- 1Geomathematics Key Laboratory of Sichuan Province, College of Mathematics and Physics, Chengdu University of Technology, Chengdu, China
- 2State Key Laboratory of Geohazard Prevention and Geoenvironment Protection, Chengdu University of Technology, Chengdu, China
- 3College of Earth Sciences, Chengdu University of Technology, Chengdu, China
- 4Sichuan Geological Survey, Chengdu, China
- 5Aerospace Information Research Institute, Chinese Academy of Sciences, Beijing, China
- 6Development Research Center of China Geological Survey, Beijing, China
Multispectral-sensor images are advantageous in terms of discriminating major lithologies due to their high spatial resolution and intermediate spectral resolution, in addition to their low cost and high accessibility in comparison to hyperspectral images. In this study, Landsat-5 Thematic Mapper ™ and the Advanced Spaceborne Thermal Emission and Reflection Radiometer (ASTER) data—which are the most widely used multispectral data for the discrimination of the mixed rock units—are utilized to identify basalts in our study area. Further, prior knowledge regarding basalt-distribution areas in our study region is obtained from the geological-survey results conducted by the Sichuan Geological Survey at 2005, which is used as the reference of correction to assess our identified results. Small portions of this prior area of basalt distribution were verified through field checks, which were then determined as sites for use as training data for remote-sensing imagery. Three supervised-classification algorithms within ENVI 5.3—k-nearest neighbors (KNN), maximum likelihood classification (MLC), and support vertical machine (SVM)—were utilized for model identification. As a result, six models were constructed, including the KNN prediction of basalts by ASTER images, SVM prediction by ASTER, MLC prediction by ASTER, KNN prediction by Landsat-5 images, SVM prediction by Landsat-5, and MLC prediction by Landsat-5. The performances of the six models, in terms of precision and accuracy, show that the optimum model is Landsat-5 by SVM, with a precision of 70.92% and accuracy of 99.97%, followed by the ASTER by SVM model, with a precision of 67.72% and accuracy of 99.89% and the Landsat-5 by KNN model, with a precision of 57.23% and accuracy of 99.85%.
1 Introduction
Generally, various rock types—with their own specific rock-forming minerals—have their own reflectance signatures, and can thus be discriminated based on their spectral characteristics using different spectral wavelengths of optical remote-sensing images (Kang et al., 2001; Corumluoglu et al., 2015; Hassan and Ramadan, 2015). Satellite images have been used to map the Earth for decades (Dou et al., 2015a; Dou et al., 2015b; Lillesand et al., 2015; Moghtaderi et al., 2022), and multispectral sensors are widely used for lithological discrimination in areas where rock units are exposed (Haselwimmer et al., 2010; Nair and Mathew, 2012; Arivazhagan and Anbazhagan, 2017). In comparison to hyperspectral imagery, multispectral imagery is more advantageous in terms of classifying major lithologies (e.g., basalt, granite, and rhyolite) due to its high quality, high spatial resolution, low cost, and high accessibility (Ge et al., 2018). Nevertheless, hyperspectral imagery is more suitable for the finer classifications of rocks (e.g., tholeiitic basalt, alkali basalt, etc.).
Landsat Thematic Mapper™, the Advanced Spaceborne Thermal Emission and Reflection Radiometer (ASTER), and Sentinel-2 are the most widely used multispectral sensors for the discrimination of mixed rock units. Of the three, ASTER and Sentinel-2 are regarded to be more suitable due to their increased availability of infrared bands and better spatial resolution than the Landsat Thematic Mapper (Gomez et al., 2005; Perry and Kruse, 2010; Ehlers and Klonus, 2014). In this study, we use the geological survey of the basalt distribution by the Sichuan Geological Survey in 2005 as prior knowledge to assess the remote-sensing identifications. Further, the Sentinel-2 sensor began collecting images after 2015; therefore, we did not use Sentinel-2 imageries here considering that the vegetation coverage changed significantly in the geological-survey area from 2005 to 2015. Instead, we used ASTER and Landsat-5 TM images collected from 2003 to 2007 and in 2004. Three machine learning methods, including k-nearest neighbors (KNN), maximum likelihood classification (MLC), and support vertical machine (SVM), were compared to identify basalts based on both ASTER and Landsat-5 data. Therefore, the prediction results of six models, including the KNN prediction of basalts by ASTER images, SVM prediction by ASTER, MLC prediction by ASTER, KNN prediction by Landsat-5 images, SVM prediction by Landsat-5, and MLC prediction by Landsat-5, were compared based on the area of bare basalt in the geological-survey scope, to calculate the performance metrics (precision and accuracy). The geological-survey basalt is partly covered by vegetation that is thus hard to be identified by remote-sensing imageries, so we just used the bare-basalt distribution of the geological-survey, that is without vegetation coverage, to assess the identified results. The bare-basalt distribution is extracted through land-use classification for the geological-survey scope (bare land and vegetation).
