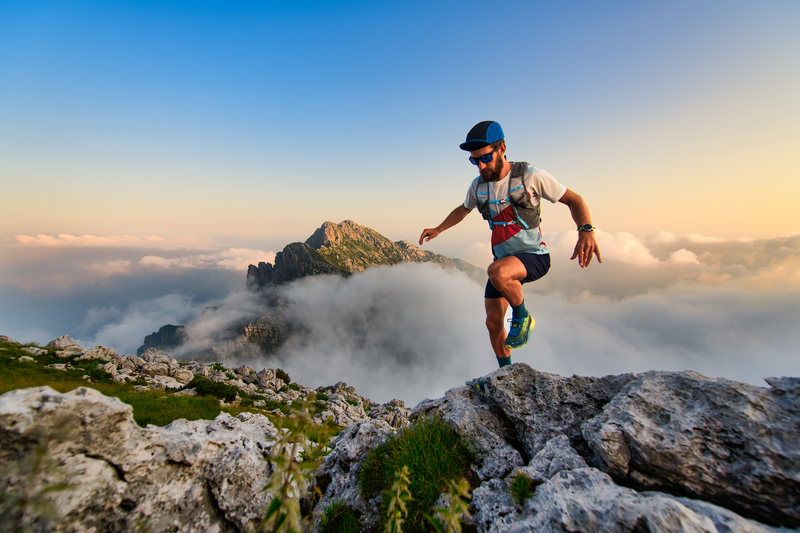
95% of researchers rate our articles as excellent or good
Learn more about the work of our research integrity team to safeguard the quality of each article we publish.
Find out more
MINI REVIEW article
Front. Earth Sci. , 26 April 2022
Sec. Cryospheric Sciences
Volume 10 - 2022 | https://doi.org/10.3389/feart.2022.791760
This article is part of the Research Topic Climate Impacts on Snowpack Dynamics View all 6 articles
Mountain rain-on-snow (ROS) generates large flooding events worldwide. Climate warming will enhance the frequency, magnitude, and widespread nature of these events. Past studies indicate rainfall, not snowmelt, typically drives much of the runoff response during ROS. However, there is substantial event-to-event variability—resulting from shifting atmospheric drivers and nuanced physical mechanisms governing water flow through a snowpack. Historically, turbulent fluxes were assumed to dominate the energy balance for snowmelt during ROS. Recent research nonetheless suggests that other components of the energy balance might be larger drivers depending on: 1) the time of year; 2) the elevation; and 3) the aspect of the slope. This mini review summarizes the literature on the physical processes governing ROS and proposes that moving forward we utilize the terms “active” and “passive” to describe a snowpack’s contribution (via snowmelt) to terrestrial water input (TWI) during ROS. Active snowpacks readily contribute meltwater to TWI via the energy balance, bolstering rainfall-runoff totals. Passive snowpacks do not melt, but simply convey rainwater through the snow matrix. In both snowpack cases, preferential flow paths enhance transmissivity. This proposed classification scheme will help researchers and water managers better communicate and interpret past findings, and aid in forecasting discussions of future events.
Snow-fed mountain watersheds supply freshwater to ∼2 billion people worldwide (Viviroli et al., 2007; Sturm et al., 2017; Immerzeel et al., 2020). However, climate change is endangering this natural service. Declines in winter snowfall and snow albedo are reducing snow’s natural reservoir function by producing both smaller and earlier snowmelt (Dudley et al., 2017; Huss et al., 2017; Mote et al., 2018; Skiles et al., 2018; Lynn et al., 2020; Kraaijenbrink et al., 2021; Siirila-Woodburn et al., 2021). Winter rain-on-snow (ROS), particularly on high elevation alpine snow (Freudiger et al., 2014; Beniston and Stoffel, 2016; Musselman et al., 2018; Hock et al., 2019), further diminishes the snowpack’s ability to accumulate, and simultaneously escalates the risk of extreme hazards including ROS floods, ROS initiated avalanches, and debris flows (Harr, 1981; DeGraff, 1994; Stimberis and Rubin, 2011; Surfleet and Tullos, 2013; Hatchett et al., 2020).
What is ROS? The simplest definition is: measurable rainfall on an existing snow cover (Pomeroy et al., 2016). However, observing and/or measuring the process is easier said than done (Harpold et al., 2017), which gives rise to numerous “definitions”. For example, McCabe et al. (2007) defined ROS as any day in which precipitation occurs and snow depth declines—a good definition given available instrumentation, but the metric fails to quantify flood risk. Alternatively, Musselman et al. (2018) and Huang et al. (2022) defined ROS (with flood potential) as heavy rainfall
Definitions aside, rain falling on a snowpack tends to occur between a few to ∼10 times a season (Moore and Prowse, 1988; Cohen et al., 2015; Würzer et al., 2016; Li et al., 2019; Juras et al., 2021; López-Moreno et al., 2021), and is more frequent in maritime mid-latitude mountains vs. continental, or arctic locations (López-Moreno et al., 2021). Most ROS events do not cause flooding (i.e., Merz and Blöschl, 2003; Yang et al., 2020). But, when floods do arise—they can be historic (e.g., Kattelmann, 1997; Marks et al., 1998; Rössler et al., 2014; Garvelmann et al., 2015; Pomeroy et al., 2016).
This review covers the physical processes governing ROS for midlatitude mountain snowpacks and touches on flood-generating mechanisms. Conceptually, ROS floods exist on a continuum—on one end, rainfall on a snow-dominated landscape producing TWI, and on the other, TWI resulting from both rainfall and snowmelt. Improvements to ROS flood forecasting skill is predicated on knowing the contributions of both fluxes as they impact the timing, duration, and volume of runoff (Tarasova et al., 2019). To help process-based discussion we introduce two new terms— “passive” vs. “active” snowpacks. Passive snowpacks convey rainfall but provide minimal melt, whereas active snowpacks readily melt, enhancing TWI.
Many past ROS floods have been largely rainfall driven (Kattelmann, 1997; Merz and Blöschl, 2003; Wayand et al., 2015; Li et al., 2019). A good example of a more “extreme” snowmelt contribution would be Henn et al. (2020) who found that snowmelt enhanced TWI by 37% in California’s Feather River. But, broadly speaking, what drives a large ROS runoff response?
