- 1Key Laboratory of Meteorological Disaster, Ministry of Education, Collaborative Innovation Center on Forecast and Evaluation of Meteorological Disasters, Nanjing University of Information Science and Technology, Nanjing, China
- 2School of Atmospheric Sciences, Nanjing University of Information Science and Technology, Nanjing, China
The connections between the Madden–Julian Oscillation (MJO) and the Arctic Oscillation (AO) are examined in both observations and model forecasts. In the observations, the time-lag composites are carried out for AO indices and anomalies of 1,000-hPa geopotential height after an active or inactive initial MJO. The results show that when the AO is in its positive (negative) phase at the initial time, the AO activity is generally enhanced (weakened) after an active MJO. Reforecast data of the 11 operational global circulation models from the Sub-seasonal to Seasonal (S2S) Prediction Project are further used to examine the relationship between MJO activity and AO prediction. When the AO is in its positive phase on the initial day of the S2S prediction, an initial active MJO can generally improve the AO prediction skill in most of the models. This is consistent with results found in the observations that a leading MJO can enhance the AO activity. However, when the AO is in its negative phase, the relationship between the MJO and AO prediction is not consistent among the 11 models. Only a few S2S models provide results that agree with the observations. Furthermore, the S2S prediction skill of the AO is examined in different MJO phases. There is a significantly positive relationship between the MJO-related AO activity and the AO prediction skill. When the AO activity is strong (weak) in an MJO phase, including the inactive MJO, the models tend to have a high (low) AO prediction skill. For example, no matter what phase the initial AO is in, the AO prediction skill is generally high in MJO phase 7, in which the AO activity is generally strong. Thus, the MJO is an important predictability source for the AO forecast in the S2S models.
Introduction
The Madden–Julian Oscillation (MJO; Madden and Julian, 1971; Madden and Julian, 1972) is taken as a predictability source for the sub-seasonal to seasonal (S2S; 10–90 days) climate forecast (Ferranti et al., 1990; Jones et al., 2004; Reichler and Roads, 2005; Zhang et al., 2013; Jones and Dudhia, 2017; Specq and Batté, 2020). It can impact global weather and climate (Zhou and Miller, 2005; Lin and Brunet, 2009; Zhou et al., 2011; Alvarez et al., 2016; Zhou et al., 2016), in which the MJO can significantly affect the Arctic Oscillation (AO; Zhou and Miller, 2005; L’Heureux and Higgins, 2008; Cassou, 2008). In addition, the connection between the MJO and the AO plays an important role in adjusting the Northern Hemisphere climate on sub-seasonal timescales (Flatau and Kim, 2013; Song and Wu, 2019a; Song and Wu, 2019b). However, in numerical models, whether the MJO can provide predictability for the S2S forecast of the AO is still not well explored.
The MJO, which is a large-scale ocean–atmosphere-coupled system accompanied by strong deep convection and the wind anomaly traveling eastwardly in the tropics (Yoneyama et al., 2013), can modulate the climate in the Northern Hemisphere (Madden and Julian, 1994; Zhang, 2005). Initiating over the Indian Ocean, the MJO affects active and break of the Indian summer monsoon spell (Singh and Bhatla, 2019; Yang and Huang, 2021). When the MJO travels eastwardly to the Maritime Continent, the local precipitation is dominated by MJO convection (Vincent and Lane, 2018). In the tropics of the western Hemisphere, the wind anomaly caused by the MJO can induce the rainfall anomaly in the Amazon Basin (Mayta et al., 2020). In West Africa, an active MJO event enhances the heavy daily rainfall (Sossa et al., 2017). Outside of the tropics, the Rossby wave stirred by the MJO propagates northwardly (Matthews et al., 2004; Moon et al., 2011) and affects the climate from Central Asia to North America (Lin and Brunet, 2009; Zhou et al., 2011; Zhou et al., 2016; Wu and Takahashi, 2018; Wei et al., 2020). Moreover, the MJO can also influence the predictability of the air temperature in the mid-latitudes on sub-seasonal timescales in S2S models (Zhou et al., 2019). In the high latitudes, the anomaly of the surface air temperature over the Arctic region is significantly associated with the MJO (Yoo et al., 2012). The atmospheric circulation around the North Pole (e.g., AO) is also adjusted by the MJO (Zhou and Miller, 2005). Strength and tendency of the AO significantly respond to MJO phases (L'Heureux and Higgins, 2008; Flatau and Kim, 2013), which generally describe locations of MJO convective centers (Wheeler and Hendon, 2004). The connection between the MJO and the AO can be generally attributed to the northward and upward propagations of the wave fluxes in the troposphere and stratosphere (Garfinkel and Schwartz, 2017; Schwartz and Garfinkel, 2017).
The AO is another important atmospheric system impacting the weather and climate in the Northern Hemisphere. It was firstly proposed through performing the empirical orthogonal function (EOF) analysis on sea-level pressure in order to find the reflection of the polar vortex on the lower troposphere (Thompson and Wallace, 1998). Since then, many pieces of evidence have proven that the AO is the leading annular mode in the atmosphere over the Northern Hemisphere (Thompson and Wallace, 1998; Wallace and Thompson, 2002). Strength and phase of this annular mode strongly impact the weather and climate regimes in the Northern Hemisphere (He et al., 2017). During the positive (negative) phase of the AO, East Asia usually experiences a weak (strong) winter monsoon (Gong et al., 2001). Furthermore, the cold surges over Eurasia in winter are stronger during the negative phase of the AO than during the positive phase (Jeong and Ho, 2005; Park et al., 2011). Heavy fog tends to occur more frequently in northern China during the positive phase of the AO than during the negative phase (Liu et al., 2020). Besides the AO phase, the drought in China is found to be closely associated with AO strength (Gong and Ho, 2003; Yang et al., 2012). For the numerical S2S forecast, the AO is also taken as a predictability source for the 500-hPa geopotential height in the Northern Hemisphere (Minami and Takaya, 2020).
Recently, the S2S forecast in the polar region became a focus of many studies. The S2S Prediction Project (http://www.s2sprediction.net), including forecasts of 11 operational global circulation models, provides both opportunity and database for understanding this issue. For example, Garfinkel et al. (2020) tried to find impacts of Eurasian snow cover on the AO prediction in the S2S operational models. Lin et al. (2020) pointed out that S2S prediction skill of the air temperature over the Northern Hemisphere is lower in the polar region than in the other regions. Besides, in the polar region, the S2S forecast of the temperature initialized with the negative AO phase tends to have better prediction skill than the forecasts initialized with the positive AO phase. Furthermore, the linkage between the MJO and polar atmospheric circulation is regarded as an important aspect to evaluate the performance of the S2S models. For example, Vitart (2017) pointed out that predictability of the North Atlantic Oscillation is MJO phase-dependent in the S2S operational models. Therefore, it is worthwhile to explore the relationship between the MJO and AO predictability for the operational models in the S2S Prediction Project.
This paper is organized as follows. The Data and Methods section presents data and methods. The impacts of the MJO on AO prediction are provided in the Impacts of Madden–Julian Oscillation Activity on Arctic Oscillation Prediction section. The Arctic Oscillation Prediction Varying in Madden–Julian Oscillation Phases section further presents S2S prediction skill associated with MJO phases. Lastly, the Summary and Discussion section is the summary.
Data and Methods
Madden–Julian Oscillation and Arctic Oscillation Indices
The Australian Bureau of Meteorology (BoM) provides the daily bivariate MJO index during 1999–2010 at the website of http://www.bom.gov.au/climate/mjo/. This index was proposed by Wheeler and Hendon (2004) and includes RMM1 and RMM2, which are principal components of the first two EOFs of outgoing longwave radiation (OLR) and 850- and 200-hPa zonal winds. Before conducting the EOF, the seasonal cycle and inter-annual variability have been removed from the OLR and winds. The MJO amplitude and phase can be illustrated through using RMM1 and RMM2 and are
The daily AO index during 1999–2010 is downloaded from the ftp of the United States National Weather Service Climate Prediction Center (CPC) at ftp://ftp.cpc.ncep.noaa.gov/cwlinks/. This index is produced through projecting daily anomalies of 1,000-hPa geopotential height (GH) onto the loading pattern of the AO. The loading pattern is the first EOF of anomalies of the monthly GH at 1,000 hPa to the north of 20°N during 1979–2000. The downloaded AO index and its loading pattern were produced using the United States National Centers for Environmental Prediction Reanalysis I (NCEP RI). However, for model results, the monthly data of NCEP-the United States Department of Energy Reanalysis II (NCEP RII) are used to calculate the loading pattern of the AO. This is because more types of observations are assimilated into the NCEP RII data than the NCEP RI (Kalnay et al., 1996; Kanamitsu et al., 2002). The NCEP RII was obtained from the website at https://psl.noaa.gov/. This reanalysis has the horizontal resolution of 2.5° × 2.5°, and its monthly 1,000-hPa GH during 1979–2000 is used for computing the AO loading pattern to obtain the AO indices for model outputs. So far, the differences of results between usage of NCEP RI and NCEP RII are negligible in the present study (figures not shown). Before calculating AO prediction skill of model forecasts, the seasonal cycle has been removed from the observed AO index, but AO phases are identified based on the original one.
Sub-Seasonal to Seasonal Reforecast
The reforecast data during 1999–2010 are provided by 11 operational centers joining the S2S Prediction Project Phase I (Table 1; http://www.s2sprediction.net). These centers are the BoM, the China Meteorological Administration (CMA), the Environment and Climate Change Canada (ECCC), the European Centre for Medium-Range Weather Forecasts (ECMWF), the Hydrometeorological Centre of Russia (HMCR), the Italy Institute of Atmospheric Sciences and Climate of the National Research Council (ISAC-CNR), the Japan Meteorological Agency (JMA), the Korea Meteorological Administration (KMA), the Météo-France/Centre National de Recherche Meteorologiques (Meteo-France), the NCEP, and the United Kingdom Met Office (UKMO).
