- 1Meteorological Research Institute, Japan Meteorological Agency, Tsukuba, Japan
- 2Seismology and Volcanology Department, Japan Meteorological Agency, Tokyo, Japan
In Japan, the nationwide earthquake early warning (EEW) system has been being operated by the Japan Meteorological Agency (JMA) since 2007, disseminating information on imminent strong ground motion to the general public and advanced technical users. In the beginning of the operation, the system ran based mainly on standard source-based algorithms with a point-source location estimate and ground motion prediction equation. The point-source algorithms successfully provided ground motion predictions with high accuracy during the initial operation; however, the 2011
Introduction
It has been 10 years since the 2011 off the Pacific coast of Tohoku earthquake (Tohoku-Oki earthquake), a
To mitigate earthquake damage, EEW systems have been developed and/or operated in many earthquake-prone regions around the world such as Japan (e.g., Nakamura, 1988; Hoshiba et al., 2008), Mexico (Espinosa-Aranda et al., 2009), the west coast of the United States (Böse et al., 2013; Kilb et al., 2021), Taiwan (Chen et al., 2015), Europe (Clinton et al., 2016), China (Zhang et al., 2016), Korea (Sheen et al., 2017), and Israel (Nof and Kurzon, 2021). In Japan, the Japan Meteorological Agency (JMA) has been operating the nationwide EEW system since 2007 to disseminate information on imminent strong ground motion to the general public and advanced technical users (Hoshiba et al., 2008).
In the beginning of the operation, the system ran based mainly on standard source-based algorithms that used a point-source model (PSM) estimate and ground motion prediction equation (GMPE) (Kamigaichi, 2004). The PSM algorithms successfully provided ground motion predictions with high accuracy during the initial operation (Doi, 2011; JMA, 2014). However, the prediction performance was poor for the Tohoku-Oki event and subsequent intense earthquake sequences in 2011 (Hoshiba et al., 2011; Kodera et al., 2018). For the mainshock, the system successfully issued a public warning for the Tohoku region (the nearest region from the epicenter) before the S-wave hit; on the other hand, the system underpredicted ground motions for the Kanto region, ∼300 km to the southwest of the epicenter, due to the fault finiteness (i.e., the PSM algorithms were unable to capture the spatial extent of the fault rupture). Just after the mainshock, the system missed several large aftershocks; the P-wave picking algorithm did not work because the P-wave onsets overlapped with large coda waves of the mainshock or previous aftershocks. In addition, the system overpredicted ground motions for multiple simultaneous earthquakes, failing to associate P-wave travel times and mislocating the hypocenters.
Over the past 10 years, JMA has taken various measures to overcome those technical limitations and to enhance the system performance further. One of significant updates of the JMA EEW system was the implementation of two novel approaches: the integrated particle filter (IPF) and propagation of local undamped motion (PLUM) algorithms (Tamaribuchi et al., 2014; Kodera et al., 2018). Another notable development was the incorporation of new observation facilities. In this article, we report the system improvements on prediction algorithms and seismic networks from 2011 to 2020 and investigate how those updates influenced the system performance using actual EEW reports issued from April 2016 to December 2020.
The Initial Japan Meteorological Agency Earthquake Early Warning System
JMA launched EEW service for the general public in October 2007 (Hoshiba et al., 2008). The initial EEW system was operated by incorporating two different seismic networks (Kamigaichi et al., 2009; Doi, 2011), versatile seismometers of JMA (Harada, 2007; ∼220 accelerometers) and Hi-net of the National Research Institute for Earth Science and Disaster Resilience (NIED; Okada et al., 2004; Aoi et al., 2020; ∼800 high-sensitivity velocity meters) (Figure 1A). Versatile means that the seismometers can calculate and transmit various observation quantities related to EEW such as P-wave arrival, displacement amplitude, and epicentral distance given by the B-Δ algorithm (Odaka et al., 2003; Tsukada et al., 2004; Harada, 2007). The system provided ground motion predictions based on the PSM estimation. Source parameter estimates were given by several algorithms running in parallel. The B-Δ, territory, and grid-search algorithms (Kamigaichi, 2004) were employed as the main source estimation methods. Real-time data from the JMA versatile seismometers were fed into these algorithms. The not-yet-arrived-data (NYAD) algorithm (Horiuchi et al., 2005), which provided source parameters from the Hi-net stations, was also implemented as an independent subsystem. In addition, the system was receiving source estimates from an external subsystem based on a classical hypocenter determination approach using automatic P- and S-wave picks at the JMA and Hi-net stations (we refer to this algorithm as Hypoon). The system received hypocenter estimates from NYAD and Hypoon only if the location errors were smaller than rejection thresholds. Source estimates given by different algorithms were combined into a single event in the event identification process if the source parameters were similar to each other. After that, ground motion predictions were calculated from the estimated source parameters and GMPE. The GMPE employed in the JMA EEW system was one proposed by Si and Midorikawa (1999), which determines a peak ground velocity (PGV) from a point-source location, magnitude, and hypocentral distance. The PGV was converted into a JMA seismic intensity (JMA, 1996) with the empirical equation proposed by Matsuoka and Midorikawa (1994). Finally, the system issued EEW reports including predicted JMA intensities and estimated source parameters.