Furthermore, the flood basalts are the signature feature of the Emeishan Large Igneous Province (LIP) in spite that there are also ultramafic and silicic volcanic rocks and layered mafic-ultramafic and silicic plutonic rocks exposed (Shellnutt, 2014). There were much substantial researches on ELIP in the past, and the reason why it is of particular interest is that it contains numerous world-class orthomagmatic Fe-Ti-V deposits and series of smaller economically important Ni-Cu-(OGE) sulphide deposits but also is contemporaneous with the Late Capitanian (∼260 Ma) mass extinction (Zhou et al., 2002; Zhang et al., 2006; Ganino and Arndt 2009). Numerous researches on ELIP in the past covered a wide scope of geology, paleomagnetism, geochronology, geochemistry, biostratigraphy and so on. But remote sensing technology was seldom used in the researches on ELIP flood basalts. This paper conducted the studies of remote-sensing identification on flood basalts in the Panxi part of ELIP.
2 Materials and methods
2.1 Study area
The study area filled in red in Figure 1, including the three counties of Miyi, Huili, and Ningnan, is located in the Panzhihua-Xichang (Panxi) region, Sichuan Province, SW China, which is part of the Emeishan LIP, and lies between the latitudes of 26°02′54″N to 27°18′33″N and longitudes of 101°59′53″E to 102°54′50″E, covering an approximate area of 8,328 km2. The Panxi region lies in the central-western part of the Emeishan LIP, where the flood basalts include high-Ti and Low-Ti lavas in addition to many other continental flood basalts (Xiao et al., 2004; Zhong et al., 2005). Magmatic Fe-Ti oxide deposits are documented in several layered intrusions in this region and, thus, account for a total ore reserve of ∼7,209 Mt total Fe, ∼559 Mt TiO2, and ∼17.4 Mt V2O5 (Vapnik, 1982).
2.2 Data
2.2.1 Geological-survey basalts
The regional geological map of basalt distribution was provided by the Sichuan Geological Survey (Figure 2). The geological map, at a scale of 1:50,0000, was created in 2005. This map served as prior knowledge for the flood basalt distribution in our study area and to determine the sampling sites and to assess our identifications by remote-sensing imageries.
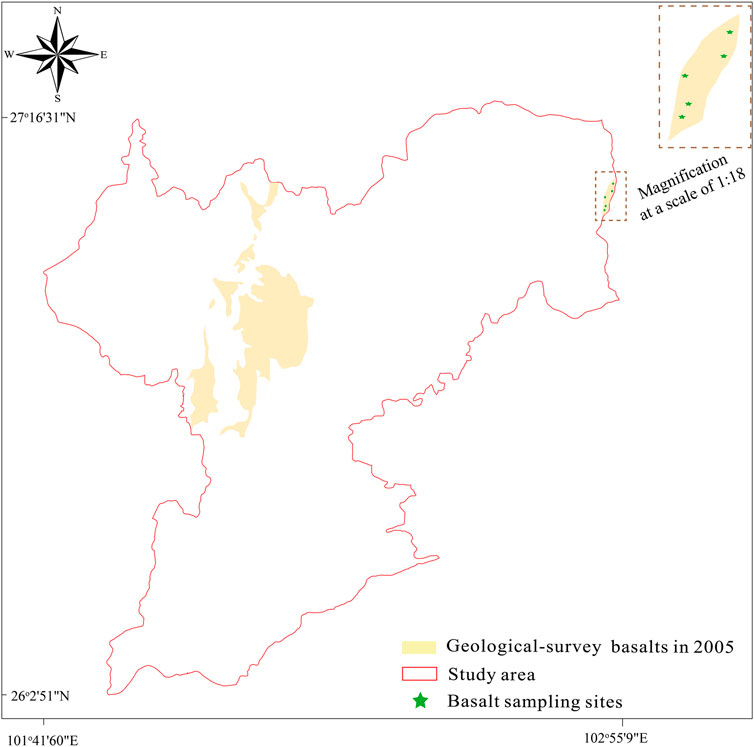
FIGURE 2. Location of geological-survey basalts in 2005 by the Sichuan Geological Survey and the sampling sites of basalts in 2022.
2.2.2 Sampling sites
The five sampling sites of basalts (Figure 2) were initially selected based on both the prior knowledge of the location of geological-survey basalts in 2005 and the visual interpretation of optical remote-sensing imagery; this was verified through field surveys in 2022. The five sampling sites have the central locations of (27°07′59″N, 102°54′22″E), (27°07′05″N,102°54′14″E), (27°06′14″N, 102°53′20″E), (27°05′10″N, 102°53′24″E), and (27°43′59″N, 102°53′17″E). The areas of the five sampling sites on remote-sensing imageries are utilized as the training data in our modeling analyses.
2.2.3 Landsat-5 and ASTER imagery
Developed by NASA, Landsat-5 is a low Earth orbit satellite that was launched on 1 March 1984, and decommissioned on 5 June 2013, and carried multispectral scanner (MSS) and the thematic mapper (TM) instruments. The Landsat-5 MSS supplies four bands, from .5 to 1.1 μm, with a 60-m spatial resolution; the Landsat-5 TM supplies seven bands, with a 30-m spatial resolution (Markham et al., 1998; Chander and Markham, 2003). In our study, we use Landsat-5 TM imagery.
