- 1Group Terrestrial Ecohydrology, Institute of Geoscience, Friedrich Schiller University Jena, Jena, Germany
- 2Centre for Environmental Biotechnology, Helmholtz Centre for Environmental Research – UFZ, Leipzig, Germany
- 3Chair of Soil Science, Institute of Geography, Friedrich Schiller University Jena, Jena, Germany
- 4German Center for Integrative Biodiversity Research (iDiv) Halle-Jena-Leipzig, Leipzig, Germany
- 5Department of Computational Hydrosystems, Helmholtz Centre for Environmental Research – UFZ, Leipzig, Germany
While net precipitation entering the soil is commonly measured in woody ecosystems, there is a lack of field measurements for herbaceous vegetation. Small canopy heights and fragile stem structures are the primary challenges for net precipitation sampling in grasslands under field conditions. We designed a new in situ device, “interception tubes”, for throughfall sampling in temperate grasslands. The instrument allows a natural development of grass canopy and sampling at multiple locations. Although it does not strictly separate throughfall and stemflow, the dominant part of the collected water is throughfall. We tested the interception tubes for splash loss with a drip experiment. Next, we evaluated the tubes’ measurements in a field installation at 25 locations both with and without vegetation cover. Also, we used measurements of gross precipitation, canopy height and soil water content to check the plausibility of the measurements. The experiment showed splash loss for the tubes is small ( < 3%) for the typical rain drop size for the growing season in the region, as well as for throughfall drops of lower falling velocity. In the uncovered period, splash loss corrected tubes’ measurements were generally smaller than classical funnel measurements. But the statistical model revealed that the slope of their relationship is close to unity (0.92) when accounting for topography and was probably related to wind effects. During the covered period, grass height systematically reduced below canopy precipitation measured by the tubes, indicating that they can capture spatial canopy drip patterns under denser grass foliage. The canopy height also altered the wind effect on the tube measurements. As in forest ecosystems, below canopy precipitation patterns were temporally stable and smaller events increased the spatial heterogeneity. The measured below canopy precipitation was between 95% and 22% that above, and grass height amplified the loss. The soil water balance showed the tubes underestimated soil water input at peak grass height, which suggests enhanced occurrence of stemflow in tall grass. Despite the underestimation of stemflow, the interception tubes are a suitable method for estimating the canopy effect on throughfall patterns in temperate grasslands, and stemflow can be quantified by additional soil moisture measurements.
1 Introduction
Precipitation is intercepted and redistributed by vegetation canopy before reaching the ground. While net precipitation enters the soil in the form of throughfall and stemflow, the intercepted fraction is evaporated back into the atmosphere referred to as interception loss. Throughfall may reach soil freely through gaps in canopy or release by dripping from leaves and branches (Dunkerley, 2000; Levia and Frost, 2006; Levia et al., 2017) whereas stemflow flows along the stem of the plant (Crockford and Richardson, 2000). Vegetation features such as type, leaf orientation and shape, plant structure, stem surface regulate net precipitation components and interception loss (Crockford and Richardson, 2000; Levia and Frost, 2003, 2006). Next to biotic factors, also rainfall characteristics namely rainfall intensity, event size (Staelens et al., 2008; Dunkerley, 2014; Zhang et al., 2016; Magliano et al., 2019), intra-event rainfall intermittency (Dunkerley, 2015), drop size (Calder et al., 1996; Nanko et al., 2006; Levia et al., 2019) and meteorological factors like wind speed (Staelens et al., 2008; Van Stan et al., 2011; Zhang et al., 2020) control net precipitation. Consequently, canopy interception does not only decrease water entering soil but also introduces spatio-temporal heterogeneity.
Interception loss can be estimated by measuring the net precipitation components, stemflow and throughfall, along with gross precipitation. While short canopies were paid much less attention to (Dunkerley, 2000; Llorens and Domingo, 2007; Sadeghi et al., 2020), forested ecosystems were extensively investigated to measure below canopy precipitation (Keim et al., 2005; McJannet et al., 2007; Zimmermann et al., 2009; Klos et al., 2014; Metzger et al., 2017; Molina et al., 2019). In forest ecosystems, elevated funnel gauges or troughs are commonly used to measure throughfall (Levia and Frost, 2006). Stemflow is typically measured with collectors or collars, which are wrapped in a spiral or ring around a tree stem (Levia and Germer, 2015). Flexible collars or spirals were also used to collect stemflow for shrubs and understory (Návar and Bryan, 1990; Jian et al., 2014; Yang et al., 2019; Gordon et al., 2020). However, these methods are not applicable to short plant communities in grasslands in temperate climates. The small leaves, branches and the non-woody, softer stem structure are the biggest challenges for measuring net precipitation under natural conditions in short herbaceous vegetation with the methods frequently used in forests.
Grasslands provide important ecosystem services such as carbon storage, erosion control and water supply by modifying infiltration rate and surface runoff (Bengtsson et al., 2019; Fischer et al., 2019). Moreover, grasslands (except croplands) cover a quarter of the global terrestrial surface, which is approximately equal to woody ecosystem areas (Lemaire et al., 2011). Because of the smaller leaf area and lower aerodynamic conductance, in temperate climates grasslands are often expected to have less interception loss than forests (David et al., 2005; Muzylo et al., 2009; Madani et al., 2017; Douinot et al., 2019), although some studies showed the opposite (Breuer et al., 2003; Williams et al., 2012). Understanding the role of grasslands in rainfall partitioning is important to solve this puzzle. Yet our current knowledge on how the grassland canopy spatially alters precipitation and impacts hydrological processes in soil is limited due to scanty experimental evidence (Breuer et al., 2003; Sadeghi et al., 2020).
Some of the rare net precipitation studies in herbaceous vegetation were based on just laboratory simulated rainfall experiments or indirect variable estimation such as effective rainfall (Burgy and Pomeroy, 1958; Ataroff and Naranjo, 2009; Ochoa-Sánchez et al., 2018). Other researchers used troughs, boxes and gypsum tablet integrated funnels to run field experiments under simulated or natural rainfall in grasses and dense closed heath shrubs (Wollny, 1890; Beard, 1962; Couturier and Ripley, 1973; Seastedt, 1985; Gilliam et al., 1987; Dunkerley, 2010). Clark (1940) firstly combined laboratory experiments with simulated rainfall and limited natural rainfall measurements in the field to investigate interception loss of several types of grass. He placed five troughs for the field measurements. He recorded a substantial amount of interception loss for almost all examined grass species. However, later Seastedt (1985) showed that through measurements depended on the stem density which was altered by placing troughs. Also, Couturier and Ripley (1973) found that the trough measurements estimated 15% less net precipitation than laboratory experiments. An alternative method used for field investigations is sealing a defined surface area while providing holes for the grass boles to allow for the growth of the grass (Crouse et al., 1966; Butler and Huband, 1985). In a dry climate, up to 40% interception loss was estimated using the sealed surface approach, while no data is available so far using this method in temperate climate. All those previous measurements agree on the sizeable throughfall amount in grasslands, although the contribution of stemflow is still under discussion: while some studies suggest stemflow can be a substantial portion of net precipitation in grasses (Beard, 1962; de Ploey, 1982; Seastedt, 1985; van Dam et al., 1991), others concluded that it is negligible (Clark, 1940; Butler and Huband, 1985).
The former measurements to estimate net precipitation in herbaceous plants under natural rainfall were conducted using comparatively few throughs (n < 10) (Clark, 1940; Beard, 1962; Butler and Huband, 1985; Seastedt, 1985; Gilliam et al., 1987; Heil et al., 1988) that does not allow estimation of the spatial variation. Although troughs can be used to sample at more locations in grassland, due to the disturbance of the natural orientation and stem density of the canopy, a trough sampling might lead to errors in estimation of net precipitation. Furthermore, sealed surface and gypsum tablet integrated funnel methods can be employed at multiple locations. However, owing to the problems related to the sealing material, sealed surface is not always suitable for net precipitation measuring (Zou et al., 2015). Also, net precipitation chemical composition and precipitation intensity could possibly induce error for gypsum tablet integrated funnel measurements (Dunkerley, 2010; Filipzik et al., 2019). However, as shown in forest studies, not only the amount of mean net precipitation but also its spatial variation is important to understand hydrological processes such as drainage, subsurface storm flow and deep percolation (Guswa and Spence, 2012; Coenders-Gerrits et al., 2013; Klos et al., 2014; Metzger et al., 2017). An accurate estimation of the spatially heterogenous below canopy drip requires many samples at multiple locations (Kimmins, 1973; Kostelnik et al., 1989; Zimmermann et al., 2010; Voss et al., 2016). Therefore, the previous measurement methods in the literature lack the possibility of estimates of spatial representative assessment of net precipitation and its patterns in grasslands in temperate climate under natural rainfall.
In this study, we propose an in-situ net precipitation measurement device for dense temperate grasslands to capture canopy induced heterogeneity in water input, which we refer to as the ‘interception tubes, or shortly “the tubes” in the following. We tested the performance of interception tubes in the lab and field and present the first data on vegetation impact on net precipitation dynamics to assess the plausibility of the measurements.
2 Materials and Methods
2.1 Interception Tubes
The interception tubes (Figure 1) consist of four thin partly opened plexiglass pipes (20 mm in diameter and 360 mm in length, the opening is 12 mm wide and 262 mm long) which are connected to a below ground plastic sampling bottle (2L). The pipes collect rain and throughfall depending on the foliage density and canopy height, and water flow is driven by gravity into the sampling bottle. We trimmed and rounded the edges of the opened part of the pipes to minimize splash. According to the definition of extent, spacing and support (Blöschl and Sivapalan, 1995; Western et al., 2002), the extent of the tubes’ is around 1 m. The support of each tubes is around 0.26 m in four axial direction which yields a receiving surface of roughly 0.01 m2. Each interception tube is inclined roughly 26° and held in place by metal support sticks to provide unhindered gravity-driven precipitation flow into the sampling bottle. The average height of dug metal supports from the ground level was 5 cm at sampling bottle joints and 14 cm at the end of the pipes, which varied depending on the ground conditions in the field. We prevented weathering and intruding animals by storing the bottle in an embedded case in the field.
