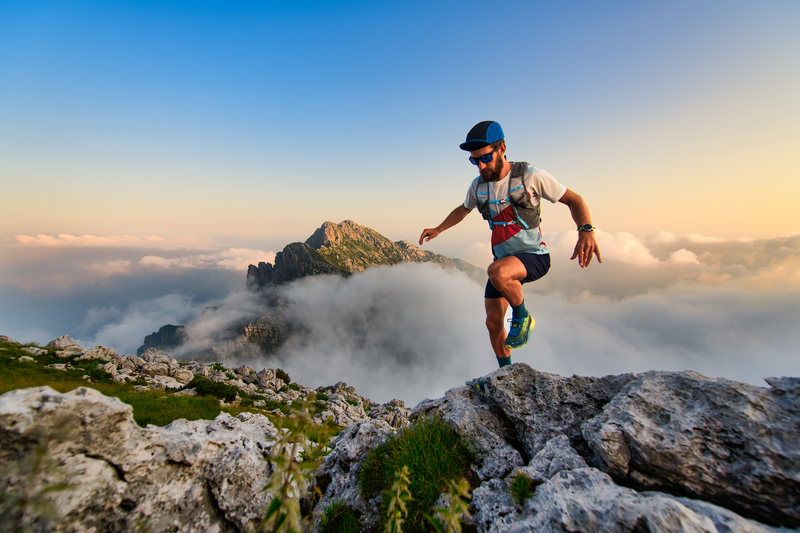
94% of researchers rate our articles as excellent or good
Learn more about the work of our research integrity team to safeguard the quality of each article we publish.
Find out more
ORIGINAL RESEARCH article
Front. Earth Sci. , 08 November 2021
Sec. Atmospheric Science
Volume 9 - 2021 | https://doi.org/10.3389/feart.2021.727169
The sub-daily variability of aerosols affects the estimates of daily mean aerosol loading. However, large spatial scale estimates of their climate effects are mostly based on snapshots from low orbit satellites that may bias the mean estimate for daily, monthly, or annual timescales. In this study, an attempt is made to estimate the magnitude of such bias based on ground and satellite-based datasets. Using ground-based measurements, we show an apparent asymmetry (of the order of 10–50%) in the sub-daily variability of aerosol loading over the Indian region. For the first time, it is reported that this sub-daily variability has a spatial pattern with an increasing amplitude toward the east of the subcontinent. We also find this variability in aerosol loading is well-captured by the satellites but with a lower amplitude. Our study shows that such differences could alter the annual surface radiative forcing estimates by more than ∼15 W m−2 over this region. We find that NASA’s Modern-Era Retrospective analysis for Research and Applications version 2 (MERRA-2), a state-of-the-art model-based chemical reanalysis, is unable to capture these sub-daily variabilities. This implies that both model and satellite-based radiative forcing estimates for large spatial scales should improve aerosol sub-daily information/variabilities for obtaining reliable radiative forcing estimates.
The importance of atmospheric aerosols and their influence on climate are well-known. Atmospheric aerosols can influence climate directly by scattering or absorbing solar radiation (Atwater, 1970; Ensor et al., 1971; Gao et al., 2018) and indirectly by affecting cloud droplet concentrations (Menon et al., 2002; Rotstayn and Lohmann, 2002; Lohmann and Feichter, 2004; Takemura et al., 2005; Panicker et al., 2010; Gu et al., 2012; Dipu et al., 2013; Ning et al., 2015). The presence of absorbing aerosols like Black Carbon (BC) or dust under favorable conditions can change the top of the atmosphere forcing from negative to positive (Babu et al., 2002; Chand et al., 2009; Liu et al., 2018). Numerous studies have reported the change in radiative forcing due to enhanced aerosol concentration over the Indian region (Jayaraman et al., 1998; Ramanathan et al., 2001; Rajeev and Ramanathan, 2001; Babu et al., 2002; Babu and Moorthy, 2002; Vinoj et al., 2004b; Ganguly et al., 2005; Ramachandran et al., 2006; Dey and Tripathi, 2008; Pathak et al., 2010; Vinoj et al., 2010; Tiwari and Singh, 2013; Vinoj et al., 2014; Pathak et al., 2016; Biswas et al., 2017; Panicker et al., 2018). It is shown that even rural and island locations are exposed to high BC concentrations (Vinoj et al., 2010; Rehman et al., 2011; Ravi Kiran et al., 2018). Several studies have reported the rapid increase of aerosol loading over the Indian region (Vinoj et al., 2004b; Sarkar et al., 2006; Kaskaoutis et al., 2012; Ramachandran et al., 2012; Kiran Kumar et al., 2013; Krishna Moorthy et al., 2013; Sreekanth, 2016; Mukherjee et al., 2018).
In addition to the long-term trends and spatial variabilities, aerosols also exhibit diurnal variation. For example, fine-mode aerosols like BC has a typical diurnal variation, depending on the boundary layer characteristics (Babu and Moorthy, 2002; Pant et al., 2006; Dey and Tripathi, 2008; Baxla et al., 2009; Vinoj et al., 2010; Bhat et al., 2017; Ravi Kiran et al., 2018) at the surface. Furthermore, large diurnal variability of column aerosol optical properties (AOP) is reported throughout the world (Smirnov, 2002; Pandithurai et al., 2007; Rana et al., 2009; Mazzola et al., 2010; Zhang et al., 2012; Guo et al., 2017; Zayakhanov et al., 2018) using point observations.
Since the Earth Observation Satellite era, low orbit satellites and their data have provided significant insights into large-scale features of aerosol distribution, transport, and variability at different timescales. However, due to their orbital characteristics, most insights are also based on a few snapshots available within a day. Thus, the daily aerosol loading is estimated from single imagery rather than actual estimates of daily mean through in situ measurement throughout the day. Despite this uncertainty, most aerosol impact assessments on regional and global scales use satellite observations to constrain model simulations. Therefore, the sub-daily variations in aerosol loading could alter the mean aerosol loading estimated from these satellites, inducing significant bias in estimated radiative effects of aerosols (Christopher, 2003; Kassianov et al., 2013; Kuang et al., 2015; Wang et al., 2015; Xu et al., 2016). Insitu observation-based studies have discussed the diurnal variation of column aerosols (Devara et al., 1996; Moorthy et al., 2005; Pandithurai et al., 2007; Rana et al., 2009; Gogoi et al., 2014; Joshi et al., 2016; Mukherjee and Vinoj, 2019) over India. However, the understanding of their spatial extent is limited. Such insights from satellites require data from multiple satellites orbiting at different times within a day or availability of high-quality aerosol retrievals at a regular time interval from geostationary satellites Such aerosols retrievals from the geostationary satellites, like SEVERI (Bernard et al., 2011) and INSAT 3D, offer the opportunity to observe the same area over a long period. However, aerosol retrievals from these platforms have considerable uncertainty (Mishra, 2018). Therefore, under current circumstances, studying the sub-daily variability of aerosols over large spatial scales requires a synergy between the station and multiple satellite measurements. The MODIS (Moderate Resolution Imaging Spectroradiometer) aerosol optical depth (AOD) data are known for its high-quality and long period of data availability (Vinoj et al., 2004b; Prasad et al., 2004; Vinoj et al., 2010; Pandey et al., 2016). Multiple studies have also validated (Vinoj et al., 2004b; Jethva et al., 2007; Dey and Di Girolamo, 2011; Mhawish et al., 2017; Mukherjee and Vinoj, 2019) the aerosol products over this region.
This study attempts to elucidate the sub-daily variability of aerosols using ground and satellite-based aerosol optical depth measurements and their impact on the radiative effect over the Indian region.
The aerosol optical depth (AOD) data at 500 nm were obtained from Aerosol Robotic NETwork (AERONET) version 2 level 1.5 all points datasets for seven stations (Jaipur, Gual Pahari, Nainital, New Delhi, Kanpur, Gandhi College, and Bhola) across the Indo-Gangetic Plains for the period since 2001 depending on their availability (at least more than a year). AERONET provides an extensive network of observatories operating in a similar operating protocol (Holben et al., 1998). The stations use the CIMEL electronique spectral radiometer to generate the spectral data of direct Sun and sky radiances within 340–1,020 and 440–1,020 nm. The accuracy limit of the AOD retrieval lies within ± 0.01 (for λ > 440 nm) to ± 0.02 (for λ < 440 nm) (Holben et al., 1998).
