- 1Ocean Modeling and Data Assimilation Division, Centro Euro-Mediterraneo Sui Cambiamenti Climatici, Bologna, Italy
- 2Department of Physics and Astronomy, University of Bologna, Bologna, Italy
Global ocean reanalyses provide consistent and comprehensive records of ocean and sea ice variables and are therefore of pivotal significance for climate studies, particularly in data-sparse regions such as Antarctica. Here, for the first time, we present the temporal and spatial variability of sea ice area in the ensemble of global ocean reanalyses produced by the Copernicus Marine Environment Monitoring Service (CMEMS) for the period 1993–2019. The reanalysis ensemble robustly reproduces observed interannual and seasonal variability, linear trend, as well as record highs and lows. While no consensus has been reached yet on the physical source of Antarctic-wide ice changes, our study also emphasizes the importance of understanding the different responses of ice classes, marginal ice zone (MIZ) and pack ice, to climate changes. Modifications of the distribution of MIZ and pack ice have implications for the level of air/sea exchanges and for the marine ecosystem. Analysis of the spatial and temporal variability of ice classes can provide further insights on long-term trends and help to improve predictions of future changes in Antarctic sea ice. We assess the ability of the reanalysis ensemble to adequately capture variability in space and time of the MIZ and pack ice area, and conclude that it can provide consistent estimates of recent changes in the Antarctic sea ice area. Our results show that the Antarctic sea ice area agrees well with satellite estimates, and the hemispheric and regional sea ice area variability are properly reproduced on seasonal and interannual time scales. Although the ensemble reanalysis product tends to slightly overestimate MIZ in summer, results show that it properly represents the variability of MIZ minima and maxima as well as its interannual variability during the growing and melting seasons. Our results confirm that Global Reanalysis Ensemble Product is able to reproduce the observed substantial regional variability, in regions covered by marginal ice.
Introduction
Antarctic sea ice plays a critical role in the polar and global climate and ecosystems, modulating the exchanges of momentum, gases and heat between the ocean and the atmosphere. A deep knowledge of sea ice variability is necessary for adequately simulating these fluxes and thus for climate modelling. In stark contrast to the Arctic, where sea ice has declined significantly in all areas and seasons (e.g., Parkinson and Cavalieri, 2012; Serreze and Stroeve 2015; Onarheim et al., 2018), Antarctic sea ice has not experienced a drastic and continuous decline during recent decades. Satellite records show a slight increasing trend in total annual-mean Antarctic sea ice extent (SIE) at a rate of ∼1.5% per decade for the 1979–2015 period, with modest increases in the maxima and minima (Turner et al., 2015; Comiso et al., 2017), albeit individual regions experienced much larger gains and losses that almost offset each other overall (Parkinson, 2019). After record maxima successively occurred in 2012, 2013, and 2014, Antarctic sea ice decreased below the long-term average in 2015, with unprecedented record low minima in 2016, 2017 and 2018 (Parkinson, 2019). However, the recent decrease does not signify a change in the sign of the long-term trend, which remains positive over the period 1979–2019, though with lower magnitude compared to the 1979–2015 trend (Wachter et al., 2021).
Understanding this quasi-stable situation in Antarctic sea ice and its link to climate change is still a significant scientific challenge (Kennicutt et al., 2015). Rather than by a single mechanism, the long-term sea ice variability is driven by a combination of processes, such as local changes in the atmospheric dynamics and wind patterns (e.g., Holland and Kwok, 2012; Meehl et al., 2016; Vichi et al., 2019; Blanchard-Wrigglesworth et al., 2021), shifts in the dominant modes of large-scale atmospheric circulation in the southern hemisphere (Stammerjohn et al., 2008), changes in the vertical structure of the near-surface water column (Goosse and Zunz, 2014; Venables and Meredith, 2014), changes in ice albedo feedback (Riihelä et al., 2021), ice-ocean feedbacks (Goosse and Zunz, 2014; Frew et al., 2019), and variability of the ice sheet water discharge (Bintanja et al., 2013; Haid et al., 2017; Pauling et al., 2017). These processes combine in different ways at regional scales. Significant regional contrasts and variability are nested within the Antarctic-wide changes: while the Ross and Weddell Seas dominate the overall upward trend, the Amundsen-Bellingshausen Seas have undergone a considerable decrease (Massom and Stammerjohn, 2010; Parkinson, 2019). High-magnitude seasonal variability is also disguised in long-term expansion of total sea ice cover: a complex seasonal pattern of trends emerges across the regions, with positive expansion trend in one season and negative in another (Holland, 2014; Hobbs et al., 2016; Parkinson, 2019). Considering the spatial and seasonal heterogeneity of trends, the Antarctic-wide changes could not aid in the attribution of those trends. The focus instead should be on the regional and seasonal variability which may give a better understanding of the long-term changes in Antarctic sea ice area.
While changes in total sea ice at different spatial/temporal scales remain puzzling, it is likely that these changes also affect the distribution and variability of ice classes in different ways (Stroeve et al., 2016; Iovino et al., 2022). Here, we define ice classes to distinguish between consolidated pack ice and the marginal ice zone (MIZ). Understanding how the spatial patterns of different ice classes change may help to elucidate the mechanisms contributing to the expansion of Antarctic ice in some regions and contraction in others (Maksym et al., 2012). In spite of the large winter cover, sea ice around Antarctica forms a vast field of small broken ice floes, with compact and consolidated ice remaining all year around only in a few coastal regions (e.g., Holland et al., 2014). The MIZ is highly dynamic and its response to climate variability differs from the inner pack ice: it undergoes faster melting due to a larger lateral melt rate (Tsamados et al., 2015), responds more easily to winds and current forcing (Manucharyan and Thompson, 2017; Alberello et al., 2020), and is highly vulnerable to waves and swell (Kohout et al., 2014). The MIZ is fundamental for climate dynamics and polar ecosystems, given its roles as a region of intense atmosphere-sea ice interactions and as a physical buffer between the consolidated pack ice zone and the effects of open ocean dynamics (e.g., Squire 2007). Monitoring changes of the MIZ environment can help us understand the associated changes in the climate system. An accurate assessment of Antarctic MIZ variability is still missing, as well as a deep insight into how ice conditions correlate with atmospheric fields and surface oceanic waves (Meylan et al., 2014, Sutherland and Balmforth 2019). The MIZ can be operationally defined through sea ice concentration (SIC) thresholds as the transitional region between open water and consolidated pack ice, where the ocean is covered by SIC between 15 and 80% (e.g., Pauling et al., 2017).
There is growing demand for comprehensive records of the historical ocean state. Ocean Reanalyses (ORAs) represent an essential tool to monitor long-term variability of various climate indices, especially in areas with sparse data such as the Antarctic Ocean. Observations alone can not reasonably reproduce consistent and homogeneous time series of three-dimensional gridded fields of ocean and ice parameters. Model simulations, on other hand, can provide somewhat accurate information regarding the ocean and ice mean states and variability, despite being prone to errors related to model formulation, initialization and forcing. A number of experiments with global ocean-sea ice models were carried out in the framework of the Coordinated Ocean-ice Reference Experiments (CORE-II) and the Ocean Model Intercomparison Project (OMIP), albeit with little focus on sea ice performance in polar regions (e.g., Downes et al., 2015; Farneti et al., 2015; Tsujino et al., 2020). Most CORE-II models are found to underestimate Antarctic SIC in summer and reproduce the sea ice edge further south compared to observations (Downes et al., 2015). The OMIP simulations reproduce a very wide range of models spread in sea ice concentration and volume, with ratios of the maximum to the minimum reaching a factor of two to three (Tsujino et al., 2020). Inaccurate representation of sea ice and a large spread across model output is due to the fact that these model systems are not constrained by observations through data assimilation schemes. The advantage of ORAs with respect to observation-only products and ocean models, is the combination of ocean/sea ice models and observational data sets driven by atmospheric forcing. The errors from models and forcing datasets are reduced through assimilation of observations. Ocean reanalyses are a fundamental tool for climate investigation, as indicated by the large number of studies that make use of them. Within the Ocean Reanalyses Intercomparison Project (Balmaseda et al., 2015), several exercises were undertaken to study the variability of many well-constrained ocean fields, such as steric sea level (Storto et al., 2017), air-sea heat fluxes (Valdivieso et al., 2017), ocean heat content (Palmer et al., 2017). ORAs are also a key tool for evaluating key climate diagnostics that are not directly observed, such as deep ocean warming (Balmaseda et al., 2013), the reconstruction of the overturning circulation (Jackson et al., 2016). Few ORAs studies so far have focused on their performance in polar regions. Chevallier et al. (2017) used 14 global ORAs to analyze the seasonal variability of the sea ice area and sea ice edge position in the Arctic region. They showed that the ensemble-mean SIC agrees quite well with the observations but there is significant disagreement among systems in simulated sea ice thickness (which is not directly assimilated in any of the ORAs). However, they also revealed a large spread in the representation of pack ice and the MIZ extent. Using a set of 10 ORAs, Uotila et al. (2019) found an overall agreement with observations in the location of both Arctic and Antarctic sea ice edges, and showed that ORAs are able to capture seasonal variability of sea ice area (SIA). The large differences in the 10 reanalysis systems resulted in a poor representation of the seasonal variability of the MIZ and pack ice area. Nevertheless, Uotila et al. (2019) discussed the fidelity of ensemble mean estimates and proved that the multi-system concept provides the most robust results owing to the cancellation of the individual errors.
In this study, we investigate the interannual and seasonal changes of Antarctic SIA on hemispheric and regional scales with the purpose of identifying the differences between MIZ and total/consolidated pack ice. We use an ensemble-mean of four global ocean-sea ice reanalyses (ORAs) together with long-term passive microwave sea ice estimates. We examine the quality of the Global ocean Reanalysis Ensemble Product (version 2, hereafter called GREP) provided by the Copernicus Marine Environment Monitoring Service (CMEMS) of the European Union. GREP is an ensemble of four global ocean-sea ice reanalyses produced at eddy-permitting resolution for the period from 1993 to present. GREP has already been successfully validated with respect to a range of ocean variables (Masina et al., 2015; Storto et al., 2019) and have been largely adopted for evaluating key climate diagnostics that are not easily observed. In this study, we evaluate the capability of GREP in reproducing the Antarctic sea ice area in the marginal ice and pack ice regions, in the 1993–2019 period. We analyse the interannual and seasonal variability in five sectors of the Antarctic Ocean. The main objectives of this work are to validate GREP Antarctic SIA against satellite estimates and to investigate the benefits of a multi-system ensemble approach. Since the multi-model mean can offset systematic errors of individual systems, we expect GREP to perform generally better than single reanalysis and provide the most consistent estimates of sea ice state and variability. We also intend to encourage the use of GREP in a wide range of applications.