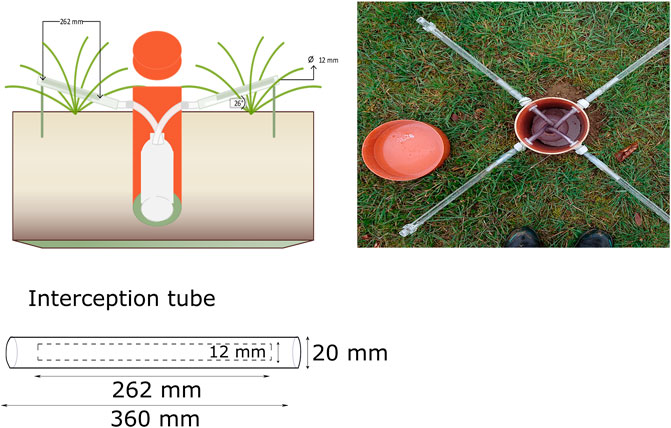
FIGURE 1. Design of the interception tubes with explicit pipe dimensions. (left) cross-section view of the interception tubes and (right) top view.
Because of their small size, the tubes allow the grass canopy to grow naturally when it is installed early in the growing season. As early field installation is crucial to create close contact between interception tubes and developed canopy, which is a key for net precipitation sampling, the method is limited to be applied in relatively short plant communities in temperate grasslands or mowing may be needed.
This method does not strictly separate stemflow and throughfall. The tubes are in close contact with the dense foliage potentially allowing the collection of both stemflow and throughfall, but the round cross section of the tubes probably interrupts stemflow paths and diverts them outwards. Therefore, the bulk portion of the collected water is expected to be throughfall.
2.2 Drip Experiment
The narrow structure of the tubes might cause a substantial amount of splash loss, particularly for big and fast rain drops because of high kinetic energy. Short canopy height, on the other hand, reduces kinetic energy of rain drops despite bigger throughfall drops (Zhou et al., 2002; Nanko et al., 2008, 2015; Frasson and Krajewski, 2011; Goebes et al., 2016). As the interception tubes is an apparatus for short temperate grassland canopy we initially focused on splash loss caused by large open rainfall drops. Therefore, we conducted a laboratory experiment to estimate splash loss for the expected big rain drops in the spring and summer season storms. For this we first estimated the drop size range of precipitation events in the target research site (Hainich Critical Zone Exploratory, Germany), by using the empirical relation between rainfall intensity and the median volume drop diameter (Eq. 1) (Brandt, 1989).
where
We used a 15-year rainfall data set (2004–2019) from a nearby German Weather Service precipitation gauge (DWD climate data center, www.dwd.de/cdc, ID 336, located 12 km to the Southeast) to calculate the drop sizes. In addition, we used a nearby project internal weather station -Reckenbuel- (1.4 km to the Northeast) to estimate the drop size range at the site during the field measurements (see below) to design our lab experiment.
Splash loss is mainly governed by the kinetic energy of rain drops, which reach the ground at a constant velocity, the terminal velocity. In the lab experiment, we aimed to reproduce the kinetic energy of a range of drop sizes, in order to estimate the splash loss. Only rain drops smaller than 0.7 mm reach terminal velocity within 2 m of falling distance (van Boxel, 1998), whereas drop sizes for larger storms can reach up to 2.16 mm in the research site (Figure 2). Since the intended investigation of the splash loss of larger drops would have exceeded the dimensions of the laboratory, we targeted at only 75% of the terminal velocity (taken from van Boxel, 1998) while generating larger drops. This way, we simulated the same kinetic energy as the one of the target rain drop size at 100% terminal velocity. Drops were generated by pumping water through a needle tip. To generate different sized drops, we used six different blunt needle tips; the sizes varied from 0.6 to 1.83 mm connected to a peristaltic pump (Watson-Marlow 101U) via a rubber tube. The needle tips produce bigger drops than their tip size regardless of the end shape (Tripp et al., 2016). Therefore, in preliminary experiment we determined calibration curve and estimated the mean size of the generated drops at certain pumping rate by continuously measuring the mass of droplets. We adjusted the pumping rate for each needle size and tube length to generate target sized drops based on the preliminary experiment. Also, we arranged the falling height for each generated drop by using the results of the model by van Boxel (1998) to reach the target kinetic energy. In order to minimize environmental impact on falling drops such as air currents, we placed the needle tip inside of a plastic shield. (Photos of the lab experiment setup and further details can be found in the Supplementary Material).
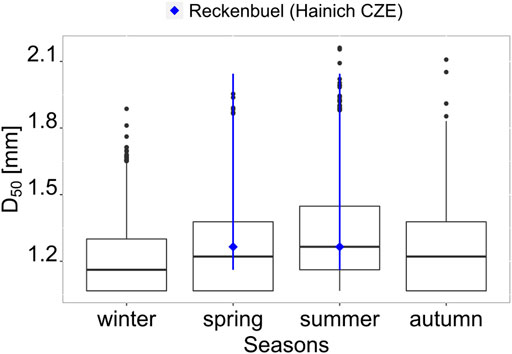
FIGURE 2. Median rain drop size (D50 in mm) derived from hourly rain intensity measured over 15 years (2004–2019) from a nearby German Weather Service precipitation gauge (DWD climate data centre, www.dwd.de/cdc, ID 336, located 12 km to the Southeast). Blue lines mark the drop size range derived from data measured by the nearby Reckenbuel station (1.4 km to the Northeast) from 13 March 2019 to 28 August 2019.
For each needle tip and the corresponding setup (adjusted height and pumping rate), first we checked the produced drop size, and started the experiment. We employed each setup for 10 minutes run and repeated three times. Due to the narrow structure of the interception tube, some falling drops missed the pipe despite the protection. Therefore, the same person observed the orifice visually and counted missed drops for 15 s, and repeated this 25 times with short breaks, concluding one round of measurements for each drop size. Three rounds of measurements were performed, of which we chose the run with the smallest standard deviation of missed drop counts. Further, we cross checked the mass balance for total generated, missed and collected drops, before estimating the drop size specific splash loss. Then, we calculated splash loss as the difference between the cross-checked pump supplied mass of drops - the received mass,
We used the kinetic energy estimated in the lab to infer the median drop sizes of real rain events at the field site. To simplify the mass contribution in the kinetic energy, we assumed sphere shaped droplets. Besides the kinetic energy formula, we used the relation (Eq. 4) for drop diameter and the terminal velocity to derive the represented rainfall drop size (Uplinger, 1981).
Throughfall drops can be much larger than open rainfall (Geißler et al., 2012; Nanko et al., 2015). According to reviewed and listed throughfall drop sizes of different plant species by (Levia et al., 2017), drop sizes can reach more than 7 mm in diameter. We assumed 8 mm as possible maximum throughfall drop diameter and 4 mm median throughfall drop size, based on the literature (Levia et al., 2017). We calculated the falling velocity of these drop sizes at ground level at peak canopy height (0.8, 0.9, 1 m) to estimate the kinetic energy based on a numerical model (van Boxel, 1998) which accounts turbulence around the drop. We then calculated the corresponding raindrop size, which has the same kinetic energy at terminal velocity in order to estimate the maximum splash loss in the uncovered period.
2.3 Field Setup and Sampling
We installed the interception tubes in a grassland, which is part of Hainich Critical Zone Exploratory (CZE) (Thuringia, Germany). The temperate grassland plot -0.045 ha-is cut two to three times per year. Grassland plant community at the directly adjacent plot to our field setup, is characterized by diverse functional groups such as graminoides (e.g., Dactylis glomerata), legumes (e.g., Trifolium repens), and herbs (e.g., Taraxacum spec.) (Potthast et al., 2017).
The research site (Figure 3) was equipped with a wireless soil moisture network (SoilNet; Bogena et al., 2010) since 2016. The network is composed of SMT100 frequency domain sensors (Truebner GmbH, Neustadt, Germany). The soil moisture sensors were installed at 30 points in a nested triangular design in two soil depths (7.5 cm, 27.5 cm). Based on soil profile examination in March 2015 (during the soil moisture network installation), the soil depth is 30 cm that varies between 17 cm and 51 cm. Moreover, the majority of fine roots was found in topsoil down to 15 cm to sometimes 20 cm depth, with some thicker roots extending deeper.
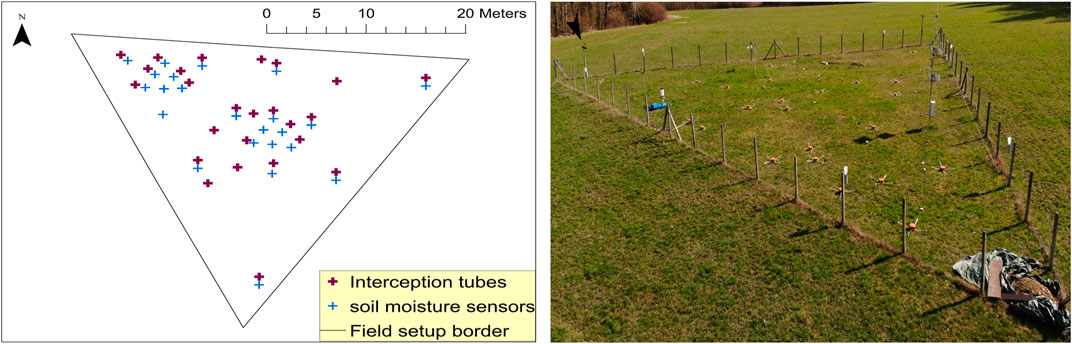
FIGURE 3. Overview of the field setup. (left) Map of the location of the interception tubes and soil moisture sensors. (right) A photograph from a drone in the uncovered period in March 2019.
The soil moisture sensors provided data with a 6-min time interval. We placed the interception tubes along a slight slope in early spring 2019 at 25 locations to ensure that the installation design of the interception tubes did not interfere the soil moisture measurements.
Weekly precipitation measurements were conducted with the tubes along with gross precipitation (above canopy ca.1.5 m) in 2019 (March–August) and in 2020 (January–February). Four gross precipitation collectors using conventional circular funnels (12 cm in diameter), with the orifice fabricated from two polyethylene bottles connected at necks with the bottom of the upper bottle removed to form a funnel and the lower bottle serving as a storage container (Zimmermann et al., 2010) were used. In order to ensure of representative rainfall sampling, we did not measure precipitation during rain events. Hence, precipitation measurement intervals were typically 7 days, but occasionally also six or 8 days.
We measured grass height (April–August 2019) at each interception tube and categorized the data set into two groups (uncovered and covered, Figure 4) according to foliage cover status and grass height. We set 15 cm grass height as a threshold value to define the covered period based on the height of the supported interception tubes and the field observations about the foliage status. During 5 weeks of sampling in 2019 and 7 weeks in 2020, the grass canopy was short and left the tubes uncovered, and we captured total rainfall at ground level (Pg,tube). We corrected the tube measurements according to the weekly cumulative splash loss (Pg,tube,cor) for the uncovered period samplings. For 14 weeks in 2019, we measured net precipitation via tubes and grass height. However, for four sampling weeks grass height data were not available because of measurement errors. We calculated weekly cumulative interception loss based on this period as the difference between total rainfall and net precipitation.