Simply put, a large ROS runoff response requires an anomalously low snowline, a large snow-covered area (SCA) and prolonged, high-intensity rainfall over most of the SCA. A seasonally low snowline can result from either a preceding cold storm, or within a single storm (Hatchett et al., 2016)—but both scenarios lead to a large SCA. Prolonged, high intensity rainfall can occur under a range of atmospheric conditions. These include: atmospheric rivers (ARs; e.g., Guan et al., 2016; Ralph et al., 2020), cloud microphysical processes such as seeder-feeder mechanisms and localized convection (e.g., Rössler et al. (2014)), enhanced mid-tropospheric moisture fluxes (e.g., Kaplan et al. (2012)), and orographic effects (Houze, 2012). We note that these atmospheric conditions operate in unison or independently, but their identification is fundamental to understanding the spatiotemporal variability in the basin-wide runoff response.
While atmospheric conditions favor prolonged, high-intensity precipitation for a large runoff response, surface processes can either amplify or dampen the runoff. Primary factors include: the snow energy balance, preferential flow, and the antecedent snowpack and soil conditions (Figure 1; Dunne and Black, 1971; Berris and Harr, 1987; Kattelmann, 1997; Singh et al., 1997; Marks et al., 1998; Kattelmann and Dozier, 1999; Rössler et al., 2014; Guan et al., 2016). An active snowpack driven by a large positive energy balance can bolster rainfall driven TWI—particularly across ephemeral (i.e., transient), low-elevation snow with little cold content (Jennings et al., 2018). However, without a saturated landscape and a snowpack with established preferential flow routing (see Section 4), runoff responses could still be dampened and/or delayed (Kattelmann, 1989; McGurk and Marsh, 1995; Singh et al., 1997; Garvelmann et al., 2015; Würzer et al., 2016).
FIGURE 1. (A) A conceptual diagram of the key drivers for a large runoff response for a ROS event. Key ingredients include a low snowline with a large SCA combined with a high intensity (i.e., heavy), long duration rainfall over the majority of the SCA. Modulating factors that can enhance runoff volumes include saturated snow and soils, and a positive energy balance during the event that results in snowmelt contributions to TWI (i.e., an active snowpack). (B) a histogram of available data on snowmelt vs. rainfall contributions to TWI from 10 studies covering 203 ROS events from the following papers: Beaudry and Golding (1983), Bergman (1983), Marks et al. (1998), Rössler et al. (2014), Garvelmann et al. (2015), Wayand et al. (2015), Pomeroy et al. (2016), Corripio and López-Moreno (2017), Mateo-Lázaro et al. (2019), and Henn et al. (2020). Note: not all events are independent, ROS definitions vary by study, and spatial scales represented range from point to basin using either observations or models. Moving forward we would encourage new studies to record the percent contributions to TWI to facilitate future intercomparison studies. (C) the evolution of the snowline in California’s Sierra Nevada (focused on the Tuolumne) using data from NASA’s Moderate Resolution Imaging Spectroradiometer (MODIS) obtained from Worldview (https://worldview.earthdata.nasa.gov/). The first image (ci) demonstrates how a cold storm can initially exacerbate ROS flood risk by increasing SCA beyond traditional mountain regions and expanding ephemeral vs. seasonal (Petersky and Harpold, 2018) snowpack areas. However, flood risk abated as ephemeral snow melted away (cii), dampening some of its potentially “active” impact prior to ROS that occurred after 5 February. (ciii) Shows the snow cover retreat after ROS. This sequence highlights the importance of storm sequencing in ROS flooding—had the 5 February storm occurred earlier, the flood risk would have been far greater. The snow seasonality metric and snowlines were estimated from data obtained via the Snow Property Inversion from Remote Sensing product [SPIReS; Bair et al. (2021)].
Many midlatitude snow-dominated basins have extensive forest coverage, and vegetation structure/density are known to influence snow accumulation via interception, reduced wind effects, and an altered energy balance (Storck et al., 2002; Lundquist et al., 2013; Broxton et al., 2015; Stevens, 2017). Given that ROS involves some of the same processes, it would follow that vegetation should also exert control on resulting ROS runoff. However, results from field studies remain inconclusive. For example, some studies suggest that ROS produces more outflow from glades (forest open areas) compared to forests (Beaudry and Golding, 1983; Berris and Harr, 1987; Storck et al., 2002), while others observed little difference (Kattelmann, 1987; Berg et al., 1991; Garvelmann et al., 2015). A good discussion can be found in Garvelmann et al. (2015)—but, more research is needed.
While our binary classification (passive vs. active) is by design simple, we note that snowpacks exist on a spectrum that can vary both temporally and spatially (Figure 2). For example, at a point location, antecedent snowpack conditions (e.g., the pre-event cold content) coupled with the meteorological conditions will initially drive a singular response (passive or active), however a shift in the meteorological drivers (i.e., a change in weather fronts) could elicit a switch. From a basin perspective, snowpacks are likely composed of a transition from active to passive snowpacks because of elevation-driven temperature differences—particularly when basins encompass large topographic relief. Forest coverage and/or structure will only increase the variability in the snowpack response. Regardless, both snowpack modes (passive vs. active) can dampen and/or delay TWI through an unsatisfied irreducible water content, but only active snowpacks will amplify TWI.
FIGURE 2. A conceptual diagram connecting meteorological drivers for active vs. passive snowpacks to snowpack liquid water routing and finally the speed of water outflow. Note: for a more detailed description of hydraulic barriers with regards to water routing within the snowpack see Webb et al. (2018a).
We define a passive snowpack as one that primarily conveys rainfall (i.e., TWI contributions of snowmelt
Regular snowfall events build cold content (Jennings et al., 2018), enabling a seasonal, deep snowpack to buffer modest meteorological drivers (Haleakala et al., 2021). Shallower snowpacks (i.e., ephemeral snow) are more at risk of melt during ROS. However, the presence of preferential flow—even for an ephemeral snowpack—can modulate the impacts of sensible heat due to rainfall energy. Preferential flow can move large volumes of water through small pores (between 3 and 8% of a cross-sectional area according to McGurk and Marsh, 1995), essentially “insulating” the rest of the pack (Marsh and Woo, 1984; Kattelmann, 1985; McGurk and Marsh, 1995; Schneebeli, 1995). While we study active snowpacks because of their large flooding impacts (i.e., Marks et al. (1998), Rössler et al. (2014), and Henn et al. (2020)), passive snowpacks may be equally potent flood drivers (see Appendix A in Wayand et al., 2015).
