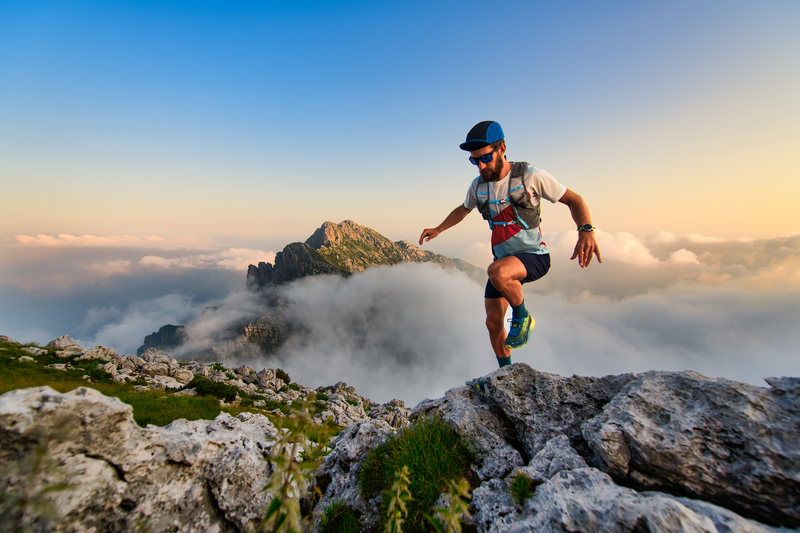
94% of researchers rate our articles as excellent or good
Learn more about the work of our research integrity team to safeguard the quality of each article we publish.
Find out more
REVIEW article
Front. Earth Sci. , 09 February 2022
Sec. Interdisciplinary Climate Studies
Volume 9 - 2021 | https://doi.org/10.3389/feart.2021.758361
This article is part of the Research Topic Arctic Amplification: Feedback Process Interactions and Contributions View all 10 articles
Arctic amplification (AA) is a coupled atmosphere-sea ice-ocean process. This understanding has evolved from the early concept of AA, as a consequence of snow-ice line progressions, through more than a century of research that has clarified the relevant processes and driving mechanisms of AA. The predictions made by early modeling studies, namely the fall/winter maximum, bottom-heavy structure, the prominence of surface albedo feedback, and the importance of stable stratification have withstood the scrutiny of multi-decadal observations and more complex models. Yet, the uncertainty in Arctic climate projections is larger than in any other region of the planet, making the assessment of high-impact, near-term regional changes difficult or impossible. Reducing this large spread in Arctic climate projections requires a quantitative process understanding. This manuscript aims to build such an understanding by synthesizing current knowledge of AA and to produce a set of recommendations to guide future research. It briefly reviews the history of AA science, summarizes observed Arctic changes, discusses modeling approaches and feedback diagnostics, and assesses the current understanding of the most relevant feedbacks to AA. These sections culminate in a conceptual model of the fundamental physical mechanisms causing AA and a collection of recommendations to accelerate progress towards reduced uncertainty in Arctic climate projections. Our conceptual model highlights the need to account for local feedback and remote process interactions within the context of the annual cycle to constrain projected AA. We recommend raising the priority of Arctic climate sensitivity research, improving the accuracy of Arctic surface energy budget observations, rethinking climate feedback definitions, coordinating new model experiments and intercomparisons, and further investigating the role of episodic variability in AA.
Anthropogenic carbon dioxide (CO2) emissions and other greenhouse gases are changing Earth’s climate. Global mean surface temperature has risen by ≥ 1.0°C relative to the pre-industrial period, making this the warmest period in the history of modern civilization (Wuebbles et al., 2017). As the impacts of warming cascade through the physical climate and natural systems, society grapples with decisions on the countermeasures needed to offset the increased vulnerability in the systems that underpin modern society: food, energy, water, health, security, and economy. Global temperature targets (e.g., Paris Climate Accord) serve as the basis to gauge the required aggressiveness of countermeasures. Global targets, however, fail to consider the uncertainty and high impact of dramatic regional changes, such as in the Arctic where consequential ice sheet melt and untenable global sea level rise cannot be ruled out at 1.5°C of global warming (IPCC 2018; Meredith et al., 2019; IPCC 2021). Global temperature targets leave substantial climate risks unconsidered; using regional indicators as policy targets helps account for the uneven spatial distribution of climate change impacts and risks.
Climate change is spread unevenly across the globe. The Arctic surface has warmed more than twice as fast as the global average surface temperature (Figure 1; Lenssen et al., 2019), a phenomenon known as Arctic Amplification (AA). AA is part of the broader polar amplification phenomenon that also applies to the Antarctic. However, amplified Antarctic warming is expected to be weaker and delayed because of the Antarctic continent surface height, smaller albedo and lapse rate feedbacks, and Southern Ocean heat uptake (Salzmann 2017; Hahn et al., 2021). Rapid Arctic surface warming is driving changes in several physical climate characteristics (e.g., sea ice and snow cover) and impacting ecosystems and vegetation distribution (Taylor et al., 2017). The use of climate change indicators from regions with the largest expected changes (e.g. Arctic surface temperature change and sea ice extent and thickness) ensures that high-impact regional climate change outcomes are considered in climate risk assessment.
FIGURE 1. Arctic and zonal mean linear surface temperature trends since 1960. (A) The spatial pattern of the surface temperature trend at 2° × 2° resolution and (B) the zonal mean surface temperature trend (K decade−1) assessed by applying a ordinary least squares fit linear regression to the GISTEMP time series (Lenssen et al., 2019; GISTEMP Team 2021).
Accurate long-term observations and trustworthy climate projections are needed to effectively inform regional targets; however, the harsh and complex Arctic environment makes the necessary observations and climate projections challenging to obtain, resulting in substantial uncertainty. A meaningful adoption of Arctic climate indicators as policy targets requires an improved process understanding to reduce uncertainty in AA projections—the topic of this review.
Research over the last 50 years has identified the fundamental characteristics of AA and advanced our understanding. It is widely accepted that AA manifests as a surface-based warming profile (Manabe and Wetherald 1975; hereafter MW75); it is strongest in fall and winter and absent in summer (Manabe and Stouffer 1980; hereafter MS80); it is strongest in regions of sea ice retreat (Washington and Meehl 1984) and that the seasonal energy transfer from summer to fall via ocean heat storage plays a critical role in its seasonality and magnitude (MS80; Washington and Meehl 1986). Moreover, the melting of sea ice and snow represents a fundamental feedback mechanism (e.g., Arrhenius 1896; Budyko 1966).
As our knowledge has deepened, additional considerations have been identified that make it harder to reduce Arctic climate projection uncertainty. Natural variability complicates our ability to quantify the forced Arctic climate change signal and distinguish the processes driving observed AA. While our understanding of interannual variability has advanced, it remains incomplete (e.g., Ding et al., 2017; Wu et al., 2021). Natural variability also represents an irreducible uncertainty in decadal and multi-decadal predictions (Kay et al., 2011; Swart et al., 2015; Swart 2017). In addition, the quantitative assessment of specific process contributions to AA is affected by the metric used to define AA and the feedback diagnostic approach applied (Hind et al., 2016).
Important advances in AA science have occurred in the last decade. The aim of this manuscript is to synthesize this knowledge and guide future research. Section 2 provides a brief history of AA science, highlighting the most pertinent results and contributing factors. Section 3 provides an overall context of the observed Arctic changes over the last several decades. Section 4 provides a discussion of the modeling approaches and feedback diagnostic techniques. Section 5 describes our current understanding of the processes driving AA. Section 6 provides a conceptual model of the key physical mechanisms. Lastly, Section 7 proposes a collection of recommendations to accelerate progress in AA science and reduce uncertainty in Arctic climate projections.
The expectation that the polar regions are more sensitive to climate forcing has been around since Arrhenius (1896) wrote on the ebb and flow of glacial periods in a seminal paper on the impact of CO2 concentrations on temperature. However, the phrase “amplified polar warming” or “polar amplification” did not appear until nearly a century later (Broecker 1975; Schneider 1975). The explanation for polar amplification has evolved from the earliest idea as a consequence of the progression of the snow-ice line (e.g., Arrhenius 1896) to modern ideas of a coupled atmosphere-sea ice-ocean process (e.g., MS80). While impossible to definitively say, it seems likely that the origin of polar amplification within the context of ice ages favored hypotheses pertaining to ice and snow. Computational expediency could also have played a role, as the surface albedo feedback is easily manipulated within energy balance models (EBMs). Be it by intuition or luck, early scientists correctly identified the leading role of the surface albedo feedback. Despite this early success, large gaps remain in our understanding of the Arctic climate system that preclude more accurate predictions. In constructing a roadmap for improving Arctic climate projections, we consider the historical evolution of polar amplification science.
Early studies employed EBMs—models representing the relationship between Earth’s surface temperature and the top-of-atmosphere (TOA) energy budget—containing many shortcomings and yet captured the essence of polar amplification. Budyko (1966) and Rakipova (1966) demonstrated the fundamental role of surface albedo and the latitudinal position of the snow-ice line in determining polar surface temperature sensitivity to climate forcing. An impressive accomplishment considering that EBMs were informed by little snow and sea ice data and contained invalid assumptions. The most consequential assumption was the exclusion of vertical and horizontal heat transports.
The influence of vertical and horizontal heat transports on polar climate was considered in EBMs later in the 1960s. Manabe and Wetherald (1967) found that the damping of vertical heat transport by strong stability at high latitudes caused a surface albedo perturbation to have a larger effect on near-surface atmospheric temperature than at higher altitudes. Budyko (1969) and Sellers (1969) represented horizontal poleward heat transport in zonally-averaged EBMs as horizontal diffusion proportional to the meridional temperature gradient. Sellers (1969) concluded that the specific representation of poleward heat transport had the potential to offset polar amplification. This research illustrated the substantial sensitivity of the polar climate to poleward heat transport and the need to fully resolve the large-scale atmospheric circulation.
With this knowledge in hand, MW75 employed a GCM to resolve atmospheric eddies and cemented polar amplification as a prominent feature of the global climate response to increased CO2. MW75 established the surface-based vertical structure of polar warming, confirmed in modern studies (e.g., Graversen et al., 2008; Serreze et al., 2009), the role of strong atmospheric stability in confining warming near the surface (e.g., Bintanja et al., 2011), and the compensation between increased latent heat (LH) and decreased poleward sensible heat (SH) transport (e.g., Hwang et al., 2011). While including many simplifications (e.g., idealized geography, fixed clouds, temperature-dependent sea ice and snow albedo, and annual mean insolation), much of our current understanding of polar amplification can be traced to the Nobel prize-winning work in MW75.
MS80 extended MW75 by incorporating a mixed-layer ocean and the annual cycle of insolation revealing that polar amplification is strongest in fall and winter and non-existent in summer. The seasonality of polar amplification is partly attributed to the seasonal energy transfer from summer to fall by the ocean (MS80); an explanation also supported by later studies (Washington and Meehl 1984; Wilson and Mitchell 1987). While adding important ocean physics to resolve the annual cycle, MW75 and MS80 did not consider oceanic poleward heat transport.
The eventual inclusion of poleward heat transport by ocean currents revealed a relationship between high latitude control climate and global climate sensitivity. Spelman and Manabe (1984) presented fully-coupled atmosphere-ocean simulations capturing the observed climate state with some realism. The inclusion of poleward ocean heat transport yielded warmer high latitude surface temperatures, a poleward shift of the snow and sea ice margin, a weakened albedo feedback, and a reduced climate sensitivity. The influence of control climate surface temperature and sea ice extent on high latitude climate sensitivity was recognized in other studies in relation to the surface albedo parameterization (Budyko 1969; Washington and Meehl 1986) and recently shown to influence CMIP5 inter-model spread (Hu et al., 2017). Rind et al. (1995) illustrated a dependence of simulated sea ice decline on sea ice thickness. Control climate-climate sensitivity relationships are attractive because of the potential ability to constrain model predictions; however, as noted by Washington and Meehl (1986), control climate-climate sensitivity relationships may only be valid when considering the same model.
Adding more climate models to the fold revealed the importance of interactions between the ocean and sea ice to the polar climate response. Washington and Meehl (1984; 1986; 1989) performed model simulations with increasingly complex representations of the ocean (swamp, slab, and a coupled ocean circulation model) finding a smaller climate sensitivity and less polar amplification than MW75 and MS80. These differences were attributed to different sea ice albedo-temperature relationships (Washington and Meehl 1984). Additionally, MW75 allowed melt pond formation to change sea ice albedo whereas Washington and Meehl (1984) did not. Washington and Meehl (1989) showed that the regional sea ice distribution was sensitive to the representation of the ocean circulation due to changes in poleward ocean heat transport and deep ocean convection. Further, the way that ocean heat is applied to sea ice (e.g., to the bottom or to the bottom and laterally) also strongly influences sea ice melt (Hansen et al., 1984). These early model intercomparisons demonstrated their value for identifying key uncertainties.
Extracting maximum value from model comparisons requires diagnostic techniques that consistently quantify the causes of model differences (Coakley 1977; Ramanathan 1977; Hansen et al., 1984; Washington and Meehl 1986; Dickinson et al., 1987; Wetherald and Manabe 1988; Section 4). Many of these studies focus on the surface albedo feedback, diagnosing it using slightly different methods, and finding large inter-model differences. However, the inter-model differences in the surface albedo feedback were mainly due to methodological differences (Ingram et al., 1989). Methods were also developed to diagnose all TOA radiative feedbacks (Hansen et al., 1984; Wetherald and Manabe 1988). Moreover, Cess and Potter (1988) developed a methodology designed to assess cloud feedback. Feedback diagnostic methods paved the way for broader model intercomparisons and enabled a consistent understanding of why projections differ (see Section 4).
Early multi-model intercomparisons identified snow and sea ice albedo feedbacks and their interactions with cloud feedback as a key polar climate uncertainty. The first large-scale, coordinated climate model intercomparison occurred in the late 1980s finding a three-fold difference in global climate sensitivity mainly due to cloud feedback differences (Cess et al., 1989; 1990). Using a similar set of models, Cess et al. (1991) reported substantial snow-albedo feedback differences; interestingly, these differences stemmed not only from the snow-albedo treatment but also from interactions with clouds. Given the demonstrated value of using the large-scale model intercomparisons to indicate uncertainty, model intercomparison projects (MIPs) emerged as a major research theme and continue to be a valuable resource for hypothesis testing, identifying projection uncertainty, and informing climate observation system design (e.g., Wielicki et al., 2013).
In the 1990s, aided by improved computational capabilities, transient climate change simulations became widespread alongside MIPs and advanced our understanding of the interactions between ocean and atmosphere circulation and polar climate. A decade earlier, Bryan et al. (1982) made the first attempt to simulate the transient climate response using a 1% per year CO2 increase experiment finding different high- and low-latitude transient responses. Subsequent transient experiments show a profound influence of the ocean circulation on the spatial distribution of Arctic warming with slower warming over the ocean and in regions of deep water formation (e.g., northern North Atlantic) and faster warming over land (Washington and Meehl 1989; Manabe et al., 1991; Washington and Meehl 1996; Meehl et al., 2000). Manabe et al. (1992) argued that the land-ocean warming contrast affects the land precipitation and soil moisture response by delaying latent heat transport from ocean to land. Washington and Meehl (1989) found a time-dependence of the high-latitude atmospheric circulation response suggesting that there may not be a single atmospheric circulation pattern that amplifies monotonically with increased forcing. While advancing our knowledge of the transient Arctic climate response, these studies did not change the underlying understanding of the physical drivers of polar amplification.
In the 2000s, the drastic sea ice retreat and warming in the Arctic seems to have spurred a newfound urgency and polar amplification began appearing as a unique research topic, as opposed to an aspect of CO2-induced climate change. Studies using multi-decadal records of Arctic temperature, snow cover, and sea ice became prominent and enabled the verification of many early predictions of AA including its fall/winter maximum, bottom-heavy structure, and the prominence of surface albedo feedback (e.g., Graversen et al., 2008; Serreze et al., 2009; Pistone et al., 2014). This application of observations is in sharp contrast to the 1980s when the quality and quantity of observations limited their use to control climate tuning. Multi-decadal observations further enabled studies of emergent constraints—relationships between an uncertain aspect of climate projections and an observable quantity (e.g., Hall and Qu 2006; Caldwell et al., 2014; Hall et al., 2019). MIP activities revealed that sea ice extent and thickness, ocean heat transport, and clouds are key sources of inter-model differences in AA (Holland and Bitz 2003) and potential emergent constraints.
Several studies in the early 2000s altered the trajectory of polar amplification research by showing that polar amplification was possible without the surface albedo feedback. First, aquaplanet experiments by Alexeev (2003) illustrated polar amplification in the absence of sea ice. Second, coupled GCM experiments with a suppressed surface albedo feedback showed polar amplification, albeit weaker (Hall 2004). These results appear at odds with earlier studies also suppressing the surface albedo feedback that concluded the sea ice albedo feedback was necessary for polar amplification (e.g., Ingram et al., 1989; Rind et al., 1995). The Ingram et al. (1989) modeling setup prohibited ocean energy transfer across seasons, which may explain the different conclusion; the reason for the difference with Rind et al. (1995) is unclear. Studies argue that poleward heat transport produces polar amplification due to an increased efficiency, as poleward traveling air is warmer and moister than before (Alexeev et al., 2005; Cai 2005; Cai 2006). Differences in insolation and clouds are also possible explanations as they can control the existence of polar amplification (Kim et al., 2018). This debate continues (Section 5e) and these studies mark an inflection point in our thinking on the role of atmospheric poleward heat transport in polar amplification.
Since 2010, studies have focused on using observations and coupled models synergistically to understand polar amplification, including a reemergence of idealized model set-ups (e.g., Chung and Räisänen, 2011; Feldl et al., 2017a; Yoshimori et al., 2017; Park et al., 2018; Shaw and Tan, 2018; Stuecker et al., 2018; Previdi et al., 2020; Semmler et al., 2020). New satellite data sets (Winker et al., 2010; Boisvert et al., 2013; Kato et al., 2018; Loeb et al., 2018; Duncan et al., 2020) and more sophisticated meteorological reanalyses have been enabling factors (Screen and Simmonds, 2010; Boisvert and Stroeve 2015). Key outcomes of recent work include confirming the role of ocean heat storage, seasonal energy transfer, and the surface turbulent flux response on AA and inter-model spread (Screen and Simmonds, 2010; Boeke and Taylor 2018; Kim et al., 2019; Dai et al., 2019). Studies continue to focus on understanding atmosphere, sea ice, and ocean processes with a keen focus on coupling. Idealized model simulations have been combined with observations to understand shorter time scale atmosphere-ocean-sea ice interactions, including links between air-mass transformation and Arctic climate (e.g., atmospheric rivers and cold air outbreaks; Pithan et al., 2018). Additionally, large single-model initial condition ensembles (e.g., Kay et al., 2015) hold incredible value for understanding the impact of internal variability on observed and projected trends. Studies continue to leverage the trove of information available from MIP activities including the first Polar Amplification MIP (PAMIP; Smith et al., 2019). While our understanding of polar amplification has advanced since Arrhenius, substantial uncertainty remains in polar climate projections warranting continued research.
Sustained polar observations (satellite, ground-based, and airborne) have enabled the identification of many fundamental characteristics of AA and the verification of early modeling results. Technological advances in polar observation have led to higher quality data records and a broader set of observed variables. Developments in meteorological, oceanic, and sea ice reanalysis have made these a primary source of Arctic climate information and are invaluable to AA science. In addition to multi-decadal records, observational capabilities now provide near-real time monitoring of the Arctic, elevating the episodic nature and interconnectedness of the region to the forefront of Arctic research. Detailed process-oriented observations reveal how sea ice, ocean and atmosphere interact and which processes shape the surface energy budget (SEB; e.g., Uttal et al., 2002; Shupe et al., 2020).
Since 1960, the Arctic has warmed faster than any other region of the planet (Figure 1). The zonal average surface temperature trends poleward of 60°N range from ∼0.3 to 0.7 K decade−1 and are strongest near the pole. Spatially, Arctic surface temperature trends range from ∼0.1 to 0.8 K decade−1 with the largest warming coinciding with substantial sea ice concentration declines (Figure 2). The seasonal contrast in Arctic surface warming is also evident (Figure 3) with maximum warming in December-January-February (DJF), minimum warming in June-July-August (JJA), and substantial warming in September-October-November (SON) and March-April-May (MAM). Figure 3 indicates a spatial variation of the seasonal surface warming pattern that coincides with the seasonality of sea ice loss modulated by atmospheric circulation variability (Ding et al., 2017; Dai et al., 2019; Wu et al., 2021). The characteristic surface-based warming profile is evident in the 1979–2020 ERA5 annual, zonal mean atmospheric temperature trends with surface trends exceeding 0.8 K decade−1 decreasing to ∼0.4 K decade−1 at 300 hPa (Figure 4).