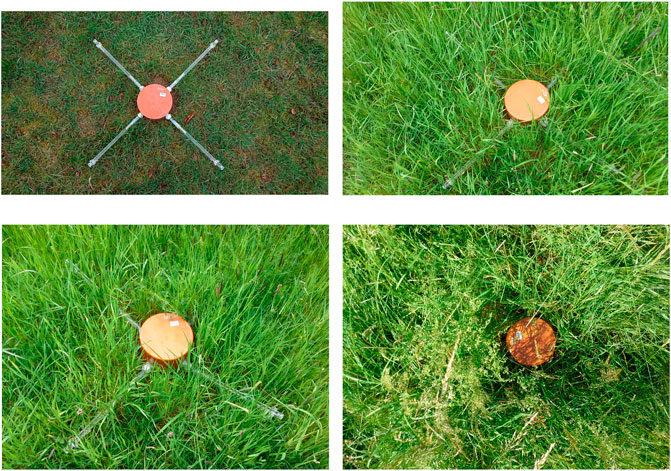
FIGURE 4. Example of one interception tube (upper left) uncovered period, and the same measurement location during the covered period (upper right) at 07.05.2019, (lower left) at 15.05.2019 and (lower right) at 13.06.2019.
We estimated weekly cumulative spatial mean of the increase in the soil water storage in both measurement depths (
where i is the sensor location, w the sampling week, n the event, h the soil depth represented by the sensor.
If more than one event occurred during the sampling week, they were summed to obtain a total weekly increase in soil water storage. All events with less than 8-h dry periods were merged into one event, in order to allow a minimum rain free period for canopy drip. Separation was based on the Reckenbuel weather station data (10 min cumulative precipitation). A list of rainfall event details for the covered period can be found in the Supplementary Material.
2.4 Data Analysis
2.4.1 Descriptive Statistics
We calculated weekly mean wind speed (
where n is the tube location, w is the sampling weeks.
Similarly, we calculated the spatial deviation of net precipitation from the mean (
where
2.4.2 Linear Mixed Effects Model
We used linear mixed effects models to check the performance of the tubes for precipitation measurement for the uncovered period, and to understand governing factors of net precipitation dynamics.
In the uncovered period, we checked whether the tubes reproduced the funnel measured gross precipitation. We also added variables which have a potential impact on the measurement performance of the interception tubes to the model. Next to spatial mean of weekly measured gross precipitation (
In the covered period, we investigated fixed factors potentially controlling measured net precipitation by interception tubes, while we selected random factors similarly. We tested the effects of spatial mean of grass height (
All statistical analyses were done in R environment (R Core Team, 2021). We used scaled variables with Z transformation by using ‘scale’ function which is provided in R base package (R Core Team, 2021) before running the linear mixed effects models. We did all linear mixed effects model analysis with ‘lme4’ package (Bates et al., 2015) and we calculated R2 in two types (conditional and marginal) of the model with ‘MuMIn’ package (Bartoń, 2020). Conditional R2 includes the variance determined by the entire model, whereas marginal R2 is explained by the fixed effects (Bartoń, 2020).
The model selection steps were the same for both uncovered and covered periods. Only fixed factors were evaluated, while the random factors were not tested in the model selection procedure. We initially included all potential variables (see supplements, Supplementary Table S3) and their interactions -beyond optimal model-to evaluate them step by step. In each selection step, we detected the least significant (the lowest p-value) fixed factor and formulated the new candidate model without it. We decided whether the model did not deteriorate without the detected fixed factor by evaluating and comparing Akaike’s Information Criterion (AIC) numbers. We used the maximum likelihood -ML- to compare the models with the same random effects (Zuur et al., 2009). If the AIC did not significantly decreased without the detected factor, that factor was removed, otherwise it was kept, and the next less significant variable was tested similarly to form the next candidate model. We repeated these steps until we obtained the model, which had the lowest AIC number, so it was the best model. Lastly, we refitted the best model with restricted maximum likelihood -REML- (Zuur et al., 2009).
3 Results
3.1 Splash Loss Experiment
Figure 2 shows that the median drop rain sizes were smaller than 1.5 mm despite seasonal differences. The larger drops (>1.2 mm) mainly occurred in spring and summer within the last 15 years of meteorological data (DWD climate data center, www.dwd.de/cdc, ID 336). The data from the nearby weather station (Reckenbuel) showed that 2019 spring-summer events resulted in a similar drop sizes range (1.16–2 mm) as 15-year data trend. Our drip experiment setup covered those large drops of the spring - summer events (Table 1).
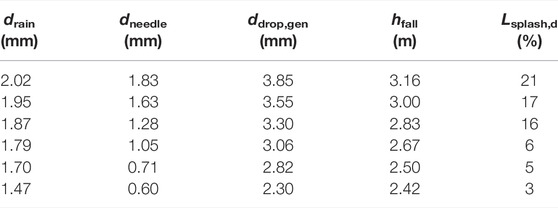
TABLE 1. Drip experiment results. Variables shown are: observed splash loss (Lsplash,d) for the represented rain drop size (drain), together with the diameter of the needles (dneedle) used for generating the drops of diameter (ddrop,gen) and the adjusted falling height (hfall) to obtain the kinetic energy representing the rain drop diameter.
In the splash experiment (Table 1) the largest tested rainfall drops (≥1.87 mm) caused more than 16% of splash loss. Yet, the loss decreased drastically to less than 3% for smaller drops (
The interaction of precipitation with the canopy alters drop sizes and velocity of falling drops. Since the canopy height rapidly changes with time, the size and the velocity of drops are unquantifiable during the covered period. However, since the canopy interaction slows down the falling drops and the grass height is comparatively small, their kinetic energy is substantially lower than drops of the same size in free rainfall. Table 2 shows the equivalent raindrop size corresponding to larger (4 mm and 8 mm in diameter) throughfall drops having the same kinetic energy when falling from the indicated height (corresponding to the canopy height). The kinetic energy of those large throughfall drops is equivalent to rain drop sizes between 0.91 mm and 1.06 mm, which is smaller than those covered in the lab experiment and producing splash loss of less than 3% in the interception tubes. Therefore, we did not apply a splash loss correction for the net precipitation measurements in the covered period.
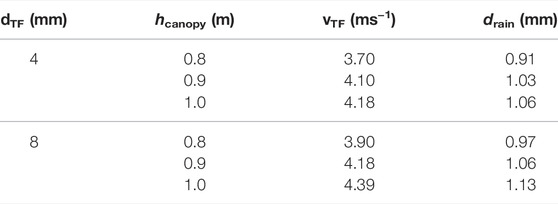
TABLE 2. Throughfall droplet size corresponding in terms of kinetic energy with given rain drop size at terminal velocity. Variables shown are: Throughfall drop size (dTF), the maximum canopy height (hcanopy) corresponding to the falling height of the throughfall droplet, the corresponding velocity of throughfall drop (vTF), and equivalent rainfall drop diameter (drain) with same kinetic energy at terminal velocity.
3.2 Uncovered Period
In the field experiment generally, the coefficient of variation of interception tubes measurements was smaller than that of the funnel measurements. Stronger wind speed increased the variation within the tubes’ measurements. The ground level precipitation measurements by interception tubes were lower than the funnel measurements on average, despite the splash loss correction (Table 3). For the mid-size cumulated events (5 mm–33 mm), the discrepancy between two measurement methods was marginal. Yet it reached up to 50% for the cumulated small events (
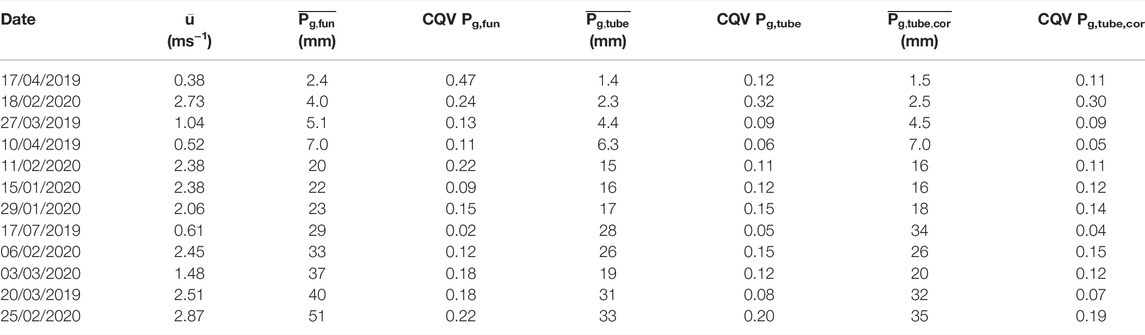
TABLE 3. Overview of the sampled gross precipitation and wind conditions during the uncovered period ordered according to the cumulated events size. Weeks without rain are not listed. Variables shown are: recorded weekly average of wind speed (
We used linear mixed effects model to investigate governing factors on the interception tubes rainfall measurements. We confirmed visually the normality and homogeneity of the residuals. The fixed factors were major contributor to R2 of the model, indicating a strong explanatory power of the model. The total R2 is 0.94, and fixed and random factors’ part are 0.85 and 0.09 respectively. Figure 5 shows the fixed effects estimates (slopes) for the corrected tubes’ measurements (Pg,tube,cor). The gross precipitation was the major positive driver of the tubes’ measurement. The fixed effect estimate of the gross precipitation was 0.92 with the high significance. Also, the elevation of the measurement location became only significant in interaction with the precipitation amount. Interception tubes located at higher elevations received more water under smaller cumulated precipitation events while these tubes collected less water during bigger cumulated events compared to the lower elevations (Figure 6). Wind speed by itself and its effect on precipitation were no drivers directly altering interception tubes measurements in the absence of foliage cover.
