. On the one hand, human activities promoted vegetation growth in most regions; on the other hand, human activities inhibited vegetation growth in other regions. On the whole, human activities played a dominant role in promoting regional vegetation growth.
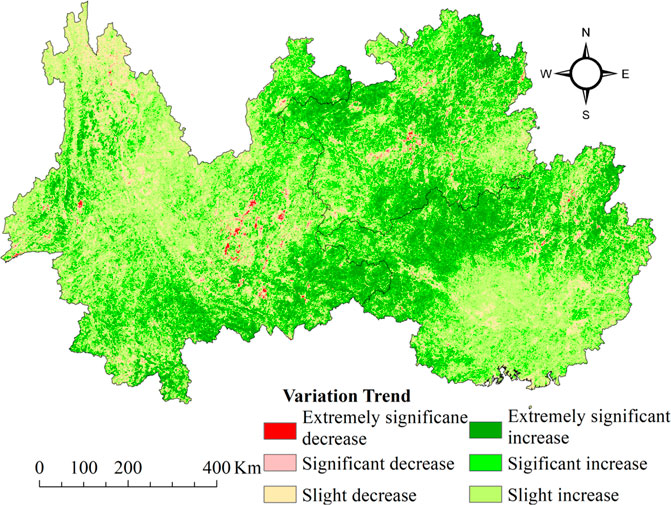
FIGURE 10. Variation trend of residual NDVI in the three karst provinces of southwest China from 2001 to 2019.
Under the influence of human activities, the extremely significant increasing areas with vegetation cover accounted for 11.20% of the total study area, mainly distributed in southeastern Yunnan, northwestern Guangxi and most parts of Guizhou (Figure 10). Numerous studies (Wang et al., 2015; Qiao et al., 2020) have shown a vegetation greening trend in southwest China over the past two decades and attributed it to the afforestation and conservation of natural forests. The great changes of NDVI resulting from ecological restoration projects in recent decades were considered to be the driver for vegetation greening in southwest China (Brandt et al., 2017). To combat rocky desertification of karst in southwest China, a great deal of ecological restoration engineering, including the GTGP, Natural Forest Protection Project and the Public welfare Forest Protection, has been carried out since 2000. Additionally, the central government initiated “A General Plan Outline for a Program to Comprehensively Address Karst Rocky Desertification (2005–2015)” in southwest China. During the process of rocky desertification control and restoration engineering, the annual afforestation area (forest plantation, closing hill for afforestation and degraded forest restoration) increased by approximately 2.3*105 km2 from 2006 to 2016, while the cumulative afforestation area reached nearly 3.4*106 km2.
The areas with a negative trend of NDVI residuals were scattered, of which the areas with significant decline and extremely significant decline accounted for only 0.74% of the total study area, primarily around cities (Figure 10). Those areas are usually in proximity to roads or around county seats, suggesting that numerous engineering projects and rapid urbanization play a leading role for the degradation of vegetation, such as some problems from the population-economy pressure, were considered to be one of the main reasons for vegetation degradation (Yu et al., 2014; Zhou et al., 2015; Zheng et al., 2019). However, the relationships among various factors remain unclear, and the response of vegetation to climate change and anthropogenic factors in the karst area of China has not been well established. Scholars hold differing views about the relationship among vegetation growth and anthropogenic factors (Zhou et al., 2015; Li et al., 2019), which need further validation.
Substantial population growth, rapid economic development and drastic land use/cover change have been the major sources of human activities that influenced the vegetation change. Therefore, the PD and NTL data were measured as the anthropogenic factors to reflect the relationship between NDVI and human activities for the three karst provinces. According to Figure 11, the NDVI in the southwest karst provinces was negatively correlated with the PD and NTL (p < 0.05), which suggests that the population pressures and economic growth could potentially will ultimately influence on the vegetation patterns and urban development of a region (Abdus et al., 2017). From Figure 11A, 62.35% of the study area is negatively correlated with NDVI, with 19.95% of the area having a significant negative correlation from PD (p < 0.05). From Figure 11B, 48.32% of the study area is negatively correlated with NDVI, with 12.95% of the area having a significant negative correlation from NTL (p < 0.05).