Active snowpacks produce relatively large snowmelt volumes (i.e., contributing
The turbulent heat fluxes—particularly the latent heat flux—are dominant drivers of ROS melt. Warm and wet winter storms, often atmospheric rivers (AR), feature high specific humidity and above-average wind speeds (Ralph et al., 2004; Ralph et al., 2005; Gimeno et al., 2014; Hatchett et al., 2017). These conditions favor the latent heat flux, with energy release taking two forms: 1) the refreezing of rain within the snowpack; and 2) condensation at the snow’s surface (DeWalle and Rango, 2008). It was 2) that drove large floods after a 1996 AR in the Pacific Northwest, with 60–90% of the energy for snowmelt attributed to condensation (Marks et al., 1998). The literature is rich with other case studies demonstrating the potency of this energy balance term e.g., Berris and Harr (1987), Rössler et al. (2014) and Garvelmann et al. (2015). Nonetheless, a key modulating factor is vegetation (i.e., Berris and Harr, 1987; Marks et al., 1998), so forest density and coverage need to be carefully considered when attributing snowmelt to the turbulent fluxes.
Another important, but less-recognized energy balance driver of ROS melt, is downwelling longwave radiation. During ROS, longwave contributes to active snowmelt via warm, moist atmospheric conditions (Garvelmann et al., 2014; Bilish et al., 2018; Li et al., 2019). Over the continental US, Li et al. (2019) demonstrated that net radiation (dominated by longwave) is the leading source of energy (68%) for ROS snowmelt in the mountainous Western US. The study is supported by Mazurkiewicz et al. (2008) who also found that ROS snowmelt was governed by longwave contributions in the Pacific Northwest—a surprise given that the turbulent fluxes were hypothesized to be a key driver.
Historically, studies have often neglected the ground heat flux due to near- or below-freezing surface temperatures (DeWalle and Rango, 2008). However, during fall and spring near-surface soil temperatures can exceed 0°C and contribute to basal layer snowmelt. Pomeroy et al. (2016) found that a late-season snowpack was primarily melted by the ground heat flux during a 2013 Canadian Rockies ROS flood. They reasoned summer ROS should be considered “distinct” from winter ROS.
Lastly, advected (sensible) heat from percolating rain into snow (a porous media) has the potential to induce melt (Kattelmann, 1997; DeWalle and Rango, 2008; Li et al., 2019). However, in British Columbia, Trubilowicz and Moore (2017) showed advected heat contributed <10% to total energy consumed for melt over 286 ROS events (10 years period). However, the calculation neglects preferential flow (Marsh and Woo, 1985; McGurk and Marsh, 1995; Singh et al., 1997; Eiriksson et al., 2013), which if present further diminishes the role of advected heat. Nonetheless, prolonged, high-intensity rainfall has potential for non-negligible advected heat, so the term should not be overlooked.
The rate of liquid water transmission through snow governs outflow timing and magnitude. Despite being studied—from theoretical (e.g., Colbeck, 1972; Colbeck, 1973; Colbeck, 1975; 1976; 1978), to experimental (e.g., Colbeck, 1974; Singh et al., 1997; Juras et al., 2017), and observational standpoints (e.g., Marsh and Woo, 1984; Conway and Benedict, 1994; McGurk and Marsh, 1995; Kattelmann and Dozier, 1999; Eiriksson et al., 2013; Rücker et al., 2019)—we have yet to fully grasp the complexities of water routing through snow. Much of the “unknown” lies in the variability of the snow’s stratigraphy and the complex exchanges of water and energy between layers.
Percolation within a porous material is driven by a fluid’s potential energy but modulated by conductance (following Darcy’s Law). In the absence of a hydraulic barrier, vertical energy gradients (i.e., gravity) induce vertical flow. Vertical flow falls into two classes of mechanisms: 1) matrix flow; and 2) preferential flow (Schneebeli, 1995; Waldner et al., 2004). Under matrix flow, an even wetting front propagates following Darcy’s law of flow—mathematically simplistic. Preferential flow, on the other hand, consists of spatially heterogeneous saturated “preferential flow paths” that exploit density differences and conveys water due to a lower porosity (Marsh and Woo, 1984; Kattelmann, 1985; McGurk and Marsh, 1995; Schneebeli, 1995). Dye tracer studies have revealed that: 1) elements of both matrix (usually at the surface), and preferential flow (usually deeper) can be present (e.g., Conway and Benedict (1994)), and that 2) preferential flow can “pool” or generate lateral flow if hydraulic barriers (either ice lenses or capillary barriers) are present (Conway and Benedict, 1994; Pfeffer and Humphrey, 1996; Singh et al., 1997; Kattelmann and Dozier, 1999; Albert and Perron, 2000; Waldner et al., 2004; Eiriksson et al., 2013; Würzer et al., 2017; Katsushima et al., 2020). Importantly, despite preferential flow only representing a small volume of the overall snowpack (between 3 and 8% as measured by McGurk and Marsh, 1995), this conveyance mechanism cannot be overlooked as it can heavily influence basin outflow response times (Eiriksson et al., 2013; Würzer et al., 2017). But, because preferential flow is predicated on fine-scale differences in density and porosity (which shift with grain metamorphism)—prediction is challenging (Colbeck, 1978; Schneebeli, 1995; Avanzi et al., 2019). Nonetheless, continued use of dye tracer studies and new techniques like near-infrared hyperspectral imagery will help address knowledge gaps (e.g., Donahue et al., 2022). Important questions include: when does preferential flow form, and how spatially heterogenous are these features?
Rainfall simulation experiments attempt to improve our understanding of water routing through the whole snowpack, although results can be difficult to compare (Conway and Benedict, 1994; Singh et al., 1997; Eiriksson et al., 2013; Juras et al., 2017). For example, Singh et al. (1997) found “conditioned” snowpacks, i.e., isothermal snowpacks with a high liquid water content (LWC; 6.8%) due to previous rainfall, generate rapid runoff through preferential flow. Nonetheless, in contrast to Singh et al. (1997); Juras et al. (2017) found that highly-stratified mid-winter snowpacks (LWC of 0.9%) generated a faster outflow response due to preferential flow when compared with three isothermal snowpacks (mean LWC of 3.7%). Despite these contrary findings, stratified snowpacks appear more likely to generate preferential flow, yielding faster outflow response times. However, the initial snow depth and LWC will act as secondary controls regulating the response times (Würzer et al. (2016). We advocate for more rainfall simulation experiments as they bolster natural ROS studies, but can be conducted on our own time, and across different snow environments (i.e., elevation, aspect and vegetation classes). While these experiments are time intensive and difficult to perform, this should not deter their usage.
Because mountain ROS environments are arguably one of the most complex encountered, parsing (and forecasting) active vs. passive snowpacks requires a full, physically-based characterization of the energy balance, as opposed to a degree day model—temperature alone does not drive ROS melt (Qi et al., 2017). With reasonable meteorological forcing data, energy balance models are capable of realistic estimates of snow state, i.e., the “bulk” cold content (Jennings et al., 2018). However, the quality of model output is largely predicated on the quality of the meteorological forcing—which can be poor even in observation-rich regions like California’s Sierra Nevada (Lundquist et al., 2019; Terzago et al., 2020). Denser, and improved station networks to capture complex meteorological drivers would greatly enhance our modeling capabilities.
