- 1Southwest Oil and Gas Field Company, PetroChina, Chengdu, China
- 2State Key Laboratory of Oil and Gas Reservoir Geology and Exploitation, Southwest Petroleum University, Chengdu, China
- 3China National Petroleum Corporation, Beijing, China
Based on logging, seismic, fracturing and production data from 301 productive wells in Longmaxi Formation in Changning shale gas field of southern Sichuan basin, the various influence factors of shale gas stimulated potential have been analyzed to carry out the correlation study with estimated ultimate recovery (EUR) of the same fracturing operation intensity to fully clarify the main controlling factors for shale gas stimulated potential. The results show that matrix brittleness, fracture propagation and reservoir properties are the 3 key secondary potentials that control shale gas stimulation potential. The matrix brittleness is controlled by elastic modulus, Poisson’s ratio, Type I and II fracture toughness, which reflects the uniformity of hydraulic fracture propagation. Fracture propagation is controlled by critical net pressure, which reflects the scale of hydraulic fracture propagation. Reservoir properties are controlled by porosity, total organic carbon content (TOC) and horizontal interval differences, which reflect the enrichment conditions and dynamic production capacity of reservoir resources. The matrix brittleness index, fracture propagation index, reservoir properties index and their combined stimulated potential index were formed by using the above 7 parameters to verify and apply the wells in Changning shale gas field. Results show that the matrix brittleness index and length-width ratio of hydraulic fracture was significantly negative correlation, the fracture propagation index and stimulated reservoir volume (SRV) were significantly positive correlation, stimulated potential index was developed taking into account enrichment & exploitation potential. In areas where the value is greater than 0.5, increasing the fracturing scale can effectively improve the well productivity; in areas less than 0.5, increasing the fracturing scale has an upper limit on the increase of productivity, so the physical properties of the reservoir itself play a significant role in controlling the production of shale gas wells.
Introduction
For thick shales with stable sedimentary structures condition and continuous distribution in North America, such as Barnett, Utica, Marcellus and Eagle Ford, it is necessary to obtain large enough reservoir stimulated volume (SRV) through hydraulic fracturing of horizontal Wells to achieve resource production (Zhao et al., 2018). However, such as the Haynesville shale in North America and the southern Sichuan Basin in China with larger burial depth, more complex seepage law and large in-situ stress difference, only aiming to obtain larger SRV can no longer guarantee that the larger SRV in the region or area can obtain higher productivity (Shen et al., 2021). Numerical simulation studies show that the maximum distance that the fluid in the matrix of shale gas reservoir can fully flow within 10 years is 7–10 m in the radius (Ma, 2021), the degree to which hydraulic fractures interactive with natural fractures to form complex fracture network directly determines whether the gas well can be produced for a long time. It is crucial to evaluate the complexity of hydraulic fractures. Therefore, different evaluation theories and methods have been formed, including concept evaluation using brittle mineral mass fraction (Rickman et al., 2008; Rybacki et al., 2016; Tang et al., 2016; Kumar et al., 2018; Arijit and Milan, 2019; Ayyaz et al., 2019; Vafaie and Rahimzadeh Kivi, 2020); emphasized the influence of natural weak plane, and established the three-dimensional evaluation method of natural weak surface through sedimentary facies-control theory (Fu et al., 2015; Geng et al., 2016; Ou and Li, 2017; Yi et al., 2019); evaluation of rock stress-strain properties based on experiments (Jin et al., 2014; Govindarajan et al., 2017); evaluation of the potential of forming three-dimensional maps based on rock and fracture mechanics (Yuan et al., 2013; Ji et al., 2019); A brittleness ductility evaluation method considering the effect of burial depth changes in ancient and modern times (Yuan et al., 2018; He et al., 2019); Coupling evaluation of mineral and rock mechanics which carried out with consideration of petrophysical fabric (Liu and Sun, 2015); The potential evaluation method considered stress state at fracture interaction (Wang H. et al., 2016; Wang S. et al., 2016; Sheng and Li, 2016; Liu et al., 2019); A superposition evaluation method considering multiple factors was established (Tang et al., 2012; Zhao et al., 2015; Chen et al., 2017; Cui et al., 2019; Shen et al., 2017). From the perspective of engineering, some scholars proposed that stage length, operation (net) pressure, well (segment, cluster) spacing, fracturing fluid scale and performance, pumping rate and proppant dosage can also be used as evaluation indexes (Zhao et al., 2013; Huang et al., 2016; Liao and Lu, 2018).
Practice shows that it is insufficient to use single factor to analyze the stimulation effect of gas wells, and even in Changning gas field and Jiaoshiba gas field in south China, the phenomenon that the higher the brittle mineral mass fraction is, the lower the production is. The main controlling factors are seldom analyzed and comprehensive evaluation methods are established by using multi-factor analysis. More importantly, static geological parameters are mostly taken as the index and the test yeild as the target in evaluation, and static parameters are considered to directly control the productivity of gas wells. Rock mechanics, fracture mechanics and geomechanical parameters are not fully considered, and the main controlling factors of cumulative production in different production stages of gas wells are not analyzed. Therefore, under the same technology conditions and stimulated intensity, it is necessary to comprehensively evaluate whether shale gas reservoirs can form large enough SRV and complex fracture network and achieve stimulated potential of commercial productivity, so as to form a comprehensive geological evaluation system with shale gas quality and fracturing quality as the core (Hou et al., 2021; Ma., 2021).
To solve the problems above, this study took the shale gas of 1st member of Longmaxi Formation in Changning gas field, southern Sichuan Basin, China as an example. Based on a large number of well testing, monitoring and productive data, the key factors affecting reservoir stimulated potential were analyzed, and the main controlling factors affecting SRV, fracture complexity and gas well productivity were selected to provide the basis for shale gas geological engineering double sweet spot optimization and targeted fracturing parameter combination system.
Geological Setting and Productive Situation
There are six sets of shale formations widely distributed in Sichuan Basin, among which the Qiongzhusi Formation and Longmaxi Formation are favorable strata for marine shale exploration and development. At present, the main exploration and development target is Longmaxi Formation, which is a set of organic-rich black shale deposited in deep water shelf facies. From the southwest to the northeast, it mainly includes the Changning-Weiyuan demonstration area and the Luzhou-West Chongqing deep evaluation area, with the burial depth gradually transitioning from 2000–3500 m to 3,500–4500 m, and the reservoir thickness is about 30–210 m. Among them, the main producing layers are located in the Wufeng Formation and the 1st sub-member of the 1st member of Longmaxi Formation, with a thickness of about 30–60 m, and the high quality shale is about 5–20 m thick (Figure 1). From bottom to top, it is subdivided into five sub-layers, namely, Wufeng Formation, L111, L112, L113 and L114. The overlying stratum is sand and mudstone of Long12, and the underlying stratum is nodular limestone of Linxiang Formation. Total organic carbon content (TOC) of the reservoir is about 2.6–5.3%, with an average of 3.6%. The porosity is about 3.7–7.9%, with an average of 4.4%. The mass fraction of siliceous minerals (quartz, feldspar) is 22.8–80.6%, with an average of 48.7%. The mass fraction of carbonate minerals (calcite and dolomite) is about 0–27.8%, with an average of 13.8%. The mass fraction of clay minerals (kaolinite and illite) is about 24–40%, with an average of 26.7%. The elastic modulus was about 12.6–55.8 GPa, with an average of 34.6 GPa. Poisson’s ratio was about 0.14–0.31, with an average of 0.21; The minimum horizontal principal stress is about 55–95 MPa, with an average of 81 MPa. The horizontal principal stress difference is about 8–20MPa, with an average of 12.6 MPa.