The Advanced Spaceborne Thermal Emission and Reflection Radiometer (ASTER) is a high spatial resolution instrument on the Terra satellite launched by NASA in 1999, and has been collecting data since February 2000. ASTER is a 15-m, 14-band multispectral resolution instrument, including six shortwave infrared (SWIR) bands, three visible and near-infrared (VNIR) bands, and five thermal infrared (TIR) bands (Argany et al., 2018). It can be used for land cover and change detection, calibration, validation, and land-surface studies. +
Generally, we used images around 2005, when the geological survey of basalt was conducted. Landsat-5 and ASTER were utilized as comparison data here. Three Landsat-5 images in 2004 covered our study area, and eleven ASTER images—from 2003 to 2007—covered our study area. All imagery techniques mentioned herein are described in Table 1. Both Landsat-5 and ASTER images were obtained through the U.S. Geological Survey Earth Resources Observation and Science Center (EROS) (http://earthexplorer.usgs.gov). The cloud coverage of these images ranged approximately from 2% to 8%, which is a relatively low level.
2.3 Methods
2.3.1 Land-use classification
Generally, the remote-sensing identification of lithologic units prefers bare land, and is less efficient when detecting lithology under dense vegetation. However, geological field surveys by geological experts can help observe lithology under vegetation. The geological-survey mapping of basalts in 2005 contains vegetated subareas. Here, we used Landsat-5 data in 2004 for land-use classification and, thus, extracted the bare-land type in our study area (Figure 3A). Figure 3B shows a magnification of the coverage of geological-survey basalts with land-use types.
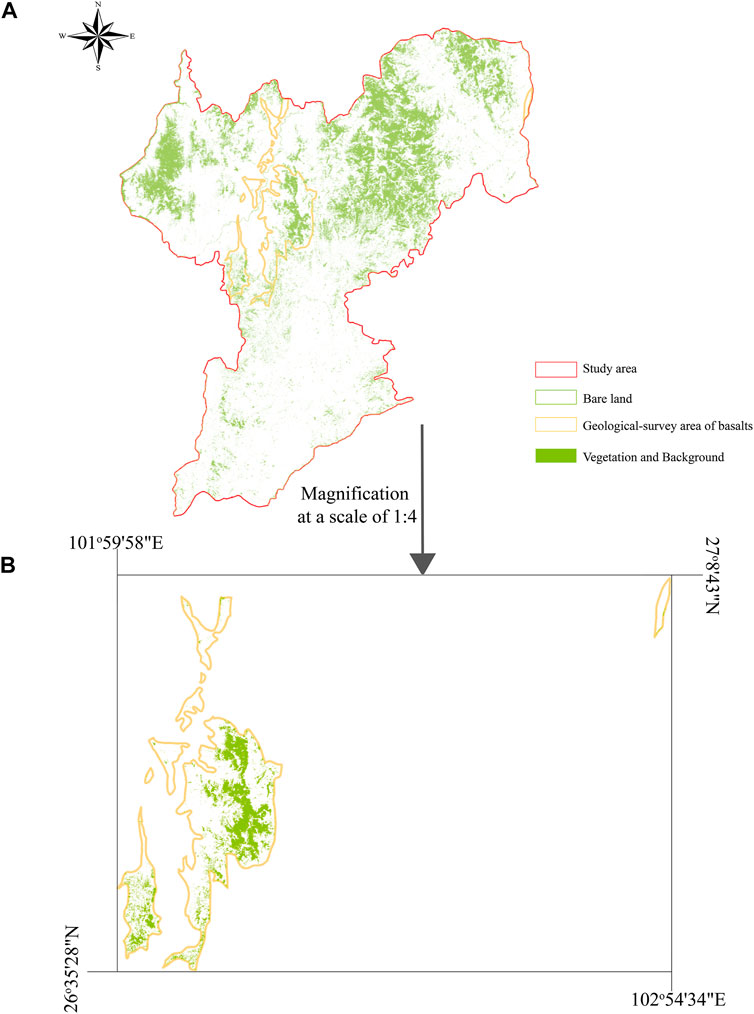
FIGURE 3. (A) Land-use type distribution in the study area. (B) The land-use type distribution in the area of geological-survey basalts.
2.3.2 Supervised-classification algorithms
2.3.2.1 KNN
The k-nearest neighbors (KNN) algorithm is a non-parametric supervised learning method (Altman, 1992). When using KNN for the binary classification of remote-sensing images in our study, the output is either a basalt class or non-basalt class. In this study, an unknown object is classified by a plurality vote of its neighbors that are metricized in the spectral–spatial distance. Here, we calculate the spectral–spatial distances of the unknown object with all training samples of two categories (basalt category and non-basalt category), and the five nearest neighbors to vote for classification: if three or more of the five nearest neighbors are related to basalt, then an unknown sample is classified as basalt, and vice versa.
