- 1College of Economics and Management, Shenyang Agricultural University, Shenyang, China
- 2Finacial Department, Shenyang Agricultural University, Shenyang, China
Agriculture has become a main source of greenhouse gas emissions globally, so limiting agricultural carbon emissions is an important part of achieving carbon neutrality in China. The reduction of agricultural carbon emissions needs the support of green credit. This paper explores the spatial effect and mechanism of green credit on agricultural carbon emissions. Specifically, based on panel data of 30 Chinese provinces from 2009 to 2019, the relationship between green credit and agricultural carbon emissions is empirically analyzed using spatial econometric and mediating effect models. The results show that green credit has a significant inhibitory effect on agricultural carbon emissions. Meanwhile, green credit can also limit agricultural carbon emissions in surrounding areas through spatial spillover effects. Moreover, the study reveals that green credit can indirectly suppress agricultural carbon emissions by promoting agricultural green technology innovation. Based on the above conclusions, this paper puts forward policy recommendations for the development of green credit and the reduction of agricultural carbon emissions.
1 Introduction
Climate change is related to the survival and development of human beings, which has attracted widespread attention from all over the world. China officially proposed a “dual carbon” goal of peaking carbon dioxide emissions by 2030 and achieving carbon neutrality by 2060 at the 75th United Nations General Assembly on 22 September 2020. As the world’s largest carbon emitter, China’s realization of the “dual carbon” goal is critical to mitigating global climate change. At the same time, agriculture has become an important source of greenhouse gas emissions globally. According to the Food and Agriculture Organization of the United Nations, greenhouse gas emissions from agriculture and food production accounted for more than 30% of global greenhouse gas emissions in 2019. Meanwhile, agriculture is the second largest source of greenhouse gas emissions in China, and greenhouse gas emissions from agricultural production activities account for about 12.54% of the total global greenhouse gas emissions (FAOSTAT, 2019). Therefore, how to control the carbon emissions from agriculture and related sectors has become the key to China’s realization of the “dual carbon” goal. Agriculture is greatly influenced by the environment and climate with significant environmental and climate externalities, which should be one of the key areas for green finance support (Ma et al., 2021). As the most important part of green finance, green credit introduces environmental risk factors into traditional credit decision-making (Thompson & Cowton, 2004), which is an effective way to promote agricultural carbon emission reduction. Previous studies have shown that green credit has obvious carbon emission reduction effects. Jiang et al. (2020) empirically studied the relationship between green credit on carbon emissions and found that green credit has a significant negative effect on carbon emissions. In terms of the mechanism, green credit mainly indirectly restrains carbon emissions by promoting technological innovation (Lyu et al., 2022) and optimizing industrial structure (Hu and Zheng, 2022). However, few studies have explored the agricultural carbon emission reduction effect of green credit. So, does green credit limit agricultural carbon emissions? If there is such an effect, what is the mechanism behind it? Is there a spatial spillover effect of green credit on agricultural carbon emissions? Studying the above issues has important practical significance for exploring the ecological and environmental effects of green credit and promoting agricultural carbon emission reduction.
Based on China’s provincial panel data from 2009 to 2019, this paper measures the intensity of agricultural carbon emissions and studies the mechanism of green credit impacting agricultural carbon emissions and empirically analyzes the impact of green credit on agricultural carbon emissions from the perspective of spatial spillover effects and mediation effects. The results show that green credit has a significant inhibitory effect on agricultural carbon emissions. Moreover, the impact of green credit on agricultural carbon emissions has a significant spatial spillover effect. In addition, green credit can promote agricultural green technology innovation and indirectly restrain agricultural carbon emissions.
The main contributions of this paper are as follows. Firstly, based on the realistic background that agriculture has become the key to achieving the “dual carbon” goal, this paper focuses on the agricultural carbon emission reduction effect of green credit, which is rarely studied in previous papers and fills the relevant research gaps. Secondly, this paper uses a spatial econometric model to test the spatial spillover effect of green credit on agricultural carbon emissions in terms of research methods. This paper examines the direct effects and spatial effects of green credit on agricultural carbon emissions. Finally, this paper analyzes the mechanism of green credit impacting agricultural carbon emissions from the perspective of green technology innovation based on the Porter hypothesis, which is also lacking in previous studies and enriches the relevant theories of green credit.
The rest of this paper is organized as follows. Section 2 shows a literature review. Section 3 introduces the theoretical framework and research hypotheses. Section 4 details the research methodology and data. Section 5 presents the empirical results and discusses them. Section 6 reports the discussion, conclusion and future researches.
2 Literature review
Along with the rapid economic growth, ecological imbalance, environmental pollution, and resource shortage are becoming increasingly serious, hence green credit has become a key force to promote the coordinated development of economic growth and environmental protection. Previous studies show that green credit has a significant contribution to green economic growth (Guo et al., 2022; Li et al., 2022) and green total factor productivity (Hu et al., 2022). It can also promote green technological innovation (Hong et al., 2021; Hu et al., 2021; Yu et al., 2021) and industrial structure upgrading (Xu et al., 2018; Li et al., 2020). At the micro level, green credit can significantly improve the financial performance (Lian et al., 2022; Xi et al., 2022) and high-quality development (Ding et al., 2022) of commercial banks, helping to improve the quality and efficiency of credit services. In addition, green credit has a clear dual impact on enterprise financing. Liu and Dong (2022) found that green credit exacerbates the financing cost of enterprises with high consumption and high pollution (“two high” enterprises). In contrast, it will play a role in alleviating financing constraints for green enterprises (Xu and Li, 2020). Following the development of carbon peaking and carbon-neutral targets, some scholars have shifted their focus from the economic effects of green credits to the carbon emission reduction effects. Jiang et al. (2020) used a dynamic panel data model to empirically examine the effects of green credits on carbon emissions and found that green credits have a significant inhibitory effect on carbon emissions. In terms of the mechanism, green credit can indirectly suppress carbon emissions through two paths: promoting technological innovation (Lyu et al., 2022) and optimizing industrial structure (Hu and Zheng, 2022). Additionally, Qin and Cao (2022) used a DID model to evaluate the carbon reduction effects of green credit policies and showed that green credit could also reduce carbon emissions. Although the industrial carbon emission reduction effect of green credit has been confirmed, there is little research and discussion on the impact of green credit on agricultural carbon emissions. Therefore, future research should pay more attention to the agricultural carbon emission reduction effect of green credit.
