- 1Key Laboratory of Western China’s Environmental Systems (Ministry of Education), College of Earth and Environmental Sciences, Lanzhou University, Lanzhou, Gansu, China
- 2Key Laboratory of Land Surface Process and Climate Change in Clod and Arid Regions, Northwest Institute of Eco-Environment and Resources (NIEER), Chinese Academy of Sciences (CAS), Lanzhou, Gansu, China
- 3Pingliang Land Surface Process and Severe Weather Research Station, Chinese Academy of Sciences (CAS), Pingliang, Gansu, China
China implemented a one-month lockdown after the 2020 Spring Festival to prevent the spread of COVID-19. The closure measures provide a rare opportunity to understand the resulting changes in air pollution levels and to test the effectiveness of previous environmental protection measures. We used the time series decomposition method to quantify the air pollution in Lanzhou during the closure period. The results showed that during the epidemic lockdown period, although the concentration of SO2 in Lanzhou decreased substantially, there was a significant increase in the concentration of O3 (by 19.14%), followed by a gradual return to the normal level. Most of the changes during the COVID-19 lockdown were within the range of fluctuations over the past five years. The trend of decreasing SO2 and CO in 2020 was less than that during 2015–2019, and the continuous decline of the PM10 concentration exceeded expectations. NO2, PM2.5 and O3 maintained the trend of the previous five years. Our results show that temporary social closure measures have a limited effect on improving air quality in Lanzhou, and they emphasize the importance of reducing the O3 concentration in the future.
1 Introduction
The outbreak of the Corona Virus Disease 2019 (COVID-19) pandemic in 2020 has had major impacts on economic activity and human health. To control the spread of COVID-19, China implemented lockdown policies and urban traffic and industrial production were strictly limited. Lanzhou, the capital city of Gansu Province, announced the Level Ⅰ response (shut down commercial activities, restrict travel, and require people to stay at home) to major public health emergencies on 25th January, two days after the confirmation of two pneumonia cases with new coronavirus infections in the city. Control measures such as self-isolation at home, store closures, and traffic control were implemented. After 27 days of these strict control measures, more than 90% of areas in Gansu Province were designated as being of low-risk of infection, and the emergency response level for COVID-19 prevention and control in Gansu Province was reduced to Level Ⅲ on 21st February.
This short-term lockdown severely restricted the movement of people and economic activities (He et al., 2020), the economy was adversely affected, and industrial energy consumption decreased; especially, there was significant reduction in traffic volume. Reduced pollution levels during the COVID-19 lockdown have been reported in different countries and regions worldwide (Chen et al., 2020; Donzelli et al., 2020; Li et al., 2020; Singh et al., 2020). However, several studies have pointed out that air quality improvements are notably more limited than some earlier reports or observational data suggest (Pei et al., 2020; Shi et al., 2021; Wang et al., 2021). For example, Wang et al. (2020) reported that the concentrations of NO2 and particulate matter in Beijing during the period of strictest travel restrictions (Level Ⅰ control measures) were significantly lower than before the closure of the city, while Brimblecombe & Lai (2021) found no obvious decrease in pollutant concentrations in Beijing during the lockdown, compared to the same period in 2019. Similar differences between the effects of lockdown on pollutant concentrations among different studies for the same area were reported for the United Kingdom (Munir et al., 2021). These findings suggest that evaluating the impact of the COVID-19 lockdown on air quality is more complicated than initially reported. Due to differences in research methods, slightly different results were obtained for the extent of the COVID-19 impact in the same region. Compared with the sequential method (comparing the lockdown period with the period before lockdown), the parallel method is more suitable for this type of intervention analysis (Munir et al., 2021).
With the implementation of the Air Pollution Prevention and Control Measures since 2012, the air quality in urban Lanzhou has improved significantly and the phenomenon of “Lanzhou Blue” is frequent (Zhao et al., 2018). Nevertheless, the local government is faced with major challenges in further improving the air quality. In this context, the strict control measures implemented during the COVID-19 epidemic provide the opportunity to observe the relationship between human activities and environmental quality, which may help formulate future air pollution control strategies for Lanzhou.
In this study we compared measured pollution data during the interval of 1st January–30th September 2020 with those for the previous five years (2015–2019) in Lanzhou. Based on the changes in the trends of six pollutants (PM2.5, PM10, SO2, NO2, O3, and CO) from 2015 to 2019, the air pollution level that would have occurred in 2020 without an epidemic was reconstructed. The impact of the COVID-19 lockdown on the air quality in the city is discussed using the difference between the reconstructed and observed pollutant concentrations. Our results may help formulate forward-looking intervention policies for improving the air quality in the city.
2 Materials and methods
To quantify the impact of the COVID-19 lockdown intervention on the air quality in Lanzhou, we divided the study interval, from 1st January 2020 to 30th September 2020, into four periods. P1 (1st–24th January 2020) is pre-lockdown, P2 (25th January–20th February 2020) is the Level Ⅰ response period, with strict controls on traffic and prohibition on gathering. P3 (21st February–10th May 2020) is the Level Ⅲ response period with public places opening in an orderly manner, and schools and living services resuming, P4 (11st May–30th September 2020) is the relaxation period.
2.1 Study area
Lanzhou, the capital city of Gansu province, is located in the semi-arid area of Northwest China (102°35′-104°34′E, 35°34′-37°07′N), in a long and narrow valley. The average altitude of the valley floor is ∼1,500 m. Lanzhou has a temperate continental climate, with an annual average air temperature of 11.5°C, relative humidity of 26.8%, wind speed of 1.49 m/s, and an average annual precipitation of 327 mm (which is concentrated in the summer months) (Ma et al., 2020). Lanzhou is located on the sandstorm transport path originating from the Taklimakan Desert, the Badain Jaran Desert, and the Hexi Corridor, the dust intrusions from upstream regions in spring time make Lanzhou one of the most severely air-polluted cities in China. Lanzhou is also an important industrial city in Northwest China (Yan et al., 2021), major industries in the urban area are petrochemical refinery and manufacturing (Wang et al., 2009).
2.2 Air quality data
Hourly concentrations of particulate matter (PM2.5, PM10), nitrogen dioxide (NO2), sulfur dioxide (SO2), carbon monoxide (CO) and 8 h moving average ozone (O3−8h) at four air quality monitoring stations in Lanzhou City from 2015 to 2020 were obtained from the China National Environmental Monitoring Network (http://www.cnemc.cn). Three of the monitoring stations are in the urban area and one is in a suburban of Lanzhou. The averages of the four sites is used to represent the overall pollution situation in Lanzhou. The following quality control measures were applied to the monitoring data. When the hourly concentration of PM2.5 exceeded the concentration of PM10 at the same hour, both the PM2.5 and PM10 for that hour were discarded; if more than three consecutive hours had the same value, the records were discarded; all zero values and outliers (values exceeding the measurement range of the instrument) were removed (Liu et al., 2016; Wu and Zhang, 2018). After the application of these criteria, if there were more than four missing data in a day, the daily average for that day was recorded as missing.