Data and Methods
The Global Reanalysis Ensemble Product (GREP version 2) consists of four global ocean-sea ice reanalyses (C-GLORSv7, Storto et al., 2016; FOAM-GloSea5, MacLachlan et al., 2015; GLORYS2v4, Lellouche et al., 2013; ORAS5, Zuo et al., 2019), all constrained by satellite and in-situ observations, and driven by the ECMWF ERA-Interim atmospheric reanalysis (Dee et al., 2011). Monthly means of ocean and sea ice variables, for individual reanalysis as well as the ensemble mean and spread, are produced and freely disseminated by CMEMS through the CMEMS catalogue (product reference GLOBAL_REANALYSIS_PHY_001_031).
The four reanalyses share the ocean components of the state-of-the-art NEMO model, and are produced on the same tripolar ORCA025 grid at an eddy-permitting resolution (approximately ¼ degree of horizontal resolution and 75 depth levels). Three reanalyses use the LIM2 thermodynamic-dynamic sea-ice model, while the other (FOAM-GloSea5) employs CICE4.1 which includes more complex physics parameterizations compared to LIM2. Although many physical and numerical schemes are similar in the four reanalyses, there are a number of significant changes including the ocean model version and some parameterizations, thus introducing differences in the four ocean model configurations. There are also differences in the data assimilation methods used by the single products, in terms of data assimilation scheme, code, frequency of analysis and assimilation time-windows, input observational data-sets, error definitions and bias correction schemes, which introduce a large number of uncertainties as ensemble spread. The main characteristics of the GREP members are summarized in Table 1 – a detailed description of model setup and data assimilation methods is outside of the scope of this study. GREP and its constituent reanalyses cover the altimetric period from 1993. Our analysis extends to 2019.
We consider a set of sea ice satellite products in order to evaluate the GREP performance. We use SIC fields from NOAA/NSIDC Climate Data Record (version 3, Meier et al., 2017, hereafter CDR), EUMETSAT OSISAF Climate Data Record and Interim Climate Data Record (release 2, products OSI-450 and OSI-430-b, Lavergne et al., 2019), and IFREMER/CERSAT (Ezraty et al., 2007). Firstly, the CDR algorithm output is a combination of SIC estimates from two well-established algorithms: the NASA Team (NT) algorithm (Cavalieri et al., 1984) and the Bootstrap (BT) algorithm (Comiso 1986). CDR SIC is based on gridded brightness temperatures (TBs) from the Nimbus-7 SMMR and the DMSP series of SSM/I and SSMIS passive microwave radiometers; the final product is provided at daily and monthly frequency on a 25 km × 25 km grid.
Secondly, the EUMETSAT OSI-450 is a level 4 product that covers the period from 1979 to 2015. The sea ice concentration is computed from the SMMR (1979–1987), SSM/I (1987–2008), and SSMIS (2006–2015) instruments, as well as ECMWF ERA-Interim data. The Interim OSI-430-b extends OSI-450 from 2016 onwards; it is an off-line product based on the same algorithms as OSI-450, and uses SSMIS data available through the NOAA CLASS, as well as operational analysis and forecast from ECMWF. The data processing introduced an open-water filter aimed at removing weather-induced false ice over open water, which unfortunately may remove some true low-concentration ice in the MIZ (Lavergne et al., 2019). OSISAF products are delivered at daily frequency on a 25 × 25 km grid. Lastly, the IFREMER/CERSAT product used here is derived from high frequency channels of SSM/I that yield a spatial resolution of 12.5 × 12.5 km. SIC is provided at daily and monthly frequency.
It is worth mentioning that OSISAF and IFREMER/CERSAT sea ice concentration are ingested by the data assimilation systems employed in the ORAs constituting GREP, while CDR is not assimilated in any ORA. The use of CDR is, hence, considered an advantage for the robustness of the GREP validation; OSISAF and IFREMER/CERSAT datasets are anyway used in our analysis. It has been shown that NT generally underestimates SIC (Andersen et al., 2007; Meier et al., 2014), and overestimates MIZ and underestimates pack ice by a factor of two compared to BT (Stroeve et al., 2016). On the other hand, BT produces too low SIC under extremely cold conditions (Comiso et al., 1997). The CDR algorithm blends NT and BT output concentration by selecting, for each grid cell, the higher concentration value, taking advantage of the strengths of each algorithm to produce concentration fields more accurate than those from either algorithm alone. Since passive microwave instruments tend to underestimate SIC, the aforementioned approach is considered to be more accurate (Meier et al., 2014). Given that observational datasets and ORAs use different horizontal grids, we interpolated the former onto the ORCA025 grid for the grid-point diagnostics.
In this paper, sea ice variability is described in terms of sea ice area (SIA) rather than sea ice extent (SIE). Sea ice extent is defined as the integral sum of the areas of all grid cells with at least 15% ice concentration, whereas sea ice area is the sum of the product of each grid cell area with at least 15% ice concentration and the respective ice concentration. Hence, sea ice area excludes open water areas between ice floes. Although these two metrics are highly correlated, uncertainties in SIC retrievals from passive microwave sensors have a larger impact on SIA that results in a weaker agreement across data records.
In addition to the total sea ice area, we consider two sea ice classes defined through SIC thresholds. The MIZ is here identified as the region extending from the outer sea ice–open-ocean boundary (defined by SIC equal to or higher than 15%) to the boundary of the consolidated pack ice (defined by 80% SIC). This definition has been previously used by Stroeve et al. (2016) to assess observed MIZ changes in Antarctica. The consolidated pack ice is then defined as the area with ice concentrations higher than 80%.
The seasonal variability of SIA is analysed for total, pack and MIZ sea ice on the hemispheric domain and in selected regions where satellite records have highlighted large differences in the ice response to climate. As in previous studies (e.g., Parkison and Cavalieri, 2012), the Antarctic domain is divided in the following five sectors (Figure 1): Weddell Sea (60° W–20° E, plus the small ocean area between the east coast of the Antarctic Peninsula and 60° W), Indian Ocean (20–90° E), western Pacific Ocean (90–160° E), Ross Sea (160° E–130° W), and the combined Amundsen-Bellingshausen Seas (130–60° W).
Results
We begin with the assessment of the interannual variability of total SIA reproduced by GREP and derived from satellite data sets. The GREP and observational products monthly-mean SIA is presented for the Southern Ocean as a whole, from January 1993 to December 2019, in Figure 2A. GREP SIA ranges from the summer minima occurring in February to winter maxima occurring generally in September, with a huge amount of sea ice growing and melting each year in very good agreement with observations. While the reanalysis ensemble slightly underestimates minima and maxima SIA, it correctly reproduces the large interannual variability, and properly depicts the record high in September 2014 (16.73 × 106 km2 in GREP and 17.42 × 106 km2 in CDR) and the marked decreases in the subsequent 3 years, with the record low in February 2017 (1.16 × 106 km2 in GREP and 1.57 × 106 km2 in CDR). GREP and CDR monthly anomalies of SIA show similar patterns and trends are basically consistent (Figure 2B), with an upward trend in yearly average SIA of 0.32 × 106 km2/decade in GREP and 0.31 × 106 km2/decade in CDR for 1993–2014, and trend close to zero (−0.04 × 106 km2/decade in GREP and −0.036 × 106 km2/decade in CDR) for the entire period 1993–2019. The good agreement between the three observational products (gray shading) and the four ORAs (pink shading) is notable; differences are greatest at the winter maxima.
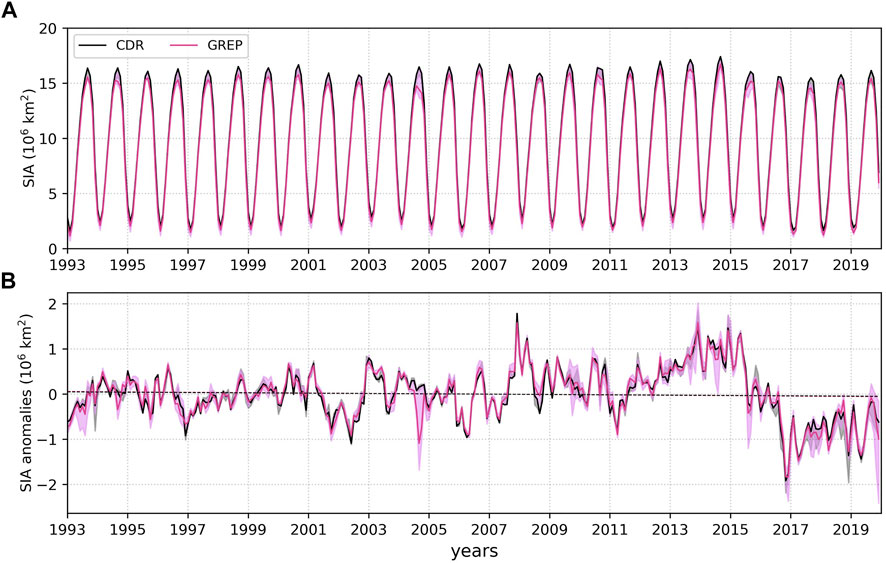
FIGURE 2. Time series of (A) monthly averages and (B) monthly anomalies of Antarctic sea ice area in GREP (magenta) and CDR (black) from January 1993 to December 2019, for GREP (magenta) and CDR (black). Pink (gray) shading denotes the envelope of GREP members (CDR, OSISAF, Ifremer CERSAT satellite records).
To quantify the inconsistency between GREP and satellite estimates, we use the integrated ice area error (IIAE) approach of Roach et al. (2018, 2020). The IIAE identifies the area of sea ice on which ORAs and observations disagree; it is computed as the sum of overestimated (O) and underestimated (U) sea ice area. These two O and U components are calculated as the sum of the product of the area and the SIC of each grid cell where GREP has a higher or lower concentration compared to observations. We apply the same metric also to pack ice and MIZ to determine how each sea ice class contributes to the overall error. The location of sea ice classes in CDR estimates is taken as the “true state”. The time series of IIAE O and U components for total ice, pack ice and the MIZ area computed relative to CDR are shown for the period 1993–2019 (Figure 3).
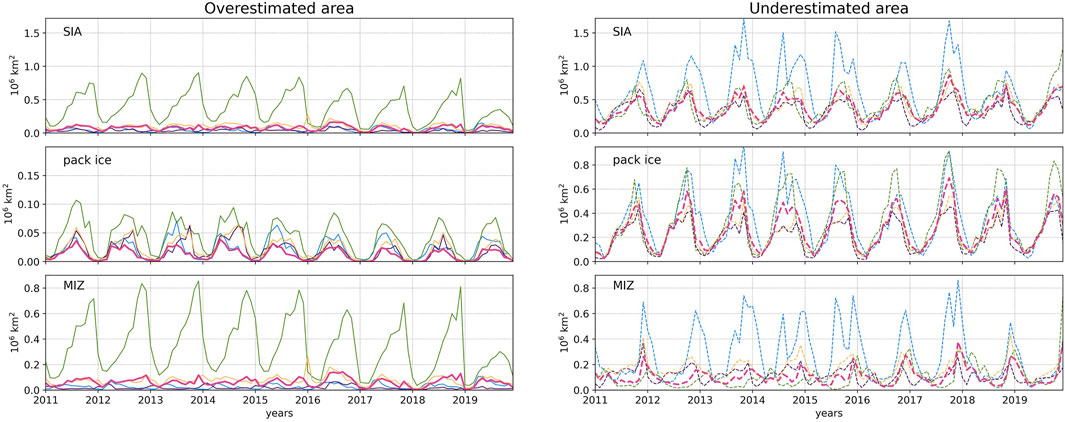
FIGURE 3. Time series of the GREP integrated ice area error components (in magenta) calculated with respect to CDR for total SIA (top panel), pack ice (middle panel) and the MIZ (bottom panel). Area of sea ice where GREP simulates higher (lower) SIC is on the left (right) column. The y-axis scales for pack ice are different. Thin lines represent the individual ORAs: CGLORS in light blue, FOAM-GloSea5 in violet, GLORYS2 in green, and ORAS5 in yellow–the same colors will be used in the following figures for single ORAs.
