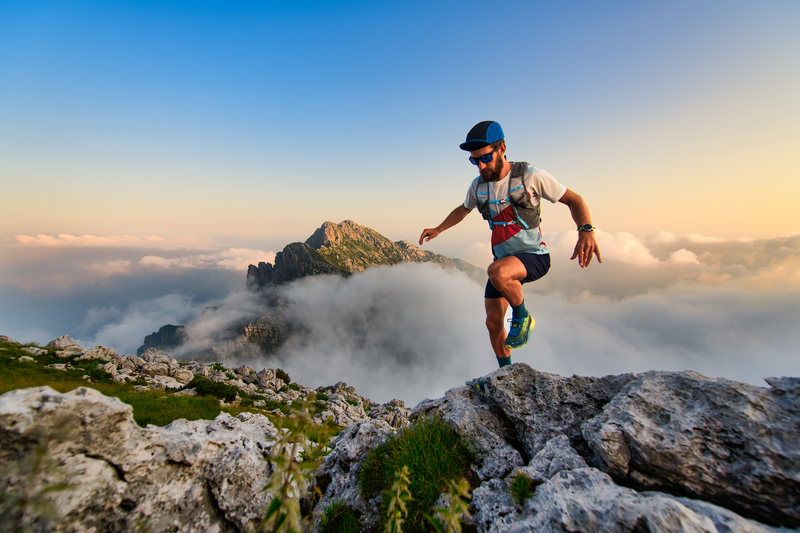
94% of researchers rate our articles as excellent or good
Learn more about the work of our research integrity team to safeguard the quality of each article we publish.
Find out more
ORIGINAL RESEARCH article
Front. Earth Sci. , 06 January 2023
Sec. Quaternary Science, Geomorphology and Paleoenvironment
Volume 10 - 2022 | https://doi.org/10.3389/feart.2022.1032578
This article is part of the Research Topic Geological Approaches and Thoughts in Chinese Archaeology View all 6 articles
Strontium isotope ratios (87Sr/86Sr) are a widely applied tool in provenance applications in archaeology, paleoecology, forensics, and food science. Bedrock Sr is naturally and anthropogenically transferred to other reservoirs on the Earth’s surface through interactions with the hydrosphere, atmosphere, and biosphere. We attempt to reassess the contribution of the spatial distribution of bioavailable strontium isotopes using the geographic detector model (GDM). Our study reveals that the watershed factor explains 50.35% of the spatial variation of bioavailable Sr isotopes, while the climate, terrain, geology, and soil explain 14.41%, 4.94%, 4.63%, and 4.70% of the spatial variation of bioavailable Sr isotopes. The factors influencing the spatial variation of bioavailable Sr isotopes in China were ranked as follows: basin > climate > terrain > geology > soil type. The nonlinear enhancements of the interactions between the watershed and geology explained 59.90% of the spatial variation in bioavailable Sr isotopes, indicating that natural processes still control the bioavailability of strontium isotopes in China. Based on the synthesized river-water isotopic data, the large-scale bioavailable Sr isoscape discussed herein can be useful for migratory studies in China. The enrichment of strontium isotope data to refine Sr isotopes, as well as the search for better data on possible controlling factors, will make the analysis more comprehensive and provide a solid foundation for explaining strontium isotope migration.
Strontium is a member of Group IIA alkaline earth with four naturally stable isotopes: 84Sr, 86Sr, 87Sr, and 88Sr Notably, only 87Sr is radiogenic with a half-life of 48.8 × 109 years, produced by the radioactive β-decay of 87Rb (Rubidium-87) (Capo et al., 1998). Rocks or minerals have different ages and Rb/Sr ratios, which allow the Earth to indicate unique and predictable patterns of variations in strontium isotopes (Bataille and Bowen, 2012). Strontium isotope ratios (87Sr/86Sr) have been a widely applied tool in provenance applications in archaeology, paleoecology, forensics, and food science during the past 4 decades (Ericson, 1985; Font et al., 2015; Coelho et al., 2017; Crowley et al., 2017).