FIGURE 2. Recent changes in the Arctic surface climate. Linear, annual mean trends from 2002–2020 for (A) skin temperature (K decade−1), (B) surface air temperature (K decade−1), (C) skin and surface air temperature difference (K decade−1), and (D) sea ice concentration (% decade−1) from the Atmospheric Infrared Sounder (AIRS; Susskind et al., 2014) and passive microwave sea ice concentration data (Cavalieri et al., 1996).
FIGURE 3. Arctic surface warming seasonality. The spatial pattern of the 2010–2020 surface temperature anomalies (units: K) relative to the 1960–2020 average are shown for (A) DJF, (B) MAM, (C) JJA, and (D) SON. Panel (E) shows the zonal mean temperature anomalies for each season. All temperature anomalies are computed from GISTEMP (GISTEMP team 2021).
FIGURE 4. Vertical structure of recent Arctic warming. Annual, zonal mean air temperature linear trends (K decade−1) between for 1979–2020 computed from ERA-5 (Hersbach et al., 2019).
Arctic sea ice cover and thickness have declined dramatically since 1979, further evidence that sea ice is a key aspect of observed AA (Screen and Simmonds, 2010; Boeke and Taylor 2018; Dai et al., 2019). September sea ice extent has declined more rapidly than during any other month, ∼–13% decade−1 (e.g., Parkinson and DiGirolamo 2016; Taylor et al., 2017). September sea ice volume has declined by >70% since the early 1980s (Schweiger et al., 2011; Kwok 2018). The Arctic sea ice melt season has also lengthened by 5–10 days decade−1 over the last four decades (earlier melt onset and later freeze-up) with larger regional changes (Markus et al., 2009; Stroeve et al., 2014; Bliss and Anderson 2018). The thinner and less expansive sea ice cover is also more susceptible to thermodynamic and dynamic forcing (Hibler 1979; Maslanik et al., 2011; Hegyi and Deng 2017; Huang et al., 2019a) promoting earlier and more rapid spring melting (Markus et al., 2009; Maslanik et al., 2011; Stroeve et al., 2014; Bliss and Anderson, 2018), contributing to the observed AA.
The Arctic SEB has responded to the sea ice and temperature trends. Clouds and Earth’s Radiant Energy System (CERES) data show strong trends in TOA and surface energy fluxes in the Arctic (Loeb et al., 2018; Kato et al., 2018). Surface albedo has declined by ∼0.03–0.04 decade−1 over the central Arctic (Duncan et al., 2020) suggesting an additional ∼1.2 Wm-2 decade−1 of shortwave (SW) energy deposited in the Arctic Ocean since 2000 (Figure 5). Strong SH and LH flux increases (Figure 5) have also occurred, coinciding with sea ice loss (Screen and Simmonds, 2010; Boisvert et al., 2013; Boisvert et al., 2015; Taylor et al., 2018). Importantly, polar TOA and surface radiative (SW and longwave (LW)) and turbulent (SH and LH) energy flux observations contain substantial uncertainties—5–20 Wm-2 and 20%, respectively—that stymie studies of climate-relevant processes (Boisvert et al., 2015; Kato et al., 2018; Taylor et al., 2018).
FIGURE 5. Recent changes in the Arctic surface energy budget. Linear, annual mean trends from 2002–2020 for surface (A) downwelling LW radiation, (B) upwelling LW radiation, (C) downwelling SW radiation, and (D) upwelling SW radiation, (E) sensible heat, and (F) latent heat flux trends (W m−2 decade−1). Radiation data is taken from CERES (Kato et al., 2018) and SH and LH fluxes are derived from AIRS (Boisvert et al., 2013).
Several key insights are gleaned from the observed Arctic changes. First, the spatially coincident changes in the Arctic surface temperature, sea ice, and SEB demonstrates the importance of atmosphere-sea ice-ocean coupling to observed Arctic changes. Second, observations verify the existence of AA and key characteristics including its seasonal, vertical, and spatial structure. Lastly, the expected SEB changes (e.g., reduced surface albedo and increased SH and LH fluxes) are observed, although observational uncertainty limits progress.
A hierarchy of models have been used to advance AA science. This evolution in modeling studies coincided with advances in computational capabilities and trends towards increased complexity beginning with EBMs (Budyko 1966, 1969; Sellers 1969), simplified/idealized atmospheric GCMs (MW75; MS80; Alexeev et al., 2005), atmospheric GCMs (Washington and Meehl 1984), coupled atmospheric-ocean GCMs (Bryan et al., 1982; Spelman and Manabe 1984; Washington and Meehl 1989), and now Earth System Models. The march from idealized to complex progressed piecewise, one new component at a time, providing insight into the influence of various climate system components on Arctic climate.
The less complex, computationally-constrained models of the 1980s identified fundamental features of AA that have withstood observational evidence and the scrutiny of more complex models. These features include the magnitude of AA (∼2–3 times global mean warming), seasonality and spatial variation of Arctic warming, bottom-heavy/surface-based profile, increased poleward LH transport, and the acceleration of the hydrologic cycle. Reduced-complexity models captured the fundamental processes influencing the Arctic response to increased CO2 including the sea ice and snow surface albedo, poleward atmospheric and oceanic heat transports, seasonal energy transfer, atmosphere-sea ice-ocean coupling, and cloud radiative effects. While increasingly complex and more realistic contemporary models provide similar insights into AA (Figure 6), their value is from the refined quantitative estimates of process contributions and more reliable projections of future climate. While no one argues for a return to reduced-complexity representations of sea ice, clouds, and the ocean to produce climate projections, reduced-complexity models enable an intuitive understanding of climate processes that is hard to glean from comprehensive models (Held 2005; Jeevanjee et al., 2017; Maher et al., 2019).
FIGURE 6. Arctic Amplification in CMIP6. (A) Zonal mean temperature trends (K decade−1) for 22 CMIP6 models from the SSP5-8.5 simulation. The inset depicts the seasonal cycle of temperature trends for the Arctic domain (poleward of 60°N). (B) The vertical profile of zonal mean temperature trends (K decade−1) for CMIP6 ensemble mean is shown.
Climate community organization around MIP activities has played a key role in AA science by providing inputs for climate projections and uncertainty assessments. Model intercomparison activities have grown from 14 models (Cess et al., 1989) to >40 models in Coupled MIP 5 and 6 (CMIP5 and 6) allowing for robust assessments of inter-model spread. Considering the two most recent CMIPs, the overall spread in the AA factor (defined as the ratio of Arctic-to-global mean surface warming) at the end of the 21st Century for the CMIP5 RCP8.5 (Taylor et al., 2012) and CMIP6 SSP8.5 scenarios (Eyring et al., 2016) has not narrowed significantly (Figure 7). However, no CMIP6 model with the available output simulates an AA factor <2. MIPs have also expanded to dedicated projects organized around scientific themes, including Polar Amplification MIP (Smith et al., 2019). Details on advances from model intercomparison studies can be found in Sections 2 and 5.
FIGURE 7. Arctic Amplification and Contemporary Climate Models. Zonal mean Arctic Amplification factor (ratio of zonal average to global mean surface temperature change) for (A) CMIP5 RCP8.5 and (B) CMIP6 SSP5-8.5. The surface temperature change is computed as the difference between the 2080–2100 and the 2015–2025 periods.
Innovative modeling approaches and experimental designs are being developed to test AA hypotheses, including a revitalization of idealized experiments. Specific results are covered in the feedback diagnostics section and Section 5. The complementary use of complex and idealized model experiments is a critical component of advancing AA science.
Frameworks quantifying how forcings and feedbacks contribute to AA can be classified into the following: energy budget-based diagnostics, mechanism denial experiments, latitudinally-constrained or otherwise idealized forcing, and sea ice forcing experiments.
Energy budget decompositions have been widely used to diagnose climate feedback contributions to surface warming. Individual feedback contributions are evaluated as climate feedback parameters that quantify the global mean TOA energy flux perturbation per unit of global mean surface warming (Wetherald and Manabe 1988; Soden and Held 2006; Shell et al., 2008; Huang et al., 2017a; Pendergrass et al., 2018). Although this method assumes that feedbacks are linear and additive, neural networks can account for nonlinearity (Zhu et al., 2019; Huang et al., 2021a). The energy budget decomposition method can also quantify the influence of regional feedbacks on the warming pattern alongside the radiative forcing, atmospheric energy transport, and ocean heat uptake (e.g., Crook et al., 2011; Taylor et al., 2011a,b; Feldl and Roe 2013; Armour et al., 2013; Pithan and Mauritsen 2014; Hu et al., 2020).
A complementary approach uses the SEB, which is important in the Arctic where the physical validity of the TOA framework is questioned (Pithan and Mauritsen 2014; Payne et al., 2015; Goosse et al., 2018; Henry et al., 2021). Like TOA, the contributions of individual SEB terms to surface temperature change can be diagnosed (Lu and Cai 2009a; Pithan and Mauritsen 2014; Sejas et al., 2014; Laîné et al., 2016; Sejas and Cai 2016; Boeke and Taylor 2018). A SEB decomposition includes additional non-radiative terms (surface turbulent fluxes and ocean heat storage) that are especially important when considering the surface temperature response seasonality.
An expansion of the SEB approach is the coupled atmosphere surface climate feedback response analysis method (CFRAM)—a vertically-resolved version of the energy budget decomposition method (Lu and Cai 2009b; Cai and Lu 2009; Taylor et al., 2013). CFRAM provides a three-dimensional analysis of feedback contributions to the surface and atmospheric temperature response from radiative and non-radiative processes (convection, condensational heating, surface turbulent fluxes, and horizontal heat transport) (Song et al., 2014; Yoshimori et al., 2014). CFRAM does not include a lapse rate feedback and provides a clearer diagnosis of the process contributions to the vertical warming structure. However, the CFRAM is computationally expensive and computes heat transports as a residual; explicitly calculated heat transport terms are straightforward to include in CFRAM however these terms are not routine model outputs. A disadvantage of all energy budget decompositions is that they do not provide clear insights into how different feedbacks are coupled. For example, the radiative sensitivity to albedo changes varies by a factor of two across climate models in the Arctic and Southern Ocean due to inter-model differences in mean-state cloudiness (Donohoe et al., 2020).
Mechanism denial experiments—model simulations where a physical process is “turned off” or locked—also provide insights into the role of various feedbacks (e.g., Wetherald and Manabe 1988; Ingram et al., 1989; Rind et al., 1995; Hall 2004; Vavrus 2004; Graversen and Wang 2009). These studies analyze differences between climate model simulations with a specific process “turned off” and experiments with the process “turned on,” such as sea ice albedo locking (e.g., Graversen et al., 2014), cloud locking (Vavrus 2004; Middlemas et al., 2020), and atmospheric heat transport divergence locking experiments (Graversen and Langen 2019). This approach highlights the coupling between processes that energy budget decomposition approaches cannot (Merlis 2014). The disadvantages of mechanism denial experiments are that they can modify the reference climate, introduce compensating effects, are challenging to apply to comprehensive climate models, and the results are difficult to compare with observations.
Lastly, different modelling protocols have been designed to understand the local and remote mechanisms to AA. Regionally applied greenhouse gas forcing experiments (Section 5e) are one such protocol designed to separate these contributions to Arctic warming (Alexeev et al., 2005; Chung and Räisänen 2011; Yoshimori et al., 2017; Shaw and Tan 2018; Stuecker et al., 2018). Another protocol isolates local and remote mechanisms by prescribing local and remote changes in sea surface temperature and sea ice concentration. Using this approach, Screen et al. (2012) attribute near-surface Arctic warming to local feedbacks and upper tropospheric warming to remote processes. Recent years have seen a proliferation of modeling experiments in which the sea ice component of a coupled ocean-atmosphere model is perturbed, including albedo reduction (e.g., Blackport and Kushner 2016; Liu and Fedorov, 2019), LW emissivity manipulation (e.g., Liu et al., 2019); sea-ice ghost forcing (e.g., Deser et al., 2015), ocean heat flux adjustment (Oudar et al., 2017), and sea ice nudging (McCusker et al., 2017; Smith et al., 2017). Although they all produce a consistent atmospheric circulation response (Screen et al., 2018), the various protocols make different and confounding assumptions regarding conservation of energy and melt water.
Each diagnostic method has strengths and weaknesses (Table 1) associated with technical aspects and underlying assumptions. These differences confound the ability to clearly assess the process contributions to AA. The community needs to address this issue to advance AA science.
TABLE 1. Summary of feedback diagnostic frameworks. The selected example reference in the right column represents a single study that demonstrates each framework.
Sea ice and snow cover changes via the positive surface albedo feedback are a principal driver of AA (Arrhenius 1896; Budyko 1969; MW75; Hall 2004). The surface albedo feedback operates when (high albedo) sea ice and snow cover melts and reduces surface albedo by uncovering the (low albedo) ocean and land surfaces underneath. Reducing surface albedo causes greater absorption of solar radiation that warms the surface and drives additional sea ice and snow melt. Studies estimate that the sea ice-snow albedo feedback is responsible for 30–60% of the total CO2-induced Arctic warming (Dickinson et al., 1987; Hall 2004; Taylor et al., 2013; Boeke and Taylor 2018; Duan et al., 2019) and is the largest local Arctic feedback (Taylor et al., 2013; Yoshimori et al., 2014; Goosse et al., 2018). Multi-centennial climate simulations show that Arctic warming slows after most of the sea ice melts, further highlighting the importance of sea ice (Bintanja and van der Linden 2013; Dai et al., 2019).
The surface albedo feedback has substantially contributed to the observed Arctic warming. Observations of a reduced snowpack (Warren et al., 1999; Brown and Robinson 2011; Webster et al., 2014) and significant declines in sea ice extent, thickness, and age since 1979 indicate a reduced Arctic surface albedo (Nghiem et al., 2007; Maslanik et al., 2011; Parkinson and DiGirolamo, 2016; Kwok, 2018). Additionally, the albedo of multi-year sea ice has decreased (Riihelä et al., 2013). Perovich et al. (2007) computed that reduced surface albedo has increased the solar energy deposited into the Arctic Ocean by 89% from 1979–2005. CERES data indicate a −0.025 ± 0.004 decade−1 Arctic average albedo decline and a +1.2–1.3 Wm−2 decade−1 increase in absorbed TOA solar radiation between 2000 and 2018 (Duncan et al., 2020).
The surface albedo feedback has contributed substantially to the inter-model spread in Arctic warming across multiple generations of intercomparisons (Cess et al., 1991; Holland and Bitz, 2003; Hu et al., 2020). This uncertainty results from the complexities of modeling the continuously evolving sea ice and snow coverage, thickness, and optical properties (Zhang et al., 2000; Laxon et al., 2003)—processes for which available data is insufficient. Furthermore, the rapidly evolving factors that govern surface albedo (e.g., snow and sea ice thickness distribution, topography, drift, melt pond and floe size distribution) occur at small scales making parameterization difficult (Holland et al., 2010, 2012; Schweiger et al., 2011; Jahn et al., 2012; Stroeve et al., 2014).
Sea ice and snow also modulate surface turbulent energy fluxes giving rise to the sea ice insulation feedback. This feedback operates when changes in sea ice concentration and snow and ice thickness alter the non-radiative surface fluxes (sea ice conductance and surface turbulent fluxes; Burt et al., 2016). Sea ice loss exposes a larger area of the Arctic Ocean to the atmosphere and allows for a freer exchange of water vapor, aerosol particles, energy, and momentum with the atmosphere. The sea ice insulation feedback is strongest where there are large surface and near surface air temperature differences collocated with reduced sea ice cover (Serreze et al., 2009; Screen and Simmonds 2010a; Screen and Simmonds, 2010b; Serreze and Barry 2011; Boisvert et al., 2015; Boisvert and Stroeve, 2015; Boeke and Taylor 2018; Taylor et al., 2018). In addition, thinner and less snow-covered sea ice promotes greater heat conduction through sea ice (MS80; Rind et al., 1995; Persson et al., 2017). Through these mechanisms, the ice insulation feedback warms and moistens the lower Arctic atmosphere promoting additional warming via an enhanced greenhouse effect (Kim et al., 2016; Boeke and Taylor 2018; Kim et al., 2019; Feldl et al., 2020; Chung et al., 2021).
Sea ice cover influences the Arctic SEB differently during polar day and night and in both cases strongly impacts surface temperature. Less sea ice cover during polar day decreases the surface albedo and increases SW absorption (Figures 8A,B). Less sea ice cover also promotes larger ocean waves due to longer fetches that have the potential to mechanically break-up sea ice (Rogers et al., 2016). The greater effective heat capacity of the ocean relative to sea ice suppresses warming caused by the surface energy gain during polar day, leading to ocean heat storage and a delayed sea ice freeze up (Dwyer et al., 2012). During polar night, less sea ice cover corresponds to a warmer surface temperature, weaker static stability, and larger upwards surface turbulent fluxes (Figures 8C,D); climatologically cold temperatures over sea ice enable large surface warming when the ice thins and transitions to open ocean (Feldl and Merlis 2021). Atmospheric temperature tends to be warmer in regions with less sea ice in part due to the warming and moistening of the lower atmosphere by increased surface turbulent fluxes, increasing downwelling LW (DLW) radiation (Figure 8D). The greater ocean effective heat capacity also changes the relationship between DLW and upwelling LW (ULW); over sea ice surface DLW anomalies do not lead to strong net LW flux imbalances because sea ice temperature quickly warms in response (Persson et al., 2017; Hegyi and Taylor 2018). These differences in the SEB response to a sea ice change during polar day and night are key components of our conceptual model (Section 6).
FIGURE 8. Modulation of surface energy fluxes by sea ice. A schematic illustration of the sea ice albedo and ice insulation feedbacks and associated surface energy budget changes from sea ice loss: shortwave (SW), downwelling longwave (DLW), upwelling LW (ULW), sensible heat (SH), latent heat (LH), conductive heat flux through sea ice (Fc) and oceanic heat flux to sea ice (Qw). The black line represents the characteristic atmospheric temperature profiles (TA) over ocean and sea ice during polar (A,B) day and (C,D) night.
Temperature feedbacks are major contributors to AA and contribute substantially to the inter-model differences in CMIP5 (Pithan and Mauritsen 2014). Temperature feedbacks are related to the efficiency of radiative cooling to space and are decomposed into contributions from a vertically-uniform temperature change (the Planck feedback) and the effect of the deviation from a vertically-uniform warming (the lapse rate feedback).
The Planck feedback contribution to AA originates from the nonlinearity of blackbody radiation with temperature, such that at colder temperatures, a larger increase in temperature is required to increase outgoing LW radiation (OLR) by 1 W m−2. The Planck feedback is negative at all latitudes and contributes to AA because it is more negative at low latitudes. However, this nonlinearity effect may be small. Henry and Merlis (2019) replace the nonlinear temperature dependence of blackbody radiation with a linearized version in an idealized moist GCM and find that it does not modify the surface temperature change pattern, as energy transport and lapse rate changes compensate.
The lapse rate feedback contribution to AA originates from the meridional gradient of the feedback sign, negative at low latitudes and positive at high latitudes. In the tropics, convection pins the atmospheric temperature profile to the moist adiabat leading to a larger warming in the upper troposphere than at the surface. This “top-heavy” vertical warming structure leads to a larger increase in OLR per unit increase in surface temperature—a negative lapse rate feedback. By contrast, the Arctic lapse rate feedback is positive because stable stratification promotes bottom-heavy warming. At high-latitudes, the atmosphere is close to radiative-advective equilibrium causing the lapse rate feedback to depend on the type of perturbation: a change in greenhouse forcing, for example, has a more bottom-heavy temperature response than a change in atmospheric heat transport (Payne et al., 2015; Cronin and Jansen, 2016).