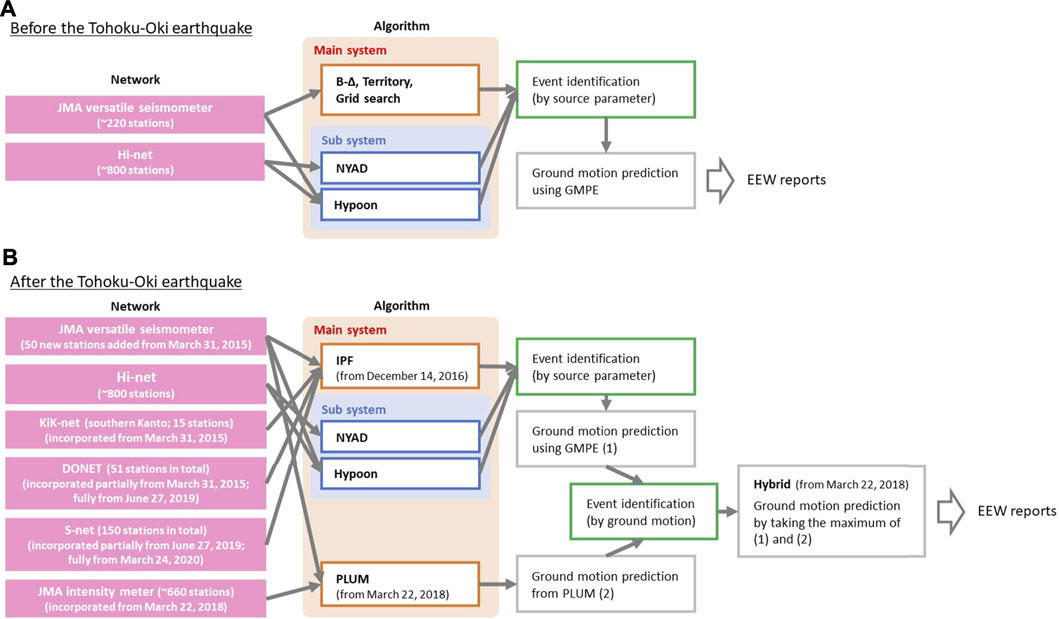
FIGURE 1. Data flow diagrams with seismic networks and ground motion prediction algorithms of the JMA EEW system (A) before and (B) after the Tohoku-Oki earthquake.
In the JMA EEW system, JMA seismic intensity is used as the ground motion metric. The JMA intensity is represented in two different ways: the 10-degree discrete representation
JMA evaluated the system prediction accuracy by calculating a prediction score, defined as the ratio of sub-prefectural areas whose intensity prediction error is within one unit on
The Japan Meteorological Agency Earthquake Early Warning System After the 2011 Tohoku-Oki Earthquake
Over the 10 years after the Tohoku-Oki earthquake, JMA has upgraded the EEW system by introducing new ground motion prediction algorithms and seismic observation facilities (Figure 1B).
New Ground Motion Prediction Algorithms
The Integrated Particle Filter Algorithm
IPF (Tamaribuchi et al., 2014) is a PSM algorithm that has been employed in the JMA EEW system since December 14, 2016 (JMA, 2016) to reduce the risk of overprediction due to the misassociation of P-wave picks for multiple simultaneous earthquakes. IPF estimates source parameters based on the Bayesian inference (Liu and Yamada, 2014; Wu et al., 2015); hypocenter locations are determined using not only P-wave travel times but also various observed quantities such as velocity amplitude, untriggered station distribution, and epicentral distance estimated from B-Δ. For the association of P-wave picking data, IPF also takes into account velocity amplitudes in addition to travel time differences, to discriminate the occurrence of multiple simultaneous earthquakes from a single large event. Retrospective simulations with the intense earthquake sequences caused by the 2011 Tohoku-Oki and 2016 Kumamoto earthquakes showed that IPF could reduce the substantial number of overpredicted warnings compared to the PSM algorithms in the initial JMA EEW system (Tamaribuchi et al., 2014; Kodera et al., 2016).