Provenancing biological materials using strontium isotope ratios requires a map of bioavailable strontium. Strontium isotopes essentially depend on the regional geological background, i.e., the older and more Rb-enriched bedrock contains more 87Sr During the isotope transfer and migration process from rock to biological tissues, the strontium isotope composition might deviate from the bedrock composition due to the differentiated weathering of rocks, mixing of soils and water, bio-averaging mechanisms, and human modification. Since the first Sr isoscape of the United States was calculated by age variations in basement rocks (Beard and Johnson, 2000), many bioavailable strontium isoscapes have been generated on different substrates such as plants, soils, animals, and water (Evans et al., 2010; Thornton, 2011; Bataille et al., 2020; Wang and Tang, 2020) using various methods (Holt et al., 2021). However, the strontium transfer processes are significantly modified by anthropogenic activities such as landscape utilization, deforestation, mining exploitation, agricultural fertilization, and mass logistics (Maurer et al., 2012; Thomsen and Andreasen, 2019). To better understand the strontium isotopes of archaeological samples and tentatively geolocate their possible provenances, it is necessary to evaluate the contributions of various contributing factors, including the environment and human activities.
The geographic detector model (GDM) is a statistical method developed by Wang and Hu, 2012) to detect spatial heterogeneity and reveal the driving forces behind it. This method is robust for identifying driving forces and influencing factors as well as conducting spatial analysis. The GDM has some significant advantages compared to classical statistical methods since it considers spatial effects and does not require a linear hypothesis (Wang et al., 2016). The relationship between X and Y established by the GDM is more reliable than that determined by a classical regression (Wang and Xu, 2017), since any potential factors can be included in the analysis without considering the problem of multiple collinearities (Zhou et al., 2018). Finally, the GDM can rank the determination power of each factor and describe its changes over time (Ding et al., 2019). Therefore, the GDM has been widely utilized in many fields of natural and social sciences, including public health (Wang et al., 2010), land use (Ju et al., 2016), PM2.5 (Wang et al., 2021), air quality indexes (Zhan et al., 2018), heavy metals (Wu et al., 2021), carbon emissions (Zhang and Zhao, 2018), and soil erosion (Wang et al., 2019), and it has been gradually adopted for research on agricultural eco-efficiency (Liao et al., 2021). Although various fields have been explored, GDM has rarely been utilized to analyze the factors affecting Sr isotopes.
In China, a large-scale bioavailable strontium isotope map has been generated based on 1872 water samples across the whole country (Wang and Tang, 2020), providing a baseline for migration studies. Herein, we intend to elucidate the impacts of various surficial factors and their interactions on Chinese bioavailable strontium patterns using the GDM. Given the importance of bioavailable Sr isotopes, this study aimed to identify the factors controlling bioavailable Sr isotopes using GDM and multiple data sources. The results are expected to reveal environmental factors’ driving contributions and provide a geo-statistical approach to explore the factors controlling the bioavailability of Sr isotopes.
All Sr isotopes samples were collected from rivers, plant leaves, local animals, and humans (from archaeological sites), which resulted in 1935 sample locations published in the dataset of bioavailable strontium isotopes in China by Wang and Tang (2020). Most isotopic ratio measurements were conducted at the State Key Laboratory of Lithospheric Evolution at the Institute of Geology and Geophysics, Chinese Academy of Sciences (IGGCAS) with a Triton Plus thermal ionization mass spectrometer (TIMS) (Thermo Fisher Scientific). Considering that some data points from the 1935 samples were obtained from published references, we normalized the ratios relative to an 87Sr/86Sr for a NIST SRM-987 of 0.710,250 when available to eliminate any potential interlaboratory bias. If the measured ratio of the standard sample was not available in the reference, we used the reported ratios without further corrections (Figure 1).
The 87Sr/86Sr ratios across China exhibit considerable heterogeneity with a distinct strontium isotope distribution based on geological and isotopic data. Given the spatial distribution characteristics of Sr isotopes in inter- and intra-tectonic blocks, the regions with the highest ratio of 87Sr/86Sr (>0.722) are mainly found in the Guotai block and the southeastern margin of the Tibetan Plateau; the lowest percentage (0.701–0.708) is primarily distributed in the Junggar Terrane, the Erguna-Xing’an block, and the Jiamusi-Hanka block (Wang and Tang, 2020).