In addition, the land use/cover change (LUCC) information is also a key aspect of regional environment change, and in a sense indicates the impacts of human activities on natural environment. Figure 12 shows Spatial transformation variation map of land use type in the three karst provinces between 2000 and 2020. Results demonstrate that study area’s land use pattern has experienced significant variations, which were primarily characterized by the transformation of cropland to forest land, forest land to cropland and grassland, and grassland to forest land, with the conversion areas of 75,318 km2, 70,043 km2, 59,195 km2, and 56,970 km2, respectively. LULC change frequently have a effect on regional environmental ecology and adaptability of biodiversity when the results of land use change were beneficial to economic development and social benefits and interests.
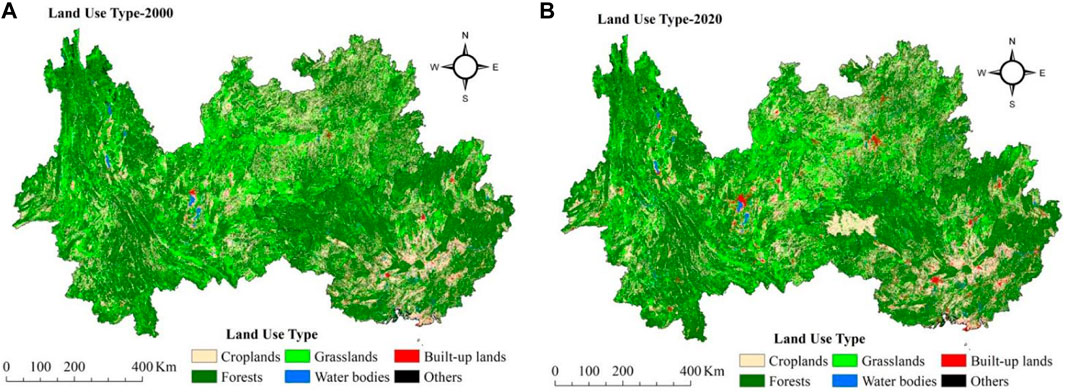
FIGURE 12. Spatial transformation variation map of land use type in the three karst provinces of southwest China between 2000 and 2020 (km2).
Land use transfer matrix is one of the most effective and common ways to explore the internal conversions of land use between different land use types (Huang et al., 2021). Furthermore, it was found that forest land extremely significant increase by 33415 and 26,010 km2 over the past two decades, largely because of transitions from cropland and grassland, which accounted for 47.71 and 43.94%, respectively. Afforestation not only gives farmers higher economic benefits in mountains of Southwest China, but also plays a positive role in suppressing soil degradation and improving biodiversity conservation, which further promotes the conversion of cropland or grassland to forest land (Huang et al., 2021). The increased forest land mainly occurred in the western mountainous areas of the three karst provinces (Figure 13). This might be due to the three provinces are mainly plateau and mountain areas with poor living environments. Actually, the implementation of the GTGP since 2000 prevents the rebound of returning land and enhances sustainable developments (Qu et al., 2020). It was obvious from Figure 13 that rapid big city expansion resulted in agricultural land loss from cropland, forest land and grassland, such as Nanning, Liuzhou, Guilin, Guiyang, Zunyi and Kunming.