Agriculture, as one of the important sources of carbon emissions, has attracted more attention in recent years. At present, the research on agricultural carbon emissions mainly focuses on three aspects: carbon source measurement, regional differences, and influencing factors. Li et al. (2011) classified and measured the carbon sources of agricultural land use. Hu and Wang (2010) measured the greenhouse gas emissions from animal husbandry in China. Taking the influence of regional differences into account, Min and Hu (2012) simultaneously measured the carbon emissions of China’s planting industry and animal husbandry, so their calculation results were more accurate. Some scholars also found significant regional differences in agricultural carbon emissions. For example, Tian et al. (2014) and Huang et al. (2019) found that the agricultural carbon emission intensity in western China is much higher than that in eastern and central regions. And some scholars extend the research perspective to the influencing factors of agricultural carbon emissions. It is found that agricultural carbon emissions are affected by the structure of the agricultural industry (He et al., 2018), the level of agricultural economic development (Pang, 2014), the progress of agricultural technology (Han et al., 2018), the degree of agricultural mechanization (Long et al., 2018) and the level of urbanization (Wu, 2015), etc. Apart from that, in terms of spatial effects, agricultural carbon emissions also have significant spatial spillover effects (Chen et al., 2020). However, few studies link financial development to agricultural carbon emissions. As a new financial model that considers environmental, social, and governance factors, green finance has been deeply integrated with agricultural carbon sequestration and emission reduction, and its impact needs to be analyzed in depth.
To sum up, there are still many deficiencies in previous studies. First of all, although some studies have discussed the impact of green credit on industrial carbon emissions (Lyu et al., 2022; Qin and Cao, 2022), agricultural carbon emissions have certain particularities compared to carbon emissions from other sectors and few studies specifically discuss the effect of green credit on agricultural carbon emissions reduction. Secondly, in the current research on agricultural carbon emissions, some studies are not comprehensive enough in measuring agricultural carbon emissions, such as only measuring agricultural material inputs (Wu et al., 2021), which overlooked many important sources of carbon emissions in agricultural production. Finally, most studies focus on the overall effect and ignore the spatial spillover effect of green credits on carbon emissions, which may lead to biased estimation results.
3 Theoretical framework and hypothesis
This study will demonstrate the impact of green credit on agricultural carbon emissions from three aspects: direct effect, spatial spillover effect, and indirect effect. Figure 1 depicts our theoretical framework and research hypotheses.
3.1 Direct effect of green credit on agricultural carbon emissions
Green credit optimizes the allocation of financial resources through differentiated credit policies and attracts more capital to green agriculture. On the one hand, green credit raises the loan threshold and financing costs of “two high” enterprises (Liu et al., 2019; Xu and Li, 2020; Peng et al., 2022), and also inhibits the scale of investment (Wang et al., 2019; Yao et al., 2021), which will limit the scale of “two high” enterprises’ development and force them to transform and upgrade. On the other hand, green credit increases financial support for energy-saving and environmental protection-oriented enterprises, eases financing constraints (Zhou et al., 2021) through tax preferences, loan preferences, and broadening financing channels. Green credit can also improve investment efficiency (Zhang et al., 2022), provide guarantees for the development of environmental enterprises, and promote the formation of a green development model.
In addition, the green credit policy which is a national macro policy transmits the signal of a “developing green economy” to the outside world. Commercial banks are the main implementers of green credit. They impose punitive high-interest rates on the “two high” enterprises (Xu et al., 2018) which will motivate enterprises to improve their carbon emission reduction efficiency. As the efficiency of carbon emission reduction increases, the level of the environmental performance of enterprises also increases, which will enhance the motivation for environmental information disclosure and improves the level of environmental information disclosure (Zhan, 2021). This will strengthen the environmental responsibility of enterprises, reduce investment in the “two high” projects, and increase investment in green projects and pollution control (Zhang and Zhang, 2017). This mechanism applies to the whole macro economy as well as agriculture. Therefore, the following hypothesis is proposed:
Hypothesis 1. Green credit has a direct disincentive effect on agricultural carbon emissions.
3.2 Spatial spillover effect of green credit on agricultural carbon emissions
Green finance as an innovative financial model can break through the limitations of space to achieve cross-regional operation and promotion. Chen and Chen (2021) found a significant spatial spillover effect of green finance on industrial carbon emissions by empirically examining panel data from 30 Chinese provinces. Huang and Chen (2022) extended the scope of the study from industrial carbon emissions to environmental quality indices (including three indicators of industrial solid waste, industrial wastewater, and industrial emissions), which also supported the conclusion that green finance had spatial spillover effects. In addition, there are also spatial spillover effects of green finance on the ecological environment (Li and Gan, 2021), energy quality development (Wang et al., 2021), and green innovation (Huang et al., 2022). Therefore, we believe that the impact of green credit as an important component of green finance on agricultural carbon emissions should also have spatial spillover effects. This leads to the following hypothesis:
Hypothesis 2. Green credit has spatial spillover effects on agricultural carbon emissions.
3.3 Indirect effects of green credit on agricultural carbon emissions
According to Porter’s hypothesis, appropriate environmental policies can stimulate firms to innovate and thus offset the costs of environmental regulations (Porter & Van der Linde, 1995). As a kind of environmental economic policy, green credit can also stimulate technological innovation and generate an “innovation compensation” effect. Green credit affects firms’ green technological innovation in three main ways. Firstly, green credit improves the accessibility and convenience of credit financing for energy-efficient and environmentally friendly enterprises (Wang et al., 2022), which further provides sufficient financial support for their green technology innovation. Secondly, green credit adds environmental protection factors to the loan application conditions, which promotes enterprises to improve their level of environmental protection awareness and actively develop green technologies to achieve active emission reduction (Ji et al., 2021). Thirdly, green credit can alleviate information asymmetry, and when banks give green loans to enterprises, they also release important signals to external investors (He & Liu, 2018), which reduces investors’ information risk and improves investment efficiency, thus forming a good investment environment for enterprises’ green technology innovation. At the same time, technological innovation is believed to reduce agricultural carbon emissions significantly (Long et al., 2018). Accordingly, this hypothesis is proposed:
Hypothesis 3. Green credit can promote agricultural green technology innovation to indirectly limit agricultural carbon emissions.