Natural background factors (i.e., the interaction of the hydrosphere, atmosphere, and biosphere) (Holt et al., 2021) and human activities (e.g., mining exploitation, agricultural fertilization) (Maurer et al., 2012; Thomsen and Andreasen, 2019) can lead to changes in the spatial pattern of bioavailable strontium isotopes. In this study, indicators such as geology, soil, topography, climate, vegetation, river basins, and land use were selected to detect their effects on the spatial distribution of bioavailable strontium isotopes and their interactions using geographic probes. The magnitudes of these effects and their interactions could be detected to assess their various roles in the bioavailable strontium isotopes.
Topographic data were obtained from NASA’s data sharing platform using a 1 × 1 km digital elevation model. The land-use data were obtained from a web station of the 2015 ESA CCI Land Cover project, and the river-basin data were obtained from the 2020 Water Resources Statistical Yearbook. The soil, plant, and geology of China data were obtained from the Data Center for Resources and Environmental Sciences of the Chinese Academy of Sciences. The climate data are derived from the map of climate types in China, which was published in the Atlas of Physical Geography of China (Yang et al., 2010). The selected factors are listed with their associated data sources in Table 1 and Figure 2.
FIGURE 2. Controlling factors. (A) Geology (B) Soil, (C) Terrain, (D) Land use, (E) Climate, (F) Plant, (G) Watershed. The soil-type legend is as follows: 10 leached soils, 11 semi-leaching soils, 12 calcium-layer soils, 13 dry soils, 15 primary soils, 16 semi-hydrated soils, 17 hydrated soils, 18 saline-alkali soils, 19 artificial soils, 20 alpine soils, 21 ferrallites, 22 urban districts, 24 lakes and reservoirs, 25 rivers, 26 sandbars and islands in rivers, 28 coral reefs and sea islands, and 30 coastal salt farms.
GDM is mainly used to analyze the correlation among Sr isotopes, the selected eight impact factors, and multiple impact factor interactions. The q values in the GeoDetector have a clear physical meaning without an assumption of linearity and objectively detect ×100 q% of the dependent variable (Wang et al., 2019). In this study, we employed factor and interaction detectors in the GDM to more comprehensively analyze the correlations between Sr isotopes and the selected impact factors (Figure 3).
FIGURE 3. Rationale of the geographical detector (Wang et al., 2010; Wang et al., 2017). (A) Detection of driving forces, and (B) interaction detection.
The factor detector method was used to measure the spatially stratified heterogeneity of geographic variable Y, and the q-statistic was defined as follows (Wang et al., 2016):
where
An “interaction detector” was defined to assess the interaction between two different factors, namely
The spatial agglomeration can be identified within the context of neighboring features (Getis and Ord, 1992). In order to identify the local spatial autocorrelation, Getis–Ord Gi is introduced and can be expressed as follows:
where
The descriptive statistics of bioavailable Sr isotopes are shown in Table 2. The variation of bioavailable Sr isotopes ranges from 0.701,937 to 0.755,434, with mean and median values of 0.711,207 and 0.71, respectively. According to Figure 4, the results of Getis–Ord Gi showed a significant feature of local spatial autocorrelation. Regions with high Z scores (hot spots) were located in Sichuan, Yunnan, Jiangxi, Guangxi, Gansu, and Henan. The regions with low Z scores (cold spots) were mainly located in Guizhou, Heilongjiang, Jilin, and Xinjiang. The firm spatial heterogeneity of bioavailable Sr isotopes provides a robust basis for GDM analysis.
In Table 3, the q-statistic results indicated that the watershed was the dominant factor in the distribution of bioavailable Sr isotopes in China, which explains 50.35% of the spatial variation of the bioavailable Sr isotopes. Then, the climate, terrain, geology, and soil explain 14.41%, 4.94%, 4.63%, and 4.70% of the spatial variation of the bioavailable Sr isotopes, respectively. The land use and plants did not pass the significance testing at the 0.05 level.
According to the results of the GDM detection factors, the factors influencing the spatial variation of the bioavailable Sr isotopes in China were ranked as follows: watershed > climate > terrain > geology > soil type (Figure 5).
FIGURE 5. Factors influencing the Sr isotopes with q values. The black vertical bar on the left represents the determination of each driver of a Sr isotope. The black vertical bar on the right represents the Sr isotope.