While the meteorological environment during ROS is difficult to observe, arguably harder still are the exchanges of water and energy within the snowpack itself. To represent these processes, models require multi-layer snowpacks, with both Darcy and Richard’s equations for flow, and ways to incorporate preferential flow (i.e., Wever et al. (2016)). But our physical understanding of these processes and their variability is still in its infancy. Advancing modeling efforts requires more rigorous measurements, e.g., TWI from networks of lysimeters (Marsh and Woo, 1985; Kattelmann, 2000; Eiriksson et al., 2013; Webb et al., 2018b; Rücker et al., 2019), observations of stratigraphy and measurements of LWC (e.g., Koch et al. (2019), Mavrovic et al. (2020); Eole and Michel (2021), Capelli et al. (2022)). This “measurement space” needs clever, new engineering solutions to achieve a predictive understanding of ROS.
Impactful ROS floods are rare, unique, and occur during varied meteorological conditions with swings in flood driving mechanisms. The causative components for ROS floods (rainfall vs. snowmelt) can vary storm-by-storm depending on whether a snowpack is passive or active, and the routing mechanisms at play. Moving forward, we first encourage a more vigorous debate on the definition(s) of ROS—having agreed upon definitions will foster better intercomparison studies and understanding of future ROS risk. Second, we encourage funding for established and novel measurements and improved networking across systems (Hatchett et al., 2020). Specifically, we suggest: 1) improved and denser observation networks for the phase, temperature, and intensity of precipitation; and 2) the development of quickly deployable and movable instruments to measure the snow energy balance, snow cold content, and snowpack flow regimes. These new observations would help to constrain model physics with regards to passive vs. active snowpacks, and ensure extreme events are skillfully forecast for the correct physically-based reasons (Kirchner, 2006).
WB conceptualized the paper and wrote the first draft. KH, BH, and MP provided revisions. MP supervised the project.
WB drafted the review based on funds from UCAR (subaward 001987), and NOAA (NOAA-OAR-OWAQ-2019-2005820). Publication fees were paid by the UCAR subaward.
The authors declare that the research was conducted in the absence of any commercial or financial relationships that could be construed as a potential conflict of interest.
All claims expressed in this article are solely those of the authors and do not necessarily represent those of their affiliated organizations, or those of the publisher, the editors and the reviewers. Any product that may be evaluated in this article, or claim that may be made by its manufacturer, is not guaranteed or endorsed by the publisher.
The authors greatly appreciate the constructive feedback and insightful suggestions from the reviewer (CT) and from Anne Heggli.
Albert, M. R., and Perron, F. E. (2000). Ice Layer and Surface Crust Permeability in a Seasonal Snow Pack. Hydrol. Process. 14 (18), 3207–3214. doi:10.1002/1099-1085(20001230)14:18<3207::aid-hyp196>3.0.co;2-c
Avanzi, F., Johnson, R. C., Oroza, C. A., Hirashima, H., Maurer, T., and Yamaguchi, S. (2019). Insights into Preferential Flow Snowpack Runoff Using Random Forest. Water Resour. Res. 55 (12), 10727–10746. doi:10.1029/2019WR024828
Bair, E. H., Stillinger, T., and Dozier, J. (2021). Snow Property Inversion from Remote Sensing (SPIReS): a Generalized Multispectral Unmixing Approach with Examples from MODIS and Landsat 8 OLI. IEEE Trans. Geosci. Remote Sensing 59 (9), 7270–7284. doi:10.1109/TGRS.2020.3040328
Beaudry, P., and Golding, D. L. (1983). “Snowmelt during Rain-On-Snow in Coastal British Columbia,” in 51st Annual Western Snow Conference (Vancouver, Washington: Western Snow Conference).
Beniston, M., and Stoffel, M. (2016). Rain-on-Snow Events, Floods and Climate Change in the Alps: Events May Increase with Warming up to 4 °C and Decrease Thereafter. Sci. Total Environ. 571, 228–236. doi:10.1016/j.scitotenv.2016.07.146
Berg, N., Osterhuber, R., and Bergman, J. (1991). Rain-induced Outflow from Deep Snowpacks in the central Sierra Nevada, California. Hydrological Sci. J. 36 (6), 611–629. doi:10.1080/02626669109492547
Bergman, J. A. (1983). “Hydrologic Response of central Sierra Nevada Snowpacks to Rainfall,” in 51st Annual Western Snow Conference (Vancouver, Washington: Western Snow Conference).
Berris, S. N., and Harr, R. D. (1987). Comparative Snow Accumulation and Melt during Rainfall in Forested and clear-cut Plots in the Western Cascades of Oregon. Water Resour. Res. 23 (1), 135–142. doi:10.1029/WR023i001p00135
Bilish, S. P., McGowan, H. A., and Callow, J. N. (2018). Energy Balance and Snowmelt Drivers of a Marginal Subalpine Snowpack. Hydrological Process. 32 (26), 3837–3851. doi:10.1002/hyp.13293
Broxton, P. D., Harpold, A. A., Biederman, J. A., Troch, P. A., Molotch, N. P., and Brooks, P. D. (2015). Quantifying the Effects of Vegetation Structure on Snow Accumulation and Ablation in Mixed‐conifer Forests. Ecohydrol. 8 (6), 1073–1094. doi:10.1002/eco.1565
Capelli, A., Koch, F., Henkel, P., Lamm, M., Appel, F., Marty, C., et al. (2022). GNSS Signal-Based Snow Water Equivalent Determination for Different Snowpack Conditions along a Steep Elevation Gradient. Cryosphere 16 (2), 505–531. doi:10.5194/tc-16-505-2022
Cohen, J., Ye, H., and Jones, J. (2015). Trends and Variability in Rain‐on‐snow Events. Geophys. Res. Lett. 42 (17), 7115–7122. doi:10.1002/2015GL065320
Colbeck, S. C. (1972). A Theory of Water Percolation in Snow. J. Glaciol. 11 (63), 369–385. doi:10.1017/s0022143000022346
Colbeck, S. C. (1973). Effects of Stratigraphic Layers on Water Flow through Snow. Hanover, New Hampshire: Corps of Engineers, US Army Cold Regions Research and Engineering Laboratory.