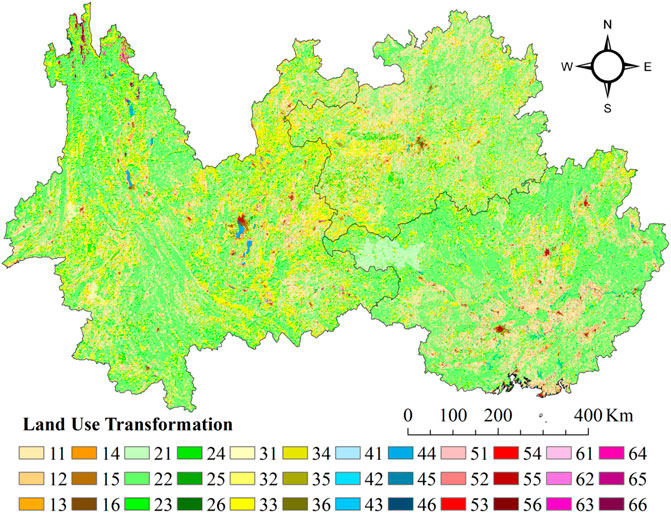
FIGURE 13. Transfer matrix of land use in the three karst provinces of southwest China between 2000 and 2020 (km2). Notes: 11-Transfer cropland to cropland, 12-Transfer cropland to forest land, 13-Transfer cropland to grassland, 14-Transfer cropland to water bodies, 15-Transfer cropland to bulit-up land, 21-Transfer forest land to cropland, 22-Transfer forest land to forest land, 23-Transfer forest land to grassland, 24-Transfer forest land to water bodies, 25-Transfer forest land to bulit-up land, 26-Transfer forest land to others, 31-Transfer grassland to cropland, 32-Transfer grassland to forest land, 33-Transfer grassland to grassland, 34-Transfer grassland to water bodies, 35-Transfer grassland to bulit-up land, 36-Transfer grassland to others, 41-Transfer water bodies to cropland, 42-Transfer water bodies to forest land, 43-Transfer water bodies to grassland, 44-Transfer water bodies to water bodies, 45-Transfer water bodies to bulit-up land, 46-Transfer water bodies to others, 51-Transfer bulit-up land to cropland, 52-Transfer bulit-up land to forest land, 53-Transfer bulit-up land to grassland, 54-Transfer bulit-up land to water bodies, 55-Transfer bulit-up land to bulit-up land, 56-Transfer bulit-up land to others, 61-Transfer others to cropland, 62-Transfer others to forest land, 63-Transfer others to grassland, 64-Transfer others to water bodies, 65-Transfer others to bulit-up land, 66-Transfer others to others.
Limitations
In this study, a methodology that can be used to quantitatively evaluate the contributions of climatic and human factors to vegetation cover in southwest China was designed by selecting NDVI as the evaluation indicator. However, the limitations of this study should also be recognized. First, the results of this study were not verified by experimental data. Second, due to the lack of data on local rocky desertification, determining the contributions of some human activity factors, such as GDP, human population density, and afforestation area, to vegetation NDVI requires further research. Therefore, research that focuses on more accurately quantifying the influences of climatic and human factors and on establishing a quantitative method of isolating the contributions of different human factors needs to be further explored. Third, the scale represents the temporal and spatial dimensions that are used for measuring ecological processes (Guo et al., 2021). The relationship between vegetation NDVI and its driving forces at one scale may not be applicable to another scale (Xu et al., 2014). Thus, future research that assesses the contributions of climatic and human factors to NDVI changes of the southwest karst provinces in China can be improved by fusing multi-source data at a higher spatial resolution and on a long-term scale.
Finally, mathematical models were constructed to establish regression equations and reveal the influence of human activities on vegetation (Wang et al., 2020). According to the residual sequence model obtained from the trend analysis, if the trend is positive, then the image element vegetation affected by human activities is improved. This condition indicates that the image element affects vegetation and disturbance variation. Thus, the quantitative relationship among vegetation cover, climate factors, and human activities should be further investigated in future works.
Uncertainties remain in this study. Since this model ignores the complex interaction between climate change and human activities and reduces the nonlinear relationship between vegetation dynamics and influencing factors to a linear relationship (Jiang et al., 2019), the accuracy of the quantification in this study may be affected. Additionally, the spatial resolution of the MODIS NDVI dataset used in this study was 1 km, leading to the possibility that scattered vegetation in unaltered natural vegetation was only affected by climate change in the mountainous areas of southwest China, which is another source of uncertainty. Lastly, the climate data utilized in this paper were obtained through spatial interpolation based on sparse site data, which could be another potential source of uncertainty.
Conclusion
Vegetation is highly responsive to external conditions. We investigated the influence of climate change (temperature, precipitation, sunshine duration and relative humidity) and human factors (GDP, population density, and land use/cover change) on gradual greening trends in southwest China, which exists the largest karst area all over the country. NDVI time series data from 2001 to 2019 were obtained from the MODIS NDVI dataset at a spatial resolution of 1 km and temporal resolution of 1 month. The coefficient of variation, Theil-Sen median trend, M-K test and relative role analysis were used to assess the relative roles of climate change and human activities in the process of vegetation cover change of the southwest karst provinces in China from 2001 to 2019. The main conclusions are as follows:
1) NDVI of vegetation in the study area progressively improved from 2001 to 2019, with a linear trend of 0.003/a. Prior to 2008, NDVI rose significantly in the southwest karst provinces, which indicates the vegetation continued to improve and recover. From 2008 to 2019, NDVI increased with the fluctuations which might relate to the occurrence of extreme weather.