The interaction detector was applied to reveal the interaction effects between the two driving factors, thereby indicating whether two driving forces enhance or weaken each other or if they independently affect the spatial heterogeneity of Sr isotopes.
In Figure 6, nonlinear enhancements of the interactions were observed between the watershed and the geology (q = 0.5990), climate (q = 0.5853), soil (q = 0.5674), and terrain (q = 0.5629), which explained 59.90%, 58.53%, 56.74%, and 56.29% of the spatial variation in the bioavailable Sr isotopes, respectively. Moreover, the climate, geology, terrain, and soil contributed to one another, respectively explaining 31.07%, 29.43%, and 24.15% of the spatial pattern of the bioavailable Sr isotopes. The q values for the watershed and other factors were around 50%, indicating that the watershed was the critical factor influencing the spatial pattern of bioavailable Sr isotopes.
FIGURE 6. The interaction detector for bioavailable Sr isotopes with q values and the interaction model. The red arrows indicate the interaction class of two influencing factors. The red arrow is located to the right of the yellow mark, showing nonlinear enhancement; the red arrow is located between the green and yellow mark, indicating bi-enhancement factors. Then, the red arrow is located to the left of the green dot, indicating weakened and nonlinearly weakened factors.
The results of the synergistic effects of two factors showed mostly nonlinear enhancement, except for the synergistic effect of the watershed and climate, which was nonlinearly attenuated; this may be related to the similarity in spatial pattern of the watershed and climate.
The geological background shapes the topography and determines the strontium isotopic composition of minerals and rocks. Strontium isotopes across China show significant heterogeneity on large spatial scales. For example, the areas with the lowest strontium isotope ratios (0.701–0.708) are distributed in the Junggar Terrane, Erguna-Xing’an block, and Jiamusi-Hanka block. In contrast, the areas with the highest ratios (>0.722) are mainly found in the Cathaysia block and the southeastern margin of the Tibetan Plateau. The Cathaysia block, where the lower Pearl River and Ganjiang River flow through the ancient Craton, is dominated by acidic rocks with high strontium isotope ratios. The probable reason for this is that the predominant outcrops are exposures of Proterozoic–Phanerozoic sedimentary rocks and Phanerozoic igneous rocks, especially the widespread Mesozoic granitoids (Li et al., 2014; Yu et al., 2010), indicating higher 87Sr/86Sr ratios (>0.711) in this block.
The Erguna and Xing’an Blocks in the western part of northeast China consist mainly of the Proterozoic to Paleozoic volcano-sedimentary strata and granites with thick Mesozoic volcanic cover, as well as some Mesozoic mafic-ultramafic rocks, Early Paleozoic limestone, and Late Paleozoic clastic sediments in the north (Wu et al., 2011). Therefore, the area is mainly a young basaltic volcanic area with relatively low strontium isotope ratios. The Yangtze Block comprises the Proterozoic metamorphic basement complexes and is primarily covered by Paleozoic–Early Mesozoic marine carbonates, clastic rocks, and some mafic rocks in the southwest (Chen, 1994). Therefore, the 87Sr/86Sr ratios are expected to be relatively homogenous and low (∼0.707–0.710) due to marine carbonates, which are easily weathered. Meanwhile, influenced by the weathering of carbonate rocks, the Yangtze Block and the Junggar Terrane–Tian Shannorthern Block also have relatively low 87Sr/86Sr ratios.
Soils primarily inherit their strontium isotopic composition from geomaterials and parent rock, and, consequently, strontium isotope information in the soil follows that of the underlying geology. Due to differential weathering and soil mixing processes, 87Sr/86Sr compositions usually show a more homogenized signature. The soil mainly reflects strontium combined with bedrock minerals and soil-forming methods.
From the q values mentioned above, geology is one of the main controlling factors but only explains 4.64% of the strontium isotope variance. Strontium isotopes in river water may be far from the rock due to differences in the abundance and weathering rates of strontium in minerals.