Colbeck, S. C. (1974). The Capillary Effects on Water Percolation in Homogeneous Snow. J. Glaciol. 13 (67), 85–97. doi:10.3189/S002214300002339X
Colbeck, S. C. (1975). A Theory for Water Flow through a Layered Snowpack. Water Resour. Res. 11 (2), 261–266. doi:10.1029/WR011i002p00261
Colbeck, S. C. (1976). An Analysis of Water Flow in Dry Snow. Water Resour. Res. 12 (3), 523–527. doi:10.1029/WR012i003p00523
Colbeck, S. C. (1978). The Difficulties of Measuring the Water Saturation and Porosity of Snow. J. Glaciol. 20 (82), 189–201. doi:10.3189/S0022143000198089
Conway, H., and Benedict, R. (1994). Infiltration of Water into Snow. Water Resour. Res. 30 (3), 641–649. doi:10.1029/93WR03247
Corripio, J., and López-Moreno, J. (2017). Analysis and Predictability of the Hydrological Response of Mountain Catchments to Heavy Rain on Snow Events: a Case Study in the Spanish Pyrenees. Hydrology 4 (2), 20. doi:10.3390/hydrology4020020
DeGraff, J. V. (1994). “The Geomorphology of Some Debris Flows in the Southern Sierra Nevada, California,” in Geomorphology and Natural Hazards. Editor M. Morisawa (Amsterdam: Elsevier), 231–252. doi:10.1016/b978-0-444-82012-9.50020-7
DeWalle, D. R., and Rango, A. (2008). Principles of Snow Hydrology. New York, NY: Cambridge University Press.
Donahue, C., Skiles, S. M., and Hammonds, K. (2022). Mapping Liquid Water Content in Snow at the Millimeter Scale: an Intercomparison of Mixed-phase Optical Property Models Using Hyperspectral Imaging and In Situ Measurements. Cryosphere 16 (1), 43–59. doi:10.5194/tc-16-43-2022
Dudley, R. W., Hodgkins, G. A., McHale, M. R., Kolian, M. J., and Renard, B. (2017). Trends in Snowmelt-Related Streamflow Timing in the Conterminous United States. J. Hydrol. 547, 208–221. doi:10.1016/j.jhydrol.2017.01.051
Dunne, T., and Black, R. D. (1971). Runoff Processes during Snowmelt. Water Resour. Res. 7 (5), 1160–1172. doi:10.1029/WR007i005p01160
Eiriksson, D., Whitson, M., Luce, C. H., Marshall, H. P., Bradford, J., Benner, S. G., et al. (2013). An Evaluation of the Hydrologic Relevance of Lateral Flow in Snow at Hillslope and Catchment Scales. Hydrol. Process. 27 (5), 640–654. doi:10.1002/hyp.9666
Eole, V., and Michel, B. (2021). “Impact of the Spatial and Temporal Variability of Snowpack Condition on Internal Liquid Water Fluxes,” in 88th Annual Western Snow Conference, Bozeman, MT.
Freudiger, D., Kohn, I., Stahl, K., and Weiler, M. (2014). Large-scale Analysis of Changing Frequencies of Rain-On-Snow Events with Flood-Generation Potential. Hydrol. Earth Syst. Sci. 18 (7), 2695–2709. doi:10.5194/hess-18-2695-2014
Garvelmann, J., Pohl, S., and Weiler, M. (2014). Variability of Observed Energy Fluxes during Rain-On-Snow and clear Sky Snowmelt in a Midlatitude Mountain Environment. J. Hydrometeorol. 15 (3), 1220–1237. doi:10.1175/jhm-d-13-0187.1
Garvelmann, J., Pohl, S., and Weiler, M. (2015). Spatio-temporal Controls of Snowmelt and Runoff Generation during Rain-On-Snow Events in a Mid-latitude Mountain Catchment. Hydrol. Process. 29 (17), 3649–3664. doi:10.1002/hyp.10460
Gimeno, L., Nieto, R., Vã¡zquez, M., and Lavers, D. A. (2014). Atmospheric Rivers: a Mini-Review. Front. Earth Sci. 2 (2), 1–6. doi:10.3389/feart.2014.00002
Guan, B., Waliser, D. E., Ralph, F. M., Fetzer, E. J., and Neiman, P. J. (2016). Hydrometeorological Characteristics of Rain‐on‐snow Events Associated with Atmospheric Rivers. Geophys. Res. Lett. 43 (6), 2964–2973. doi:10.1002/2016GL067978
Haleakala, K., Gebremichael, M., Dozier, J., and Lettenmaier, D. P. (2021). Factors Governing Winter Snow Accumulation and Ablation Susceptibility across the Sierra Nevada, U.S.A. J. Hydrometeorol. 22 (6), 1455–1472. doi:10.1175/jhm-d-20-0257.1
Harpold, A. A., Kaplan, M. L., Klos, P. Z., Link, T., McNamara, J. P., Rajagopal, S., et al. (2017). Rain or Snow: Hydrologic Processes, Observations, Prediction, and Research Needs. Hydrol. Earth Syst. Sci. 21 (1), 1–22. doi:10.5194/hess-21-1-2017
Harr, R. D. (1981). Some Characteristics and Consequences of Snowmelt during Rainfall in Western Oregon. J. Hydrol. 53 (3), 277–304. doi:10.1016/0022-1694(81)90006-8
Hatchett, B. J., Kaplan, M. L., and Burak, S. (2016). “Some Characteristics of Upside-Down Storms in the Northern Sierra Nevada, California-Nevada, USA,” in Proceedings of the International Snow Science Workshop, Breckenridge, CO.
Hatchett, B. J., Burak, S., Rutz, J. J., Oakley, N. S., Bair, E. H., and Kaplan, M. L. (2017). Avalanche Fatalities during Atmospheric River Events in the Western United States. J. Hydrometeorol. 18 (5), 1359–1374. doi:10.1175/jhm-d-16-0219.1
Hatchett, B. J., Cao, Q., Dawson, P. B., Ellis, C. J., Hecht, C. W., Kawzenuk, B., et al. (2020). Observations of an Extreme Atmospheric River Storm with a Diverse Sensor Network. Earth Space Sci. 7 (8), e2020EA001129. doi:10.1029/2020EA001129
Henn, B., Musselman, K. N., Lestak, L., Ralph, F. M., and Molotch, N. P. (2020). Extreme Runoff Generation from Atmospheric River Driven Snowmelt during the 2017 Oroville Dam Spillways Incident. Geophys. Res. Lett. 47 (14), e2020GL088189. doi:10.1029/2020GL088189
Hock, R., Rasul, G., Alder, C., Cáceres, B., Gruber, S., Hirabayashi, Y., et al. (2019). “High Mountain Areas,” in IPCC Special Report on the Ocean and Cryosphere in a Changing Climate. Editors D.C.R. H.-O. Pörtner, V. Masson-Delmotte, P. Zhai, M. Tignor, E. Poloczanska, K. Mintenbecket al.