Along with the ground-based observation data, AOD at 550 nm was also obtained from two satellite platforms (NASA’s Terra and Aqua satellites). The satellites use MODIS as a common sensor and utilize a similar algorithm (Dark target & deep blue combined, collection-6, MOD08_D3_v6.1, and MYD_08_D3_v6.1) to retrieve the AOD data. However, the overpass timing of these two satellites is different. Thus, quality-assured level 3 AOD values with a spatial resolution of 1° from two satellites having different overpass times (MODIS Terra at 10:30 IST (5 UTC) and MODIS Aqua at 13:30 IST (8 UTC)) are utilized in this study. The MODIS collection-6 level-2 AOD has a retrieval uncertainty of 0.05 ± 0.15 (Levy et al., 2013). The level 3 data are derived by following strict quality control measures, which substantially reduces the bias of the data.
The inter-satellite difference provides an opportunity to estimate the sub-daily variability in aerosol loading. Therefore, the AOD difference between 10:30 IST and 13:30 IST was calculated (± 15 min averaging window) for ground-based AERONET observations data and is termed AERONET AOD Difference Range (ADR). Similarly, the AOD difference between Terra and Aqua satellite AOD’s is called Satellite AOD Difference Range (SDR).
The annual and seasonal variability of ADR and SDR is computed to show the daytime (hereafter called sub-daily) variation of aerosol loading spatially over the Indian region. The variability of both ADR and SDR is then used to understand their spatial distribution.
Besides the ground-based and satellite observations, model-derived data are also used to understand the aerosol sub-daily variability. For that, NASA’s Modern-Era Retrospective analysis for Research and Applications version 2 (MERRA-2) (Bosilovich, 2015) generated Aerosol Total Extinction at 500 nm is used. The model assimilates several meteorological parameters and NASA’s Goddard Earth Observation System (GEOS-5) model data (Gelaro et al., 2017). The aerosol reanalysis product assimilates aerosol species from Goddard Chemistry Aerosol Radiation and Transport (GOCART) module along with space and ground-based observations (Buchard et al., 2017). The MERRA-2 data are validated with observation (Buchard et al., 2017; Pandey et al., 2017; McCoy et al., 2018; Navinya et al., 2020), and their biases are well-understood.
The relative humidity (RH) data from ECMWF (four times daily) (Dee et al., 2011) are also used in this study. The difference between RH at 06 UTC and 09 UTC is calculated for the annual and seasonal scales based on its temporal proximity to the satellite overpass times.
In the final section, we have calculated the change in aerosol radiative forcing (a lower estimate) due to the sub-daily variability in aerosols. The Aerosol Radiative Forcing Efficiency (ARFE) estimates from AERONET were utilized to estimate the forcing difference. The ARFE or ΔFeff is defined as the rate at which the atmosphere is forced per unit of aerosol optical depth at 550 nm. As the influence of aerosol loading is normalized, the magnitude of ARFE permits understanding the direct radiative effect of different aerosol types characterized by their size distribution and absorption property. The overall uncertainty of ARFE is within 28 ± 30 W m−2 (García et al., 2008). Multiplying AOD with ARFE provides the aerosol radiative forcing. Similarly, multiplying the daily, seasonal/annual averaged ARFE with ADR (ΔAOD from observations) SDR (ΔAOD form satellites) will provide ARF difference due to sub-daily/diurnal variability. These expressions are provided below:
To generate the spatial ARF difference over the Indian region, the lowest minimum ARFE value (by magnitude) among all the stations is used and multiplied with SDR. Therefore, all the values provided in this study are low estimates. A significant assumption made here is that the ARFE is constant spatially. Though this assumption is not entirely correct, this was made to explore the radiative effect that may be attributed only to the aerosol loading and not their composition and differing forcing efficiencies (that vary both spatially and temporally). The ARFE was chosen based on their actual estimates from AERONET seasonally.
The ARF difference calculated from the observed ARFE is the ARF difference generated only because of the AOD differences. For additional confidence, a similar exercise was also carried out using Cloud and the Earth’s Radiant Energy System (CERES) SYN1deg product, containing CERES geostationary (CG) enhanced temporally interpolated short-wave fluxes. SYN1deg data have a systematic bias in the short-wave models (∼15% for daily and ∼6% for monthly data) for global clear-sky cases (Rutan et al., 2015). However, the CG method reduces bias by 20% (Doelling et al., 2013). Monthly 1° data of incoming and outgoing shortwave fluxes are used to calculate the direct radiative forcing map, further divided by the SDR to generate the ARF difference map. CERES data are well-validated at the top of the atmosphere. However, the derived surface data have biases due to noise (Wielicki et al., 2001). Therefore, only the top of the atmosphere (TOA) forcing estimates are used in this study to elucidate forcing differences induced by sub-daily variability in aerosol loading.
Figure 1 shows the diurnal variation of columnar AOD over different AERONET stations over the Indian region. The AOD values for the entire study period are utilized to calculate the diurnal variability. The magnitude of AOD for different stations varied widely. Therefore, for ease of comparison, each hourly mean AOD between 9 AM and 5 PM. is subtracted by the hourly mean AOD at 9 AM. This normalizes the values of AOD at all stations and shows the diurnal variability clearly.
It is found that the AOD over Jaipur has low diurnal variability compared to the other stations. Northern and high-altitude stations like Nainital show an increase in AOD while other stations show a decrease with time. Rana et al. (2009) reported a higher evening time AOD over a northern station, Dehradun, similar to our study. Ground-based observation over Pune has also reported a high evening AOD during pre-monsoon (Pandithurai et al., 2007). Bhaskar et al. (2015) reported low morning and higher afternoon values of AOD over Jodhpur. However, a study conducted over Bhubaneswar reported a fall of 20% in the afternoon AOD value indicating higher morning AOD over the eastern part (Mukherjee and Vinoj, 2019). In terms of actual AOD, the average diurnal variability w.r.to the daily mean increases from west to east. In terms of percentages, Jaipur depicts ∼–20% (w.r.to daily mean) change diurnally while Gandhi College shows ∼+49% change. It is also evident that the morning time AOD becomes higher than the evening, with the most significant differences observed for stations to the east. To further explore the spatial pattern of SDR and ADR, their annual mean from west to east is shown in Figure 2. It may be mentioned that diurnal variability refers to whole-day change. In contrast, sub-daily variability indicates the difference between the 3 h (10:30 AM to 1:30 PM) for SDR and is similarly estimated as ADR. The mean aerosol loading and location of AERONET stations used in this study are shown in Figure 3.
FIGURE 3. Locations of the AERONET stations taken for analysis. The background shows the annual climatology of aerosol optical depth from 2002 to 2019 from MODIS Terra.
It is observed that SDR is always positive, indicating the higher value of Terra AOD (morning), while ADR is negative over the western stations and becomes positive from west to east. Both SDR and ADR show a gradient from west to east. It is not clear why the ground-based stations and satellites show different sub-daily variability in terms of their signs. However, satellites can track spatial gradients reasonably well. The focus of this study was to identify the spatial pattern of sub-daily variability and its radiative effect, if any. Hence, the differences (in sign) between ground and satellite measurements within the day are not explored any further. The similarity in the gradient (west to east) of SDR and ADR provides us the confidence that satellites can track their spatial pattern. A previous study over Hyderabad also indicated this difference (SDR) by comparing the MODIS and Microtops II data (Kharol et al., 2011). Similar results were also obtained over Nainital, Kanpur, and Gandhi College (Choudhry et al., 2012) and Bhubaneswar (Mukherjee and Vinoj, 2019). However, it is now essential to investigate whether this west to east gradient exists on a large spatial domain. Thus, the seasonal variation of SDR is further explored over the Indian region (Figure 4).