Weathering and mixing in the basin result in more homogeneous strontium isotope ratios in the watershed. The q values show that the watershed explains most of the variance. Water samples taken from streams, lakes, and rivers have been used to represent a bioavailable Sr library. The spatial pattern of strontium isotopes in a river depends on the rock masses at the surface and underwater. Each of these blocks experienced different tectonic activities throughout their geologic history and contained significantly different lithologies in their outcrops. Consequently, the spatial patterning of 87Sr/86Sr in the hydrosphere reflects that of rocks exposed on the surface and in aquifers. The 87Sr/86Sr value is higher in rivers draining cratons and lower in rivers draining mafic rock units (Peucker-Ehrenbrink and Fiske, 2019).
Watersheds could explain the most strontium isotopic variance. River water signals upstream homogenization within a watershed, similarities within a watershed, and differences between watersheds. The river water is a mixture of strontium isotopic input from the upper reaches of the rivers as well as precipitation in riverbed sediments.
Climatic factors, such as temperature, humidity, and seasonal changes, influence weathering. The variance explained by the climate factor alone is 14.4%, but, when combined with information from the watershed and geology, its variance significantly increases to 59.9% and 31.1%, respectively (Figure 6).
Weathering at the watershed scale has prominent seasonal characteristics. The general pattern is that the weathering rate decreases during the dry period, increases after the rainy season, and increases and decreases abruptly during the ice melt and heavy rainfall periods, respectively. The weathering rate in southeast–northwest China shows a gradualdecrease (Wang and Tang, 2020). Differences in weathering rates in different climatic zones will affect the spatial distribution of bioavailable strontium isotopes. The lower Pearl River basin is located in southeastern China and has a subtropical climate. The silicate weathering rate (SWR) of the lower reaches of the Pearl River is 5.60 ± 5.05, and the carbonate weathering rate (CWR) is 25.58 ± 13.99. The Yangtze River basin is located primarily in the central part of China and belongs to the subtropical monsoon climate zone; the SWR and CWR of the Yangtze River are 2.76 ± 1.06 and 14.28 ± 5.13, respectively. The Heihe River basin is located in the middle and high latitudes with a temperate continental monsoon climate; it has an SWR and CWR of 1.49 ± 4.62 and 5.36 ± 13.4, respectively, in its upper reaches. The Kaidu and Aksu Rivers are located in northwestern China, which belongs to the temperate arid continental and semi-arid climate zone. The Kaidu River is in the middle of the Tianshan Mountains, and the Aksu River is in the western part of the Tarim Basin. The SWR and CWR of the Kaidu and Aksu River are 0.75 ± 0.48 and 4.03 ± 1.51, respectively.
In our study, the land-use and plant factors did not pass the significance test, indicating that natural processes still dominate the bioavailable strontium isotopes in China, and the long history of farming in eastern China has not changed the strontium isotope pattern in the watershed on this scale. This scenario might result from a sampling bias, i.e., the water samples for the bioavailable Sr isoscape were mainly obtained along the course of large drainages in China, which would minimize 87Sr/86Sr ratio variations as the river crosses different lithologic units and would overstate the anthropogenic influences. If small-to-medium sized rivers were selected to evaluate the Sr isotope composition of discrete watersheds, a better picture of the true Sr isotope variations could be captured over unique geographic/geologic areas.
Although regional strontium variation essentially depends on the area’s geological background, the bioavailable strontium signals could have their own controlling factors when various processes are involved. As discussed above, the river water-based bioavailable Sr isoscape is largely controlled by the watershed rather than geological heterogeneity. This also implies great differences in the spatiotemporal scale among various substrates that were used to reconstruct the bioavailable strontium isoscape.
Conversely, bioavailable Sr isoscapes constructed solely on the basis of their geological background may have significant errors. Various factors and processes contribute to these errors, including but not limited to rock types, chemical weathering rates, climate, vegetation, the home range of animals, and human activities. A promising approach to reduce this uncertainty is to compile the currently used data with observed bioavailable Sr isotopic signals, such as those from local animals, soil substrates, or vegetation.
Based on the synthesized river-water isotopic data, the large-scale bioavailable Sr isoscape discussed herein demonstrates value for migratory studies in China that would be effective on the scale of the watershed given the river-induced mixing process. At any specific site, it is necessary to refine the isoscape using other proxies, such as archaeological animals and local plants.
In general, the variation in the bioavailable Sr isoscape occurs on a long time-scale. Among the controlling factors, the terrain, soil, watershed, and geology are variables that demonstrate slow change. However, land cover, climate, and vegetation are variable and exhibit rapid change. Therefore, there are some time gaps between the dependent variables (controlling factors) and independent variable (bioavailable Sr isoscape), which will impact the accuracy of the GDM analysis and require further study.