Houze, R. A. (2012). Orographic Effects on Precipitating Clouds. Rev. Geophys. 50 (1). doi:10.1029/2011RG000365
Huang, H., Fischella, M. R., Liu, Y., Ban, Z., Fayne, J. V., Li, D., et al. (2022). Changes in Mechanisms and Characteristics of Western U.S. Floods over the Last Sixty Years. Geophys. Res. Lett. 49 (3), e2021GL097022. doi:10.1029/2021GL097022
Huss, M., Bookhagen, B., Huggel, C., Jacobsen, D., Bradley, R. S., Clague, J. J., et al. (2017). Toward Mountains without Permanent Snow and Ice. Earth's Future 5 (5), 418–435. doi:10.1002/2016EF000514
Immerzeel, W. W., Lutz, A. F., Andrade, M., Bahl, A., Biemans, H., Bolch, T., et al. (2020). Importance and Vulnerability of the World's Water Towers. Nature 577 (7790), 364–369. doi:10.1038/s41586-019-1822-y
Jennings, K. S., Kittel, T. G. F., and Molotch, N. P. (2018). Observations and Simulations of the Seasonal Evolution of Snowpack Cold Content and its Relation to Snowmelt and the Snowpack Energy Budget. Cryosphere 12 (5), 1595–1614. doi:10.5194/tc-12-1595-2018
Juras, R., Würzer, S., Pavlásek, J., Vitvar, T., and Jonas, T. (2017). Rainwater Propagation through Snowpack during Rain-On-Snow Sprinkling Experiments under Different Snow Conditions. Hydrol. Earth Syst. Sci. 21 (9), 4973–4987. doi:10.5194/hess-21-4973-2017
Juras, R., Blöcher, J. R., Jenicek, M., Hotovy, O., and Markonis, Y. (2021). What Affects the Hydrological Response of Rain-On-Snow Events in Low-Altitude Mountain Ranges in Central Europe? J. Hydrol. 603, 127002. doi:10.1016/j.jhydrol.2021.127002
Kaplan, M. L., Vellore, R. K., Marzette, P. J., and Lewis, J. M. (2012). The Role of Windward-Side Diabatic Heating in Sierra Nevada Spillover Precipitation. J. Hydrometeorol. 13 (4), 1172–1194. doi:10.1175/jhm-d-11-06.1
Katsushima, T., Adachi, S., Yamaguchi, S., Ozeki, T., and Kumakura, T. (2020). Nondestructive Three-Dimensional Observations of Flow finger and Lateral Flow Development in Dry Snow Using Magnetic Resonance Imaging. Cold Regions Sci. Technol. 170, 102956. doi:10.1016/j.coldregions.2019.102956
Kattelmann, R., and Dozier, J. (1999). Observations of Snowpack Ripening in the Sierra Nevada, California, U.S.A. J. Glaciol. 45 (151), 409–416. doi:10.3189/S002214300000126X
Kattelmann, R. (1985). Macropores in Snowpacks of Sierra Nevada. A. Glaciology. 6, 272–273. doi:10.1017/s0260305500010594
Kattelmann, R. (1987). Water Release from a Forested Snowpack during Rainfall. For. Hydrol. Watershed Manage. 167, 265–272.
Kattelmann, R. (1989). Spatial Variability of Snow-Pack Outflow at a Site in Sierra Nevada, U.S.A. A. Glaciology. 13, 124–128. doi:10.3189/S026030550000775810.1017/s0260305500007758
Kattelmann, R. (1997). Flooding from Rain-On-Snow Events in the Sierra Nevada. IAHS Publ.-series Proc. Reports-Intern Assoc Hydrol. Sci. 239, 59–66.
Kattelmann, R. (2000). Snowmelt Lysimeters in the Evaluation of Snowmelt Models. Ann. Glaciol. 31, 405–410. doi:10.3189/172756400781820048
Kirchner, J. W. (2006). Getting the Right Answers for the Right Reasons: Linking Measurements, Analyses, and Models to advance the Science of Hydrology. Water Resour. Res. 42 (3), W03S04. doi:10.1029/2005WR004362
Koch, F., Henkel, P., Appel, F., Schmid, L., Bach, H., Lamm, M., et al. (2019). Retrieval of Snow Water Equivalent, Liquid Water Content, and Snow Height of Dry and Wet Snow by Combining GPS Signal Attenuation and Time Delay. Water Resour. Res. 55 (5), 4465–4487. doi:10.1029/2018WR024431
Kraaijenbrink, P. D. A., Stigter, E. E., Yao, T., and Immerzeel, W. W. (2021). Climate Change Decisive for Asia's Snow Meltwater Supply. Nat. Clim. Chang. 11 (7), 591–597. doi:10.1038/s41558-021-01074-x
Li, D., Lettenmaier, D. P., Margulis, S. A., and Andreadis, K. (2019). The Role of Rain‐on‐Snow in Flooding over the Conterminous United States. Water Resour. Res. 55 (11), 8492–8513. doi:10.1029/2019WR024950
López-Moreno, J. I., Pomeroy, J. W., Morán-Tejeda, E., Revuelto, J., Navarro-Serrano, F. M., Vidaller, I., et al. (2021). Changes in the Frequency of Global High Mountain Rain-On-Snow Events Due to Climate Warming. Environ. Res. Lett. 16 (9), 094021. doi:10.1088/1748-9326/ac0dde
Lundquist, J. D., Dickerson-Lange, S. E., Lutz, J. A., and Cristea, N. C. (2013). Lower forest Density Enhances Snow Retention in Regions with Warmer winters: A Global Framework Developed from Plot-Scale Observations and Modeling. Water Resour. Res. 49 (10), 6356–6370. doi:10.1002/wrcr.20504
Lundquist, J., Hughes, M., Gutmann, E., and Kapnick, S. (2019). Our Skill in Modeling Mountain Rain and Snow Is Bypassing the Skill of Our Observational Networks. Bull. Am. Meteorol. Soc. 100 (12), 2473–2490. doi:10.1175/bams-d-19-0001.1
Lynn, E., Cuthbertson, A., He, M., Vasquez, J. P., Anderson, M. L., Coombe, P., et al. (2020). Technical Note: Precipitation-phase Partitioning at Landscape Scales to Regional Scales. Hydrol. Earth Syst. Sci. 24 (11), 5317–5328. doi:10.5194/hess-24-5317-2020