This dependence on perturbation type presents a challenge in determining the relative importance of radiative, surface-based, and advective controls on the lapse rate feedback. In the absence of a surface albedo feedback, Henry et al. (2021) finds that the increase in CO2 and water vapor alone cause a surface-enhanced warming, consistent with analytic column model results (Cronin and Jansen 2016). Song et al. (2014) argue that the water vapor and albedo feedbacks cause the positive Arctic lapse rate feedback. Mechanism denial experiments reveal that the surface albedo feedback enhances the high-latitude lapse rate feedback (Graversen et al., 2014; Feldl et al., 2017a), or equivalently surface-amplified warming is found in targeted sea ice loss experiments (e.g., Screen et al., 2018). Further, the Arctic lapse rate feedback is strongly correlated across models with summer sea ice loss and cold-season increases in surface turbulent heat fluxes (Feldl et al., 2020; Boeke et al., 2021). Atmospheric energy transport changes tend to reduce the Arctic lapse rate feedback (Feldl et al., 2020) via increases in moist energy transport and decreases in dry energy transport that warm the mid-troposphere and cool the near-surface atmosphere (Henry et al., 2021). Moreover, the decrease in dry transport is strongly controlled by the surface albedo feedback strength (Feldl et al., 2017b; Henry et al., 2021). The high latitude lapse rate feedback results from the sum of these different processes with strong evidence for the importance of surface processes (Cai and Lu 2009; Boeke et al., 2021).
From the surface perspective, the temperature feedback manifests as increased DLW radiation due to atmospheric warming, warming the surface and increasing ULW radiation. The coupling between increased DLW and ULW via the greenhouse effect constitutes a positive feedback loop amplifying surface and atmospheric warming (Sejas and Cai 2016; Zeppetello et al., 2019). Previous studies argue that this feedback accounts for most of the Arctic surface warming (Pithan and Mauritsen 2014; Laîné et al., 2016; Sejas and Cai 2016). Additional studies point to the importance of increased clear-sky DLW on the fall/winter Arctic warming maximum (Lu and Cai 2009a; Boeke and Taylor 2018). Though important to AA, the surface perspective of the temperature feedback does not provide clear insight into the processes that trigger it.
Cloud processes modulate the radiative fluxes and thermodynamic structure of the Arctic atmosphere. The TOA Arctic cloud feedback in CMIP5 is generally negative (Zelinka et al., 2012) and is positive from the surface perspective (Taylor et al., 2013; Boeke and Taylor 2018), indicating that cloud feedback both increases TOA reflected SW and increases surface DLW (Taylor et al., 2011b; Taylor et al., 2013; Pithan and Mauritsen 2014). The magnitude and large inter-model spread of the Arctic cloud feedback comes from model discrepancies in the projected changes in cloud fraction, particularly at low-levels, and optical depth (Vavrus 2004, Vavrus et al., 2008, Vavrus et al., 2011; Liu et al., 2012; Morrison et al., 2019; English et al., 2014, Vignesh et al., 2020). Multiple interacting processes, illustrated in Figure 9, contribute to inter-model differences in the Arctic cloud feedback: surface-atmosphere coupling, cloud microphysics and precipitation, and interactions with large-scale atmospheric dynamics and thermodynamics (e.g., Curry et al., 1996).
FIGURE 9. Dominant Arctic cloud responses to warming during summer and non-summer months. Summer cloud fraction and optical depth changes between a (A) cooler to a (B) warmer Arctic are small due weak surface-cloud coupling and a weak cloud-phase feedback. Cloud masking (not depicted) damps the TOA radiative budget response to reduced surface albedo by blocking sunlight from reaching the newly uncovered, underlying, and darker ocean. Non-summer (mainly spring and fall) cloud fraction and optical depth between a (C) cooler and a (D) warmer Arctic generally increase as a result of stronger surface-atmospheric coupling and enhanced latent and sensible heat fluxes (orange arrows), a positive cloud phase feedback (warmer temperature replace ice crystals with liquid droplets), and reduced lower tropospheric stability (black line represents the characteristic atmospheric temperature profiles, TA). In summer and non-summer months, newly exposed sea ice exposes the atmosphere to new local sources of marine aerosols that can increase CCN and INP with an uncertain influence on cloud microphysical properties. Note that depicted sea ice conditions are illustrative.
The Arctic optical depth feedback is shaped by changes in cloud thermodynamic phase. In response to warming, cloud ice transitions to water increasing cloud albedo and causing a negative feedback (Figures 9C,D; Mitchell et al., 1989; Li and Le Treut 1992). This feedback is sensitive to cloud ice in the control climate, by determining the amount of ice available to transition. The cloud phase feedback magnitude is likely biased negative in most contemporary climate models due to excessive cloud ice and too little supercooled liquid under present-day conditions, yielding unrealistically large increases in mixed-phase cloud optical thickness with warming (Tsushima et al., 2006; Klein et al., 2009; Komurcu et al., 2014; McCoy et al., 2016; Tan et al., 2016). This cloud optical depth feedback bias may have broader implications to AA by enhancing the Arctic lapse rate feedback (Tan and Storelvmo 2019). Recent model experiments revealed that while global cloud feedbacks warm the Arctic, the local feedback contributes negligibly to Arctic warming (Middlemas et al., 2020) suggesting a potential remote influence (Section 5e). However, the model exhibits a low mixed-phase supercooled liquid bias and likely an optical depth feedback that is too negative.
The stability of the lower troposphere affects cloud processes and constitutes a cloud feedback mechanism. Arctic cloud fraction and optical thickness tend to increase with reduced lower tropospheric stability (LTS; Barton et al., 2012; Solomon et al., 2014; Taylor et al., 2015; Yu et al., 2019). In response to increased CO2, LTS is expected to decrease, promoting increased cloud fraction and optical depth with a seasonally varying character (Boeke et al., 2021). CMIP5 models show substantial cloud-induced warming in fall and winter coincident with large reductions in LTS (Figures 9C,D; Boeke and Taylor 2018). These reductions in LTS are in part due to the large reductions in sea ice (Pavelsky et al., 2011). Thus, cloud changes induced by the LTS mechanism are coincident with cloud-surface turbulent coupling (Kay and Gettelman 2009; Shupe et al., 2013; Solomon et al., 2014; Taylor et al., 2015; Yu et al., 2019).
Cloud-surface coupling represents an important mechanism through which sea ice influences cloud feedback. Sea ice loss tends to increase cloud fraction and optical depth through increased surface evaporation (Curry et al., 1996; Taylor et al., 2015; Abe et al., 2015; Huang et al., 2017b; Morrison et al., 2019; Huang et al., 2021b). However, the sensitivity of clouds to sea ice loss depends on the cloud-surface coupling state and the air-surface temperature gradient. This condition-dependent behavior is responsible for the seasonality of the cloud response to sea ice loss (Figures 9A,B); observational studies find that more liquid clouds result from reduced sea ice in all seasons except summer (Kay and Gettelman 2009; Boisvert et al., 2015; Taylor et al., 2015; Morrison et al., 2018; Huang et al., 2019a; Huang et al., 2019b). Weak air-surface temperature gradients and decoupled cloud layers are typical in Arctic summer conditions (Shupe et al., 2013). Recent research suggests that LH and SH flux increases may elicit different cloud responses (Li et al., 2020). The evidence suggests that the cloud-sea ice feedback promotes surface warming in non-summer months.
Cloud masking influences AA by modifying the strength of other feedbacks. Cloud masking is defined as the difference in the cloud feedback determined using the cloud radiative effect and that using the partial radiative perturbation approach. The cloud masking effect operates by altering the TOA radiative perturbation from a feedback relative to clear-sky and is sensitive to present-day cloud properties. Most pertinent for the Arctic, cloud masking damps the magnitude of the surface albedo feedback by reducing the TOA radiative perturbation from a surface albedo change (Soden et al., 2004). Several studies indicate that the cloud masking effect reduced the TOA radiative impact of the observed surface albedo decline by ∼50% (Sledd and L’Ecuyer 2019; He et al., 2019; Alkama et al., 2020; Stapf et al., 2020). While not a feedback, the cloud masking effect highlights a mechanism through which present-day cloud properties influence Arctic climate change.
Lastly, microphysical processes influence the evolution of cloud radiative properties and modulate cloud feedback (Figure 9). Cloud microphysical processes represent sources and sinks of mixed-phase cloud liquid and ice and modulate the water amount, phase partitioning, and the number and size of hydrometeors (Curry et al., 1996; Beesley and Moritz 1999; Klein et al., 2009; Yang et al., 2015; Tan and Storelvmo 2016; Barrett et al., 2017; Furtado & Field 2017; Wang et al., 2018). However, cloud microphysical processes and their interactions with aerosols are poorly represented in climate models. Ice nucleation mechanisms and ice-nucleating particle (INP) properties and sources are either poorly constrained or not represented in models (Xie et al., 2013; English et al., 2014; Komurcu et al., 2014; Schmale et al., 2021). Mixed-phase cloud INP recycling (Fan et al., 2016; Solomon et al., 2018), secondary ice production (Rangno and Hobbs 2001; Lawson et al., 2017; Sotiropoulou et al., 2020; Zhao et al., 2021) and biological INP-sea ice interactions (Wilson et al., 2015; Irish et al., 2017; Hartmann et al., 2019; Creamean et al., 2020) remain unresolved or unrepresented. In addition, the efficiency of the Wegener-Bergeron-Findeisen process (Tan and Storelvmo 2016), updraft velocity, and ice crystal fall speeds (Ervens et al., 2011; Tan and Storelvmo 2019) are also poorly constrained. These gaps in our understanding of cloud microphysical processes preclude a more quantitative assessment of the Arctic cloud feedback and its influence on AA. Observational constraints that statistically characterize the range of Arctic cloud types are needed to improve parameterized processes and reduce cloud-related uncertainty.
The diversity of Arctic surface types (e.g., sea ice, ocean, and land) dictates features of the spatial structure and seasonality of AA. Surface-type dependent characteristics and processes such as albedo, surface turbulent fluxes, vertical and horizontal heat transport, and heat capacity control the impact of each surface type. Understanding how specific surface types influence the spatial distribution and seasonality of AA may help reduce the inter-model spread.
Explanations of regional variations in AA must consider the underlying surface. Observed temperature changes indicate that regions with the largest sea ice loss are warming most rapidly (Robock 1983; Screen and Simmonds, 2010a; Bekryaev et al., 2010; Boisvert and Stroeve 2015; Figure 2). Moreover, the regional characteristics of warming within a climate model is driven by differences in surface properties and feedbacks (Laîné et al., 2016). Figure 10 illustrates CMIP6 model projections showing that the magnitude and seasonality of warming is a function surface type: namely, sea ice-retreat, sea ice-covered, ice-free ocean, and land (Figure 10; definitions in caption).
FIGURE 10. Hovmoller plot of the monthly time series of the CMIP6 ensemble average Arctic surface temperature changes in SSP5-8.5 for (A) ice-retreat regions (present-day sea ice concentration >15% and future sea ice concentration <15%), (B) ice-covered regions (sea ice concentration >15% in present and future), (C) ice-free ocean (present-day sea ice concentration <15%), and (D) land. The right panels show the total surface warming (K) by 2100 as the difference between the 2090–2100 and 2015–2025 periods for each surface type (solid black line) and the across-model standard deviation (dotted line).
Several processes conspire to cause the largest Arctic warming in sea ice-retreat and sea ice-covered regions (Figure 10). Surface albedo and sea ice insulation feedbacks strongly enhance surface warming (MS80; Screen and Simmonds, 2010a; Taylor et al., 2013; Pistone et al., 2014; Boeke and Taylor 2018). Cloud feedbacks are also positive in these regions, especially in fall/winter (Section 5c). Strong LTS, seasonal ocean energy transfer drive the release of stored ocean heat via SH and LH fluxes (Figures 11C,F), and changes in surface thermal inertia contribute to the maximum winter warming in these regions (Robock 1983; Sejas et al., 2014; Laîné et al., 2016; Sejas and Cai 2016; Boeke and Taylor 2018; Feldl et al., 2020).
FIGURE 11. Surface energy budget response by surface type. CMIP6 SSP5-8.5 ensemble mean surface energy budget changes during polar night and day for (A,D) land, (B,E) ocean, and (C,F) sea ice retreat. Surface types are defined as in Figure 10. Changes are computed as the difference between the 2080–2100 period and the first 20-years of the simulation (2015–2035).
The characteristics of the warming response in ice-free ocean regions differ from sea ice regions. Ice-free regions have a weaker and almost seasonally uniform warming (Figure 10) resulting from the large ocean heat capacity (Dwyer et al., 2012) and weaker positive feedbacks (especially the surface albedo feedback; Boeke and Taylor 2018). Thus, the SEB response is smaller than in sea ice regions and shows an opposite net flux change during winter from differing SH flux responses (Figures 11B,E). Additionally, changes in ocean heat transport also influence the warming (Section 5f), however it is unclear if these changes affect these regions differently.
While warming in land regions has a similar seasonal structure as sea ice, different surface characteristics indicate that a different set of processes cause this signal. Seasonal differences in the surface albedo feedback occur due to the earlier spring peak in land snowmelt compared to sea ice melt (Taylor et al., 2011b). Additionally, the surface albedo feedback is weaker (smaller increases in surface absorbed SW; Figure 11D) over snow-covered land than over sea ice because of smaller albedo differences with the underlying surface, despite being at a lower latitude (Taylor et al., 2011a). Surface turbulent flux changes cool the land during summer as opposed to during winter as in sea ice regions (Figures 11A,D; Laîné et al., 2016; Letterly et al., 2018); the summer warming minimum over land results from increased cooling and earlier snowmelt rather than increased heat storage as in sea ice regions (Boeke et al., 2021). The small heat capacity of land combined with the nonlinearity of the temperature dependence of LW surface cooling (Henry and Vallis 2021) and increased local atmospheric heat transport from sea ice loss to land regions (Deser et al., 2010; Burt et al., 2016; Boeke and Taylor 2018) also contribute to the winter amplification over land.
Despite considerable efforts particularly over the last decade, the role of remote influences on AA is still debated. Here, we define remote impacts on Arctic warming as any warming that occurs due to non-Arctic changes (equatorward of 60°N). Thereby, remote effects are not merely associated with changes in meridional heat transports but include the local feedbacks they initiate or mediate (e.g., water vapor and cloud feedbacks). Understanding the partitioning between local and remotely-induced warming (Figure 12) is crucial for reducing uncertainty in the impacts of non-well mixed climate forcings (e.g., aerosols; Chung and Räisänen, 2011). Further, simulated Arctic warming and variability may depend on the models’ representation of tropical Pacific variability (e.g., Baxter et al., 2019; Ding et al., 2019) and improving Arctic projections may require improved modeling of teleconnections.
FIGURE 12. Schematic illustrates how moisture transport from lower latitudes into the Arctic contributes to the water vapor triple effect (warming from condensation and the greenhouse effects of moisture and clouds) drives direct Arctic warming and induces additional warming though local feedbacks (yellow arrows). Warming by local Arctic feedbacks induced by local forcing mechanisms (red arrows) is depicted separately.
Early EBM studies identified the strong impact of meridional heat transports on polar temperatures (Budyko 1969; Sellers, 1969, North, 1975), and, in GCMs, the opposing responses of dry static energy (DSE) and LH transports due to reductions in the meridional temperature gradient and increases in the moisture gradient (MW80). Flannery (1984) extended the dry EBM approach to include the separate effect of increased LH transport with warming; EBMs continue to be used to study polar warming (e.g., Hwang et al., 2011; Rose et al., 2014; Roe et al., 2015; Merlis and Henry 2018; Armour et al., 2019; Feldl and Merlis 2021).
Despite different meridional shapes of the forcing due to CO2 and solar constant changes, Wetherald and Manabe (1975) and MW80 found that the meridional shape of the response was similar. Langen and Alexeev (2007) identified a preferred polar amplified response mode whose shape is determined by the strength of the TOA radiative restoring feedback and the DSE and LH transports (also see Merlis and Henry (2018)). The concept of a preferred mode is strengthened by the linearity between Arctic and global mean temperature change inferred from the paleoclimate record (Miller et al., 2010) and CMIP5 models (Yoshimori et al., 2017).
GCM experiments have been performed to gauge the remote impact on Arctic warming. Some used a direct extra energy term added to the SEB (“ghost forcing”, Alexeev et al., 2005; Park et al., 2018), some used latitudinally confined CO2 increases (Chung and Räisänen, 2011; Shaw and Tan, 2018; Stuecker et al., 2018; Semmler et al., 2020) while others specified SST increases at lower latitudes (Yoshimori et al., 2017). Common to these approaches is that any Arctic warming that occurs, does so due to the indirect effects of the remote warming. Chung and Räisänen (2011) attribute 60–85% of Arctic warming to non-local drivers, Yoshimori et al. (2017) find 60–70%, Park et al. (2018) ∼50%, Shaw and Tan (2018) ∼60%, and Stuecker et al. (2018) ∼50%. These studies indicate that non-Arctic forcing increases non-Arctic temperatures, which in turn increase Arctic temperatures. Local-Arctic feedbacks then amplify this remotely induced Arctic warming (Figure 12) to produce a final remotely induced warming that accounts for half or more of the full Arctic warming.
AA therefore arises in part due to an asymmetry between low-to-high and high-to-low latitude impacts: low-latitude warming is efficiently communicated poleward while high-latitude warming is less efficiently communicated equatorward (Alexeev et al., 2005; Chung and Räisänen, 2011; Park et al., 2018; Shaw and Tan, 2018; Stuecker et al., 2018; Semmler et al., 2020). Non-Arctic warming tends to produce a rather uniform meridional warming pattern and thereby does not itself cause AA (Park et al., 2018; Stuecker et al., 2018). Nevertheless, the fact that non-Arctic warming does not stay localized, as opposed to local-Arctic induced warming, implies that remote effects contribute significantly to Arctic warming. Similarly, moist EBMs and idealized GCMs produce polar amplification in the absence of a surface albedo feedback due to the down-gradient transport of moist static energy (Alexeev et al., 2005; Langen and Alexeev 2007; Roe et al., 2015; Armour et al., 2019; Russotto and Biasutti 2020).
Tropical impacts on Arctic warming (e.g., Rodgers et al., 2003) have been elaborated in the “tropically excited Arctic warming mechanism” (TEAM, Lee et al., 2011; Lee 2012; 2014). Enhanced convection in the Pacific warm pool leads to strengthened or more frequent excitement of poleward propagating Rossby waves. Through dynamic heating and increased moisture transport into the Arctic, the wave dynamics increase the DLW radiation and lead to warming. The role of tropical Pacific Rossby wave-driven teleconnections to the Arctic has been highlighted for observed warming over northeastern Canada and Greenland (Ding et al., 2014) and Arctic sea ice trends and variability (Ding et al., 2017; Baxter et al., 2019; Ding et al., 2019; Topál et al., 2020). Planetary waves dominate the transport of heat and moisture into the Arctic and can drive temperature increases (Graversen and Burtu 2016; Baggett and Lee 2017). Synoptic waves also transport heat and moisture to the Arctic, but in smaller amounts and only in conjunction with a background of amplified planetary waves (Baggett and Lee 2017).
Several studies have concluded that atmospheric heat transport changes play a small or negligible role in AA, finding a negative correlation between polar amplification and atmospheric heat transport changes (Hwang et al., 2011; Kay et al., 2012; Boeke and Taylor 2018). However, this conclusion may result from neglecting the amplified warming effect of latent heat transport discussed below. Due to the nearly canceling effects of increased LH transport and decreasing DSE transport, models with high AA tend to simulate only small or even negative net heat transport changes. Similar conclusions of a subsidiary role for atmospheric heat transport were drawn by Pithan and Mauritsen (2014), Stuecker et al. (2018) and Feldl et al. (2020) using a TOA kernel-based approach and Taylor et al. (2013) using CFRAM. The discrepancy between these studies and those showing the importance of low-latitude impacts and LH transports is likely due to 1) the effect of transport-driven increases in LH is amplified by accompanying changes in specific humidity and clouds (i.e., a “water vapor triple effect” Figure 12; Cai 2006; Graversen and Burtu, 2016; Baggett and Lee, 2017; Lee et al., 2017; Yoshimori et al., 2017; Graversen and Langen, 2019), 2) differing attribution of warming to local and remote processes, and 3) a focus on vertically-integrated energy transport, which does not account for a disproportionate effect of lower versus upper tropospheric transport on surface temperature (Cardinale et al., 2021).