Bedrock Sr is naturally and anthropogenically transferred to other reservoirs on the Earth’s surface through interactions with the hydrosphere, atmosphere, and biosphere. We attempted to reassess the contribution of the spatial distribution of bioavailable strontium isotopes using the geographic detector model. Our study revealed that the factors influencing the spatial variation of bioavailable Sr isotopes in China were ranked as follows: watershed > climate > terrain > geology > soil type. The nonlinear enhancements of the interactions between the watershed and geology explained 59.90% of the spatial variation in the bioavailable Sr isotopes, indicating that natural processes still dominate the bioavailable strontium isotopes in China.
Our analysis also provided some areas to consider with respect to migratory studies using strontium isotopes. The strontium isotope data must be strengthened and refined to improve the Sr isoscape; in addition, we can seek better controlling factor data to make the analysis more comprehensive. The controlling factors will provide a solid ground on which to interpret the inferred migration of the strontium isotopes (Thornton, 2011).
The raw data supporting the conclusions of this article will be made available by the authors, without undue reservation.
All authors read and approved the final version of the manuscript. XM conceived of the study. XZ designed the methodology, conducted the analysis, and wrote the first draft of the manuscript. XW reviewed and edited the manuscript.
This study was supported by the National Key R&D Program of China (Nos. 2019YFA0607403 and 2021xjkk0903), National Natural Science Foundation of China (Nos. 42072210, 32271638, and 32171561), and Central Public-interest Scientific Institution Basal Research Fund (Nos. Y2020PT05 and BSRF201901).
The authors declare that the research was conducted in the absence of any commercial or financial relationships that could be construed as a potential conflict of interest.
All claims expressed in this article are solely those of the authors and do not necessarily represent those of their affiliated organizations, or those of the publisher, the editors and the reviewers. Any product that may be evaluated in this article, or claim that may be made by its manufacturer, is not guaranteed or endorsed by the publisher.
Bataille, C. P., and Bowen, G. J. (2012). Mapping 87Sr/86Sr variations in bedrock and water for large scale provenance studies. Chem. Geol. 304-305, 39–52. doi:10.1016/j.chemgeo.2012.01.028
Bataille, C. P., Crowley, B. E., Wooller, M. J., and Bowen, G. J. (2020). Advances in global bioavailable strontium isoscapes. Palaeogeogr. Palaeoclimatol. Palaeoecol. 555, 109849. doi:10.1016/j.palaeo.2020.109849
Beard, B. L., and Johnson, C. M. (2000). Strontium isotope composition of skeletal material can determine the birth place and geographic mobility of humans and animals. J. Foren. Sci. 45 (5), 1049–1061. doi:10.1520/JFS14829J
Capo, R. C., Stewart, B. W., and Chadwick, O. A. (1998). Strontium isotopes as tracers of ecosystem processes: Theory and methods. Geoderma 82, 197–225. doi:10.1016/s0016-7061(97)00102-x
Chen, Y. Q. (1994). An introduction of regional geology in China. Beijing: Geological Publishing House. (In Chinese).