4 Methodology and data
4.1 Method
4.1.1 Construction of a weight matrix
The selection of a suitable spatial weight matrix is a prerequisite and basis for spatial autocorrelation testing and spatial econometric model regression. To ensure the robustness of the regression results, both 0–1 and geographic distance spatial weight matrices are selected for analysis in this paper. The 0–1 weight matrix (W1) and the geographic distance weight matrix (W2) are defined as follows:
where dij is the distance between the centers of gravity in province i and province j.
4.1.2 Spatial autocorrelation analysis
According to the first law of geography, there is a certain dependence on the spatial distribution of anything. And the closer the distance, the stronger the spatial dependence. The spatial dependence of each variable should be examined before applying the spatial econometric model regression. In this paper, the global Moran index is chosen to test the spatial dependence of agricultural carbon emission intensity and green credit. The global Moran’s I index is defined as follows:
where I is the global Moran index value; xi and xj are the average values of agricultural carbon emission intensity and green credit in province i and province j, respectively;
To further determine the spatial clustering pattern of agricultural carbon emission intensity and green credit, this paper uses the local Moran index to identify the local autocorrelation characteristics of agricultural carbon emission intensity and green credit. The local Moran’s I index is defined as follows:
where Ii is the local Moran index value of province i.
4.1.3 Spatial econometric model
To study the direct effect (Hypothesis 1) and spatial spillover effect (Hypothesis 2) of green credit on agricultural carbon emissions, the traditional general panel data model is further extended to a general form of the spatial econometric model by adding the spatially lagged variables of agricultural carbon emission intensity, green credit, and other control variables, the model expression is as follows:
where lnCIit is the agricultural carbon emission intensity of each province; lnGCit is the green credit of each province; Xit is a series of control variables; ρ is the spatial autoregressive coefficient of agricultural carbon emission intensity; θ1 and θc are the spatially lagged term coefficients of green credit and other control variables, respectively; Wij is the spatial weight matrix; λ is the spatial error coefficient; μit and εit are random error terms.
The general form of spatial econometric models can be transformed into the corresponding models according to the different spatial transmission mechanisms. When ρ ≠ 0, θ = 0 and λ = 0, the model will be transformed into a spatial autoregressive model (SAR); when ρ = 0, θ = 0 and λ ≠ 0, the model (5) will be transformed into a spatial error model (SEM); when ρ ≠ 0, θ ≠ 0 and λ = 0, the model (5) will be transformed into a spatial Durbin model (SDM). A reasonable selection of spatial econometric models will be made later based on the statistical test results.
4.1.3 Mediating effect model
To further investigate the mediating effect of green credit on agricultural carbon emissions (Hypothesis 3). In this paper, agricultural green technology innovation is taken as the mediating variable and the mediating effect model is constructed as follows:
where lnATit is the agricultural green technology innovation in each province; the coefficient α1 in Eq. 5 represents the total effect of green credit on agricultural carbon emissions; β1*γ2 which is the product of the coefficient β1 in Eq. 6 and the coefficient γ2 in Eq. 7 represents the mediating effect; the coefficient γ1 in Eq. 7 represents the direct effect.
4.2 Variable selection
4.2.1 Explained variable
Agricultural carbon intensity is the ratio of agricultural carbon emissions to total agricultural output, representing the carbon emissions per unit of growth in agricultural output. Compared with total agricultural carbon emission or agricultural carbon emission per capita, agricultural carbon emission intensity takes into account the scale of the agricultural economy in each region, and its comparability is stronger. Therefore, this paper chooses agricultural carbon emission intensity as the explanatory variable to measure the level of agricultural carbon emissions in a region, which is calculated as follows:
where CI is agricultural carbon emission intensity (as shown in Figure 2); E is total agricultural carbon emission; AGDP is total agricultural output value.
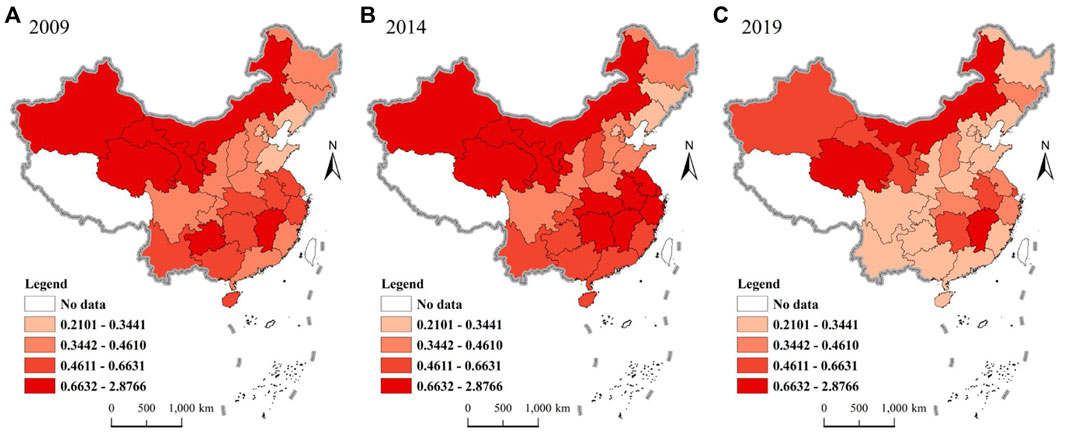
FIGURE 2. The spatial and temporal patterns of China’s agricultural carbon emission intensity in 2009, 2014, and 2019.
Referring to existing studies, to measure agricultural carbon emissions more accurately and comprehensively, this paper divides agricultural carbon emission sources into two major sectors: plantation and animal husbandry. According to the IPCC, the formula for calculating agriculture carbon emissions is as follows:
where E is the total agricultural carbon emission; Ei is the agricultural carbon emission of each carbon source; Ti is the consumption of each carbon source; δi is the carbon emission factor of each carbon source (as shown in Table 1).