The “water vapor triple effect” represents the multiple influences of water vapor on the Arctic energy budget relating to the misattribution of local and remote Arctic warming (Figure 12). Water vapor transport from mid-latitudes into the Arctic has multiple effects on the Arctic energy budget beyond the release of latent heat at condensation; before condensation, the added water vapor increases the greenhouse effect and after condensation it leads to increased cloudiness, which in Arctic winter has a warming effect. We call this the water vapor triple effect and it means that, per unit of energy transported into the Arctic, latent heat transport is more efficient than DSE transport at warming the Arctic. Graversen and Burtu (2016) show that LH transport eventually leads to an order of magnitude more Arctic warming than DSE transport per unit of energy. When looking at net heat transport changes, this amplified effect is overlooked and the change in total atmospheric heat transport is an unreliable measure of the full effect of atmospheric dynamics (Yoshimori et al., 2017). In offline feedback diagnostic approaches, the water vapor triple effect is attributed to local feedbacks (e.g., water vapor, cloud, lapse rate). Thus, many local feedbacks, as conventionally defined, are not exclusively local in nature. Attribution of total Arctic warming should be considered as four contributions: 1) the local forcing, 2) local feedbacks activated by the locally-induced warming, 3) the remote impacts/forcing and 4) local feedbacks activated by the remotely-induced warming. Impacts 1) and 2) should be counted as locally-induced warming while impacts 3) and 4) should be counted as remotely-induced.
The transport of energy by the oceanic circulation modulates Arctic temperature and sea ice and thus can influence AA. Observations show enhanced ocean heat transports into the Arctic through the Fram Strait and the Barents Sea in recent years (Karcher et al., 2003; Schauer et al., 2004; Dmitrenko et al., 2008; Skagseth et al., 2008; Spielhagen et al., 2011; Årthun et al., 2012). Climate models simulate enhanced high-latitude ocean heat transport under global warming (e.g., Holland and Bitz, 2003; Bitz et al., 2006; Hwang et al., 2011; van der Linden et al., 2019). Several studies suggest that this increased ocean heat transport contributes to Arctic warming (Holland and Bitz, 2003; Hwang et al., 2011; Mahlstein and Knutti, 2011; Singh et al., 2017); in contrast, other studies argue that changes in ocean transport are not correlated with Arctic warming (e.g., Pithan and Mauritsen, 2014; Laîné et al., 2016). This discrepancy mostly comes from the difference of the latitudes where the ocean heat transport is focused (Nummelin et al., 2017). Ocean heat transport increases poleward of 60oN are positively correlated with AA (Holland and Bitz 2003; Hwang et al., 2011; Mahlstein and Knutti 2011).
Several mechanisms contribute to enhanced poleward ocean heat transport under anthropogenic warming. Several studies indicate that increased ocean heat transport in the subpolar North Atlantic is mainly due to warmer Atlantic water (Jungclaus et al., 2014; Koenigk and Brodeau 2014; Nummelin et al., 2017), while other studies highlight ocean circulation changes (Bitz et al., 2006; Rugenstein et al., 2013; Winton et al., 2013; Marshall et al., 2015; Oldenburg et al., 2018; van der Linden et al., 2019). In the latter mechanism, changes in the North Atlantic subpolar gyre or the Atlantic Meridional Overturning Circulation (AMOC) are argued to be important. For example, a strengthened subpolar gyre causes increased oceanic heat transport into the Barents Sea that decreases sea ice and increases oceanic heat release. An anomalous cyclonic circulation is then induced over the Barents Sea that intensifies westerly winds and further promotes oceanic heat transport and warming in the Barents Sea (Ådlandsvik and Loeng, 1991; Goosse et al., 2003; Bengtsson et al., 2004; Arzel et al., 2008; Guemas and Salas-Melia, 2008; Semenov et al., 2009).
Alternatively, the role of AMOC change in high-latitude ocean heat transport and AA is debated. In GFDL models, a stronger AMOC weakening is linked with less high-latitude warming (Rugenstein et al., 2013; Winton et al., 2013). van der Linden et al. (2019) show that changes in the North Atlantic subpolar gyre play a prominent role in modulating ocean heat transport into the Arctic, while AMOC change is a secondary factor in the EC-Earth model. Additionally, the relationship between AMOC and high-latitude ocean heat transport could be different under internal variability and anthropogenic warming (Oldenburg et al., 2018). AMOC is not a one-way forcing on Arctic climate; Arctic sea ice melt under anthropogenic warming may also slow the AMOC after multiple decades (Sévellec et al., 2017; Liu et al., 2019; Li et al., 2021). A recent study (Liu and Fedorov, 2021) reconciles the two-way interaction between Arctic sea ice and the AMOC, which modulates the changes in both variables in a warming climate.
Ocean heat transported into the Arctic from the Atlantic influences Arctic warming and relates to the inter-model spread. Inter-model differences across 18 CMIP6 models (Figure 13) illustrate the relationship between Arctic warming and ocean heat transport across different latitudes. The correlation is positive and becomes statistically significant near 70oN and strengthens moving poleward (Figure 13A), a result consistent with previous studies (Holland and Bitz 2003; Hwang et al., 2011; Mahlstein and Knutti 2011). At 80oN where much of the Atlantic ocean heat enters the Arctic via the Fram Strait, the correlation between Arctic warming and ocean heat transport reaches 0.91. Thus, models with more (less) ocean heat imported into the Arctic via the Atlantic sector simulate stronger (weaker) warming during 2015–2,100 under SSP5-8.5 (Figure 13B).
FIGURE 13. Ocean heat transport and Arctic Warming. (A) The correlation between the trend of average SST over 60–90oN during 2015–2,100 and northward ocean heat transport averaged over 2015–2,100 across different latitudes in the Atlantic basin among 18 CMIP6 climate models under the SSP5-8.5 scenario. For each model, only the first ensemble simulation is used to ensure an equal weight among models. Dark blue indicates the latitudes where the correlation is significant with 95% confidence by Pearson’s r test. (B) The scatter plot of SST trends during 2015–2,100 and northward ocean heat transport averaged over 2015–2,100 across 80oN in the Atlantic sector, with the regression line of the two variables (black).
Long-term climate change and mean energy budgets symbolize the accumulation of short timescale, episodic events. The nature of episodic events has implications for our understanding and projecting of AA. In the seasonal mean, the wintertime Arctic SEB and lower tropospheric temperature profiles are dominated by radiative cooling and strong stable stratification (Serreze et al., 1992). However, at any point in time and space, the Arctic winter boundary layer over sea ice or land tends to be either in a radiatively clear state with no clouds or ice clouds or a cloudy state with low-level liquid containing clouds (Stramler et al., 2011). In the radiatively clear state over sea ice, surface radiative cooling (∼-40 W m−2) drives surface-based temperature inversions with strengths of ∼10–15 K. In the radiatively cloudy state, the surface is in approximate radiative balance with the cloud layer and a weaker temperature inversion is elevated to or above the cloud-top (Sedlar et al., 2012; Pithan et al., 2014).
These two states occur at different stages of air-mass transformations (Pithan et al., 2018; Nygård et al., 2019). Following the intrusion of warm, moist air masses from lower latitudes, radiative cooling leads to cloud formation driving the boundary layer into the cloudy state. After several days over Arctic sea ice or land, cooling and drying of the air-mass causes the mixed-phase cloud to glaciate or decay, transitioning to the clear state. The moisture supply aloft and cloud-top radiative cooling lead to cloud top moisture inversions (e.g., increases in specific humidity with height). Given the differences in the thermodynamic profile and the SEB between these states, changes in their frequency of occurrence can impact wintertime sea ice growth, near-surface air temperature and lapse-rate, water vapor and cloud feedbacks.
Episodic variability can influence AA through multiple mechanisms. Changes in the frequency of radiatively clear and cloudy states due to a change in the magnitude or frequency of moist air mass intrusions and atmospheric rivers could alter the SEB and cloud feedback. Observational analyses suggest an increase in the number of moist intrusions has already contributed to wintertime Arctic warming and reduced sea ice growth (Woods and Caballero 2016a; Woods and Caballero 2016b; Graham et al., 2017a; Graham et al., 2017b; Hegyi and Taylor 2018). The initial properties of incoming air-masses could also change, influencing the longevity of mixed-phase clouds; warmer, moister, and potentially more aerosol laden air-masses are possible due to warming at lower latitudes. The potential impact of AA and sea ice loss on the frequency of circulation states with strong meridional advection has been intensely investigated over the past decade and continues to be debated (e.g., Cohen et al., 2020). Lastly, surface turbulent fluxes over the ice-free ocean represent another mechanism by which episodic variability can influence AA as the magnitude of SH and LH fluxes can change by ∼100 Wm-2 depending upon whether the prevailing winds are from sea ice to ice-free ocean or vice versa (Taylor et al., 2018). A quantitative understanding of the Arctic system response to episodic heat and moisture transport events, air-mass transformation, and cloud formation is needed to reduce uncertainty in Arctic projections.
AA results from a collection of interacting processes. Based upon the available evidence, we deduce five fundamental concepts for AA (Figure 14): (C1) local positive feedbacks amplify the initial local forcing more strongly in the Arctic than elsewhere, (C2) the predominance of stable atmospheric stratification (inversion denoted by the color bar in Figure 14) restricts convective mixing and focuses warming in a shallow near-surface layer, (C3) the seasonal transfer of energy from summer to fall/winter by ocean heat storage in combination with sea ice loss exposing the larger thermal inertia of the ocean and drives the maximum warming in winter, (C4) increased poleward LH transport amplifies Arctic warming through a “water vapor triple effect”, and (C5) activation of local feedbacks by remote atmospheric and oceanic processes drive additional warming. Next, we employ these concepts to describe the AA process.
Initially, rising CO2 levels increase DLW radiation warming the Arctic surface and overlying air with a surface-based vertical structure. Arctic warming excites a suite of positive local feedbacks (C1; cloud, water vapor, and surface albedo) that lead to further warming. The surface albedo feedback represents the strongest positive local feedback and favors a surface-based warming profile that is further promoted by strong atmospheric stable stratification (C2).
Each local feedback has a unique seasonal signature that shapes its contributions to AA. Sea ice decline is strongest in summer, increasing absorbed solar radiation into the Arctic Ocean; however, summer warming is small due to the Arctic Ocean’s large heat capacity and the LH associated with sea ice melt. These processes sequester the surplus energy and transfer it to fall/winter (C3) producing larger warming during these months. The increased upper Arctic Ocean heat content delays fall sea ice freeze onset, exposes the ocean to the atmosphere for a longer time, and increases surface turbulent fluxes from ocean-to-atmosphere. The combination of delayed freeze onset and warmer temperatures promotes less winter sea ice growth and thinner spring sea ice that is more susceptible to earlier summer melt out. This provides more time to accumulate solar radiation in summer, further delaying fall freeze-up.
Simultaneously with these local processes, the rest of the globe warms and moistens in response to increased CO2 causing the air transported into the Arctic to have a larger moist static energy. The poleward moisture transport contributes not only to the LH release associated with condensation but also to an increased greenhouse effect prior to condensation and subsequent increased cloudiness. Through this water vapor triple effect, increased LH transport (C4) overcomes the countering effect of reduced DSE transports due to a weakened equator-to-pole temperature gradient. As a result, remote atmospheric and oceanic processes drive additional surface warming that triggers interactions with local feedbacks (from C1) that cause further warming (C5).
Our conceptual model describes five overall ideas fundamental to AA. We acknowledge that an improved understanding of individual processes is critical for producing reliable Arctic warming projections and resolving inter-model differences. However, our conceptual model highlights the need to accurately account for local feedback and remote process interactions within the context of the annual cycle to constrain the likelihood that future AA will be on the high-end of model projections.
These five ideas fundamental to AA are not all-encompassing. We acknowledge that the highly coupled nature of the atmosphere, hydrosphere, cryosphere, land, and biosphere means other processes such as permafrost thawing, aerosol-cloud interactions, glacier melt, land use change, among others can influence future AA. These processes, however, are either not included or overly simplified in model simulations or are considered of secondary importance. An improved understanding of individual processes is critical for producing reliable Arctic climate projections and resolving inter-model differences. As model fidelity advances and our knowledge expands, we envision that new knowledge will build upon the fundamentals described in our conceptual model.
Arctic Amplification is a fundamental aspect of Earth’s climate as documented in a range of contexts: paleoclimate, present-day observations, and models of varying complexities. Despite these observations and our current understanding, a complete theory of Arctic Amplification remains elusive. Gaps in our understanding have thwarted reliable surface temperature and sea ice projections due to anthropogenic forcing. After reviewing the current understanding of Arctic Amplification and proposing a conceptual model, we have identified key knowledge gaps and recommendations to accelerate progress.
1. A sustained observing system that resolves key Arctic processes is vital. A pursuit is underway (e.g., integrated Arctic Observing Network (AON)) and this work must continue. In addition, we recommend routine Arctic field expeditions with a MOSAiC-like (https://mosaic-expedition.org) scope to provide the missing data needed to advance understanding (Shupe et al., 2020) for all seasons. Our vision is a permanent, floating Central Arctic observatory.
2. Arctic surface energy budget uncertainties inhibit robust conclusions of critical atmosphere-sea ice-ocean processes with signals <10–20 W m−2. We recommend a focus on advancing satellite-based measurement approaches to obtain Arctic-wide surface energy budget information (e.g., advanced IR sounder radiance assimilation; Smith et al., 2021).
3. A quantitative understanding of how individual physical parameterization schemes influence feedback uncertainty is lacking. We recommend modeling experiments, intercomparison studies, and sophisticated statistical analyses (e.g., data-driven causality discovery methods) to quantify the sensitivity of Arctic feedbacks to physical parameterization schemes. An experimental protocol enabling the community to characterize these links across models and parameterization schemes is needed.
4. Surface turbulent flux schemes vary across climate models, producing fluxes that differ markedly from observations. We recommend a coordinated intercomparison of high-latitude surface turbulent flux parameterizations for “standard” cases (e.g., on-sea ice flow, off-sea ice flow, ocean with and without sea ice, etc.) with adequate observational constraints to identify the magnitude and source of model bias.
5. Reliable Arctic projections require an accurate accounting of local feedback and remote process interactions within the context of the annual cycle. We recommend that research on how local feedback and remote process interactions influence the sea ice annual cycle should be a near-term research focus. An improved understanding of these energy exchanges and interactions will accelerate our understanding of Arctic Amplification.
6. While energy balance models and feedback diagnostic frameworks are indispensable, these frameworks obscure the episodic nature of time-averaged quantities and the links between small-scale processes and long-term change. We recommend the influence of episodic variability on Arctic Amplification as a key research focus area, complementary to recommendation 5. Specifically, research into how the Arctic system dissipates energy from heat and moisture transport events, air mass transformation, and cloud formation is needed.
7. Regional climate change indicators for policy targets should be adopted to account for the uneven spatial distribution of climate change impacts and risks. The adoption of regional climate change targets would help to raise the priority of Arctic science.
8. Feedback diagnostic frameworks contain ambiguities and inconsistencies that make physical interpretation unclear. For instance, the lapse rate feedback is defensible in the tropics where moist convection couples the surface and upper troposphere, however its interpretation at high latitudes is less clear. We recommend a working group tasked to rethink feedback definitions and diagnostic frameworks, making them more process-oriented.
Polar amplification has been studied in depth for at least 50 years. While the leading explanation for amplified polar warming remains the surface albedo feedback and strong stratification at high latitudes, new details highlight the important role of atmosphere, ocean, and sea ice coupling processes. The highly coupled nature of the polar regions is a source of substantial uncertainty in regional climate projections. Our understanding of polar amplification has been wedded to computational and technological advances that have enabled more complex climate simulations with more detailed physical parameterizations. The role of observations has also evolved from a tool for model tuning to now being used for direct analysis.
While these advances have contributed to our understanding of polar amplification and must continue, an important step remains; to raise Arctic climate sensitivity on the climate modeling priority list, giving it equal priority to global climate sensitivity. Currently, state-of-the-art knowledge of Arctic processes (e.g., surface turbulent flux bulk formula) have not yet been widely implemented in climate models (Bourassa, 2013). Given the rapidly changing Arctic sea ice conditions, older parameterizations developed under thicker, multi-year sea ice conditions are likely to be less applicable in the ‘new’ Arctic with a predominantly seasonal sea ice cover. Giving Arctic climate sensitivity a high priority ensures the rapid integration of knowledge into climate models and will accelerate the reduction in Arctic climate projection uncertainty.
All authors listed have made a substantial, direct, and intellectual contribution to the work and approved it for publication.
PT, RB, and LB are supported by the NASA Interdisciplinary Studies Program (NNH19ZDA001N-IDS). PT, RB, SS, and YH are support by the NASA Radiation Budget Science Project. IT is funded through the NSERC RGPIN-2021-02720 grant. NF is supported by the US National Science foundation (NSF AGS-1753034). WL is supported by the Alfred P. Sloan Foundation as a Research Fellow, and is also supported by U.S. National Science Foundation (AGS-2053121). Matthew Henry is supported by NERC grant number NE/T00942X/1, under a NERC-NSF partnership named "Dynamics of Warm Past and Future Climates".
The authors declare that the research was conducted in the absence of any commercial or financial relationships that could be construed as a potential conflict of interest.
All claims expressed in this article are solely those of the authors and do not necessarily represent those of their affiliated organizations, or those of the publisher, the editors and the reviewers. Any product that may be evaluated in this article, or claim that may be made by its manufacturer, is not guaranteed or endorsed by the publisher.