For every month, errors are very low relative to the mean SIA values, even for February and September. In general, GREP tends to underestimate total SIA area with the error ranging from 0.1 × 106 km2 in March-April to 0.7 × 106 km2 in October-December. Reanalyses generally tend to reproduce lower SIC than CDR, within the pack ice region: while IIAE O component in pack ice is relatively small (∼0.05 × 106 km2 all year round) and similar among the individual ORAs, IIAE U component grows up to 0.6 × 106 km in August-November and doubles for two reanalysis products. The MIZ also contributes to the total overestimated and underestimated area, but the error does not generally exceed 0.2 × 106 km2. There is one ORA outlier (GLORYS2) that generally contributes to overestimating SIA, and one (CGLORS) to underestimating it. The former (the latter) reproduces too high (low) SIC in the MIZ. Overall, GREP performs well owing to minimization of systematic errors in individual products. Additionally, the error in the ensemble mean is consistent throughout the years, which is not the case for single ensemble members.
The accuracy of GREP and individual ORAs in reproducing the spatial distribution of SIC is shown in Figure 4, where maps of the SIC root mean square errors (RMSE) for GREP and individual ORAs against CDR are presented for September and February, which are typically the months of maximum and minimum ice coverage respectively. The monthly climatologies are computed over the years 1993–2019. The sign of the errors has also been analyzed through the spatial distribution of the average bias (not shown). In September, RMSEs are lower than 5% along the Antarctic coast for all ORAs and tend to grow towards the ice edge, with the highest values generally smaller than 15% except for one single product, GLORYS2, which overestimates SIC by up to 20% in the Ross Sea and the Bellingshausen and Amundsen Seas. In February, the largest disagreement with CDR is located near the Antarctic coast, in particular in the Indian Ocean and the Western Pacific Ocean sectors, where three of the four ORAs underestimate the observed concentration. This error may be primarily linked to the reanalyses representation of sea ice drift and the Antarctic coastal current in the eastern Antarctica (not shown). One product (CGLORS) exhibits an unique behavior with the RMSE for SIC exceeding 30% along the entire Antarctic coastline - this indicates a lower concentration compared to CDR that may be related to a large warm bias in sea surface temperature along the coast, in particular in the Indian and the Western Pacific Oceans (not shown). GREP compares well with satellite estimates considering that the RMSEs are of the same order as the uncertainties from SIC retrievals using passive microwave radiometry (Ivanova et al., 2015). Time evolution of the mean over area RMSE (not shown) indicates that the RMSE for GREP concentration is up to ∼10% in summer months (January-February) and does not exceed 7% in other months.
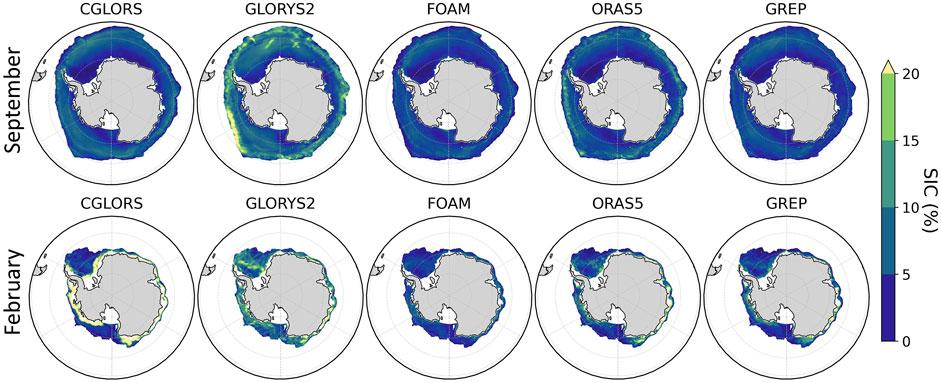
FIGURE 4. Mean root mean square error for SIC at every grid cell for the single ORAs and GREP against CDR in September (upper row) and February (lower row).
We also analyze the ensemble spread (ES) in order to assess the overall consistency across ORAs (Figures 5A,B). The largest ES in SIC (∼35% in February) is found during the melting season everywhere along the Antarctic coast, except in the Weddell and the Ross Seas. Increased ES is consistent with uncertainties coming from the assimilated satellite data - SIC retrievals present larger uncertainties within the melting season due to surface wetness and a broad variety of sea ice forms that affect sea ice emissivity (Ivanova et al., 2015; Meier and Stewart, 2019). In September, there is high consistency among ORAs due to the larger portion of stable and compact pack ice. Larger ES is located in the MIZ and does not exceed 10%. Finally, we compare RMSE of GREP SIC calculated against CDR, with the SIC ES to evaluate whether the ensemble is over-dispersive or under-dispersive. The spatial distribution of the metric (GREP RMSE minus ES) is shown for September and February in Figures 5C,D. GREP is over-dispersive when RMSE < ES (blue/negative) and under-dispersive when RMSE > ES (red/positive). In September, it appears that ensemble dispersion depends on sea ice class: GREP is over-dispersive in the MIZ (represented by the region between contour lines), whereas GREP is under-dispersive within the pack ice. This means that ORAs agree better on the representation of high concentration in the region of stable pack ice, where the ORAs performances are less challenging compared to the MIZ. In February, there does not seem to be a direct relationship between ensemble dispersion and sea ice class. The pattern of the difference is heterogeneous, particularly along the coast of the eastern Antarctic. In the Weddell and Ross Seas, the GREP remains over-dispersive. Time series of the difference between GREP RMSE and ES better presents the opposite behavior of sea ice classes and the contribution to total sea ice changes (Figure 5E). The compensation between sea ice classes in all seasons, except in summer, translates into close-to-zero values for the total ice concentration. From December to February, GREP RMSE exceeds ES in both pack ice and the MIZ, leading to an increased difference for the total ice area.
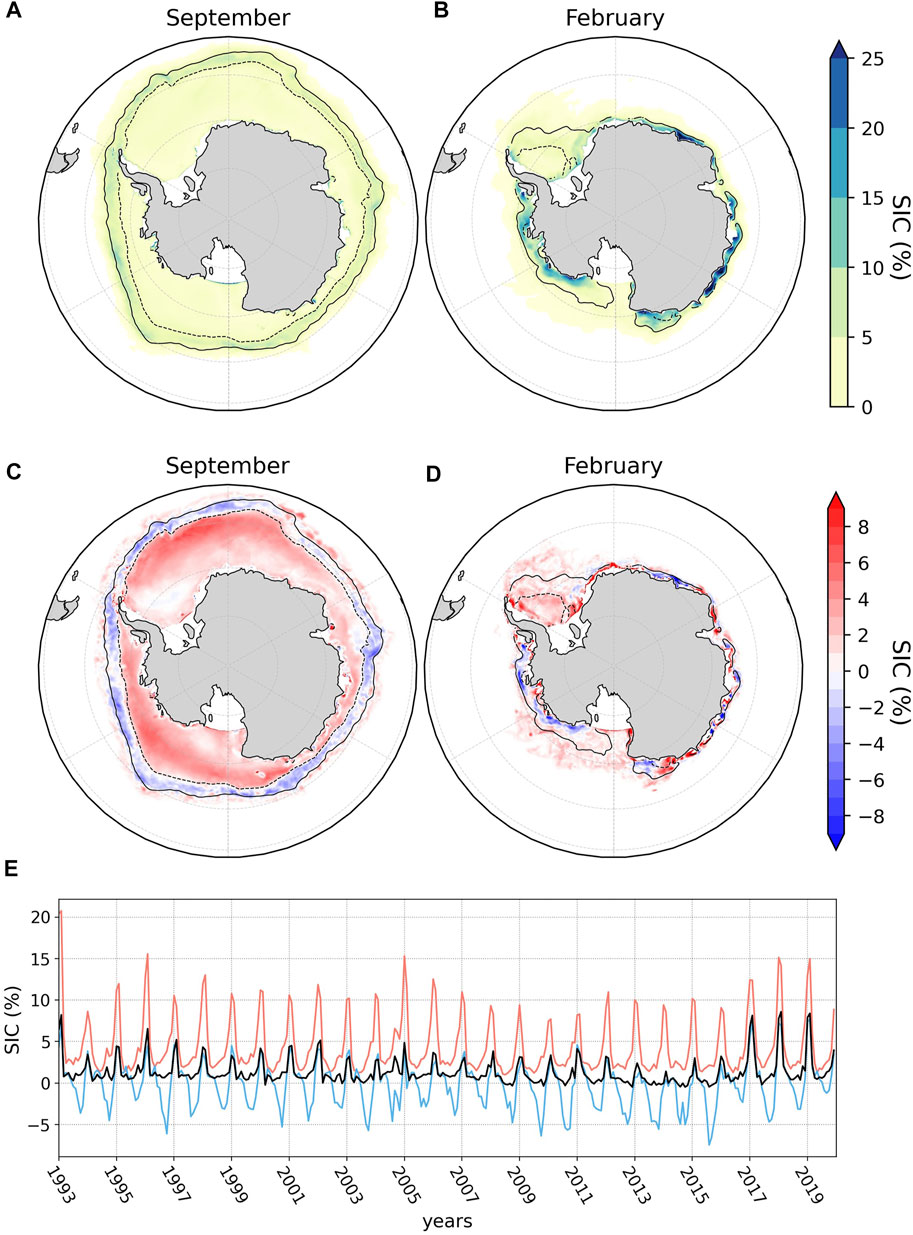
FIGURE 5. Spatial distribution of (A,B) ensemble spread (ES) and (C,D) difference (in %) between GREP RMSE and ES for SIC in September and February. Contours indicate the mean position of 15% (solid) and 80% (dashed) ice concentration over the period 1993–2019 from CDR. (E) Time series of the difference (in %) between GREP RMSE and ES spread of SIC for total area (black), pack ice (red), the MIZ (blue).