2) Under the joint action of natural and anthropogenic-driven factors, vegetation coverage in the southwest karst provinces showed an overall upward trend from 2001 to 2019, and the annual difference of vegetation NDVI was small. Vegetation variations in the study area had an obvious spatial heterogeneity. Climate change and human activities are two driving forces in vegetation NDVI. Though the interaction of the two, about 90.31% of the study area experienced an increase in vegetation coverage and about 9.69% a decrease, with the significantly decreased values mainly concentrated in the major cities.
3) Human activities over the past 19 years were the leading factors in the process of vegetation cover improvement and degradation in the southwest karst provinces of China. For areas with increasing vegetation, as identified by remote sensing observations, the relative roles of climate variations and human activities in vegetation dynamics were calculated as 38 and 62%, respectively, indicating that human activities played an important role in vegetation increase. For areas of decreasing vegetation, as identified by remote sensing observations, the relative roles of climate variations and human activities in vegetation change were calculated as 41 and 59%, respectively, indicating that climate variations had large negative effects on vegetation condition.
4) During 2001–2019, vegetation NDVI in the southwest karst provinces was positively correlated with precipitation, air temperature and relative humidity, with partial correlation coefficients of 0.116, 0.120 and 0.107, respectively. In contrast, NDVI was negatively correlated with sunshine duration, with a partial correlation coefficient of −0.15. Thus, vegetation growth in the southwest karst provinces was both positively promoted and negatively inhibited by climate change.
5) Under the influence of human activities, the vegetation coverage increased significantly for about 11.20% of the total study area, scattered around cities. The NDVI of vegetation in the three provinces was negatively correlated with PD and NTL. LUCC results demonstrate that study area’s land use pattern has experienced significant variations, which were primarily characterized by the transformation of cropland to forest land, forest land to cropland and grassland, and grassland to forest land. Afforestation further promotes the conversion of cropland or grassland to forest land, and rapid big city expansion resulted in agricultural land loss from cropland, forest land and grassland in the three provinces of southwest China.
Data Availability Statement
The original contributions presented in the study are included in the article/Supplementary Material, further inquiries can be directed to the corresponding author.
Author Contributions
HY and YX conceived the original ideas, and designed and performed the experiments. Writing—original draft preparation, HY. Formal analysis and software from JH and SZ. Visualization, LX. All authors have read and agreed to the published version of the manuscript.
Funding
This research were funded by Key Laboratory of Ecology of Rare and Endangered Species and Environmental Protection (Guangxi Normal University), Ministry of Education, China (No.ERESEP2020Z05), and the Guangxi Natural Science Foundation Program (No. 2020GXNSFBA297160).
Conflict of Interest
The authors declare that the research was conducted in the absence of any commercial or financial relationships that could be construed as a potential conflict of interest.
Publisher’s Note
All claims expressed in this article are solely those of the authors and do not necessarily represent those of their affiliated organizations, or those of the publisher, the editors and the reviewers. Any product that may be evaluated in this article, or claim that may be made by its manufacturer, is not guaranteed or endorsed by the publisher.