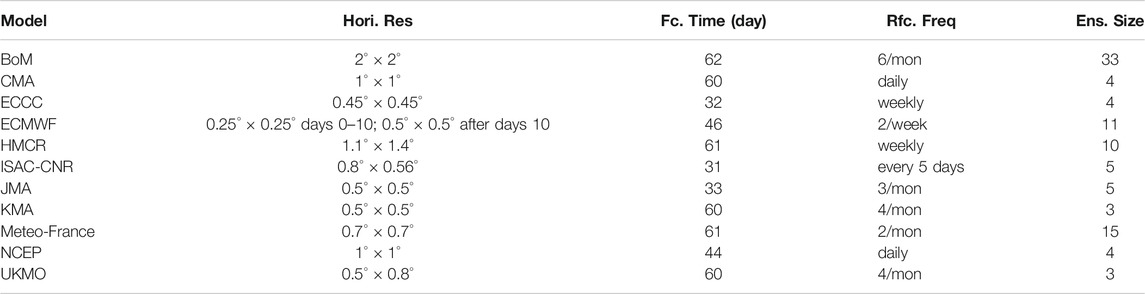
TABLE 1. Horizontal resolution (Hori. Res.), forecast time (Fc. Time), reforecast frequency (Rfc. Freq.), and ensemble size (Ens. Size) of the 11 sub-seasonal to seasonal (S2S) models (http://www.s2sprediction.net).
The S2S models were running with various horizontal resolutions (Hori. Res.) and had produced reforecast data with different forecast times (Fc. Time), reforecast frequencies (Rfc. Freq.), and ensemble sizes (Ens. Size). In Table 1, the horizontal resolutions of the models vary from the finest one of 0.25° × 0.25° to the coarsest one of 2° × 2°. Before being released by the project, the model results were resized into the same horizontal resolution of 1.5° × 1.5°, except that the BoM provided the data with horizontal resolution of 2.5° × 2.5°. The forecast time of the 11 models ranges from 31 to 62 days (Table 1). The reforecast of the first 26 days, including the initial day, is used for the analysis in this study. The reforecast frequencies vary from daily to twice a month (Table 1), and we use all the reforecast during 1999–2010 because this is the common period of the model results. In Table 1, the ensemble members are from 3 to 33. In the present study, we mainly focus on the impacts of the MJO on the S2S forecast, and thus the effects of ensemble members on AO forecast are not analyzed, which may need further research to explore.
The 1,000-hPa GH of the S2S model reforecasts was used to produce the AO index of prediction. The GH of all the 11 models was firstly interpolated into the horizontal resolution of 2.5° × 2.5°, which is the same as resolution of the NCEP reanalysis. Secondly, the model climatology during 1999–2010 was removed from the GH. At last, the GH anomalies were projected onto the observed loading pattern of AO to obtain the AO index of the S2S reforecast. It is noted that the loading pattern is obtained from the observations. The climatology of seasonal cycle during the reforecast period of each model is removed before calculating the AO prediction skill. The seasonal cycle is calculated for every forecast time of each model; for example, the climatology at forecast day 1 initiated on a date is computed using data on forecast day 1 that is initiated on the same date of each year during 1999–2010, and so on. For each model, the ensemble mean of the AO indices of all the members in each model is used to analyze the relationship between the MJO and the AO prediction. In this study, the prediction during the extended boreal winter (November to March) is mainly explored because the MJO and the AO have strong activities during boreal winter.
Significant Test
The significant test for the differences (Diff) of AO amplitude and prediction skill between the active and inactive MJO is carried out based on the Monte Carlo technique (Ebisuzaki,1997; Zhou et al., 2011; Zhou et al., 2016; Zhou et al., 2019). 1) We randomly shuffle the time sequence of MJO amplitude during the extended winter. This can randomly change the matches between time and MJO amplitude, and then an artificial time series of MJO amplitude is obtained. 2) The time with the active or inactive MJO is picked out according to the new shuffled sequence. 3) After that, the mean difference of a variable between the active and inactive MJO is calculated and defined as Diff_shuff. 4) The steps 1–3 are repeated 1,000 times to obtain the probability distribution of Diff_shuff. 5) At last, probability (p-value) of Diff_shuff ≥ Diff > 0 or Diff_shuff ≤ Diff < 0 can be obtained. If the p-value is less than or equal to 0.05 (0.1), it means that Diff_shuff ≥ Diff > 0 or Diff_shuff ≤ Diff < 0 is a small probability event, which means that the Diff value is not obtained by chance. Thus, the value of Diff is significant at the 5% or 10% level. On the contrary, if p > 0.05 (>0.1), the Diff is not significant.
Impacts of Madden–Julian Oscillation Activity on Arctic Oscillation Prediction
Observed Relationship Between Madden–Julian Oscillation and Arctic Oscillation Activities
We first check the observed relationship between MJO and AO activities. Figure 1 shows the time-lag composite of AO amplitude leading by the MJO activity. The AO amplitude can represent strength of the AO activity in its positive or negative phase, which exhibits the annular circulation mode in the low-level troposphere over the Northern Hemisphere. Therefore, the composites of the effects of the MJO on AO amplitudes are further separated into circumstances of positive (Figure 1A) and negative (Figure 1B) AO phases. It is noted that the AO phase is identified according to the original AO index on the initial day (day 0), but the composites are conducted using the AO index with the seasonal cycle being removed.
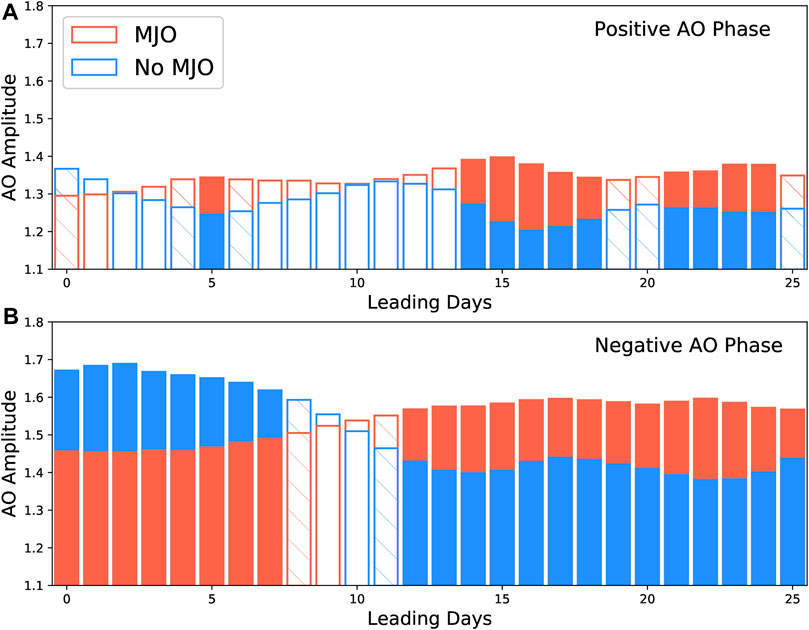
FIGURE 1. Time-lag composites of Arctic Oscillation (AO) amplitudes when the active (orange) or inactive (blue) Madden–Julian Oscillation (MJO) event leads the AO. The x-axis represents leading days, and y-axis is AO amplitude. The legend is shown in panel A. Panels A and B are for AO amplitude when the AO phases on leading day 0 are positive and negative, respectively. The bars filled with color (hatch) are significant at 5% (10%) level in terms of the differences between the AO amplitudes with and without the MJO on day 0. The significant test is based on the Monte Carlo method introduced in the Significant Test section.
When the AO is in its positive phase on the initial day, the time-lag composite of the AO amplitude with and without a leading initial MJO event is shown in Figure 1A. The x-axis shows the leading time of MJO activity to AO activity. When the leading days are 0 and 1, the AO activity is weaker with a leading MJO than an inactive MJO but only significant at the 10% level on day 0. On days 4–6, the AO activity with a leading MJO is stronger than an inactive MJO, and the differences are significant at the 5% (10%) level on day 5 (days 4 and 6). During days 14–25, the AO activity is significantly stronger with a leading MJO than an inactive MJO at the 5% level, except that the differences on days 19, 20, and 25 are significant at the 10% level. Those results indicate that in the positive phase of the AO, its activity tends to be enhanced after an MJO event happening for 4–6 and 14–25 days.
When the AO is in its negative phase on the initial day (Figure 1B), the AO activity during days 0–7 (on day 8) is significantly weaker with a leading MJO than an inactive MJO at the 5% (10%) level. After day 11, the AO activity with a leading MJO is significantly stronger than an inactive MJO at the 5% level, except that the difference on day 11 is significant at the 10% level. Thus, in the negative AO phase, the AO tends to have weak activity with an active MJO leading for 0–8 days but strong activity after the MJO event happening for 11 days.
As a supplement for the AO index, anomalies of 1,000-hPa GH are composed to further check the connections between MJO activity and the AO pattern on sub-seasonal timescales (Figures 2, 3). Before conducting the composite, the 1,000-hPa GH during 1999–2010 is firstly band-pass filtered with a cutoff of 10–90 days using the three-order Butterworth filter. After that, the GH anomalies during November–March are picked out for the composite, which is based on the MJO activity (A value).