2.3.2.2 MLC
Maximum likelihood classification (MLC) is a supervised classification method based on Bayes theorem, which makes use of a discriminant function to assign a pixel to the class with the highest likelihood (Ahmad and Quegan 2012a). The advantage of MLC as a parametric classifier is that it takes into account the variance–covariance within the class distributions and for normally distributed data (Ahmad and Quegan, 2012a; Ahmad and Quegan, 2012b).
2.3.2.3 SVM
support vertical machine (SVM), as a non-parametric supervised machine learning algorithm, is often used in binary-classification problems (Merghadi et al., 2020). SVM is one of the most robust prediction methods, based on the statistical learning frameworks proposed by Vapnik (1982), Vapnik (1995). An SVM training algorithm builds a model that assigns new examples to one category or the other, making it a non-probabilistic binary linear classifier. SVM maps training examples to points in space to maximize the width of the gap between the two categories.
2.3.3 Model evaluation metrics
To evaluate the performance of the three aforementioned supervised-classification methods, two metrics were utilized here: precision and accuracy. Precision refers to the correctness of basalt identification based on the geological-survey mapping basalts as shown in Figure 3, and accuracy is the ratio of the number of correct predictions made to all predictions.
where 1) FPs (false positives) mean that pixels belonging to non-basalt were misclassified as belonging to basalt, 2) FNs (false negatives) mean that pixels belonging to basalt were misclassified as belonging to non-basalt, 3) TPs (true positives) mean that pixels belonging to basalt were correctly classified as belonging to basalt, and 4) TNs (true negatives) mean that pixels belonging to non-basalt were correctly classified as belonging to non-basalt (Costa et al., 2019; Zeng et al., 2022).
3 Results
We analyzed three supervised-classification algorithms and two kinds of remote-sensing imageries, as mentioned previously, to identify basalts using the training images. These training images are part of the Landsat-5 imagery data located at the sampling sites, as described in Figure 2, when identification uses Landsat-5 images; they are also part of ASTER imagery, when identification uses ASTER images. In total, six identification methods are used: the KNN prediction of basalts by ASTER images, SVM prediction by ASTER, MLC prediction by ASTER, KNN prediction of basalts by Landsat-5 images, SVM prediction by Landsat-5, and MLC prediction by Landsat-5. All identifications are carried out using ENVI 5.3.
When using MLC, the probability threshold is set at 99%. This threshold is a probability minimum for inclusion in a class, and the ENVI does not classify pixels with a value lower than this value. As a binary-classification method, we tried changing the probability threshold of MLC from 51% to 99.9%; it was found that a threshold below 99% led to anomalous data that deviated from prior-knowledge basalt areas.
The results of the six identification methods are shown in Figure 4. Generally, the identified locations of basalts are concentrated in the prior areas of geological-survey basalt, which indicates that the methods of identification used (including the selections of both remote-sensing images and algorithms) are reasonable. Furthermore, in past research, remote-sensing images for identifying the lithology exhibit poor performance with significant vegetation coverage (Grebby et al., 2014). Therefore, in our study, the samples used for training are chosen to be bare and without vegetation coverage; here, remote-sensing images simply identify basalts that are exposed and cannot detect basalts under vegetation. We overlap bare land (Figure 3) with the identified results (Figure 4), taking the area of geological-survey basalts as prior knowledge to calculate the precision and accuracy for the six identification methods. Figure 5A shows that how the three ASTER identification results fall into the prior area of basalts, and Figure 5B shows that how the three Landsat-5 identification results fall into the prior area of basalts. Assuming that the geological-survey basalt areas are correct, the performance metrics (including precision and accuracy) of the six identification methods are shown in Table 1.