Supplementary Material
The Supplementary Material for this article can be found online at: https://www.frontiersin.org/articles/10.3389/feart.2022.799493/full#supplementary-material
References
Baniya, B., Tang, Q., Huang, Z., Sun, S., and Techato, K. A. (2018). Spatial and Temporal Variation of NDVI in Response to Climate Change and the Implication for Carbon Dynamics in Nepal. Forests 9, 329. doi:10.3390/f9060329
Bao, G., Qin, Z., Bao, Y., Zhou, Y., Li, W., and Sanjjav, A. (2014). NDVI-based Long-Term Vegetation Dynamics and its Response to Climatic Change in the Mongolian Plateau. Remote Sensing 6, 8337–8358. doi:10.3390/rs6098337
Brandt, M., Rasmussen, K., Peñuelas, J., Tian, F., Schurgers, G., Verger, A., et al. (2017). Human Population Growth Offsets Climate-Driven Increase in Woody Vegetation in Sub-saharan Africa. Nat. Ecol. Evol. 1, 81. doi:10.1038/s41559-017-0081
Breil, M., Panitz, H. J., and Schädler, G. (2017). Impact of Soil-Vegetation-Atmosphere Interactions on the Spatial Rainfall Distribution in the Central Sahel. Metz 26, 379–389. doi:10.1127/metz/2017/0819
Burrell, A. L., Evans, J. P., and Liu, Y. (2018). The Impact of Dataset Selection on Land Degradation Assessment. ISPRS J. Photogrammetry Remote Sensing 146, 22–37. doi:10.1016/j.isprsjprs.2018.08.017
Cai, H., Yang, X., Wang, K., and Xiao, L. (2014). Is forest Restoration in the Southwest China Karst Promoted Mainly by Climate Change or Human-Induced Factors? Remote Sensing 6, 9895–9910. doi:10.3390/rs6109895
Chen, H., Liu, X., Ding, C., and Huang, F. (2018). Phenology-based Residual Trend Analysis of MODIS-NDVI Time Series for Assessing Human-Induced Land Degradation. Sensors 18, 3676. doi:10.3390/s18113676
Chu, H., Venevsky, S., Wu, C., and Wang, M. (2019). NDVI-based Vegetation Dynamics and its Response to Climate Changes at Amur-Heilongjiang River Basin from 1982 to 2015. Sci. Total Environ. 650, 2051–2062. doi:10.1016/j.scitotenv.2018.09.115
Deng, Y., Wang, S., Bai, X., Tian, Y., Wu, L., Xiao, J., et al. (2018). Relationship Among Land Surface Temperature and LUCC, NDVI in Typical Karst Area. Sci. Rep. 8, 641. doi:10.1038/s41598-017-19088-x
Eckert, S., Hüsler, F., Liniger, H., and Hodel, E. (2015). Trend Analysis of MODIS NDVI Time Series for Detecting Land Degradation and Regeneration in Mongolia. J. Arid Environments 113, 16–28. doi:10.1016/j.jaridenv.2014.09.001
Erb, K. H., Kastner, T., Plutzar, C., Bais, A. L. S., Carvalhais, N., Fetzel, T., et al. (2018). Unexpectedly Large Impact of forest Management and Grazing on Global Vegetation Biomass. Nature 553, 73–76. doi:10.1038/nature25138
Evans, J., and Geerken, R. (2004). Discrimination between Climate and Human-Induced Dryland Degradation. J. Arid Environments 57, 535–554. doi:10.1016/S0140-1963(03)00121-6
Geldmann, J., Joppa, L. N., and Burgess, N. D. (2014). Mapping Change in Human Pressure Globally on Land and within Protected Areas. Conservation Biol. 28, 1604–1616. doi:10.1111/cobi.12332
Gemitzi, A., Banti, M. Α., and Lakshmi, V. (2019). Vegetation Greening Trends in Different Land Use Types: Natural Variability versus Human-Induced Impacts in Greece. Environ. Earth Sci. 78, 172. doi:10.1007/s12665-019-8180-9
Guo, L., Liu, R., Men, C., Wang, Q., Miao, Y., Shoaib, M., et al. (2021). Multiscale Spatiotemporal Characteristics of Landscape Patterns, Hotspots, and Influencing Factors for Soil Erosion. Sci. Total Environ. 779, 146474. doi:10.1016/j.scitotenv.2021.146474
He, G., Zhen, X., Li, Z., Shen, W., Han, J., Zhang, L., et al. (2020). Influence of Variations of Hydrothermal Conditions on Normalized Difference Vegetation index in Typical Temperature Zones along the East Coast of China. Front. Earth Sci. 8, 574101. doi:10.3389/feart.2020.574101
Hou, W., Gao, J., Wu, S., and Dai, E. (2015). Interannual Variations in Growing-Season NDVI and its Correlation with Climate Variables in the Southwestern Karst Region of China. Remote Sensing 7, 11105–11124. doi:10.3390/rs70911105
Huang, H., Zhou, Y., Qian, M., and Zeng, Z. (2021). Land Use Transition and Driving Forces in Chinese Loess Plateau: a Case Study from Pu County, Shanxi Province. Land 10, 67. doi:10.3390/land10010067
Hutchinson, M. F. (1998). Interpolation of Rainfall Data with Thin Plate Smoothing Splines - Part I: Two Dimensional Smoothing of Data with Short Range Correlation. J. Geogr. Inf. Decis. Anal. 2, 139–151. Available at: https://www.researchgate.net/publication/2570048.