Marks, D., Kimball, J., Tingey, D., and Link, T. (1998). The Sensitivity of Snowmelt Processes to Climate Conditions and forest Cover during Rain-On-Snow: a Case Study of the 1996 Pacific Northwest Flood. Hydrol. Process. 12 (10-11), 1569–1587. doi:10.1002/(sici)1099-1085(199808/09)12:10/11<1569::aid-hyp682>3.0.co;2-l
Marsh, P., and Woo, M.-K. (1984). Wetting Front advance and Freezing of Meltwater within a Snow Cover: 1. Observations in the Canadian Arctic. Water Resour. Res. 20 (12), 1853–1864. doi:10.1029/WR020i012p01853
Marsh, P., and Woo, M.-K. (1985). Meltwater Movement in Natural Heterogeneous Snow Covers. Water Resour. Res. 21 (11), 1710–1716. doi:10.1029/WR021i011p01710
Mateo-Lázaro, J., Castillo-Mateo, J., Sánchez-Navarro, J., Fuertes-Rodríguez, V., García-Gil, A., and Edo-Romero, V. (2019). Assessment of the Role of Snowmelt in a Flood Event in a Gauged Catchment. Water 11 (3), 506. doi:10.3390/w11030506
Mavrovic, A., Madore, J.-B., Langlois, A., Royer, A., and Roy, A. (2020). Snow Liquid Water Content Measurement Using an Open-Ended Coaxial Probe (OECP). Cold Regions Sci. Technol. 171, 102958. doi:10.1016/j.coldregions.2019.102958
Mazurkiewicz, A. B., Callery, D. G., and McDonnell, J. J. (2008). Assessing the Controls of the Snow Energy Balance and Water Available for Runoff in a Rain-On-Snow Environment. J. Hydrol. 354 (1), 1–14. doi:10.1016/j.jhydrol.2007.12.027
McCabe, G. J., Clark, M. P., and Hay, L. E. (2007). Rain-on-snow Events in the Western United States. Bull. Am. Meteorol. Soc. 88 (3), 319–328. doi:10.1175/BAMS-88-3-319
McGurk, B. J., and Marsh, P. (1995). “Flow-finger Continuity in Serial Thick-Sections in a Melting Sierran Snowpack,” in Biogeochemistry of Seasonally Snow-Covered Catchments. Editors K.A. Tonnessen, M.W. Williams, and M. Tranter (Wallingford: International Association of Hydrological Sciences), 81–88.
Merz, R., and Blöschl, G. (2003). A Process Typology of Regional Floods. Water Resour. Res. 39 (12). doi:10.1029/2002WR001952
Moore, R. D., and Prowse, T. D. (1988). Snow Hydrology of the Waimakariri Catchmet, South Island, New Zealand. J. Hydrol. (New Zealand) 27 (1), 44–68.
Mote, P. W., Li, S., Lettenmaier, D. P., Xiao, M., and Engel, R. (2018). Dramatic Declines in Snowpack in the Western US. Npj Clim. Atmos. Sci. 1 (1), 2. doi:10.1038/s41612-018-0012-1
Musselman, K. N., Lehner, F., Ikeda, K., Clark, M. P., Prein, A. F., Liu, C., et al. (2018). Projected Increases and Shifts in Rain-On-Snow Flood Risk over Western North America. Nat. Clim Change 8 (9), 808–812. doi:10.1038/s41558-018-0236-4
Petersky, R., and Harpold, A. (2018). Now You See it, Now You Don't: a Case Study of Ephemeral Snowpacks and Soil Moisture Response in the Great Basin, USA. Hydrol. Earth Syst. Sci. 22 (9), 4891–4906. doi:10.5194/hess-22-4891-2018
Pfeffer, W. T., and Humphrey, N. F. (1996). Determination of Timing and Location of Water Movement and Ice-Layer Formation by Temperature Measurements in Sub-freezing Snow. J. Glaciol. 42 (141), 292–304. doi:10.1017/s0022143000004159
Pomeroy, J. W., Fang, X., and Marks, D. G. (2016). The Cold Rain-On-Snow Event of June 2013 in the Canadian Rockies - Characteristics and Diagnosis. Hydrol. Process. 30 (17), 2899–2914. doi:10.1002/hyp.10905
Qi, J., Li, S., Jamieson, R., Hebb, D., Xing, Z., and Meng, F.-R. (2017). Modifying SWAT with an Energy Balance Module to Simulate Snowmelt for Maritime Regions. Environ. Model. Softw. 93, 146–160. doi:10.1016/j.envsoft.2017.03.007
Ralph, F. M., Neiman, P. J., and Wick, G. A. (2004). Satellite and CALJET Aircraft Observations of Atmospheric Rivers over the Eastern north Pacific Ocean during the winter of 1997/98. Mon. Wea. Rev. 132 (7), 1721–1745. doi:10.1175/1520-0493(2004)132<1721:sacaoo>2.0.co;2
Ralph, F. M., Neiman, P. J., and Rotunno, R. (2005). Dropsonde Observations in Low-Level Jets over the Northeastern Pacific Ocean from CALJET-1998 and PACJET-2001: Mean Vertical-Profile and Atmospheric-River Characteristics. Mon. Wea. Rev. 133 (4), 889–910. doi:10.1175/mwr2896.1
Ralph, F. M., Dettinger, M. D., RutzDuane, J. J., and Waliser, E. (2020). Atmospheric Rivers. Cham, Switzerland: Springer.
Rössler, O., Froidevaux, P., Börst, U., Rickli, R., Martius, O., and Weingartner, R. (2014). Retrospective Analysis of a Nonforecasted Rain-On-Snow Flood in the Alps - a Matter of Model Limitations or Unpredictable Nature? Hydrol. Earth Syst. Sci. 18 (6), 2265–2285. doi:10.5194/hess-18-2265-2014
Rücker, A., Boss, S., Kirchner, J. W., and von Freyberg, J. (2019). Monitoring Snowpack Outflow Volumes and Their Isotopic Composition to Better Understand Streamflow Generation during Rain-On-Snow Events. Hydrol. Earth Syst. Sci. 23 (7), 2983–3005. doi:10.5194/hess-23-2983-2019
Schneebeli, M. (1995). Development and Stability of Preferential Flow Paths in a Layered Snowpack, 228. IAHS Publ.