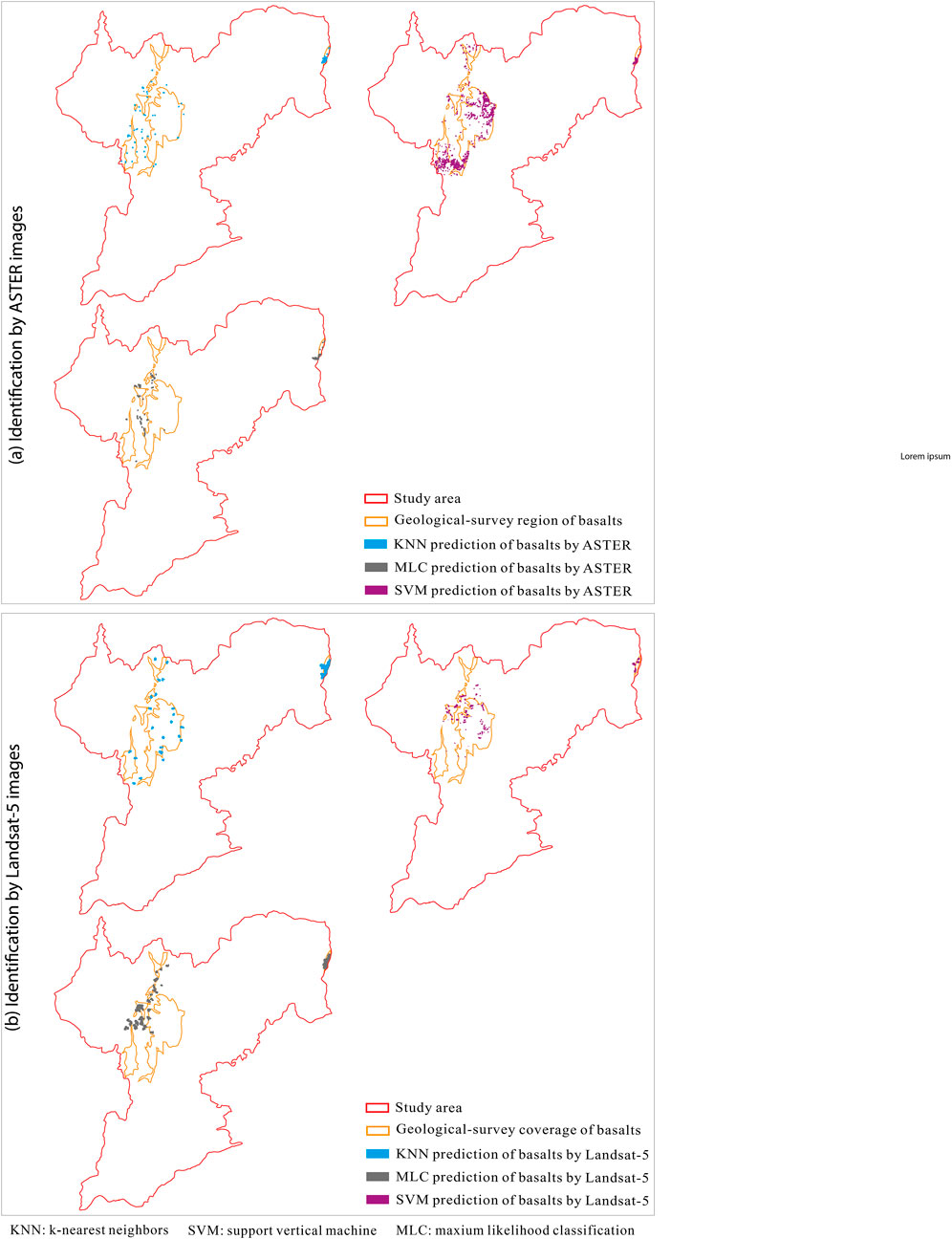
FIGURE 4. Results of basalt identification by the three algorithms respectively on Landsat-5 and ASTER imageries.
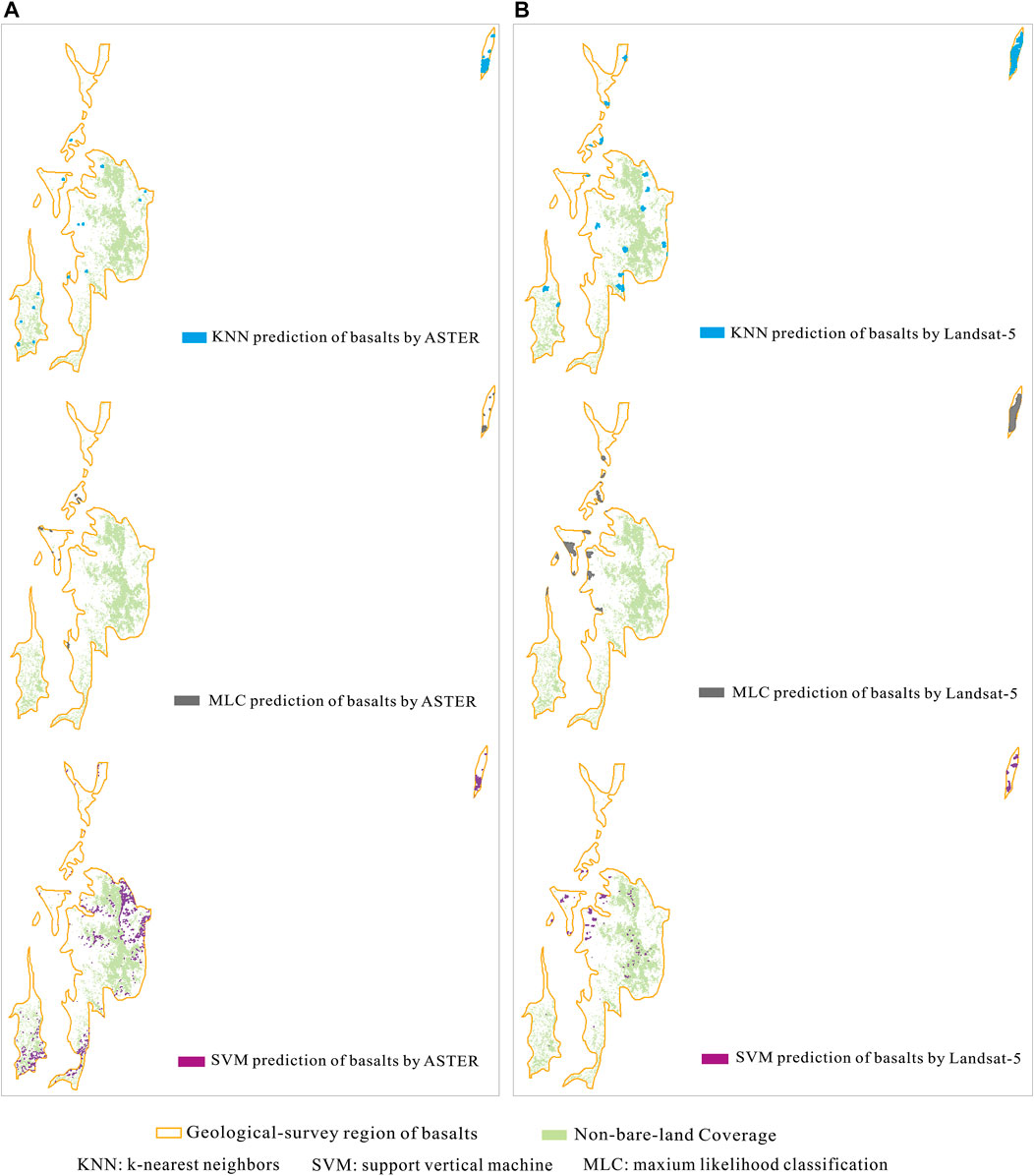
FIGURE 5. (A) Three identifications by ASTER falling into the scope of geological-survey basalts. (B) Three identifications by Landsat-5 falling into the scope of geological-survey basalts.
4 Discussion
As shown in Figure 5, most of the identified area falling into the geological-survey scope is on bare land, except a minor portion of the identified area from SVM by Landsat-5, which slightly overlaps with vegetation. It is inevitable that all methods exhibit certain errors, but the overall identification of basalts by remote-sensing images, with the training data of bare-basalt images, is still correct for bare land.
The three algorithms of KNN, MLC, and SVM are all object-oriented identifications; therefore, the identified basalts have many clusters with various sizes. Generally, the identified clusters by Landsat-5 show larger sizes than those by ASTER (Figure 4; Figure 5), possibly because Landsat-5 has a lower spatial resolution of 30 m for identification, than ASTER’s 15 m, which is better for our study area with the total area of 8328 km2.
Moreover, it seems that the SVM algorithm performs most effectively on both ASTER and Landsat-5 imageries than the other two algorithms, because the total identified area of basalts by SVM is slightly more. KNN performed very well on Landsat-5; MLC performed poorest on both ASTER and Landsat-5 imageries (Figure 5 and Table 2), compared to SVM and KNN. The SVM algorithm is essentially a two-category classifier of deep learning and is possibly more robust than the other two algorithms of simple machine learning. When the probability threshold of MLC is set lower, it produces results that deviate from prior knowledge; when it is set higher, it produces a smaller area of prediction. The error emergence of singular solutions possibly occurs when solving the covariance matrix in the MLC algorithm. KNN performs intermediately between SVM and MLC, and predicts more area based on Landsat-5 than based on ASTER, possibly only because the spatial resolution of Landsat-5 TM imagery is 30 m, in contrast to the 15-m resolution of ASTER imagery.
5 Conclusion