Jiang, H., Xu, X., Guan, M., Wang, L., Huang, Y., and Jiang, Y. (2020). Determining the Contributions of Climate Change and Human Activities to Vegetation Dynamics in Agro-Pastural Transitional Zone of Northern China from 2000 to 2015. Sci. Total Environ. 718, 134871. doi:10.1016/j.scitotenv.2019.134871
Jiang, L., Guli·Jiapaer, G. L., Bao, A., Guo, H., and Ndayisaba, F. (2017). Vegetation Dynamics and Responses to Climate Change and Human Activities in Central Asia. Sci. Total Environ. 599-600, 967–980. doi:10.1016/j.scitotenv.2017.05.012
Jiang, Z., Lian, Y., and Qin, X. (2014). Rocky Desertification in Southwest China: Impacts, Causes, and Restoration. Earth-Science Rev. 132, 1–12. doi:10.1016/j.earscirev.2014.01.005
Jong, R., Verbesselt, J., Schaepman, M. E., and Bruin, S. (2012). Trend Changes in Global Greening and browning: Contribution of Short-Term Trends to Longer-Term Change. Glob. Change Biol. 18, 642–655. doi:10.1111/j.1365-2486.2011.02578.x
Levin, N. (2016). Human Factors Explain the Majority of MODIS-Derived Trends in Vegetation Cover in Israel: A Densely Populated Country in the Eastern Mediterranean. Reg. Environ. Change 16, 1197–1211. doi:10.1007/s10113-015-0848-4
Li, A., Wu, J., and Huang, J. (2012). Distinguishing between Human-Induced and Climate-Driven Vegetation Changes: a Critical Application of RESTREND in Inner Mongolia. Landscape Ecol. 27, 969–982. doi:10.1007/s10980-012-9751-2
Li, J., Peng, S., and Li, Z. (2017). Detecting and Attributing Vegetation Changes on China's Loess Plateau. Agric. For. Meteorology 247, 260–270. doi:10.1016/j.agrformet.2017.08.005
Li, S., Yang, S., Liu, X., Liu, Y., and Shi, M. (2015). NDVI-based Analysis on the Influence of Climate Change and Human Activities on Vegetation Restoration in the Shaanxi-Gansu-Ningxia Region, central China. Remote Sensing 7, 11163–11182. doi:10.3390/rs70911163
Li, Y., Xie, Z., Qin, Y., and Zheng, Z. (2019). Estimating Relations of Vegetation, Climate Change, and Human Activity: A Case Study in the 400 Mm Annual Precipitation Fluctuation Zone, China. Remote Sensing 11, 1159. doi:10.3390/rs11101159
Liu, F., Qin, T., Girma, A., Wang, H., Weng, B., Yu, Z., et al. (2018). Dynamics of Land-Use and Vegetation Change Using Ndvi and Transfer Matrix: a Case Study of the Huaihe River basin. Pol. J. Environ. Stud. 28, 213–223. doi:10.15244/pjoes/82900
Liu, Y. Y., Evans, J. P., McCabe, M. F., De Jeu, R. A. M., Van Dijk, A. I. J. M., Dolman, A. J., et al. (2013). Changing Climate and Overgrazing Are Decimating Mongolian Steppes. Plos One 8, e57599. doi:10.1371/journal.pone.0057599
Meng, M., Huang, N., Wu, M., Pei, J., Wang, J., and Niu, Z. (2019). Vegetation Change in Response to Climate Factors and Human Activities on the Mongolian Plateau. PeerJ 7, e7735. doi:10.7717/peerj.7735
Nanzad, L., Zhang, J., Tuvdendorj, B., Nabil, M., Zhang, S., and Bai, Y. (2019). NDVI Anomaly for Drought Monitoring and its Correlation with Climate Factors over Mongolia from 2000 to 2016. J. Arid Environments 164, 69–77. doi:10.1016/j.jaridenv.2019.01.019
Nesha, M. K., Herold, M., De Sy, V., Duchelle, A. E., Martius, C., Branthomme, A., et al. (2021). An Assessment of Data Sources, Data Quality and Changes in National forest Monitoring Capacities in the Global Forest Resources Assessment 2005-2020. Environ. Res. Lett. 16, 054029. doi:10.1088/1748-9326/abd81b