Coelho, I., Castanheira, I., Bordado, J. M., Donard, O., and Silva, J. A. L. (2017). Recent developments and trends in the application of strontium and its isotopes in biological related fields. TrAC Trends Anal. Chem. 90, 45–61. doi:10.1016/j.trac.2017.02.005
Crowley, B. E., Miller, J. H., and Bataille, C. P. (2017). Strontium isotopes (87Sr/86Sr) in terrestrial ecological and palaeoecological research: Empirical efforts and recent advances in continental-scale models. Biol. Rev. 92, 43–59. doi:10.1111/brv.12217
Ding, Y., Zhang, M., Qian, X., Li, C., Chen, S., and Wang, W. (2019). Using the geographical detector technique to explore the impact of socioeconomic factors on PM2.5 concentrations in China. J. Clean. Prod. 211, 1480–1490. doi:10.1016/j.jclepro.2018.11.159
Ericson, J. E. (1985). Strontium isotope characterization in the study of prehistoric human ecology. J. Hum. Evol. 14, 503–514. doi:10.1016/s0047-2484(85)80029-4
Evans, J. A., Montgomery, J., Wildman, G., and Boulton, N. (2010). Spatial variations in biosphere 87Sr/86Sr in Britain. J. Geol. Soc. Lond. 167, 1–4. doi:10.1144/0016-76492009-090
Font, L., Jonker, G., van Aalderen, P. A., Schiltmans, E. F., and Davies, G. R. (2015). Provenancing of unidentified World War II casualties: Application of strontium and oxygen isotope analysis in tooth enamel. Sci. Justice 55, 10–17. doi:10.1016/j.scijus.2014.02.005
Getis, A., and Ord, J. K. (1992). The analysis of spatial association by use of distance statistics. Geogr. Anal. 24, 189–206. doi:10.1111/j.1538-4632.1992.tb00261.x
Holt, E., Evans, J. A., and Madgwick, R. (2021). Strontium(87Sr/86Sr) mapping: A critical review of methods and approaches. Earth-Science Rev. 216, 103593. doi:10.1016/j.earscirev.2021.103593
Ju, H., Zhang, Z., Zuo, L., Wang, J., Zhang, S., Wang, X., et al. (2016). Driving forces and their interactions of built-up land expansion based on the geographical detector – a case study of beijing, ChinaDetrital zircon U–Pb age and Hf isotope constrains on the generation and reworking of precambrian continental crust in the Cathaysia block, south China: A synthesis. Gondwana Res. Res. 3025 (11), 21881202–22071215. doi:10.1016/j.gr.2014.01.003
Li, X. H., Li, Z. X., and Li, W. X. (2014). Detrital zircon U-Pb age and Hf isotope constrains on the generation and reworking of Precambrian continental crust in the Cathaysia Block, South China: A synthesis. Gondwana Res. 25, 1202–1215.
Liao, J., Yu, C., Feng, Z., Zhao, H., Wu, K., and Ma, X. (2021). Spatial differentiation characteristics and driving factors of agricultural eco-efficiency in Chinese provinces from the perspective of ecosystem services. J. Clean. Prod. 288, 125466. doi:10.1016/j.jclepro.2020.125466
Luo, L. C., Hui, X. L., Wang, Z. H., Zhang, X., Xie, Y. H., Gao, Z. Q., et al. (2019). Multi-site evaluation of plastic film mulch and nitrogen fertilization for wheat grain yield, protein content and its components in semiarid areas of China. Field Crops Res. 240, 86–94. doi:10.1016/j.fcr.2019.06.002
Maurer, A. F., Galer, S. J., Knipper, C., Beierlein, L., Nunn, E. V., Peters, D., et al. (2012). Bioavailable 87Sr/86Sr in different environmental sampleseffects of anthropogenic contamination and implications for isoscapes in past migration studies. Sci. Total Environ. 433, 216–229. doi:10.1016/j.scitotenv.2012.06.046
Peucker-Ehrenbrink, B., and Fiske, G. J. (2019). A continental perspective of the seawater 87Sr/86Sr record: A review. Chem. Geol. 510, 140–165. doi:10.1016/j.chemgeo.2019.01.017
Su, Y. J., Guo, Q. H., Hu, T. Y., Guan, H. C., Jin, S. C., An, S. Z., et al. (2020). An updated vegetation map of China (1:1000000). Sci. Bull. 65, 1125–1136. doi:10.1016/j.scib.2020.04.004
Thomsen, E., and Andreasen, R. (2019). Agricultural lime disturbs natural strontium isotope variations: Implications for provenance and migration studies. Sci. Adv. 5, eaav8083. doi:10.1126/sciadv.aav8083
Thornton, E. K. (2011). Reconstructing ancient Maya animal trade through strontium isotope (87Sr/86Sr) analysis. J. Archaeol. Sci. 38, 3254–3263. doi:10.1016/j.jas.2011.06.035
Wang, J. F., Li, X. H., Christakos, G., Liao, Y. L., Zhang, T., Gu, X., et al. (2010). Geographical detectors-based health risk assessment and its application in the neural tube defects study of the Heshun region, China. Int. J. Geogr. Inf. Sci. 24, 107–127. doi:10.1080/13658810802443457
Wang, J. F., Zhang, T. L., and Fu, B. J. (2016). A measure of spatial stratified heterogeneity. Ecol. Indic. 67, 250–256. doi:10.1016/j.ecolind.2016.02.052
Wang, H., Gao, J., and Hou, W. (2019). Quantitative attribution analysis of soil erosion in different geomorphological types in karst areas: Based on the geodetector method. J. Geogr. Sci. 29 (2), 271–286. doi:10.1007/s11442-019-1596-z
Wang, Y., Liu, C., Wang, Q., Qin, Q., Ren, H., and Cao, J. (2021). Impacts of natural and socioeconomic factors on PM2.5 from 2014 to 2017. J. Environ. Manag. 284, 112071. doi:10.1016/j.jenvman.2021.112071
Wang, J. F., and Xu, C. D. (2017). Geodetector: Principle and prospective. Acta Geogr. Sin. 72, 116–134.