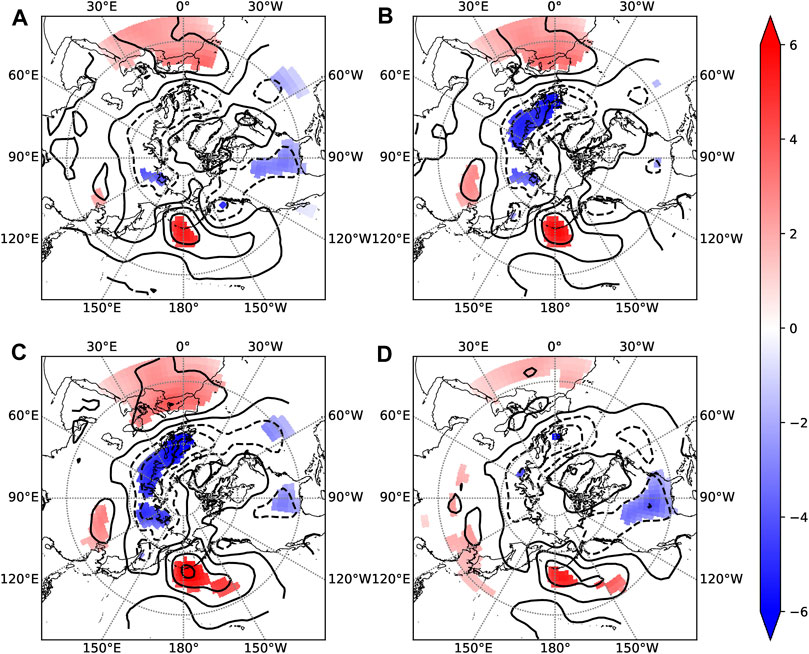
FIGURE 2. Composite of 1,000-hPa geopotential height (GH; contour) anomalies that are band-pass filtered with a cutoff of 10–90 days. The composite is carried out in terms of active Madden–Julian Oscillation (MJO) events (A ≥ 1). Panels (A–D) present the composites that the MJO leads GH for 0, 5, 10, and 15 days, respectively. Negative (positive) values are presented with dash (solid) lines. Shading areas are significant at the 5% level based on the Student’s t-test.
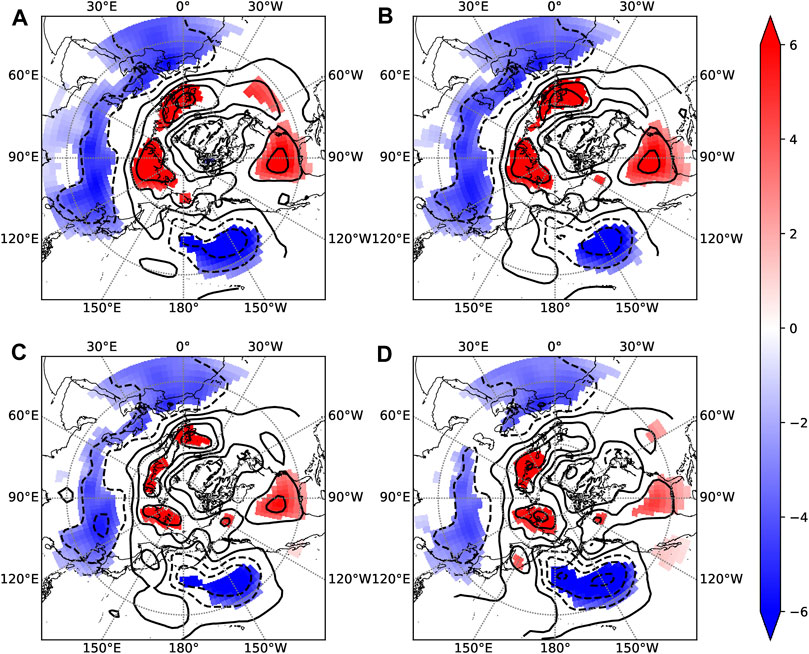
FIGURE 3. Same as Figure 2 but for the composites of inactive Madden–Julian Oscillation (MJO) events (A < 1). Panels (A–D) present the composites that the MJO leads GH for 0, 5, 10, and 15 days, respectively.
Figure 2 shows the composite of GH anomalies leading by an active MJO (A ≥ 1) for 0, 5, 10, and 15 days. On day 0 (Figure 2A), the GH anomalies and active MJO happen contemporarily. There are negative anomalies over northern Eurasia but not significant, except that the anomalies around 120°E are significant at the 5% level based on the Student’s t-test. Besides, the negative anomalies over southeastern America and part of the Atlantic are also significant. For positive anomalies, they are significant over northern Europe and the northern Pacific. When the MJO leads the composite for 5 and 10 days (Figures 2B, C), the patterns of GH anomalies are generally the same as that in Figure 2A. Moreover, the strength of the anomalies is increased, especially for the center of significantly negative GH anomalies over northern Eurasia. The distribution of the GH anomalies lagging the MJO for 15 days Figure 2D is quite similar to those in Figures 2A–C, but the strength of the anomalies decreases. The pattern presented in Figure 2 is quite similar (opposite) to the AO pattern in its positive (negative) phase. This indicates that when there is a leading active MJO, the positive (negative) AO pattern tends to be enhanced (weakened).
When the MJO is inactive (A < 1), time-lag composite of GH anomalies is shown in Figure 3. On day 0, the GH anomalies are significantly positive along northern Eurasia, and the significantly negative anomalies are found over Europe and the northern Pacific. Moreover, there are also significantly negative (positive) anomalies along the 30°N over Eurasia (over southeastern America and part of the Atlantic). On days 5, 10, and 15, the very similar pattern as that in Figure 3A is found in Figures 3B–D, respectively, but the strength of the GH anomalies decreases. The distribution of the significant anomalies in Figure 3 over northern Eurasia, Europe, and northern Pacific is reminiscent of the pattern of the AO in its negative phase. Thus, when the MJO is inactive, the negative (positive) AO pattern tends to be enhanced (weakened).
Overall, the results shown in Figures 2 and 3 are generally consistent with those presented in Figure 1. When the AO is in its positive phase with (without) an active MJO, the strength of the AO during the following 15 days can be generally enhanced (weakened). On the contrary, when the AO is in its negative phase with (without) an active MJO, the strength of the AO during the following 15 days is generally weakened (enhanced).
Influence of the Madden–Julian Oscillation on Arctic Oscillation Prediction
As there is a relationship between the AO and the MJO in the observations, it is interesting to explore whether this relationship can be reflected by the S2S models in their AO prediction. Figures 4 and 5 provide the skill of the 11 S2S model predictions of the AO in its positive and negative phases, respectively. In each figure, the prediction skill is separated into the circumstances that forecast is initiated with or without an active MJO (decided by A ≥ 1 or A < 1). The prediction skill is the correlation between AO indices of model forecasts and the observations. When the correlation skill is greater than or equal to 0.5, the forecast is taken as useful prediction. The significance of the differences between the prediction skill with and without the MJO is also presented based on the Monte Carlo concept introduced in Significant Test.
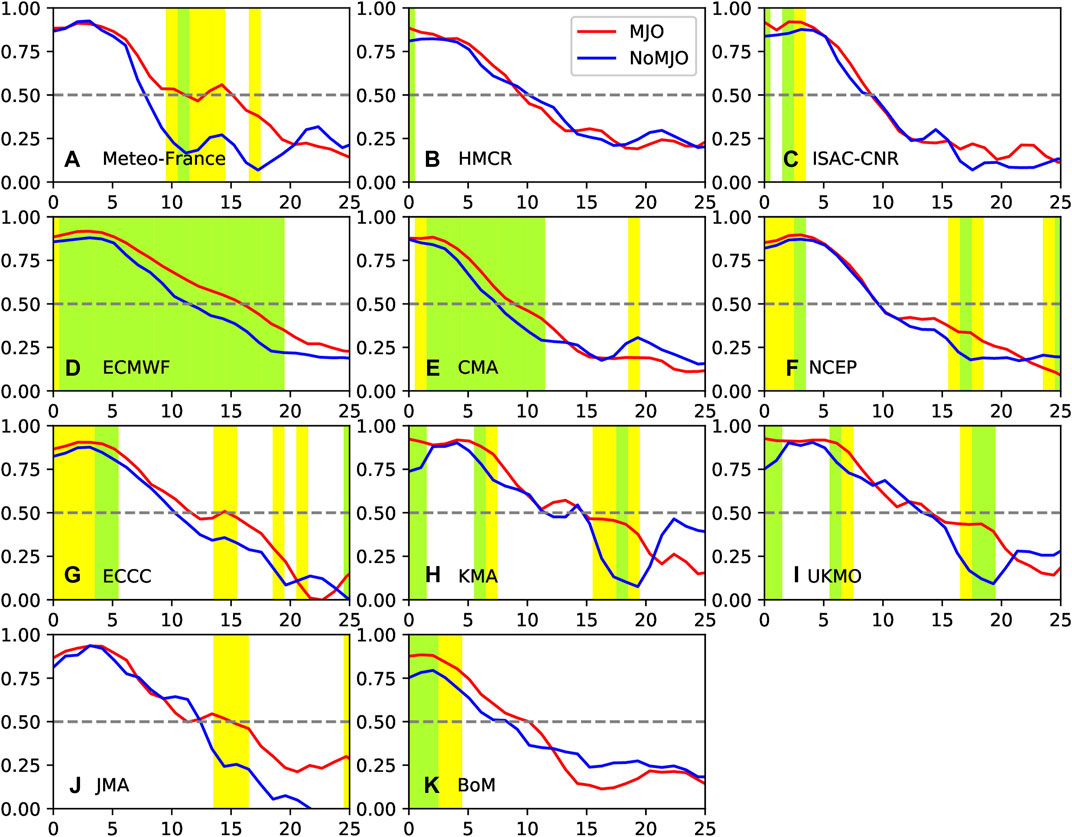
FIGURE 4. Arctic Oscillation (AO) prediction skill of the 11 sub-seasonal to seasonal (S2S) models during the first 25 forecast days when the Arctic Oscillation (AO) is in the positive phase on the initial day. The prediction skill is the correlation between the predicted and observed AO with the seasonal cycle being removed. In each panel, the red (blue) line indicates prediction initialed with (without) an active Madden–Julian Oscillation (MJO), and the legend is presented in panel B. The gray dash line presents the prediction skill of 0.5, above (below) which the skill is (not) useful. The significance of the differences between the prediction skill with and without the MJO (red and blue lines) is presented using shading areas. The green (yellow) shading indicates that the significance is at the 5% (10%) level according to the method introduced in the Significant Test section. Panel (A-K) are for models of Meteo-France, HMCR, ISAC-CNR, ECMWF, CMA, NCEP, ECCC, KMA, UKMO, JMA, and BoM, respectively.
