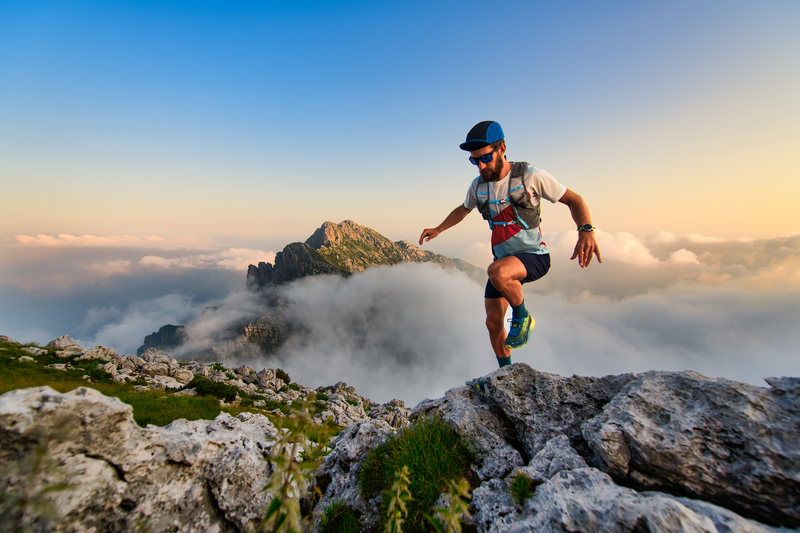
94% of researchers rate our articles as excellent or good
Learn more about the work of our research integrity team to safeguard the quality of each article we publish.
Find out more
EDITORIAL article
Front. Earth Sci. , 04 January 2023
Sec. Environmental Informatics and Remote Sensing
Volume 10 - 2022 | https://doi.org/10.3389/feart.2022.1090016
This article is part of the Research Topic Artificial Intelligence and Machine Learning in Earth Science View all 5 articles
Editorial on the Research Topic
Artificial intelligence and machine learning in Earth science
In recent years, Artificial Intelligence (AI) and Machine learning (ML) methods have demonstrated practical usage in several spheres of Earth system science including the hydrosphere, geosphere, biosphere, and cryosphere. With technological evolution, countless numbers of ground, air, space, and marine-based sensors provide information regarding the health of our planet; Modern technologies such as AI and ML have become very useful tools in many areas of Earth science studies because of their capability to interpret petabytes of data collected by a variety of sensors.
The aim of the current Research Topic is to collect recent advances related to artificial intelligence and machine learning in geophysics. The Research Topic demonstrates the use of AI in leaf area index product generation, improved core lithofacies predictions, water level predictions, and forecasting monthly precipitation. In the first paper, (Li) a Nonlinear Auto Regressive neural network with eXogenous input (NARXNN) model generated using spectral reflectance is implemented.
In the second paper, (Koeshidayatullah et al.) a vision transformer model Facies ViT for improved core lithofacies classification useful for subsurface reservoir characterization in different basins worldwide is developed, three machine learning approaches 1) Multilayer perceptron neural network (MLP-NN), 2) Elman neural network (ENN), and 3) integration of particle swarm optimization algorithm to Elman neural network (PSO-ENN) are used to predict the water level in the Dongting lake.
Authors of the third paper (Luo et al.) have implemented a hybrid EEMD-BMA model based on ensemble empirical mode decomposition (EEMD) and Bayesian model averaging (BMA) for monthly precipitation series data at Kunming station from January 1951 to December 2020 to predict precipitation.
In the last paper, (Deng et al.) effective usage of AI methods in water level prediction, precipitation prediction and LAI has been demonstrated.
We believe that the content of the Research Topic is an important information source for researchers working in the area of artificial intelligence and machine learning.
All authors listed have made a substantial, direct, and intellectual contribution to the work and approved it for publication.
S-AO were employed by the Sonatrach.
The remaining authors declare that the research was conducted in the absence of any commercial or financial relationships that could be construed as a potential conflict of interest.
All claims expressed in this article are solely those of the authors and do not necessarily represent those of their affiliated organizations, or those of the publisher, the editors and the reviewers. Any product that may be evaluated in this article, or claim that may be made by its manufacturer, is not guaranteed or endorsed by the publisher.
Keywords: AI, ML, earth science, advances, research
Citation: Ouadfeul S-A, Jawak SD, Shirzadi A and Idrees MO (2023) Editorial: Artificial intelligence and machine learning in Earth science. Front. Earth Sci. 10:1090016. doi: 10.3389/feart.2022.1090016
Received: 04 November 2022; Accepted: 05 December 2022;
Published: 04 January 2023.
Edited and reviewed by:
Alexander Kokhanovsky, German Research Centre for Geosciences, GermanyCopyright © 2023 Ouadfeul, Jawak, Shirzadi and Idrees. This is an open-access article distributed under the terms of the Creative Commons Attribution License (CC BY). The use, distribution or reproduction in other forums is permitted, provided the original author(s) and the copyright owner(s) are credited and that the original publication in this journal is cited, in accordance with accepted academic practice. No use, distribution or reproduction is permitted which does not comply with these terms.
*Correspondence: Sid-Ali Ouadfeul, b3VhZGZldWw3N0BnbWFpbC5jb20=
Disclaimer: All claims expressed in this article are solely those of the authors and do not necessarily represent those of their affiliated organizations, or those of the publisher, the editors and the reviewers. Any product that may be evaluated in this article or claim that may be made by its manufacturer is not guaranteed or endorsed by the publisher.
Research integrity at Frontiers
Learn more about the work of our research integrity team to safeguard the quality of each article we publish.