Wang, J., and Hu, Y. (2012). Environmental health risk detection with GeogDetector. Environ. Model. Softw. 33, 114–115. doi:10.1016/j.envsoft.2012.01.015
Wang, X., and Tang, Z. (2020). The first large-scale bioavailable Sr isotope map of China and its implication for provenance studies. Earth-Science Rev. 210, 103353. doi:10.1016/j.earscirev.2020.103353
Wu, F. Y., Sun, D. Y., Ge, W. C., Zhang, Y. B., Grant, M. L., Wilde, S. A., et al. (2011). Geochronology of the Phanerozoic granitoids in northeastern China. J. Asian Earth Sci. 41, 1–30. doi:10.1016/j.jseaes.2010.11.014
Wu, Q., Hu, W., Wang, H., Liu, P., Wang, X., and Huang, B. (2021). Spatial distribution, ecological risk, and sources of heavy metals in soils from a typical economic development area, southeastern China. Sci. Total Environ. 780, 146557. doi:10.1016/j.scitotenv.2021.146557
Yu, J. H., O’Reilly, S. Y., Wang, L., Griffin, W. L., Zhou, M. F., Zhang, M., et al. (2010). Components and episodic growth of Precambrian crust in the Cathaysia Block, South China: Evidence from U–Pb ages and Hf isotopes of zircons in Neoproterozoic sediments. Precambrian Res. 181, 97–114. doi:10.1016/j.precamres.2010.05.016
Zhan, D., Kwan, M., Zhang, W., Yu, X., Meng, B., and Liu, Q. (2018). The driving factors of air quality index in China. J. Clean. Prod. 197, 1342–1351. doi:10.1016/j.jclepro.2018.06.108
Zhang, X., and Zhao, Y. (2018). Identification of the driving factors’ influences on regional energy-related carbon emissions in China based on geographical detector method. Environ. Sci. Pollut. Res. 25, 9626–9635. doi:10.1007/s11356-018-1237-6
Keywords: strontium isotope, geographic detector model, natural and anthropogenic influences, controlling factor, China
Citation: Zhang X, Ma X and Wang X (2023) Using the geographic detector model to identify factors controlling the bioavailability of Sr isotopes in China. Front. Earth Sci. 10:1032578. doi: 10.3389/feart.2022.1032578
Received: 31 August 2022; Accepted: 28 October 2022;
Published: 06 January 2023.
Edited by:
Hassan Fazeli Nashli, University of Tehran, IranReviewed by:
Zhan Tian, Southern University of Science and Technology, ChinaCopyright © 2023 Zhang, Ma and Wang. This is an open-access article distributed under the terms of the Creative Commons Attribution License (CC BY). The use, distribution or reproduction in other forums is permitted, provided the original author(s) and the copyright owner(s) are credited and that the original publication in this journal is cited, in accordance with accepted academic practice. No use, distribution or reproduction is permitted which does not comply with these terms.
*Correspondence: Xin Ma, bWF4aW4wMkBjYWFzLmNu
Disclaimer: All claims expressed in this article are solely those of the authors and do not necessarily represent those of their affiliated organizations, or those of the publisher, the editors and the reviewers. Any product that may be evaluated in this article or claim that may be made by its manufacturer is not guaranteed or endorsed by the publisher.
Research integrity at Frontiers
Learn more about the work of our research integrity team to safeguard the quality of each article we publish.