2.3 Time series decomposition
The level of air pollutants in Lanzhou has shown a clear downward trend in recent years (Tan et al., 2009; Yin et al., 2020), and therefore the data needed to be detrended before the effect of the COVID-19 lockdown could be evaluated by comparing the concentrations of each pollutant in previous years with that in 2020. Besides the trend of the inter-annual change, seasonal variations and the Spring Festival effect were also considered.
The non-parametric Mann-Kendall trend test (http://www.mathworks.com/matlabcentral/fileexchange/authors/23983) (Burkey, 2006) was used to obtain the trend of the interannual change for the six pollutants during 2015–2019, and the Theil-Sen method (Theil, 1992; Sen, 1968) was used to determine the magnitude (Sen’s slope) of the trend. All the slopes were calculated in MATLAB using the daily averaged pollutant concentrations.
The Theil-Sen method has been widely used in long-term trend analysis (Neeti and Eastman, 2011; Munir et al., 2013). It is a non-parametric technique for robustly fitting a line to data while minimizing the influence of outliers. Sen’s slope (Q) is determined by finding the median of all slopes between pairs of data points in a time series (i.e., time series of the daily averaged pollutant concentrations from 2015 to 2019):
Where xi and xj are daily average concentrations of PM2.5, PM10, NO2, SO2, CO, or the daily 8-h maximum O3 (O3–8 h) for day i and j, and n is the number of days in the five years. A positive Q value represents an increasing trend, while a negative Q value represents a decreasing trend.
The daily PM2.5 concentrations from 2015 to 2019 were used to calculate the inter-annual trend (orange line in Figure 1A). The seasonal variation was calculated after removing the interannual trend from the daily averaged data (Figure 1B). An average seasonal variation for 2015–2019 was obtained (red line in Figure 1B) and further removed from the detrended data to obtain the residual (similar Figures for other pollutants are presented in the Supplementary Information, see Supplementary Figure S1). The graphical fitting process was performed on the data shown in Figure 1 using locally weighted scatterplot smoothing (LOWESS) (Cleveland et al., 2017). LOWESS smoothing was conducted in Origin 2021 software. The 5-year daily data were processed using the LOWESS data smoothing algorithm with a 30-day window. After removing the inter-annual trend (blue line in Figure 1A), the pollutant concentrations in different years were found to be more comparable, which facilitated the analysis of the impact of COVID-19.
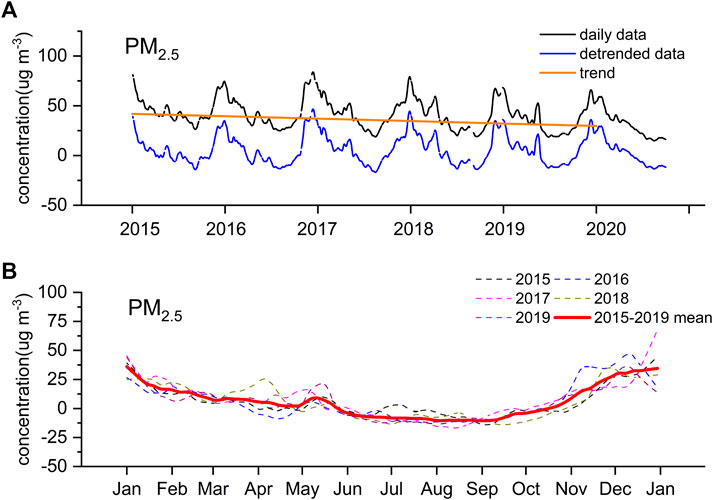
FIGURE 1. (A) Time series of daily PM2.5 concentration averaged across all sites in Lanzhou (black) and the trend (orange) calculated using the data for 2015–2019. (B) Seasonal cycle of PM2.5 after removing the inter-annual trend. The data are smoothed using a LOWESS filter (30 days).
Lanzhou announced its lockdown on 25th January 2020, which is also the first day of the Chinese New Year (CNY) and the most important festival in China. Therefore, the holiday effect would potentially cause errors in assessing the impact of the COVID-19 lockdown. Obvious differences in air pollutant concentrations during CNY and non-CNY have been confirmed by many studies (Tan et al., 2009; Shi et al., 2014; Zhao et al., 2014), and thus proper treatment of the CNY effect is important when comparing pollutant concentrations across years (Silver et al., 2020). The date of the CNY is determined according to the Lunar calendar in China, and the official Chinese New Year Festival lasts for seven days. Here, we consider the 7 days prior to and the 7 days after (total of 15 days) the CNY as the period affected by the Chinese Spring Festival. The 15-day time series after detrending and deseasonalizing were averaged for each pollutant to obtain the CNY signal (red line in Figure 2).
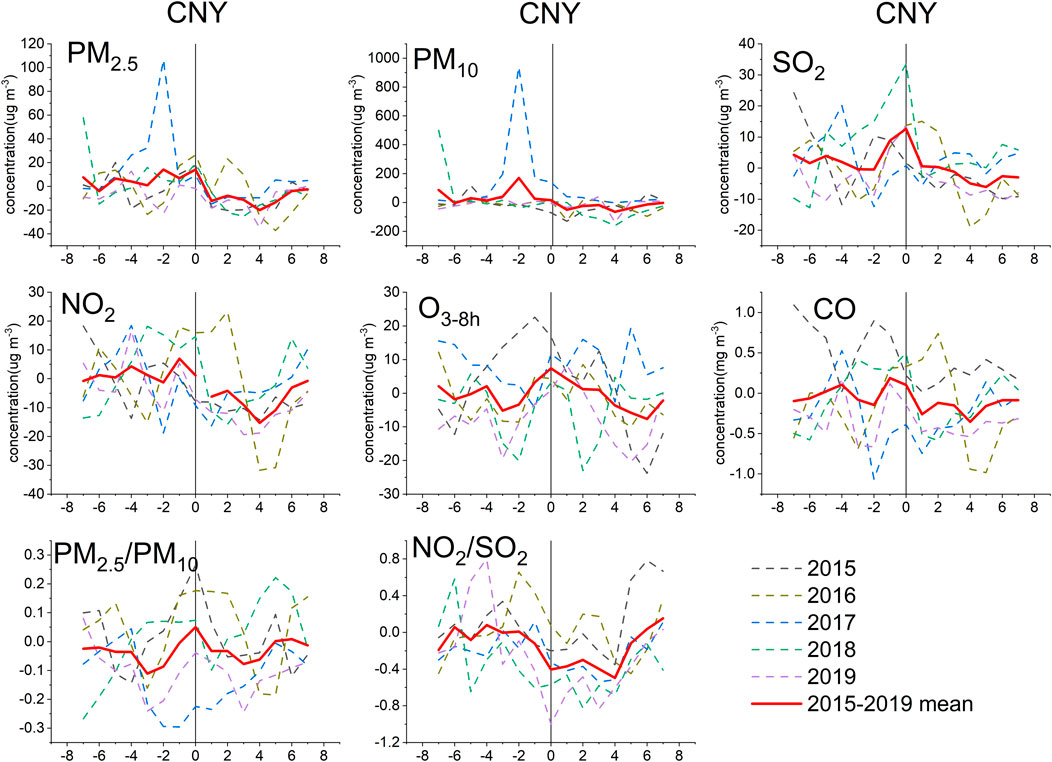
FIGURE 2. Average 2015–2019 detrended and deseasonalized concentrations of six pollutants, PM2.5/PM10 and NO2/SO2 during the Chinese New Year (CNY). The Spring Festival data for each year are stripped of trend and seasonal cycle.