4.2.2 Explanatory variable
Combing the existing literature, we find that existing studies mainly measure green credit in four major aspects, namely, the proportion of green credit, the proportion of loans for energy conservation and environmental protection projects, the “bank loans” in industrial pollution control investments, and the proportion of interest expenses in six high-energy-consuming industries (Xie and Liu, 2019). The data on the proportion of green credit and the proportion of loans for energy conservation and environmental protection projects are obtained from bank-level statistics (Ji et al., 2021), which are not consistent with the statistical caliber of this paper which needs the panel data of 30 provinces in China. And the data on “bank loans” in industrial pollution control investments have not been updated since 2010. To sum up, considering that interest expenditure can reflect the scale of credit, this paper refers to Lyu et al. (2022) and adopts the indicator of total interest expenditure of non-six energy-consuming industries to measure green credit.
4.2.3 Mediating variable
Most studies have measured green technology innovation from three perspectives: studies from the input perspective mainly include R&D expenditure and its proportion, the number of research personnel, etc.; studies from the output perspective mainly use the number of patent applications and grants; innovation efficiency is mainly measured by input-output ratio, data envelopment analysis, and stochastic Frontier analysis, etc. Considering the availability and comparability of data, this paper screens agricultural patents according to the IPC classification numbers of agricultural and forestry patents in the green patent list published by WIPO, and finally selects the number of agricultural green invention patent applications to measure agricultural green technology innovation.
4.2.4 Control variables
In fact, agricultural carbon emission intensity is also influenced by a combination of factors. To study the impact of green credit on agricultural carbon emissions more comprehensively, control variables that may influence agricultural carbon emission intensity need to be considered.
The increase in farmers’ income will promote farmers’ input to production factors and thus increase agricultural carbon emissions (Zhou et al., 2022), so rural income level (PCDI) is considered. As the increase of farmers’ literacy will help to understand and use advanced carbon reduction technologies, thus indirectly promoting carbon reduction in agriculture (Wu et al., 2021), rural human capital (HUM) is considered. Crop damage levels (DA) can seriously affect agricultural carbon emissions because crop damage leads to significant yield reductions. The proportion of the primary industry is an important indicator of the level of agricultural development in a province at the macro level (Han et al., 2021), and the development of the primary industry can promote the reduction of carbon emissions per unit of agricultural output value, so industrial structure (STR) is considered. Urbanization makes the migration of rural employment intensify the outflow of agricultural labor, and the construction of urbanized urban areas reduces the space and scale of agricultural production, thus reducing agricultural carbon emissions (Wu, 2015), so the urbanization level (UL) is considered. The specific description of each variable is shown in Table 2.
4.3 Data sources
This paper uses panel data from 30 provinces in China from 2009 to 2019. The data is mainly from the China Industrial Statistical Yearbook, China Economic Census Yearbook, China Rural Statistical Yearbook, China Agricultural Statistical Yearbook, China Agricultural Statistics, China Animal Husbandry Yearbook, China Statistical Yearbook, statistical yearbooks of Chinese provinces (cities, districts), and China Intellectual Property Office. Economic variables such as agricultural output value and disposable income of rural residents are all based on 2009 and are deflated by corresponding price indices. For the missing values of some variables in individual years, the interpolation method is used to fill them. In addition, to alleviate the influence of heteroscedasticity, the explained variable and other partial variable data are processed logarithmically before the empirical analysis. All of the regressions are performed in Stata15.0. The results of descriptive statistics for each variable are shown in Table 3.
5 Results and discussion
5.1 Spatial autocorrelation test
The global Moran’s I of agricultural carbon emission intensity and green credit for each province from 2009 to 2019 are calculated based on Eqn. 3, and the results are shown in Tables 4, 5. The results show that agricultural carbon emission intensity and green credit have a significant positive spatial correlation both under the 0–1 weight matrix (W1) and under the geographic distance weight matrix (W2). Therefore, it is more accurate to use spatial econometric models to explore the effects of green credits on agricultural carbon emissions compared with ordinary panel data models.
To further analyze the spatial clustering patterns of agricultural carbon emission intensity and green credit in each province, the local Moran indexes of agricultural carbon emission intensity and green credit in each province of China from 2009 to 2019 are calculated based on Eq. 4, and the local Moran scatter plots of agricultural carbon emission intensity and green credit are drawn accordingly. Considering the space limitation, this paper only reports the results for 3 years 2009, 2014, and 2019 under the geographic distance weight matrix (W2). As shown in Figure 3, the local Moran scatter points of each province are mostly distributed in the first and third quadrants, which indicates again that agricultural carbon emission intensity and green credit have a strong positive spatial correlation in local space.
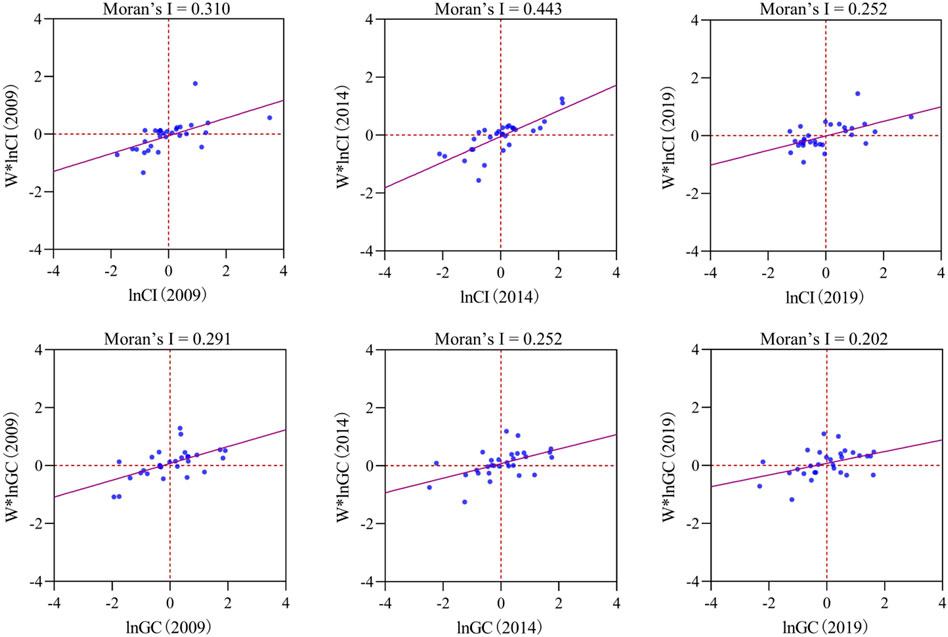
FIGURE 3. Moran scatter plot of agricultural carbon intensity and green credit in 2009, 2014, and 2019.