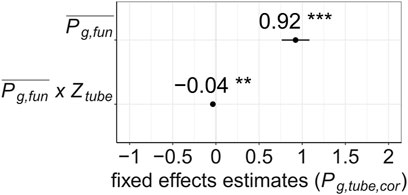
FIGURE 5. Factors affecting splash loss corrected precipitation measurement with the tubes (Pg,tube corrected) during the uncovered period. Values on the x-axis indicate the slope of the relations. Significant predictors are: weekly measured mean gross precipitation (
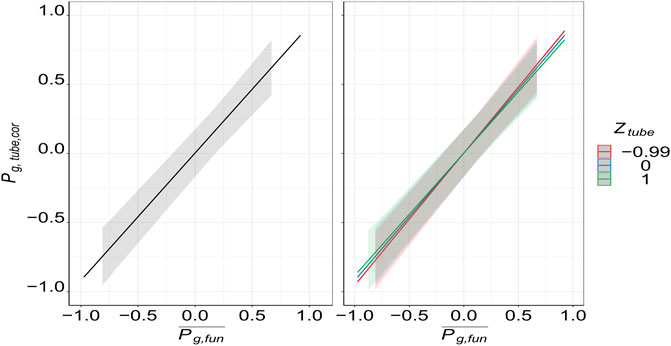
FIGURE 6. Visualisation of the significant relations shown in Figure 5, representing the significant drivers of corrected tube gross precipitation measurements (Pg,tubes,cor) during the uncovered period. Relation to (left) mean gross precipitation (
3.3 Covered Period
Table 4 gives an overview of net precipitation and environmental conditions during the active growing season, i.e., the covered period. There, the spatial variation in gross precipitation was smaller, which may be attributed to lower wind speed. The interception tubes measurements revealed that the spatial variation in net precipitation was higher for smaller events compared to larger events. Coincidentally, the smaller events mostly took place when the grass height was taller.
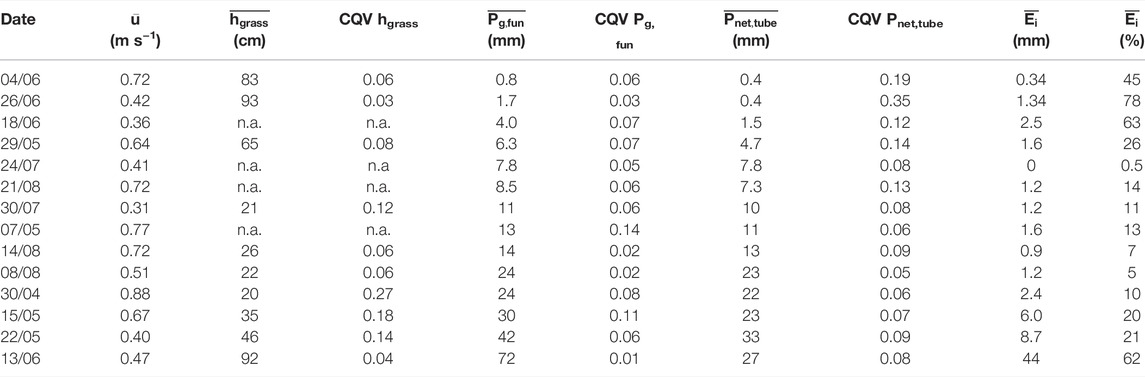
TABLE 4. Overview of the properties of the net precipitation sampling periods during the covered period in 2019. Weeks without rain are not listed. n.a. indicates missing data. Variables shown are: recorded weekly average of wind speed (
Moreover, increase in soil water storage response to water input measured by gross precipitation and interception tubes measurements (Figure 7) showed that the tubes measurements notably underestimated the water input in particularly under tall sized grass (up to 93 cm). The interception tubes might miss stemflow possibly due to the diversion of stemflow away from collecting orifice, and this could be the reason for the underestimation. In other words, the interception tubes captured mainly throughfall.
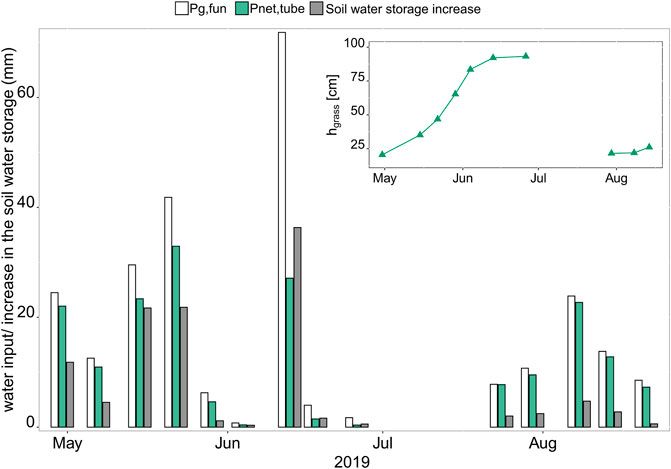
FIGURE 7. Weekly spatial mean of gross precipitation (Pg, fun), net precipitation (Pnet, tube) and increase in the soil water storage for the soil column 0–37.5 cm depth during the covered period. Soil water storage was calculated based on the soil moisture sensors in the topsoil (7.5 cm) and sub soil (27.5 cm). Inlet: Weekly spatial average of grass height. Only values of hgrass > 15 cm are shown, the interruption in the time series is due to the annual mowing.
Figure 8 shows the interception tubes captured the spatial pattern of throughfall, which was temporally persistent and potentially governed by the vegetation (see below). Some measurement locations typically received less water than the average (δPnet,tube < 0) while others remained on the average or became wetter (δPnet,tube > 0) throughout the growing period. Notably, more and less water collected locations differed from the uncovered period (Figure 8), indicating that other factors but the interception tubes themselves were causing the persistence of the pattern in the covered period Also, the tubes measurements reproduced the expected trend in interception loss in the developed grassland canopy (
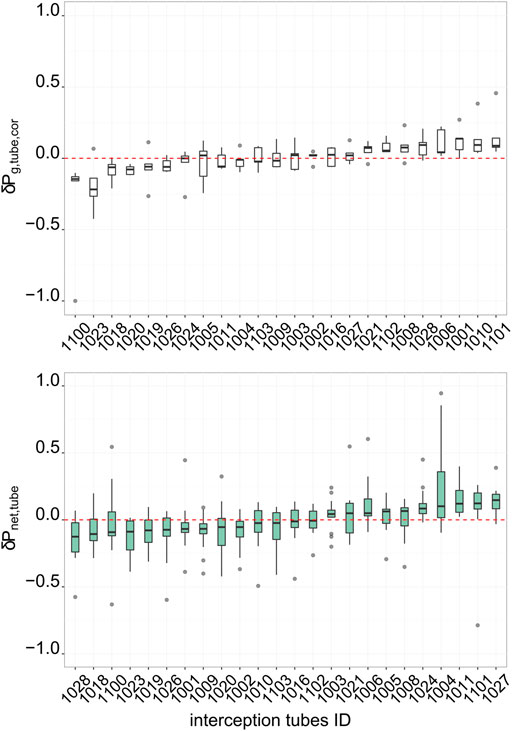
FIGURE 8. Temporal stability of precipitation patterns based on the spatial deviation of the interception tube measurements in 2019 (April–August) for (top) uncovered period and (bottom) covered period. X axis indicates location identification, and Y axis gives the spatial deviation from the mean of the tubes’ measurements for the particular location for all sampling weeks.
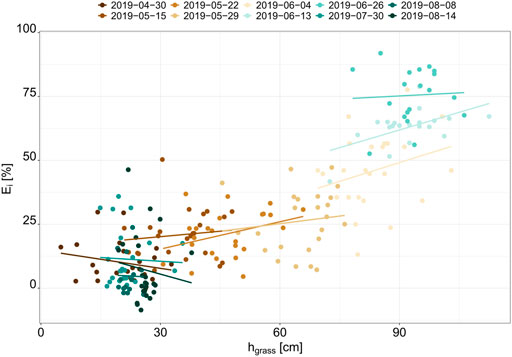
FIGURE 9. Relation between cumulative weekly interception loss (Ei) and grass height (hgrass). Each point stands for one location. Measurement weeks are differently coloured.
The mixed effects model results (Figure 10) show that the key drivers of the interception tubes measurements were gross precipitation and followed by the mean grass height, representing the seasonal evolution of the grass canopy. Also, the interaction between the spatial deviation of grass height from the mean (e.g., the spatial pattern) and the average wind speed influenced the net precipitation measurements. Major contribution for R2 was high from the fixed factors -0.85- while the random factors contribution was 0.11.
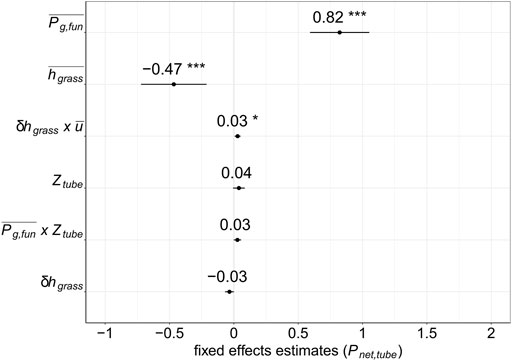
FIGURE 10. Factors affecting net precipitation measurement (Pnet, tube) during the covered period. Values on the x-axis indicate the slope of the relations. Significant predictors are: weekly measured mean gross precipitation (
Seasonal increase in average grass height reduced the collected throughfall (Figure 10 and Figure 11), while the spatial deviation of grass height from the mean (δhgrass) had no significant influence in the model by itself. However, together with the wind speed, it had a significant effect. In other words, the canopy created wind shield effect relied on spatial deviation of grass height. Stronger wind speed increased throughfall where the grass was taller and inversely reduced the captured volume where the grass was shorter (Figure 11). Elevation, its interaction with gross precipitation and the spatial deviation of grass height improved the model yet, they were not significant drivers.
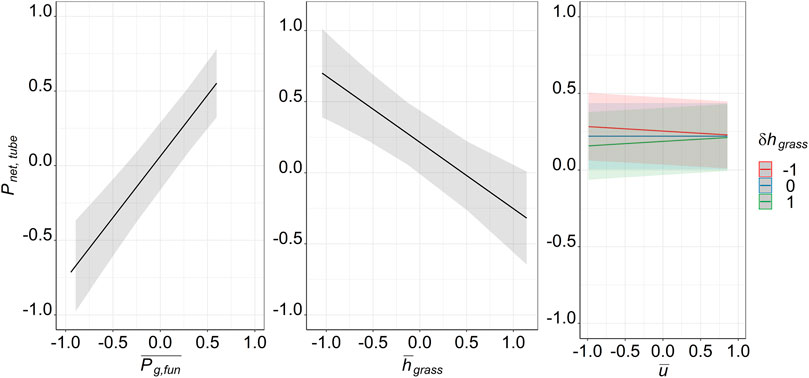
FIGURE 11. Visualisation of the significant relations shown in Figure 10, representing the significant drivers of the net precipitation measurements (Pnet, tube) during the covered period. Relation to (left) mean gross precipitation (
4 Discussion
4.1 Splash Loss
The lab experiment indicated that splash loss did not lead to high uncertainty for the interception tubes measurements, even for typical big drops of the spring-summer rainfall events, and largest throughfall drops. At the research site, spring-summer events mostly produced smaller drops associated with low splash loss (less than 5%) during the uncovered period. The partly opened - sectorial opening-shape pipes efficiently reduced the splash loss also in the sampling with the interception tubes, as already found by Friesen and Köhler (2014). Nevertheless, the interception tubes design can be improved by adapting knife-edge designed opening to handle better off-center drops which decreases uncertainty in sampled volume in the orifice collection area.