The above processing was applied to each pollutant at each site, using the daily averaged data series during 2015–2019. As a result, the time series for each pollutant were divided into four components: inter-annual trends, season variations, fixed events (i.e., the CNY effect), and noise or residuals. The patterns of the first three components were used to reconstruct the daily concentrations of each pollutant at each site in 2020. The residuals for each pollutant were used to assess the deviation of each pollutant concentration due to the COVID-19 lockdown from their expected value in the absence of COVID-19. In 2020, the most likely source impacting these residual concentrations was the COVID-19 lockdown, although meteorological contributions cannot be excluded.
3 Results
3.1 Temporal variations of the pollutants
As shown in Figure 3, during the period of 2015–2019, PM2.5, PM10, SO2 and CO in Lanzhou all show a decreasing trend, while O3 shows a significant increasing trend. There is no obvious trend in NO2. The inter-annual trends of all pollutants are all significant at p <0.05. PM2.5 and CO have the strongest negative trend, with a median trend of −6.81% year−1 or −2.44 μg m−3 year−1 and −8.43% year−1 or −0.08 mg m−3 year−1, respectively. PM10 and SO2 have a negative trend of −0.55% year−1 or −0.55 μg m−3 year−1 and −5.54% year−1 or −0.73 μg m−3 year−1, respectively, while O3 has a positive trend of 1.69% year−1 or 1.53 μg m−3 year−1. NO2 increased slightly with a median trend of 0.05% year−1 or 0.02 μg m−3 year−1. These values are comparable to those in Silver et al. (2018), who found that the annual average PM2.5 concentration of 1,689 monitoring sites in China decreased by 3.4 μg m−3 year−1 or 7.2% year−1 between 2015 and 2017, and that there was no median trend in annual mean NO2 concentration (0.0 μg m−3 year−1 or 0.1% year−1). The increase in O3 concentration and decrease in PM2.5 and SO2 were also observed in other regions in China in recent years (Fan et al., 2020; Kuerban et al., 2020). The decreasing trend indicates the effectiveness of the Air Pollution Prevention and Control Measures implemented since 2012 in reducing high loading PM2.5 and SO2 therein. For ozone, several studies have reported that decreases in PM2.5 could increase ozone through decrease in aerosol sink of hydroperoxy (HO2) radicals and increase of photolysis rates (Li et al., 2019). For example, a recent study by Zhao et al. (2021) in Lanzhou noticed an increase of mean ambient temperature and net radiation at noon during 2017–2019 compared with 2015–2016, and attributed it partly to large PM2.5 reductions. Both the increase of mean ambient temperature and net radiation could increase the production of ozone. The role played by meteorological conditions and its interaction with aerosols in ozone trends in the study area need further investigation.
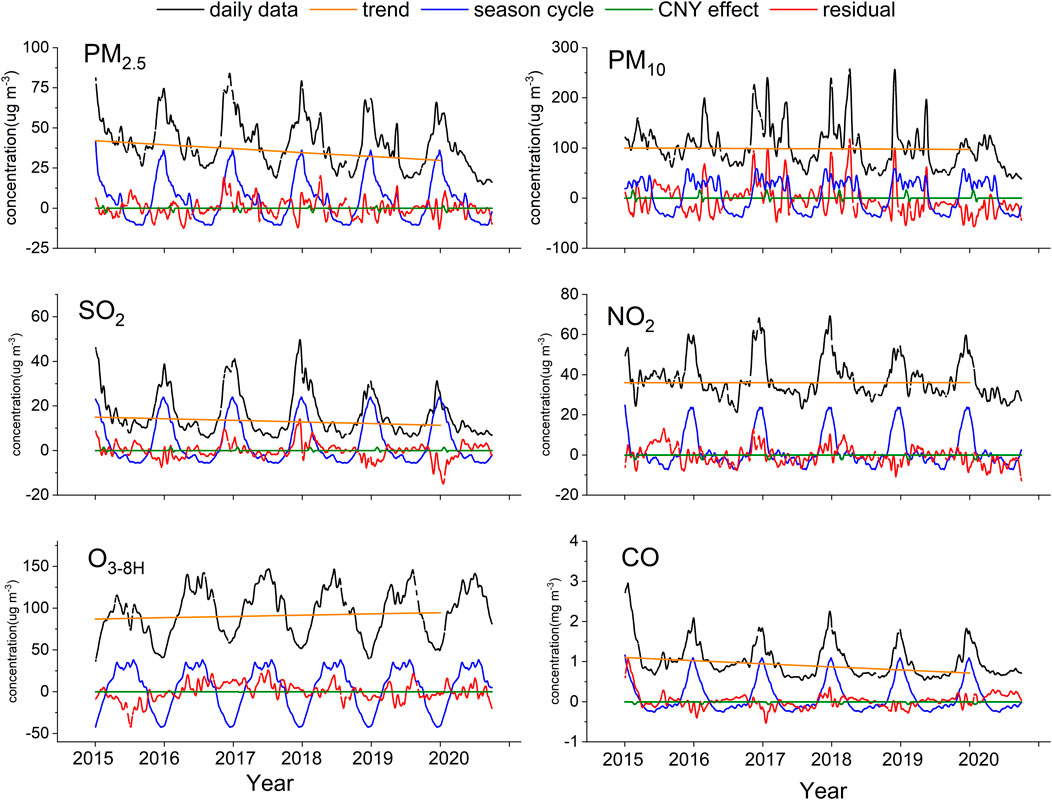
FIGURE 3. Daily time series for six pollutants (black), decomposed into the trend (orange), seasonal cycle (blue), Chinese New Year (CNY) effect (green) and residual (red) components. The data are smoothed using a LOWESS filter.