FIGURE 4. Variation of SDR in percentages (%) over the Indian region for (A) Winter (DJF), (B) Pre-monsoon (MAM), (C) Monsoon (JJA), (D) Post-monsoon (SON), and (E) Annual. The black dots denote statistical significance at a 95% level.
The seasonal variation of SDR exhibits the same pattern from west to east (more pronounced during winter and pre-monsoon). Annually, the western desert region experiences higher AOD in the afternoon while the eastern coastal parts are higher in the morning, which declines by afternoon. The annual change in SDR lies between ∼5% to the west and increasing to ∼20% in the east (Figure 4E). Figure 4 shows the seasonal and annual variation of SDR in terms of percentages over the Indian region. The difference between the two satellites is significant throughout the study region. SDR during winter (DJF) (Figure 4A) builds a clear gradient from west to east. The gradient is well-pronounced over Indo-Gangetic Plain (IGP). The SDR at the far western part of IGP is ∼5% and increases to ∼20% to the east, similar to the annual pattern. During pre-monsoon season (MAM) (Figure 4B), the gradient still exists though there is a slight shift toward the deserts in the west. There is a possibility that dust activity speeds up with surface heating and convective activities in the afternoon compared to morning over dry arid and desert regions. This may partly explain the large gradient observed around the northwest part of India (adjoining the Thar desert). This gradient is not very clear during the monsoon period (Figure 4C). The monsoon with heavy and uneven rainfall both spatially and temporally may alter these gradients. SON also depicts lower western and higher eastern SDR (Figure 4D) of the magnitude of about 10%.
Besides the west-east gradient, a significant north-south asymmetry can also be observed for the winter and monsoon period (Figures 4A,C). During winter, the southern part of the subcontinent experiences high morning AOD (∼+20% change in SDR) while the northern territory does not show much difference. However, the scenario reverses during monsoon when a negative SDR is observed over southern India, and a weak positive SDR can be observed in the north. The high winds coupled with the presence of semi-arid regions in the rainshadow of the Western Ghats may explain the lower SDR during JJA, an observation similar to the arid deserts in the northwest. On the other hand, DJF, due to the possibility of morning inversion, leads to a significant accumulation of aerosols (east of the Western Ghats) and hence high SDR in the rain shadow regions. Unlike the IGP, these aspects are unexplored for this region and require rigorous investigations.
It should be noted that irrespective of the season, the SDR percentage is always negative or lower at the western part indicating the higher Aqua AOD value in the west region. On the other hand, positive values of SDR represent the high Terra value over the eastern part. It is surprising to witness that two different satellites with only 3 h of difference between their overpass times can experience ∼20% of the change in the AOD value over the eastern Indian region. Thus, the difference may be much higher if the sub-daily variation can be calculated over the east part considering the whole daytime (or the diurnal variability). Therefore, the mean AOD value will be much different from the satellite-derived value. So, viewing satellite data as a daily mean can create a significant difference in radiative forcing estimates.
From the above discussions, it is clear that the sub-daily variation of AOD can be observed from both ground-based and satellite observations with certain limitations. Furthermore, it is explored whether any available model-derived AOD can capture these diurnal variations. The MERRA-2 hourly total extinction data at 550 nm are taken, and the Model AOD Diurnal Range (MDR), similar to SDR percentage, is calculated (Supplementary Figure S1). Our study reveals that MERRA-2 is unable to capture the sub-daily change over the Indian region. Though it captures some positive changes during winter, the amount of change is negligible compared to SDR. As the model cannot capture the diurnal variation of AOD, it is difficult to account for the sub-daily variation to calculate the forcing accurately by these systems.
Understanding the exact reason for this sub-daily variability of aerosols is challenging as AOD depends on several (natural, anthropogenic emissions, and prevailing meteorology) factors. The researchers provided several explanations like long-range transport of particles, sources of pollutants in the surroundings, photochemical conversion of gas to the particles to interpret this sub-daily variability (Devara et al., 1996; Kharol et al., 2011). Based on the ground-based measurements over Pune, Pandithurai et al. (2007) reported that high humidity, calm winds, and low-level inversions could explain the high morning AOD. Therefore, we have explored the relative humidity (RH) changes over the Indian region. Several researchers have already reported the near relation between AOD and RH over different parts of the world (Yoon and Kim, 2006; Altaratz et al., 2013; Brock et al., 2016; Zang et al., 2019; Eck et al., 2020; Li et al., 2020). AOD shows an increase to factors 1.24, 1.51, 2.16, and 3.20 at different RH levels 70, 80, 90, and 95% (Yoon and Kim, 2006). The relationship between relative humidity and aerosols over the Indian subcontinent is also well-established (Pandithurai et al., 1997; Dey and Tripathi, 2007). A recent study reported that the change in mid-tropospheric RH could alter the AOD values (Pandey and Vinoj, 2021). Typically, AOD shows an increase with the rise of relative humidity up to a critical barrier (∼70–80%). The AOD is averaged in bins with each 5% rise of RH (Supplementary Figure S2). As most of the observational stations were located over the Indo-Gangetic Plain, the relation between AOD and RH was studied over the IGP. It is seen that AOD increases with RH of about 75–80% RH. Since the range of RH over the IGP is also expected to be in this range, a similar analysis like SDR was also estimated for RH (Figure 5).
FIGURE 5. The difference in relative humidity (%) (06 UTC—09 UTC) over the Indian region for (A) Winter (DJF), (B) Pre-monsoon (MAM), (C) Monsoon (JJA), (D) Post-monsoon (SON), and (E) Annual. Black dots denote 95% significance level.
It may be noted that the RH difference is primarily positive in all the seasons, which indicates a higher RH percentage in the morning. The pattern of the RH difference shows a clear spatial pattern similar to SDR and ADR. These differences are highly statistically significant. The high morning RH may favor the formation of new particles and deliquescence growth of the existing particles through aqueous chemistry, which can increase morning time AOD values (Yoon and Kim, 2006; Altaratz et al., 2013). For example, it is reported that globally, the RH can explain 20% of the AOD variation (Altaratz et al., 2013). Thus, the relative humidity difference can potentially cause the AOD difference as the day advances. The ADR and SDR are calculated using 10:30 AM IST and 1:30 PM IST data (closest to AOD measurements) while the RH difference is computed using 11:30 AM IST and 2:30 PM IST data. It may be mentioned that these temporal patterns over eastern India may not be possible due to anthropogenic emissions as human activities are expected to peak during the later part of the day.
As discussed, several factors can cause the sub-daily variability of AOD. Detailed studies regarding all these factors can identify the region-specific contributing factor for the ADR/SDR. However, this is beyond the scope of this work and may be subjected to more detailed research with reliable long-term observational data. It may be reiterated that the primary focus of this study is to explore the sub-daily variability of the aerosols over a large spatial scale and its radiative effect. Therefore, the causes of the sub-daily variability are not further explored.
Finally, an attempt is made to estimate the difference in aerosol radiative forcing (both surface and top of the atmosphere) because of the sub-daily variability of aerosols. The intensity of forcing difference due to SDR is explored in the next section.
The annual and seasonal variation of Aerosol Radiative Forcing Efficiency (ARFE) at the bottom of the atmosphere (BOA) for different AERONET stations is calculated to estimate the ARF difference. Annual (Supplementary Figure S3) and seasonal (Figure 6) variation of ARFE (BOA) shows a clear decreasing trend from west to east. The northern stations like New Delhi and Nanital exhibits higher ARFE than the western stations. The monsoon period shows large fluctuation with negligible west to east gradient, perhaps due to the rainfall and subsequent changes to aerosol loading and optical properties (Vinoj et al., 2004b). A recent study has also reported similar seasonal ARFE gradients using four AERONET stations over IGP (Bibi et al., 2017). Though the ARFE at the surface shows a distinct west to east gradient, the ARFE at the top of the atmosphere (Supplementary Figure S4) is not clear. In order to obtain the ARF changes as a consequence of the diurnal or sub-daily variability, the minimum magnitude of ARFE (BOA) within all AERONET stations in India over IGP (Gandhi College) is used. This is done to obtain a minimum estimate of ARF, which these sub-daily variabilities may create. In the real world, the estimates are expected to be much higher. This assumption is a very crude approximation of aerosol property and their distribution across the Indian region. Therefore, CERES-based fluxes are used to calculate ARF differences due to sub-daily variability to obtain a more realistic distribution spatially. It may be noted that the same spatial pattern of SDR is used in all analyses for easy comparison.