Although ASTER is widely used for the discrimination of mixed rock units, Landsat-5 data has advantages in certain cases, especially when the total area for identification is large. However, ASTER data would be more advantageous for more detailed identification in smaller areas. Furthermore, for machine learning algorithms for the supervised classification within ENVI, SVM may perform relatively more robustly.
Generally, in this study, we use both ASTER and Landsat-5 data for discrimination of the mixed rocks by three supervised-classification algorithms, and in the training, small-size training images are utilized. As a result, a total of six models are constructed. The modeling results by training small-size images are generally in accordance with the trends of the prior area of geological-survey basalt distributions. This verifies the capability of remote-sensing imagery for lithological discrimination and the capability of the SVM algorithm for deep learning in supervised classification. However, there are still issues in terms of how remote-sensing imagery can identify rocks with high vegetation coverage. Under the constraints of present remote-sensing technology, the only possibility may be to develop algorithms of deep learning neural networks.
Besides, this work of the remote-sensing identification of flood basalts in Panxi part of Emeishan LIP is significant, which will promote us to launch a wider-scope identification across Emeishan LIP in the future. Remote-sensing identification of flood basalts all over the Emeishan LIP will not only help map the distribution of flood basalts, but also help explore the world-class Fe-Ti-V deposits and Ni-Cu-(OGE) sulphide deposits that are both closely related to flood basalt distributions.
Data availability statement
The original contributions presented in the study are included in the article/Supplementary Material, further inquiries can be directed to the corresponding authors.
Author contributions
LZ: Conceptualization, methodology, investigation, formal analysis, writing-original draft; TL: Supervision, funding acquisition, validation, writing–review and editing; HH: Visualization, data curation; PZ: Funding acquisition, supervision; YH: Resource; LJ: Resource, writing–review and editing; YY: Visualization; SJ: Conceptualization, investigation, formal analysis, validation, project administration, writing–review and editing.