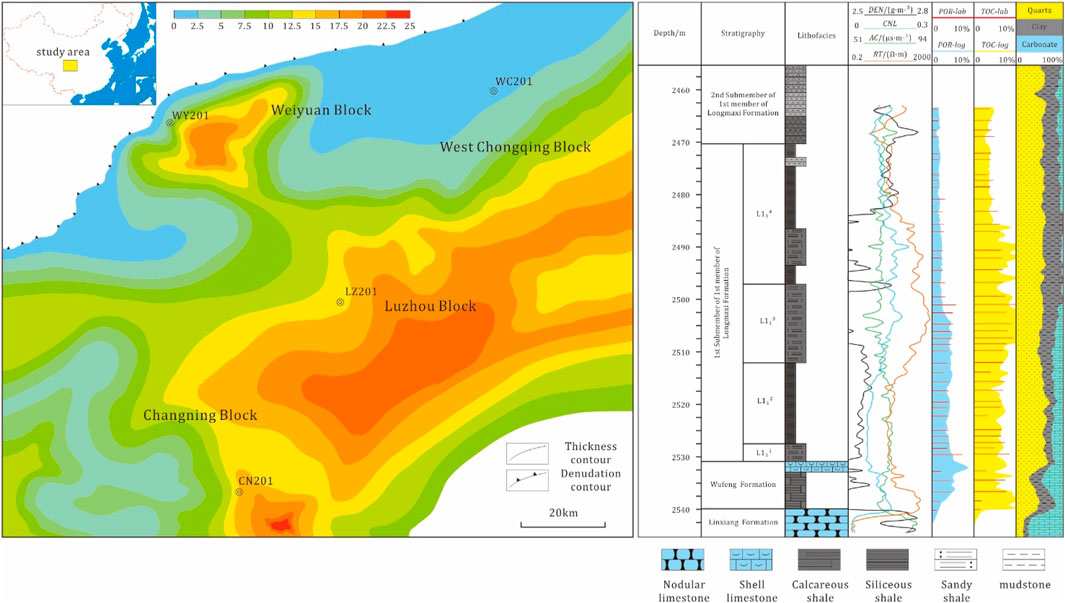
FIGURE 1. Map showing the high-quality reservoir thickness of the 1st member of Longmaxi Formation, and locations of sampling well and its comprehensive stratigraphic column.
Data and Methods
Data Sources
The data in this paper focus on logging interpretation, seismic interpretation, fracturing operation and dynamic monitoring data of 161 horizontal wells in the 1st Member of Longmaxi Formation in Changning shale gas field. The logging interpretation data includes well path, reservoir property, rock mechanics, in-situ stress, the seismic interpretation data include the occurrence of natural fracture zone, the fracturing operation data include single well fracturing stage length, liquid and proppant strength, pumping rate, the dynamic monitoring data include micro-seismic monitoring results, test and cumulative production, EUR, which provide basic data for the study of the main controlling factors of shale gas well stimulation potential.
Methods for Factors
The study first extracted the required source factors (SF) and their derived factors (DF) as much as possible through logging and seismic interpretation data, which can be divided into matrix brittleness (MB), fracture propagation (FP) and reservoir properties (RP) (Table 1).
Derived factors refer to all kinds of parameters that are combined with source factors or represent reservoir geological characteristics indirectly. They include dynamic modulus of elasticity (Edyn), Poisson’s ratio (ν), the total brittleness mineral mass fraction (VTotal), type I fracture toughness (KI) and type II fracture toughness (KII), natural fracture belt approaching angle (θ), in-situ stress difference (Δσ), micro natural fracture development index (WNF), micro bedding development index (WLM), the fracture pressure (Pp), critical net pressure (Pnet), free gas volume (GasF), absorbed gas volume (GasA), vertical depth at the starting point (DA) and ending point (DB) of horizontal well fracturing stage, total gas content (GasT), vertical depth difference of horizontal well (ΔD, DA is always subtracted from DB to obtain the difference, which can distinguish updip well from downdip well).
We also give the calculation formulas of the above parameters to make the evaluation factors as complete as possible (Table 1). It is worth noting that the better the matrix brittleness factor is, the greater the brittleness is, the more beneficial to improve the complexity of the fracture network (Rickman et al., 2008). The better the fracture propagation factors are, the wider the hydraulic fracture propagation may be. The better the reservoir resource factor, the more resources can be recovered within the transformed volume. In more detail, we introduce a large number of parameters not involved in conventional evaluation: ①Generally, the azimuth angle β of natural fracture zone is of great significance for well trajectory design, but for drilled and fractured wells, the approach angle θ is more valuable for analysis; ②WNF and WLM reflect the development scale of micro-fractures and micro-bedding inside the matrix rock, which is controlled by sedimentary facies and is positively correlated with the content of mineral components, and can be obtained by multiple regression of VSi and VCa in the source factors; ③Pp is a newly added parameter in this study that comprehensively considers three-dimensional stress and natural fracture zone angle to evaluate whether fractures can be opened. The smaller the value is, the easier the natural fracture zone is to be opened, and the more conducive it is to hydraulic fracturing; ④Pnet is introduced as a newly added parameter, the smaller the value is, it indicates that the penetration and steering of hydraulic fractures are less difficult under the same construction intensity, and the fracture complexity is higher; ⑤Horizontal well path also has a crucial influence on long-term production effect. Both updip and downdip wells have large dip angles, which will lead to fluid accumulation at heel and toe, respectively, which will affect long-term productivity.
Methods for Stimulation Effects
The study also collected source targets (ST) and derived targets (DT) of dynamic monitoring, which can be divided into two categories: microseismic monitoring and production dynamic monitoring. Microseismic monitoring includes SRV (SRV), spread length (L), spread width (W), spread height (H) and derived parameters spread length-width ratio (R). Production dynamic monitoring includes daily test yeild (QTest), 30/60/90/180/330 days’ cumulative yeild (Q30, Q60, Q90, Q180, Q330), EUR (EUR) and derivative parameters equivalent construction intensity production data (QTest+, Q30+, Q60+, Q90+, Q180+, Q330+, EUR+).
The Method for Micro-seismic Monitoring
It is generally believed that the larger the SRV, the larger the L, W and H are, indicating the larger the area that can be utilized. The constructed derivative parameter R is defined as the ratio of spread length to spread width, as shown in Eq. 1. The smaller R is, the higher the uniformity is, the more uniform the SRV is, and the fewer the blind zones between intervals and wells are.
The Method for Production Dynamic Monitoring
Productive monitoring source data are read by metering equipment and visually reflect well productivity, while EUR is obtained through the Duong model (Duong, 2011). The DT are mainly aimed at eliminating the influence of fracturing operation parameters. For the same stimulated intensity, this study is defined as returning to the average of the main parameters of shale gas fracturing in Sichuan Basin. Therefore, for any horizontal well, the corresponding productivity data of different periods are regression to the strength of fracturing section length of 1500m, cluster space of 10m, hydraulic strength of 30 m³/m (hydraulic volume used in horizontal well per meter), proppant strength of 2 t/m (supporting dose used in horizontal well per meter), and pumping rate of 15 m³/min for unified evaluation, as shown in Eq. 2. Minimize the impact of fracturing parameters.