FIGURE 6. Variation of ARFE over different AERONET Stations for (A) Winter, (B) Pre-monsoon, (C) Monsoon, and (D) Post-monsoon. The ARFE is defined as the radiative forcing per unit of aerosol optical depth at 550 nm estimated using simple linear regression.
Annually, the ARF difference due to sub-daily variation of AOD over Jaipur is ∼1 W m−2 while at Gandhi College, it increases to ∼5 W m−2. However, seasonally, the Gandhi College site experiences a 16 W m−2 difference during the post-monsoon period.
Annual and seasonal ARF difference (BOA) because of SDR is computed for the Indian region (Figure 7). As we multiplied the SDR with the minimum ARFE value, the ARF difference generated is due to the AOD loading difference (Eq. 4) and not its actual optical property. In this analysis, the annual and seasonal data show a minimum ARF difference in the western part while maximum at the east (primarily due to the high loading difference). Spatially, the ARF difference at the west is ∼6 W m−2 while it increases to ∼20 W m−2 gradually toward the east. For completeness, a similar analysis was carried out for the top of the atmosphere (Supplementary Figure S5). It is surprising to witness that even at the top of the atmosphere, the ARF difference reaches more than 4 W m−2 during winter. Previous studies have already reported that diurnal variation can induce substantial errors in the aerosol radiative forcing calculations and introduces a large bias in estimating direct radiative forcing on regional scales (Christopher, 2003; Arola et al., 2013). Sreekanth et al. (2007) reported that the wintertime ARF at the BOA over Vishakhapattanam is ∼36 W m−2, while at the TOA, it is ∼8.4 W m−2. Several other studies have reported that the ARF over the Bay of Bengal ranges from 15 to 24 W m−2 at the BOA and 2–10 W m−2 at the TOA (Vinoj et al., 2004a; Kedia et al., 2010). Dey and Tripathi (2008) reported that the clear-sky ARF at BOA ranges from −5.8 to −62.3 W m−2 while the TOA forcing ranges from −19.6 to +6.1 W m−2. Thus, though the ARF difference itself appears to be low, they could substantially alter the TOA forcing (up to 50%) with enormous implications for cooling/warming regionally. Thus, we find that the most significant effects due to sub-daily variability happen at the TOA, and in the eastern part of India though, their ARFE is lower than western India. This implies that aerosol loading differences dominate over the eastern Indian region. These ARF differences between BOA and TOA can cause significant changes in atmospheric absorption by more than 10 W m−2, with potential implications to atmospheric heating, convection/circulation, and weather. A 10 W m−2 difference in magnitude for atmospheric radiative effect is almost more than 50% of the overall warming reported for the winter period for the INDOEX campaign (Ramanathan et al., 2001) and highly polluted oceanic regions adjacent Indian sub-continent (Satheesh et al., 2010) and hence a pretty substantial magnitude.
FIGURE 7. Variation of ARF difference (BOA) over Indian region (W°m−2) for (A) Winter (DJF), (B) Pre-monsoon (MAM), (C) Monsoon (JJA), and (D) Post-monsoon (SON) using minimum ARFE.
The gradient of the ARF difference (both BOA and TOA) is more pronounced over IGP. It is already recognized that instantaneous forcing at the surface is linearly proportional to AOD (McComiskey et al., 2008) and related to polluted areas (Li et al., 2010). As IGP is one of the most polluted regions globally, the changes in forcing due to AOD change are more predominant here. Furthermore, whether the results remain the same if an actual ARFE spatial map is used to generate the ARF difference is investigated.
To generate the ARFE map, the Direct Radiating Forcing (DRF) at the top of the atmosphere (TOA) over the Indian region is estimated using CERES short-wave flux. The Direct Radiative Forcing Efficiency (DRFE) has been created by dividing the DRF with satellite-derived AOD. The calculated DRFE is multiplied with SDR to generate the sub-daily forcing difference (TOA) seasonally. From Figure 8, it is clear that even in TOA, the gradient of forcing difference exists, though the values are not high. During winter periods, most of the eastern part experiences more than 1.5 W m−2 difference in forcing at the top of the atmosphere because of aerosol sub-daily variation. In some places, it reaches values up to 3 W m−2.
FIGURE 8. Variation of ARF (W°m−2) difference (TOA) calculated from derived ARFE map over Indian region for (A) Winter (DJF), (B) Pre-monsoon (MAM), (C) Monsoon (JJA), and (D) Post-monsoon (SON) from CERES SW-Flux data.
The aerosol radiative forcing depends on the nature of aerosols and the overall aerosol loading. Besides, the radiative forcing estimations primarily utilize observational data (either from ground-based or satellite observations) of the aerosol loading. The present study depicts that AOD can vary as much as 50% from the daily mean over the eastern part. Satellites can also capture this variability as the AOD difference within 3 h shows more than 20% change in aerosol loading. As discussed earlier, these changes can create significant ARF differences in BOA and TOA (∼50%). The long-term change in aerosol radiative forcing impacts the local climate by either warming or cooling the atmosphere, altering the wind circulation and precipitation pattern. Therefore, it is essential to estimate the spatial patterns of forcing precisely. It may be mentioned that the sub-daily variability of aerosols is a global phenomenon (Smirnov, 2002; Mazzola et al., 2010; Zhang et al., 2012; Guo et al., 2017; Zayakhanov et al., 2018). A recent study over South Korea shows that AOD can vary up to 20–30% in the inland sites and ± 20% in the coastal areas (Lennartson et al., 2018).
This study thus shows that ARF estimations without incorporating proper diurnal variability can induce substantial errors in the spatial distribution of forcing regionally and globally, modifying their climate effects.
The study reveals a distinct sub-daily variability and gradient of AOD from the west to the east of the Indian subcontinent, reaching up to ∼50% using ground-based measurements. Similar analysis using satellite datasets reveals spatially such estimates could be ∼20% (even within a short period of 3 h). These differences appear to be driven in part by the gradient in relative humidity. This high sub-daily variability over eastern India creates a significant forcing difference of ∼15 W m−2 at the surface while over 1.5 W m−2 at the TOA. Thus, the non-accountability of aerosol sub-daily variations can lead to substantial error in diurnally averaged or daily, seasonal, or annual forcing estimates. Interestingly, even the state-of-the-art model MERRA-2 is unable to capture this sub-daily variation of AOD. In a region like the Indian subcontinent, where the aerosol loading is very high, such a difference can substantially alter daily and, hence, long-term forcing estimates, thereby biasing the aerosol climate effects.
Publicly available datasets were analyzed in this study. This data can be found here: https://ladsweb.modaps.eosdis.nasa.gov; https://aeronet.gsfc.nasa.gov; http://ceres.larc.nasa.gov; https://apps.ecmwf.int/datasets/data/interim-full-daily/levtype=pl.
TM: conceptualization; data curation; methodology; formal analysis; writing–original draft. VV: conceptualization; supervision; methodology; writing–review and editing.
The authors declare that the research was conducted in the absence of any commercial or financial relationships that could be construed as a potential conflict of interest.
All claims expressed in this article are solely those of the authors and do not necessarily represent those of their affiliated organizations, or those of the publisher, the editors and the reviewers. Any product that may be evaluated in this article, or claim that may be made by its manufacturer, is not guaranteed or endorsed by the publisher.