Seasonal Variability
We proceed with an assessment of the consistency of the seasonal sea ice variability between the reanalysis ensemble and satellite estimates. The climatological mean seasonal cycle of the circumpolar SIA as represented by GREP, single ORAs and observational estimates, is shown for total sea ice, pack ice, and MIZ, in Figure 6A. The seasonal cycle of Antarctic sea ice is consistent among ORAs and in phase with observations. All systems have a maximum in total SIA in September, and a minimum in February; it takes about 7 months to expand sixfold from summer minimum of ∼2.5 × 106 km2 to winter maximum of ∼15 × 106 km2, and about 5 months to melt again. It is worth noting that the ensemble spread of ORA SIA is limited throughout the year, and is comparable to the estimated observational uncertainty. The seasonal cycle of Antarctic-wide total SIA is dominated by the variability of pack ice, whose area evolves at the same rate as total ice. GREP slightly underestimates the area of pack ice from August for the melting season (only one reanalysis, GLORYS2, is larger than observational products), but all ORAs align well with observations during refreezing in autumn.
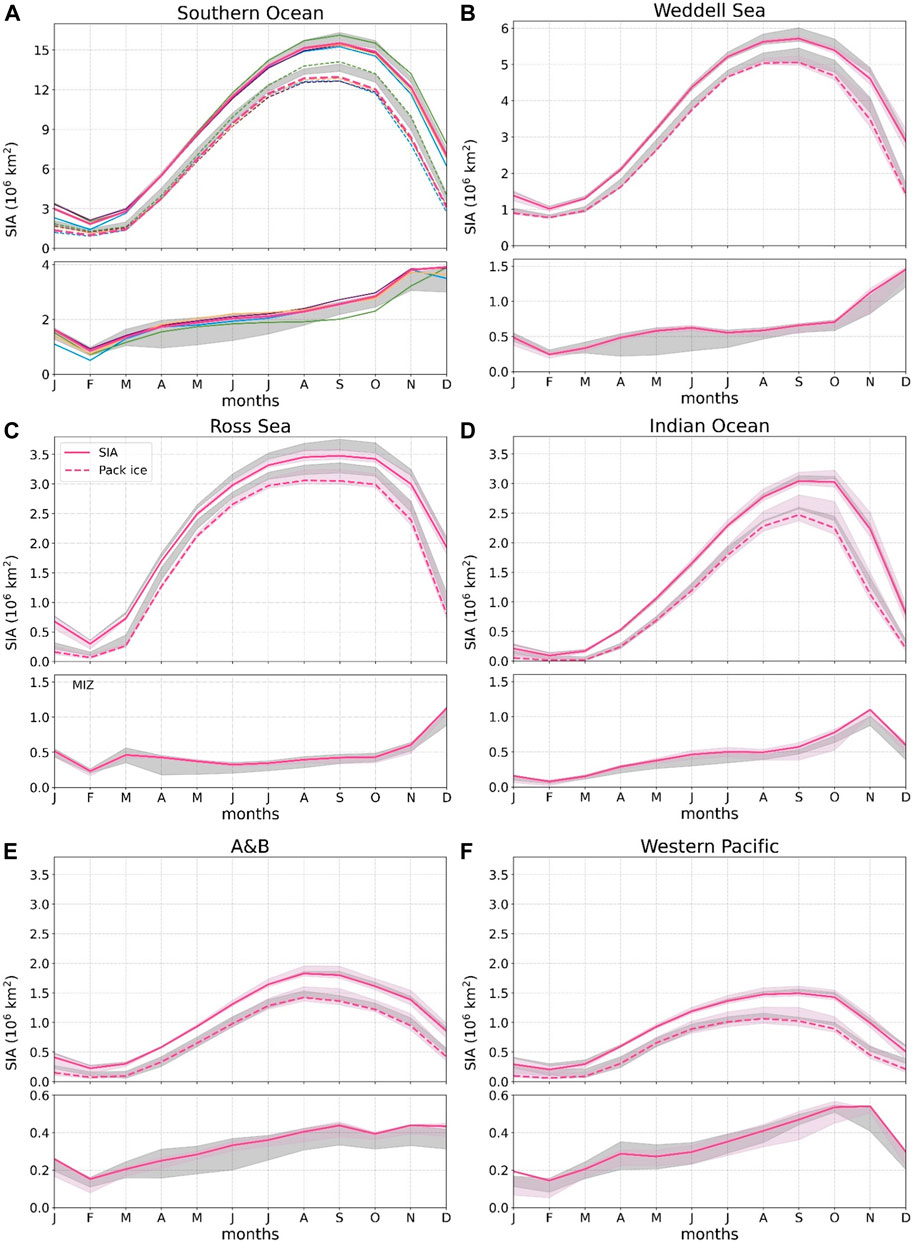
FIGURE 6. Mean seasonal cycle (1993–2019) in the total SIA (solid), pack ice (dashed) (upper subplots), and the area covered by Marginal Ice Zone (MIZ) (lower subplots) computed for GREP (in magenta) and the individual ORA (thin colored lines) in the Antarctic-wide region (A), for GREP in the five sub-sectors (B-F). Pink shading denotes the envelope of GREP members. Gray shading denotes the envelope of observational estimates (CDR, OSISAF, Ifremer CERSAT). Please note the different y-axis scales for the Southern Ocean and Weddell Sea.
The seasonal changes in the MIZ are quite different from those in total ice and pack ice. On average, the MIZ advance needs about 10 months to progress from near the coast (in February) to its most equatorward maximum (in November or December) and about only 2 months to revert to a minimum. After summer, the MIZ area grows simultaneously with pack ice, in part transforming into it, and continues to expand in spring after the total (and pack) SIA peaks. The further increase in the MIZ area after the consolidated ice pack begins to melt implies that, as it starts to retreat, the pack ice converts in part to MIZ over a wider area. We note the Antarctic MIZ/pack-ice ratio is close to 1 from December to March. GREP is always in the observed envelope; the ensemble spread of ORA SIA is generally smaller or comparable to the estimated observational uncertainty. Here, the larger spread among the observed MIZ area (found also between NT and BT algorithms by Stroeve et al., 2016) reflects the different ability of high and low frequency channels used in the different data algorithms to retrieve low fraction sea ice. However, GLORYS2 underestimates the MIZ area from July to December, and this can be attributed to faster sea ice consolidation in the growing season. This is consistent with the IIAE analysis (Figure 3), which indicates that this system simulates higher SIC in those grid cells that are considered to belong to the MIZ in observations, and with the RMSE of SIC (Figure 4) with larger errors in the outer ice region where MIZ is located. CGLORS underestimates the MIZ area in summer from December to February, causing a large impact on the minimum of total SIA (as seen in Figures 3, 4).
For all sea ice classes, the highest consistency among datasets is observed throughout autumn freezing, from March to June. Overall, due to the realistic performance of all single members and the cancellation of systematic errors, GREP reproduces robust estimates of the seasonal cycle of Antarctic total ice area and the two sea ice classes.
The different seasonality of sea ice classes is a notable result that confirms a different interplay of ice classes with the ocean and the atmosphere. Seasonal variability of Antarctic sea ice is governed by the position of the circumpolar trough relative to the ice edge and associated wind field and Ekman transport (Enomoto and Ohmura, 1990; Eayrs et al., 2019). In spring, when the circumpolar trough is north of the ice edge, hastened conversion of pack ice to the MIZ is supported by divergence which results in opening of pack ice. This consequently facilitates solar absorption in the upper ocean and accelerates lateral melting of ice floes (e.g., Perovich and Jones, 2014) which contributes to the MIZ growth. From December to February, the MIZ area rapidly retreats together with pack ice, driven by southward Ekman forcing and sea ice convergence. However, the MIZ represents a significant part of the overall ice cover from December to March (the proportion between the MIZ and pack ice area is in the range between 0.8 and 1.2).
Analysis in the Sub-regions
Since Antarctic sea ice variability and trends are spatially heterogeneous (e.g., Parkinson and Cavalieri, 2012; Parkinson 2019), the analysis of the Antarctic circumpolar sea ice is rather limited. In this section, we investigate the accuracy of GREP performance on regional scales by analysing the seasonal variability of total ice, pack ice and MIZ area for each of the five Antarctic sectors (shown in Figure 1), and by comparing GREP output with the CDR product.
As expected, there are significant differences among the five sectors in the amount of ice classes, the timing of maxima and minima, the rate of sea ice expansion and the retreat phase (Figures 6B–F). This contrast in the regional patterns of sea ice growth and melt is associated with geographic differences and interplay of leading climate processes (Maksym et al., 2012).
There is a very good agreement between GREP and CDR variability in all regions (Figures 6B–F, Figure 7). It is worth noting that the spread in observational products (and in the reanalyses) varies not only among sea ice classes, but also among regions. The spread of observational estimates of MIZ area is generally larger than the spread of the reanalysis ensemble, in particular in the Weddell Sea and Ross Sea in autumn months and in the Amundsen-Bellingshausen (A-B) Seas from March to December.
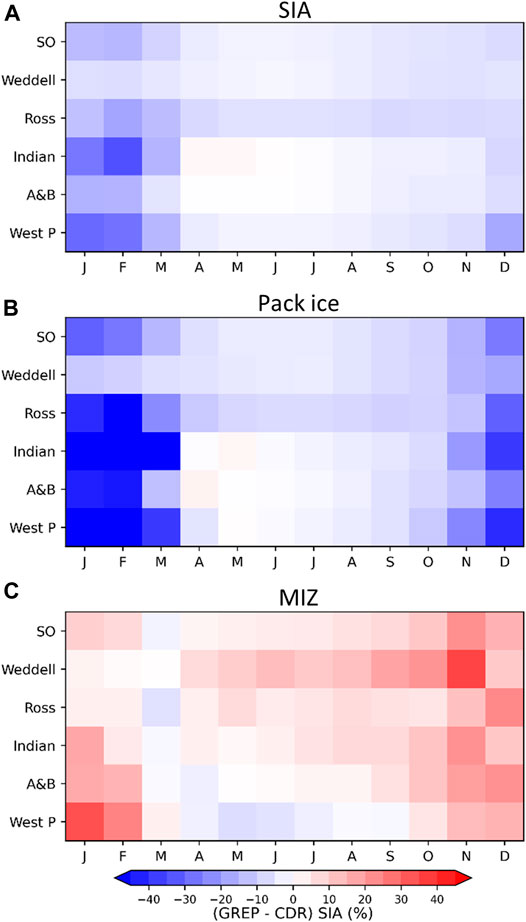
FIGURE 7. Seasonal cycle of (GREP minus CDR, in %) monthly climatology of (A) total ice (upper panel), (B) pack ice (middle) and (C) MIZ area in the Southern Ocean and its five sectors. Differences are shown as a percentage of CDR values computed for the years 1993–2019. Red (blue) indicates that GREP reproduces higher (lower) SIA compared to CDR.
As in the Southern Ocean as a whole, all sectors exhibit a large annual cycle of monthly total SIA (Figure 6), with asymmetric growth and melt seasons. However, there are large differences in the timing and magnitude of the sub-region seasonality, given that the rate of waxing and waning of sea ice and the interplay with air-sea components vary across the sectors. Minima of total SIA always occur in February and maxima occur frequently in September (Figure 6), although with much greater interannual variability than in the Southern Ocean as a whole (not shown). The pattern and ratio of pack and marginal ice widely varies among the regions.