Results
There are three keys to reservoir stimulation potential: high SRV, high fracture complexity, and high economic productivity. The correlation study between the factors above and the dynamic monitoring objectives can reveal the main controlling factors of the dynamic effect of horizontal shale gas wells, so as to achieve the purpose of comprehensive evaluation of reservoir stimulated potential. In the first place, without considering whether the reservoir contains resources, the study aims at maximizing SRV and fracture complexity to explore the reservoir parameters related to SRV and spread length-width ratio in micro-seismic monitoring. Combined with the reservoir property of gas-bearing shale, the study comprehensively evaluates the gas well productivity and selects the parameter group with the highest influence degree.
Relationship Between the Factors and Micro-seismic Monitoring Results
Micro-seismic monitoring can be divided into two modes: downhole micro-seismic monitoring and surface micro-seismic monitoring. To eliminate the influence of different monitoring modes, this study selected 554 stages of 25 downhole micro-seismic wells with similar fracturing operation parameters in the Changning gasfield as the target, using the seismic and logging data to read the matrix brittleness and fracture propagation factors of each stage of horizontal well, and the correlation analysis is conducted with the target parameters of each stage monitored by micro-seismic.
Univariate analysis was carried out based on the calculation results of 554 stages of 25 wells in Changning gas field and SRV or R of each stage (Table 2). Due to the influence of the distance between the downhole micro-seismic monitoring sensor and the monitoring well, the monitoring data of each well is susceptible to the influence of the signal strength in the processing and has the difference in the value size of SRV. Therefore, the collected 554 segments of data are not completely linear. Taking 40 stages in three wells on platform H6 as an example, the factors with the most significant correlation with SRV were obtained, including Pnet, Δσ, Edyn, σv, σh, σH (Figure 2). The factors with the most significant correlation with R mainly included Edyn, ν, WNF, WLM, KII, KI (Figure 3). The ranking of other relevant factors can be seen in Table 2.
Relationship Between the Factors and Productivity
The evaluation of the stimulation effect in Sichuan Basin usually takes the test yield as the main goal and tends to ignore the long-term stable yield ability. On the other hand, reservoir quality also determines the upper limit of production. Since it is difficult to evaluate productivity by stages as a unit, this study selected 161 wells in Changning gasfield as the analysis target, and took into account the factors that affect SRV and R of micro-seismic monitoring. At the same time, several factors affecting the reservoir quality and production effect, such as POR, TOC, GasF, GasA, GasT, VTotal, ΔD, were considered to analyze the influence on the production parameters of the same stimulated intensity.
Univariate analysis was conducted by using the calculation results of 161 wells in Changning gasfield and QTest+, Q30+, Q60+, Q90+, Q180+, Q330+, EUR+ of a single well (Table 3). The analysis shows that there are significant differences in the main controlling factors of gas well production in different production stages.
Discussion
Influence Mechanism on SRV and R
For SRV, Pnet is a parameter derived from Δσ and θ, and its influence on SRV is much higher than that of Δσ. The smaller Pnet is, the greater the probability of hydraulic fractures penetration and diversion towards natural fractures is, and the wider the spread hydraulic fractures is. Therefore, the development direction of natural fracture belt and the comprehensive influence of horizontal stress difference play a key role in hydraulic fracture propagation. We also can see that Pnet can basically embody the characteristics of Δσ, σH, σh. Therefore, Pnet and Edyn are selected as the main analysis objects. it can be seen from the statistical figure that the fracturing stages with smaller Pnet and larger Edyn in a single well generally have higher SRV (Figures 4A,B), which can still show strong negative correlation and positive correlation, respectively, on the whole. According to ΔPnet = 1 MPa as the change step size, the mean value of Pnet and SRV in the interval corresponding to the segment of the line (Figure 4C) was calculated. Similarly, according to ΔEdyn = 2.5 MPa as the change step size, the mean value of Edyn and SRV in the corresponding interval of Edyn was calculated (Figure 4D). It can be seen that both Pnet and Edyn have a good power relationship with SRV, and the accuracy of the trend line is high. Therefore, Edyn is obtained based on the comprehensive calculation of mineral and saturated fluid matrix, and also has the significance of rock ore itself. Therefore, Pnet and Edyn are selected as the main factors to evaluate the formation of large SRV in the reservoir under the precondition of not repeated consideration.
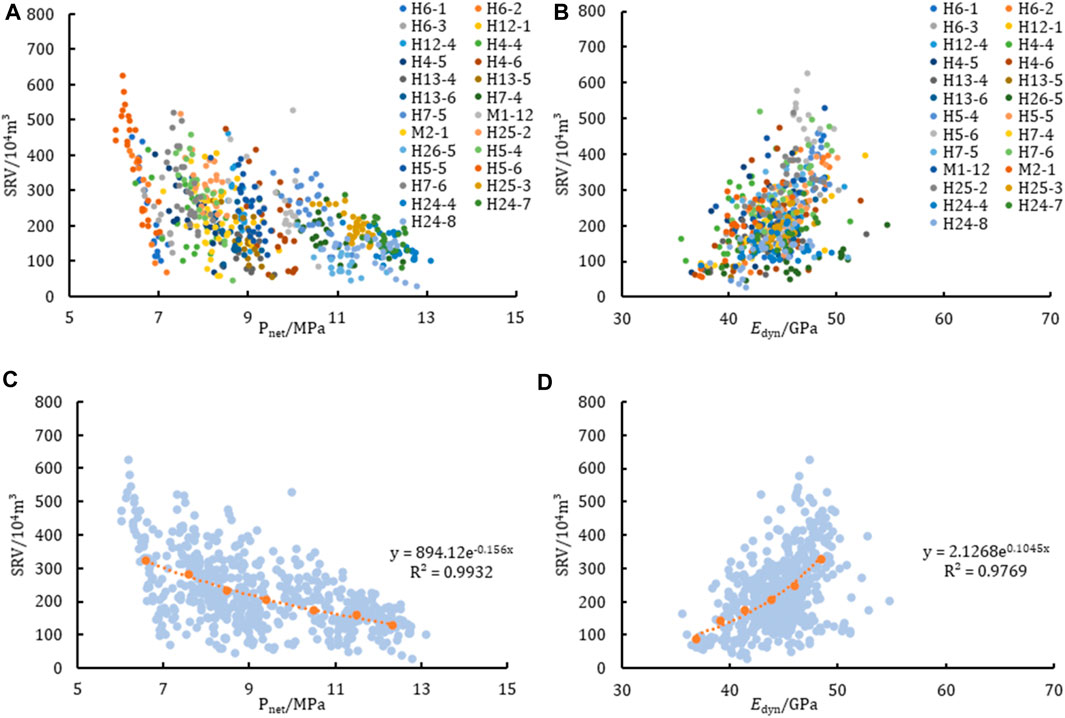
FIGURE 4. Relationship among Pnet, Edyn of single segment and SRV of underground micro-seismic in Changning gas field.