The authors acknowledge the Indian Space Research Organization (ISRO) for supporting this work through its Aerosol Radiative Forcing over India (ARFI) Project. The authors acknowledge NASA for providing various datasets used in this study, including MODIS Terra, Aqua, CERES, and MERRA-2 reanalysis through the GIOVANNI portal. The authors also like to acknowledge AERONET for providing ground-based measurements. The authors acknowledge ECMWF for providing the reanalysis data.
The Supplementary Material for this article can be found online at: https://www.frontiersin.org/articles/10.3389/feart.2021.727169/full#supplementary-material
Altaratz, O., Bar-Or, R. Z., Wollner, U., and Koren, I. (2013). Relative Humidity and its Effect on Aerosol Optical Depth in the Vicinity of Convective Clouds. Environ. Res. Lett. 8, 034025. doi:10.1088/1748-9326/8/3/034025
Arola, A., Eck, T. F., Huttunen, J., Lehtinen, K. E. J., Lindfors, A. V., Myhre, G., et al. (2013). Influence of Observed Diurnal Cycles of Aerosol Optical Depth on Aerosol Direct Radiative Effect. Atmos. Chem. Phys. 13, 7895–7901. doi:10.5194/acp-13-7895-2013
Atwater, M. A. (1970). Planetary Albedo Changes Due to Aerosols. Science 170, 64–66. doi:10.1126/science.170.3953.64
Babu, S. S., and Moorthy, K. K. (2002). Aerosol Black Carbon Over a Tropical Coastal Station in India. Geophys. Res. Lett. 29, 13-1–13–4. doi:10.1029/2002GL015662
Babu, S. S., Satheesh, S. K., and Moorthy, K. K. (2002). Aerosol Radiative Forcing Due to Enhanced Black Carbon at an Urban Site in India. Geophys. Res. Lett. 29, 27-1–27–4. doi:10.1029/2002GL015826
Baxla, S. P., Roy, A. A., Gupta, T., Tripathi, S. N., and Bandyopadhyaya, R. (2009). Analysis of Diurnal and Seasonal Variation of Submicron Outdoor Aerosol Mass and Size Distribution in a Northern Indian City and its Correlation to Black Carbon. Aerosol Air Qual. Res. 9, 458–469. doi:10.4209/aaqr.2009.03.0017
Bernard, E., Moulin, C., Ramon, D., Jolivet, D., Riedi, J., and Nicolas, J.-M. (2011). Description and Validation of an AOT Product over Land at the 0.6 μm Channel of the SEVIRI Sensor Onboard MSG. Atmos. Meas. Tech. 4, 2543–2565. doi:10.5194/amt-4-2543-2011
Bhaskar, V. V., Safai, P. D., and Raju, M. P. (2015). Long Term Characterization of Aerosol Optical Properties: Implications for Radiative Forcing over the Desert Region of Jodhpur, India. Atmos. Environ. 114, 66–74. doi:10.1016/j.atmosenv.2015.05.043
Bhat, M. A., Romshoo, S. A., and Beig, G. (2017). Aerosol Black Carbon at an Urban Site-Srinagar, Northwestern Himalaya, India: Seasonality, Sources, Meteorology and Radiative Forcing. Atmos. Environ. 165, 336–348. doi:10.1016/j.atmosenv.2017.07.004
Bibi, H., Alam, K., and Bibi, S. (2017). Estimation of Shortwave Direct Aerosol Radiative Forcing at Four Locations on the Indo-Gangetic plains: Model Results and Ground Measurement. Atmos. Environ. 163, 166–181. doi:10.1016/j.atmosenv.2017.05.043
Biswas, J., Pathak, B., Patadia, F., Bhuyan, P. K., Gogoi, M. M., and Babu, S. S. (2017). Satellite-retrieved Direct Radiative Forcing of Aerosols over North-East India and Adjoining Areas: Climatology and Impact Assessment. Int. J. Climatol 37, 298–317. doi:10.1002/joc.5004
Bosilovich, M. (2015). In Technical Report Series on Global Modeling and Data Assimilation NASA/TM–2015-104606 43. 145.
Brock, C. A., Wagner, N. L., Anderson, B. E., Beyersdorf, A., Campuzano-Jost, P., Day, D. A., et al. (2016). Aerosol Optical Properties in the southeastern United States in Summer - Part 2: Sensitivity of Aerosol Optical Depth to Relative Humidity and Aerosol Parameters. Atmos. Chem. Phys. 16, 5009–5019. doi:10.5194/acp-16-5009-2016
Buchard, V., Randles, C. A., da Silva, A. M., Darmenov, A., Colarco, P. R., Govindaraju, R., et al. (2017). The MERRA-2 Aerosol Reanalysis, 1980 Onward. Part II: Evaluation and Case Studies. J. Clim. 30, 6851–6872. doi:10.1175/JCLI-D-16-0613.1
Chand, D., Wood, R., Anderson, T. L., Satheesh, S. K., and Charlson, R. J. (2009). Satellite-Derived Direct Radiative Effect of Aerosols Dependent on Cloud Cover. Nat. Geosci 2, 181–184. doi:10.1038/ngeo437
Choudhry, P., Misra, A., and Tripathi, S. N. (2012). Study of MODIS Derived AOD at Three Different Locations in the Indo Gangetic Plain: Kanpur, Gandhi College and Nainital. Ann. Geophys. 30, 1479–1493. doi:10.5194/angeo-30-1479-2012
Christopher, S. A. (2003). Estimation of Diurnal Shortwave Dust Aerosol Radiative Forcing during PRIDE. J. Geophys. Res. 108, 8596. doi:10.1029/2002JD002787
Dee, D. P., Uppala, S. M., Simmons, A. J., Berrisford, P., Poli, P., Kobayashi, S., et al. (2011). The ERA-Interim Reanalysis: Configuration and Performance of the Data Assimilation System. Q.J.R. Meteorol. Soc. 137, 553–597. doi:10.1002/qj.828
Devara, P. C. S., Pandithurai, G., Raj, P. E., and Sharma, S. (1996). Investigations of Aerosol Optical Depth Variations Using Spectroradiometer at an Urban Station, Pune, India. J. Aerosol Sci. 27, 621–632. doi:10.1016/0021-8502(95)00550-1
Dey, S., and Di Girolamo, L. (2011). A Decade of Change in Aerosol Properties over the Indian Subcontinent. Geophys. Res. Lett. 38, L14811. doi:10.1029/2011GL048153
Dey, S., and Tripathi, S. N. (2008). Aerosol Direct Radiative Effects over Kanpur in the Indo-Gangetic basin, Northern India: Long-Term (2001-2005) Observations and Implications to Regional Climate. J. Geophys. Res. 113, 1–20. doi:10.1029/2007JD009029
Dey, S., and Tripathi, S. N. (2007). Estimation of Aerosol Optical Properties and Radiative Effects in the Ganga basin, Northern India, during the Wintertime. J. Geophys. Res. 112, 1–16. doi:10.1029/2006JD007267
Dipu, S., Prabha, T. V., Pandithurai, G., Dudhia, J., Pfister, G., Rajesh, K., et al. (2013). Impact of Elevated Aerosol Layer on the Cloud Macrophysical Properties Prior to Monsoon Onset. Atmos. Environ. 70, 454–467. doi:10.1016/J.ATMOSENV.2012.12.036
Doelling, D. R., Loeb, N. G., Keyes, D. F., Nordeen, M. L., Morstad, D., Nguyen, C., et al. (2013). Geostationary Enhanced Temporal Interpolation for ceres Flux Products. J. Atmos. Ocean. Technol. 30, 1072–1090. doi:10.1175/JTECH-D-12-00136.1
Eck, T. F., Holben, B. N., Kim, J., Beyersdorf, A. J., Choi, M., Lee, S., et al. (2020). Influence of Cloud, Fog, and High Relative Humidity during Pollution Transport Events in South Korea: Aerosol Properties and PM2.5 Variability. Atmos. Environ. 232, 117530. doi:10.1016/j.atmosenv.2020.117530