The regional variability as reproduced by the GREP ensemble-mean is described for individual sectors. In the Weddell Sea (Figure 6B), the SIA is much higher than other regions and has the largest distribution of pack ice. Its seasonality is consistent with the Southern Ocean as a whole. From the February minima (∼1 × 106 km2), total and pack ice areas begin to expand in March and peak (at ∼ 5.7 × 106 km2 and ∼5 × 106 km2 respectively) on average in September, but maximum timing varies frequently from August to October (not shown). The Weddell Sea provides the greatest contribution (∼55%) to the summer sea ice area in the Southern Ocean, due to the presence of consolidated pack ice all year around. In agreement with CDR, the ensemble-mean shows that the Weddell Sea holds the largest percentage (∼75%) of February pack ice. The MIZ area also starts to advance in March and continues to increase until December (∼1.45 × 106 km2), as the pack ice quickly retreats. In this region, the sea ice cover expands northwards until it reaches a region with strong air-sea dynamics. North of the consolidated pack ice region, ice continues to advance, thanks to further freezing or breaking by the winds and currents.
The second largest contribution to the Antarctic-wide ice area comes from the Ross Sea (Figure 6C). In this sector, the total ice and pack ice areas present a large asymmetric seasonal cycle, with, approximately, a 9-months growth period and a 3-months melting period. With almost no pack ice, the total sea ice and MIZ areas have a marked minimum always occurring in February. There is a large variability in the timing of total and consolidated pack ice maxima occurring generally from August to October and reaching ∼3.5 × 106 km2 and ∼3 × 106 km2 respectively. In February, the minimum SIA mainly consists of MIZ that covers ∼0.25 106 km2; the MIZ fraction is then nearly constant throughout the expansion and retreat of the pack ice, with a maximum in December (1.13 × 106 km2) as the pack ice rapidly decays. The Ross Sea, like the Western Pacific (Figure 6F), exhibits a second peak in the MIZ area in March, in the freezing season, when the area of MIZ and pack ice starts to expand and the increasing sea ice consolidation is accompanied by MIZ-to-pack ice transformation.
In the Indian Ocean, the total SIA maximum (3 × 106 km2) is reached in October rather than September (Figure 6D), about 1 month later the pack ice peak (2.4 × 106 km2) is reached. The pack ice tends to disappear completely in summer and when MIZ comprises the largest portion of the overall ice cover. The MIZ advances from March until November when its area (∼1.1 × 106 km2) is comparable to that of pack ice.
At their largest, the A-B Seas and Western Pacific Ocean together account for less than 20% of the Antarctic-wide SIA, with the lowest winter maxima (1.83 and 1.5 × 106 km2, respectively); they can weakly affect the Antarctic sea ice seasonal cycle. In both sectors, the areas of consolidated pack ice and MIZ are generally comparable in the winter months. The A-B Seas are in major contrast with the rest of the Southern Ocean (Parkinson, 2019), and are characterized by an overall downward sea ice trend (not shown) related to the upper ocean warming at the west of the Antarctic Peninsula (e.g., Ducklow et al., 2012). Seasonality of ice expansion and retreat are almost symmetric for total ice and pack ice areas (Figure 6D) that both peak in August (the maximum timing varies from July to October from year to year) and are minimum between February and March. The MIZ area increases during most of the year, from February to December. There is a large interannual variability in the timing of the maximum that results in the double peaks in September and November (approximately 0.42 × 106 km2). Here, the MIZ area does not further increase when pack ice starts to retreat, in contrast to other regions. The MIZ gives the largest contribution to total area from January to April. In this sector the spread of observational estimates of MIZ is very large compared to the ORAs spread- GREP is always located within the observed envelope. In the Western Pacific Ocean, the total SIA reaches the highest value from August to October (Figure 6F), with the maximum generally occurring in September (∼1.5 × 106 km2). While pack ice area exhibits very low values and stays nearly constant throughout the summer period, MIZ area presents a prominent minimum in February and then begins to quickly expand until November when it exceeds pack ice area. The MIZ area remains larger until autumn.
Figure 7 shows how GREP representation of the seasonal variability of total ice, pack ice and the MIZ area differs from CDR estimates; due to the large regional contrasts in the amount of sea ice, the differences are expressed as a percentage of the average of CDR values. For total SIA, the difference between GREP and CDR is almost everywhere within 15% from April to December (Figure 7A). Thus, GREP seasonal variability is consistent in time and space with the observed sea ice changes over the period 1993–2019. The largest differences are generally found in summer, in particular in the Indian Ocean and the Western Pacific where GREP area is about 25% lower than CDR. The accuracy of GREP stands out in the Weddell Sea where total sea ice area differs from CDR data by -7% at the most. The high quality of total sea ice in the reanalysis ensemble results from the contrasting behaviour of pack and MIZ area. Differences have a similar pattern for pack ice areas, but with different magnitudes. The highest values are found from December to March when GREP tends to generally underestimate the area of consolidated ice in all sectors. Due to the very low amount of pack ice area in both GREP and CDR in spring and summer, this metric typically detects small differences with respect to CDR. For example, GREP pack ice area differs by ∼70% from CDR in the Indian Ocean in February, when pack ice area has almost disappeared in the region, with values lower than 0.1 × 106 km2. As for the total ice, it is in the Weddell Sea sector that GREP better reproduces the seasonal variability of the pack sea ice area. Overall, the ensemble-mean reproduces a larger area of the MIZ almost everywhere. As for pack ice, GREP and CDR differences are the smallest in the growing season when GREP MIZ extends 10% more than CDR at most - differences stay small but reverse in the Western Pacific Ocean during autumn-winter months. The GREP MIZ area is 20–30% larger than observed estimates generally in November-December, when it approaches its maximum values. The largest departures from CDR are found in the Western Pacific sector in January and the Weddell Sea in November. It is worth noting that the MIZ area reproduced by GREP has generally the largest differences from the observational estimates when they present large spread (Figure 6).
Discussion
Understanding the mechanisms and rates of Antarctic sea ice change is crucial from a climate-change perspective. Sea ice concentration retrieved from satellite microwave radiometers has been available on a daily basis since the late 1980s at a horizontal resolution finer than 25 km. However, these observational estimates are highly dependent on which passive microwave methods and sea ice algorithms are used (Ivanova et al., 2014; 2015). There are dozens of such algorithms available. Although these products agree quite well on area trends, absolute values of total SIC and SIA are not necessarily consistent with each other. There are also large differences among observed products in the regional ice distribution and trends, as well as in the contribution of consolidated ice or MIZ in the total ice cover (Stroeve et al., 2016). This is of particular importance for accurate assessment of processes contributing to climate change and assimilation of sea-ice in models. Reliable estimates of sea ice concentration and relative parameters are necessary to constrain also other ice parameters in modelling studies of past, present and future variability.
Simulation of Antarctic sea ice remains a fundamental challenge for state-of-the-art climate models (e.g., Holmes et al., 2019; Roach et al., 2020). Despite advances in climate modeling capabilities, the CMIP5 and CMIP6 intermodel spread in Antarctic sea ice extent is large, especially in summer, and the observed weak upward trend of the Antarctic ice extent is not captured yet (Turner et al., 2013; Roach et al., 2020; Shu et al., 2020; Shu et al., 2020). The poor accuracy of Antarctic sea ice changes in the CMIP exercises limits our understanding on what drives regional and seasonal Antarctic sea ice changes, including feedback and competing processes.
Our analysis confirms that ocean reanalyses are a fundamental tool for investigating climate variability and for evaluating key climate diagnostics that are not directly observed (e.g., Masina and Storto, 2017). Given the robustness of its mean and the implicit quantification of uncertainty by means of the spread, the multi-model ensemble provides a robust representation of the spatial and temporal variability of Antarctic sea ice. Although sea ice concentration is the most well-constrained sea ice parameter, the ensemble spread mainly comes from differences in implemented data assimilation schemes but also from other sources of uncertainty such as differences in models, observational datasets and air-sea flux formulations.
We found strong consistency between the reanalysis ensemble and the satellite products, and GREP generally outperforms or at least equals individual reanalyses in approaching observation-based estimates of sea ice area. The advantage of the multi-model approach is highlighted by the fact that it is practically impossible to determine which one of four performs the best for all metrics and seasons. GREP smooths the strengths and weaknesses of single systems and provides the most consistent and reliable estimates of the mean state and variability of sea ice area. Nevertheless, advancement in model formulations and data assimilation schemes in single members could reduce the impact of ORAs shortcomings on the realism and accuracy of the ensemble-mean solution.
Although the main objective of the study is the evaluation of the GREP ability to reproduce the observed sea ice area on interannual and seasonal scales, our results also confirm the importance of regional variability and the distinction in sea ice classes. They should be considered when assessing how Antarctic sea ice varies in model simulations and when investigating the different processes that are likely contributing to ice interannual and seasonal.
We focus on how consolidated pack ice and the marginal ice change in relation to their different characteristics and therefore their different sensitivities to the external forcing. Differences in the seasonality of ice classes suggest that their variability is driven by changes in wind and ocean conditions in a different way. While the description of processes controlling the distribution of the MIZ and pack ice is out of scope of this study, we emphasize that a better knowledge of temporal and spatial variability in the MIZ and pack ice can provide a deeper insight of possible driving mechanisms behind these changes. We show that both GREP (and individual ORAs) and satellite products present considerable differences in the climatological mean seasonal cycle in the area of ice classes. The net circumpolar changes in sea ice area is the result of the interplay of MIZ and pack ice, and their different response to changing wind and ocean conditions. The annual waxing and waning of sea ice cover implies redistribution of ice floes between the MIZ and pack ice from month to month as well as spatial expansion and contraction of sea ice edge. When pack ice starts to melt and its area to retreat in spring, the breaking of ice floes contributes to the MIZ expansion that continues for 2–3 months. That results in a strong asymmetry in the MIZ seasonal cycle in all Antarctic regions, with approximately 9–10 months of advance and 2–3 months of retreat. Contractions and expansions of pack ice and the MIZ do not necessarily follow the changes in the location of the outer sea ice edge: ice classes can contribute to changes in sea ice coverage in different ways or even exhibit an opposite behavior (Stroeve et al., 2016). GREP reproduces regional differences in the proportion between pack and MIZ, the timing and duration of freezing and melting seasons, in close agreement with observation-based results (e.g., Stroeve et al., 2016; Parkinson, 2019; Wang et al., 2021).
The reanalysis ensemble agrees well with the CDR product on the different contributions of MIZ and pack ice to changes in the Antarctic-wide total ice. Monthly trends (computed as function of longitude and month) in the total, pack and marginal ice area (Figure 8) indicate a large degree of seasonal and regional variability around Antarctica. In all sectors and for all months, the spatial patterns and magnitude of statistically significant positive and negative trends in total ice area are highly consistent between GREP and CDR in all sectors. Results highlight the necessity to distinguish between sea ice classes in order to assess the quality of numerical systems. Although GREP and CDR are similar in SIA trends, there are some inconsistencies when looking at sea ice classes: GREP barely reproduces the correct magnitude of trends in the Eastern Antarctic and does not simulate the MIZ area expansion in December in the Ross Sea. Generally, in both GREP and CDR, significant trends in the MIZ are less pronounced but more heterogeneous in space, and they tend to offset the significant trends in pack ice. This is for example evident in the Ross Sea, where no trend is found in the observed total sea ice area in December, due to compensation between the opposite trends in the MIZ and pack ice. Positive trends in total SIA are generally dominated by statistically significant positive trends in the consolidated pack ice as in the western Weddell Sea from January to March. Both ice classes contribute to the statistically significant negative trends in the eastern Ross Sea and eastern A-B Seas in summer. The regional variability of the MIZ area trends during spring and autumn is consistent with a complex pattern of changes in timing of sea ice advance, retreat and duration (e.g., Eayrs et al., 2019).