Although there are fluctuations in some months, the PM2.5, PM10, SO2, NO2 and CO concentrations during 2015–2019 generally showed high values in winter and spring, and low values in summer and autumn, which is a combination effect of both strong emissions of pollutants and relatively stable atmospheric condition in winter (Ma et al., 2019). The variation of the O3 concentration, with a peak in summer, is different from the other pollutants, which is mainly due to the enhanced photochemical reactions with abundant sunlight and high temperature in summer (Li et al., 2014; Li et al., 2019). However, there are still debates on whether anthropogenic pollution or stratospheric intrusion plays a more dominant role (Liu et al., 2019; Li et al., 2020).
Figure 3 demonstrates the effect of the Spring Festival on the concentration of pollutants, which show a generally increasing trend during the 7 days interval prior to CNY and a decreasing trend during the 7 days after CNY. PM2.5 peaked on two days before CNY (-2) and CNY day (0). PM2.5/PM10 was small during CNY (-2), largely caused by the floating dust event occurred during 25–26 January 2017 (Sand-dust Weather Almanac, 2017). A peak in PM2.5/PM10 occurred on the CNY day, when SO2 and O3–8 h also peaked, which may be related to emissions from fireworks. The concentrations of the six pollutants were lower than usual during the 7 days after the CNY; PM2.5 and NO2 decreased by 10.19 μg m−3 and 7.05 μg m−3 respectively. O3 concentrations decreased by 0.59 μg m−3 on average during the 7-day holiday, and a significant fall 3 days before the CNY was observed before the upward trend, which was contrary to the change in PM10.
3.2 Residuals analysis
The time-series decomposed components (i.e., the trend, seasonal cycle and effect of the CNY based on the 2015–2019 time series) were used to reconstruct the time series of the daily concentrations of each pollutant in 2020 (red lines in Figure 4). As shown in Figure 4, the reconstructed time series are in overall good agreement with the observations. The highest correlation coefficient (0.73) is for CO, while that for NO2 is the smallest (0.43). This indicates that the changes in the CO concentration were relatively stable, while NO2 was more variable.
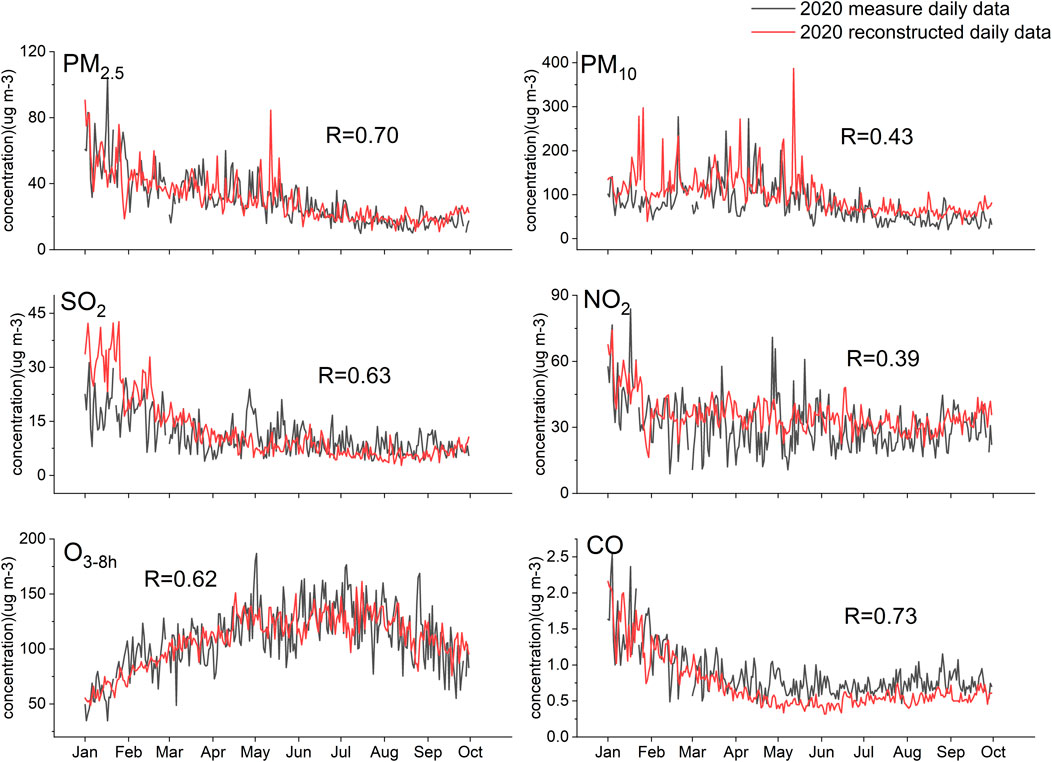
FIGURE 4. Comparison of the observed pollutant concentrations in 2020 with those reconstructed using the three components of the inter-annual trends, seasonal variations and CNY events, based on the data for 2015–2019.
Figure 5 shows the 7-day moving average residuals (observation minus reconstructed values) of the six pollutant concentrations in 2020, at different stages of the COVID-19 epidemic. The residuals of the six pollutants fluctuate around zero. The variation of the residuals are very well correlated at the urban and suburban sites, except for NO2 and O3–8h during P1, with the residuals at urban sites being more positive and generally larger than that at the suburban sites. Reference to the average residuals for the different periods (Supplementary Table S1) shows that the pollutant concentrations in Lanzhou did not decrease as sharply as might have been expected during P2.