5.2 Statistical tests for model selection
A reasonable selection of spatial econometric models is needed to study the effect of green credit on agricultural carbon emissions. In this paper, the spatial econometric models will be tested and selected in three steps.
Firstly, LM tests are conducted to regress the sample data using a mixed-effects OLS model. The LM-Lag, robust LM-Lag, LM-Error, and robust LM-Error tests are used to determine the type of spatial transmission mechanism. Then the corresponding spatial econometric model is selected. Table 6 reports the LM test results, and all four LM tests under the 0–1 weight matrix (W1) passed the 10% significance level test, indicating that the SDM model should be selected for analysis. In contrast, the robust LM-Lag test under the geographic distance weight matrix (W2) failed the significance test, indicating that the SEM model should be selected for the analysis.
Secondly, to ensure the robustness of the results, both SEM and SDM models are used in this paper for comparative analysis. Based on the Hausman test results (as shown in Table 7), all models rejected the original hypothesis of choosing the random-effects model at the 5% significance level, so the fixed-effects model should be chosen. Further, by comparing the fitting effects of the three models of individual fixed effects, time fixed effects, and two-way fixed effects, the time fixed effects model should be selected. Meanwhile, the AIC and BIC values of the SDM model are smaller compared with the SEM model, indicating that the SDM model has a better fitting effect. Therefore, the SDM model with time-fixed effects is more suitable to be selected.
Finally, the LR and Wald tests are performed to determine whether the SDM model could be an SEM model. According to Table 7, it can be seen that the LR and Wald tests passed the 1% significance level test under both spatial weight matrices, indicating that the SDM model cannot degrade into an SEM model. In summary, the SDM model with time-fixed effects is finally chosen to explore the impact of green credit on agricultural carbon emissions, and the regression results are analyzed mainly with the geographic distance weight matrix (W2).
5.3 Spatial panel model regression analysis and spillover effect decomposition
Table 7 reports the regression results of the OLS, SEM, and SDM models. The results show that the coefficients of green credit are negative in all five models, and all of them pass the 1% significance level test, indicating that higher levels of green credit can significantly limit agricultural carbon emissions. Therefore, Hypothesis 1 is supported. From column (5), the coefficient of the spatial lag term of green credit is −0.169 and passes the significance level test of 10%, which indicates that green credit has a significant spatial spillover effect. So, green credit in one province of China also has a significant inhibitory effect on agricultural carbon emissions in its neighboring provinces.
However, the regression results of the SDM model do not directly reflect the extent of the impact of green credit on agricultural carbon emissions. This paper draws on LeSage and Pace (2009) and decomposes the regression coefficients of green credit on agricultural carbon emissions into direct effect, indirect effect (spatial spillover effect), and total effect by using the partial differencing method. The direct effect represents the impact of green credit on agricultural carbon emissions in a province; the indirect effect represents the impact of green credit on agricultural carbon emissions in neighboring provinces; the total effect represents the sum of the direct effect and the indirect effect.
As shown in Table 8, the direct, indirect, and total effects of green credits under two different spatial weights are all significantly negative at the 1% significance level, indicating that green credits not only reduce agricultural carbon emissions in the province but also suppress agricultural carbon emissions in neighboring provinces. Therefore, the spatial spillover effects are significant which verifies Hypothesis 2. Compared with the regression coefficients of the OLS model, the direct effect of green credit on agricultural carbon emissions is larger in the SDM model, which indicates to some extent that the suppressive effect of green credit on agricultural carbon emissions would be underestimated if the spatial effect is ignored.
Among the control variables, all three effects of rural income level (PCDI) on agricultural carbon emissions are positive and pass the significance test, which is consistent with the expected hypothesis. The direct effect of rural human capital (HUM) is significantly negative, which is consistent with the expected hypothesis, while the indirect and total effects of rural human capital (HUM) are positive. The probable reason might be that human capital is more restricted by local policies and institutions, which makes it difficult to move across provinces. All three effects of crop damage level (DA) are significantly negative, which may be explained by the relatively stable carbon source of crops, the decrease in agricultural output due to damage, and the increase in agricultural carbon emissions per unit of output value (Wu et al., 2021). The direct effect, indirect effect, and the total effect of industrial structure (STR) and urbanization level (UL) on agricultural carbon emissions are all significantly negative, which is also consistent with the expected hypothesis.
5.4 Mediating effect analysis
According to the previous theoretical analysis, green credit can exert a green technological innovation effect and indirectly suppress agricultural carbon emissions by improving agricultural green technological innovation. To test Hypothesis 3 and investigate the mechanism of the effect of green credit on agricultural carbon emissions, this paper tests it by constructing a mediating effect model.
Table 9 reports the regression results of the mediated effects model. In the first step, the regression coefficient of green credit on agricultural carbon emissions in column (1) is significantly negative and should be further tested. In the second step, the regression coefficient of green credit on agricultural green technology innovation in column (2) is 0.862 and passes the 1% significance level test, indicating that green credit has a significant contribution to agricultural green technology innovation. In the third step, the regression coefficients of −0.246 and −0.141 for green credit and agricultural green technology innovation in column (3) are both significantly negative, indicating that both green credit and agricultural green technology innovation can significantly suppress agricultural carbon emissions. Therefore, Agricultural green technology innovation plays an intermediary role between green credit and agricultural carbon emissions. The value of the mediating effect is −0.122 (0.862* −0.141), which accounts for 32.88% [(−0.122/−0.371)*100%] of the total effect, indicating that there is a “green credit → agricultural green technology innovation → agricultural carbon emissions” transmission mechanism in the process of green credit’s impact on agricultural carbon emissions. Therefore, Hypothesis 3 is verified.