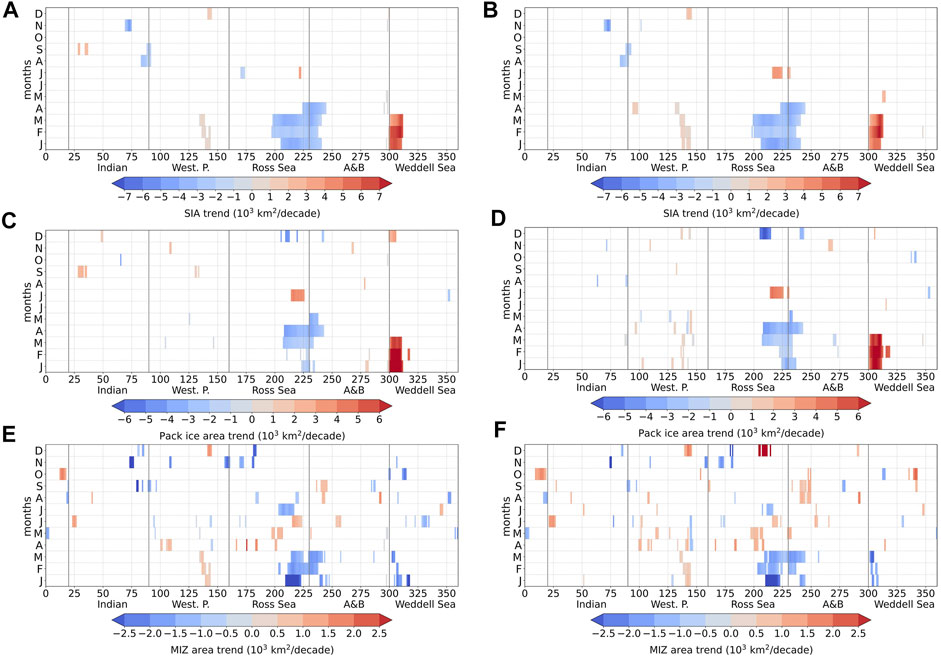
FIGURE 8. Monthly trends (1993–2019) in regional areas of total ice (A,B), pack ice (C,D), and MIZ (E,F), as a function of longitude. Left (right) columns correspond to GREP (CDR). Only significance higher than 95% are shown. Note that colorbar scales are different for ice classes.
Differences between GREP and CDR can be also explained by some limitations in our analysis. The first caveat concerns the methodology: we distinguish sea ice classes through sea ice concentration thresholds. Although the SIC-based definition is the one most often used (e.g., Strong and Rigor, 2013; Stroeve et al., 2016; Rolph et al., 2020), Vichi (2021) showed that it is not able to adequately capture the features of the Antarctic MIZ, in which ice dynamics is determined by oceanic and atmospheric processes. Indeed, this definition of the MIZ is not physically or dynamically explained; the lower boundary is linked to uncertainty from SIC retrievals (Comiso & Zwally, 1984) while the upper boundary corresponds to the WMO definition of “close ice” (WMO, 2009). In fact, the properties of Antarctic ice cover do not directly depend on the degree of coverage. In-situ measurements carried out in the Southern Ocean showed that close pack ice with SIC up to 100% do have the dynamical properties of the MIZ (Alberello et al., 2019; Vichi et al., 2019; Brouwer et al., 2021), which discredits the reliability of threshold-based definition. Vichi (2021) proposed an alternative MIZ definition that is based on statistical properties of the SIC and its spatial and temporal variability. The new method indicates the measure of variability, which is a key feature of the marginal ice. It also overcomes the disparity among the algorithms that could considerably differ in their representations of sea ice concentration, area and extent.
Given the highly dynamic nature of the MIZ, another limitation of this study is the temporal resolution of GREP and ORAs output provided by CMEMS. Our analysis is constrained by monthly means of SIC from reanalyses. The use of daily fields might be more appropriate to investigate the MIZ variability and its linkage to regional interactions with ocean and atmosphere.
Conclusion
We assessed the accuracy of the CMEMS Global Reanalysis Ensemble Product (GREP) in reproducing the evolution in time and space of Antarctic total sea ice and discriminating the consolidated pack ice from the MIZ. Antarctic sea ice area from GREP is compared to a set of sea ice satellite products. GREP properly reproduces interannual and seasonal variability of total sea ice area both on hemispheric and regional scales. GREP is shown to properly represent the interannual and seasonal variability of pack and MIZ areas during the growing and melting seasons, as well as their minima and maxima. More evident discrepancies between GREP and satellite products occur during summer, when the spread among individual ORA increases; one product tends to underestimate MIZ area and another to overestimate pack ice area. Nonetheless, due to minimization of the single errors, the ensemble mean provides the most consistent and reliable estimates. The spatial distribution of RMSE in SIC also indicates that GREP smooths out strengths and weaknesses of individual systems.
For all ice classes, the ensemble spread is comparable to the spread among the observational estimates. The quality of GREP is generally comparable to that of satellite data sets and the differences between GREP and CDR are comparable or even smaller than differences between different algorithms (Stroeve et al., 2016). The seasonal cycle of the total sea ice area is within the observational uncertainty almost all year round, while the pack ice area is generally underestimated and the MIZ area is in the upper end of the observational range. This compensation between sea ice classes partly reflects misplacement of sea ice across the basin compared to the “true state”.
Dispersion of GREP in sea ice concentration also appears to depend on sea ice classes. Due to the compensation between the opposite behavior in pack ice (GREP is under-dispersive) and the MIZ (GREP is over-dispersive), the difference between GREP RMSE and GREP ES is close to zero for the total ice area.
On a regional scale, the Weddell Sea is the region where GREP provides the most accurate representation of sea ice area, while the largest and most persistent discrepancies occur in the Indian and the Western Pacific sectors. This spatial distinction in GREP performance is attributed to the proportion of pack ice and the MIZ in the regions. Given its highly dynamic nature, the MIZ is more challenging to simulate compared to pack ice.
Considering that ocean reanalyses are widely used as boundary and initial conditions in forecasting systems, sub-optimal representation of the SIC distribution and variability can affect the quality of the output. The results of the current work proved the quality of the GREP product with regard to sea ice concentration and associated metrics. GREP agrees well with satellite products, and can be used to get a robust estimate of current sea ice state and recent trends in sea ice area and extent. However, improvement in data assimilation techniques, space-time data coverage in the ice-covered Southern Ocean regions, and availability of other ice properties (such as thickness and drift) from satellite measurements will most probably enhance the quality of ORAs and GREP in polar regions.
Data Availability Statement
ORAs data and satellite datasets analyzed in this study are all freely available online. GREP output (product identifier GLOBAL_REANALYSIS_PHY_001_031) and its full documentation are provided by the Copernicus Marine Environment Monitoring Service, and are available to download through CMEMS webpage https://resources.marine.copernicus.eu/products Sea Ice Concentration data sets can be downloaded at the following links:
• NOAA/NSIDC Climate Data Record data set: https://nsidc.org/data/g02202/versions/3/
• EUMETSAT OSISAF: https://osi-saf.eumetsat.int/products/sea-ice-products
• Ifremer/CERSAT: ftp://ftp.ifremer.fr/ifremer/cersat/products/gridded/psi-concentration/
Author Contributions
DI conceived and designed this study, and wrote the manuscript. JS analyzed, simulated and observed datasets, and contributed to the interpretation of results and editing. SM and AC reviewed the manuscript.
Funding
DI was funded by the European Union's Horizon 2020 research and innovation program under Grant agreement No 101003826 via the project CRiceS (Climate Relevant interactions and feedbacks: the key role of sea ice and Snow in the polar and global climate system). JS was supported by the Italian National Program for Research in Antarctica (PNRA) via the project INVASI (Interannual Variability of the Antarctic Sea Ice/ocean system from ocean reanalyses, Reference Number PNRA18-00244). AC and SM were supported by Copernicus Marine Service “Global Ocean Reanalysis for the GLO MFC” (Reference number: 114-R&DGLO-RAN-CMEMS).
Conflict of Interest
The authors declare that the research was conducted in the absence of any commercial or financial relationships that could be construed as a potential conflict of interest.
Publisher’s Note
All claims expressed in this article are solely those of the authors and do not necessarily represent those of their affiliated organizations, or those of the publisher, the editors, and the reviewers. Any product that may be evaluated in this article, or claim that may be made by its manufacturer, is not guaranteed or endorsed by the publisher.