Abe, M., Nozawa, T., Ogura, T., and Takata, K. (2015). Effect of Retreating Sea Ice on Arctic Cloud Cover in Simulated Recent Global Warming. Atmos. Chem. Phys. Discuss. 15 (12), 17527–17552. doi:10.5194/acpd-15-17527-2015
Ådlandsvik, B., and Loeng, H. (1991). A Study of the Climatic System in the Barents Sea. Polar Res. 10 (1), 45–50. doi:10.3402/polar.v10i1.6726
Alexeev, V. A., Langen, P. L., and Bates, J. R. (2005). Polar Amplification of Surface Warming on an Aquaplanet in “Ghost Forcing” Experiments without Sea Ice Feedbacks. Clim. Dyn. 24 (7–8), 655–666. doi:10.1007/s00382-005-0018-3
Alexeev, V. A. (2003). Sensitivity to CO2 Doubling of an Atmospheric GCM Coupled to an Oceanic Mixed Layer: a Linear Analysis. Clim. Dyn. 20 (7), 775–787. doi:10.1007/s00382-003-0312-x
Alkama, R., Taylor, P. C., Garcia-San Martin, L., Douville, H., Duveiller, G., Forzieri, G., et al. (2020). Clouds Damp the Radiative Impacts of Polar Sea Ice Loss. The Cryosphere 14 (8), 2673–2686. doi:10.5194/tc-14-2673-2020
Armour, K. C., Bitz, C. M., and Roe, G. H. (2013). Time-Varying Climate Sensitivity from Regional Feedbacks. J. Clim. 26 (13), 4518–4534. doi:10.1175/JCLI-D-12-00544.1
Armour, K. C., Siler, N., Donohoe, A., and Roe, G. H. (2019). Meridional Atmospheric Heat Transport Constrained by Energetics and Mediated by Large-Scale Diffusion. J. Clim. 32 (12), 3655–3680. doi:10.1175/jcli-d-18-0563.1
Arrhenius, S. (1896). XXXI.On the Influence of Carbonic Acid in the Air upon the Temperature of the Ground. Lond. Edinb. Dublin Phil. Mag. J. Sci. 41 (251), 237–276. doi:10.1080/14786449608620846
Årthun, M., Eldevik, T., Smedsrud, L. H., Skagseth, Ø., and Ingvaldsen, R. B. (2012). Quantifying the Influence of Atlantic Heat on Barents Sea Ice Variability and Retreat*. J. Clim. 25 (13), 4736–4743. doi:10.1175/JCLI-D-11-00466.1
Arzel, O., Fichefet, T., Goosse, H., and Dufresne, J.-L. (2007). Causes and Impacts of Changes in the Arctic Freshwater Budget during the Twentieth and Twenty-First Centuries in an AOGCM. Clim. Dyn. 30 (1), 37–58. doi:10.1007/s00382-007-0258-5
Baggett, C., and Lee, S. (2017). An Identification of the Mechanisms that Lead to Arctic Warming during Planetary-Scale and Synoptic-Scale Wave Life Cycles. J. Atmos Sci 74 (6), 1859–1877. doi:10.1175/JAS-D-16-0156.1
Barrett, A. I., Hogan, R. J., and Forbes, R. M. (2017). Why Are Mixed-phase Altocumulus Clouds Poorly Predicted by Large-Scale Models? Part 1. Physical Processes. J. Geophys. Res. Atmos. 122 (18), 9903–9926. doi:10.1002/2016JD026321
Barton, N. P., Klein, S. A., Boyle, J. S., and Zhang, Y. Y. (2012). Arctic Synoptic Regimes: Comparing Domain-wide Arctic Cloud Observations with CAM4 and CAM5 during Similar Dynamics. J. Geophys. Res. 117 (D15), a–n. doi:10.1029/2012JD017589
Baxter, I., Ding, Q., Schweiger, A., L’Heureux, M., Baxter, S., Wang, T., et al. (2019). How Tropical Pacific Surface Cooling Contributed to Accelerated Sea Ice Melt from 2007 to 2012 as Ice Is Thinned by Anthropogenic Forcing. J. Clim. 32 (24), 8583–8602. doi:10.1175/JCLI-D-18-0783.1
Beesley, J. A., and Moritz, R. E. (1999). Toward an Explanation of the Annual Cycle of Cloudiness over the Arctic Ocean. J. Clim. 12 (2), 395–415. doi:10.1175/1520-0442(1999)012<0395:TAEOTA>2.0.CO;2
Bekryaev, R. V., Polyakov, I. V., and Alexeev, V. A. (2010). Role of Polar Amplification in Long-Term Surface Air Temperature Variations and Modern Arctic Warming. J. Clim. 23 (14), 3888–3906. doi:10.1175/2010JCLI3297.1
Bengtsson, L., Semenov, V. A., and Johannessen, O. M. (2004). The Early Twentieth-Century Warming in the Arctic-A Possible Mechanism. J. Clim. 17 (20), 40452–44057. doi:10.1175/1520-0442(2004)017<4045:tetwit>2.0.co;2
Bintanja, R., Graversen, R. G., and Hazeleger, W. (2011). Arctic winter Warming Amplified by the thermal Inversion and Consequent Low Infrared Cooling to Space. Nat. Geosci 4 (11), 758–761. doi:10.1038/ngeo1285
Bintanja, R., and van der Linden, E. C. (2013). The Changing Seasonal Climate in the Arctic. Sci. Rep. 3 (1), 1556. doi:10.1038/srep01556
Bitz, C. M., Gent, P. R., Woodgate, R. A., Holland, M. M., and Lindsay, R. (2006). The Influence of Sea Ice on Ocean Heat Uptake in Response to Increasing CO2. J. Clim. 19 (11), 2437–2450. doi:10.1175/JCLI3756.1
Blackport, R., and Kushner, P. J. (2016). The Transient and Equilibrium Climate Response to Rapid Summertime Sea Ice Loss in CCSM4. J. Clim. 29 (2), 401–417. doi:10.1175/JCLI-D-15-0284.1
Bliss, A. C., and Anderson, M. R. (2018). Arctic Sea Ice Melt Onset Timing from Passive Microwave-Based and Surface Air Temperature-Based Methods. J. Geophys. Res. Atmos. 123 (17), 9063–9080. doi:10.1029/2018jd028676
Boeke, R. C., and Taylor, P. C. (2018). Seasonal Energy Exchange in Sea Ice Retreat Regions Contributes to Differences in Projected Arctic Warming. Nat. Commun. 9 (1), 5017. doi:10.1038/s41467-018-07061-9
Boeke, R. C., Taylor, P. C., and Sejas, S. A. (2021). On the Nature of the Arctic's Positive Lapse‐Rate Feedback. Geophys. Res. Lett. 48 (1), e2020GL091109. doi:10.1029/2020GL091109
Boisvert, L. N., Markus, T., and Vihma, T. (2013). Moisture Flux Changes and Trends for the Entire Arctic in 2003-2011 Derived from EOS Aqua Data. J. Geophys. Res. Oceans 118 (10), 5829–5843. doi:10.1002/jgrc.20414
Boisvert, L. N., and Stroeve, J. C. (2015). The Arctic Is Becoming Warmer and Wetter as Revealed by the Atmospheric Infrared Sounder. Geophys. Res. Lett. 42 (11), 4439–4446. doi:10.1002/2015GL063775
Boisvert, L. N., Wu, D. L., and Shie, C.-L. (2015). Increasing Evaporation Amounts Seen in the Arctic between 2003 and 2013 from AIRS Data. J. Geophys. Res. Atmos. 120 (14), 6865–6881. doi:10.1002/2015JD023258
Bourassa, M. A., Gille, S. T., Bitz, C., Carlson, D., Cerovecki, I., Clayson, C. A., et al. (2013). High-Latitude Ocean and Sea Ice Surface Fluxes: Challenges for Climate Research. Bull. Amer. Meteorol. Soc. 94 (3), 403–423. doi:10.1175/BAMS-D-11-00244.1
Broecker, W. S. (1975). Climatic Change: Are We on the Brink of a Pronounced Global Warming? Science 189 (4201), 460–463. doi:10.1126/science.189.4201.460
Brown, R. D., and Robinson, D. A. (2011). Northern Hemisphere spring Snow Cover Variability and Change over 1922-2010 Including an Assessment of Uncertainty. The Cryosphere 5 (1), 219–229. doi:10.5194/tc-5-219-2011
Bryan, K., Komro, F. G., Manabe, S., and Spelman, M. J. (1982). Transient Climate Response to Increasing Atmospheric Carbon Dioxide. Science 215, 56–58. doi:10.1126/science.215.4528.56
Budyko, M. I. (1966). “Polar Ice and Climate,” in Proceedings of the Symposium on the Arctic Heat Budget and Atmospheric Circulation (Santa Monica, California: Rand Corporation).
Budyko, M. I. (1969). The Effect of Solar Radiation Variations on the Climate of the Earth. Tellus 21 (5), 611–619. doi:10.3402/tellusa.v21i5.10109
Burt, M. A., Randall, D. A., and Branson, M. D. (2016). Dark Warming. J. Clim. 29 (2), 705–719. doi:10.1175/jcli-d-15-0147.1
Cai, M. (2005). Dynamical Amplification of Polar Warming. Geophys. Res. Lett. 32 (22), a–n. doi:10.1029/2005GL024481
Cai, M. (2006). Dynamical Greenhouse-Plus Feedback and Polar Warming Amplification. Part I: A Dry Radiative-Transportive Climate Model. Clim. Dyn. 26 (7–8), 661–675. doi:10.1007/s00382-005-0104-6
Cai, M., and Lu, J. (2009). A New Framework for Isolating Individual Feedback Processes in Coupled General Circulation Climate Models. Part II: Method Demonstrations and Comparisons. Clim. Dyn. 32 (6), 887–900. doi:10.1007/s00382-008-0424-4
Caldwell, P. M., Bretherton, C. S., Zelinka, M. D., Klein, S. A., Santer, B. D., and Sanderson, B. M. (2014). Statistical Significance of Climate Sensitivity Predictors Obtained by Data Mining. Geophys. Res. Lett. 41 (5), 1803–1808. doi:10.1002/2014GL059205
Cardinale, C. J., Rose, B. E. J., Lang, A. L., and Donohoe, A. (2021). Stratospheric and Tropospheric Flux Contributions to the Polar Cap Energy Budgets. J. Clim. 34 (11), 4261–4278. JCLI-D-20-0722.1.xml. doi:10.1175/jcli-d-20-0722.1
Cavalieri, D. J., Parkinson, C. L., Gloersen, P., and Zwally, H. (1996). “Sea Ice Concentrations from Nimbus-7 SMMR and DMSP SSM/I-SSMIS Passive Microwave Data,” in [2003–2016], NASA DAAC at the Natl. Snow and Ice Data Cent. (Boulder, Colo: NSIDC). [Updated yearly].
Cess, R. D., and Potter, G. L. (1988). A Methodology for Understanding and Intercomparing Atmospheric Climate Feedback Processes in General Circulation Models. J. Geophys. Res. 93 (D7), 8305–8314. doi:10.1029/JD093iD07p08305
Cess, R. D., Potter, G. L., Blanchet, J. P., Boer, G. J., Del Genio, A. D., Déqué, M., et al. (1990). Intercomparison and Interpretation of Climate Feedback Processes in 19 Atmospheric General Circulation Models. J. Geophys. Res. 95 (D10), 16601–16615. doi:10.1029/JD095iD10p16601
Cess, R. D., Potter, G. L., Blanchet, J. P., Boer, G. J., Ghan, S. J., Kiehl, J. T., et al. (1989). Interpretation of Cloud-Climate Feedback as Produced by 14 Atmospheric General Circulation Models. Science 245 (4917), 513–516. doi:10.1126/science.245.4917.513
Cess, R. D., Potter, G. L., Zhang, M.-H., Blanchet, J.-P., Chalita, S., Colman, R., et al. (1991). Interpretation of Snow-Climate Feedback as Produced by 17 General Circulation Models. Science 253 (5022), 888–892. doi:10.1126/science.253.5022.888
Chung, C. E., and Räisänen, P. (2011). Origin of the Arctic Warming in Climate Models. Geophys. Res. Lett. 38 (21), a–n. doi:10.1029/2011GL049816
Chung, E. S., Ha, K. J., Timmermann, A., Stuecker, M. F., Bodai, T., and Lee, S. K. (2021). Cold‐Season Arctic Amplification Driven by Arctic Ocean‐Mediated Seasonal Energy Transfer. Earth's Future 9 (2), e2020EF001898. doi:10.1029/2020EF001898
Coakley, J. A. (1977). Feedbacks in Vertical-Column Energy Balance Models. J. Atmos. Sci. 34, 465–470. doi:10.1175/1520-0469(1977)034<0465:FIVCEB>2.0.CO;2
Cohen, J., Zhang, X., Francis, J., Jung, T., Kwok, R., Overland, J., et al. (2020). Divergent Consensuses on Arctic Amplification Influence on Midlatitude Severe winter Weather. Nat. Clim. Chang. 10 (1), 20–29. doi:10.1038/s41558-019-0662-y
Creamean, J. M., Hill, T. C. J., DeMott, P. J., Uetake, J., Kreidenweis, S., and Douglas, T. A. (2020). Thawing Permafrost: an Overlooked Source of Seeds for Arctic Cloud Formation. Environ. Res. Lett. 15 (8), 084022. doi:10.1088/1748-9326/ab87d3
Cronin, T. W., and Jansen, M. F. (2016). Analytic Radiative‐advective Equilibrium as a Model for High‐latitude Climate. Geophys. Res. Lett. 43 (1), 449–457. doi:10.1002/2015GL067172
Crook, J. A., Forster, P. M., and Stuber, N. (2011). Spatial Patterns of Modeled Climate Feedback and Contributions to Temperature Response and Polar Amplification. J. Clim. 24 (14), 3575–3592. doi:10.1175/2011JCLI3863.1
Curry, J. A., Schramm, J. L., Rossow, W. B., and Randall, D. (1996). Overview of Arctic Cloud and Radiation Characteristics. J. Clim. 9 (8), 1731–1764. doi:10.1175/1520-0442(1996)009<1731:OOACAR>2.0.CO;2
Dai, A., Luo, D., Song, M., and Liu, J. (2019). Arctic Amplification Is Caused by Sea-Ice Loss under Increasing CO2. Nat. Commun. 10 (1), 121. doi:10.1038/s41467-018-07954-9
Deser, C., Tomas, R., Alexander, M., and Lawrence, D. (2010). The Seasonal Atmospheric Response to Projected Arctic Sea Ice Loss in the Late Twenty-First Century. J. Clim. 23 (2), 333–351. doi:10.1175/2009jcli3053.1
Deser, C., Tomas, R. A., and Sun, L. (2015). The Role of Ocean-Atmosphere Coupling in the Zonal-Mean Atmospheric Response to Arctic Sea Ice Loss. J. Clim. 28 (6), 2168–2186. doi:10.1175/JCLI-D-14-00325.1
Dickinson, R. E., Meehl, G. A., and Washington, W. M. (1987). Ice-albedo Feedback in a CO2-doubling Simulation. Climatic Change 10 (3), 241–248. doi:10.1007/BF00143904
Ding, Q., Schweiger, A., L’Heureux, M., Battisti, D. S., Po-Chedley, S., Johnson, N. C., et al. (2017). Influence of High-Latitude Atmospheric Circulation Changes on Summertime Arctic Sea Ice. Nat. Clim Change 7, 289–295. doi:10.1038/NCLIMATE3241
Ding, Q., Schweiger, A., L’Heureux, M., Steig, E. J., Battisti, D. S., Johnson, N. C., et al. (20192019). Fingerprints of Internal Drivers of Arctic Sea Ice Loss in Observations and Model Simulations. Nat. Geosci 12, 28–33. doi:10.1038/s41561-018-0256-8
Ding, Q., Wallace, J. M., Battisti, D. S., Steig, E. J., Gallant, A. J. E., Kim, H.-J., et al. (2014). Tropical Forcing of the Recent Rapid Arctic Warming in Northeastern Canada and Greenland. Nature 509, 209–212. doi:10.1038/nature13260
Dmitrenko, I. A., Polyakov, I. V., Kirillov, S. A., Timokhov, L. A., Frolov, I. E., Sokolov, V. T., et al. (2008). Toward a Warmer Arctic Ocean: Spreading of the Early 21st century Atlantic Water Warm Anomaly along the Eurasian Basin Margins. J. Geophys. Res. 113 (C5). doi:10.1029/2007JC004158
Donohoe, A., Blanchard-Wrigglesworth, E., Schweiger, A., and Rasch, P. J. (2020). The Effect of Atmospheric Transmissivity on Model and Observational Estimates of the Sea Ice Albedo Feedback. J. Clim. 33 (13), 5743–5765. doi:10.1175/JCLI-D-19-0674.1
Duan, L., Cao, L., and Caldeira, K. (2019). Estimating Contributions of Sea Ice and Land Snow to Climate Feedback. J. Geophys. Res. Atmos. 124 (1), 199–208. doi:10.1029/2018JD029093
Duncan, B. N., Ott, L. E., Abshire, J. B., Brucker, L., Carroll, M. L., Carton, J., et al. (2020). Space‐Based Observations for Understanding Changes in the Arctic‐Boreal Zone. Rev. Geophys. 58 (1), e2019RG000652. doi:10.1029/2019RG000652
Dwyer, J. G., Biasutti, M., and Sobel, A. H. (2012). Projected Changes in the Seasonal Cycle of Surface Temperature. J. Clim. 25 (18), 6359–6374. doi:10.1175/JCLI-D-11-00741.1
English, J. M., Kay, J. E., Gettelman, A., Liu, X., Wang, Y., Zhang, Y., et al. (2014). Contributions of Clouds, Surface Albedos, and Mixed-phase Ice Nucleation Schemes to Arctic Radiation Biases in CAM5. J. Clim. 27 (13), 5174–5197. doi:10.1175/JCLI-D-13-00608.1
Ervens, B., Feingold, G., Sulia, K., and Harrington, J. (2011). The Impact of Microphysical Parameters, Ice Nucleation Mode, and Habit Growth on the Ice/liquid Partitioning in Mixed-phase Arctic Clouds. J. Geophys. Res. 116 (D17). doi:10.1029/2011JD015729
Eyring, V., Bony, S., Meehl, G. A., Senior, C. A., Stevens, B., Stouffer, R. J., et al. (2016). Overview of the Coupled Model Intercomparison Project Phase 6 (CMIP6) Experimental Design and Organization. Geosci. Model. Dev. 9, 1937–1958. doi:10.5194/gmd-9-1937-2016
Fan, J., Wang, Y., Rosenfeld, D., and Liu, X. (2016). Review of Aerosol-Cloud Interactions: Mechanisms, Significance, and Challenges. J. Atmos. Sci. 73 (11), 4221–4252. doi:10.1175/JAS-D-16-0037.1
Feldl, N., Anderson, B. T., and Bordoni, S. (2017b). Atmospheric Eddies Mediate Lapse Rate Feedback and Arctic Amplification. J. Clim. 30 (22), 9213–9224. doi:10.1175/JCLI-D-16-0706.1
Feldl, N., Bordoni, S., and Merlis, T. M. (2017a). Coupled High-Latitude Climate Feedbacks and Their Impact on Atmospheric Heat Transport. J. Clim. 30 (1), 189–201. doi:10.1175/JCLI-D-16-0324.1
Feldl, N., and Merlis, T. M. (2021). Polar Amplification in Idealized Climates: The Role of Ice, Moisture, and Seasons. Geophys. Res. Lett. 48, e2021GL094130. doi:10.1029/2021GL094130
Feldl, N., Po-Chedley, S., Singh, H. K. A., Hay, S., and Kushner, P. J. (2020). Sea Ice and Atmospheric Circulation Shape the High-Latitude Lapse Rate Feedback. Npj Clim. Atmos. Sci. 3 (1), 1–9. doi:10.1038/s41612-020-00146-7
Feldl, N., and Roe, G. H. (2013). Four Perspectives on Climate Feedbacks. Geophys. Res. Lett. 40 (15), 4007–4011. doi:10.1002/grl.50711
Flannery, B. P. (1984). Energy Balance Models Incorporating Transport of Thermal and Latent Energy. J. Atmos. Sci. 41 (3), 414–421. https://journals.ametsoc.org/view/journals/atsc/41/3/1520-0469_1984_041_0414_ebmito_2_0_co_2.xml.
Furtado, K., and Field, P. (2017). The Role of Ice Microphysics Parametrizations in Determining the Prevalence of Supercooled Liquid Water in High-Resolution Simulations of a Southern Ocean Midlatitude Cyclone. J. Atmos. Sci. 74 (6), 2001–2021. doi:10.1175/JAS-D-16-0165.1
GISTEMP Team (2021). GISS Surface Temperature Analysis (GISTEMP), Version 4. New York, NY: NASA Goddard Institute for Space Studies. Available at: https://data.giss.nasa.gov/gistemp/ (Accessed 26 April, 2021).
