- 1State Key Laboratory on Internet of Things for Smart City and Department of Civil and Environmental Engineering, University of Macau, Macau, China
- 2China Construction Eighth Engineering Bureau Co., Ltd., Shanghai, China
- 3South Company of China Construction Eighth Engineering Bureau Co., Ltd., Nanning, China
Editorial on the Research Topic
Spatial modelling and failure analysis of natural and engineering disasters through data-based methods
Numerous natural and engineering disasters are observed frequently due to the climate change and the human engineering activities. In recent years, a large number of tunnels, metros and underground spaces were constructed to reduce congestion and store potential resources with the development of infrastructure construction (Font-Capoet al., 2011; Liu and Zou 2022). A respective example in China is that the length of constructed, under constructing, and planning railway tunnels were up to 15,781 km, 8,200 km, and 12,800 km, as reported by Guo (2018). The rapid construction caused significant challenges of engineering disasters when encountering the unfavorable conditions, e.g., karst, fault, and weathered slot (Høien and Nilsen, 2014; Liu et al., 2018a, b; Golian et al., 2021). As shown in Figure 1, main engineering disasters related to the engineering construction include the water and mud inrush, soil/rock collapse, erosion, surface subsidence and crack (Liu et al., 2019, 2022; Zhang et al., 2019). These disasters may induce the serious influences on the hydraulic-geological-ecological environment (e.g., groundwater decline) and usually cause the stoppages for the projects, huge property damages, and deaths to the constructors and surface residents (Hayashi et al., 2009; Wang et al., 2019). Thus, it is necessary to establish the effective model or method to predict these disasters and their related influences on the ground and surface environment.
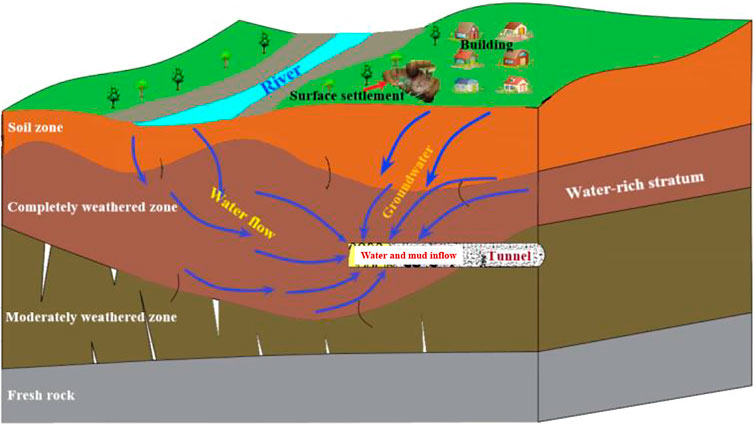
FIGURE 1. Typical unfavorable ground and the related geological disaster induced by tunnel construction.
Considering this practical demand, a special topic, entitled “Spatial Modelling and Failure Analysis of Natural and Engineering Disasters through Data-based Methods” was initiated with several editors to process the timely peer-review and publication of relevant manuscript by 12 March 2021. The main objective of this Research Topic is to provide a set of peer-reviewed publications that propose innovative data-based methods and techniques such as machine/deep learning and advanced numerical methods to investigate the various engineering and natural disasters. In this Research Topic, 41 manuscripts were accepted in total, indicating the acute need for the engineers and scholars to understand the aspects of engineering and natural disasters.
During the published papers, many machine learning methods were applied into the analysis of various engineering and natural disasters. For example, Wang et al. used an advanced gradient boosting algorithms to analysis the slope stability under the seismic effect. Zhu and Guan developed an improved machine model to predict the size of water-conducting region which was the key to prevent the roof water disasters during coal engineering. Liu et al. proposed an integrated model by considering the generative adversarial network and support vector machine, aiming to identify the seismic events more accurately. Also, some new numerical techniques as well as the theoretical models were proposed for many geological disasters, such as rockburst and large deformation in tunnel, deformation and stability in pile, and slope failure and rockfall (Fu et al.; Liang et al.; Zheng et al.). For example, Fan et al. analyzed the tunnel rockburst based on a field case (i.e., Jinping hydropower station) and they discussed the prediction method of rockburst based on the Hoek-Brown strength criterion and the energy release process. Zhou et al. implemented the discrete element method (DEM) to investigate the landslide failure process. Hu et al. developed a theoretical model to evaluate the large deformation of tunnel when tunnel constructed in a soft ground.
The studies in current Research Topic reflect the substantial improvements in the data-based methods and techniques in the analysis of the various engineering and natural disasters, which may provide the important references for the prediction and prevention of these disasters.
Author contributions
All authors listed have made a substantial, direct, and intellectual contribution to the work and approved it for publication.
Acknowledgments
The authors would like to thank the hundreds of reviewers and scientists who contributed to this Research Topic. Particular thanks go to the board of the Frontiers Publishing house for their supports of this Research Topic.