Ensor, D. S., Porch, W. M., Pilat, M. J., Charlson, R. J., Ensor, D. S., Porch, W. M., et al. (1971). Influence of the Atmospheric Aerosol on Albedo. J. Appl. Meteorol. 10, 1303–1306. doi:10.1175/1520-0450(1971)010<1303:iotaao>2.0.co;2
Ganguly, D., Gadhavi, H., Jayaraman, A., Rajesh, T. A., and Misra, A. (2005). Single Scattering Albedo of Aerosols Over the Central India: Implications for the Regional Aerosol Radiative Forcing. Geophys. Res. Lett. 32, L18803. doi:10.1029/2005GL023903
Gao, J., Zhu, B., Xiao, H., Kang, H., Pan, C., Wang, D., et al. (2018). Effects of Black Carbon and Boundary Layer Interaction on Surface Ozone in Nanjing, China. Atmos. Chem. Phys. 18, 7081–7094. doi:10.5194/acp-18-7081-2018
García, O. E., Díaz, A. M., Expósito, F. J., Díaz, J. P., Dubovik, O., Dubuisson, P., et al. (2008). Validation of AERONET Estimates of Atmospheric Solar Fluxes and Aerosol Radiative Forcing by Ground-Based Broadband Measurements. J. Geophys. Res. 113, 1–16. doi:10.1029/2008JD010211
Gelaro, R., McCarty, W., Suárez, M. J., Todling, R., Molod, A., Takacs, L., et al. (2017). The Modern-Era Retrospective Analysis for Research and Applications, Version 2 (MERRA-2). J. Clim. 30, 5419–5454. doi:10.1175/JCLI-D-16-0758.1
Gogoi, M. M., Moorthy, K. K., Kompalli, S. K., Chaubey, J. P., Babu, S. S., Manoj, M. R., et al. (2014). Physical and Optical Properties of Aerosols in a Free Tropospheric Environment: Results from Long-Term Observations over Western Trans-himalayas. Atmos. Environ. 84, 262–274. doi:10.1016/j.atmosenv.2013.11.029
Gu, Y., Liou, K. N., Jiang, J. H., Su, H., and Liu, X. (2012). Dust Aerosol Impact on North Africa Climate: a GCM Investigation of Aerosol-Cloud-Radiation Interactions Using A-Train Satellite Data. Atmos. Chem. Phys. 12, 1667–1679. doi:10.5194/acp-12-1667-2012
Guo, J., Xia, F., Zhang, Y., Liu, H., Li, J., Lou, M., et al. (2017). Impact of Diurnal Variability and Meteorological Factors on the PM2.5 - AOD Relationship: Implications for PM2.5 Remote Sensing. Environ. Pollut. 221, 94–104. doi:10.1016/j.envpol.2016.11.043
Holben, B. N., Eck, T. F., Slutsker, I., Tanré, D., Buis, J. P., Setzer, A., et al. (1998). AERONET-A Federated Instrument Network and Data Archive for Aerosol Characterization. Remote Sens. Environ. 66, 1–16. doi:10.1016/S0034-4257(98)00031-5
Jayaraman, A., Lubin, D., Ramachandran, S., Ramanathan, V., Woodbridge, E., Collins, W. D., et al. (1998). Direct Observations of Aerosol Radiative Forcing over the Tropical Indian Ocean during the January-February 1996 Pre-INDOEX Cruise. J. Geophys. Res. 103, 13827–13836. doi:10.1029/98jd00559
Jethva, H., Satheesh, S. K., and Srinivasan, J. (2007). Assessment of Second-Generation MODIS Aerosol Retrieval (Collection 005) at Kanpur, India. Geophys. Res. Lett. 34, 1–5. doi:10.1029/2007GL029647
Joshi, H., Naja, M., Singh, K. P., Kumar, R., Bhardwaj, P., Babu, S. S., et al. (2016). Investigations of Aerosol Black Carbon from a Semi-Urban Site in the Indo-Gangetic Plain Region. Atmos. Environ. 125, 346–359. doi:10.1016/j.atmosenv.2015.04.007
Kaskaoutis, D. G., Singh, R. P., Gautam, R., Sharma, M., Kosmopoulos, P. G., and Tripathi, S. N. (2012). Variability and Trends of Aerosol Properties over Kanpur, Northern India Using AERONET Data (2001-10). Environ. Res. Lett. 7, 024003. doi:10.1088/1748-9326/7/2/024003
Kassianov, E., Barnard, J., Pekour, M., Berg, L. K., Michalsky, J., Lantz, K., et al. (2013). Do diurnal Aerosol Changes Affect Daily Average Radiative Forcing. Geophys. Res. Lett. 40, 3265–3269. doi:10.1002/grl.50567
Kedia, S., Ramachandran, S., Kumar, A., and Sarin, M. M. (2010). Spatiotemporal Gradients in Aerosol Radiative Forcing and Heating Rate over Bay of Bengal and Arabian Sea Derived on the Basis of Optical, Physical, and Chemical Properties. J. Geophys. Res. 115, 1–17. doi:10.1029/2009JD013136
Kharol, S. K., Badarinath, K. V. S., Sharma, A. R., Kaskaoutis, D. G., and Kambezidis, H. D. (2011). Multiyear Analysis of Terra/Aqua MODIS Aerosol Optical Depth and Ground Observations over Tropical Urban Region of Hyderabad, India. Atmos. Environ. 45, 1532–1542. doi:10.1016/j.atmosenv.2010.12.047
Kiran Kumar, T., Gadhavi, H., Jayaraman, A., Sai Suman, M. N., and Vijaya Bhaskara Rao, S. (2013). Temporal and Spatial Variability of Aerosol Optical Depth over South India as Inferred from MODIS. J. Atmos. Solar-Terrestrial Phys. 94, 71–80. doi:10.1016/j.jastp.2012.12.010
Krishna Moorthy, K., Suresh Babu, S., Manoj, M. R., and Satheesh, S. K. (2013). Buildup of Aerosols over the Indian Region. Geophys. Res. Lett. 40, 1011–1014. doi:10.1002/grl.50165
Kuang, Y., Zhao, C. S., Tao, J. C., and Ma, N. (2015). Diurnal Variations of Aerosol Optical Properties in the North China Plain and Their Influences on the Estimates of Direct Aerosol Radiative Effect. Atmos. Chem. Phys. 15, 5761–5772. doi:10.5194/acp-15-5761-2015
Lennartson, E. M., Wang, J., Gu, J., Castro Garcia, L., Ge, C., Gao, M., et al. (2018). Diurnal Variation of Aerosol Optical Depth and PM2.5 in South Korea: a Synthesis from AERONET, Satellite (GOCI), KORUS-AQ Observation, and the WRF-Chem Model. Atmos. Chem. Phys. 18, 15125–15144. doi:10.5194/acp-18-15125-2018
Levy, R. C., Mattoo, S., Munchak, L. A., Remer, L. A., Sayer, A. M., Patadia, F., et al. (2013). The Collection 6 MODIS Aerosol Products Over Land and Ocean. Atmos. Meas. Tech. 6, 2989–3034. doi:10.5194/amt-6-2989-2013
Li, L., Li, Q., Huang, L., Wang, Q., Zhu, A., Xu, J., et al. (2020). Air Quality Changes during the COVID-19 Lockdown over the Yangtze River Delta Region: An Insight into the Impact of Human Activity Pattern Changes on Air Pollution Variation. Sci. Total Environ. 732, 139282. doi:10.1016/j.scitotenv.2020.139282
Li, Z., Lee, K.-H., Wang, Y., Xin, J., and Hao, W.-M. (2010). First Observation-Based Estimates of Cloud-free Aerosol Radiative Forcing across China. J. Geophys. Res. 115, 1–9. doi:10.1029/2009JD013306
Liu, L., Shawki, D., Voulgarakis, A., Kasoar, M., Samset, B. H., Myhre, G., et al. (2018). A PDRMIP Multimodel Study on the Impacts of Regional Aerosol Forcings on Global and Regional Precipitation. J. Clim. 31, 4429–4447. doi:10.1175/JCLI-D-17-0439.1
Lohmann, U., and Feichter, J. (2004). Global Indirect Aerosol Effects: a Review. Atmos. Chem. Phys. Discuss. 4, 7561–7614. doi:10.5194/acpd-4-7561-2004