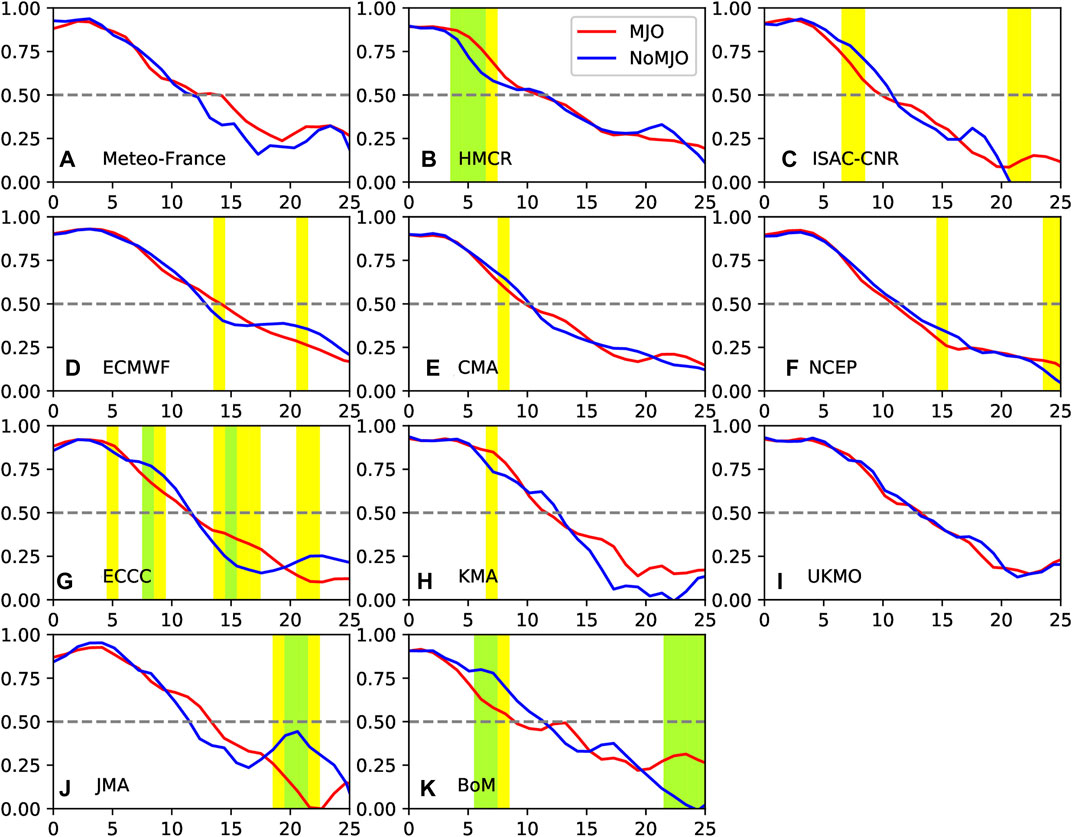
FIGURE 5. Same as Figure 4 but for the Arctic Oscillation (AO) prediction initialed with the negative AO phase, and the legend is shown in panel A. Panel (A-K) are for models of Meteo-France, HMCR, ISAC-CNR, ECMWF, CMA, NCEP, ECCC, KMA, UKMO, JMA, and BoM, respectively.
Figure 4 shows the skill of AO prediction initiated in the AO positive phase. Among the 11 S2S models, the AO prediction skill is generally useful (≥0.5) in the first 15 forecast days, which are much smaller than those of the prediction of the MJO in the tropics (Vitart,2017) and the temperature prediction in mid-latitudes (Zhou et al., 2019). The models of ECMWF (Figure 4D), UKMO (Figure 4I), and JMA (Figure 4J) can still provide the useful AO prediction around 15 forecast days, which is the largest among the 11 models. In most of the 11 models, the prediction skill with an initial MJO is generally larger than that without an initial MJO during the first 25 forecast days. In Meteo-France (Figure 4A), the prediction skill initiated with the MJO is significantly greater than that of the inactive MJO at the 10% (5%) level during 10–14 days and on day 17. In HMCR (Figure 4B), ISAC-CNR (Figure 4C), and BoM (Figure 4K), prediction initiated with the MJO is only significantly greater than those without the MJO during the first several forecast days (≤5 days). In ECMWF (Figure 4D), the prediction is significantly improved during forecast days 1–19 at the 5% level when the forecast is initiated with the MJO. In CMA (Figure 4E), prediction initiated with the MJO is significantly better than that without the MJO at the 5% level during forecast days 3–11 (at the 10% level on day 2). In NCEP (Figure 4F), ECCC (Figure 4G), KMA (Figure 4H), and UKMO (Figure 4I), predictions are generally significantly improved on the first several forecast days and during days 15–20. In JMA, prediction skill with the MJO is only significantly greater than that without the MJO at the 10% level during days 14–16. Moreover, it is also found that prediction skill without the MJO is significantly greater than that with the MJO around day 25 in NCEP and day 21 in ECCC. It is interesting to find that when the AO is in its positive phase with an active MJO event on the initial day in the model, the AO prediction skill in most of the S2S models can be significantly improved, especially in the ECMWF and CMA models. In the observations (Figures 1, 2), it is also found that the AO activity can be enhanced in its positive phase when there is a leading MJO.
For the predictions initiated in the negative AO phase (Figure 5), the effects of a leading MJO on the prediction are not as strong as those in the positive AO phase. In Meteo-France (Figure 5A) and UKMO (Figure 5I), no significant difference is found between the prediction skill with and without the MJO. The prediction skill with the MJO is significantly greater than that without the MJO during forecast days 4–7 in HMCR (Figure 5B), on days 21 and 22 in ISAC-CNR (Figure 5C), on day 14 in ECMWF (Figure 5D), on days 24 and 25 in NCEP (Figure 5F), on day 7 in KMA (Figure 5H), and during days 22–25 in BoM (Figure 5K). The significance is all at the 10% level, except that the significance in HMCR and BOM is at the 5% level during days 4–6 and during days 22–25, respectively. The positive differences between the skill with and without an initial MJO before day 11 are in the opposite of the observations (Figures 1B, 3). The differences between the prediction skill with and without the MJO are significantly negative on forecast day 21 in ECMWF (Figure 5D), on day 8 in CMA (Figure 5E), on day 15 in NCEP (Figure 5F), during days 19–22 in JMA (Figure 5J), and during days 6–8 in BoM (Figure 5K). The significance is generally at the 10% level, except that the differences are significantly at the 5% level on days 6 and 7 in BoM. The results before forecast day 11 in BoM are generally consistent with the observations that the MJO can weaken AO activity when the forecast is initiated in negative AO phase. In ECCC (Figure 5G), the differences of the prediction skill are significantly positive (negative) on days 5 and 14–17 (days 8–9 and 21–22) at the 10% level, except that it is significant at the 5% level on days 7 and 15. So far, when the prediction is initiated in the negative AO phase, less consistence of the effects of the MJO on the AO prediction skill is found among the 11 models. This may be due to the weather and climate in the negative AO phase that are more complicated than those in the positive phase (Gong et al., 2001; Jeong and Ho, 2005; Park et al., 2011).
In general, the MJO can be a significant predictability source for the AO S2S prediction. Moreover, in most of the S2S models, the connections between the MJO activity and AO prediction in the positive AO phase are stronger than those in the negative phase. Thus, improvement of the connections between the MJO and the AO in the negative AO phase could be a challenge for the S2S models.
Arctic Oscillation Prediction Varying in Madden–Julian Oscillation Phases
Correlations Between Madden–Julian Oscillation-Related Arctic Oscillation Activity and Arctic Oscillation Prediction
To further check the relationship between MJO activity and AO prediction skill, the correlations between the MJO-related AO activity in the observations and the AO S2S prediction skill in the models are presented in Table 2. To obtain Table 2, the time-lag AO activities in the observations are the same as those shown in Figure 1 but are separated into circumstances of eight active MJO phases and one inactive MJO phase (phase 0). Furthermore, the AO prediction skill is also divided into the nine phases according to the MJO activity on the initial day. Besides the nine MJO phases, the correlations are conducted during the first 15 lag (forecast) days in the observations (models), and thus the sample size for the correlation in Table 2 is 15 × 9 = 135. The first 15 days are chosen because most of the models have useful prediction skill less than 15 forecast days. As the prediction skill decreases during the first 15 forecast days, and there is a decrease trend. The mean of the prediction in the nine MJO phases is removed on each forecast day before calculating the correlation.

TABLE 2. Correlations between the observed time-lag AO activities after the occurrence of the MJO event for 15 days and the AO S2S prediction skill during the first 15 forecast days in the 11 S2S models. On each forecast day, the AO activity and prediction skill are further separated into eight MJO phases and no MJO (phase 0) according to the MJO activity on the initial day. Therefore, the sample size for each correlation is 15 (days) × 9 (phases) = 135. Moreover, correlations are also separated into circumstances of the AO positive (Pos.) and negative (Neg.) phases on the initial day. As the prediction skill decreases during the first 15 forecast days, the mean of the prediction skill during the nine MJO phases on each forecast day is removed before conducting the correlation. Thus, the decreasing trend is removed in the prediction skill. The correlation coefficients in bold are significant at the 5% level based on the Student’s t-test.