For R, it can be seen that the influence of Edyn and ν on R is much higher than that of other factors, indicating that the reservoir with higher matrix brittleness is more conducive to be uniformly stimulated. WNF and WLM also have a large influence, indicating that the development of natural weak plane may aggravate the non-uniform propagation of hydraulic fractures, resulting in the imbalance of spread length-width ratio. The increase of KII also leads to the imbalance of fracture uniformity, indicating that the stronger the fracture resistance of rock is, the smaller the possibility of hydraulic fracture bending is. The influence of mineral composition on fracture uniformity is small, which may be related to the difficulty of mineral composition to directly reflect the rock structure and mechanical properties. Compared with SRV, the influence of in-situ stress and its derived parameters on R is small. Edyn and ν are extracted as the main analysis objects according to the correlation, and 554 stages of data are also analyzed statistically. It can be seen from the statistical figure that the lower the ν and the greater the Edyn in a single well, the smaller R is generally (Figures 5A,B). According to ΔEdyn = 2 GPa as the change step size, the mean Edyn and R in the interval corresponding to Edyn was calculated (Figure 5C). In the same way, according to Δν = 0.02 as the changing step size, the mean ν and R in the interval corresponding to the ν is calculated (Figure 5D). It can be seen that both Edyn and ν have a good power relationship with R, and the precision of the trend line is high. Therefore, Edyn and ν are selected as the main controlling factors to evaluate the uniform expansion of the reservoir.
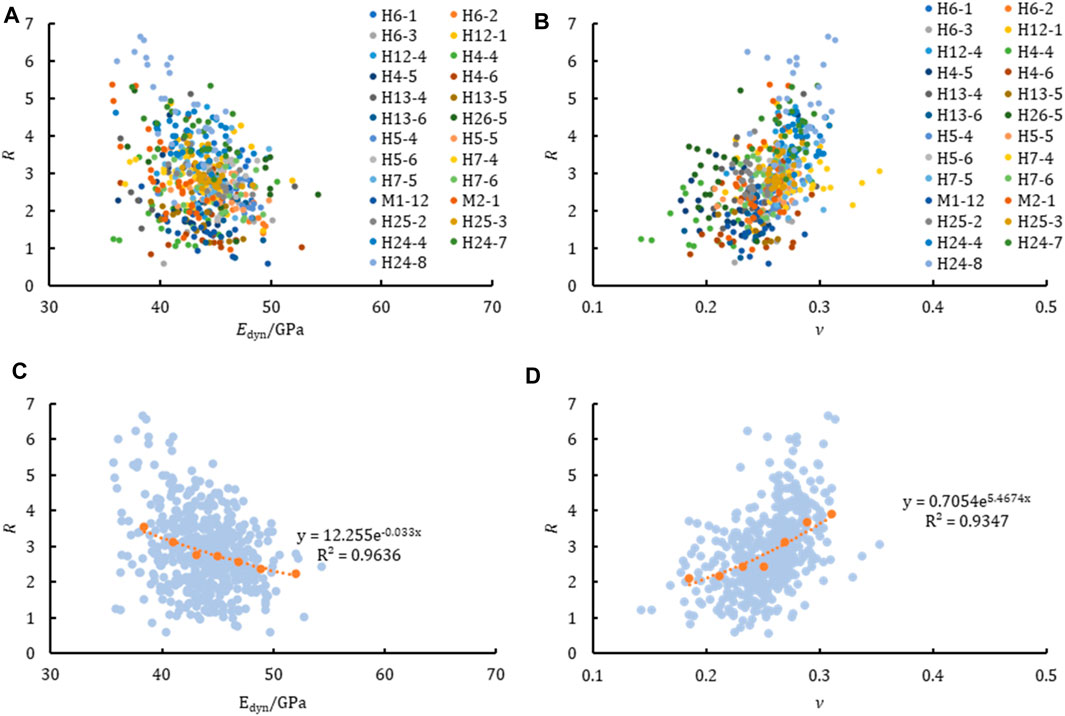
FIGURE 5. Relationship among Edyn, ν of single segment and R of underground micro-seismic in Changning gas field.
Influence Mechanism on Productivity
For QTest+, GasT, TOC, GasA, POR, GasF are the main factors affecting the test daily production, which indicates that the reservoir quality plays a decisive role in the initial productivity. The obvious negative correlation between VTotal and QTest+ is not because the high brittleness of the reservoir affects the production, but more likely because the gas wells with high brittleness do not have high quality reservoir quality under the influence of deposition and diagenesis (Shen et al., 2020b). It also shows that it is not sufficient to evaluate the reservoir stimulation potential only by using brittle minerals as an engineering factor. The influence of ΔD on the daily test yield is different from other factors. When it is negative, it means that the heel of the horizontal well is higher than the toe, indicating downdip well; otherwise, it means updip well; and its absolute value represents the inclination of the horizontal well. Influenced by the structure of the Sichuan Basin, the dip angle of 0–10° is always present in the main gas producing layers, and the larger dip angle has an obvious risk of fluid accumulation, thus affecting the production (Wei et al., 2019). Therefore, there is a binomial relationship between the dip Angle and the measured daily production.
But for EUR+, it shows that the main controlling factors have inherited the changing trend from the daily test yield to the EUR of the period mentioned above. The main controlling factors are changed, and changed in order. From the results of univariate analysis, the dominant factors have four characteristics. The first is the instability of the main control factors. The main control factors always change, with the passage of production time, the overall change is great. Second, and most important, with the passing of production time, the weight of Pnet and Edyn, which influence the effect of fracture network stimulation, is gradually increased, and the primary factor is gradually changed from “geological parameters” to “engineering parameters.” Third, reservoir property parameters could not to be ignored to productivity effect, which is the basic guarantee for the production after the formation of complex fracture network. Fourth, ΔD can not be ignored. The analysis shows that the influence of the horizontal well trajectory inclination on the long-term stable production is gradually increasing, which may be related to the fluid accumulation at the heel and toe of the upward dip well after a long production time. Comprehensive analysis shows that long-term stable and high production of gas well is not only affected by static geological parameters of reservoir, but also by rock mechanics, fracture mechanics, geomechanics and well path. Thus, the influence model of shale gas well geology-engineering-production model is established (Figure 6).
In the early stage of production, under the precondition of smooth fracturing operation, gas well productivity is mainly controlled by RP, that is, the stronger the reservoir properties, the higher the gas content, and the overall high production of the gas well. Factors as production time, MB and FP gradually highlight the influence of factors on gas well productivity, show the resources of enrichment of reservoir formed by hydraulic fracturing complex network, get bigger the SRV, is the basis of the gas wells to maintain long-term stable and high yield eventually safeguard, also shows that the reservoir itself have the ability to form complex joint network and the larger the SRV, under the same construction intensity, relatively high yields can be obtained. The main controlling factors of productivity in different stages also show that the main controlling factors of gas well are RP factor, MB factor and FP factor successively: Regardless of the technology, the near-wellbore zone can be fully stimulated. Therefore, in the early stage of production, production mainly comes from the near-wellbore zone where complex fracture networks are formed by fracturing, and the reservoir and gas content of the reservoir itself absolutely control the productivity (Figures 6A,B). As production time goes by, the fracture network formed by hydraulic fracturing connects the reservoir matrix and communicates with natural fractures, which becomes the main factor affecting production replacement. The more channels gas flows from the matrix to the wellbore, the more effectively the production of the gas well can be maintained (Figures 6C,D). At the end of production, gas well productivity is more reflected in the initiative of stimulated reservoir volume, emphasizing that the wider control area of hydraulic fracture in three-dimensional space, the more conducive to obtain the final cumulative high production (Figures 6E,F).