6 Discussion, conclusion, and future research
Based on the panel data of 30 provinces in China from 2009 to 2019, this study measures the intensity of agricultural carbon emissions in each province. Then, this paper empirically investigated the impact of green credit on agricultural carbon emissions, the spatial spillover effect, and its mechanism of action using spatial econometric and mediating effect models. The main conclusions are as follows. Firstly, green credit has a significant inhibitory effect on agricultural carbon emissions. Previous studies have demonstrated the negative impact of green credit on industrial carbon emissions (Jiang et al., 2020; Hu and Zheng, 2022; You et al., 2022). However, few studies have explored the effect of green credit on agricultural carbon emissions reduction, and our study fills this gap. Secondly, the inhibitory effect of green credit on agricultural carbon emissions has a spatial spillover effect. This finding also confirms the conclusion that green finance has a spatial spillover effect on carbon emissions (Chen and Chen, 2021). Thirdly, green credit can indirectly limit agricultural carbon emissions by promoting agricultural green technology innovation. This finding is consistent with previous research conclusions (Li et al., 2022; Lyu et al., 2022), which proposed that green credit could not only directly suppress carbon emissions, but also promote low-carbon technological innovation to indirectly reduce carbon emissions. This study highlights the important role of green technology innovation in agricultural carbon emission reduction and enriches the theoretical mechanism of agricultural carbon emission reduction effects of green credit.
Based on the above findings, this study proposes the following suggestions. First of all, green credit can effectively curb agricultural carbon emissions, so the local government should provide more support for green credit policies and improve the development level and utilization efficiency of green credit. On the one hand, we should implement the “innovation-driven development strategy” to increase the scale of investment in green credit and improve the development level of green credit in the region. On the other hand, we should also introduce more local or foreign talents to improve the management of green credit and the efficiency of green credit utilization. Secondly, as green credit has a significant spatial spillover effect on agricultural carbon emissions, we should strengthen the cross-regional promotion and cooperation of green credit. Then we can give full play to the diffusion effect of green credit agricultural carbon emission reduction and reduce agricultural carbon emissions. Finally, green credit can indirectly limit agricultural carbon emissions by promoting agricultural green technology innovation, so we should build an incentive system for agricultural green technology innovation. We also need to guide green credit funds to support high-tech agricultural enterprises. It is necessary to increase the financial investment in technology research and development of agricultural carbon sequestration and emission reduction.
Although this study has figured out the spatial effect and mechanism of green credit on agricultural carbon emissions, there are still some limitations. Firstly, we only study the impact of green credit which is only one instrument of green finance on agricultural carbon emissions. In fact, green finance has developed quickly in recent years and has involved various financial instruments such as green securities, green investment, green insurance, and carbon finance. We will build a comprehensive index of green finance and figure out its impact on agricultural carbon emissions in the future. Secondly, we will compare the effects of green credit and other green financial instruments on agricultural carbon emissions. Thirdly, due to data limitations, the agricultural carbon emissions data in this study are provincial-level data, we will further explore the impact of green finance on agricultural carbon emissions at the prefecture level in the future.
Data availability statement
The original contributions presented in the study are included in the article/Supplementary Material, further inquiries can be directed to the corresponding authors.
Author contributions
LQ was the first author who contributed to funding acquisition, project administration, and manuscript revision. SL was the co-first author who contributed to data collection, statistical analysis, and manuscript writing. YH helped with data collection and statistical analysis. YZ is responsible for the production of pictures and tables. DW was the corresponding author who helped read and approved the final manuscript. DY was the co-corresponding author who helped read and approved the final manuscript.
Funding
This research was funded by National Social Science Foundation of China (Grant Number. 21CJY026), China Postdoctoral Science Foundation (Grant Number. WSNJC201909), Fundamental scientific research project of Liaoning Provincial Department of Education (Grant Number. WSNJC201909), Entrusted Project of Philosophy, and Social Sciences Young Talents Training in Liaoning Province (Grant Number. 2022lslqnrcwtkt-49).
Conflict of interest
The authors declare that the research was conducted in the absence of any commercial or financial relationships that could be construed as a potential conflict of interest.
Publisher’s note
All claims expressed in this article are solely those of the authors and do not necessarily represent those of their affiliated organizations, or those of the publisher, the editors and the reviewers. Any product that may be evaluated in this article, or claim that may be made by its manufacturer, is not guaranteed or endorsed by the publisher.
References
Chen, W., Peng, Y., and Yu, G. (2020). The influencing factors and spillover effects of interprovincial agricultural carbon emissions in China. PLoS One 15 (11), e0240800. doi:10.1371/journal.pone.0240800
Chen, X., and Chen, Z. (2021). Can green finance development reduce carbon emissions? Empirical evidence from 30 Chinese provinces. Sustainability 13 (21), 12137. doi:10.3390/su132112137
Ding, L., Zhuang, Y., and Jiang, S. (2022). Green credit and high-quality sustainable development of banks. Environ. Sci. Pollut. Res. Int. doi:10.1007/s11356-022-21438-w
FAOSTAT (2019). Food and agriculture organization of the united Nations. Available at: https://www.fao.org/faostat/zh/#data/GT.