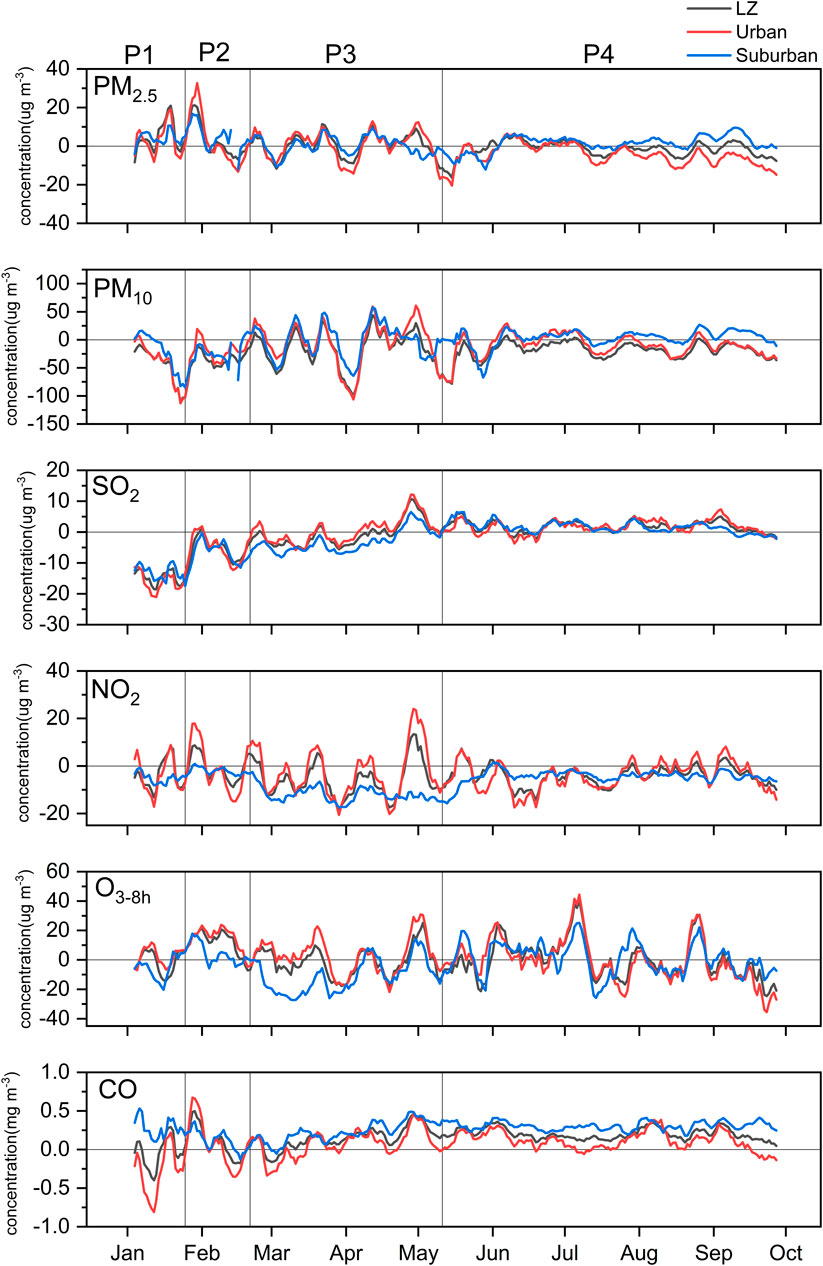
FIGURE 5. Changes in 7-day moving average residuals of six pollutants for all, urban and rural sites in 2020. P1, pre-lockdown; P2, Level Ⅰ response period; P3, Level Ⅲ response period; P4, relaxation period.
During P2 the PM2.5 concentration in Lanzhou increased by 2.48 μg m−3, an increase of 15.86% compared to the reconstructed value, and a decrease of 3.26 μg m−3 compared to pre-lockdown (Supplementary Table S1). In Figure 6, we compare the residuals of different pollutants during the four phases. PM2.5, SO2 and CO show monotonic changes during the four periods, which indicates that COVID-19 had a limited effect on the concentrations of the three pollutants. The residuals of PM2.5 decreased monotonically and that of CO increased monotonically during the study period, which may be related to the large seasonal decrease in the background concentration or a reduction of the inter-annual trend. Although SO2 showed a monotonic increase during the four stages of the study period, the concentration of SO2 was lower than the reconstructed value, except during P4.
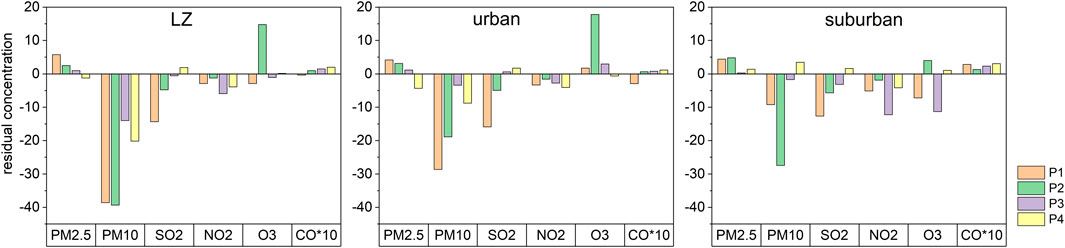
FIGURE 6. Comparison of the 7-day moving average residuals of the six pollutants at different stages of the epidemic. Note: CO is multiplied by ten for clarity.
PM10 decreased during the COVID-19 lockdown, falling by 23.40% if only the P2 stage, with the strictest controls, is considered. PM10 decreased by 1.81 μg m−3 compared to pre-lockdown (Supplementary Table S1). The variation of PM10 differed between urban and suburban areas during the study period. The PM10 at urban sites during P2 increased by 9.79 μg m−3, an increase of 14.86%, compared with pre-lockdown. PM10 in the suburban site decreased by 13.56% compared to pre-lockdown. During the period when the COVID-19 lockdown was relaxed (P4) and the restrictions were lifted, the concentration of PM10 at the suburban site rebounded to the reconstructed value, although it did not reach the expected values.
NO2 was low during the Level Ⅲ response period (P3) (-5.91 μg m−3, -14.65%), while a slight decrease occurred during the strict lockdown period (P2). The decline during P3 was mainly at the suburban site. The NO2 in the suburban of Lanzhou decreased by 53.91% compared to the expected values. NO2 decreased more during P3 than during P2, which may be due to the overlap of the CNY and the epidemic during this period. We removed the New Year effect to determine the impact of the epidemic, but during CNY, people gather in families to celebrate the holiday, which is to some extent the same behavior as the response to the home isolation measures undertaken to control the epidemic. As a result, the impact of the epidemic may have been reduced by removing the effect of CNY. Ignoring the CNY holiday, it can be inferred that the NO2 concentration 14 days after CNY decreased by 0.48% compared to the 14 days before CNY, and by 8.63% compared to the reconstructed values during the same period. During the P3 stage, the difference between urban and suburban areas indicates that the suburban areas were more affected by the control measures implemented during the COVID-19 lockdown than urban areas. In general, for Lanzhou, the COVID-19 lockdown had a relatively small impact on NO2 concentrations.
Among the pollutants studied, O3 was the most obviously affected by the COVID-19 lockdown, and the difference in residuals between the urban and the suburban areas during the lockdown period was also the largest. During the strict lockdown (P2), the O3 concentration in Lanzhou increased by 19.14% compared to the reconstructed value. Both urban and suburban sites showed an increase during the P2 stage, with the urban areas increasing more, with an increase of 24.60%. This rise in O3 during the lockdown period has been widely reported in China and even globally (Silver et al., 2020; Grange et al., 2021). In particular, during the Level Ⅲ response (P3), the O3 concentration in Lanzhou decreased by 19.80% compared to the strict control phrase (P2). Both urban and suburban sites showed a decrease during P3. The O3 concentration in suburban areas decreased by -11.31 μg m−3 (-7.97%) compared with the reconstructed value during P3, and it decreased by 13.07% compared to P2. The O3 concentration in urban areas increased by 2.97 μg m−3 (3.54%) compared to the reconstructed value in P3, but it decreased by 21.06% compared to P2. The analysis of O3 is complicated by the spatio-temporal heterogeneity of its production and the nonlinear chemical response to NOX and VOC emissions (Jin and Halloway, 2015). Due to the influence of atmospheric chemistry, changes in the NO2 concentration do not reflect the same relative change in NOX emissions (Keller et al., 2021). Previous studies have reported that the elevated O3 during lockdowns was mainly attributed to the enhanced atmospheric oxidation capacity in northern China (Le et al., 2020), the North China emission reduction during lockdown (Zhu et al., 2021). Chemistry of secondary aerosols and ozone as well as the meteorological conditions may have contributed positively to the anomalous enhancement in O3 during the lockdown in Lanzhou, which merit further investigation.