Stimulation Effects Evaluation
According to the classification of different influencing factors, combined with their different influencing mechanisms, EUR+ was taken as the evaluation target, and the top factors were selected as the main control factors. Among them, Pnet is the main controlling factor of fracture propagation, KII, KI, Edyn, ν are the main controlling factors of matrix brittleness, and POR, TOC, and ΔD are the main controlling factors of reservoir properties, which are used for parameters required by subsequent evaluation methods.
Evaluation Model Establishment
Matrix brittleness is fused with Edyn and ν, considering the difference of the two orders of magnitude, the two parameters are firstly dimensionless processing:
Then, the dimensionless elastic modulus and the dimensionless Poisson’s ratio were dimensionless again to establish the matrix brittleness potential index:
Pnet is dimensionless to obtain the fracture propagation potential index:
Since it is difficult to combine POR and TOC, they should be combined by considering the weight. Therefore, the corresponding correlations of 0.1768 and 0.1734 in single factor analysis of POR and TOC were selected to evaluate EUR of gas wells. ΔD is introduced, and 1000 m vertical depth difference is taken as the gas well limit, and enrichment and development potential index is established to reflect the impact of gas well inclination on production:
Finally, the three factors are combined to form the stimulation potential index:
Field Evaluation
The study collected EUR+ data from 301 wells, including 161 analysis wells above and 140 validation wells. A total of 301 wells were used for the analysis, and once the majority of wells met the evaluation requirements, the method proved to be of widespread value. With EUR+ setting 0.5 × 108 m³ as step length, the productivity interval was divided. CF and PF were used as horizontal and vertical coordinates respectively to analyze the distribution interval of high-producing and low-producing Wells (Figure 7A). Wells with EUR+ at 1.5 × 108 m³ are mainly distributed in the dominant zones (CF and PF greater than 0.5), but the red dominant zones also have a significant number of low-producing wells, indicating that effective fracturing potential alone is not sufficient to reflect stimulation potential.
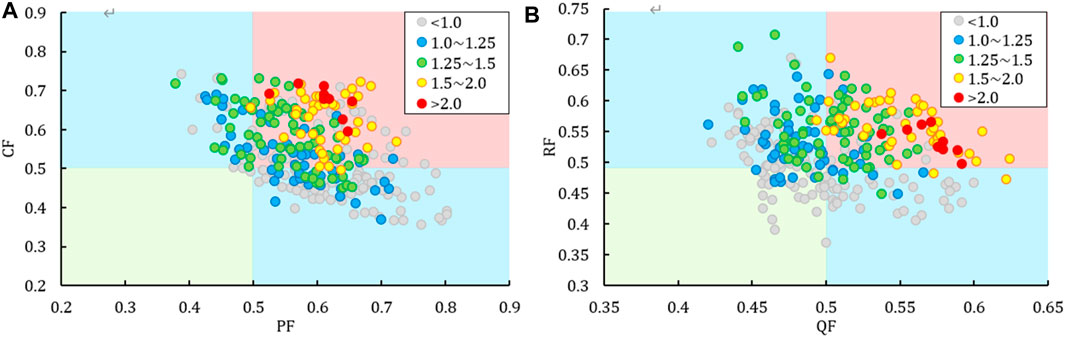
FIGURE 7. Gas well production distribution corresponding to different evaluation charts (Sample well number 301).
For convenience analysis, CF and PF are equivalent combined as QF (Eq. 9) to comprehensively evaluate stimulated effect and form a Cartesian coordinate system with RF (Figure 7B). In terms of the distribution of gas Wells, the high-producing wells are always distributed in the dominant area (QF and RF are greater than 0.5), while the low-producing wells are “eliminated” to other areas, with a more obvious differentiation, which confirms that the factors selected in this study and the indicators formed are effective in the evaluation of the stimulated potential of gas wells.
A geological model was established based on 301 logging data to reflect the burial depth of the reservoir (Figure 8A) and the comprehensive index MF (Figure 8B). The higher the MF, the better the stimulated potential of the reservoir, and the more conducive to achieving high yield. It can be seen that the high part of the structure and the slope area (MF > 0.5) are the main positions to obtain high yield (Liu et al., 2020, 2021), while the axial part of the tectonic syncline (MF < 0.5) is more complicated due to stress concentration.
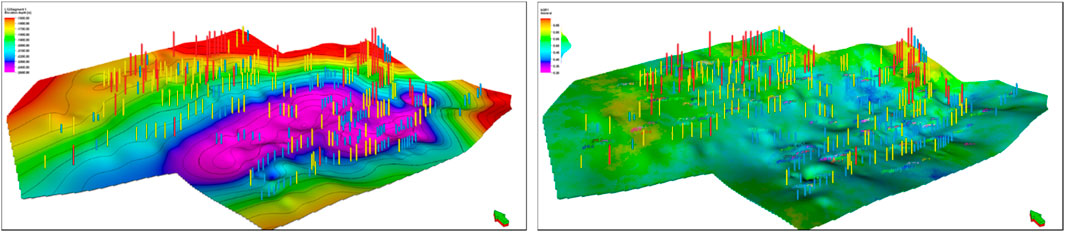
FIGURE 8. Distribution of gas Wells with different production rates in buried depth contour and MF contour.
Moreover, in the fracturing process of horizontal wells in Changning gasfield, the liquid strength, proppant strength and pumping rate in a single well are within the range of 20–45 m³/m, 1∼5 t/m and 8–18 m³/min respectively. A total of 181 wells in high part of structure and slope areas saw a significant increase in EUR as the fracturing parameters increased, and it seems that the fracturing parameters could be further increased to achieve the purpose of production increase (Figures 9A,C,E). However, from the parameter changes of 120 wells in the synclinal axis, the increase rate of EUR per well is low, and the increase of production is no longer obvious after fracturing operation parameters increase to a certain scale, that is, reservoir conditions control the upper limit of gas well productivity in this area (Figures 9B,D,F).
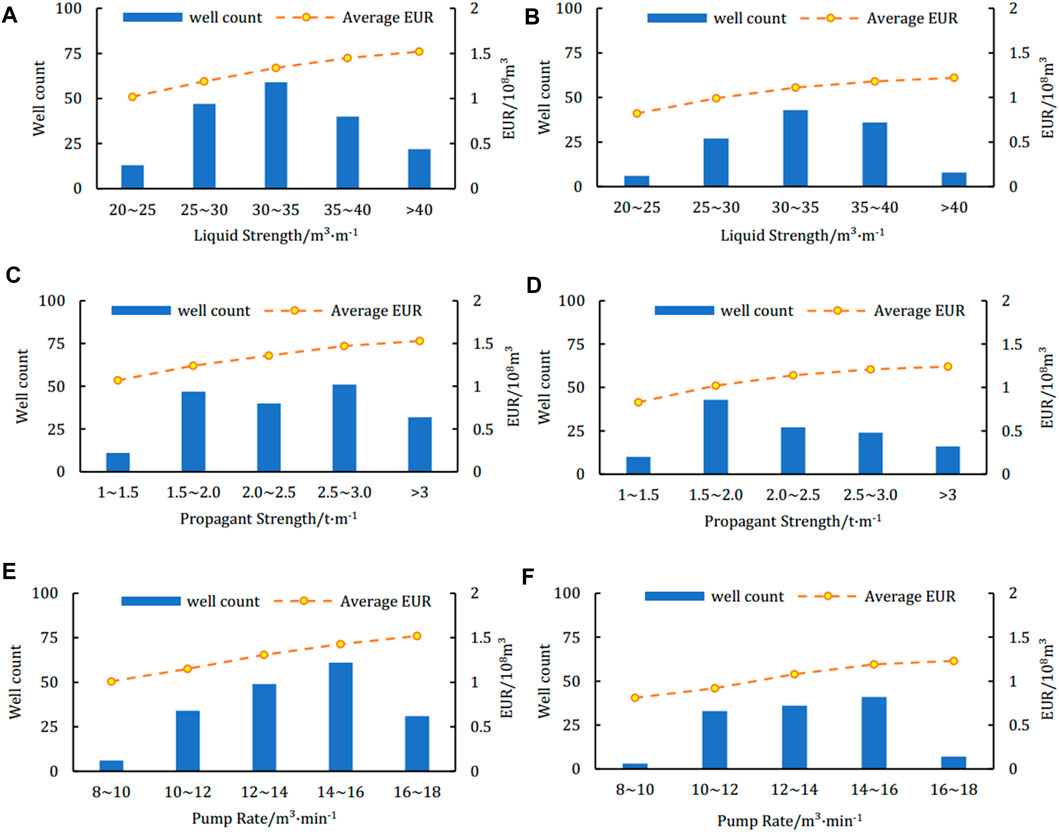
FIGURE 9. Relationship between frac-parameters and EUR of gas wells in regions with MF greater and less than 0.5.