Throughfall drop size is a function of several spatio-temporally varied biotic and abiotic elements. Next to rain intensity, canopy alters the throughfall drop size distribution (Hall and Calder, 1993; Nanko, 2016; Levia et al., 2019). Orientation of surface and shape of leaf determine throughfall drop sizes along with the angle and count of branches besides height of the first branch (Hall and Calder, 1993; Nanko et al., 2013; Levia et al., 2017). It is challenging to determine splash loss with weekly samplings during the covered period owing to fast changes in the foliage cover. Further, interaction with the short canopy would generally slow throughfall drops (Zhou et al., 2002; Frasson and Krajewski, 2011). Since the grass canopy height never exceeded 1 m, canopy drip would not reach terminal velocity (van Boxel, 1998; Nanko et al., 2008). Short vegetation height reduces kinetic energy also due to short falling distance (Nanko et al., 2015; Goebes et al., 2016) Moreover, typical for temperate grasslands at our site at peak grass height (0.9 m) maximum throughfall drop size can reach only equivalent kinetic energy of small raindrops drops, and negligible splash loss according to the drip experiment. As a result, we deduce that splash loss does not lead to substantial measurement error for tube measurements during the covered period.
4.2 Validation of the Tube Measurements in the Uncovered Period With Conventional Funnel Measurements
We used the conventional gross precipitation measurements to validate the interception tubes’ measurements during the uncovered period. The tubes’ measurements were directly proportional to the funnel measurements, yet on average, the tubes received less precipitation than funnels despite the splash loss correction. We observed that, in the absence of the foliage cover, stronger wind caused higher spatial variability in interception tubes measurements. However, the tubes measurements generally showed less variation around the mean compared to the funnel measurements, which gives confidence in the interception tubes measurement. This could be due to lower wind speed at the ground level. Wind usually is one of the major challenges in rainfall measurement (Nešpor and Sevruk, 1999; Sevruk et al., 2009). Position and shape of the rainfall collectors influence wind induced error, and the collectors placed above ground become an obstacle for the wind field (Sevruk, 1994; Sevruk and Nespor, 1994). However, although the tubes were placed at the ground level, where wind speeds are generally lower, the long interception tubes and the lid of the embedded case may have induced wind currents, causing more under-catch than a classical funnel would have.
To further investigate drivers of the interception tubes measurements, we used linear mixed effects model for both the uncovered and the covered period. Low contribution of random factors to the models indicates that the primary drivers, represented by the fixed factors, were captured by the models. The fixed effect estimates of the model for the uncovered period showed that corrected interception tubes measurements strongly aligned with mean gross precipitation. The positive slope of the relation between two precipitation measurement methods was near unity (0.92) which suggests that the interception tubes properly reproduced precipitation despite the differences in average sampled volume between two methods.
Wind influence on the measurement was not detected with the model during the uncovered period, whereas it became a significant driver for the covered period. Instead, the elevation ranges between 353 m and 355 m above sea level, which probably reflected the wind field along the slight slope, where the tubes were located. The interaction factor between elevation and gross precipitation showed inverse proportional relation to the tube measurements. Topography influences the wind field at the small scale (Sandsborg, 1970) which likely reduced the collected precipitation by the interception tubes.
During the covered period, the average wind speed altered the relation of the interception tubes measurements and the spatial variation of grass height. More water was received at locations with taller grass at higher wind speed compared to lower grass locations. One potential reason may be that the canopy created a windshield, which is known to enhance precipitation sampling (Sevruk, 1994). Since the grass height spatially varied, the wind shading effect was heterogeneous. The significant impact of the topography instead disappeared during the covered period, which further indicates that the grass canopy acted as a windshield and reduced interference of the tubes in wind currents. Therefore, during the covered period, we expect less wind induced error in interception tubes measurements compared to the uncovered period. On the other hand, wind can affect measurements as it might flex or bend leaves and stems. Further wind induced vibration or shaking can influence measurements. These impacts are dependent on how wind sways canopy, and level of wind speed. In the covered periods, the wind speed was generally lower than 0.9 ms−1 whereas during the uncovered period, wind speed increased up to 2.87 ms−1. Moreover, wind speed by itself was not an important factor for the tubes measurements according to the statistical model results, which would be expected if wind generally increased the throughfall amount, e.g. by decreasing stemflow and increasing the dripping part of the net precipitation. Overall, we deduce that wind shielding by the canopy is the most plausible cause for the observed interaction.
4.3 Plausibility of Net Precipitation Measurements in Covered Conditions
4.3.1 Validation of Net Precipitation Measurements Based on Soil Water Balance
In order to validate our interception tubes measurements during covered period, we used the increase in the soil water storage. Since net precipitation is the amount of water that enters the ground, it should be almost equivalent to an increase in soil water content according to the simple mass balance. Early in the growing season, the increase in the soil water content was lower than net precipitation, which we attribute to the presence of preferential flow paths. However, in the presence of tall grass (>60 cm), the tubes collected net precipitation was lower than the increase in soil water storage (Figure 7). This can only be explained by the interception tubes underestimating net precipitation, particularly for tall grass and is most likely related to stemflow bypassing interception tubes. As a result of seasonal development of canopy, taller grass stems might have diverted water away from the interception tubes and sampled stemflow amount might change over time. Moreover, stemflow partitioning in herbaceous vegetation is altered by thickness, height and angle of stems together with the rain intensity (de Ploey, 1982). The growth of stems and stem elongation, are promoted by taller canopy height (Gusmão Filho et al., 2020; Macedo et al., 2021). Thus, taller grass is expected to generate sizable stemflow, whereas shorter grass produces had a negligible amount of stemflow as found in prairie grass and wheat (Clark, 1940; Butler and Huband, 1985). Through the growing season, leaf and stem structure changes in grasslands due to the physiological developments. For instance, Sahramaa and Jauhiainen (2003) observed that stem elongation rate of reed canary grass increased during flag leaf development and blossoming. Yet it decreased during the anthesis, and plant height still increased. We also observed in our field site that canopy architecture differed through growing season with tall flower and seed tillers developing as the grassland reached maximum height in some weeks before the summer cut. Because of these physiological changes, the portioning of stemflow likely varied through the growing season, affecting the proportion of net precipitation captured by the interception tubes. The change in the differences between the increase in soil water storage and the interception tubes measurements through the sampling period supports this argument. Also, we showed by the soil water balance that under tall sized grass, especially, the tubes quantitively overestimate interception loss amount due to the mismeasured stemflow. Interception loss can be quantified more accurately by large lysimeters and micro-lysimeters in temperate grasslands as they measure reliably evaporation and evapotranspiration (Harsch et al., 2009; Wegehenkel and Gerke, 2013; Pütz et al., 2016; Groh et al., 2019). However, large lysimeters might not allow easily to observe small scale variation induced by grassland canopy. Further micro lysimeters in temperate climate needs to be controlled for how natural canopy and root growth might be altered due to the restricted root structure (Riedl et al., 2022) since, as we observed, the amount of net precipitation components, thereby interception, changes through seasonal plant development.
4.3.2 Plausibility Check of the Net Precipitation Measurements by Spatial Patterns
The statistical model for the covered period showed that vegetation is a significant driver to determine tube measurements, next to gross precipitation. The average grass height systematically reduced the tubes’ measurements, which implies that the growth of vegetation increased interception loss. The seasonal development of a foliage cover has earlier been found to affect interception loss in grassland (Zou et al., 2015). Also, Crouse et al. (1966) showed that storage capacity, thereby interception loss, depended on grass height in Mediterranean climate. Moreover, for interception loss estimation in temperate climate canopy height is an important element also in short canopies (Breuer et al., 2003). Hence, the result confirms that the interception tubes measurements captured the expected effect of grassland canopy on net precipitation. On the other hand, the spatial pattern of vegetation height was only significant in interaction with wind speed. This further hints at the wind protection created by spatially heterogenous canopy (see above) and the stronger impact of seasonal dynamics of vegetation on the net precipitation measurements.
Our interception tubes measurements during the covered period also showed the direct impact of grass height on the calculated average interception loss, besides the average net precipitation. The average interception loss ranged between 5% and 78% depending on the cumulated event precipitation volumes and grass height (Table 4). The high interception loss in the grassland during small events has been observed with other type of measurements in different climates, which found over 60% interception loss and rise in the interception loss for the smaller events (Clark, 1940; Gilliam et al., 1987; Zou et al., 2015). Thus, while at our site, the estimated interception loss is quantitively overestimated due to underestimated stemflow (see above), the variation across event sizes agrees with expectations. Grass height spatial variation influenced interception loss between the tubes locations also within the same sampling week, so the interception tubes measurements caught the effect of grass height on interception loss both in space and time.
We also looked at the similarity of spatial patterns of interception tubes measurements between different sampling weeks as a further plausibility check of the tubes measurements. The spatial deviation of the interception tubes precipitation measurements from the mean (Figure 8) showed that vegetation altered the spatial patterns of net precipitation which were temporally stable even though the spatial differences in grass height were not significant by itself in the statistical model (Figure 10). The temporal stability of net precipitation patterns in our grassland site agrees with time persistent throughfall and stemflow patterns in forest ecosystems (Keim et al., 2005; Zimmermann et al., 2009; Metzger et al., 2017, 2019; Cisneros Vaca et al., 2018). This similarity of net precipitation patterns between the available studies in forest ecosystems and our data signifies that the tubes captured anticipated vegetation induced precipitation patterns.
Furthermore, our net precipitation data revealed that the spatial variation increased with smaller events (Table 4), which generally agrees with observations in the forested ecosystems (Carlyle-Moses, 2004; Metzger et al., 2017). However, smaller events occurred, coincidentally, when grass was tall which obscures understanding of the reasons behind the higher variation in net precipitation. We are not aware of any other study in the literature about the influence of vegetation features on the spatial variation of net precipitation in grassland to compare our result with. However, in forest ecosystems, the variation of net precipitation is increased not only due to the small event size but also because of the more complex canopy (Zimmermann et al., 2016; Van Stan et al., 2020) which also increased number of potential drip points (Levia and Frost, 2006). The tube measurements generally reflect expected patterns of the influence of vegetation and event size on net precipitation variability, which also suggests that the interception tubes could capture the dynamics of net precipitation.