Siirila-Woodburn, E. R., Rhoades, A. M., Hatchett, B. J., Huning, L. S., Szinai, J., Tague, C., et al. (2021). A Low-To-No Snow Future and its Impacts on Water Resources in the Western United States. Nat. Rev. Earth Environ. 2 (11), 800–819. doi:10.1038/s43017-021-00219-y
Singh, P., Spitzbart, G., Hübl, H., and Weinmeister, H. W. (1997). Hydrological Response of Snowpack under Rain-On-Snow Events: a Field Study. J. Hydrol. 202 (1), 1–20. doi:10.1016/S0022-1694(97)00004-8
Skiles, S. M., Flanner, M., Cook, J. M., Dumont, M., and Painter, T. H. (2018). Radiative Forcing by Light-Absorbing Particles in Snow. Nat. Clim Change 8 (11), 964–971. doi:10.1038/s41558-018-0296-5
Stevens, J. T. (2017). Scale-dependent Effects of post-fire Canopy Cover on Snowpack Depth in Montane Coniferous Forests. Ecol. Appl. 27 (6), 1888–1900. doi:10.1002/eap.1575
Stimberis, J., and Rubin, C. M. (2011). Glide Avalanche Response to an Extreme Rain-On-Snow Event, Snoqualmie Pass, Washington, USA. J. Glaciol. 57, 468–474. doi:10.3189/002214311796905686
Storck, P., Lettenmaier, D. P., and Bolton, S. M. (2002). Measurement of Snow Interception and Canopy Effects on Snow Accumulation and Melt in a Mountainous Maritime Climate, Oregon, United States. Water Resour. Res. 38 (11), 5–1516. doi:10.1029/2002WR001281
Sturm, M., Goldstein, M. A., and Parr, C. (2017). Water and Life from Snow: A Trillion Dollar Science Question. Water Resour. Res. 53 (5), 3534–3544. doi:10.1002/2017WR020840
Surfleet, C. G., and Tullos, D. (2013). Variability in Effect of Climate Change on Rain-On-Snow Peak Flow Events in a Temperate Climate. J. Hydrol. 479, 24–34. doi:10.1016/j.jhydrol.2012.11.021
Tarasova, L., Merz, R., Kiss, A., Basso, S., Blöschl, G., Merz, B., et al. (2019). Causative Classification of River Flood Events. Wires. Water 6 (4), e1353. doi:10.1002/wat2.1353
Terzago, S., Andreoli, V., Arduini, G., Balsamo, G., Campo, L., Cassardo, C., et al. (2020). Sensitivity of Snow Models to the Accuracy of Meteorological Forcings in Mountain Environments. Hydrol. Earth Syst. Sci. 24 (8), 4061–4090. doi:10.5194/hess-24-4061-2020
Trubilowicz, J. W., and Moore, R. D. (2017). Quantifying the Role of the Snowpack in Generating Water Available for Run-Off during Rain-On-Snow Events from Snow Pillow Records. Hydrol. Process. 31 (23), 4136–4150. doi:10.1002/hyp.11310
Viviroli, D., Dürr, H. H., Messerli, B., Meybeck, M., and Weingartner, R. (2007). Mountains of the World, Water Towers for Humanity: Typology, Mapping, and Global Significance. Water Resour. Res. 43 (7). doi:10.1029/2006WR005653
Waldner, P. A., Schneebeli, M., Schultze-Zimmermann, U., and Flühler, H. (2004). Effect of Snow Structure on Water Flow and Solute Transport. Hydrol. Process. 18 (7), 1271–1290. doi:10.1002/hyp.1401
Wayand, N. E., Lundquist, J. D., and Clark, M. P. (2015). Modeling the Influence of Hypsometry, Vegetation, and Storm Energy on Snowmelt Contributions to Basins during Rain‐on‐snow Floods. Water Resour. Res. 51 (10), 8551–8569. doi:10.1002/2014WR016576
Webb, R. W., Fassnacht, S. R., Gooseff, M. N., and Webb, S. W. (2018a). The Presence of Hydraulic Barriers in Layered Snowpacks: TOUGH2 Simulations and Estimated Diversion Lengths. Transp Porous Med. 123 (3), 457–476. doi:10.1007/s11242-018-1079-1
Webb, R. W., Williams, M. W., and Erickson, T. A. (2018b). The Spatial and Temporal Variability of Meltwater Flow Paths: Insights from a Grid of over 100 Snow Lysimeters. Water Resour. Res. 54 (2), 1146–1160. doi:10.1002/2017WR020866
Wever, N., Würzer, S., Fierz, C., and Lehning, M. (2016). Simulating Ice Layer Formation under the Presence of Preferential Flow in Layered Snowpacks. Cryosphere 10 (6), 2731–2744. doi:10.5194/tc-10-2731-2016
Würzer, S., Jonas, T., Wever, N., and Lehning, M. (2016). Influence of Initial Snowpack Properties on Runoff Formation during Rain-On-Snow Events. J. Hydrometeorol. 17 (6), 1801–1815. doi:10.1175/jhm-d-15-0181.1
Würzer, S., Wever, N., Juras, R., Lehning, M., and Jonas, T. (2017). Modelling Liquid Water Transport in Snow under Rain-On-Snow Conditions - Considering Preferential Flow. Hydrol. Earth Syst. Sci. 21 (3), 1741–1756. doi:10.5194/hess-21-1741-2017
Keywords: rain-on-snow, snowmelt, terrestrial water input, snow energy balance, hydraulic conductivity
Citation: Brandt WT, Haleakala K, Hatchett BJ and Pan M (2022) A Review of the Hydrologic Response Mechanisms During Mountain Rain-on-Snow. Front. Earth Sci. 10:791760. doi: 10.3389/feart.2022.791760
Received: 08 October 2021; Accepted: 14 March 2022;
Published: 26 April 2022.
Edited by:
Anna-Kaisa Ronkanen, Finnish Environment Institute (SYKE), FinlandReviewed by:
Christina (Naomi) Tague, University of California, Santa Barbara, United StatesCopyright © 2022 Brandt, Haleakala, Hatchett and Pan. This is an open-access article distributed under the terms of the Creative Commons Attribution License (CC BY). The use, distribution or reproduction in other forums is permitted, provided the original author(s) and the copyright owner(s) are credited and that the original publication in this journal is cited, in accordance with accepted academic practice. No use, distribution or reproduction is permitted which does not comply with these terms.
*Correspondence: W. Tyler Brandt, d2JyYW5kdEB1Y3NkLmVkdQ==
Disclaimer: All claims expressed in this article are solely those of the authors and do not necessarily represent those of their affiliated organizations, or those of the publisher, the editors and the reviewers. Any product that may be evaluated in this article or claim that may be made by its manufacturer is not guaranteed or endorsed by the publisher.
Research integrity at Frontiers
Learn more about the work of our research integrity team to safeguard the quality of each article we publish.