Conclusion
Shale gas stimulated potential can intuitively reflect the gas well productivity, can be divided into matrix brittleness, fracture propagation and enrichment and exploitation of three factors. It is mainly controlled by elastic modulus, Poisson’s ratio, Type I and II fracture toughness, critical net pressure, porosity, TOC, and well trajectory, which are related to well trajectory, reservoir property, rock mechanics, in-situ stress, occurrence of natural fracture belt and their derived parameters.
The matrix brittleness index can be used to evaluate the hydraulic fracture homogenicity, and the fracture propagation index can be used to evaluate the SRV scale. Comprehensive index (MF) can be used to evaluate the stimulation potential of gas well. The higher the MF, the higher the ratio of gas Wells to high production, indicating that the reservoir itself must not only be favorable for fracturing, but also have sufficient resource enrichment. In areas with MF greater than 0.5, wells can achieve significant production gains through enhanced fracturing parameters. In the area less than 0.5, when the fracturing parameters reach a certain level, the productivity increase of the well is no longer obvious, and the physical properties of the reservoir itself play a significant role in controlling the production of shale gas wells.
Data Availability Statement
The data analyzed in this study is subject to the following licenses/restrictions: The data belongs to the internal production data of the oil and gas field company and can be used inside the oil field. The methods in the article can be extended to other fields for use. Requests to access these datasets should be directed to U2hlbkNfVmljdG9yQDE2My5jb20=
Author Contributions
CS: data analysis, method research, and paper writing ZZ: Theoretical research and methodological research JX: methodological research and thinking arrangement FW: Data analysis QF: data analysis and method research LR: Data analysis.
Conflict of Interest
Authors CS, QF, and FW were employed by the Southwest Oil and Gas Field Company. Author JX was employed by the China National Petroleum Corporation.
The remaining authors declare that the research was conducted in the absence of any commercial or financial relationships that could be construed as a potential conflict of interest.
Publisher’s Note
All claims expressed in this article are solely those of the authors and do not necessarily represent those of their affiliated organizations, or those of the publisher, the editors and the reviewers. Any product that may be evaluated in this article, or claim that may be made by its manufacturer, is not guaranteed or endorsed by the publisher.
References
Ayyaz, M., Abdulazeez, A., Mohamed, I. A., and Ali, S. (2019). “Lithofacies Controls on Mechanical Properties and Brittleness in Qusaiba Shale, Rub’s Al-Khali Basin, Saudi Arabia,” in International Petroleum Technology Conference, Beijing, 1–20. doi:10.2118/19084-MS
Biot, M. A. (1956). Theory of Propagation of Elastic Waves in a Fluid‐Saturated Porous Solid. I. Low‐Frequency Range. The J. Acoust. Soc. America 28, 168–178. doi:10.1121/1.190824110.1121/1.1908239
Chen, J. Z., Cao, H., and Sun, P. H. (2017). Fracability Evaluation of Shale in the Niutitang Formation in Northwestern Hunan. Earth Sci. Front. 24, 390–398.
Cui, C. L., Dong, Z. G., and Wu, D. S. (2019). Rock Mechanics Study and Fracability Evaluation for Longmaxi Formation of Baojing Block in Hunan Province. Nat. Gas Geosci. 30, 626–634.
Duong, A. N. (2011). Rate-decline Analysis for Fracture-Dominated Shale Reservoirs. SPE Reservoir Eval. Eng. 14, 377–387. doi:10.2118/137748-PA
Fu, H., Wang, X., Zhang, L., Gao, R., Li, Z., Zhu, X., et al. (2015). Geological Controls on Artificial Fracture Networks in continental Shale and its Fracability Evaluation: A Case Study in the Yanchang Formation, Ordos Basin, China. J. Nat. Gas Sci. Eng. 26, 1285–1293. doi:10.1016/j.jngse.2015.08.034
Gassmann, F. (1951). Elastic Waves through a Packing of Spheres. Geophysics 16, 673–685. doi:10.1190/1.1437718
Geng, Z., Chen, M., Jin, Y., Fang, X., and Jing, N. (2016). Integrated Fracability Assessment Methodology for Unconventional Naturally Fractured Reservoirs: Bridging the gap between Geophysics and Production. J. Pet. Sci. Eng. 145, 640–647. doi:10.1016/j.petrol.2016.06.034
Govindarajan, S., Gokaraju, D. V., Mitra, A., Patterson, R., and Aldin, M. (2017). “Evaluation of Fracability index for Reservoir Rock- A Laboratory Study,” in Proceedings of 51st U.S. Rock Mechanics/Geomechanics Symposium, California, USA. ARMA-2017-0381.
He, Z., Li, S., Nie, H., Yuan, Y., and Wang, H. (2019). The Shale Gas "sweet Window": "The Cracked and Unbroken" State of Shale and its Depth Range. Mar. Pet. Geology. 101, 334–342. doi:10.1016/j.marpetgeo.2018.11.033
Hill, R. (1952). The Elastic Behaviour of a Crystalline Aggregate. Proc. Phys. Soc. A. 65, 349–354. doi:10.1088/0370-1298/65/5/307
Hou, L. H., Yu, Z. C., Luo, X., Lin, S. H., Zhao, Z. Y., and Yang, Z. (2021). Key Geological Factors Controlling the Estimated Ultimate Recovery of Shale Oil and Gas: A Case Study of the Eagle Ford Shale. Gulf Coast Basin, USA. Pet. Exploration Dev. 48, 654–665. doi:10.1016/S1876-3804(21)60062-9
Huang, J., Wu, L. Z., and You, Y. (2016). The Evaluation and Application of Engineering Sweet Spots in a Horizontal Well in the Fuling Shale Gas Reservoir. Pet. Drilling Tech. 44, 16–20. doi:10.11911/syztjs.201603003
Ji, G. F., Li, S. C., and Li, K. D. (2019). A Method for Evaluation Shale Fracability Based on Shear Slip Fractures under Plane Strain and Intergranular Fracture. J. Chongqing Univ. 42, 1–9. doi:10.11835/j.issn.1000-582X.2019.255
Jin, X., Shah, S. N., Roegiers, J.-C., and Zhang, B. (2015). An Integrated Petrophysics and Geomechanics Approach for Fracability Evaluation in Shale Reservoirs. Eng. Pet. 20, 518–526. doi:10.2118/168589-PA
Kumar, S., Das, S., Bastia, R., and Ojha, K. (2018). Mineralogical and Morphological Characterization of Older Cambay Shale from north Cambay Basin, India: Implication for Shale Oil/gas Development. Mar. Pet. Geology. 97, 339–354. doi:10.1016/j.marpetgeo.2018.07.020
Kuster, G. T., and Toksöz, M. N. (1974). Velocity and Attenuation of Seismic Waves in Two‐phase Media: Part I. Theoretical Formulations. Geophysics 39, 587–606. doi:10.1190/1.1440450
Liao, D. L., and Lu, B. P. (2018). An Evaluation Method of Engineering Sweet Spots of Shale Gas Reservoir Development: A Case Study from the Jiaoshiba Gas Field, Sichuan Basin. Nat. Gas Industry 38, 43–50.