5 Conclusion
We designed the interception tubes to measure net precipitation in the small canopy at multiple locations under natural conditions. The purpose of the design was to capture throughfall patterns while allowing for little disturbance of the development of the herbaceous canopy in a temperate climate. We tested the interception tubes in the lab with a drip experiment and field measurements in the absence of foliage cover (uncovered period) also later in the growing season (covered period). Plausibility was checked based on precipitation patterns and the change in soil water storage.
After accounting for wind speed in the mixed effects model, the interception tubes could reproduce the conventional gross precipitation measurements during the uncovered period, with a slope of the relation close to unity. Splash loss did not cause a significant measurement problem, and the developed canopy reduced possible wind induced measurement errors. Also, during the covered period, the interception tubes reproduced the expected throughfall patterns, and seasonal canopy growth systematically decreased net precipitation.
The soil water balance suggests that interception tubes underestimate net precipitation in very tall grass, which we attribute to the increase of stemflow and reduction of throughfall in the progressing growing season due to the physiological changes of the vegetation. Moreover, tall grass stems might divert stemflow away from the tubes, namely stemflow might bypass the collection area. Therefore, although the interception tubes have a strong connection to the canopy, it mainly captures throughfall.
The interception tubes capture throughfall patterns under field conditions because of its applicability at multiple locations and possibility for natural canopy growth. Yet, the proposed method has some limitations quantitively estimating of interception loss due to underestimation of stemflow. Event scale field sampling together with additional measurements, such as soil moisture, can assist the interception tubes to improve stemflow assessment, but requires deeper soil moisture profiles in order to allow closing the soil water balance in spite of preferential flow. Despite underestimating stemflow, the interception tubes address the needs of throughfall sampling at natural grasslands in temperate climate conditions.
Data Availability Statement
The raw data supporting the conclusions of this article will be made available by the authors, without undue reservation.
Author Contributions
GD and JFi designed and developed the interception tubes with contribution of BM and AH. GD designed and performed the lab experiment, together with JFr and AH. GD and JFi designed the field setup, and conducted the field work with the help of students named in the acknowledgements. GD analyzed the data and evaluated them with AH. GD and AH prepared the first version of the manuscript and all authors contributed to discussions and writing of the final version.
Funding
This study is part of the Collaborative Research Centre AquaDiva of the Friedrich Schiller University Jena, funded by the Deutsche Forschungsgemeinschaft (DFG, German Research Foundation) – SFB 1076 – Project Number 218627073.
Conflict of Interest
The authors declare that the research was conducted in the absence of any commercial or financial relationships that could be construed as a potential conflict of interest.
Publisher’s Note
All claims expressed in this article are solely those of the authors and do not necessarily represent those of their affiliated organizations, or those of the publisher, the editors and the reviewers. Any product that may be evaluated in this article, or claim that may be made by its manufacturer, is not guaranteed or endorsed by the publisher.
Acknowledgments
We thank AquaDiva subproject D03 for weather station (Reckenbuel) data. Also, we are thankful to Johanna Clara Metzger and people who contributed to installation of soil moisture sensors in the research site. We thank the Hainich CZE site manager Robert Lehmann and the Hainich National Park. We thank the bachelor and master students Carla Peter, Xiaoyu Zhao, Stephan Bock.
Supplementary Material
The Supplementary Material for this article can be found online at: https://www.frontiersin.org/articles/10.3389/feart.2022.799419/full#supplementary-material
References
Ataroff, M., and Naranjo, M. E. (2009). Interception of Water by Pastures of Pennisetum Clandestinum Hochst. ex Chiov. and Melinis Minutiflora Beauv. Agric. For. Meteorol. 149, 1616–1620. doi:10.1016/j.agrformet.2009.05.003
Bartoń, K. (2020). MuMIn: Multi-Model Inference. Available at: https://CRAN.R-project.org/package=MuMIn. (Accessed July 5, 2021).
Bates, D., Mächler, M., Bolker, B., and Walker, S. (2015). Fitting Linear Mixed-Effects Models Using lme4. J. Stat. Soft. 67, 1–48 (Accessed May 7, 2021). doi:10.18637/jss.v067.i01
Beard, J. S. (1962). Rainfall Interception by Grass. South Afr. For. J. 42, 12–15. doi:10.1080/00382167.1962.9629728
Bengtsson, J., Bullock, J. M., Egoh, B., Everson, C., Everson, T., O'Connor, T., et al. (2019). Grasslands-More Important for Ecosystem Services than you Might Think. Ecosphere 10, e02582. doi:10.1002/ecs2.2582
Blöschl, G., and Sivapalan, M. (1995). Scale Issues in Hydrological Modelling: A Review. Hydrol. Process. 9, 251–290. doi:10.1002/hyp.3360090305
Bogena, H. R., Herbst, M., Huisman, J. A., Rosenbaum, U., Weuthen, A., and Vereecken, H. (2010). Potential of Wireless Sensor Networks for Measuring Soil Water Content Variability. Vadose Zone J. 9, 1002–1013. doi:10.2136/vzj2009.0173
Brandt, C. J. (1989). The Size Distribution of Throughfall Drops Under Vegetation Canopies. CATENA 16, 507–524. doi:10.1016/0341-8162(89)90032-5
Breuer, L., Eckhardt, K., and Frede, H.-G. (2003). Plant Parameter Values for Models in Temperate Climates. Ecol. Model. 169, 237–293. doi:10.1016/S0304-3800(03)00274-6
Burgy, R. H., and Pomeroy, C. R. (1958). Interception Losses in Grassy Vegetation. Trans. AGU 39, 1095. doi:10.1029/TR039i006p01095
Butler, D. R., and Huband, N. D. S. (1985). Throughfall and Stem-Flow in Wheat. Agric. For. Meteorol. 35 (1–4), 329–338. doi:10.1016/0168-1923(85)90093-0
Calder, I. R., Hall, R. L., Rosier, P. T. W., Bastable, H. G., and Prasanna, K. T. (1996). Dependence of Rainfall Interception on Drop Size: 2. Experimental Determination of the Wetting Functions and two-Layer Stochastic Model Parameters for Five Tropical Tree Species. J. Hydrol. 185, 379–388. doi:10.1016/0022-1694(95)02999-0
Carlyle-Moses, D. E. (2004). Throughfall, Stemflow, and Canopy Interception Loss Fluxes in a Semi-Arid Sierra Madre Oriental Matorral Community. J. Arid Environ. 58, 181–202. doi:10.1016/S0140-1963(03)00125-3
Cisneros Vaca, C., Ghimire, C., and Van der Tol, C. (2018). Spatial Patterns and Temporal Stability of Throughfall in a Mature Douglas-fir Forest. Water 10, 317. doi:10.3390/w10030317
Clark, O. R. (1940). Interception of Rainfall by Prairie Grasses, Weeds, and Certain Crop Plants. Ecol. Monogr. 10, 243–277. doi:10.2307/1948607
Coenders-Gerrits, A. M. J., Hopp, L., Savenije, H. H. G., and Pfister, L. (2013). The Effect of Spatial Throughfall Patterns on Soil Moisture Patterns at the Hillslope Scale. Hydrol. Earth Syst. Sci. 17, 1749–1763. doi:10.5194/hess-17-1749-2013
Couturier, D. E., and Ripley, E. A. (1973). Rainfall Interception in Mixed Grass Prairie. Can. J. Plant Sci. 53, 659–663. doi:10.4141/cjps73-130
Crockford, R. H., and Richardson, D. P. (2000). Partitioning of Rainfall Into Throughfall, Stemflow and Interception: Effect of Forest Type, Ground Cover and Climate. Hydrol. Process. 14, 2903–2920. doi:10.1002/1099-1085(200011/12)14:16/17<2903:aid-hyp126>3.0.co;2-6
Crouse, R. P., Corbett, E. S., and Seegrist, D. W. (1966). Methods of Measuring and Analyzing Rainfall Interception by Grass. Int. Assoc. Sci. Hydrol. Bull. 11, 110–120. doi:10.1080/02626666609493463
David, J. S., Valente, F., and Gash, J. H. (2005). “Evaporation of Intercepted Rainfall,” in Encyclopedia of Hydrological Sciences. Editors M. G. Anderson, and J. J. McDonnell (Chichester, UK: John Wiley & Sons). doi:10.1002/0470848944.hsa046
de Ploey, J. (1982). A Stemflow Equation for Grasses and Similar Vegetation. CATENA 9, 139–152. doi:10.1016/S0341-8162(82)80010-6
Douinot, A., Tetzlaff, D., Maneta, M., Kuppel, S., Schulte‐Bisping, H., and Soulsby, C. (2019). Ecohydrological Modelling with EcH2O-iso to Quantify Forest and Grassland Effects on Water Partitioning and Flux Ages. Hydrol. Process. 33, 2174–2191. doi:10.1002/hyp.13480
Dunkerley, D. (2000). Measuring Interception Loss and Canopy Storage In Dryland Vegetation: A Brief Review and Evaluation of Available Research Strategies. Hydrol. Process. 14, 669–678. doi:10.1002/(sici)1099-1085(200003)14:4<669::aid-hyp965>3.0.co;2-i
Dunkerley, D. (2010). A New Method for Determining the Throughfall Fraction and Throughfall Depth in Vegetation Canopies. J. Hydrol. 385, 65–75. doi:10.1016/j.jhydrol.2010.02.004
Dunkerley, D. (2014). Stemflow on the Woody Parts of Plants: Dependence on Rainfall Intensity and Event Profile From Laboratory Simulations. Hydrol. Process. 28, 5469–5482. doi:10.1002/hyp.10050
Dunkerley, D. (2015). Intra-Event Intermittency of Rainfall: an Analysis of the Metrics of Rain and No-Rain Periods. Hydrol. Process. 29, 3294–3305. doi:10.1002/hyp.10454
Filipzik, J., Michalzik, B., Demir, G., Kunze, D., and Hildebrandt, A. (2019). “Method proposal for net precipitation measurements on grassland,” in EGU 2019, Vienna, Austria.
Fischer, C., Leimer, S., Roscher, C., Ravenek, J., de Kroon, H., Kreutziger, Y., et al. (2019). Plant Species Richness and Functional Groups Have Different Effects on Soil Water Content in a Decade‐Long Grassland Experiment. J. Ecol. 107, 127–141. doi:10.1111/1365-2745.13046
Frasson, R. P. d. M., and Krajewski, W. F. (2011). Characterization of the Drop-Size Distribution and Velocity-Diameter Relation of the Throughfall Under the Maize Canopy. Agric. For. Meteorol. 151, 1244–1251. doi:10.1016/j.agrformet.2011.05.001
Friesen, J., and Köhler, A. (2014). “Analysis of Splash Loss for Different Throughfall Trough Designs,” in EGU 2014, Vienna, Austria.