Funding
The funding for this work was supported by the National Natural Science Foundation of China (No. 42002294, No. 41972308, No. U19A20111, No. 42130719), China Geological Survey project (No. DD20221785), State Key Laboratory of Geohazard Prevention and Geoenvironment Pretection Research Project (SKLGP 2017Z001), Research Foundation for Talents of Chengdu University of Technology (10912-KYQD2021-07430), and the Second Tibetan Plateau Scientific Expedition and Research (2019QZKK0806)
Conflict of interest
The authors declare that the research was conducted in the absence of any commercial or financial relationships that could be construed as a potential conflict of interest.
Publisher’s note
All claims expressed in this article are solely those of the authors and do not necessarily represent those of their affiliated organizations, or those of the publisher, the editors and the reviewers. Any product that may be evaluated in this article, or claim that may be made by its manufacturer, is not guaranteed or endorsed by the publisher.
References
Ahmad, A., and Quegan, S. (2012a). Analysis of maximum likelihood classification on multispectral data. Appl. Math. Sci. 6, 6425–6436.
Ahmad, A., and Quegan, S. (2012b). “Analysis of maximum likelihood classification technique on Landsat 5 TM satellite data of tropical land covers,” in 2012 IEEE International Conference on Control System, Computing and Engineering, Penang, Malaysia, 23-25 November 2012, 280–285. doi:10.1109/ICCSCE.2012.6487156
Altman, N. S. (1992). An introduction to kernel and nearest-neighbor nonparametric regression. Am. Stat. 46, 175–185. doi:10.1080/00031305.1992.10475879
Argany, M., Ramezani, A., and Ahmadi, A. (2018). Determination of basalt zones using basalt extraction index (BEI) and ASTER image classification. Cogent. Geosci. 4 (1), 1466672. doi:10.1080/23312041.2018.1466672
Arivazhagan, S., and Anbazhagan, S.Centre for Geoinformatics and Planetary Studies, Periyar University, Salem – 636 011, India (2017). ASTER data analyses for lithological discrimination of sittampundi anorthositic complex, southern India. Geosciences Res. 2, 196–209. doi:10.22606/gr.2017.23005
Chander, G., and Markham, B. (2003). Revised Landsat-5 TM radiometric calibration procedures and postcalibration dynamic ranges. IEEE Trans. Geosci. Remote Sens. 41, 2674–2677. doi:10.1109/tgrs.2003.818464
Corumluoglu, O., Vural, A., and Asri, I. (2015). Determination of Kula basalts (geosite) in Turkey using remote sensing techniques. Arab. J. Geosci. 8, 10105–10117. doi:10.1007/s12517-015-1914-4
Costa, M. G. F., Campos, J. P. M., de Aquino, E. A. G., de Albuquerque Pereira, W. C., and Costa Filho, C. F. F. (2019). Evaluating the performance of convolutional neural networks with direct acyclic graph architectures in automatic segmentation of breast lesion in US images. Bmc. Med. Imaging. 19, 85. doi:10.1186/s12880-019-0389-2
Dou, J., Chang, K. T., Chen, S., Yunus, A. P., Liu, J. K., Xia, H., et al. (2015a). Automatic case-based reasoning approach for landslide detection: Integration of object-oriented image analysis and a genetic algorithm. Remote Sens. 7, 4318–4342. doi:10.3390/rs70404318
Dou, J., Li, X., Yunus, A. P., Paudel, U., Chang, K. T., Zhu, Z., et al. (2015b). Automatic detection of sinkhole collapses at finer resolutions using a multi-component remote sensing approach. Nat. Hazards 78, 1021–1044. doi:10.1007/s11069-015-1756-0
Ehlers, M., and Klonus, S. (2014). “Scale issues in multisensory image fusion,” in Scale issues in remote sensing. Editor Q. Weng (Hoboken, New Jersey: John Wiley & Sons), 13–33.
Ganino, C., and Arndt, N. T. (2009). Climate changes caused by degassing of sediments during the emplacement of large igneous provinces. Geology 37, 323–326. doi:10.1130/g25325a.1
Ge, W., Cheng, Q., Tang, Y., Jing, L., and Gao, C. (2018). Lithological classification using sentinel-2A data in the shibanjing ophiolite complex in inner Mongolia, China. Remote. Sens. 10, 638–722. doi:10.3390/rs10040638
Gomez, C., Delacourt, C., Allemand, P., Ledru, P., and Wackerle, R. (2005). Using ASTER remote sensing dataset for geological mapping, in Namibia. Phys. Chem. Earth. 30, 97–108. doi:10.1016/j.pce.2004.08.042
Grebby, S., Cunninghan, D., Tansey, K., and Naden, J. (2014). The impact of vegetation on lithological mapping using airborne multispectral data: A case study for the north troodos region, Cyprus. Remote. Sens. 6, 10860–10887. doi:10.3390/rs61110860
Haselwimmer, C. E., Riley, T. R., and Liu, J. G. (2010). Assessing the potential of multispectral remote sensing for lithological mapping on the Antarctic Peninsula: Case study from eastern Adelaide Island, Graham Land. Antarct. Sci. 22, 299–318. doi:10.1017/s0954102010000015
Hassan, S. M., and Ramadan, T. M. (2015). Mapping of the late Neoproterozoic Basement rocks and detection of the gold-bearing alteration zones at Abu Marawat-Semna area, Eastern Desert, Egypt using remote sensing data. Arab. J. Geosci. 8, 4641–4656. doi:10.1007/s12517-014-1562-0
Kang, K. K., Song, K. Y., Ahn, C. H., and Won, J. S. (2001). Reflectance of geological media by using a field spectrometer in the Ungsang area, Kyungsang Basin. Korean. J. remote. Sens. 17, 165–181.