Liu, R., Hao, F., Engelder, T., Shu, Z., Yi, J., Xu, S., et al. (2019). Stress Memory Extracted from Shale in the Vicinity of a Fault Zone: Implications for Shale-Gas Retention. Mar. Pet. Geology. 102, 340–349. doi:10.1016/j.marpetgeo.2018.12.047
Liu, R., Hao, F., Engelder, T., Zhu, Z., Yi, J., Xu, S., et al. (2020). Influence of Tectonic Exhumation on Porosity of Wufeng-Longmaxi Shale in the Fuling Gas Field of the Eastern Sichuan Basin, China. Bulletin 104, 939–959. doi:10.1306/08161918071
Liu, R., Jiang, D., Zheng, J., Hao, F., Jing, C., Liu, H., et al. (2021). Stress Heterogeneity in the Changning Shale-Gas Field, Southern Sichuan Basin: Implications for a Hydraulic Fracturing Strategy. Mar. Pet. Geology. 132, 105218. doi:10.1016/j.marpetgeo.2021.105218
Liu, Z., and Song, L. (2017). Wang Changsheng, Sun Ting, Yang Xiaoming and Li Xia. Evaluation Method of the Least Horizontal Principal Stress by Logging Data in Anisotropic Fast Formations[J]. Pet. Exploration Dev. 44, 745–752. doi:10.1016/S1876-3804(17)30089-7
Liu, Z., and Sun, Z. (2015). New Brittleness Indexes and Their Application in Shale/clay Gas Reservoir Prediction[J]. Pet. Exploration Dev. 42, 117–124. doi:10.1016/S1876-3804(15)60016-7
Ma, X. (2021). “Extreme Utilization” Development Theory of Unconventional Natural Gas[J]. Pet. Exploration Dev. 48, 1–11. doi:10.1016/S1876-3804(21)60030-7
Mavko, G., Mukerji, T., and Dvorikin, J. (1998). The Rock Physics Handbook: Tools for Seismic Analysis in Porous Media. Cambridge: Cambridge University Press.
Ou, C., and Li, C. (2017). 3D Discrete Network Modeling of Shale Bedding Fractures Based on Lithofacies Characterization[J]. Pet. Exploration Dev. 44, 309–318. doi:10.1016/S1876-3804(17)30039-3
Ren, H. L., Liu, C. L., Liu, W. P., Yang, X. Y., and Li, Y. W. (2020). Stress Field Simulation and Fracture Development Prediction of the Wufeng Formation- Longmaxi Formation in the Fushun-Yongchuan Block, Sichuan Basin. J. Geomechanics 26, 74–83. doi:10.12090/j.issn.1006-6616.2020.26.01.008
Reuss, A. (1929). Stresses Constant in Composite, Rule of Mixtures for Compliance Components. J. Appl. Maths. Mech. 9, 49–58. doi:10.1002/zamm.19290090104
Rickman, R., Mullen, M. J., Petre, J. E., Grieser, W. V., and Kundert, D. (2008). “A Practical Use of Shale Petrophysics for Stimulation Design Optimization: All Shale Plays Are Not Clones of the Barnett Shale,” in SPE Annual Technical Conference and Exhibition, Denver, Colorado, USA. doi:10.2118/115258-MS21-24 September
Rybacki, E., Meier, T., and Dresen, G. (2016). What Controls the Mechanical Properties of Shale Rocks? - Part II: Brittleness. J. Pet. Sci. Eng. 144, 39–58. doi:10.1016/j.petrol.2016.02.022
Sahu, A., and Das, M. K. (2019). Petrophysical Evaluation of Organic Richness and Brittleness of Shale for Unconventional Hydrocarbon Prospecting: A Case Study on Vadaparru Shale, Krishna Godavari Basin, India. Bahrain. doi:10.15530/AP-URTEC-2019-198280
Shen, C., Ren, L., Zhao, J., Tan, X., and Wu, L. (2017). New Comprehensive index and its Application on Evaluation in Shale Gas Reservoirs: A Case Study of Upper Ordovician Wufeng Formation to Lower Silurian Longmaxi Formation in southeastern Margin of Sichuan Basin. Pet. Exploration Dev. 44, 649–658. doi:10.1016/S1876-3804(17)30078-2
Shen, C., Xie, J., Zhao, J., Fan, Y., and Ren, L. (2021). Whole-life Cycle Countermeasures to Improve the Stimulation Effect of Network Fracturing in Deep Shale Gas Reservoirs of the Southern Sichuan Basin. Nat. Gas Industry 41, 169–177. doi:10.3787/j.issn.1000-0976.2021.01.015
Shen, C., Xie, J., Zhao, J., Fan, Y., and Song, Y. (2020b). Evolution Difference of Fracability of marine Shale Gas Reservoir in Luzhou and West Chongqing Block, Sichuan Basin. J. China Univ. Mining Tech. 49, 736–748. doi:10.13247/j.cnki.jcumt.001135
Shen, C., Zhao, J., Ren, L., and Fan, Y. (2019). A New Fracturing Sweet Spot Identification Method in Longmaxi Formation of Sichuan Basin, SW China. J. Nat. Gas Geosci. 4, 279–286. doi:10.1016/j.jnggs.2019.09.001
Shen, C., Zhao, J., Xie, J., Fan, Y., and Song, Y. (2020a). Target Window Spatial Distribution Prediction Based on Network Fracability: A Case Study of Shale Gas Reservoirs in the Changning Block, Southern Sichuan Basin. J. Geomechanics 26, 881–891.
Sheng, Q., and Li, W. (2016). Evaluation Method of Shale Fracability and its Application in Jiaoshiba Area. Prog. Geophys. 31, 1473–1479.
Tang, X., Xu, S., Zhuang, C., Su, Y., and Chen, X. (2016). Quantitative Evaluation of Rock Brittleness and Fracability Based on Elastic-Wave Velocity Variation Around Borehole. Pet. Exploration Dev. 43, 457–464. doi:10.1016/S1876-3804(16)30053-2
Tang, Y., Xing, Y., Li, L. Z., Zhang, B. H., and Jiang, S. X. (2012). Influence Factors and Evaluation Methods of the Gas Shale Fracability. Earth Sci. Front. 19, 356–363.