Geißler, C., Kühn, P., Böhnke, M., Bruelheide, H., Shi, X., and Scholten, T. (2012). Splash Erosion Potential Under Tree Canopies in Subtropical SE China. CATENA 91, 85–93. doi:10.1016/j.catena.2010.10.009
Gilliam, F. S., Seastedt, T. R., and Knapp, A. K. (1987). Canopy Rainfall Interception and Throughfall in Burned and Unburned Tallgrass Prairie. Southwest. Nat. 32, 267. doi:10.2307/3671570
Goebes, P., Schmidt, K., Härdtle, W., Seitz, S., Stumpf, F., Oheimb, G. v., et al. (2016). Rule-Based Analysis of Throughfall Kinetic Energy to Evaluate Biotic and Abiotic Factor Thresholds to Mitigate Erosive Power. Prog. Phys. Geogr. Earth Environ. 40, 431–449. doi:10.1177/0309133315624642
Gordon, D. A. R., Coenders-Gerrits, M., Sellers, B. A., Sadeghi, S. M. M., and Van Stan, J. T. (2020). Rainfall Interception and Redistribution by a Common North American Understory and Pasture Forb, Eupatorium capillifolium (Lam. dogfennel). Hydrol. Earth Syst. Sci. 24, 4587–4599. doi:10.5194/hess-24-4587-2020
Groh, J., Pütz, T., Gerke, H. H., Vanderborght, J., and Vereecken, H. (2019). Quantification and Prediction of Nighttime Evapotranspiration for Two Distinct Grassland Ecosystems. Water Resour. Res. 55, 2961–2975. doi:10.1029/2018WR024072
Gusmão Filho, J. D., Deitos Fries, D., Maia de Lana Sousa, B., Lara Fagundes, J., Acosta Backes, A., Santos Dias, D. L., et al. (2020). Growth Dynamics and Senescence of Digit Grass as a Response to Several Canopy Heights. Rev. Mex. Cienc. Pecu. 11, 38–52. doi:10.22319/rmcp.v11i1.4913
Guswa, A. J., and Spence, C. M. (2012). Effect of throughfall Variability on Recharge: Application to Hemlock and Deciduous Forests in Western Massachusetts. Ecohydrol. 5, 563–574. doi:10.1002/eco.281
Hall, R. L., and Calder, I. R. (1993). Drop Size Modification by Forest Canopies: Measurements Using a Disdrometer. J. Geophys. Res. 98, 18465–18470. doi:10.1029/93JD01498
Harsch, N., Brandenburg, M., and Klemm, O. (2009). Large-Scale Lysimeter Site St. Arnold, Germany: Analysis of 40 Years of Precipitation, Leachate and Evapotranspiration. Hydrol. Earth Syst. Sci. 13, 305–317. doi:10.5194/hess-13-305-2009
Heil, G. W., Werger, M. J. A., de Mol, W., van Dam, D., and Heijne, B. (1988). Capture of Atmospheric Ammonium by Grassland Canopies. Science 239, 764–765. doi:10.1126/science.239.4841.764
Jian, S., Zhao, C., Fang, S., and Yu, K. (2014). Characteristics of Caragana korshinskii and Hippophae rhamnoides stemflow and their significance in soil moisture enhancement in Loess Plateau, China. J. Arid. Land 6, 105–116. doi:10.1007/s40333-013-0189-4
Keim, R. F., Skaugset, A. E., and Weiler, M. (2005). Temporal Persistence of Spatial Patterns in Throughfall. J. Hydrol. 314, 263–274. doi:10.1016/j.jhydrol.2005.03.021
Kimmins, J. P. (1973). Some Statistical Aspects of Sampling Throughfall Precipitation in Nutrient Cycling Studies in British Columbian Coastal Forests. Ecology 54, 1008–1019. doi:10.2307/1935567
Klos, P. Z., Chain-Guadarrama, A., Link, T. E., Finegan, B., Vierling, L. A., and Chazdon, R. (2014). Throughfall Heterogeneity in Tropical Forested Landscapes as a Focal Mechanism For Deep Percolation. J. Hydrol. 519, 2180–2188. doi:10.1016/j.jhydrol.2014.10.004
Kostelnik, K. M., Lynch, J. A., Grimm, J. W., and Corbett, E. S. (1989). Sample Size Requirements for Estimation of Throughfall Chemistry Beneath a Mixed Hardwood Forest. J. Environ. Qual. 18, 274–280. doi:10.2134/jeq1989.00472425001800030005x
G. Lemaire, J. Hodgson, and A. Chabbi (Editors) (2011). Grassland Productivity and Ecosystem Services (Wallingford, Oxfordshire ; Cambridge, MA: CABI).
Levia, D. F., and Frost, E. E. (2003). A Review and Evaluation of Stemflow Literature in the Hydrologic and Biogeochemical Cycles of Forested and Agricultural Ecosystems. J. Hydrol. 274, 1–29. doi:10.1016/S0022-1694(02)00399-2
Levia, D. F., and Frost, E. E. (2006). Variability of Throughfall Volume and Solute Inputs in Wooded Ecosystems. Prog. Phys. Geogr. Earth Environ. 30, 605–632. doi:10.1177/0309133306071145
Levia, D. F., and Germer, S. (2015). A Review of Stemflow Generation Dynamics and Stemflow-Environment Interactions in Forests and Shrublands. Rev. Geophys. 53, 673–714. doi:10.1002/2015RG000479
Levia, D. F., Hudson, S. A., Llorens, P., and Nanko, K. (2017). Throughfall Drop Size Distributions: A Review and Prospectus for Future Research: Throughfall Drop Size Distributions. WIREs Water 4, e1225. doi:10.1002/wat2.1225
Levia, D. F., Nanko, K., Amasaki, H., Giambelluca, T. W., Hotta, N., Iida, S. i., et al. (2019). Throughfall Partitioning by Trees. Hydrol. Process. 33, 1698–1708. doi:10.1002/hyp.13432
Llorens, P., and Domingo, F. (2007). Rainfall Partitioning by Vegetation under Mediterranean Conditions. A Review of Studies in Europe. J. Hydrol. 335, 37–54. doi:10.1016/j.jhydrol.2006.10.032
Macedo, V. H. M., Cunha, A. M. Q., Cândido, E. P., Domingues, F. N., da Silva, W. L., Lara, M. A. S., et al. (2021). Canopy Structural Variations Affect the Relationship Between Height and Light Interception in Guinea Grass. Field Crops Res. 271, 108249. doi:10.1016/j.fcr.2021.108249
Madani, E. M., Jansson, P. E., and Babelon, I. (2017). Differences in Water Balance Between Grassland and forest Watersheds Using Long-Term Data, Derived Using the CoupModel. Hydrol. Res. 49, 72–89. doi:10.2166/nh.2017.154
Magliano, P. N., Whitworth‐Hulse, J. I., Florio, E. L., Aguirre, E. C., and Blanco, L. J. (2019). Interception Loss, Throughfall and Stemflow By Larrea Divaricata : The Role of Rainfall Characteristics and Plant Morphological Attributes. Ecol. Res. 34, 753–764. doi:10.1111/1440-1703.12036
McJannet, D., Wallace, J., and Reddell, P. (2007). Precipitation Interception in Australian Tropical Rainforests: I. Measurement of Stemflow, Throughfall and Cloud Interception. Hydrol. Process. 21, 1692–1702. doi:10.1002/hyp.6347
Metzger, J. C., Wutzler, T., Dalla Valle, N., Filipzik, J., Grauer, C., Lehmann, R., et al. (2017). Vegetation Impacts Soil Water Content Patterns by Shaping Canopy Water Fluxes and Soil Properties. Hydrol. Process. 31, 3783–3795. doi:10.1002/hyp.11274
Metzger, J. C., Schumacher, J., Lange, M., and Hildebrandt, A. (2019). Neighbourhood and Stand Structure Affect Stemflow Generation in a Heterogeneous Deciduous Temperate Forest. Hydrol. Earth Syst. Sci. 23, 4433–4452. doi:10.5194/hess-23-4433-2019
Molina, A. J., Llorens, P., Garcia-Estringana, P., Moreno de las Heras, M., Cayuela, C., Gallart, F., et al. (2019). Contributions of Throughfall, Forest and Soil Characteristics to Near-Surface Soil Water-Content Variability at the Plot Scale in a Mountainous Mediterranean Area. Sci. Total Environ. 647, 1421–1432. doi:10.1016/j.scitotenv.2018.08.020
Muzylo, A., Llorens, P., Valente, F., Keizer, J. J., Domingo, F., and Gash, J. H. C. (2009). A review of Rainfall Interception Modelling. J. Hydrol. 370, 191–206. doi:10.1016/j.jhydrol.2009.02.058
Návar, J., and Bryan, R. (1990). Interception Loss and Rainfall Redistribution by Three Semi-Arid Growing Shrubs in Northeastern Mexico. J. Hydrol. 115, 51–63. doi:10.1016/0022-1694(90)90197-6
Nanko, K., Hotta, N., and Suzuki, M. (2006). Evaluating the influence of canopy species and meteorological factors on throughfall drop size distribution. J. Hydrol. 329, 422–431. doi:10.1016/j.jhydrol.2006.02.036
Nanko, K., Onda, Y., Ito, A., and Moriwaki, H. (2008). Effect of Canopy Thickness and Canopy Saturation on the Amount and Kinetic Energy of Throughfall: An Experimental Approach. Geophys. Res. Lett. 35. doi:10.1029/2007GL033010
Nanko, K., Watanabe, A., Hotta, N., and Suzuki, M. (2013). Physical Interpretation of the Difference in Drop Size Distributions of Leaf Drips Among Tree Species. Agric. For. Meteorol. 169, 74–84. doi:10.1016/j.agrformet.2012.09.018
Nanko, K., Giambelluca, T. W., Sutherland, R. A., Mudd, R. G., Nullet, M. A., and Ziegler, A. D. (2015). Erosion Potential under Miconia Calvescens Stands on the Island of Hawai'i. Land Degrad. Dev. 26, 218–226. doi:10.1002/ldr.2200
Nanko, K. (2016). Differences in Throughfall Drop Size Distributions in the Presence and Absence of Foliage. Hydrol. Sci. J. 61, 8. doi:10.1080/02626667.2015.1052454
Nešpor, V., and Sevruk, B. (1999). Estimation of Wind-Induced Error of Rainfall Gauge Measurements Using a Numerical Simulation. J. Atmos. Ocean. Technol. 16, 450–464.