Mazzola, M., Lanconelli, C., Lupi, A., Busetto, M., Vitale, V., and Tomasi, C. (2010). Columnar Aerosol Optical Properties in the Po Valley, Italy, from MFRSR Data. J. Geophys. Res. 115, 1–17. doi:10.1029/2009JD013310
McComiskey, A., Schwartz, S. E., Schmid, B., Guan, H., Lewis, E. R., Ricchiazzi, P., et al. (2008). Direct Aerosol Forcing: Calculation from Observables and Sensitivities to Inputs. J. Geophys. Res. 113, 1–16. doi:10.1029/2007JD009170
McCoy, D. T., Bender, F. A.-M., Grosvenor, D. P., Mohrmann, J. K., Hartmann, D. L., Wood, R., et al. (2018). Predicting Decadal Trends in Cloud Droplet Number Concentration Using Reanalysis and Satellite Data. Atmos. Chem. Phys. 18, 2035–2047. doi:10.5194/acp-18-2035-2018
Menon, S., Genio, A. D. D., Koch, D., and Tselioudis, G. (2002). GCM Simulations of the Aerosol Indirect Effect: Sensitivity to Cloud Parameterization and Aerosol Burden. J. Atmos. Sci. 59, 692–713. doi:10.1175/1520-0469(2002)059<0692:gsotai>2.0.co;2
Mhawish, A., Banerjee, T., Broday, D. M., Misra, A., and Tripathi, S. N. (2017). Evaluation of MODIS Collection 6 Aerosol Retrieval Algorithms over Indo-Gangetic Plain: Implications of Aerosols Types and Mass Loading. Remote Sens. Environ. 201, 297–313. doi:10.1016/j.rse.2017.09.016
Mishra, M. K. (2018). Retrieval of Aerosol Optical Depth from INSAT-3D Imager over Asian Landmass and Adjoining Ocean: Retrieval Uncertainty and Validation. J. Geophys. Res. Atmos. 123, 5484–5508. doi:10.1029/2017JD028116
Moorthy, K. K., Babu, S. S., and Satheesh, S. K. (2005). Aerosol Characteristics and Radiative Impacts over the Arabian Sea during the Intermonsoon Season: Results from ARMEX Field Campaign. J. Atmos. Sci. 62, 192–206. doi:10.1175/JAS-3378.1
Mukherjee, T., Asutosh, A., Pandey, S. K., Yang, L., Gogoi, P. P., Panwar, A., et al. (2018). Increasing Potential for Air Pollution over Megacity New Delhi: A Study Based on 2016 Diwali Episode. Aerosol Air Qual. Res. 18, 2510–2518. doi:10.4209/aaqr.2017.11.0440
Mukherjee, T., and Vinoj, V. (2019). Atmospheric Aerosol Optical Depth and its Variability over an Urban Location in Eastern India. Nat. Hazards 102, 591–605. doi:10.1007/s11069-019-03636-x
Navinya, C. D., Vinoj, V., and Pandey, S. K. (2020). Evaluation of PM2.5 Surface Concentrations Simulated by NASA’s MERRA Version 2 Aerosol Reanalysis over India and its Relation to the Air Quality Index. Aerosol Air Qual. Res. 20, 1329–1339. doi:10.4209/aaqr.2019.12.0615
Ning, H., Li-juan, L., and Bin, W. (2015). The Role of the Aerosol Indirect Effect in the Northern Indian Ocean Warming Simulated by CMIP5 Models. Atmos. Oceanic Sci. Lett. 7, 411–416. doi:10.1080/16742834.2014.11447199
Pandey, S. K., Bakshi, H., and Vinoj, V. (2016). Recent Changes in Dust and its Impact on Aerosol Trends over the Indo-Gangetic Plain (IGP). Remote Sensing of the Atmosphere, Clouds, and Precipitation VI, 98761Z. doi:10.1117/12.2223314
Pandey, S. K., Vinoj, V., Landu, K., and Babu, S. S. (2017). Declining Pre-monsoon Dust Loading over South Asia: Signature of a Changing Regional Climate. Sci. Rep. 7, 1–10. doi:10.1038/s41598-017-16338-w
Pandey, S. K., and Vinoj, V. (2021). Surprising Changes in Aerosol Loading over india amid Covid-19 Lockdown. Aerosol Air Qual. Res. 21, 200466. doi:10.4209/aaqr.2020.07.0466
Pandithurai, G., Devara, P. C. S., Maheskumar, R. S., Raj, P. E., and Dani, K. K. (1997). Spectral Characteristics of Urban Aerosols and Their Association with Relative Humidity. Atmos. Res. 45, 109–122. doi:10.1016/S0169-8095(97)00033-1
Pandithurai, G., Pinker, R. T., Devara, P. C. S., Takamura, T., and Dani, K. K. (2007). Seasonal Asymmetry in Diurnal Variation of Aerosol Optical Characteristics over Pune, Western India. J. Geophys. Res. 112, 1–9. doi:10.1029/2006JD007803
Panicker, A. S., Aditi, R., Beig, G., Ali, K., and Solmon, F. (2018). Radiative Forcing of Carbonaceous Aerosols over Two Urban Environments in Northern India. Aerosol Air Qual. Res. 18, 884–894. doi:10.4209/aaqr.2017.01.0056
Panicker, A. S., Pandithurai, G., and Dipu, S. (2010). Aerosol Indirect Effect during Successive Contrasting Monsoon Seasons over Indian Subcontinent Using MODIS Data. Atmos. Environ. 44, 1937–1943. doi:10.1016/J.ATMOSENV.2010.02.015
Pant, P., Hegde, P., Dumka, U. C., Sagar, R., Satheesh, S. K., Moorthy, K. K., et al. (2006). Aerosol Characteristics at a High-Altitude Location in central Himalayas: Optical Properties and Radiative Forcing. J. Geophys. Res. 111, 1–9. doi:10.1029/2005JD006768
Pathak, B., Kalita, G., Bhuyan, K., Bhuyan, P. K., and Moorthy, K. K. (2010). Aerosol Temporal Characteristics and its Impact on Shortwave Radiative Forcing at a Location in the Northeast of India. J. Geophys. Res. 115, 1–14. doi:10.1029/2009JD013462
Pathak, B., Subba, T., Dahutia, P., Bhuyan, P. K., Moorthy, K. K., Gogoi, M. M., et al. (2016). Aerosol Characteristics in north-east India Using ARFINET Spectral Optical Depth Measurements. Atmos. Environ. 125, 461–473. doi:10.1016/j.atmosenv.2015.07.038
Prasad, A. K., Singh, R. P., and Singh, A. (2004). Variability of Aerosol Optical Depth over Indian Subcontinent Using MODIS Data. J. Indian Soc. Remote Sens. 32, 313–316. doi:10.1007/BF03030855
Rajeev, K., and Ramanathan, V. (2001). Direct Observations of clear-sky Aerosol Radiative Forcing from Space during the Indian Ocean Experiment. J. Geophys. Res. 106, 17221–17235. doi:10.1029/2000JD900723
Ramachandran, S., Kedia, S., and Srivastava, R. (2012). Aerosol Optical Depth Trends over Different Regions of India. Atmos. Environ. 49, 338–347. doi:10.1016/j.atmosenv.2011.11.017
Ramachandran, S., Rengarajan, R., Jayaraman, A., Sarin, M. M., and Das, S. K. (2006). Aerosol Radiative Forcing During Clear, Hazy, and Foggy Conditions Over a Continental Polluted Location in north India. J. Geophys. Res. 111, 1–12. doi:10.1029/2006JD007142
Ramanathan, V., Crutzen, P. J., Lelieveld, J., Mitra, A. P., Althausen, D., Anderson, J., et al. (2001). Indian Ocean Experiment: An Integrated Analysis of the Climate Forcing and Effects of the Great Indo-Asian Haze. J. Geophys. Res. 106, 28371–28398. doi:10.1029/2001JD900133
Rana, S., Kant, Y., and Dadhwal, V. K. (2009). Diurnal and Seasonal Variation of Spectral Properties of Aerosols over Dehradun, India. Aerosol Air Qual. Res. 9, 32–49. doi:10.4209/aaqr.2008.06.0019