3.3 Comparison of residuals between 2020 and 2015–2019
Figure 7 compares the 7-day moving average residuals during the study period with those of the same period of the previous five years. In addition to changes related to the inter-annual trend, the seasonal cycle and the CNY effect, there are complex interactions between atmospheric chemistry and meteorology. To assess the impact of the restriction measures after the COVID-19 outbreak, we consider the change in residuals during the same period of 2015–2019 as the normal range of fluctuation of the pollutants. A Student t test at the 0.1 significance level revealed a large negative SO2 anomaly and positive O3 anomaly during the lockdown (P2), with a large positive CO anomaly and a negative PM10 anomaly during the relaxation period, compared to the anomalies during the previous five years. A large positive SO2 anomaly (significant at the 0.25 level) and a negative NO2 anomaly (significant at the 0.25 level) were also observed during P4. This indicates that there was a reduction in the trend of decreasing CO and SO2 in 2020 compared with that during 2015–2019, while PM10 continued to decrease beyond expectations. This indicates the effectiveness of air pollution control measures, and it also shows that air quality management is a long-term process. The PM2.5, NO2 and O3 residuals maintained the same rate of change as during the previous five years. The increase in O3 in urban areas during the strict lockdown period and the decrease in NO2 in suburban areas during the Level Ⅲ response were significantly beyond expectations (Supplementary Figure S2).
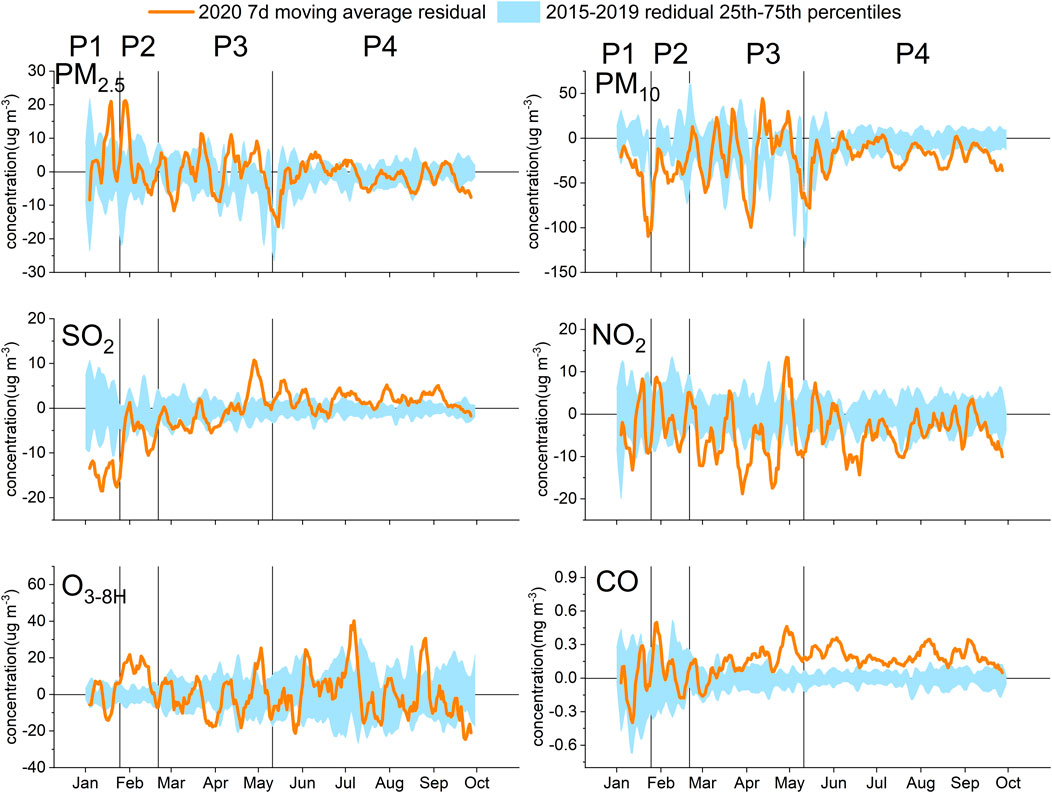
FIGURE 7. Comparison of the residual concentrations of pollutants in 2020 with those for same period in 2015–2019. The 25th and 75th percentiles of averaged detrended concentrations for 2015–2019 (blue shading) are shown.
The impact of the COVID-19 lockdown measures on air quality was not as great as we expected. A similar conclusion was reached in other studies, although different methods were used. The results of Wang et al. (2021) showed that the lockdown temporarily improved the air quality in China, but the rate of reduction of SO2, NO2, and CO was very small. Pei et al. (2020) believed that the air quality in the urban areas of China did not improve overall during the lockdown. Under the restrictions imposed to reduce COVID-19 infections, there were several improvements in air quality, but they were not as effective as we expected. To further improve the air quality in Lanzhou City, detailed source-response studies are needed.
4 Conclusion
Time-series decomposition was used to quantify the changes in air quality in Lanzhou City during the COVID-19 epidemic. During the epidemic prevention and control period in Lanzhou, only PM10 decreased (by 23.40%) compared with the expected values over the same period, with a 1.80 μg m−3 decrease compared with that before the lockdown. The NO2 concentration 14 days after CNY fell by 0.48% compared with that 14 days before, and by 8.63% compared with the reconstructed value for the same period. The O3 response depends on the season, time scale, and environment. During the period of the COVID-19 lockdown, the O3 concentration in Lanzhou increased by 19.14% compared to the reconstructed value during P2, and it then decreased by 19.80% during the Level Ⅲ response (P3). Compared with the past five years, the changes during the COVID-19 epidemic were generally within the normal range of fluctuations. The PM10 concentration in Lanzhou continued to decrease, but there was a reduction in the rate of decrease in SO2 and CO, while PM2.5, NO2 and O3 maintained the same trend as during the previous five years. It is important to determine the cause of the widespread increase in O3 in future studies. In short, we believe that the COVID-19 lockdown did indeed reduce the levels of several air pollutants in Lanzhou, but the temporary social lockdown measures were insufficient to further improve the atmospheric environment. Atmospheric pollutants require comprehensive and coordinated mitigation measures, which highlights the importance of reducing the concentration of O3 in the future.