Lillesand, T. M., Kieffer, R. W., and Chipman, J. W. (2015). Remote sensing and image interpretation. Hoboken: John Wiley & Sons.
Markham, B. L., Seiferth, J. C., Smid, J., and Barker, J. L. (1998). <title>Lifetime responsivity behavior of the Landsat-5 thematic mapper</title>. Proc. SPIE 3427, 420–431. doi:10.1117/12.328513
Merghadi, A., Yunus, A. P., Dou, J., Whiteley, J., ThaiPham, B., Bui, D. T., et al. (2020). Machine learning methods for landslide susceptibility studies: A comparative overview of algorithm performance. Earth-Science Rev. 207, 103225. doi:10.1016/j.earscirev.2020.103225
Moghtaderi, A., Moore, F., and Ranjbar, H. (2022). Testing ASTER and sentinel-2 MSI images to discriminate igneous and metamorphic rock units in the chadormalu paleocrater, central Iran. Can. J. Remote. Sens. 48, 214–238. doi:10.1080/07038992.2021.1997347
Nair, A., and Mathew, G. (2012). Lithological discrimination of the phenaimata felsic-mafic complex. Gujarat, India, using the advanced Spaceborne thermal emission and reflection radiometer (ASTER). Int. J. Remote. Sens. 33, 198–219. doi:10.1080/01431161.2011.591441
Perry, S., and Kruse, F. (2010). “ASTER data use in mining applications,” in Land remote sensing and global environmental change. Remote sensing and digital image processing. Editors B. Ramachandran, C. Justice, and M. Abrams (New York: Springer), 301–324.
Shellnutt, J. G. (2014). The Emeishan large igneous province: A synthesis. Geosci. Front. 5, 369–394.
Xiao, L., Xu, Y. G., Mei, H. J., Zheng, Y. F., He, B., and Pirajno, F. (2004). Distinct mantle sources of low-Ti and high-Ti basalts from the Western emeishan large igneous province, SW China: Implications for plume-lithosphere interaction. Earth. Planet. Sc. Lett. 228, 525–546. doi:10.1016/j.epsl.2004.10.002
Zeng, L., Li, T. B., Wang, X. K., Chen, L., Zeng, P., and Herrin, J. S. (2022). UNetGE: A U-Net-based software at automatic grain extraction for image analysis of the grain size and shape characteristics. Sensors 22, 5565. doi:10.3390/s22155565
Zhang, Z., Mahoney, J. J., Mao, J., and Wang, F. (2006). Geochemistry of picritic and associated basalt flows of the Western Emeishan flood basalt province, China. J. Pet. 47, 1997–2019. doi:10.1093/petrology/egl034
Zhong, H., Hu, R. Z., Wilson, A. H., and Zhu, W. G. (2005). Review of the link between the hongge layered intrusion and emeishan flood basalts, southwest China. Int. Geol. Rev. 47, 971–985. doi:10.2747/0020-6814.47.9.971
Keywords: identifying basalt, Landsat-5, ASTER, supervised learning, machine-learning algorithms
Citation: Zeng L, Li T, Huang H, Zeng P, He Y, Jing L, Yang Y and Jiao S (2023) Identifying Emeishan basalt by supervised learning with Landsat-5 and ASTER data. Front. Earth Sci. 10:1097778. doi: 10.3389/feart.2022.1097778
Received: 14 November 2022; Accepted: 22 December 2022;
Published: 09 January 2023.
Edited by:
Qiuming Pei, Southwest Jiaotong University, ChinaReviewed by:
Wenyan Ge, Northwest A&F University, ChinaJie Dou, China University of Geosciences Wuhan, China
Shubin Zhou, Macquarie University, Australia
Copyright © 2023 Zeng, Li, Huang, Zeng, He, Jing, Yang and Jiao. This is an open-access article distributed under the terms of the Creative Commons Attribution License (CC BY). The use, distribution or reproduction in other forums is permitted, provided the original author(s) and the copyright owner(s) are credited and that the original publication in this journal is cited, in accordance with accepted academic practice. No use, distribution or reproduction is permitted which does not comply with these terms.
*Correspondence: Ling Zeng, zengling18@cdut.edu.cn; Shoutao Jiao, jshoutao@mail.cgs.gov.cn