Ravi Kiran, V., Talukdar, S., Venkat Ratnam, M., and Jayaraman, A. (2018). Long-term Observations of Black Carbon Aerosol Over a Rural Location in Southern Peninsular India: Role of Dynamics and Meteorology. Atmos. Environ. 189, 264–274. doi:10.1016/j.atmosenv.2018.06.020
Rehman, I. H., Ahmed, T., Praveen, P. S., Kar, A., and Ramanathan, V. (2011). Black Carbon Emissions from Biomass and Fossil Fuels in Rural India. Atmos. Chem. Phys. 11, 7289–7299. doi:10.5194/acp-11-7289-2011
Rotstayn, L. D., and Lohmann, U. (2002). Tropical Rainfall Trends and the Indirect Aerosol Effect. J. Clim. 15, 2103–2116. doi:10.1175/1520-0442(2002)015<2103:trtati>2.0.co;2
Rutan, D. A., Kato, S., Doelling, D. R., Rose, F. G., Nguyen, L. T., Caldwell, T. E., et al. (2015). CERES Synoptic Product: Methodology and Validation of Surface Radiant Flux. J. Atmos. Ocean. Technol. 32, 1121–1143. doi:10.1175/JTECH-D-14-00165.1
Sarkar, S., Chokngamwong, R., Cervone, G., Singh, R. P., and Kafatos, M. (2006). Variability of Aerosol Optical Depth and Aerosol Forcing over India. Adv. Space Res. 37, 2153–2159. doi:10.1016/j.asr.2005.09.043
Satheesh, S. K., Vinoj, V., and Krishnamoorthy, K. (2010). Assessment of Aerosol Radiative Impact over Oceanic Regions Adjacent to Indian Subcontinent Using Multisatellite Analysis. Adv. Meteorol. 2010, 1–13. doi:10.1155/2010/139186
Smirnov, a., Holben, B. N., Eck, T. F., Slutsker, I., Chatenet, B., and Pinker, R. T. (2002). Diurnal Variability of Aerosol Optical Depth Observed at AERONET (Aerosol Robotic Network) Sites. Geophys. Res. Lett. 29, 30–31. doi:10.1029/2002GL016305
Sreekanth, V. (2016). Discussion on Linear Long-Term Trends in Aerosol and Cloud Properties over India and its Surrounding Waters. Adv. Space Res. 57, 2104–2114. doi:10.1016/j.asr.2016.02.015
Sreekanth, V., Niranjan, K., and Madhavan, B. L. (2007). Radiative Forcing of Black Carbon over Eastern India. Geophys. Res. Lett. 34, 1–5. doi:10.1029/2007GL030377
Takemura, T., Nozawa, T., Emori, S., Nakajima, T. Y., and Nakajima, T. (2005). Simulation of Climate Response to Aerosol Direct and Indirect Effects with Aerosol Transport-Radiation Model. J. Geophys. Res. 110, 1–16. doi:10.1029/2004JD005029
Tiwari, S., and Singh, A. K. (2013). Variability of Aerosol Parameters Derived from Ground and Satellite Measurements over Varanasi Located in the Indo-Gangetic Basin. Aerosol Air Qual. Res. 13, 627–638. doi:10.4209/aaqr.2012.06.0162
Vinoj, V., Babu, S. S., Satheesh, S. K., Moorthy, K. K., and Kaufman, Y. J. (2004a). Radiative Forcing by Aerosols over the Bay of Bengal Region Derived from Shipborne, Island-Based, and Satellite (Moderate-Resolution Imaging Spectroradiometer) Observations. J. Geophys. Res. 109, 1–11. doi:10.1029/2003jd004329
Vinoj, V., Rasch, P. J., Wang, H., Yoon, J.-H., Ma, P.-L., Landu, K., et al. (2014). Short-Term Modulation of Indian Summer Monsoon Rainfall by West Asian Dust. Nat. Geosci. 7, 308–313. doi:10.1038/NGEO2107
Vinoj, V., Satheesh, S. K., and Moorthy, K. K. (2010). Optical, Radiative, and Source Characteristics of Aerosols at Minicoy, a Remote Island in the Southern Arabian Sea. J. Geophys. Res. 115, 1–19. doi:10.1029/2009JD011810
Vinoj, V., Satheesh, S. K., Suresh Babu, S., and Krishna Moorthy, K. (2004b). Large Aerosol Optical Depths Observed at an Urban Location in Southern India Associated with Rain-Deficit Summer Monsoon Season. Ann. Geophys. 22, 3073–3077. doi:10.5194/angeo-22-3073-2004
Wang, Z., Liu, D., Wang, Y., Wang, Z., and Shi, G. (2015). Diurnal Aerosol Variations Do Affect Daily Averaged Radiative Forcing under Heavy Aerosol Loading Observed in Hefei, China. Atmos. Meas. Tech. 8, 2901–2907. doi:10.5194/amt-8-2901-2015
Wielicki, B., Charlock, T., Haeffelin, M., Kratz, D., Loeb, N., and Minnis, P. (2001). CERES Validation Summary.
Xu, H., Guo, J., Ceamanos, X., Roujean, J.-L., Min, M., and Carrer, D. (2016). On the Influence of the Diurnal Variations of Aerosol Content to Estimate Direct Aerosol Radiative Forcing Using MODIS Data. Atmos. Environ. 141, 186–196. doi:10.1016/j.atmosenv.2016.06.067
Yoon, S.-C., and Kim, J. (2006). Influences of Relative Humidity on Aerosol Optical Properties and Aerosol Radiative Forcing during ACE-Asia. Atmos. Environ. 40, 4328–4338. doi:10.1016/j.atmosenv.2006.03.036
Zang, L., Wang, Z., Zhu, B., and Zhang, Y. (2019). Roles of Relative Humidity in Aerosol Pollution Aggravation over central China during Wintertime. Int. J. Environ. Res. Public Health 16, 4422. doi:10.3390/ijerph16224422
Zayakhanov, A. S., Zhamsueva, G. S., Sungrapova, I. P., and Tsydypov, V. V. (2018). Features of Diurnal Variability of Ultrafine Aerosol in the Air of the Baikal Coastal Zone and Arid Zone of Mongolia. Atmos. Ocean. Opt. 31, 257–262. doi:10.1134/S1024856018030168
Keywords: aerosol optical depth, AERONET, diurnal variability, indo-gangetic plains, aerosol radiative forcing (ARF)
Citation: Mukherjee T and Vinoj V (2021) The Sub-Daily Variability of Aerosol Loading and Associated Radiative Forcing Over the Indian Region. Front. Earth Sci. 9:727169. doi: 10.3389/feart.2021.727169
Received: 18 June 2021; Accepted: 14 September 2021;
Published: 08 November 2021.
Edited by:
Sachchidanand Singh, National Physical Laboratory (CSIR), IndiaReviewed by:
Bijoy Vengasseril Thampi, Science Systems and Applications, Inc, United StatesCopyright © 2021 Mukherjee and Vinoj. This is an open-access article distributed under the terms of the Creative Commons Attribution License (CC BY). The use, distribution or reproduction in other forums is permitted, provided the original author(s) and the copyright owner(s) are credited and that the original publication in this journal is cited, in accordance with accepted academic practice. No use, distribution or reproduction is permitted which does not comply with these terms.
*Correspondence: V. Vinoj, dmlub2pAaWl0YmJzLmFjLmlu
Disclaimer: All claims expressed in this article are solely those of the authors and do not necessarily represent those of their affiliated organizations, or those of the publisher, the editors and the reviewers. Any product that may be evaluated in this article or claim that may be made by its manufacturer is not guaranteed or endorsed by the publisher.
Research integrity at Frontiers
Learn more about the work of our research integrity team to safeguard the quality of each article we publish.