Ochoa-Sánchez, A., Crespo, P., and Célleri, R. (2018). Quantification of Rainfall Interception in the High Andean Tussock Grasslands. Ecohydrology 11, e1946. doi:10.1002/eco.1946
Potthast, K., Meyer, S., Crecelius, A. C., Schubert, U. S., Tischer, A., and Michalzik, B. (2017). Land-Use and Fire Drive Temporal Patterns of Soil Solution Chemistry and Nutrient Fluxes. Sci. Total Environ. 605-606, 514–526. doi:10.1016/j.scitotenv.2017.06.182
Pütz, T., Kiese, R., Wollschläger, U., Groh, J., Rupp, H., Zacharias, S., et al. (2016). TERENO-SOILCan: a Lysimeter-Network in Germany Observing Soil Processes and Plant Diversity Influenced by Climate Change. Environ. Earth Sci. 75, 1242. doi:10.1007/s12665-016-6031-5
R Core Team (2021). R: A Language and Environment for Statistical Computing. Vienna, Austria: R Foundation for Statistical Computing. Available at: https://www.r-project.org/.
Riedl, A., Li, Y., Eugster, J., Buchmann, N., and Eugster, W. (2022). Technical note: High-accuracy weighing micro-lysimeter system for long-term measurements of non-rainfall water inputs to grasslands. Hydrol. Earth Syst. Sci. 26, 91–116. doi:10.5194/hess-26-91-2022
Sadeghi, S. M. M., Gordon, D. A., and Van Stan, J. T. (2020). “A Global Synthesis of Throughfall and Stemflow Hydrometeorology,” in Precipitation Partitioning by Vegetation: A Global Synthesis. Editors J. T. Van Stan John, E. Gutmann, and J. Friesen (Cham: Springer International Publishing), 49–70. doi:10.1007/978-3-030-29702-2_4
Sahramaa, M., and Jauhiainen, L. (2003). Characterization of Development and Stem Elongation of Reed Canary Grass Under Northern Conditions. Industrial Crops Prod. 18, 155–169. doi:10.1016/S0926-6690(03)00044-X
Sandsborg, J. (1970). The Effect of Wind on the Precipitation Distribution Over a Hillock. Hydrol. Res. 1, 235–244. doi:10.2166/nh.1970.0016
Seastedt, T. R. (1985). Canopy Interception of Nitrogen in Bulk Precipitation by Annually Burned and Unburned Tallgrass Prairie. Oecologia 66, 88–92. doi:10.1007/BF00378557
Sevruk, B., and Nespor, V. (1994). “The Effect of Dimensions and Shape of Precipitation Gauges on the Wind-Induced Error,” in Global Precipitations and Climate Change. Editors M. Desbois, and F. Désalmand (Berlin, Heidelberg: Springer Berlin Heidelberg), 231–246. NATO ASI Series. doi:10.1007/978-3-642-79268-7_14
Sevruk, B., Ondrás, M., and Chvíla, B. (2009). The WMO Precipitation Measurement Intercomparisons. Atmos. Res. 92, 376–380. doi:10.1016/j.atmosres.2009.01.016
Sevruk, B. (1994). “Spatial and Temporal Inhomogeneity of Global Precipitation Data,” in Global Precipitations and Climate Change . Editors M. Desbois, and F. Désalmand (Berlin, Heidelberg: Springer Berlin Heidelberg), 219–230. NATO ASI Series. doi:10.1007/978-3-642-79268-710.1007/978-3-642-79268-7_13
Staelens, J., De Schrijver, A., Verheyen, K., and Verhoest, N. E. C. (2008). Rainfall Partitioning Into Throughfall, Stemflow, and Interception Within a Single Beech (Fagus sylvatica L.) Canopy: Influence of Foliation, Rain Event Characteristics, and Meteorology. Hydrol. Process. 22, 33–45. doi:10.1002/hyp.6610
Tripp, G. K., Good, K. L., Motta, M. J., Kass, P. H., and Murphy, C. J. (2016). The Effect of Needle Gauge, Needle Type, and Needle Orientation on the Volume of a Drop. Vet. Ophthalmol. 19, 38–42. doi:10.1111/vop.12253
Uplinger, W. G. (1981). “A New Formula For Raindrop Terminal Velocity - Google Scholar,” in Proceedings of the 20th Conference on Radar Meteorology, Boston, Mass, USA, 389–391.
van Boxel, J. (1998). Numerical Model for the Fall Speed of Raindrops in a Rainfall Simulator. I.C.E. Special Report 1998/1, 77–85.
van Dam, D., Heil, G. W., Heijne, B., and Bobbink, R. (1991). Throughfall Below Grassland Canopies: A Comparison of Conventional and Ion Exchange Methods. Environ. Pollut. 73, 85–99. doi:10.1016/0269-7491(91)90016-P
Van Stan, J. T., Siegert, C. M., Levia, D. F., and Scheick, C. E. (2011). Effects of Wind-Driven Rainfall on Stemflow Generation Between Codominant Tree Species with Differing Crown Characteristics. Agric. For. Meteorol. 151, 1277–1286. doi:10.1016/j.agrformet.2011.05.008
Van Stan, J. T., Hildebrandt, A., Friesen, J., Metzger, J. C., and Yankine, S. A. (2020). “Spatial Variability and Temporal Stability of Local Net Precipitation Patterns,” in Precipitation Partitioning by Vegetation: A Global Synthesis. Editors I. Van Stan John T., E. Gutmann, and J. Friesen (Cham: Springer International Publishing), 89–104. doi:10.1007/978-3-030-29702-2_6
Voss, S., Zimmermann, B., and Zimmermann, A. (2016). Detecting Spatial Structures in Throughfall Data: The Effect of Extent, Sample Size, Sampling Design, and Variogram Estimation Method. J. Hydrol. 540, 527–537. doi:10.1016/j.jhydrol.2016.06.042
Wegehenkel, M., and Gerke, H. H. (2013). Comparison of Real Evapotranspiration Measured By Weighing Lysimeters With Simulations Based on the Penman Formula and a Crop Growth Model. J. Hydrol. Hydromech. 61, 161–172. doi:10.2478/johh-2013-0021
Western, A. W., Grayson, R. B., and Blöschl, G. (2002). Scaling of Soil Moisture: A Hydrologic Perspective. Annu. Rev. Earth Planet. Sci. 30, 149–180. doi:10.1146/annurev.earth.30.091201.140434
Williams, C. A., Reichstein, M., Buchmann, N., Baldocchi, D., Beer, C., Schwalm, C., et al. (2012). Climate and Vegetation Controls on the Surface Water Balance: Synthesis of Evapotranspiration Measured Across a Global Network of Flux Towers. Water Resour. Res. 48. doi:10.1029/2011WR011586
Wollny, E. (1890). Untersuchungen über das Verhalten der atmosphärischen Niederschläge zur Pflanze und zum Boden. Forschungen Auf Dem Geb. Agrik.-Phys. 13, 316–356.
Yang, X.-g., Chen, L., Wang, L., Wang, X., Gu, J.-l., Qu, W.-j., et al. (2019). Dynamic Rainfall-Partitioning Relationships Among Throughfall, Stemflow, and Interception Loss by Caragana Intermedia. J. Hydrol. 574, 980–989. doi:10.1016/j.jhydrol.2019.04.083
Zhang, Z.-S., Zhao, Y., Li, X.-R., Huang, L., and Tan, H.-J. (2016). Gross Rainfall Amount and Maximum Rainfall Intensity in 60-Minute Influence on Interception Loss of Shrubs: a 10-Year Observation in the Tengger Desert. Sci. Rep. 6, 26030. doi:10.1038/srep26030
Zhang, Y.-f., Wang, X.-p., Pan, Y.-x., and Hu, R. (2020). Relative Contribution of Biotic and Abiotic Factors to Stemflow Production and Funneling Efficiency: A Long-Term Field Study on a Xerophytic Shrub Species In Tengger Desert of Northern China. Agric. For. Meteorol. 280, 107781. doi:10.1016/j.agrformet.2019.107781
Zhou, G., Wei, X., and Yan, J. (2002). Impacts of Eucalyptus (Eucalyptus Exserta) Plantation on Sediment Yield in Guangdong Province, Southern China-A Kinetic Energy Approach. CATENA 49, 231–251. doi:10.1016/S0341-8162(02)00030-9
Zimmermann, A., Zimmermann, B., and Elsenbeer, H. (2009). Rainfall Redistribution in a Tropical Forest: Spatial and Temporal Patterns. Water Resour. Res. 45. doi:10.1029/2008WR007470
Zimmermann, B., Zimmermann, A., Lark, R. M., and Elsenbeer, H. (2010). Sampling Procedures for Throughfall Monitoring: A Simulation Study. Water Resour. Res. 46. doi:10.1029/2009WR007776
Zimmermann, A., Voss, S., Metzger, J. C., Hildebrandt, A., and Zimmermann, B. (2016). Capturing Heterogeneity: The Role of A Study Area's Extent for Estimating Mean Throughfall. J. Hydrol. 542, 781–789. doi:10.1016/j.jhydrol.2016.09.047
Zou, C. B., Caterina, G. L., Will, R. E., Stebler, E., and Turton, D. (2015). Canopy Interception for a Tallgrass Prairie under Juniper Encroachment. PLOS ONE 10, e0141422. doi:10.1371/journal.pone.0141422
Keywords: net precipitation, throughfall, stemflow, interception, grassland, field sampling
Citation: Demir G, Friesen J, Filipzik J, Michalzik B and Hildebrandt A (2022) A Method Proposal for Throughfall Measurement in Grassland at Plot Scale in Temperate Climate: ‘Interception Tubes’. Front. Earth Sci. 10:799419. doi: 10.3389/feart.2022.799419
Received: 21 October 2021; Accepted: 14 June 2022;
Published: 14 July 2022.
Edited by:
David Glenn Chandler, Syracuse University, United StatesReviewed by:
Xiaodong Gao, Northwest A&F University, ChinaDavid Dunkerley, Monash University, Australia
Copyright © 2022 Demir, Friesen, Filipzik, Michalzik and Hildebrandt. This is an open-access article distributed under the terms of the Creative Commons Attribution License (CC BY). The use, distribution or reproduction in other forums is permitted, provided the original author(s) and the copyright owner(s) are credited and that the original publication in this journal is cited, in accordance with accepted academic practice. No use, distribution or reproduction is permitted which does not comply with these terms.
*Correspondence: Gökben Demir, Z29la2Jlbi5kZW1pckB1bmktamVuYS5kZQ==