In Table 2, most of the models show the significant correlations between the MJO-related AO activities and the AO S2S prediction. No matter what the AO phase the initial day is in, a significantly positive relationship is found between the MJO-related AO activity and the AO prediction, except for the correlations in Meteo-France, in the positive phase in JMA, and in the negative phase in ISAC-CNR. The correlations are significantly positive at the 5% level. They are above 0.5 in NCEP, around 0.4 in ECCC, ECMWF, and HMCR, and about 0.3 in BoM and CMA. In the KMA and UKMO models, the correlations are 0.22 and 0.27 in the AO positive phase, respectively, which are much smaller than those in the AO negative phase (0.34 and 0.4, respectively). The significant correlations in ISAC-CNR and JMA are generally around 0.2. The maximum correlation 0.63 is in the negative AO phase in NCEP. The significantly positive relationship indicates that when the MJO-related AO activity is strong in the observations, there tends to be a high prediction skill in most of the S2S models. This finding further confirms that the MJO can significantly impact the AO prediction skill in the S2S models.
Observed Arctic Oscillation Activity in Each Madden–Julian Oscillation Phase
Figure 6 presents time-lag composites of the AO activity in the nine MJO phases, including the inactive MJO (phase 0), when the MJO leads the AO for 5, 10, and 15 days. The composites are also divided into circumstances of the AO positive and negative phases on the initial day. Figure 6 is very similar to Figure 1 but separates the AO activities into nine MJO phases. The AO activities in the eight MJO phases are presented by bars with different colors, and AO activities without an initial MJO are shown by the circle with black solid line in Figure 6. The eight MJO phases can represent the locations of MJO convection centers in the tropics. When the MJO is in phases 1–8, MJO convection travels from Africa, across the Indian Ocean and the Pacific, to the western Hemisphere.
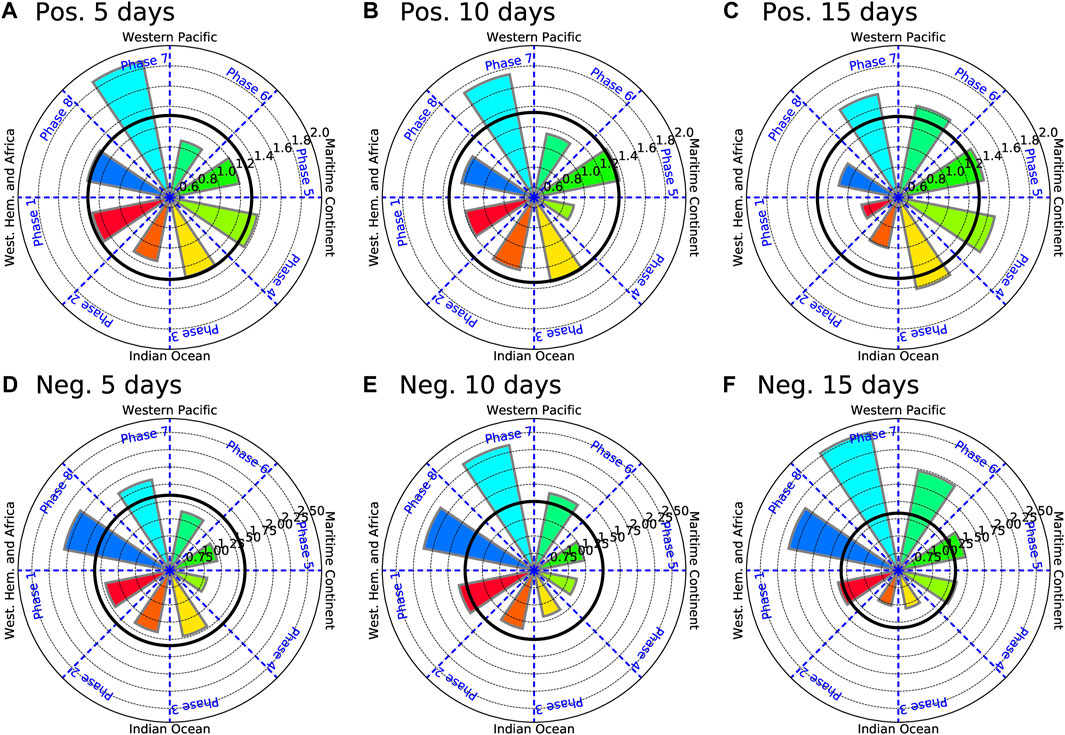
FIGURE 6. Time-lag composites of Arctic Oscillation (AO) amplitudes (bars) in eight phases of the Madden–Julian Oscillation (MJO) leading the AO activity for 5 (left panels A and D), 10 (middle panels B and E), and 15 (right panels C and F) days. The composite of the AO amplitude without the leading MJO is shown by the circle of black solid line in each panel, and the gray dash lines are reference lines for the strength of AO amplitude. The grid space of gray dash lines in the top (bottom) panels is 0.2 (0.25). The blue dash lines separate the eight MJO phases, and the composites of AO amplitude in MJO 1–8 phases also present different colors.
Figures 6A–C present the AO activities when the AO is in its positive phase on the initial day. The composites of AO activities that lagged 5 days are shown in Figure 6A. In MJO phases 2, 5, and 6, the AO activities are smaller than those in MJO phases 1, 3, and 8 and close to those in phase 0. In MJO phases 4 and 7, the AO activities are the strongest amongst all the MJO phases. In Figure 6B, the AO activities lag 10 days to the MJO activity on the initial day. The AO activity is the strongest in phase 7. In phases 3 and 5, the AO activities are the same as those without the MJO. The AO activities in phases 1, 2, 4, 6, and 8 are smaller than those without an MJO. When the AO activities lag 15 days, they are stronger (smaller) in phases 3–7 (1, 2, and 8) than those without an MJO. Figures 6D–F provide the AO activities when the AO is in its negative phase on the initial day. In Figure 6D, the AO activities are stronger (weaker) in phases 7 and 8 (phases 1–6) than those in phase 0. In Figure 6E, the AO activities are stronger (weaker) in phases 1 and 6–8 (phases 2–5) than those without an MJO. When the AO activities lag 15 days (Figure 6F), they are stronger (weaker) during phases 5–8 (2 and 3) than those in the rest of the phases. The activities in phases 0, 1, and 4 are generally the same. Overall, as the initial day is in the MJO phase 7 (phase 2), the AO activity with the positive phase at the initial time is strong (weak). When the AO is in its negative phase on the initial day, the AO activities are strong (weak) in phases 7 and 8 (phases 2 and 3).
Arctic Oscillation Prediction in Each Madden–Julian Oscillation Phase
In order to check the performance of S2S models on AO prediction associated with different MJO phases, Figures 7, 8 show the prediction skill of the AO with the MJO in different phases on the initial day. For the conciseness of this paper, we only present the results of ECMWF, CMA, and NCEP on the forecast days of 5, 10, and 15. The ECMWF model has the longest leading days with useful prediction skill among all the models. The prediction in CMA model is less skillful than most of the models. The NCEP model has the highest correlation between the observed AO activity and the AO prediction (Table 2).
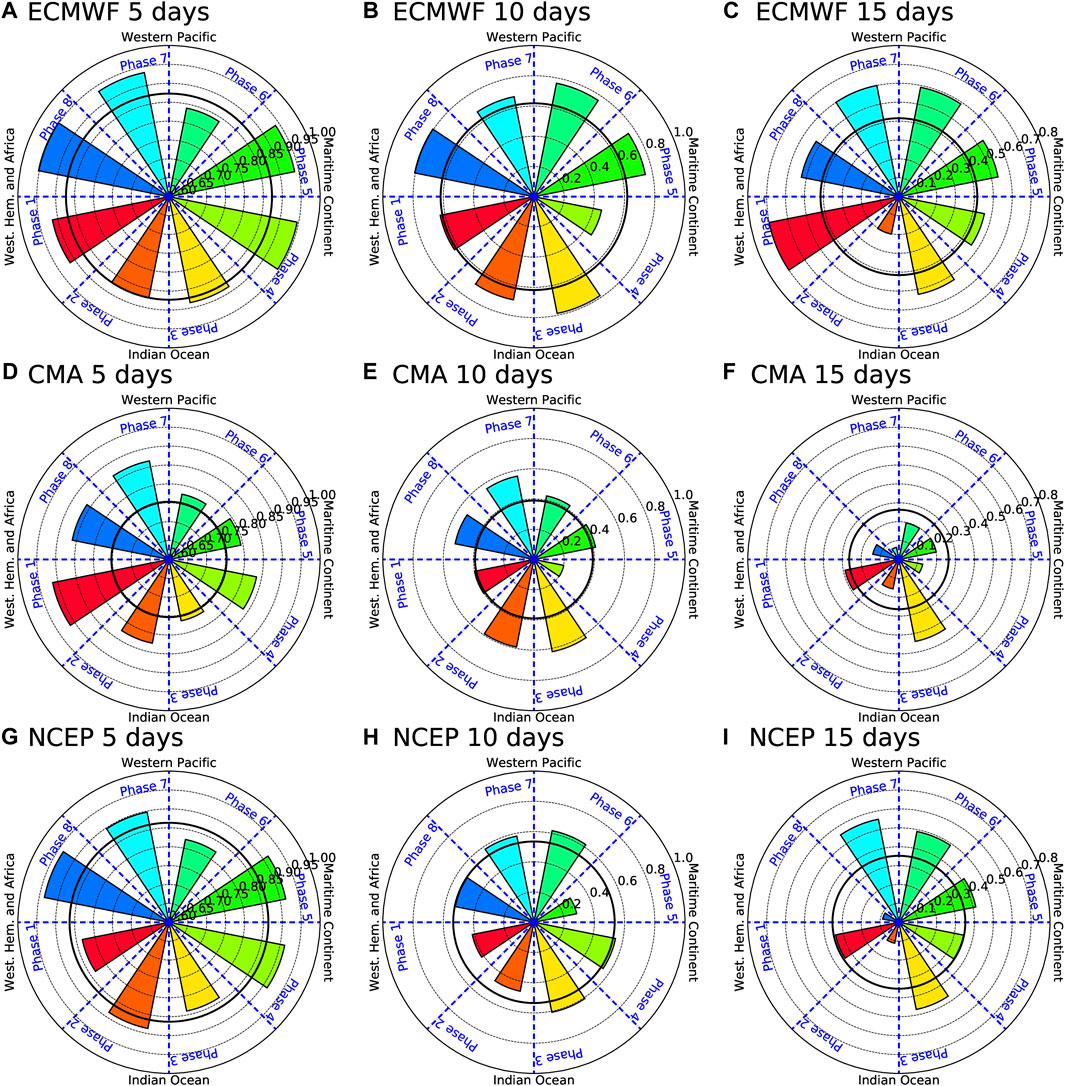
FIGURE 7. Similar to Figure 6 but for the prediction skill with Madden–Julian Oscillation (MJO) in different phases on the forecast days of 5 (left panels A, D, and G), 10 (middle panels B, E, and H), and 15 (right panels C, F, and I) for European Centre for Medium-Range Weather Forecasts (ECMWF; top panels), China Meteorological Administration (CMA; middle panels), and United States National Centers for Environmental Prediction (NCEP; bottom panels) models as the initial day is in the positive Arctic Oscillation (AO) phase. The prediction skill in the eight MJO phases (phase 0) is present by bars with different colors (the circle with black solid line).