Conflict of interest
TY, ZY, SW, GH, CoL, CnL, and RX were employed by China Construction Eighth Engineering Bureau Co., Ltd. and South Company of China Construction Eighth Engineering Bureau Co., Ltd.
The remaining author declares that the research was conducted in the absence of any commercial or financial relationships that could be construed as a potential conflict of interest.
Publisher’s note
All claims expressed in this article are solely those of the authors and do not necessarily represent those of their affiliated organizations, or those of the publisher, the editors and the reviewers. Any product that may be evaluated in this article, or claim that may be made by its manufacturer, is not guaranteed or endorsed by the publisher.
References
Font-Capó, J., Vázquez-Suñé, E., Carrera, J., Martí, D., Carbonell, R., and Pérez-Estaun, A. (2011). Groundwater inflow prediction in urban tunneling with a tunnel boring machine (TBM). Eng. Geol. 121 (1), 46–54. doi:10.1016/j.enggeo.2011.04.012
Golian, M., Teshnizi, E. S., Parise, M., Terzic, J., Milanovic, S., Vakanjac, V. R., et al. (2021). A new analytical method for determination of discharge duration in tunnels subjected to groundwater inrush. Bull. Eng. Geol. Environ. 80, 3293–3313. doi:10.1007/s10064-021-02140-6
Guo, S. Y. (2018). Technical development and outlook of the tunnels and underground works in China. Mod. Tunn. Technol. 55, 1. doi:10.13807/j.cnki.mtt.2018.S2.001
Hayashi, T., Tokunaga, T., Aichi, M., Shimada, J., and Taniguchi, M. (2009). Effects of human activities and urbanization on groundwater environments: an example from the aquifer system of tokyo and the surrounding area. Sci. Total Environ. 407 (9), 3165–3172. doi:10.1016/j.scitotenv.2008.07.012
Høien, A., and Nilsen, B. (2014). Rock mass grouting in the løren tunnel: case study with the main focus on the groutability and feasibility of drill parameter interpretation. Rock Mech. Rock Eng. 47 (3), 967–983. doi:10.1007/s00603-013-0386-7
Liu, J., Chen, W., Nie, W., Yuan, J., and Dong, J. (2019). Experimental research on the mass transfer and flow properties of water inrush in completely weathered granite under different particle size distributions. Rock Mech. Rock Eng. 52 (7), 2141–2153. doi:10.1007/s00603-018-1719-3
Liu, J., Chen, W., Yang, D., Yuan, J., Li, X., and Zhang, Q. (2018a). Nonlinear seepage–erosion coupled water inrush model for completely weathered granite. Mar. Georesources Geotechnol. 36, 484–493. doi:10.1080/1064119x.2017.1340373
Liu, J., Chen, W., Yuan, J., Li, C., Zhang, Q., and Li, X. (2018b). Groundwater control and curtain grouting for tunnel construction in completely weathered granite. Bull. Eng. Geol. Environ. 77 (2), 515–531. doi:10.1007/s10064-017-1003-x
Liu, J. Q., Sun, Y. K., Li, C. J., Yuan, H. L., Chen, W. Z., Liu, X. Y., et al. (2022). Field monitoring and numerical analysis of tunnel water inrush and the environmental changes. Tunn. Undergr. Space Technol. 122, 104360. doi:10.1016/j.tust.2021.104360
Liu, J., and Zou, T. (2022). Identifying the outlier in tunnel monitoring data: an integration model. Comput. Commun. 188, 145–155. doi:10.1016/j.comcom.2022.03.002
Wang, X. T., Li, S. C., Xu, Z. H., Li, X. Z., Lin, P., and Lin, C. J. (2019). An interval risk assessment method and management of water inflow and inrush in course of karst tunnel excavation. Tunn. Undergr. Space Technol. 92, 103033. doi:10.1016/j.tust.2019.103033
Keywords: unfavorable geology, engineering disasters, data-based models, machine learning, advanced numerical method
Citation: Liu J, Yang T, Yong Z, Wang S, Huang G, Liang C, Liu C and Xiao R (2022) Editorial: Spatial modelling and failure analysis of natural and engineering disasters through data-based methods. Front. Earth Sci. 10:1000540. doi: 10.3389/feart.2022.1000540
Received: 22 July 2022; Accepted: 25 July 2022;
Published: 26 August 2022.
Edited and reviewed by:
Alexander Kokhanovsky, Max Planck Institute for Chemistry, GermanyCopyright © 2022 Liu, Yang, Yong, Wang, Huang, Liang, Liu and Xiao. This is an open-access article distributed under the terms of the Creative Commons Attribution License (CC BY). The use, distribution or reproduction in other forums is permitted, provided the original author(s) and the copyright owner(s) are credited and that the original publication in this journal is cited, in accordance with accepted academic practice. No use, distribution or reproduction is permitted which does not comply with these terms.
*Correspondence: Jinquan Liu, jinquanliu99@163.com