Ostwald, M., and Chen, D. (2006). Land-use Change: Impacts of Climate Variations and Policies Among Small-Scale Farmers in the Loess Plateau, China. Land Use Policy 23, 361–371. doi:10.1016/j.landusepol.2005.04.004
Peng, J., Jiang, H., Liu, Q., Green, S. M., Quine, T. A., Liu, H., et al. (2021). Human Activity vs. Climate Change: Distinguishing Dominant Drivers on Lai Dynamics in Karst Region of Southwest China. Sci. Total Environ. 769, 144297. doi:10.1016/j.scitotenv.2020.144297
Peng, J., Li, Y., Tian, L., Liu, Y., and Wang, Y. (2015). Vegetation Dynamics and Associated Driving Forces in Eastern China during 1999-2008. Remote Sensing 7, 13641–13663. doi:10.3390/rs71013641
Piao, S., Mohammat, A., Fang, J., Cai, Q., and Feng, J. (2006). NDVI-based Increase in Growth of Temperate Grasslands and its Responses to Climate Changes in China. Glob. Environ. Change 16, 340–348. doi:10.1016/j.gloenvcha.2006.02.002
Qiao, Y., Jiang, Y., and Zhang, C. (2021). Contribution of Karst Ecological Restoration Engineering to Vegetation Greening in Southwest China during Recent Decade. Ecol. Indicators 121, 107081. doi:10.1016/j.ecolind.2020.107081
Qiu, C., Hu, J., Yang, F., Liu, F., and Li, X. (2018). Human Pressures on Natural Reserves in Yunnan Province and Management Implications. Sci. Rep. 8, 3260. doi:10.1038/s41598-018-21654-w
Qu, L., Huang, Y., Yang, L., and Li, Y. (2020). Vegetation Restoration in Response to Climatic and Anthropogenic Changes in the Loess Plateau, China. Chin. Geogr. Sci. 30, 89–100. doi:10.1007/s11769-020-1093-4
Salmerón Gómez, R., García Pérez, J., López MartínDel Mar, M. D. M., and García, C. G. (2016). Collinearity Diagnostic Applied in ridge Estimation through the Variance Inflation Factor. J. Appl. Stat. 43, 1831–1849. doi:10.1080/02664763.2015.1120712
Samie, A., Deng, X., Jia, S., and Chen, D. (2017). Scenario-based Simulation on Dynamics of Land-Use-Land-Cover Change in punjab Province, Pakistan. Sustainability 9, 1285. doi:10.3390/su9081285
ShengPei, D., Bo, Z., HaiJun, W., YaMin, W., LingXia, G., XingMei, W., et al. (2011). Vegetation Cover Change and the Driving Factors over Northwest China. J. Arid Land 3, 25–33. doi:10.3724/SP.J.1227.2011.00025
Sun, Y., Yang, Y., Zhang, L., and Wang, Z. (2015). The Relative Roles of Climate Variations and Human Activities in Vegetation Change in North China. Phys. Chem. Earth, Parts A/B/C 87-88, 67–78. doi:10.1016/j.pce.2015.09.017
Wang, H., Yao, F., Zhu, H., and Zhao, Y. (2020). Spatiotemporal Variation of Vegetation Coverage and its Response to Climate Factors and Human Activities in Arid and Semi-arid Areas: Case Study of the Otindag sandy Land in China. Sustainability 12, 5214. doi:10.3390/su12125214
Wang, J., Wang, K., Zhang, M., and Zhang, C. (2015). Impacts of Climate Change and Human Activities on Vegetation Cover in Hilly Southern China. Ecol. Eng. 81, 451–461. doi:10.1016/j.ecoleng.2015.04.022
Wang, M., Fu, J. E., Wu, Z., and Pang, Z. (2020). Spatiotemporal Variation of NDVI in the Vegetation Growing Season in the Source Region of the Yellow River, China. Ijgi 9, 282. doi:10.3390/ijgi9040282