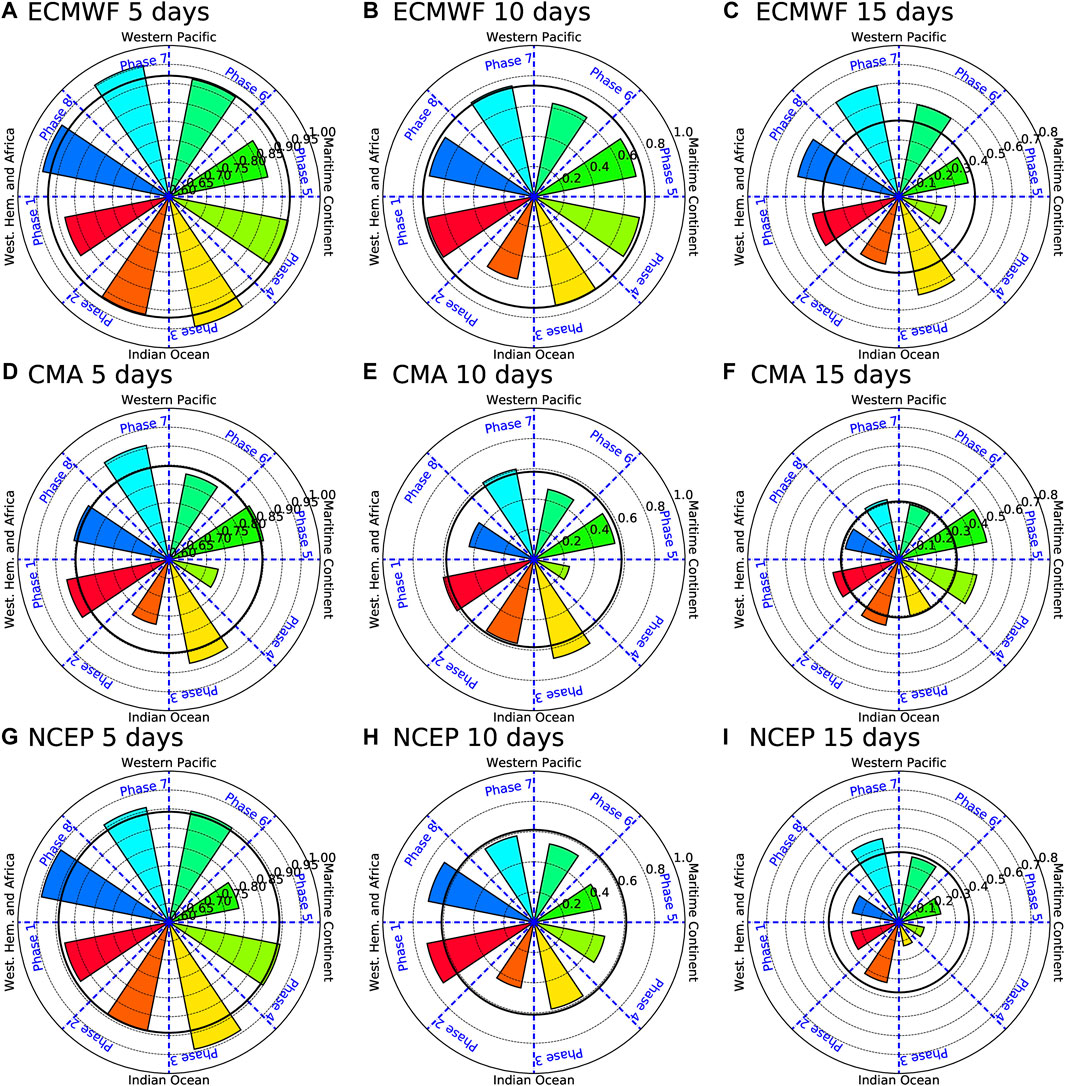
FIGURE 8. Same as Figure 7 but for the initial day in the negative Arctic Oscillation (AO) phase. Left (A, D, and G), middle (B, E, and H), and right (C, F, and I) panels are for the forecast days of 5, 10, and 15 days, respectively.
When the prediction is initiated with the positive AO phase, the prediction skill is presented in Figure 7. In ECMWF, the prediction skill on forecast day 5 is larger in phases 1, 4, 5, 7, and 8 than that in phases 0, 2, and 3 (Figure 7A). The skill in phase 6 is the smallest among all the phases. On day 10 (Figure 7B), the skill is large (small) in phases 2, 3, and 5–7 (phase 4), which is bigger (smaller) than that in phases 0 and 1. On day 15 (Figure 7C), the skill in phases 1–8 is larger than that without the MJO, except that in phase 2. In CMA, the prediction skill on day 5 is larger in phases 1–8 than that in phase 0 (Figure 7D). The skill in phases 1, 7, and 8 (phase 3) is larger (smaller) than that in the rest of the phases. On day 10 (Figure 7E), the prediction skill is larger in phases 2, 3, and 6–8 than that in the rest of the phases, among which the skill in phase 4 is the smallest. On day 15 (Figure 7F), the skill is smaller in phases 1–8 than that without an initial MJO, except that in phases 1 and 3. In Figure 7G on forecast day 5, the prediction skill in NCEP is found large (small) in phases 2, 4, 5, 7, and 8 (phases 1, 3, and 6) and is greater (smaller) than the skill in phase 0. On day 10 (Figure 7H), the skill is bigger in phases 3, 6, and 7 than that in phase 0 but smaller in phases 1, 2, and 5. The skill in phases 4 and 8 is close to that in phase 0. On day 15 (Figure 7I), the skill is large in phases 3 and 5–7, close to the skill without an initial MJO in phases 1 and 4, and small in phases 2 and 8. Generally, the prediction skill is larger in phases 3 and 7 than that without an initial MJO in the three models, except the skill in phase 7 on forecast day 15 of CMA and in phase 3 on forecast day 5 of NCEP. Moreover, the forecast in phase 2 on day 15 has less skill among all the phases in the three models. Those findings from the prediction skill are generally consistent with the MJO-related AO activity in the observations (Figures 6A–C).
When the prediction is initiated in the negative phase of the AO, its skill is provided in Figure 8. In ECMWF, the prediction skill in phases 3, 7, and 8 (phases 1 and 5) is larger (smaller) than that in phase 0 on forecast day 5 (Figure 8A). In phases 2, 4, and 6, the skill is close to that in phase 0. On day 10 (Figure 8B), the prediction skill in phases 1–8 is generally close to that in phase 0, except that the skill in phases 2 and 6 is smaller than that in phase 0. On day 15 (Figure 8C), the skill in phases 1–8 is generally greater than that without an initial MJO, except that in phases 2, 4, and 5. In CMA, compared with the skill in phase 0, it is large in phases 1, 3, and 7, small in phases 2, 4, and 6, and generally the same in phases 5 and 8 on day 5 (Figure 8D). On day 10 (Figure 8E), the skill is slightly larger in phases 1, 3, and 7 than that in phase 0 but is smaller in phases 4–6 and 8. The skill in phase 2 is alike to that in phase 0. On day 15 (Figure 8F), the skill is large in phases 1, 2, 4, and 5. In the remaining phases, the skill is generally close to that in phase 0 but slightly larger in phase 7. In NCEP on day 5, the skill is larger in phases 3, 7, and 8 than that in phase 0. In the rest of the phases, the skill is generally close to that in phase 0, except that the skill is smaller in phase 5. On day 10 (Figure 8H), the skill is generally smaller than that without an initial MJO, except that in phases 1 and 8. On day 15 (Figure 8I), only the skill in phase 7 is greater than that in phase 0, and the skill in the rest of the phases is smaller. Generally, the skill is higher in phase 7 than the skill in phase 0 in the three models, except on day 10 in NCEP. In phases 2, 4, and 5, the skill is generally smaller than or close to that in phase 0 in the three models, except in phases 2, 4, and 5 on day 15 in CMA. These findings further present that AO prediction skill is related to the MJO activity, which also agrees with that in the observations (Figures 6D–F).