Data availability statement
Publicly available datasets were analyzed in this study. This data can be found here: the China National Environmental Monitoring Network (http://www.cnemc.cn).
Author contributions
HL: Conceptualization, Methodology, Writing-original draft. YY: Conceptualization, Data curation, Methodology, Writing-review and editing, Supervision. DX: Writing-review and editing, Supervision. XM: Methodology. XL: Methodology. LD: Methodology.
Funding
This work was supported by the Gansu Science and Technology Plan (18JR2RA005).
Acknowledgments
Thanks to Jan Bloemendal from the University of Liverpool for suggestions and proofreading the article.
Conflict of interest
The authors declare that the research was conducted in the absence of any commercial or financial relationships that could be construed as a potential conflict of interest.
Publisher’s note
All claims expressed in this article are solely those of the authors and do not necessarily represent those of their affiliated organizations, or those of the publisher, the editors and the reviewers. Any product that may be evaluated in this article, or claim that may be made by its manufacturer, is not guaranteed or endorsed by the publisher.
Supplementary material
The Supplementary Material for this article can be found online at: https://www.frontiersin.org/articles/10.3389/feart.2022.1011536/full#supplementary-material.
References
Brimblecombe, P., and Lai, Y. (2021). Diurnal and weekly patterns of primary pollutants in Beijing under COVID-19 restrictions. Faraday Discuss. 226, 138–148. doi:10.1039/D0FD00082E
Burkey, J. (2006). A non-parametric monotonic trend test computing Mann-Kendall Tau, Tau-b, and Sen’s slope written in Mathworks-MATLAB implemented using matrix rotations. Seattle, Washington. USA: King County, Department of Natural Resources and Parks, Science and Technical Services Section. Available at: http://www.mathworks.com/matlabcentral/fileexchange/authors/23983 (accessed March 13, 2021).
Chen, L., Chien, L. C., Li, Y., and Lin, G. (2020). Nonuniform impacts of COVID-19 lockdown on air quality over the United States. Sci. Total Environ. 745, 141105. doi:10.1016/j.scitotenv.2020.1411055
Cleveland, W. S., Grosse, E., and Shyu, W. M. (2017). Local regression models. Statistical models in S. England,UK: Routledge, 309–376.
Donzelli, G., Cioni, L., Cancellieri, M., Llopis Morales, A., and Morales Suárez-Varela, M. M. (2020). The effect of the Covid-19 lockdown on air quality in three Italian medium-sized cities. Atmosphere 11 (10), 1118. doi:10.3390/atmos11101118
Fan, H., Zhao, C., and Yang, Y. (2020). A comprehensive analysis of the spatio-temporal variation of urban air pollution in China during 2014–2018. Atmos. Environ. X. 220, 117066. doi:10.1016/j.atmosenv.2019.117066
Grange, S. K., Lee, J. D., Drysdale, W. S., Lewis, A. C., Hueglin, C., Emmenegger, L., et al. (2021). COVID-19 lockdowns highlight a risk of increasing ozone pollution in European urban areas. Atmos. Chem. Phys. 21 (5), 4169–4185. doi:10.5194/acp-2020-1171
He, G., Pan, Y., and Tanaka, T. (2020). The short-term impacts of COVID-19 lockdown on urban air pollution in China. Nat. Sustain. 3 (12), 1005–1011. doi:10.1038/s41893-020-0581-y
Jin, X., and Holloway, T. (2015). Spatial and temporal variability of ozone sensitivity over China observed from the Ozone Monitoring Instrument. J. Geophys. Res. Atmos. 120 (14), 7229–7246. doi:10.1002/2015JD023250
Keller, C. A., Evans, M. J., Knowland, K. E., Hasenkopf, C. A., Modekurty, S., Lucchesi, R. A., et al. (2021). Global impact of COVID-19 restrictions on the surface concentrations of nitrogen dioxide and ozone. Atmos. Chem. Phys. 21 (5), 3555–3592. doi:10.5194/acp-21-3555-2021
Kuerban, M., Waili, Y., Fan, F., Liu, Y., Qin, W., Dore, A. J., et al. (2020). Spatio-temporal patterns of air pollution in China from 2015 to 2018 and implications for health risks. Environ. Pollut. 258, 113659. doi:10.1016/j.envpol.2019.113659
Le, T., Wang, Y., Liu, L., Yang, J., Yung, Y. L., Li, G., et al. (2020). Unexpected air pollution with marked emission reductions during the COVID-19 outbreak in China. Science 369 (6504), 702–706. doi:10.1126/science.abb7431
Li, K., Jacob, D. J., Liao, H., Shen, L., Zhang, Q., and Bates, K. H. (2019). Anthropogenic drivers of 2013–2017 trends in summer surface ozone in China. Proc. Natl. Acad. Sci. U. S. A. 116 (2), 422–427. doi:10.1073/pnas.1812168116
Li, L., Li, Q., Huang, L., Wang, Q., Zhu, A., Xu, J., et al. (2020). Air quality changes during the COVID-19 lockdown over the Yangtze River Delta Region: An insight into the impact of human activity pattern changes on air pollution variation. Sci. Total Environ. 732, 139282. doi:10.1016/j.scitotenv.2020.139282
Li, X., Liu, J., Mauzerall, D. L., Emmons, L. K., Walters, S., Horowitz, L. W., et al. (2014). Effects of trans-Eurasian transport of air pollutants on surface ozone concentrations over Western China. J. Geophys. Res. Atmos. 119 (21), 12, 338–412, 354. doi:10.1002/2014JD021936
Liu, J., Li, W., and Li, J. (2016). Quality screening for air quality monitoring data in China. Environ. Pollut. 216, 720–723. doi:10.1016/j.envpol.2016.06.037
Liu, N., Lin, W., Ma, J., Xu, W., and Xu, X. (2019). Seasonal variation in surface ozone and its regional characteristics at global atmosphere watch stations in China. J. Environ. Sci. 77, 291–302. doi:10.1016/j.jes.2018.08.009
Ma, X., Jia, H., Sha, T., An, J., and Tian, R. (2019). Spatial and seasonal characteristics of particulate matter and gaseous pollution in China: Implications for control policy. Environ. Pollut. 248, 421–428. doi:10.1016/j.envpol.2019.02.038
Ma, Y., Ma, B., Jiao, H., Zhang, Y., Xin, J., and Yu, Z. (2020). An analysis of the effects of weather and air pollution on tropospheric ozone using a generalized additive model in Western China: Lanzhou, Gansu. Atmos. Environ. 224, 117342. doi:10.1016/j.atmosenv.2020.117342