Vafaie, A., and Rahimzadeh Kivi, I. (2020). An Investigation on the Effect of thermal Maturity and Rock Composition on the Mechanical Behavior of Carbonaceous Shale Formations. Mar. Pet. Geology. 116, 104315–104413. doi:10.1016/j.marpetgeo.2020.104315
Voigt Crystal physics[M] (1928). Griechische Satzlehre. Leipzig: Teubner, 1–86. doi:10.1007/978-3-663-15957-5_1
Wang, H., Chen, J., Zhang, J., Xie, Q., Wei, Bo., and Zhao, Y. (2016a). A New Method of Fracability Evaluation of Shale Gas Reservoir Based on Weight Allocation. Pet. Drilling Tech. 44, 88–94. doi:10.11911/syztjs.201603016
Wang, S., Yang, H., Zhao, J., Li, N., and Li, Y. (2016b). Research and Application of Comprehensive Evaluation on Fracability of Shale Gas Well. Pet. Geology. Recovery Efficiency 23, 121–126. doi:10.13673/j.cnki.cn37-1359/te.20151106.015
Wei, Y., Qi, Y,, Jia, C,, Jin, Y,, and Yuan, H. (2019). Production Performance and Development Measures for Typical Platform Horizontal wells in the Weiyuan Shale Gas Field, Sichuan Basin. Nat. Gas Industry 39, 81–86. doi:10.3787/j.issn.1000-0976.2019.01.009
Xu, S., White, R. E., and White, (1995). A New Velocity Model for clay‐sand Mixtures 1. Geophys. Prospecting 43, 91–118. doi:10.1111/j.1365-2478.1995.tb00126.x
Yang, Y,, Shi, W,, Zhang, X,, Wang, R,, Xu, X,., Liu, Y,., et al. (2021). Identification Method of Shale Lithofacies by Logging Curves: a Case Study from Wufeng-Longmaxi Formation in Jiaoshiba Area. SW China 33, 135–146. doi:10.12108/yxyqc.20210214
Yi, J., Bao, H., Zheng, A., Zhang, B., Shu, Z., Li, J., et al. (2019). Main Factors Controlling marine Shale Gas Enrichment and High-Yield wells in South China: A Case Study of the Fuling Shale Gas Field. Mar. Pet. Geology. 103, 114–125. doi:10.1016/j.marpetgeo.2019.01.024
Yuan, J., Deng, J., Zhang, D., Li, D., Yan, W., Chen, C., et al. (2013). Fracability Evaluation of Shale-Gas Reservoirs[J]. Acta Petrolei Sinica 34 (3), 356–363. doi:10.11743/ogg20180505
Yuan, Y,, Liu, J., and Zhou, Y. (2018). Brittle-ductile Transition Zone of Shale and its Implications in Shale Gas Exploration. Oil Gas Geology. 39, 899–906. doi:10.11743/ogg20180505
Zhao, J., Shen, C., Ren, L., and Tan, X. (2017). Quantitative Prediction of Gas Contents in Different Occurrence States of Shale Reservoirs: A Case Study of the Jiaoshiba Shale Gasfield in the Sichuan Basin. Nat. Gas Industry 37, 27–33. doi:10.3787/j.issn.1000-0976.2017.04.004
Zhao, J., Ren, L., and Hu, Y. (2013). Controlling Factors of Hydraulic Fractures Extending into Network in Shale Formations. J. Southwest Pet. Univ. (Science Tech. Edition) 35, 1–9.
Zhao, J., RenShen, L. C,, Shen, C., and Li, Y. (2018). Latest Research Progresses in Network Fracturing Theories and Technologies for Shale Gas Reservoirs. Nat. Gas Industry B 5, 533–546. doi:10.1016/j.ngib.2018.03.007
Zhao, J., Xu, W., Li, Y., Hu, J., and Li, J. (2015). A New Method for Fracability Evaluation of Shale-Gas Reservoirs. Nat. Gas Geosci. 26, 1165–1172. doi:10.11764/j.issn.1672-1926.2015.06.1165
Zhong, G., Chen, L., Liao, M., Wang, G., Yang, Y,, and Gao, X. (2020). A Comprehensive Logging Evaluation Method of Shale Gas Reservoir Quality. Nat. Gas Industry 40, 54–60. doi:10.11911/syztjs.2020091
Glossary
VSi, VCa, VClay— the mass fraction of siliceous mineral, carbonate mineral, clay mineral, %
VTotal— the mass fraction of brittle minerals, %
σn— the normal stress on the natural fracture belt, MPa
ω— the dip angle of natural fracture belt, 10° in this study
θ— approximation angle, °
PP— the critical opening pressure in natural fracture belt, MPa
Pn1— the critical net pressure of hydraulic fracture penetrating natural fracture belt, MPa
Pn2— the critical net pressure of hydraulic fracture turning along the natural fracture belt, MPa
To— the tensile strength of natural fracture zone, 3MPa was taken in this study
Δpnf— pressure drop at fracture end, 1MPa is taken in this study
Q+— production dynamic data under the same stimulated intensity, Q30+, Q60+, Q90+, Q180+, Q330+, EUR+
Q— Production dynamic data, Q30, Q60, Q90, Q180, Q330, EUR
l— length of fracturing section of horizontal well, m
Liquid— horizontal well fluid strength, m³/m
Prop— proppant strength of horizontal well, t/m
Pump— horizontal well operation rate, m³/min
E′— dimensionless modulus of elasticity
Edyn— modulus of elasticity, GPa
Edynmax, Edynmin— maximum and minimum modulus of elasticity in sample, GPa
ν′— dimensionless Poisson’s ratio
ν— Poisson’s ratio
νmax, νmin— maximum and minimum Poisson’s ratio in the sample
Pnet— critical net pressure, MPa
Pnet max, Pnet min— the maximum and minimum critical net pressure in the sample, MPa
POR′— dimensionless porosity
POR— porosity, %
PORmax, PORmin— maximum and minimum porosity in sample, %
TOC′— dimensionless total organic carbon content, dimensionless
TOC— total organic carbon content, %
TOCmax, TOCmin— the maximum and minimum total organic carbon content in the sample, %
ΔD— vertical depth difference of horizontal wellbore, m
CF, PF, RF, MF— matrix brittleness potential index, fracture propagation potential index, enrichment & exploration potential index, stimulated potential
Keywords: shale gas, stimulated potential, matrix brittleness, fracture propagation, enrichment and exploitation, main factors, evaluation method, changning shale gasfield
Citation: Shen C, Zhao J, Xie J, Fu Y, Wu J and Ren L (2021) The Main Controlling Factors and Evaluation Method of the Reservoir Stimulation Potential: A Case Study of the Changning Shale Gas Field, Southern Sichuan Basin, SW China. Front. Earth Sci. 9:738668. doi: 10.3389/feart.2021.738668
Received: 09 July 2021; Accepted: 14 September 2021;
Published: 05 November 2021.
Edited by:
Gang Lei, King Fahd University of Petroleum and Minerals, Saudi ArabiaReviewed by:
Kaiqiang Zhang, Imperial College London, United KingdomNai Cao, Sinopec Research Institute of Petroleum Engineering (SRIPE), China
Copyright © 2021 Shen, Zhao, Xie, Fu, Wu and Ren. This is an open-access article distributed under the terms of the Creative Commons Attribution License (CC BY). The use, distribution or reproduction in other forums is permitted, provided the original author(s) and the copyright owner(s) are credited and that the original publication in this journal is cited, in accordance with accepted academic practice. No use, distribution or reproduction is permitted which does not comply with these terms.
*Correspondence: Cheng Shen, c2hlbmNfdmljdG9yQDE2My5jb20=