Guo, L., Tan, W., and Xu, Y. (2022). Impact of green credit on green economy efficiency in China. Environ. Sci. Pollut. Res. 29 (23), 35124–35137. doi:10.1007/s11356-021-18444-9
Han, H., Zhong, Z., Guo, Y., Xi, F., and Liu, S. (2018). Coupling and decoupling effects of agricultural carbon emissions in China and their driving factors. Environ. Sci. Pollut. Res. 25 (25), 25280–25293. doi:10.1007/s11356-018-2589-7
Han, J., Qu, J., Xu, L., Xu, H., and Xu, L. (2021). The spatial effect of agricultural finance on agricultural greenhouse gas emission intensity: An empirical analysis based on the spatial durbin model. J. Ecol. Rural Environ. 37 (11), 1404. doi:10.19741/j.issn.1673-4831.2020.0783
He, L.-Y., and Liu, L. (2018). Stand by or follow? Responsibility diffusion effects and green credit. Emerg. Mark. Finance Trade 54 (8), 1740–1760. doi:10.1080/1540496x.2018.1430566
He, Y., Chen, R., Wu, H., Xu, J., and Song, Y. (2018). Spatial dynamics of agricultural carbon emissions in China and the related driving factors. Chin. J. Eco-Agriculture 26 (9), 1269. doi:10.13930/j.cnki.cjea.171097
Hong, M., Li, Z., and Drakeford, B. (2021). Do the green credit guidelines affect corporate green technology innovation? Empirical research from China. Int. J. Environ. Res. Public Health 18 (4), 1682. doi:10.3390/ijerph18041682
Hu, G., Wang, X., and Wang, Y. (2021). Can the green credit policy stimulate green innovation in heavily polluting enterprises? Evidence from a quasi-natural experiment in China. Energy Econ. 98, 105134. doi:10.1016/j.eneco.2021.105134
Hu, Q., Li, X., and Feng, Y. (2022). Do green credit affect green total factor productivity? Empirical evidence from China. Front. Energy Res. 9, 821242. doi:10.3389/fenrg.2021.821242
Hu, X., and Wang, J. (2010). Estimation of livestock greenhouse gases discharge in China. Trans. CSAE 26 (10), 247–252. doi:10.3969/j.issn.1002-6819.2010.10.042
Hu, Y., and Zheng, J. (2022). How does green credit affect carbon emissions in China? A theoretical analysis framework and empirical study. Environ. Sci. Pollut. Res. 29, 59712–59726. doi:10.1007/s11356-022-20043-1
Huang, X., Xu, X., Wang, Q., Zhang, L., Gao, X., and Chen, L. (2019). Assessment of agricultural carbon emissions and their spatiotemporal changes in China, 1997-2016. Int. J. Environ. Res. Public Health 16 (17), 3105. doi:10.3390/ijerph16173105
Huang, Y., Chen, C., Lei, L., and Zhang, Y. (2022). Impacts of green finance on green innovation: A spatial and nonlinear perspective. J. Clean. Prod. 365, 132548. doi:10.1016/j.jclepro.2022.132548
Huang, Y., and Chen, C. (2022). The spatial spillover and threshold effect of green finance on environmental quality: Evidence from China. Environ. Sci. Pollut. Res. 29 (12), 17487–17498. doi:10.1007/s11356-021-16892-x
Ji, Y., Jiang, H., and Song, L. (2021). A study on the impact of green credits on low-carbon technological progress: Empirical test based on the provincial panel data of China. J. Yunnan Univ. Finance Econ. 37 (9), 97–110. doi:10.16537/j.cnki.jynufe.000730
Jiang, H., Wang, W., Wang, L., and Wu, J. (2020). The effects of the carbon emission reduction of China’s green finance——An analysis based on green credit and green venture investment. Finance Forum 25 (11), 39–48. doi:10.16529/j.cnki.11-4613/f.2020.11.006
LeSage, J., and Pace, R. K. (2009). Introduction to spatial econometrics. Boca Raton, Florida, United States: Chapman and Hall/CRC.
Li, B., Zhang, J., and Li, H. (2011). Empirical study on China's agriculture carbon emissions and economic development. J. Arid Land Resour. Environ. 25 (12), 8–13. doi:10.13448/j.cnki.jalre.2011.12.002
Li, C., and Gan, Y. (2021). The spatial spillover effects of green finance on ecological environment-empirical research based on spatial econometric model. Environ. Sci. Pollut. Res. 28 (5), 5651–5665. doi:10.1007/s11356-020-10961-3
Li, Y., Ding, T., and Zhu, W. (2022a). Can green credit contribute to sustainable economic growth? An empirical study from China. Sustainability 14 (11), 6661. doi:10.3390/su14116661
Li, Y., Hu, H., and Li, H. (2020). Empirical analysis of the impact of green credit on the upgrading of China's industrial structure: Based on Chinese provincial panel data. Econ. Problems 485 (1), 37–43. doi:10.16011/j.cnki.jjwt.2020.01.005
Li, Z., Feng, L., Mai, S., and Li, A. (2022b). Does green credit curb carbon emissions? ——research based on Chinese provincial panel data. Shanghai Finance 498 (1), 2–12. doi:10.13910/j.cnki.shjr.2022.01.001
Lian, Y., Gao, J., and Ye, T. (2022). How does green credit affect the financial performance of commercial banks? ——evidence from China. J. Clean. Prod. 344, 131069. doi:10.1016/j.jclepro.2022.131069
Liu, Q., and Dong, B. (2022). How does China's green credit policy affect the green innovation of heavily polluting enterprises? The perspective of substantive and strategic innovations. Environ. Sci. Pollut. Res. Int.. doi:10.1007/s11356-022-21199-6
Liu, X., Wang, E., and Cai, D. (2019). Green credit policy, property rights and debt financing: Quasi-natural experimental evidence from China. Finance Res. Lett. 29, 129–135. doi:10.1016/j.frl.2019.03.014
Long, X., Luo, Y., Wu, C., and Zhang, J. (2018). The influencing factors of CO2 emission intensity of Chinese agriculture from 1997 to 2014. Environ. Sci. Pollut. Res. 25 (13), 13093–13101. doi:10.1007/s11356-018-1549-6
Lyu, B., Da, J., Ostic, D., and Yu, H. (2022). How does green credit promote carbon reduction? A mediated model. Front. Environ. Sci. 10, 878060. doi:10.3389/fenvs.2022.878060
Ma, J., Meng, H., Shao, D., and Zhu, Y. (2021). Green finance, inclusive finance and green agriculture development. Finance Forum 26 (3), 3–8. doi:10.16529/j.cnki.11-4613/f.2021.03.002
Min, J., and Hu, H. (2012). Calculation of greenhouse gases emission from agricultural production in China. China Popul. Resour. Environ. 22 (7), 21–27. doi:10.3969/j.issn.1002-2104.2012.07.004
Pang, L. (2014). Empirical study of regional carbon emissions of agriculture in China. J. Arid Land Resour. Environ. 28 (12), 1–7. doi:10.13448/j.cnki.jalre.2014.12.001