Goosse, H., Kay, J. E., Armour, K. C., Bodas-Salcedo, A., Chepfer, H., Docquier, D., et al. (2018). Quantifying Climate Feedbacks in Polar Regions. Nat. Commun. 9 (1), 1919. doi:10.1038/s41467-018-04173-0
Goosse, H., Selten, F., Haarsma, R., and Opsteegh, J. (2003). Large Sea-Ice Volume Anomalies Simulated in a Coupled Climate Model. Clim. Dyn. 20 (5), 523–536. doi:10.1007/s00382-002-0290-4
Graham, R. M., Cohen, L., Petty, A. A., Boisvert, L. N., Rinke, A., Hudson, S. R., et al. (2017a). Increasing Frequency and Duration of Arctic winter Warming Events. Geophys. Res. Lett. 44 (13), 6974–6983. doi:10.1002/2017GL073395
Graham, R. M., Cohen, L., Petty, A. A., Boisvert, L. N., Rinke, A., Hudson, S. R., et al. (2017b). Increasing Frequency and Duration of Arctic winter Warming Events. Geophys. Res. Lett. 44 (13), 6974–6983. doi:10.1002/2017GL073395
Graversen, R. G., and Burtu, M. (2016). Arctic Amplification Enhanced by Latent Energy Transport of Atmospheric Planetary Waves. Q.J.R. Meteorol. Soc. 142 (698), 2046–2054. doi:10.1002/qj.2802
Graversen, R. G., Langen, P. L., and Mauritsen, T. (2014). Polar Amplification in CCSM4: Contributions from the Lapse Rate and Surface Albedo Feedbacks. J. Clim. 27 (12), 4433–4450. doi:10.1175/JCLI-D-13-00551.1
Graversen, R. G., and Langen, P. L. (2019). On the Role of the Atmospheric Energy Transport in 2 × CO2-Induced Polar Amplification in CESM1. J. Clim. 32 (13), 3941–3956. doi:10.1175/JCLI-D-18-0546.1
Graversen, R. G., Mauritsen, T., Tjernström, M., Källén, E., and Svensson, G. (2008). Vertical Structure of Recent Arctic Warming. Nature 451 (7174), 53–56. doi:10.1038/nature06502
Graversen, R. G., and Wang, M. (2009). Polar Amplification in a Coupled Climate Model with Locked Albedo. Clim. Dyn. 33 (5), 629–643. doi:10.1007/s00382-009-0535-6
Guemas, V., and Salas-Mélia, D. (2008). Simulation of the Atlantic Meridional Overturning Circulation in an Atmosphere-Ocean Global Coupled Model. Part II: Weakening in a Climate Change experiment: a Feedback Mechanism. Clim. Dyn. 30 (7), 831–844. doi:10.1007/s00382-007-0328-8
Hahn, L. C., Armour, K. C., Zelinka, M. D., Bitz, C. M., and Donohoe, A. (2021). Contributions to Polar Amplification in CMIP5 and CMIP6 Models. Front. Earth Sci. 9, 710036. doi:10.3389/feart.2021.710036
Hall, A., Cox, P., Huntingford, C., and Klein, S. (2019). Progressing Emergent Constraints on Future Climate Change. Nat. Clim. Chang. 9 (4), 269–278. doi:10.1038/s41558-019-0436-6
Hall, A., and Qu, X. (2006). Using the Current Seasonal Cycle to Constrain Snow Albedo Feedback in Future Climate Change. Geophys. Res. Lett. 33 (3). doi:10.1029/2005GL025127
Hall, A. (2004). The Role of Surface Albedo Feedback in Climate. J. Clim. 17 (7), 1550–1568. doi:10.1175/1520-0442(2004)017<1550:trosaf>2.0.co;2
Hansen, J., Lacis, A., Rind, D., Russell, G., Stone, P., Fung, I., et al. (1984). Climate Sensitivity: Analysis of Feedback Mechanisms. Feedback 1, 1–3. doi:10.1029/gm029p0130
Hartmann, M., Blunier, T., Brügger, S. O., Schmale, J., Schwikowski, M., Vogel, A., et al. (2019). Variation of Ice Nucleating Particles in the European Arctic over the Last Centuries. Geophys. Res. Lett. 46 (7), 4007–4016. doi:10.1029/2019GL082311
He, M., Hu, Y., Chen, N., Wang, D., Huang, J., and Stamnes, K. (2019). High Cloud Coverage over Melted Areas Dominates the Impact of Clouds on the Albedo Feedback in the Arctic. Sci. Rep. 9 (1), 9529. doi:10.1038/s41598-019-44155-w
Hegyi, B. M., and Deng, Y. (2017). Dynamical and Thermodynamical Impacts of High- and Low-Frequency Atmospheric Eddies on the Initial Melt of Arctic Sea Ice. J. Clim. 30 (3), 865–883. doi:10.1175/JCLI-D-15-0366.1
Hegyi, B. M., and Taylor, P. C. (2018). The Unprecedented 2016-2017 Arctic Sea Ice Growth Season: The Crucial Role of Atmospheric Rivers and Longwave Fluxes. Geophys. Res. Lett. 45 (10), 5204–5212. doi:10.1029/2017GL076717
Held, I. M. (2005). The Gap between Simulation and Understanding in Climate Modeling. Bull. Amer. Meteorol. Soc. 86 (11), 1609–1614. doi:10.1175/BAMS-86-11-1609
Henry, M., Merlis, T. M., Lutsko, N. J., and Rose, B. E. J. (2021). Decomposing the Drivers of Polar Amplification with a Single-Column Model. J. Clim. 34 (6), 2355–2365. doi:10.1175/JCLI-D-20-0178.1
Henry, M., and Merlis, T. M. (2019). The Role of the Nonlinearity of the Stefan-Boltzmann Law on the Structure of Radiatively Forced Temperature Change. J. Clim. 32 (2), 335–348. doi:10.1175/JCLI-D-17-0603.1
Henry, M., and Vallis, G. K. (2021). Reduced High-Latitude Land Seasonality in Climates with Very High Carbon Dioxide. J. Clim. 1 (aop), 1–38. doi:10.1175/JCLI-D-21-0131.1
Hersbach, H., Bell, B., Berrisford, P., Biavati, G., Horányi, A., Muñoz Sabater, J., et al. (2019). ERA5 Monthly Averaged Data on Pressure Levels from 1979 to Present. Copernicus Climate Change Service (C3S) Climate Data Store (CDS). Available at: https://cds.climate.copernicus.eu/cdsapp#!/dataset/reanalysis-era5-pressure-levels-monthly-means (Accessed March 3, 2021).
Hibler, W. D. (1979). A Dynamic Thermodynamic Sea Ice Model. J. Phys. Oceanogr. 9 (4), 815–846. doi:10.1175/1520-0485(1979)009<0815:adtsim>2.0.co;2
Hind, A., Zhang, Q., and Brattström, G. (2016). Problems Encountered when Defining Arctic Amplification as a Ratio. Sci. Rep. 6 (1), 30469. doi:10.1038/srep30469
Holland, M. M., Bailey, D. A., Briegleb, B. P., Light, B., and Hunke, E. (2012). Improved Sea Ice Shortwave Radiation Physics in CCSM4: The Impact of Melt Ponds and Aerosols on Arctic Sea Ice. J. Clim. 25 (5), 1413–1430. doi:10.1175/JCLI-D-11-00078.1
Holland, M. M., and Bitz, C. M. (2003). Polar Amplification of Climate Change in Coupled Models. Clim. Dyn. 21, 221–232. doi:10.1007/s00382-003-0332-6
Holland, M. M., Serreze, M. C., and Stroeve, J. (2010). The Sea Ice Mass Budget of the Arctic and its Future Change as Simulated by Coupled Climate Models. Clim. Dyn. 34 (2–3), 185–200. doi:10.1007/s00382-008-0493-4
Hu, X., Fan, H., Cai, M., Sejas, S. A., Taylor, P., and Yang, S. (2020). A Less Cloudy Picture of the Inter-model Spread in Future Global Warming Projections. Nat. Commun. 11 (1), 4472. doi:10.1038/s41467-020-18227-9
Hu, X., Taylor, P. C., Cai, M., Yang, S., Deng, Y., and Sejas, S. (2017). Inter-Model Warming Projection Spread: Inherited Traits from Control Climate Diversity. Sci. Rep. 7 (1), 4300. doi:10.1038/s41598-017-04623-7
Huang, Y., Ding, Q., Dong, X., Xi, B., and Baxter, I. (2021b). Summertime Low Clouds Mediate the Impact of the Large-Scale Circulation on Arctic Sea Ice. Commun. Earth Environ. 2 (1), 1–10. doi:10.1038/s43247-021-00114-w
Huang, Y., Dong, X., Bailey, D. A., Holland, M. M., Xi, B., DuVivier, A. K., et al. (2019a). Thicker Clouds and Accelerated Arctic Sea Ice Decline: The Atmosphere‐Sea Ice Interactions in Spring. Geophys. Res. Lett. 46 (12), 6980–6989. doi:10.1029/2019GL082791
Huang, Y., Dong, X., Xi, B., and Deng, Y. (2019b). A Survey of the Atmospheric Physical Processes Key to the Onset of Arctic Sea Ice Melt in spring. Clim. Dyn. 52 (7), 4907–4922. doi:10.1007/s00382-018-4422-x
Huang, Y., Dong, X., Xi, B., Dolinar, E. K., and Stanfield, R. E. (2017b). The Footprints of 16 Year Trends of Arctic Springtime Cloud and Radiation Properties on September Sea Ice Retreat. J. Geophys. Res. Atmos. 122 (4), 2179–2193. doi:10.1002/2016JD026020
Huang, Y., Huang, H., and Shakirova, A. (2021a). The Nonlinear Radiative Feedback Effects in the Arctic Warming. Front. Earth Sci. 9, 693779. doi:10.3389/feart.2021.693779
Huang, Y., Xia, Y., and Tan, X. (2017a). On the Pattern of CO2 Radiative Forcing and Poleward Energy Transport. J. Geophys. Res. Atmos. 122 (2010), 10,578–10,593. doi:10.1002/2017JD027221
Hwang, Y.-T., Frierson, D. M. W., and Kay, J. E. (2011). Coupling between Arctic Feedbacks and Changes in Poleward Energy Transport. Geophys. Res. Lett. 38 (17), a–n. doi:10.1029/2011GL048546
Ingram, W. J., Wilson, C. A., and Mitchell, J. F. B. (1989). Modeling Climate Change: An Assessment of Sea Ice and Surface Albedo Feedbacks. J. Geophys. Res. 94 (D6), 8609–8622. doi:10.1029/JD094iD06p08609
Irish, V. E., Elizondo, P., Chen, J., Chou, C., Charette, J., Lizotte, M., et al. (2017). Ice-nucleating Particles in Canadian Arctic Sea-Surface Microlayer and Bulk Seawater. Atmos. Chem. Phys. 17 (17), 10583–10595. doi:10.5194/acp-17-10583-2017
Jahn, A., Sterling, K., Holland, M. M., Kay, J. E., Maslanik, J. A., Bitz, C. M., et al. (2012). Late-Twentieth-Century Simulation of Arctic Sea Ice and Ocean Properties in the CCSM4. J. Clim. 25 (5), 1431–1452. doi:10.1175/JCLI-D-11-00201.1
Jeevanjee, N., Hassanzadeh, P., Hill, S., and Sheshadri, A. (2017). A Perspective on Climate Model Hierarchies. J. Adv. Model. Earth Syst. 9 (4), 1760–1771. doi:10.1002/2017MS001038
Jungclaus, J. H., Lohmann, K., and Zanchettin, D. (2014). Enhanced 20th-century Heat Transfer to the Arctic Simulated in the Context of Climate Variations over the Last Millennium. Clim. Past 10 (6), 2201–2213. doi:10.5194/cp-10-2201-2014
Karcher, M. J., Gerdes, R., Kauker, F., and Köberle, C. (2003). Arctic Warming: Evolution and Spreading of the 1990s Warm Event in the Nordic Seas and the Arctic Ocean. J. Geophys. Res. 108 (C2), a–n. doi:10.1029/2001JC001265
Kato, S., Rose, F. G., Rutan, D. A., Thorsen, T. J., Loeb, N. G., Doelling, D. R., et al. (2018). Surface Irradiances of Edition 4.0 Clouds and the Earth's Radiant Energy System (CERES) Energy Balanced and Filled (EBAF) Data Product. J. Clim. 31 (11), 4501–4527. doi:10.1175/jcli-d-17-0523.1
Kay, J. E., Deser, C., Phillips, A., Mai, A., Hannay, C., Strand, G., et al. (2015). The Community Earth System Model (CESM) Large Ensemble Project: A Community Resource for Studying Climate Change in the Presence of Internal Climate Variability. Bull. Am. Meteorol. Soc. 96 (8), 1333–1349. doi:10.1175/BAMS-D-13-00255.1
Kay, J. E., and Gettelman, A. (2009). Cloud Influence on and Response to Seasonal Arctic Sea Ice Loss. J. Geophys. Res. 114 (D18), 1. doi:10.1029/2009JD011773
Kay, J. E., Holland, M. M., Bitz, C. M., Blanchard-Wrigglesworth, E., Gettelman, A., Conley, A., et al. (2012). The Influence of Local Feedbacks and Northward Heat Transport on the Equilibrium Arctic Climate Response to Increased Greenhouse Gas Forcing. J. Clim. 25 (16), 5433–5450. doi:10.1175/JCLI-D-11-00622.1
Kay, J. E., Holland, M. M., and Jahn, A. (2011). Inter-annual to Multi-Decadal Arctic Sea Ice Extent Trends in a Warming World. Geophys. Res. Lett. 38 (15), 1. doi:10.1029/2011GL048008
Kim, D., Kang, S. M., Shin, Y., and Feldl, N. (2018). Sensitivity of Polar Amplification to Varying Insolation Conditions. J. Clim. 31 (12), 4933–4947. doi:10.1175/JCLI-D-17-0627.1
Kim, K.-Y., Hamlington, B. D., Na, H., and Kim, J. (2016). Mechanism of Seasonal Arctic Sea Ice Evolution and Arctic Amplification. The Cryosphere 10 (5), 2191–2202. doi:10.5194/tc-10-2191-2016
Kim, K.-Y., Kim, J.-Y., Kim, J., Yeo, S., Na, H., Hamlington, B. D., et al. (2019). Vertical Feedback Mechanism of Winter Arctic Amplification and Sea Ice Loss. Sci. Rep. 9 (1), 1184. doi:10.1038/s41598-018-38109-x
Klein, S. A., McCoy, R. B., Morrison, H., Ackerman, A. S., Avramov, A., Boer, G. d., et al. (2009). Intercomparison of Model Simulations of Mixed-phase Clouds Observed during the ARM Mixed-phase Arctic Cloud Experiment. I: Single-Layer Cloud. Q.J.R. Meteorol. Soc. 135 (641), 979–1002. doi:10.1002/qj.416
Koenigk, T., and Brodeau, L. (2014). Ocean Heat Transport into the Arctic in the Twentieth and Twenty-First century in EC-Earth. Clim. Dyn. 42 (11), 3101–3120. doi:10.1007/s00382-013-1821-x
Komurcu, M., Storelvmo, T., Tan, I., Lohmann, U., Yun, Y., Penner, J. E., et al. (2014). Intercomparison of the Cloud Water Phase Among Global Climate Models. J. Geophys. Res. Atmos. 119 (6), 3372–3400. doi:10.1002/2013JD021119
Kwok, R. (2018). Arctic Sea Ice Thickness, Volume, and Multiyear Ice Coverage: Losses and Coupled Variability (1958-2018). Environ. Res. Lett. 13 (10), 105005. doi:10.1088/1748-9326/aae3ec
Laîné, A., Yoshimori, M., and Abe-Ouchi, A. (2016). Surface Arctic Amplification Factors in CMIP5 Models: Land and Oceanic Surfaces and Seasonality. J. Clim. 29 (9), 3297–3316. doi:10.1175/JCLI-D-15-0497.1
Langen, P. L., and Alexeev, V. A. (2007). Polar Amplification as a Preferred Response in an Idealized Aquaplanet GCM. Clim. Dyn. 29, 305–317. doi:10.1007/S00382-006-0221-X
Lawson, P., Gurganus, C., Woods, S., and Bruintjes, R. (2017). Aircraft Observations of Cumulus Microphysics Ranging from the Tropics to Midlatitudes: Implications for a “New” Secondary Ice Process. J. Atmos. Sci. 74 (9), 2899–2920. doi:10.1175/JAS-D-17-0033.1
Laxon, S., Peacock, N., and Smith, D. (2003). High Interannual Variability of Sea Ice Thickness in the Arctic Region. Nature 425 (6961), 947–950. doi:10.1038/nature02050
Lee, S. (2014). A Theory for Polar Amplification from a General Circulation Perspective. Asia-pacific J. Atmos. Sci. 50, 31–43. doi:10.1007/s13143-014-0024-7
Lee, S., Gong, T., Feldstein, S. B., Screen, J. A., and Simmonds, I. (2017). Revisiting the Cause of the 1989-2009 Arctic Surface Warming Using the Surface Energy Budget: Downward Infrared Radiation Dominates the Surface Fluxes. Geophys. Res. Lett. 44, 10654–10661. doi:10.1002/2017GL075375
Lee, S., Gong, T., Johnson, N., Feldstein, S. B., and Pollard, D. (2011). On the Possible Link between Tropical Convection and the Northern Hemisphere Arctic Surface Air Temperature Change between 1958 and 2001. J. Clim. 24 (16), 4350–4367. doi:10.1175/2011JCLI4003.1
Lee, S. (2012). Testing of the Tropically Excited Arctic Warming Mechanism (TEAM) with Traditional El Niño and La Niña. J. Clim. 25, 4015–4022. doi:10.1175/JCLI-D-12-00055.1
Lenssen, N. J. L., Schmidt, G. A., Hansen, J. E., Menne, M. J., Persin, A., Ruedy, R., et al. (2019). Improvements in the GISTEMP Uncertainty Model. J. Geophys. Res. Atmos. 124 (12), 6307–6326. doi:10.1029/2018JD029522
Letterly, A., Key, J., and Liu, Y. (2018). Arctic Climate: Changes in Sea Ice Extent Outweigh Changes in Snow Cover. The Cryosphere 12, 3373–3382. doi:10.5194/tc-12-3373-2018
Li, H., Fedorov, A., and Liu, W. (2021). AMOC Stability and Diverging Response to Arctic Sea Ice Decline in Two Climate Models. J. Clim. 34 (13), 1–47. doi:10.1175/JCLI-D-20-0572.1
Li, X., Krueger, S. K., Strong, C., Mace, G. G., and Benson, S. (2020). Midwinter Arctic Leads Form and Dissipate Low Clouds. Nat. Commun. 11 (1), 206. doi:10.1038/s41467-019-14074-5
Li, Z.-X., and Le Treut, H. (1992). Cloud-radiation Feedbacks in a General Circulation Model and Their Dependence on Cloud Modelling Assumptions. Clim. Dyn. 7 (3), 133–139. doi:10.1007/BF00211155
Liu, W., Fedorov, A., and Sévellec, F. (2019). The Mechanisms of the Atlantic Meridional Overturning Circulation Slowdown Induced by Arctic Sea Ice Decline. J. Clim. 32 (4), 977–996. doi:10.1175/JCLI-D-18-0231.1
Liu, W., and Fedorov, A. V. (2019). Global Impacts of Arctic Sea Ice Loss Mediated by the Atlantic Meridional Overturning Circulation. Geophys. Res. Lett. 46 (2), 944–952. doi:10.1029/2018GL080602
Liu, W., and Fedorov, A. (2021). Interaction Between Arctic Sea Ice and the Atlantic Meridional Overturning Circulation in a Warming Climate. Clim. Dyn. doi:10.1007/s00382-021-05993-5
Liu, Y., Key, J. R., Liu, Z., Wang, X., and Vavrus, S. J. (2012). A Cloudier Arctic Expected with Diminishing Sea Ice. Geophys. Res. Lett. 39 (5), a–n. doi:10.1029/2012GL051251
Loeb, N. G., Doelling, D. R., Wang, H., Su, W., Nguyen, C., Corbett, J. G., et al. (2018). Clouds and the Earth's Radiant Energy System (CERES) Energy Balanced and Filled (EBAF) Top-Of-Atmosphere (TOA) Edition-4.0 Data Product. J. Clim. 31 (2), 895–918. doi:10.1175/jcli-d-17-0208.1
Lu, J., and Cai, M. (2009b). A New Framework for Isolating Individual Feedback Processes in Coupled General Circulation Climate Models. Part I: Formulation. Clim. Dyn. 32 (6), 873–885. doi:10.1007/s00382-008-0425-3
Lu, J., and Cai, M. (2009a). Seasonality of Polar Surface Warming Amplification in Climate Simulations. Geophys. Res. Lett. 36 (16). doi:10.1029/2009GL040133
Maher, P., Gerber, E. P., Medeiros, B., Merlis, T. M., Sherwood, S., Sheshadri, A., et al. (2019). Model Hierarchies for Understanding Atmospheric Circulation. Rev. Geophys. 57 (2), 250–280. doi:10.1029/2018RG000607
Mahlstein, I., and Knutti, R. (2011). Ocean Heat Transport as a Cause for Model Uncertainty in Projected Arctic Warming. J. Clim. 24 (5), 1451–1460. doi:10.1175/2010JCLI3713.1
Manabe, S., Spelman, M. J., and Stouffer, R. J. (1992). Transient Responses of a Coupled Ocean-Atmosphere Model to Gradual Changes of Atmospheric CO2. Part II: Seasonal Response. J. Clim. 5 (2), 105–126. doi:10.1175/1520-0442(1992)005<0105:TROACO>2.0.CO;2
Manabe, S., and Stouffer, R. J. (1980). Sensitivity of a Global Climate Model to an Increase of CO2concentration in the Atmosphere. J. Geophys. Res. 85 (C10), 5529–5554. doi:10.1029/JC085iC10p05529
Manabe, S., Stouffer, R. J., Spelman, M. J., and Bryan, K. (1991). Transient Responses of a Coupled Ocean-Atmosphere Model to Gradual Changes of Atmospheric CO2. Part I. Annual Mean Response. J. Clim. 4 (8), 785–818. doi:10.1175/1520-0442(1991)004<0785:TROACO>2.0.CO;2
Manabe, S., and Wetherald, R. T. (1975). The Effects of Doubling the CO2Concentration on the Climate of a General Circulation Model. J. Atmos. Sci. 32 (1), 3–15. doi:10.1175/1520-0469(1975)032<0003:TEODTC>2.0.CO;2
Manabe, S., and Wetherald, R. T. (1967). Thermal Equilibrium of the Atmosphere with a Given Distribution of Relative Humidity. J. Atmos. Sci. 24 (3), 241–259. doi:10.1175/1520-0469(1967)024<0241:teotaw>2.0.co;2
Markus, T., Stroeve, J. C., and Miller, J. (2009). Recent Changes in Arctic Sea Ice Melt Onset, Freezeup, and Melt Season Length. J. Geophys. Res. Oceans 114 (C12). doi:10.1029/2009jc005436
Marshall, J., Scott, J. R., Armour, K. C., Campin, J.-M., Kelley, M., and Romanou, A. (2015). The Ocean's Role in the Transient Response of Climate to Abrupt Greenhouse Gas Forcing. Clim. Dyn. 44 (7), 2287–2299. doi:10.1007/s00382-014-2308-0
Maslanik, J., Stroeve, J., Fowler, C., and Emery, W. (2011). Distribution and Trends in Arctic Sea Ice Age through spring 2011. Geophys. Res. Lett. 38 (13), a–n. doi:10.1029/2011GL047735
McCoy, D. T., Tan, I., Hartmann, D. L., Zelinka, M. D., and Storelvmo, T. (2016). On the Relationships Among Cloud Cover, Mixed‐phase Partitioning, and Planetary Albedo in GCMs. J. Adv. Model. Earth Syst. 8 (2), 650–668. doi:10.1002/2015MS000589
McCusker, K. E., Kushner, P. J., Fyfe, J. C., Sigmond, M., Kharin, V. V., and Bitz, C. M. (2017). Remarkable Separability of Circulation Response to Arctic Sea Ice Loss and Greenhouse Gas Forcing. Geophys. Res. Lett. 44 (15), 7955–7964. doi:10.1002/2017GL074327
Meehl, G. A., Collins, W. D., Boville, B. A., Kiehl, J. T., Wigley, T. M. L., and Arblaster, J. M. (2000). Response of the NCAR Climate System Model to Increased CO2and the Role of Physical Processes. J. Clim. 13 (11), 1879–1898. doi:10.1175/1520-0442(2000)013<1879:rotncs>2.0.co;2
Meredith, M., Sommerkorn, M., Cassotta, S., Derksen, C., Ekaykin, A., Hollowed, A., et al. (2019). “Polar Regions,” in IPCC Special Report on the Ocean and Cryosphere in a Changing Climate. Editors H.-O. Pörtner, D. C. Roberts, V. Masson-Delmotte, P. Zhai, M. Tignor, E. Poloczanskaet al. (Geneva, Switzerland: Intergovernmental Panel on Climate Change). In press.