References
Alberello, A., Bennetts, L., Heil, P., Eayrs, C., Vichi, M., MacHutchon, K., et al. (2020). Drift of Pancake Ice Floes in the winter Antarctic Marginal Ice Zone during Polar Cyclones. J. Geophys. Res. Oceans 125 (3), e2019JC015418. doi:10.1029/2019jc015418
Alberello, A., Onorato, M., Bennetts, L., Vichi, M., Eayrs, C., MacHutchon, K., et al. (2019). Brief Communication: Pancake Ice Floe Size Distribution during the winter Expansion of the Antarctic Marginal Ice Zone. The Cryosphere 13 (1), 41–48. doi:10.5194/tc-13-41-2019
Andersen, S., Tonboe, R., Kaleschke, L., Heygster, G., and Pedersen, L. T. (2007). Intercomparison of Passive Microwave Sea Ice Concentration Retrievals over the High‐concentration Arctic Sea Ice. J. Geophys. Res. Oceans 112 (C8), 1. doi:10.1029/2006jc003543
Balmaseda, M. A., Hernandez, F., Storto, A., Palmer, M. D., Alves, O., Shi, L., et al. (2015). The Ocean Reanalyses Intercomparison Project (ORA-IP). J. Oper. Oceanography 8 (Suppl. 1), s80–s97. doi:10.1080/1755876x.2015.1022329
Balmaseda, M. A., Trenberth, K. E., and Källén, E. (2013). Distinctive Climate Signals in Reanalysis of Global Ocean Heat Content. Geophys. Res. Lett. 40 (9), 1754–1759. doi:10.1002/grl.50382
Bintanja, R., van Oldenborgh, G. J., Drijfhout, S. S., Wouters, B., and Katsman, C. A. (2013). Important Role for Ocean Warming and Increased Ice-Shelf Melt in Antarctic Sea-Ice Expansion. Nat. Geosci 6 (5), 376–379. doi:10.1038/ngeo1767
Blanchard-Wrigglesworth, E., Roach, L. A., Donohoe, A., and Ding, Q. (2021). Impact of Winds and Southern Ocean SSTs on Antarctic Sea Ice Trends and Variability. J. Clim. 34 (3), 949–965. doi:10.1175/jcli-d-20-0386.1
Brouwer, J., Fraser, A. D., Murphy, D. J., Wongpan, P., Alberello, A., Kohout, A., et al. (2021). Altimetric Observation of Wave Attenuation through the Antarctic Marginal Ice Zone Using ICESat-2. The Cryosphere Discuss. [preprint], in review. doi:10.5194/tc-2021-367
Cavalieri, D. J., Gloersen, P., and Campbell, W. J. (1984). Determination of Sea Ice Parameters with the Nimbus 7 SMMR. J. Geophys. Res. 89 (D4), 5355–5369. doi:10.1029/jd089id04p05355
Chevallier, M., Smith, G. C., Dupont, F., Lemieux, J.-F., Forget, G., Fujii, Y., et al. (2017). Intercomparison of the Arctic Sea Ice Cover in Global Ocean-Sea Ice Reanalyses from the ORA-IP Project. Clim. Dyn. 49 (3), 1107–1136. doi:10.1007/s00382-016-2985-y
Comiso, J. C., Cavalieri, D. J., Parkinson, C. L., and Gloersen, P. (1997). Passive Microwave Algorithms for Sea Ice Concentration: A Comparison of Two Techniques. Remote sensing Environ. 60 (3), 357–384. doi:10.1016/s0034-4257(96)00220-9
Comiso, J. C. (1986). Characteristics of Arctic winter Sea Ice from Satellite Multispectral Microwave Observations. J. Geophys. Res. 91 (C1), 975–994. doi:10.1029/jc091ic01p00975
Comiso, J. C., Gersten, R. A., Stock, L. V., Turner, J., Perez, G. J., and Cho, K. (2017). Positive Trend in the Antarctic Sea Ice Cover and Associated Changes in Surface Temperature. J. Clim. 30 (6), 2251–2267. doi:10.1175/jcli-d-16-0408.1
Comiso, J. C., and Zwally, H. J. (1984). Concentration Gradients and Growth/decay Characteristics of the Seasonal Sea Ice Cover. J. Geophys. Res. 89 (C5), 8081–8103. doi:10.1029/jc089ic05p08081
Dee, D. P., Uppala, S. M., Simmons, A. J., Berrisford, P., Poli, P., Kobayashi, S., et al. (2011). The ERA-Interim Reanalysis: Configuration and Performance of the Data Assimilation System. Q.J.R. Meteorol. Soc. 137 (656), 553–597. doi:10.1002/qj.828
Downes, S. M., Farneti, R., Uotila, P., Griffies, S. M., Marsland, S. J., Bailey, D., et al. (2015). An Assessment of Southern Ocean Water Masses and Sea Ice during 1988-2007 in a Suite of Interannual CORE-II Simulations. Ocean Model. 94, 67–94. doi:10.1016/j.ocemod.2015.07.022
Ducklow, H., Clarke, A., Dickhut, R., Doney, S. C., Geisz, H., Huang, K., et al. (2012). “The marine Ecosystem of the Western Antarctic Peninsula,” in Antarctica: An Extreme Environment in a Changing World. Editors A. Rogers, N. Johnston, A. Clarke, and E. Murphy. First Edn. (Oxford: Blackwell Publishing Ltd).
Eayrs, C., Holland, D., Francis, D., Wagner, T., Kumar, R., and Li, X. (2019). Understanding the Seasonal Cycle of Antarctic Sea Ice Extent in the Context of Longer‐Term Variability. Rev. Geophys. 57 (3), 1037–1064. doi:10.1029/2018rg000631
Enomoto, H., and Ohmura, A. (1990). The Influences of Atmospheric Half-Yearly Cycle on the Sea Ice Extent in the Antarctic. J. Geophys. Res. 95 (C6), 9497–9511. doi:10.1029/JC095iC06p09497
Ezraty, R., Girard-Ardhuin, F., Piollé, J. F., Kaleschke, L., and Heygster, G. (2007). “Arctic and Antarctic Sea Ice Concentration and Arctic Sea Ice Drift Estimated from Special Sensor Microwave Data,” in Département d’Océanographie Physique et Spatiale, IFREMER, Brest, France and University of Bremen Germany (IFREMER), 2.
Farneti, R., Downes, S. M., Griffies, S. M., Marsland, S. J., Behrens, E., Bentsen, M., et al. (2015). An Assessment of Antarctic Circumpolar Current and Southern Ocean Meridional Overturning Circulation during 1958-2007 in a Suite of Interannual CORE-II Simulations. Ocean Model. 93, 84–120. doi:10.1016/j.ocemod.2015.07.009
Frew, R. C., Feltham, D. L., Holland, P. R., and Petty, A. A. (2019). Sea Ice-Ocean Feedbacks in the Antarctic Shelf Seas. J. Phys. Oceanography 49 (9), 2423–2446. doi:10.1175/jpo-d-18-0229.1
Goosse, H., and Zunz, V. (2014). Decadal Trends in the Antarctic Sea Ice Extent Ultimately Controlled by Ice-Ocean Feedback. The Cryosphere 8 (2), 453–470. doi:10.5194/tc-8-453-2014
Haid, V., Iovino, D., and Masina, S. (2017). Impacts of Freshwater Changes on Antarctic Sea Ice in an Eddy-Permitting Sea-Ice-Ocean Model. The Cryosphere 11 (3), 1387–1402. doi:10.5194/tc-11-1387-2017
Hobbs, W. R., Massom, R., Stammerjohn, S., Reid, P., Williams, G., and Meier, W. (2016). A Review of Recent Changes in Southern Ocean Sea Ice, Their Drivers and Forcings. Glob. Planet. Change 143, 228–250. doi:10.1016/j.gloplacha.2016.06.008
Holland, P. R., Bruneau, N., Enright, C., Losch, M., Kurtz, N. T., and Kwok, R. (2014). Modeled Trends in Antarctic Sea Ice Thickness. J. Clim. 27 (10), 378. doi:10.1175/jcli-d-13-00301.1
Holland, P. R., and Kwok, R. (2012). Wind-driven Trends in Antarctic Sea-Ice Drift. Nat. Geosci 5 (12), 872–875. doi:10.1038/ngeo1627
Holland, P. R. (2014). The Seasonality of Antarctic Sea Ice Trends. Geophys. Res. Lett. 41 (12), 4230–4237. doi:10.1002/2014gl060172
Holmes, C. R., Holland, P. R., and Bracegirdle, T. J. (2019). Compensating Biases and a Noteworthy Success in the CMIP5 Representation of Antarctic Sea Ice Processes. Geophys. Res. Lett. 46 (8), 4299–4307.
Iovino, D., Selivanova, J., Lavergne, T., Cipollone, A., Masina, S., and Garric, G. (2022). Changes in the Antarctic Marginal Ice Zone, in Copernicus Marine Service Ocean State Report, Issue 6. J. Oper. Oceanography 1, 1. accepted.
Ivanova, N., Johannessen, O. M., Pedersen, L. T., and Tonboe, R. T. (2014). Retrieval of Arctic Sea Ice Parameters by Satellite Passive Microwave Sensors: A Comparison of Eleven Sea Ice Concentration Algorithms. IEEE Trans. Geosci. Remote Sensing 52 (11), 7233–7246. doi:10.1109/tgrs.2014.2310136
Ivanova, N., Pedersen, L. T., Tonboe, R. T., Kern, S., Heygster, G., Lavergne, T., et al. (2015). Inter-comparison and Evaluation of Sea Ice Algorithms: towards Further Identification of Challenges and Optimal Approach Using Passive Microwave Observations. The Cryosphere 9 (5), 1797–1817. doi:10.5194/tc-9-1797-2015
Jackson, L. C., Peterson, K. A., Roberts, C. D., and Wood, R. A. (2016). Recent Slowing of Atlantic Overturning Circulation as a Recovery from Earlier Strengthening. Nat. Geosci 9 (7), 518–522. doi:10.1038/ngeo2715
Kennicutt, M. C., Chown, S. L., Cassano, J. J., Liggett, D., Peck, L. S., Massom, R., et al. (2015). A Roadmap for Antarctic and Southern Ocean Science for the Next Two Decades and beyond. Antartic Sci. 27 (1), 3–18. doi:10.1017/s0954102014000674
Kohout, A. L., Williams, M. J. M., Dean, S. M., and Meylan, M. H. (2014). Storm-induced Sea-Ice Breakup and the Implications for Ice Extent. Nature 509 (7502), 604–607. doi:10.1038/nature13262
Lavergne, T., Sørensen, A. M., Kern, S., Tonboe, R., Notz, D., Aaboe, S., et al. (2019). Version 2 of the EUMETSAT OSI SAF and ESA CCI Sea-Ice Concentration Climate Data Records. The Cryosphere 13 (1), 49–78. doi:10.5194/tc-13-49-2019
Lellouche, J.-M., Le Galloudec, O., Drévillon, M., Régnier, C., Greiner, E., Garric, G., et al. (2013). Evaluation of Global Monitoring and Forecasting Systems at Mercator Océan. Ocean Sci. 9 (1), 57–81. doi:10.5194/os-9-57-2013
MacLachlan, C., Arribas, A., Peterson, K. A., Maidens, A., Fereday, D., Scaife, A. A., et al. (2015). Global Seasonal Forecast System Version 5 (GloSea5): a High-Resolution Seasonal Forecast System. Q.J.R. Meteorol. Soc. 141, 1072–1084. doi:10.1002/qj.2396
Maksym, T., Stammerjohn, S., Ackley, S., and Massom, R. (2012). Antarctic Sea Ice-A Polar Opposite? oceanog 25 (3), 140–151. doi:10.5670/oceanog.2012.88
Manucharyan, G. E., and Thompson, A. F. (2017). Submesoscale Sea Ice‐Ocean Interactions in Marginal Ice Zones. J. Geophys. Res. Oceans 122, 9455–9475. doi:10.1002/2017JC012895
Masina, S., Storto, A., Ferry, N., Valdivieso, M., Haines, K., Balmaseda, M., et al. (2015). An Ensemble of Eddy-Permitting Global Ocean Reanalyses from the MyOcean Project. Clim. Dyn. 49, 813–841. doi:10.1007/s00382-015-2728-5
Masina, S., and Storto, A. (2017). Reconstructing the Recent Past Ocean Variability: Status and Perspective. J Mar. Res. 75 (6), 727–764. doi:10.1357/002224017823523973
Massom, R. A., and Stammerjohn, S. E. (2010). Antarctic Sea Ice Change and Variability - Physical and Ecological Implications. Polar Sci. 4 (2), 149–186. doi:10.1016/j.polar.2010.05.001
Meehl, G. A., Arblaster, J. M., Bitz, C. M., Chung, C. T. Y., and Teng, H. (2016). Antarctic Sea-Ice Expansion between 2000 and 2014 Driven by Tropical Pacific Decadal Climate Variability. Nat. Geosci 9 (8), 590–595. doi:10.1038/ngeo2751
Meier, W. N., Fetterer, F., Savoie, M., Mallory, S., Duerr, R., and Stroeve, J. (2017). NOAA/NSIDC Climate Data Record of Passive Microwave Sea Ice Concentration. version 3. Boulder, Colorado USA: NSIDC: National Snow and Ice Data Center.