Wang, Y., Xia, T., Shataer, R., Zhang, S., and Li, Z. (2021). Analysis of Characteristics and Driving Factors of Land-Use Changes in the Tarim River basin from 1990 to 2018. Sustainability 13, 10263. doi:10.3390/su131810263
Xiu, L., Yan, C., Li, X., Qian, D., and Feng, K. (2019). Changes in Wetlands and Surrounding Land Cover in a Desert Area under the Influences of Human and Climatic Factors: A Case Study of the Hongjian Nur Region. Ecol. Indicators 101, 261–273. doi:10.1016/j.ecolind.2019.01.025
Xu, D., Song, A., Li, D., Ding, X., and Wang, Z. (2019). Assessing the Relative Role of Climate Change and Human Activities in Desertification of North China from 1981 to 2010. Front. Earth Sci. 13, 43–54. doi:10.1007/s11707-018-0706-z
Xu, G., Zhang, H., Chen, B., Zhang, H., Innes, J., Wang, G., et al. (2014). Changes in Vegetation Growth Dynamics and Relations with Climate over China's Landmass from 1982 to 2011. Remote Sensing 6, 3263–3283. doi:10.3390/rs6043263
Xu, H. J., Wang, X. P., and Yang, T. B. (2017). Trend Shifts in Satellite-Derived Vegetation Growth in Central Eurasia, 1982-2013. Sci. Total Environ. 579, 1658–1674. doi:10.1016/j.scitotenv.2016.11.182
Yu, F. K., Huang, X. H., Yuan, H., Ye, J. X., Yao, P., Qi, D. H., et al. (2014). Spatial-temporal Vegetation Succession in Yao'an County, Yunnan Province, Southwest China during 1976-2014: A Case Survey Based on RS Technology for Mountains Eco-Engineering. Ecol. Eng. 73, 9–16. doi:10.1016/j.ecoleng.2014.09.026
Zhao, L., Dai, A., and Dong, B. (2018). Changes in Global Vegetation Activity and its Driving Factors during 1982-2013. Agric. For. Meteorology 249, 198–209. doi:10.3390/rs604326310.1016/j.agrformet.2017.11.013
Zhao, S., Pereira, P., Wu, X., Zhou, J., Cao, J., and Zhang, W. (2020). Global Karst Vegetation Regime and its Response to Climate Change and Human Activities. Ecol. Indicators 113, 106208. doi:10.1016/j.ecolind.2020.106208
Zheng, K., Wei, J. Z., Pei, J. Y., Cheng, H., Zhang, X. L., Huang, F. Q., et al. (2019). Impacts of Climate Change and Human Activities on Grassland Vegetation Variation in the Chinese Loess Plateau. Sci. Total Environ. 660, 236–244. doi:10.1016/j.scitotenv.2019.01.022
Keywords: vegetation dynamics, NDVI, climate variations, human activities, remote sensing, southwest karst
Citation: Yang H, Hu J, Zhang S, Xiong L and Xu Y (2022) Climate Variations vs. Human Activities: Distinguishing the Relative Roles on Vegetation Dynamics in the Three Karst Provinces of Southwest China. Front. Earth Sci. 10:799493. doi: 10.3389/feart.2022.799493
Received: 21 October 2021; Accepted: 03 March 2022;
Published: 12 April 2022.
Edited by:
Marco Chini, Luxembourg Institute of Science and Technology (LIST), LuxembourgReviewed by:
Yi Liu, University of New South Wales, AustraliaTianyi Zhang, Institute of Atmospheric Physics (CAS), China
Copyright © 2022 Yang, Hu, Zhang, Xiong and Xu. This is an open-access article distributed under the terms of the Creative Commons Attribution License (CC BY). The use, distribution or reproduction in other forums is permitted, provided the original author(s) and the copyright owner(s) are credited and that the original publication in this journal is cited, in accordance with accepted academic practice. No use, distribution or reproduction is permitted which does not comply with these terms.
*Correspondence: Yong Xu, eW9uZ3h1QGdsdXQuZWR1LmNu