Merlis, T. M., and Henry, M. (2018). Simple Estimates of Polar Amplification in Moist Diffusive Energy Balance Models. J. Clim. 31 (15), 5811–5824. doi:10.1175/JCLI-D-17-0578.1
Merlis, T. M. (2014). Interacting Components of the Top-Of-Atmosphere Energy Balance Affect Changes in Regional Surface Temperature. Geophys. Res. Lett. 41 (20), 7291–7297. doi:10.1002/2014GL061700
Middlemas, E. A., Kay, J. E., Medeiros, B. M., and Maroon, E. A. (2020). Quantifying the Influence of Cloud Radiative Feedbacks on Arctic Surface Warming Using Cloud Locking in an Earth System Model. Geophys. Res. Lett. 47 (15), e2020GL089207. doi:10.1029/2020GL089207
Miller, G. H., Alley, R. B., Brigham-Grette, J., Fitzpatrick, J. J., Polyak, L., Serreze, M. C., et al. (2010). Arctic Amplification: Can the Past Constrain the Future? Quat. Sci. Rev. 29, 1779–1790. doi:10.1016/j.quascirev.2010.02.008
Mitchell, J. F. B., Senior, C. A., and Ingram, W. J. (1989). C02 and Climate: a Missing Feedback? Nature 341 (6238), 132–134. doi:10.1038/341132a0
Morrison, A. L., Kay, J. E., Chepfer, H., Guzman, R., and Yettella, V. (2018). Isolating the Liquid Cloud Response to Recent Arctic Sea Ice Variability Using Spaceborne Lidar Observations. J. Geophys. Res. Atmos. 123 (1), 473–490. doi:10.1002/2017JD027248
Morrison, A. L., Kay, J. E., Frey, W. R., Chepfer, H., and Guzman, R. (2019). Cloud Response to Arctic Sea Ice Loss and Implications for Future Feedback in the CESM1 Climate Model. J. Geophys. Res. Atmos. 124 (2), 1003–1020. doi:10.1029/2018JD029142
North, G. R. (2007). Theory of Energy-Balance Climate Models. J. Atmos. Sci. 32 (11), 2033–2043. https://journals.ametsoc.org/view/journals/atsc/32/11/1520-0469_1975_032_2033_toebcm_2_0_co_2.xml.
Nghiem, S. V., Rigor, I. G., Perovich, D. K., Clemente-Colón, P., Weatherly, J. W., and Neumann, G. (2007). Rapid Reduction of Arctic Perennial Sea Ice. Geophys. Res. Lett. 34 (19). doi:10.1029/2007GL031138
Nummelin, A., Li, C., and Hezel, P. J. (2017). Connecting Ocean Heat Transport Changes from the Midlatitudes to the Arctic Ocean. Geophys. Res. Lett. 44 (4), 1899–1908. doi:10.1002/2016GL071333
Nygård, T., Graversen, R. G., Uotila, P., Naakka, T., and Vihma, T. (2019). Strong Dependence of Wintertime Arctic Moisture and Cloud Distributions on Atmospheric Large-Scale Circulation. J. Clim. 32 (24), 8771–8790. doi:10.1175/JCLI-D-19-0242.1
Oldenburg, D., Armour, K. C., Thompson, L., and Bitz, C. M. (2018). Distinct Mechanisms of Ocean Heat Transport into the Arctic under Internal Variability and Climate Change. Geophys. Res. Lett. 45 (15), 7692–7700. doi:10.1029/2018GL078719
Oudar, T., Sanchez-Gomez, E., Chauvin, F., Cattiaux, J., Terray, L., and Cassou, C. (2017). Respective Roles of Direct GHG Radiative Forcing and Induced Arctic Sea Ice Loss on the Northern Hemisphere Atmospheric Circulation. Clim. Dyn. 49 (11), 3693–3713. doi:10.1007/s00382-017-3541-0
Park, H.-S., Kim, S.-J., Seo, K.-H., Stewart, A. L., Kim, S.-Y., and Son, S.-W. (2018). The Impact of Arctic Sea Ice Loss on Mid-holocene Climate. Nat. Commun. 9 (1), 4571. doi:10.1038/s41467-018-07068-2
Parkinson, C. L., and DiGirolamo, N. E. (2016). New Visualizations Highlight New Information on the Contrasting Arctic and Antarctic Sea-Ice Trends since the Late 1970s. Remote Sensing Environ. 183, 198–204. doi:10.1016/j.rse.2016.05.020
Pavelsky, T. M., Boé, J., Hall, A., and Fetzer, E. J. (2011). Atmospheric Inversion Strength over Polar Oceans in winter Regulated by Sea Ice. Clim. Dyn. 36 (5), 945–955. doi:10.1007/s00382-010-0756-8
Payne, A. E., Jansen, M. F., and Cronin, T. W. (2015). Conceptual Model Analysis of the Influence of Temperature Feedbacks on Polar Amplification. Geophys. Res. Lett. 42 (21), 9561–9570. doi:10.1002/2015GL065889
Pendergrass, A. G., Conley, A., and Vitt, F. M. (2018). Surface and Top-Of-Atmosphere Radiative Feedback Kernels for CESM-CAM5. Earth Syst. Sci. Data 10 (1), 317–324. doi:10.5194/essd-10-317-2018
Perovich, D. K., Light, B., Eicken, H., Jones, K. F., Runciman, K., and Nghiem, S. V. (2007). Increasing Solar Heating of the Arctic Ocean and Adjacent Seas, 1979-2005: Attribution and Role in the Ice-Albedo Feedback. Geophys. Res. Lett. 34 (19). doi:10.1029/2007GL031480
Persson, P. O. G., Shupe, M. D., Perovich, D., and Solomon, A. (2017). Linking Atmospheric Synoptic Transport, Cloud Phase, Surface Energy Fluxes, and Sea-Ice Growth: Observations of Midwinter SHEBA Conditions. Clim. Dyn. 49 (4), 1341–1364. doi:10.1007/s00382-016-3383-1
Pistone, K., Eisenman, I., and Ramanathan, V. (2014). Observational Determination of Albedo Decrease Caused by Vanishing Arctic Sea Ice. Proc. Natl. Acad. Sci. USA 111 (9), 3322–3326. doi:10.1073/pnas.1318201111
Pithan, F., and Mauritsen, T. (2014). Arctic Amplification Dominated by Temperature Feedbacks in Contemporary Climate Models. Nat. Geosci 7 (3), 181–184. doi:10.1038/ngeo2071
Pithan, F., Medeiros, B., and Mauritsen, T. (2014). Mixed-phase Clouds Cause Climate Model Biases in Arctic Wintertime Temperature Inversions. Clim. Dyn. 43 (1), 289–303. doi:10.1007/s00382-013-1964-9
Pithan, F., Svensson, G., Caballero, R., Chechin, D., Cronin, T. W., Ekman, A. M. L., et al. (2018). Role of Air-Mass Transformations in Exchange between the Arctic and Mid-latitudes. Nat. Geosci 11 (11), 805–812. doi:10.1038/s41561-018-0234-1
Previdi, M., Janoski, T. P., Chiodo, G., Smith, K. L., and Polvani, L. M. (2020). Arctic Amplification: A Rapid Response to Radiative Forcing. Geophys. Res. Lett. 47 (17), e2020GL089933. doi:10.1029/2020GL089933
Rakipova, L. R. (1966). “The Influence of the Arctic Ice Cover on the Zonal Distribution of Atmospheric Temperature,” in Proceedings of the Symposium on the Arctic Heat Budget and Atmospheric Circulation (Springer).
Ramanathan, V. (1977). Interactions between Ice-Albedo, Lapse-Rate and Cloud-Top Feedbacks: An Analysis of the Nonlinear Response of a GCM Climate Model. J. Atmos. Sci. 34 (12), 1885–1897. doi:10.1175/1520-0469(1977)034<1885:ibialr>2.0.co;2
Rangno, A. L., and Hobbs, P. V. (2001). Ice Particles in Stratiform Clouds in the Arctic and Possible Mechanisms for the Production of High Ice Concentrations. J. Geophys. Res. 106 (D14), 15065–15075. doi:10.1029/2000JD900286
Riihelä, A., Manninen, T., and Laine, V. (2013). Observed Changes in the Albedo of the Arctic Sea-Ice Zone for the Period 1982-2009. Nat. Clim Change 3 (10), 895–898. doi:10.1038/nclimate1963
Rind, D., Healy, R., Parkinson, C., and Martinson, D. (1995). The Role of Sea Ice in 2×CO2Climate Model Sensitivity. Part I: The Total Influence of Sea Ice Thickness and Extent. J. Clim. 8 (3), 449–463. doi:10.1175/1520-0442(1995)008<0449:TROSII>2.0.CO;2
Robock, A. (1983). Ice and Snow Feedbacks and the Latitudinal and Seasonal Distribution of Climate Sensitivity. J. Atmos. Sci. 40, 986–997. doi:10.1175/1520-0469(1983)040<0986:iasfat>2.0.co;2
Rodgers, K. B. (2003). A Tropical Mechanism for Northern Hemisphere Deglaciation. Geochem. Geophys. Geosyst. 4 (5), 1046. doi:10.1029/2003gc000508
Roe, G. H., Feldl, N., Armour, K. C., Hwang, Y.-T., and Frierson, D. M. W. (2015). The Remote Impacts of Climate Feedbacks on Regional Climate Predictability. Nat. Geosci 8, 135–139. doi:10.1038/ngeo2346
Rogers, W. E., Thomson, J., Shen, H. H., Doble, M. J., Wadhams, P., and Cheng, S. (2016). Dissipation of Wind Waves by Pancake and Frazil Ice in the Autumn Beaufort Sea. J. Geophys. Res. Oceans 121 (11), 7991–8007. doi:10.1002/2016JC012251
Rose, B. E. J., Armour, K. C., Battisti, D. S., Feldl, N., and Koll, D. D. B. (2014). The Dependence of Transient Climate Sensitivity and Radiative Feedbacks on the Spatial Pattern of Ocean Heat Uptake. Geophys. Res. Lett. 41, 1071–1078. doi:10.1002/2013GL058955
Rugenstein, M. A. A., Winton, M., Stouffer, R. J., Griffies, S. M., and Hallberg, R. (2013). Northern High-Latitude Heat Budget Decomposition and Transient Warming. J. Clim. 26 (2), 609–621. doi:10.1175/JCLI-D-11-00695.1
Russotto, R. D., and Biasutti, M. (2020). Polar Amplification as an Inherent Response of a Circulating Atmosphere: Results from the TRACMIP Aquaplanets. Geophys. Res. Lett. 47, 12. doi:10.1029/2019GL086771
Salzmann, M. (2017). The Polar Amplification Asymmetry: Role of Antarctic Surface Height. Earth Syst. Dynam. 8 (2), 323–336. doi:10.5194/esd-8-323-2017
Schauer, U., Fahrbach, E., Osterhus, S., and Rohardt, G. (2004). Arctic Warming through the Fram Strait: Oceanic Heat Transport from 3 Years of Measurements. J. Geophys. Res. 109 (C6). doi:10.1029/2003JC001823
Schmale, J., Zieger, P., and Ekman, A. M. L. (2021). Aerosols in Current and Future Arctic Climate. Nat. Clim. Chang. 11 (2), 95–105. doi:10.1038/s41558-020-00969-5
Schneider, S. H. (1975). On the Carbon Dioxide-Climate Confusion. J. Atmos. Sci. 32 (11), 2060–2066. doi:10.1175/1520-0469(1975)032<2060:OTCDC>2.0.CO;2
Schweiger, A., Lindsay, R., Zhang, J., Steele, M., Stern, H., and Kwok, R. (2011). Uncertainty in Modeled Arctic Sea Ice Volume. J. Geophys. Res. 116 (C8), 1. doi:10.1029/2011JC007084
Screen, J. A., Deser, C., and Simmonds, I. (2012). Local and Remote Controls on Observed Arctic Warming. Geophys. Res. Lett. 39 (10), a–n. doi:10.1029/2012GL051598
Screen, J. A., Deser, C., Smith, D. M., Zhang, X., Blackport, R., Kushner, P. J., et al. (2018). Consistency and Discrepancy in the Atmospheric Response to Arctic Sea-Ice Loss across Climate Models. Nat. Geosci 11 (3), 155–163. doi:10.1038/s41561-018-0059-y
Screen, J. A., and Simmonds, I. (2010a). Increasing Fall-winter Energy Loss from the Arctic Ocean and its Role in Arctic Temperature Amplification. Geophys. Res. Lett. 37 (16), a–n. doi:10.1029/2010GL044136
Screen, J. A., and Simmonds, I. (2010b). The central Role of Diminishing Sea Ice in Recent Arctic Temperature Amplification. Nature 464 (7293), 1334–1337. doi:10.1038/nature09051
Sedlar, J., Shupe, M. D., and Tjernström, M. (2012). On the Relationship between Thermodynamic Structure and Cloud Top, and its Climate Significance in the Arctic. J. Clim. 25 (7), 2374–2393. doi:10.1175/JCLI-D-11-00186.1
Sejas, S. A., Cai, M., Hu, A., Meehl, G. A., Washington, W., and Taylor, P. C. (2014). Individual Feedback Contributions to the Seasonality of Surface Warming. J. Clim. 27 (14), 5653–5669. doi:10.1175/JCLI-D-13-00658.1
Sejas, S. A., and Cai, M. (2016). Isolating the Temperature Feedback Loop and its Effects on Surface Temperature. J. Atmos. Sci. 73 (8), 3287–3303. doi:10.1175/JAS-D-15-0287.1
Sellers, W. D. (1969). A Global Climatic Model Based on the Energy Balance of the Earth-Atmosphere System. J. Appl. Meteorol. 8 (3), 392–400. doi:10.1175/1520-0450(1969)008<0392:AGCMBO>2.0.CO;2
Semenov, V. A., Park, W., and Latif, M. (2009). Barents Sea Inflow Shutdown: A New Mechanism for Rapid Climate Changes. Geophys. Res. Lett. 36 (14). doi:10.1029/2009GL038911
Semmler, T., Pithan, F., and Jung, T. (2020). Quantifying Two-Way Influences between the Arctic and Mid-latitudes through Regionally Increased CO2 Concentrations in Coupled Climate Simulations. Clim. Dyn. 54 (7), 3307–3321. doi:10.1007/s00382-020-05171-z
Serreze, M. C., Barrett, A. P., Stroeve, J. C., Kindig, D. N., and Holland, M. M. (2009). The Emergence of Surface-Based Arctic Amplification. The Cryosphere 3 (1), 11–19. doi:10.5194/tc-3-11-2009
Serreze, M. C., and Barry, R. G. (2011). Processes and Impacts of Arctic Amplification: A Research Synthesis. Glob. Planet. Change 77 (1), 85–96. doi:10.1016/j.gloplacha.2011.03.004
Serreze, M. C., Schnell, R. C., and Kahl, J. D. (1992). Low-Level Temperature Inversions of the Eurasian Arctic and Comparisons with Soviet Drifting Station Data. J. Clim. 5 (6), 615–629. doi:10.1175/1520-0442(1992)005<0615:LLTIOT>2.0.CO;2
Sévellec, F., Fedorov, A. V., and Liu, W. (2017). Arctic Sea-Ice Decline Weakens the Atlantic Meridional Overturning Circulation. Nat. Clim Change 7 (8), 604–610. doi:10.1038/nclimate3353
Shaw, T. A., and Tan, Z. (2018). Testing Latitudinally Dependent Explanations of the Circulation Response to Increased CO 2 Using Aquaplanet Models. Geophys. Res. Lett. 45 (18), 9861–9869. doi:10.1029/2018GL078974
Shell, K. M., Kiehl, J. T., and Shields, C. A. (2008). Using the Radiative Kernel Technique to Calculate Climate Feedbacks in NCAR's Community Atmospheric Model. J. Clim. 21 (10), 2269–2282. doi:10.1175/2007JCLI2044.1
Shupe, M. D., Persson, P. O. G., Brooks, I. M., Tjernström, M., Sedlar, J., Mauritsen, T., et al. (2013). Cloud and Boundary Layer Interactions over the Arctic Sea Ice in Late Summer. Atmos. Chem. Phys. 13 (18), 9379–9399. doi:10.5194/acp-13-9379-2013
Shupe, M. D. (2020). The MOSAiC Expedition: A Year Drifting with the Arctic Sea Ice. Washington, DC USA: National Oceanic and Atmospheric Administration. doi:10.25923/9g3v-xh92
Singh, H. A., Rasch, P. J., and Rose, B. E. J. (2017). Increased Ocean Heat Convergence into the High Latitudes with CO2 Doubling Enhances Polar-Amplified Warming. Geophys. Res. Lett. 44 (2010), 10,583–10,591. doi:10.1002/2017GL074561
Skagseth, Ø., Furevik, T., Ingvaldsen, R., Loeng, H., Mork, K. A., Orvik, K. A., et al. (2008). “Volume and Heat Transports to the Arctic Ocean via the Norwegian and Barents Seas,” in Arctic–Subarctic Ocean Fluxes: Defining the Role of the Northern Seas in Climate. Editors R. R. Dickson, J. Meincke, and P. Rhines (Springer Netherlands), 45–64. doi:10.1007/978-1-4020-6774-7_3
Sledd, A., and L’Ecuyer, T. (2019). How Much Do Clouds Mask the Impacts of Arctic Sea Ice and Snow Cover Variations? Different Perspectives from Observations and Reanalyses. Atmosphere 10 (1), 12. doi:10.3390/atmos10010012
Smith, D. M., Dunstone, N. J., Scaife, A. A., Fiedler, E. K., Copsey, D., and Hardiman, S. C. (2017). Atmospheric Response to Arctic and Antarctic Sea Ice: The Importance of Ocean-Atmosphere Coupling and the Background State. J. Clim. 30 (12), 4547–4565. doi:10.1175/JCLI-D-16-0564.1
Smith, D. M., Screen, J. A., Deser, C., Cohen, J., Fyfe, J. C., García-Serrano, J., et al. (2019). The Polar Amplification Model Intercomparison Project (PAMIP) Contribution to CMIP6: Investigating the Causes and Consequences of Polar Amplification. Geosci. Model. Dev. 12 (3), 1139–1164. doi:10.5194/gmd-12-1139-2019
Smith, W., Revercomb, H., Weisz, E., Tobin, D., Knuteson, R., Taylor, J., et al. (2021). Hyperspectral Satellite Radiance Atmospheric Profile Information Content and its Dependence on Spectrometer Technology. IEEE J. Sel. Top. Appl. Earth Observations Remote Sensing 14, 4720–4736. doi:10.1109/JSTARS.2021.3073482
Soden, B. J., Broccoli, A. J., and Hemler, R. S. (2004). On the Use of Cloud Forcing to Estimate Cloud Feedback. J. Clim. 17 (19), 3661–3665. doi:10.1175/1520-0442(2004)017<3661:OTUOCF>2.0.CO;2
Soden, B. J., and Held, I. M. (2006). An Assessment of Climate Feedbacks in Coupled Ocean-Atmosphere Models. J. Clim. 19 (14), 3354–3360. doi:10.1175/JCLI3799.1
Solomon, A., de Boer, G., Creamean, J. M., McComiskey, A., Shupe, M. D., Maahn, M., et al. (2018). The Relative Impact of Cloud Condensation Nuclei and Ice Nucleating Particle Concentrations on Phase Partitioning in Arctic Mixed-phase Stratocumulus Clouds. Atmos. Chem. Phys. 18 (23), 17047–17059. doi:10.5194/acp-18-17047-2018
Solomon, A., Shupe, M. D., Persson, O., Morrison, H., Yamaguchi, T., Caldwell, P. M., et al. (2014). The Sensitivity of Springtime Arctic Mixed-phase Stratocumulus Clouds to Surface-Layer and Cloud-Top Inversion-Layer Moisture Sources. J. Atmos. Sci. 71 (2), 574–595. doi:10.1175/JAS-D-13-0179.1