Meier, W. N., Peng, G., Scott, D. J., and Savoie, M. H. (2014). Verification of a New NOAA/NSIDC Passive Microwave Sea-Ice Concentration Climate Record. Polar Res. 33 (1), 21004. doi:10.3402/polar.v33.21004
Meier, W. N., and Stewart, J. S. (2019). Assessing Uncertainties in Sea Ice Extent Climate Indicators. Environ. Res. Lett. 14 (3), 035005. doi:10.1088/1748-9326/aaf52c
Meylan, M. H., Bennetts, L. G., and Kohout, A. L. (2014). In Situ measurements and Analysis of Ocean Waves in the Antarctic Marginal Ice Zone. Geophys. Res. Lett. 41, 5046–5051. doi:10.1002/2014GL060809
Onarheim, I. H., Eldevik, T., Smedsrud, L. H., and Stroeve, J. C. (2018). Seasonal and Regional Manifestation of Arctic Sea Ice Loss. J. Clim. 31 (12), 4917–4932. doi:10.1175/jcli-d-17-0427.1
Palmer, M. D., Roberts, C. D., Balmaseda, M., Chang, Y.-S., Chepurin, G., Ferry, N., et al. (2017). Ocean Heat Content Variability and Change in an Ensemble of Ocean Reanalyses. Clim. Dyn. 49 (3), 909–930. doi:10.1007/s00382-015-2801-0
Parkinson, C. L., and Cavalieri, D. J. (2012). Arctic Sea Ice Variability and Trends, 1979–2010. The Cryosphere 6 (4), 881–889. doi:10.5194/tc-6-871-2012
Parkinson, C. L. (2019). A 40-y Record Reveals Gradual Antarctic Sea Ice Increases Followed by Decreases at Rates Far Exceeding the Rates Seen in the Arctic. Proc. Natl. Acad. Sci. USA 116 (29), 14414–14423. doi:10.1073/pnas.1906556116
Pauling, A. G., Smith, I. J., Langhorne, P. J., and Bitz, C. M. (2017). Time‐dependent Freshwater Input from Ice Shelves: Impacts on Antarctic Sea Ice and the Southern Ocean in an Earth System Model. Geophys. Res. Lett. 44 (20), 10–454. doi:10.1002/2017gl075017
Perovich, D. K., and Jones, K. F. (2014). The Seasonal Evolution of Sea Ice Floe Size Distribution. J. Geophys. Res. Oceans 119, 8767–8777. doi:10.1002/2014JC010136
Riihelä, A., Bright, R. M., and Anttila, K. (2021). Recent Strengthening of Snow and Ice Albedo Feedback Driven by Antarctic Sea-Ice Loss. Nat. Geosci. 14, 832–836. doi:10.1038/s41561-021-00841-x
Roach, L. A., Dean, S. M., and Renwick, J. A. (2018). Consistent Biases in Antarctic Sea Ice Concentration Simulated by Climate Models. The Cryosphere 12 (1), 365–383. doi:10.5194/tc-12-365-2018
Roach, L. A., Dörr, J., Holmes, C. R., Massonnet, F., Blockley, E. W., Notz, D., et al. (2020). Antarctic Sea Ice Area in CMIP6. Geophys. Res. Lett. 47, e2019GL086729. doi:10.1029/2019GL086729
Rolph, R. J., Feltham, D. L., and Schröder, D. (2020). Changes of the Arctic Marginal Ice Zone during the Satellite Era. The Cryosphere 14 (6), 1971–1984. doi:10.5194/tc-14-1971-2020
Serreze, M. C., and Stroeve, J. (2015). Arctic Sea Ice Trends, Variability and Implications for Seasonal Ice Forecasting. Phil. Trans. R. Soc. A. 373 (2045), 20140159. doi:10.1098/rsta.2014.0159
Shu, Q., Wang, Q., Song, Z., Qiao, F., Zhao, J., Chu, M., et al. (2020). Assessment of Sea Ice Extent in CMIP6 with Comparison to Observations and CMIP5. Geophys. Res. Lett. 47 (9), e2020GL087965. doi:10.1029/2020gl087965
Stammerjohn, S. E., Martinson, D. G., Smith, R. C., Yuan, X., and Rind, D. (2008). Trends in Antarctic Annual Sea Ice Retreat and advance and Their Relation to El Niño–Southern Oscillation and Southern Annular Mode Variability. J. Geophys. Res. Oceans 113 (C3), 1. doi:10.1029/2007jc004269
Storto, A., Masina, S., Balmaseda, M., Guinehut, S., Xue, Y., Szekely, F., et al. (2017). Steric Sea Level Variability (1993-2010) in an Ensemble of Ocean Reanalyses and Objective Analyses. Clim. Dyn. 49 (3), 709–729. doi:10.1007/s00382-015-2554-9
Storto, A., Masina, S., and Navarra, A. (2016). Evaluation of the CMCC Eddy‐permitting Global Ocean Physical Reanalysis System (C‐GLORS, 1982-2012) and its Assimilation Components. Q.J.R. Meteorol. Soc. 142, 738–758. doi:10.1002/qj.2673
Storto, A., Masina, S., Simoncelli, S., Iovino, D., Cipollone, A., Drevillon, M., et al. (2019). The Added Value of the Multi-System Spread Information for Ocean Heat Content and Steric Sea Level Investigations in the CMEMS GREP Ensemble Reanalysis Product. Clim. Dyn. 53 (1), 287–312. doi:10.1007/s00382-018-4585-5
Stroeve, J. C., Jenouvrier, S., Campbell, G. G., Barbraud, C., and Delord, K. (2016). Mapping and Assessing Variability in the Antarctic Marginal Ice Zone, Pack Ice and Coastal Polynyas in Two Sea Ice Algorithms with Implications on Breeding success of Snow Petrels. The Cryosphere 10 (4), 1823–1843. doi:10.5194/tc-10-1823-2016
Strong, C., and Rigor, I. G. (2013). Arctic Marginal Ice Zone Trending Wider in Summer and Narrower in winter. Geophys. Res. Lett. 40 (18), 4864–4868. doi:10.1002/grl.50928
Sutherland, B. R., and Balmforth, N. J. (2019). Damping of surface waves by floating particles. Physical Review Fluids 4 (1), 014804.
Tsamados, M., Feltham, D., Petty, A., Schroeder, D., and Flocco, D. (2015). Processes Controlling Surface, Bottom and Lateral Melt of Arctic Sea Ice in a State of the Art Sea Ice Model. Phil. Trans. R. Soc. A. 373, 20140167. doi:10.1098/rsta.2014.0167
Tsujino, H., Urakawa, L. S., Griffies, S. M., Danabasoglu, G., Adcroft, A. J., Amaral, A. E., et al. (2020). Evaluation of Global Ocean-Sea-Ice Model Simulations Based on the Experimental Protocols of the Ocean Model Intercomparison Project Phase 2 (OMIP-2). Geosci. Model. Dev. 13 (8), 3643–3708. doi:10.5194/gmd-13-3643-2020
Turner, J., Bracegirdle, T. J., Phillips, T., Marshall, G. J., and Hosking, J. S. (2013). An Initial Assessment of Antarctic Sea Ice Extent in the CMIP5 Models. J. Clim. 26 (5), 1473–1484. doi:10.1175/jcli-d-12-00068.1
Turner, J., Hosking, J. S., Bracegirdle, T. J., Marshall, G. J., and Phillips, T. (2015). Recent Changes in Antarctic Sea Ice. Phil. Trans. R. Soc. A. 373, 20140163. doi:10.1098/rsta.2014.0163
Uotila, P., Goosse, H., Haines, K., Chevallier, M., Barthélemy, A., Bricaud, C., et al. (2019). An Assessment of Ten Ocean Reanalyses in the Polar Regions. Clim. Dyn. 52 (3), 1613–1650. doi:10.1007/s00382-018-4242-z
Valdivieso, M., Haines, K., Balmaseda, M., Chang, Y.-S., Drevillon, M., Fujii, Y., et al. (2017). An Assessment of Air-Sea Heat Fluxes from Ocean and Coupled Reanalyses. Clim. Dyn. 49 (3), 983–1008. doi:10.1007/s00382-015-2843-3
Venables, H. J., and Meredith, M. P. (2014). Feedbacks between Ice Cover, Ocean Stratification, and Heat Content in Ryder Bay, Western Antarctic Peninsula. J. Geophys. Res. Oceans 119 (8), 5323–5336. doi:10.1002/2013jc009669
Vichi, M. (2021). A Statistical Definition of the Antarctic Marginal Ice Zone. Cryosphere Discuss. 1, 1–23. [preprint].
Vichi, M., Eayrs, C., Alberello, A., Bekker, A., Bennetts, L., Holland, D., et al. (2019). Effects of an Explosive Polar Cyclone Crossing the Antarctic Marginal Ice Zone. Geophys. Res. Lett. 46 (11), 5948–5958. doi:10.1029/2019gl082457
Wachter, P., Reiser, F., Friedl, P., and Jacobeit, J. (2021). A New Approach to Classification of 40 Years of Antarctic Sea Ice Concentration Data. Int. J. Climatology 41, E2683–E2699. doi:10.1002/joc.6874
Wang, M. J., Liu, T. T., Yang, Z. J., Wu, B., and Zhu, X. (2021). Variation of Antarctic Marginal Ice Zone Extent (1989–2019). Adv. Polar Sci. 32 (4), 341–355. doi:10.13679/j.advps.2021.0042
Keywords: Antarctic sea ice, marginal ice zone, pack ice, ocean reanalyses, GREP
Citation: Iovino D, Selivanova J, Masina S and Cipollone A (2022) The Antarctic Marginal Ice Zone and Pack Ice Area in CMEMS GREP Ensemble Reanalysis Product. Front. Earth Sci. 10:745274. doi: 10.3389/feart.2022.745274
Received: 21 July 2021; Accepted: 17 January 2022;
Published: 10 February 2022.
Edited by:
Stefanie Arndt, Alfred Wegener Institute Helmholtz Centre for Polar and Marine Research (AWI), GermanyReviewed by:
Alexander Fraser, University of Tasmania, AustraliaLu Zhou, University of Gothenburg, Sweden
Copyright © 2022 Iovino, Selivanova, Masina and Cipollone. This is an open-access article distributed under the terms of the Creative Commons Attribution License (CC BY). The use, distribution or reproduction in other forums is permitted, provided the original author(s) and the copyright owner(s) are credited and that the original publication in this journal is cited, in accordance with accepted academic practice. No use, distribution or reproduction is permitted which does not comply with these terms.
*Correspondence: Doroteaciro Iovino, ZG9yb3RlYS5pb3Zpbm9AY21jYy5pdA==; Julia Selivanova, aXVsaWlhLnNlbGl2YW5vdmFAdW5pYm8uaXQ=
†These authors share first authorship