Peng, B., Yan, W., Elahi, E., and Wan, A. (2022). Does the green credit policy affect the scale of corporate debt financing? Evidence from listed companies in heavy pollution industries in China. Environ. Sci. Pollut. Res. 29 (1), 755–767. doi:10.1007/s11356-021-15587-7
Porter, M. E., and Van der Linde, C. J. (1995). Toward a new conception of the environment-competitiveness relationship. J. Econ. Perspect. 9 (4), 97–118. doi:10.1257/jep.9.4.97
Qin, J., and Cao, J. (2022). Carbon emission reduction effects of green credit policies: Empirical evidence from China. Front. Environ. Sci. 10, 789072. doi:10.3389/fenvs.2022.798072
Thompson, P., and Cowton, C. J. (2004). Bringing the environment into bank lending: Implications for environmental reporting. Br. Account. Rev. 36 (2), 197–218. doi:10.1016/j.bar.2003.11.005
Tian, Y., Zhang, J., and He, Y. (2014). Research on spatial-temporal characteristics and driving factor of agricultural carbon emissions in China. J. Integr. Agric. 13 (6), 1393–1403. doi:10.1016/S2095-3119(13)60624-3
Tian, Y., Zhang, J., and Li, B. (2011). Research on spatial-temporal characteristics and factor decomposition of agricultural carbon emission based on input angle-taking Hubei Province for example. Res. Agric. Mod. 32 (6), 752–755. doi:10.3969/j.issn.1000-0275.2011.06.025
Wang, E., Liu, X., Wu, J., and Cai, D. (2019). Green credit, debt maturity, and corporate investment—evidence from China. Sustainability 11 (3), 583. doi:10.3390/su11030583
Wang, F., Wang, R., and He, Z. (2021). The impact of environmental pollution and green finance on the high-quality development of energy based on spatial Dubin model. Resour. Policy 74, 102451. doi:10.1016/j.resourpol.2021.102451
Wang, H., Qi, S., Zhou, C., Zhou, J., and Huang, X. (2022). Green credit policy, government behavior and green innovation quality of enterprises. J. Clean. Prod. 331, 129834. doi:10.1016/j.jclepro.2021.129834
West, T. O., and Marland, G. (2002). A synthesis of carbon sequestration, carbon emissions, and net carbon flux in agriculture: Comparing tillage practices in the United States. Agric. Ecosyst. Environ. 91 (1-3), 217–232. doi:10.1016/s0167-8809(01)00233-x
Wu, C. (2015). The impact of urbanization on agricultural carbon emissions in China. Econ. Surv. 32 (1), 12–18. doi:10.15931/j.cnki.1006-1096.2015.01.003
Wu, F. L., Li, L., Zhang, H. L., and Chen, H. (2007). Net carbon emissions of farmland ecosystem influenced by conservation tillage. Chin. J. Ecol. 26, 2035–2039. doi:10.13292/j.1000-4890.2007.0360
Wu, G., Liu, J., and Chen, Y. (2021). Analysis of spatial characteristics and spillover effects of agricultural carbon emission intensity in China. Environ. Sci. Technol. 44 (11), 211–219. doi:10.19672/j.cnki.1003-6504.1521.21.338
Xi, B., Wang, Y., and Yang, M. (2022). Green credit, green reputation, and corporate financial performance: Evidence from China. Environ. Sci. Pollut. Res. 29 (2), 2401–2419. doi:10.1007/s11356-021-15646-z
Xie, T., and Liu, J. (2019). How does green credit affect China’s green economy growth? China Popul. Resour. Environ. 29 (9), 83–90. doi:10.12062/cpre.20190501
Xu, S., Zhao, X., and Yao, S. (2018). Analysis on the effect of green credit on the upgrading of industrial structure. J. Shanghai Univ. Finance Econ. 20 (2), 59–72. doi:10.16538/j.cnki.jsufe.2018.02.004
Xu, X., and Li, J. (2020). Asymmetric impacts of the policy and development of green credit on the debt financing cost and maturity of different types of enterprises in China. J. Clean. Prod. 264, 121574. doi:10.1016/j.jclepro.2020.121574
Yao, S., Pan, Y., Sensoy, A., Uddin, G. S., and Cheng, F. (2021). Green credit policy and firm performance: What we learn from China. Energy Econ. 101, 105415. doi:10.1016/j.eneco.2021.105415
You, Z., Li, Z., and Li, P. (2022). Research on the impact of green finance development on regional carbon emission: Take green credit, green industrial investment and green bonds for example. Financial Theory & Pract. 511 (02), 69–77. doi:10.3969/j.issn.1003-4625.2022.02.008
Yu, C.-H., Wu, X., Zhang, D., Chen, S., and Zhao, J. (2021). Demand for green finance: Resolving financing constraints on green innovation in China. Energy Policy 153, 112255. doi:10.1016/j.enpol.2021.112255
Zhan, H. (2021). How does green credit affect corporate environmental information disclosure? Evidence based on heavily polluting enterprises in China. Nankai Econ. Stud. 2021 (3), 193–207. doi:10.14116/j.nkes.2021.03.012
Zhang, J., Luo, Y., and Ding, X. (2022). Can green credit policy improve the overseas investment efficiency of enterprises in China? J. Clean. Prod. 340, 130785. doi:10.1016/j.jclepro.2022.130785
Zhang, P., and Zhang, X. (2017). Construction and development of green credit policy system in China. Environ. Prot. 45 (19), 7–10. doi:10.14026/j.cnki.0253-9705.2017.19.002
Zhou, G., Liu, C., and Luo, S. (2021). Resource allocation effect of green credit policy: Based on DID model. Mathematics 9 (2), 159. doi:10.3390/math9020159
Keywords: green credit, green finance, agricultural carbon emissions, spatial spillover effect, mediating effect, climate change
Citation: Qin L, Liu S, Hou Y, Zhang Y, Wu D and Yan D (2023) The spatial spillover effect and mediating effect of green credit on agricultural carbon emissions: Evidence from China. Front. Earth Sci. 10:1037776. doi: 10.3389/feart.2022.1037776
Received: 06 September 2022; Accepted: 27 September 2022;
Published: 09 January 2023.
Edited by:
Jingke Hong, Chongqing University, ChinaReviewed by:
Quiang Ma, Liaoning Shihua University, ChinaShuaishuai Li, Changchun Sci-Tech University, China
Copyright © 2023 Qin, Liu, Hou, Zhang, Wu and Yan. This is an open-access article distributed under the terms of the Creative Commons Attribution License (CC BY). The use, distribution or reproduction in other forums is permitted, provided the original author(s) and the copyright owner(s) are credited and that the original publication in this journal is cited, in accordance with accepted academic practice. No use, distribution or reproduction is permitted which does not comply with these terms.
*Correspondence: Dongli Wu, wdl@syau.edu.cn; Dabo Yan, 2002510009@syau.edu.cn
†These authors share first authorship