Song, X., Zhang, G. J., and Cai, M. (2014). Quantifying Contributions of Climate Feedbacks to Tropospheric Warming in the NCAR CCSM3.0. Clim. Dyn. 42 (3), 901–917. doi:10.1007/s00382-013-1805-x
Sotiropoulou, G., Sullivan, S., Savre, J., Lloyd, G., Lachlan-Cope, T., Ekman, A. M. L., et al. (2020). The Impact of Secondary Ice Production on Arctic Stratocumulus. Atmos. Chem. Phys. 20 (3), 1301–1316. doi:10.5194/acp-20-1301-2020
Spelman, M. J., and Manabe, S. (1984). Influence of Oceanic Heat Transport upon the Sensitivity of a Model Climate. J. Geophys. Res. 89 (C1), 571–586. doi:10.1029/JC089iC01p00571
Spielhagen, R. F., Werner, K., Sørensen, S. A., Zamelczyk, K., Kandiano, E., Budeus, G., et al. (2011). Enhanced Modern Heat Transfer to the Arctic by Warm Atlantic Water. Science 331 (6016), 450–453. doi:10.1126/science.1197397
Stapf, J., Ehrlich, A., Jäkel, E., Lüpkes, C., and Wendisch, M. (2020). Reassessment of Shortwave Surface Cloud Radiative Forcing in the Arctic: Consideration of Surface-Albedo-Cloud Interactions. Atmos. Chem. Phys. 20 (16), 9895–9914. doi:10.5194/acp-20-9895-2020
Stramler, K., Del Genio, A. D., and Rossow, W. B. (2011). Synoptically Driven Arctic Winter States. J. Clim. 24 (6), 1747–1762. doi:10.1175/2010JCLI3817.1
Stroeve, J., Barrett, A., Serreze, M., and Schweiger, A. (2014). Using Records from Submarine, Aircraft and Satellites to Evaluate Climate Model Simulations of Arctic Sea Ice Thickness. The Cryosphere 8 (5), 1839–1854. doi:10.5194/tc-8-1839-2014
Stuecker, M. F., Bitz, C. M., Armour, K. C., Proistosescu, C., Kang, S. M., Xie, S.-P., et al. (2018). Polar Amplification Dominated by Local Forcing and Feedbacks. Nat. Clim Change 8 (12), 1076–1081. doi:10.1038/s41558-018-0339-y
Susskind, J., Blaisdell, J. M., and Iredell, L. (2014). Improved Methodology for Surface and Atmospheric Soundings, Error Estimates, and Quality Control Procedures: The Atmospheric Infrared Sounder Science Team Version-6 Retrieval Algorithm. J. Appl. Remote Sens 8 (1), 084994. doi:10.1117/1.JRS.8.084994
Swart, N. C., Fyfe, J. C., Hawkins, E., Kay, J. E., and Jahn, A. (2015). Influence of Internal Variability on Arctic Sea-Ice Trends. Nat. Clim Change 5 (2), 86–89. doi:10.1038/nclimate2483
Swart, N. (2017). Natural Causes of Arctic Sea-Ice Loss. Nat. Clim Change 7 (4), 239–241. doi:10.1038/nclimate3254
Tan, I., and Storelvmo, T. (2019). Evidence of Strong Contributions from Mixed‐Phase Clouds to Arctic Climate Change. Geophys. Res. Lett. 46 (5), 2894–2902. doi:10.1029/2018GL081871
Tan, I., and Storelvmo, T. (2016). Sensitivity Study on the Influence of Cloud Microphysical Parameters on Mixed-phase Cloud Thermodynamic Phase Partitioning in CAM5. J. Atmos. Sci. 73 (2), 709–728. doi:10.1175/JAS-D-15-0152.1
Tan, I., Storelvmo, T., and Zelinka, M. D. (2016). Observational Constraints on Mixed-phase Clouds Imply Higher Climate Sensitivity. Science 352 (6282), 224–227. doi:10.1126/science.aad5300
Taylor, K. E., Stouffer, R. J., and Meehl, G. A. (2012). An Overview of CMIP5 and the Experiment Design. Bull. Am. Meteorol. Soc. 93 (4), 485–498. doi:10.1175/BAMS-D-11-00094.1
Taylor, P. C., Cai, M., Hu, A., Meehl, J., Washington, W., and Zhang, G. J. (2013). A Decomposition of Feedback Contributions to Polar Warming Amplification. J. Clim. 26 (18), 7023–7043. doi:10.1175/JCLI-D-12-00696.1
Taylor, P. C., Ellingson, R. G., and Cai, M. (2011a). Geographical Distribution of Climate Feedbacks in the NCAR CCSM3.0. J. Clim. 24 (11), 2737–2753. doi:10.1175/2010JCLI3788.1
Taylor, P. C., Ellingson, R. G., and Cai, M. (2011b). Seasonal Variations of Climate Feedbacks in the NCAR CCSM3. J. Clim. 24 (13), 3433–3444. doi:10.1175/2011jcli3862.1
Taylor, P. C., Kato, S., Xu, K. M., and Cai, M. (2015). Covariance between Arctic Sea Ice and Clouds within Atmospheric State Regimes at the Satellite Footprint Level. J. Geophys. Res. Atmos. 120 (24), 12656–12678. doi:10.1002/2015JD023520
Taylor, P. C., Maslowski, W., Perlwitz, J., and Wuebbles, D. J. (2017). “Ch. 11: Arctic Changes and Their Effects on Alaska and the Rest of the United States. Climate Science Special Report: Fourth National Climate Assessment, Volume I,” in Climate Science Special Report: Fourth National Climate Assessment, Volume I. Editors D. J. Wuebbles, S. W. Fahey, K. A. Hibbard, D. J. Dokken, B. C. Steward, and T. K. Maycock (Washington, DC, USA: U.S. Global Climate Change Research Program), 303–332. doi:10.7930/J00863GK
Taylor, P., Hegyi, B., Boeke, R., and Boisvert, L. (2018). On the Increasing Importance of Air-Sea Exchanges in a Thawing Arctic: A Review. Atmosphere 9 (2), 41. doi:10.3390/atmos9020041
Topál, D., Ding, Q., Mitchell, J., Baxter, I., Herein, M., Haszpra, T., et al. (2020). An Internal Atmospheric Process Determining Summertime Arctic Sea Ice Melting in the Next Three Decades: Lessons Learned from Five Large Ensembles and Multiple CMIP5 Climate Simulations. J. Clim. 33 (17), 7431–7454. doi:10.1175/JCLI-D-19-0803.1
Tsushima, Y., Emori, S., Ogura, T., Kimoto, M., Webb, M. J., Williams, K. D., et al. (2006). Importance of the Mixed-phase Cloud Distribution in the Control Climate for Assessing the Response of Clouds to Carbon Dioxide Increase: a Multi-Model Study. Clim. Dyn. 27 (2), 113–126. doi:10.1007/s00382-006-0127-7
Uttal, T., Curry, J. A., Mcphee, M. G., Perovich, D. K., Moritz, R. E., Maslanik, J. A., et al. (2002). Surface Heat Budget of the Arctic Ocean. Bull. Amer. Meteorol. Soc. 83 (2), 255–275. doi:10.1175/1520-0477(2002)083<0255:shbota>2.3.co;2
van der Linden, E. C., Le Bars, D., Bintanja, R., and Hazeleger, W. (2019). Oceanic Heat Transport into the Arctic under High and Low CO2 Forcing. Clim. Dyn. 53 (7), 4763–4780. doi:10.1007/s00382-019-04824-y
Vargas Zeppetello, L. R., Donohoe, A., and Battisti, D. S. (2019). Does Surface Temperature Respond to or Determine Downwelling Longwave Radiation? Geophys. Res. Lett. 46 (5), 2781–2789. doi:10.1029/2019GL082220
Vavrus, S., Holland, M. M., and Bailey, D. A. (2011). Changes in Arctic Clouds during Intervals of Rapid Sea Ice Loss. Clim. Dyn. 36 (7), 1475–1489. doi:10.1007/s00382-010-0816-0
Vavrus, S. (2004). The Impact of Cloud Feedbacks on Arctic Climate under Greenhouse Forcing*. J. Clim. 17 (3), 603–615. doi:10.1175/1520-0442(2004)017<0603:TIOCFO>2.0.CO;2
Vavrus, S., Waliser, D., Schweiger, A., and Francis, J. (2008). Simulations of 20th and 21st century Arctic Cloud Amount in the Global Climate Models Assessed in the IPCC AR4. Clim. Dyn. 33 (7), 1099–1115. doi:10.1007/s00382-008-0475-6
Vignesh, P. P., Jiang, J. H., Kishore, P., Su, H., Smay, T., Brighton, N., et al. (2020). Assessment of CMIP6 Cloud Fraction and Comparison with Satellite Observations. Earth Space Sci. 7 (2), e2019EA000975. doi:10.1029/2019EA000975
IPCC (2021). “Climate Change 2021: The Physical Science Basis,” in Contribution of Working Group I to the Sixth Assessment Report of the Intergovernmental Panel on Climate Change. Editors V. Masson-Delmotte, P. Zhai, A. Pirani, S. L. Connors, C. Péan, S. Bergeret al. (Cambridge: Cambridge University Press). In Press.
IPCC (2018). “Summary for Policymakers,” in Global Warming of 1.5°C. An IPCC Special Report on the Impacts of Global Warming of 1.5°C above Pre-industrial Levels and Related Global Greenhouse Gas Emission Pathways, in the Context of Strengthening the Global Response to the Threat of Climate Change, Sustainable Development, and Efforts to Eradicate Poverty. Editors V. Masson-Delmotte, P. Zhai, H.-O. Pörtner, D. Roberts, J. Skea, P. R. Shuklaet al. (Geneva, Switzerland: World Meteorological Organization), 32.
Wang, Y., Zhang, D., Liu, X., and Wang, Z. (2018). Distinct Contributions of Ice Nucleation, Large-Scale Environment, and Shallow Cumulus Detrainment to Cloud Phase Partitioning with NCAR CAM5. J. Geophys. Res. Atmos. 123 (2), 1132–1154. doi:10.1002/2017JD027213
Warren, S. G., Rigor, I. G., Untersteiner, N., Radionov, V. F., Bryazgin, N. N., Aleksandrov, Y. I., et al. (1999). Snow Depth on Arctic Sea Ice. J. Clim. 12 (6), 1814–1829. doi:10.1175/1520-0442(1999)012<1814:SDOASI>2.0.CO;2
Washington, W. M., and Meehl, G. A. (1989). Climate Sensitivity Due to Increased CO2: Experiments with a Coupled Atmosphere and Ocean General Circulation Model. Clim. Dyn. 4 (1), 1–38. doi:10.1007/BF00207397
Washington, W. M., and Meehl, G. A. (1986). General Circulation Model CO2 Sensitivity Experiments: Snow-Sea Ice Albedo Parameterizations and Globally Averaged Surface Air Temperature. Climatic Change 8 (3), 231–241. doi:10.1007/BF00161596
Washington, W. M., and Meehl, G. A. (1996). High-latitude Climate Change in a Global Coupled Ocean-Atmosphere-Sea Ice Model with Increased Atmospheric CO2. J. Geophys. Res. 101 (D8), 12795–12801. doi:10.1029/96JD00505
Washington, W. M., and Meehl, G. A. (1984). Seasonal Cycle experiment on the Climate Sensitivity Due to a Doubling of CO2with an Atmospheric General Circulation Model Coupled to a Simple Mixed-Layer Ocean Model. J. Geophys. Res. 89 (D6), 9475–9503. doi:10.1029/JD089iD06p09475
Webster, M. A., Rigor, I. G., Nghiem, S. V., Kurtz, N. T., Farrell, S. L., Perovich, D. K., et al. (2014). Interdecadal Changes in Snow Depth on Arctic Sea Ice. J. Geophys. Res. Oceans 119 (8), 5395–5406. doi:10.1002/2014JC009985
Wetherald, R. T., and Manabe, S. (1988). Cloud Feedback Processes in a General Circulation Model. J. Atmos. Sci. 45 (8), 1397–1416. doi:10.1175/1520-0469(1988)045<1397:CFPIAG>2.0.CO;2
Wetherald, R. T., and Manabe, S. (1975). The Effects of Changing the Solar Constant on the Climate of a General Circulation Model. J. Atmos. Sci. 32 (11), 20442–22059. doi:10.1175/1520-0469(1975)032<2044:teocts>2.0.co;2
Wielicki, B. A., Young, D. F., Mlynczak, M. G., Thome, K. J., Leroy, S., Corliss, J., et al. (2013). Achieving Climate Change Absolute Accuracy in Orbit. Bull. Am. Meteorol. Soc. 94 (10), 1519–1539. doi:10.1175/BAMS-D-12-00149.1
Wilson, C. A., and Mitchell, J. F. B. (1987). A Doubled CO2climate Sensitivity experiment with a Global Climate Model Including a Simple Ocean. J. Geophys. Res. 92 (D11), 13315–13343. doi:10.1029/JD092iD11p13315
Wilson, T. W., Ladino, L. A., Alpert, P. A., Breckels, M. N., Brooks, I. M., Browse, J., et al. (2015). A marine Biogenic Source of Atmospheric Ice-Nucleating Particles. Nature 525 (7568), 234–238. doi:10.1038/nature14986
Winker, D. M., Pelon, J., Coakley, J. A., Ackerman, S. A., Charlson, R. J., Colarco, P. R., et al. (2010). The CALIPSO Mission. Bull. Am. Meteorol. Soc. 91 (9), 1211–1230. doi:10.1175/2010bams3009.1
Winton, M., Griffies, S. M., Samuels, B. L., Sarmiento, J. L., and Frölicher, T. L. (2013). Connecting Changing Ocean Circulation with Changing Climate. J. Clim. 26 (7), 2268–2278. doi:10.1175/JCLI-D-12-00296.1
Woods, C., and Caballero, R. (2016a). The Role of Moist Intrusions in Winter Arctic Warming and Sea Ice Decline. J. Clim. 29 (12), 4473–4485. doi:10.1175/JCLI-D-15-0773.1
Woods, C., and Caballero, R. (2016b). The Role of Moist Intrusions in Winter Arctic Warming and Sea Ice Decline. J. Clim. 29 (12), 4473–4485. doi:10.1175/JCLI-D-15-0773.1
Wu, F., Li, W., Zhang, P., and Li, W. (2021). Relative Contributions of Internal Atmospheric Variability and Surface Processes to the Interannual Variations in Wintertime Arctic Surface Air Temperatures. J. Clim. 34 (17), 1–48. doi:10.1175/JCLI-D-20-0779.1
Wuebbles, D. J., Fahey, D. W., Hibbard, K. A., DeAngelo, B., Doherty, S., Hayhoe, K., et al. (2017). “Executive Summary. Climate Science Special Report: Fourth National Climate Assessment, Volume I,” in Climate Science Special Report: Fourth National Climate Assessment, Volume I (Washington, DC USA: US Global Climate Change Research Program). doi:10.7930/J0DJ5CTG
Xie, S., Liu, X., Zhao, C., and Zhang, Y. (2013). Sensitivity of CAM5-Simulated Arctic Clouds and Radiation to Ice Nucleation Parameterization. J. Clim. 26 (16), 5981–5999. doi:10.1175/JCLI-D-12-00517.1
Yang, F., Ovchinnikov, M., and Shaw, R. A. (2015). Long-lifetime Ice Particles in Mixed-phase Stratiform Clouds: Quasi-Steady and Recycled Growth. J. Geophys. Res. Atmos. 120 (22), 11,617–11,635. doi:10.1002/2015JD023679
Yoshimori, M., Abe-Ouchi, A., and Laîné, A. (2017). The Role of Atmospheric Heat Transport and Regional Feedbacks in the Arctic Warming at Equilibrium. Clim. Dyn. 49 (9), 3457–3472. doi:10.1007/s00382-017-3523-2
Yoshimori, M., Watanabe, M., Abe-Ouchi, A., Shiogama, H., and Ogura, T. (2014). Relative Contribution of Feedback Processes to Arctic Amplification of Temperature Change in MIROC GCM. Clim. Dyn. 42 (5), 1613–1630. doi:10.1007/s00382-013-1875-9
Yu, Y., Taylor, P. C., and Cai, M. (2019). Seasonal Variations of Arctic Low‐Level Clouds and its Linkage to Sea Ice Seasonal Variations. J. Geophys. Res. Atmos. 124 (22), 12206–12226. doi:10.1029/2019JD031014
Zelinka, M. D., Klein, S. A., and Hartmann, D. L. (2012). Computing and Partitioning Cloud Feedbacks Using Cloud Property Histograms. Part II: Attribution to Changes in Cloud Amount, Altitude, and Optical Depth. J. Clim. 25 (11), 3736–3754. doi:10.1175/JCLI-D-11-00249.1
Zhang, J., Rothrock, D., and Steele, M. (2000). Recent Changes in Arctic Sea Ice: The Interplay between Ice Dynamics and Thermodynamics. J. Clim. 13 (17), 3099–3114. doi:10.1175/1520-0442(2000)013<3099:RCIASI>2.0.CO;2
Zhao, X., Liu, X., Phillips, V. T. J., and Patade, S. (2021). Impacts of Secondary Ice Production on Arctic Mixed-phase Clouds Based on ARM Observations and CAM6 Single-Column Model Simulations. Atmos. Chem. Phys. 21 (7), 5685–5703. doi:10.5194/acp-21-5685-2021
Keywords: arctic amplification, climate feedback mechanisms, cloud feedback, sea ice albedo feedback, remote mechanisms, sea ice
Citation: Taylor PC, Boeke RC, Boisvert LN, Feldl N, Henry M, Huang Y, Langen PL, Liu W, Pithan F, Sejas SA and Tan I (2022) Process Drivers, Inter-Model Spread, and the Path Forward: A Review of Amplified Arctic Warming. Front. Earth Sci. 9:758361. doi: 10.3389/feart.2021.758361
Received: 13 August 2021; Accepted: 20 December 2021;
Published: 09 February 2022.
Edited by:
Matthew Collins, University of Exeter, United KingdomCopyright © 2022 Taylor, Boeke, Boisvert, Feldl, Henry, Huang, Langen, Liu, Pithan, Sejas and Tan. This is an open-access article distributed under the terms of the Creative Commons Attribution License (CC BY). The use, distribution or reproduction in other forums is permitted, provided the original author(s) and the copyright owner(s) are credited and that the original publication in this journal is cited, in accordance with accepted academic practice. No use, distribution or reproduction is permitted which does not comply with these terms.
*Correspondence: Patrick C. Taylor, cGF0cmljay5jLnRheWxvckBuYXNhLmdvdg==
Disclaimer: All claims expressed in this article are solely those of the authors and do not necessarily represent those of their affiliated organizations, or those of the publisher, the editors and the reviewers. Any product that may be evaluated in this article or claim that may be made by its manufacturer is not guaranteed or endorsed by the publisher.
Research integrity at Frontiers
Learn more about the work of our research integrity team to safeguard the quality of each article we publish.