Munir, S., Habeebullah, T. M., Seroji, A. R., Gabr, S. S., Mohammed, A. M., and Morsy, E. A. (2013). Quantifying temporal trends of atmospheric pollutants in Makkah (1997–2012). Atmos. Environ. X. 77, 647–655. doi:10.1016/j.atmosenv.2013.05.075
Munir, S., Luo, Z., and Dixon, T. (2021). Comparing different approaches for assessing the impact of COVID-19 lockdown on urban air quality in Reading, UK. Atmos. Res. 261, 105730. doi:10.1016/j.atmosres.2021.105730
Neeti, N., and Eastman, J. R. (2011). A contextual mann-kendall approach for the assessment of trend significance in image time series. Trans. GIS 15 (5), 599–611. doi:10.1111/j.1467-9671.2011.01280.x
Pei, Z., Han, G., Ma, X., Su, H., and Gong, W. (2020). Response of major air pollutants to COVID-19 lockdowns in China. Sci. Total Environ. 743, 140879. doi:10.1016/j.scitotenv.2020.140879
Sen, P. K. (1968). Estimates of the regression coefficient based on Kendall's tau. J. Am. Stat. Assoc. 63 (324), 1379–1389. doi:10.1080/01621459.1968.10480934
Shi, G. L., Liu, G. R., Tian, Y. Z., Zhou, X. Y., Peng, X., and Feng, Y. C. (2014). Chemical characteristic and toxicity assessment of particle associated PAHs for the short-term anthropogenic activity event: During the Chinese new year's festival in 2013. Sci. Total Environ. 482, 8–14. doi:10.1016/j.scitotenv.2014.02.107
Shi, Z., Song, C., Liu, B., Lu, G., Xu, J., Van Vu, T., et al. (2021). Abrupt but smaller than expected changes in surface air quality attributable to COVID-19 lockdowns. Sci. Adv. 7 (3), eabd6696. doi:10.1126/sciadv.abd6696
Silver, B., He, X., Arnold, S. R., and Spracklen, D. V. (2020). The impact of COVID-19 control measures on air quality in China. Environ. Res. Lett. 15 (8), 084021. doi:10.1088/1748-9326/aba3a2
Silver, B., Reddington, C. L., Arnold, S. R., and Spracklen, D. V. (2018). Substantial changes in air pollution across China during 2015–2017. Environ. Res. Lett. 13 (11), 114012. doi:10.1088/1748-9326/aae718
Singh, V., Singh, S., Biswal, A., Kesarkar, A. P., Mor, S., and Ravindra, K. (2020). Diurnal and temporal changes in air pollution during COVID-19 strict lockdown over different regions of India. Environ. Pollut. 266, 115368. doi:10.1016/j.envpol.2020.115368
Tan, P. H., Chou, C., Liang, J. Y., Chou, C. C. K., and Shiu, C. J. (2009). Air pollution “holiday effect” resulting from the Chinese New Year. Atmos. Environ. X. 43 (13), 2114–2124. doi:10.1016/j.atmosenv.2009.01.037
Theil, H. (1992). “A rank-invariant method of linear and polynomial regression analysis,” in Henri Theil’s contributions to economics and econometrics (Berlin, Germany: Springer), 345–381.
Wang, M., Liu, F., and Zheng, M. (2021). Air quality improvement from COVID-19 lockdown: Evidence from China. Air Qual. Atmos. Health 14 (4), 591–604. doi:10.1007/s11869-020-00963-y
Wang, S., Feng, X., Zeng, X., Ma, Y., and Shang, K. (2009). A study on variations of concentrations of particulate matter with different sizes in Lanzhou, China. Atmos. Environ. 43 (17), 2823–2828. doi:10.1016/j.atmosenv.2009.02.021
Wang, Y., Wen, Y., Wang, Y., Zhang, S., Zhang, K. M., Zheng, H., et al. (2020). Four-month changes in air quality during and after the COVID-19 lockdown in six megacities in China. Environ. Sci. Technol. Lett. 7 (11), 802–808. doi:10.1021/acs.estlett.0c00605
Wu, L., and Zhang, J. (2018). Assessing population movement impacts on urban heat island of beijing during the Chinese new year holiday: Effects of meteorological conditions. Theor. Appl. Climatol. 131 (3), 1203–1210. doi:10.1007/s00704-017-2043-7
Yan, C., Wang, L., and Zhang, Q. (2021). Study on coupled relationship between urban air quality and land use in Lanzhou, China. Sustainability 13 (14), 7724. doi:10.3390/su13147724
Yin, Z., Huang, X., He, L., Cao, S., and Zhang, J. J. (2020). Trends in ambient air pollution levels and PM2. 5 chemical compositions in four Chinese cities from 1995 to 2017. J. Thorac. Dis. 12 (10), 6396–6410. doi:10.21037/jtd-19-crh-aq-004
Zhao, S. P., Yu, Y., and Qin, D. H. (2018). From highly polluted inland city of China to “Lanzhou Blue”: The air-pollution characteristics. Sci. Cold Arid. Reg. 10 (1), 12–26. doi:10.3724/SP.J.1226.2018.00012
Zhao, S. P., Yu, Y., Yin, D. Y., and Qin, D. H. (2021). Contrasting response of ultrafine particle number and PM2:5 mass concentrations to Clean Air Action in China. Geophys. Res. Lett. 48, e2021GL093886. doi:10.1029/2021GL093886
Zhao, S., Yu, Y., Yin, D., Liu, N., and He, J. (2014). Ambient particulate pollution during Chinese Spring festival in urban Lanzhou, northwestern China. Atmos. Pollut. Res. 5 (2), 335–343. doi:10.5094/APR.2014.039
Keywords: lockdown, Lanzhou, O3, particulate matter, COVID-19
Citation: Liu H, Yu Y, Ma X, Liu X, Dong L and Xia D (2023) Monitoring the impact of the COVID-19 lockdown on air quality in Lanzhou: Implications for future control strategies. Front. Earth Sci. 10:1011536. doi: 10.3389/feart.2022.1011536
Received: 04 August 2022; Accepted: 14 September 2022;
Published: 09 January 2023.
Edited by:
Dipesh Rupakheti, Nanjing University of Information Science and Technology, ChinaReviewed by:
Worradorn Phairuang, Kanazawa University, JapanShani Tiwari, Council of Scientific and Industrial Research, India
Copyright © 2023 Liu, Yu, Ma, Liu, Dong and Xia. This is an open-access article distributed under the terms of the Creative Commons Attribution License (CC BY). The use, distribution or reproduction in other forums is permitted, provided the original author(s) and the copyright owner(s) are credited and that the original publication in this journal is cited, in accordance with accepted academic practice. No use, distribution or reproduction is permitted which does not comply with these terms.
*Correspondence: Ye Yu, eXl1QGx6Yi5hYy5jbg==; Dunsheng Xia, ZHN4aWFAbHp1LmVkdS5jbg==