In general, both the correlation coefficients in Table 2 and the bar charts of AO activity and prediction skill in different MJO phases show that the AO prediction skill in the S2S models is closely associated with the MJO activity at the initial time. Due to the complicated interactions between the MJO in the tropics and the AO in the Arctic, the S2S models may only catch part of the relationship between the MJO and the AO. Thus, the prediction skill in some phases does not agree with MJO-related AO activity. However, it is still found that when the MJO activity enlarges the AO strength, it tends to lead to a large prediction skill of the AO in the S2S models.
Summary and Discussion
As a substantial condition for the S2S climate prediction in the numerical model, the initial state of the atmosphere carries lots of signals of use. Among all those signals, how do they interact with predictands that we care, and which one can be a predictability source for S2S prediction? Answering those two questions can help us to improve the understanding of S2S prediction. Following this concept, the impacts of MJO on the AO prediction in the S2S models are explored in the present study.
The MJO and the AO are the major sub-seasonal and annular modes in the tropics and the Northern Hemisphere, respectively. In the observations, significant time-lag connections are found between the MJO and AO activities. Using the MJO and AO indices, absolute value of AO amplitude is defined as AO activity for the time-lag composite in active and inactive MJO phases. In the composite, when there is an active MJO in the tropics and the AO is in its positive phase, AO activities lagging 4–6 and 14–25 days are enhanced compared to those without an MJO. On the contrary, when AO is in its negative phase, compared with the AO activity without an MJO, the active MJO leads the AO activity of decrease for 0–8 days but the AO activity of increase for at least 11 days. In the composite of 1,000-hPa GH, the AO positive (negative) pattern is enhanced (weakened) after an active MJO for 0, 5, 10, and 15 days. An inactive MJO is in favor of enhancement of the AO negative pattern but not the AO positive pattern.
The connections between the MJO and AO activities also have some reflection in the S2S models. The AO prediction skill is compared between the forecasts with and without an initial active MJO. When the initial condition of the forecast is in the AO positive phase, the prediction with an initial MJO has more useful skill than that without the MJO in most of the models, especially in ECMWF and CMA. When there is a negative AO on the initial day, no consistency is found for the impacts of the MJO on prediction skill among the 11 S2S models. Most of the models show that the prediction skill with the MJO is weaker than that without the MJO during the first 8 forecast days, but it is only significant in ISAC-CNR, CMA, ECCC, and BoM. The significant level is generally at the 10% level, except that is at the 5% level in BoM. After day 11 of the forecast, the prediction skill that can be significantly enhanced by the initial MJO is only found in ISAC-CNR, ECMWF, ECCC, and BoM. The significance is generally at the 10% level in most of the models, except that is at the 5% level in the ECCC and BoM. When the prediction is in the negative AO phase, the differences of the prediction skill between with and without an initial MJO are generally not consistent with the differences of the AO activities in the observations. This may be because that the weather and climate in the negative phase of AO are more complicated than those in the positive phase in the Northern Hemisphere, which provides much challenge for the S2S operational models.
Besides the prediction skill associated with an initial active and inactive MJO, we further explore the skill varying in the eight active MJO phases, as well as the inactive MJO phase. A significant relationship is found between the observed MJO-related AO activities and the AO prediction skill in different MJO phases in most of the S2S models. No matter what phase the AO is in, all the correlation coefficients are significantly positive at the 5% level, except in Meteo-France, in the positive phase of JMA, and in the negative phase of ISAC-CNR. The correlation in the NCEP model is the largest among all the models. The significantly positive relationship indicates that when the MJO causes a strong AO activity in the observations, it corresponds to a large AO prediction skill in the S2S model, and vice versa. This result further proves that the MJO can supply useful predictability for the AO prediction in the S2S models.
Taking the model results in ECMWF, CMA, and NCEP as examples, we can further examine that the detail of the prediction skill varied with the initial MJO phases. When the initial state with a positive AO is in MJO phases 3 and 7, the prediction skill tends to be larger than that without the MJO in the three models. In MJO phase 2, the prediction still is the smallest in the three models. When the prediction is initiated with a negative AO and in MJO phase 7, the prediction skill is larger than that without the MJO in the three models. Although there are several exceptions, the three models still present some common features of the connection between initial MJO activity and the AO prediction. Previous studies have found that the MJO can affect the AO through teleconnection and processes in the stratosphere (Garfinkel and Schwartz, 2017; Schwartz and Garfinkel, 2017), and this could be the physical explanation for the connection between the MJO activity and the AO prediction. However, the processes are complicated, and thus the models cannot catch them all. Generally, this study suggests that the MJO activity in the tropics is an important predictability source of the AO prediction, and the prediction skill of the AO depends on both initial phases of the MJO and the AO.
Data Availability Statement
The original contributions presented in the study are included in the article/Supplementary Material. Further inquiries can be directed to the corresponding author.
Author Contributions
YZ provided the original idea and wrote the article. YW conducted most of the calculations and drew some of the figures.
Funding
We thank the support from the National Natural Science Foundation of China (Grant Nos. 41930969 and 42175030).
Conflict of Interest
The authors declare that the research was conducted in the absence of any commercial or financial relationships that could be construed as a potential conflict of interest.
Publisher’s Note
All claims expressed in this article are solely those of the authors and do not necessarily represent those of their affiliated organizations, or those of the publisher, the editors, and the reviewers. Any product that may be evaluated in this article, or claim that may be made by its manufacturer, is not guaranteed or endorsed by the publisher.
Acknowledgments
The MJO index is obtained from BoM at the website of http://www.bom.gov.au/climate/mjo/. The AO index is from the ftp of NWC/CPC at ftp://ftp.cpc.ncep.noaa.gov/cwlinks/. The NCEP RII reanalysis is obtained from https://psl.noaa.gov/. The S2S reforecast can be downloaded from the port suggested by the website of S2S project at http://www.s2sprediction.net.
References
Alvarez, M. S., Vera, C. S., Kiladis, G. N., and Liebmann, B. (2016). Influence of the Madden Julian Oscillation on Precipitation and Surface Air Temperature in South America. Clim. Dyn. 46, 245–262. doi:10.1007/s00382-015-2581-6
Cassou, C. (2008). Intraseasonal Interaction between the Madden-Julian Oscillation and the North Atlantic Oscillation. Nature 455, 523–527. doi:10.1038/nature07286
Ebisuzaki, W. (1997). A Method to Estimate the Statistical Significance of a Correlation when the Data Are Serially Correlated. J. Clim. 10, 2147–2153. doi:10.1175/1520-0442(1997)010<2147:amtets>2.0.co;2
Ferranti, L., Palmer, T. N., Molteni, F., and Klinker, E. (1990). Tropical-Extratropical Interaction Associated with the 30-60 Day Oscillation and its Impact on Medium and Extended Range Prediction. J. Atmos. Sci. 47, 2177–2199. doi:10.1175/1520-0469(1990)047<2177:teiawt>2.0.co;2
Flatau, M., and Kim, Y.-J. (2013). Interaction between the MJO and Polar Circulations. J. Clim. 26, 3562–3574. doi:10.1175/jcli-d-11-00508.1
Garfinkel, C. I., and Schwartz, C. (2017). MJO-related Tropical Convection Anomalies Lead to More Accurate Stratospheric Vortex Variability in Subseasonal Forecast Models. Geophys. Res. Lett. 44, 10,054–10062. doi:10.1002/2017GL074470–
Garfinkel, C. I., Schwartz, C., White, I. P., and Rao, J. (2020). Predictability of the Early winter Arctic Oscillation from Autumn Eurasian Snowcover in Subseasonal Forecast Models. Clim. Dyn. 55, 961–974. doi:10.1007/s00382-020-05305-3
Gong, D.-Y., Wang, S.-W., and Zhu, J.-H. (2001). East Asian winter Monsoon and Arctic Oscillation. Geophys. Res. Lett. 28 (10), 2073–2076. doi:10.1029/2000gl012311
Gong, D., and Ho, C. (2003). Arctic Oscillation Signals in the East Asian Summer Monsoon. J. Geophys. Res. Atmos. 108, 171–181. doi:10.1029/2002jd002193
He, S., Gao, Y., Li, F., Wang, H., and He, Y. (2017). Impact of Arctic Oscillation on the East Asian Climate: A Review. Earth-Science Rev. 164, 48–62. doi:10.1016/j.earscirev.2016.10.014
Jeong, J., and Ho, C. (2005). Changes in Occurrence of Cold Surges over East Asia in Association with Arctic Oscillation. Geophys. Res. Lett. 32, L14704. doi:10.1029/2005gl023024
Jones, C., and Dudhia, J. (2017). Potential Predictability during a Madden-Julian Oscillation Event. J. Clim. 30, 5345–5360. doi:10.1175/jcli-d-16-0634.1
Jones, C., Waliser, D. E., Lau, K. M., and Stern, W. (2004). The Madden-Julian Oscillation and its Impact on Northern Hemisphere Weather Predictability. Mon. Wea. Rev. 132, 1462–1471. doi:10.1175/1520-0493(2004)132<1462:tmoaii>2.0.co;2
Kalnay, E., Kanamitsu, M., Kistler, R., Collins, W., Deaven, D., and Gandin, L. (1996). The NCEP/NCAR 40-year Reanalysis Project. Bull. Amer. Meteorol. Soc. 77, 437–470. doi:10.1175/1520-0477(1996)077<0437:TNYRP>2.0.CO;2:
Kanamitsu, M., Ebisuzaki, W., Woollen, J., Yang, S.-K., Hnilo, J. J., Fiorino, M., et al. (2002). NCEP-DOE AMIP-II Reanalysis (R-2). Bull. Amer. Meteorol. Soc. 83, 1631–1644. doi:10.1175/bams-83-11-1631
L’Heureux, M. L., and Higgins, R. W. (2008). Boreal Winter Links between the Madden-Julian Oscillation and the Arctic Oscillation. J. Clim. 21 (12), 3040–3050. doi:10.1175/2007jcli1955.1
Lin, H., Brunet, G., and Derome, J. (2009). An Observed Connection between the North Atlantic Oscillation and the Madden-Julian Oscillation. Mon. Wea. Rev. 22, 364–380. doi:10.1175/2008jcli2515.1
Liu, P., Tang, M., Yu, H., and Zhang, Y. (2020). Influence of Arctic Oscillation on Frequency of Wintertime Fog Days in Eastern China. Atmosphere 11, 162. doi:10.3390/atmos11020162
Madden, R. A., and Julian, P. R. (1972). Description of Global-Scale Circulation Cells in the Tropics with a 40-50 Day Period. J. Atmos. Sci. 29, 1109–1123. doi:10.1175/1520-0469(1972)029<1109:dogscc>2.0.co;2
Madden, R. A., and Julian, P. R. (1971). Detection of a 40-50 Day Oscillation in the Zonal Wind in the Tropical Pacific. J. Atmos. Sci. 28, 702–708. doi:10.1175/1520-0469(1971)028<0702:doadoi>2.0.co;2
Madden, R. A., and Julian, P. R. (1994). Observations of the 40-50-Day Tropical Oscillation-A Review. Mon. Wea. Rev. 122, 814–837. doi:10.1175/1520-0493(1994)122<0814:ootdto>2.0.co;2
Matthews, A. J., Hoskins, B. J., and Masutani, M. (2004). The Global Response to Tropical Heating in the Madden-Julian Oscillation during the Northern winter. Q. J. R. Meteorol. Soc. 130, 1991–2011. doi:10.1256/qj.02.123
Mayta, V. C., Silva, N. P., Ambrizzi, T., Dias, P. L. S., and Espinoza, J. C. (2020). Assessing the Skill of All-Season Diverse Madden-Julian Oscillation Indices for the Intraseasonal Amazon Precipitation. Clim. Dyn. 54, 3729–3749. doi:10.1007/s00382-020-05202-9
Minami, A., and Takaya, Y. (2020). Enhanced Northern Hemisphere Correlation Skill of Subseasonal Predictions in the strong Negative Phase of the Arctic Oscillation. J. Geophys. Res. Atmos. 125, e2019JD031268. doi:10.1029/2019jd031268
Moon, J.-Y., Wang, B., and Ha, K.-J. (2011). ENSO Regulation of MJO Teleconnection. Clim. Dyn. 37, 1133–1149. doi:10.1007/s00382-010-0902-3
Park, T.-W., Ho, C.-H., and Yang, S. (2011). Relationship between the Arctic Oscillation and Cold Surges over East Asia. J. Clim. 24, 68–83. doi:10.1175/2010jcli3529.1
Reichler, T., and Roads, J. O. (2005). Long-Range Predictability in the Tropics. Part II: 30-60-Day Variability. J. Clim. 18 (5), 634–650. doi:10.1175/jcli-3295.1
Schwartz, C., and Garfinkel, C. I. (2017). Relative Roles of the MJO and Stratospheric Variability in North Atlantic and European winter Climate. J. Geophys. Res. Atmos. 122, 4184–4201. doi:10.1002/2016JD025829
Singh, M., and Bhatla, R. (2019). Modulation of Active-Break Spell of Indian Summer Monsoon by Madden Julian Oscillation. J. Earth Syst. Sci. 128, 70. doi:10.1007/s12040-019-1091-z
Song, L., and Wu, R. (2019a). Combined Effects of the MJO and the Arctic Oscillation on the Intraseasonal Eastern China winter Temperature Variations. J. Clim. 32, 2295–2311. doi:10.1175/jcli-d-18-0625.1
Song, L., and Wu, R. (2019b). Different Cooperation of the Arctic Oscillation and the Madden‐Julian Oscillation in the East Asian Cold Events during Early and Late Winter. J. Geophys. Res. Atmos. 124, 4913–4931. doi:10.1029/2019jd030388
Sossa, A., Liebmann, B., Bladé, I., Allured, D., Hendon, H. H., Peterson, P., et al. (2017). Statistical Connection between the Madden-Julian Oscillation and Large Daily Precipitation Events in West Africa. J. Clim. 30, 1999–2010. doi:10.1175/jcli-d-16-0144.1
Specq, D., and Batté, L. (2020). Improving Subseasonal Precipitation Forecasts through a Statistical-Dynamical Approach : Application to the Southwest Tropical Pacific. Clim. Dyn. 55, 1913–1927. doi:10.1007/s00382-020-05355-7
Thompson, D. W. J., and Wallace, J. M. (1998). The Arctic Oscillation Signature in the Wintertime Geopotential Height and Temperature fields. Geophys. Res. Lett. 25, 1297–1300. doi:10.1029/98gl00950
Vincent, C. L., and Lane, T. P. (2018). Mesoscale Variation in Diabatic Heating Around Sumatra, and its Modulation with the Madden-Julian Oscillation. Mon. Wea. Rev. 146, 2599–2614. doi:10.1175/mwr-d-17-0392.1
Vitart, F. (2017). Madden-Julian Oscillation Prediction and Teleconnections in the S2S Database. Q. J. R. Meteorol. Soc. 143, 2210–2220. doi:10.1002/qj.3079
Wallace, J. M., and Thompson, D. W. J. (2002). The pacific center of Action of the Northern Hemisphere Annular Mode: Real or Artifact? J. Clim. 15, 1987–1991. doi:10.1175/1520-0442(2002)015<1987:tpcoao>2.0.co;2
Wheeler, M. C., and Hendon, H. H. (2004). An All-Season Real-Time Multivariate MJO index: Development of an index for Monitoring and Prediction. Mon. Weather Rev. 132, 1917–1932. doi:10.1175/1520-0493(2004)132<1917:aarmmi>2.0.co;2
Wei, K., Ouyang, C., Duan, H., Li, Y., Chen, M., Ma, J., et al. (2020). Reflections on the Catastrophic 2020 Yangtze River Basin Flooding in Southern China. Innov 2 (1), 100038.
Wu, L., and Takahashi, M. (2018). Contributions of Tropical Waves to Tropical Cyclone Genesis Over the Western North Pacific. Clim. Dyn. 50, 4635–4649. doi:10.1007/s00382-00017-03895-00383
Yang, J., Gong, D., Wang, W., Hu, M., and Mao, R. (2012). Extreme Drought Event of 2009/2010 over Southwestern China. Meteorol. Atmos. Phys. 115, 173–184. doi:10.1007/s00703-011-0172-6
Yang, X., and Huang, P. (2021). Restored Relationship Between ENSO and Indian Summer Monsoon Rainfall Around 1999/2000. Innov 2 (2), 100102.
Yoneyama, K., Zhang, C., and Long, C. N. (2013). Tracking Pulses of the Madden-Julian Oscillation. Bull. Amer. Meteorol. Soc. 94 (12), 1871–1891. doi:10.1175/bams-d-12-00157.1
Yoo, C., Feldstein, S., and Lee, S. (2012). The Impact of the Madden-Julian Oscillation Trend on the Arctic Amplification of Surface Air Temperature During the 1979–2008 Boreal Winter. Geoophys. Res. Lett. 38, L24804. doi:10.1029/2011GL049881
Zhang, C., Gottschalck, J., Maloney, E. D., Moncrieff, M. W., Vitart, F., Waliser, D. E., et al. (2013). Cracking the MJO Nut. Geophys. Res. Lett. 40, 1223–1230. doi:10.1002/grl.50244
Zhou, S., and Miller, A. J. (2005). The Interaction of the Madden-Julian Oscillation and the Arctic Oscillation. J. Clim. 18, 143–159. doi:10.1175/jcli3251.1
Zhou, Y., Lu, Y., Yang, B., Jiang, J., Huang, A., Zhao, Y., et al. (2016). On the Relationship between the Madden-Julian Oscillation and 2 M Air Temperature over central Asia in Boreal winter. J. Geophys. Res. Atmos. 121, 13,250–13,272. doi:10.1002/2016JD025651
Zhou, Y., Thompson, K. R., and Lu, Y. (2011). Mapping the Relationship between Northern Hemisphere Winter Surface Air Temperature and the Madden-Julian Oscillation. Mon. Wea. Rev. 139, 2439–2454. doi:10.1175/2011MWR3587.1
Keywords: sub-seasonal to seasonal (S2S), Madden–Julian oscillation (MJO), Arctic oscillation (AO), prediction, initial time
Citation: Zhou Y and Wang Y (2021) Influence of the Madden–Julian Oscillation on the Arctic Oscillation Prediction in S2S Operational Models. Front. Earth Sci. 9:787680. doi: 10.3389/feart.2021.787680
Received: 01 October 2021; Accepted: 22 October 2021;
Published: 17 November 2021.
Edited by:
Gen Li, Hohai University, ChinaReviewed by:
Liang Wu, Institute of Atmospheric Physics (CAS), ChinaXianyu Yang, Chengdu University of Information Technology, China
Copyright © 2021 Zhou and Wang. This is an open-access article distributed under the terms of the Creative Commons Attribution License (CC BY). The use, distribution or reproduction in other forums is permitted, provided the original author(s) and the copyright owner(s) are credited and that the original publication in this journal is cited, in accordance with accepted academic practice. No use, distribution or reproduction is permitted which does not comply with these terms.
*Correspondence: Yang Zhou, eWFuZ3pob3VAbnVpc3QuZWR1LmNu