In the updated EEW system, IPF is implemented as the main PSM algorithm, replacing the B-Δ, territory, and grid-search algorithms. The NYAD and Hypoon algorithms are still working in the updated system; JMA has decided to leave the two algorithms because the two algorithms use denser seismic networks than IPF (the difference comes from whether Hi-net is incorporated or not) and therefore could sometimes provide source parameter estimates timelier than IPF.
IPF is being operated with JMA versatile seismometers. Additionally, IPF uses three seismic networks maintained by NIED: DONET, S-net, and a part of KiK-net (Kaneda et al., 2015; Kawaguchi et al., 2015; Kanazawa et al., 2016; Uehira et al., 2016; Mochizuki et al., 2016; Okada et al., 2004; Aoi et al., 2020; Figures 1B, 2A,B; as of December 2020).
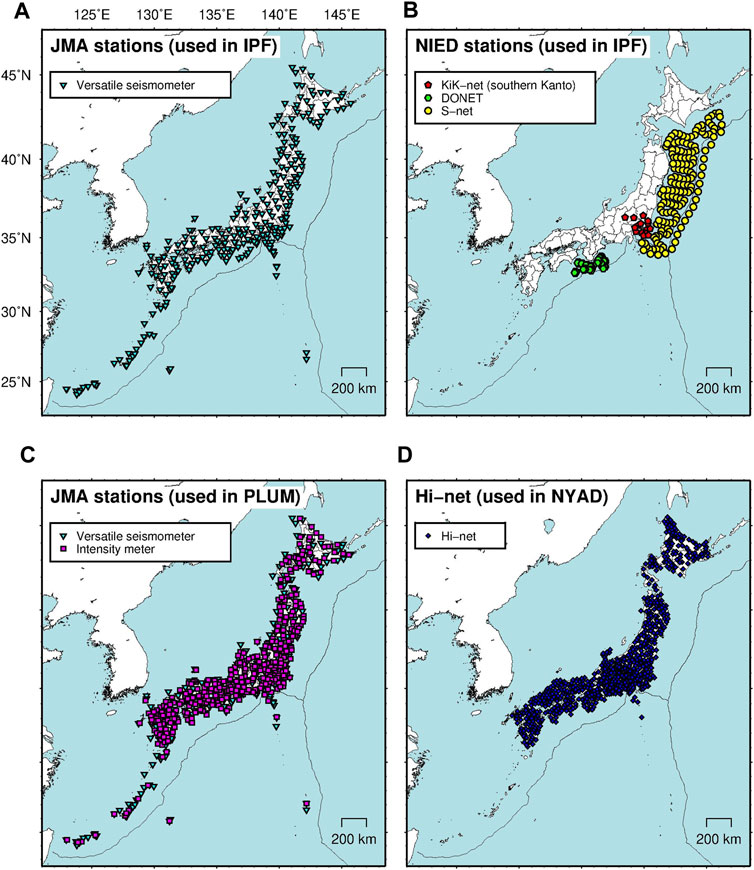
FIGURE 2. Locations of (A) JMA stations used in IPF, (B) NIED stations used in IPF, (C) JMA stations used in PLUM, and (D) Hi-net stations used in NYAD.
The Propagation of Local Undamped Motion Algorithm
PLUM (Kodera et al., 2018) is a wavefield-based algorithm (Hoshiba, 2013; Hoshiba and Aoki, 2015) that has been implemented since March 22, 2018 (JMA, 2018) to provide accurate ground motions for large earthquakes with nonnegligible finite faults and for intense earthquake sequences for which PSM algorithms could fail to estimate correct source parameters. PLUM provides ground motion predictions without assuming specific source models; instead, PLUM predicts future ground motions directly from ground shakings observed near the target sites, assuming unattenuated plane wave incident. In the JMA EEW system, a predicted intensity at target site
As of December 2020, PLUM is being operated with two JMA seismic networks, versatile seismometers and intensity meters (Figure 2C).
The Hybrid Algorithm
In the updated EEW system, two different ground motion predictions are given by the PSM and PLUM algorithms. The two ground motion predictions are combined in the ground-motion-based event identification process to obtain the final ground motion prediction result (Figure 1B). The event identification process assumes that the two ground motion predictions are from the same event if there are one or more overlapped sub-prefectural areas with predicted intensities of 3 or more. The final ground motion predictions are given by taking the maximum of predicted intensities for each area. We refer to this procedure as the hybrid algorithm (Kodera et al., 2018).
New Seismic Observation Facilities
After the Tohoku-Oki earthquake, in addition to the introduction of the new ground motion prediction algorithms, JMA has incorporated new inland and offshore seismic observation facilities for more robust and timelier EEW issuances (Figure 2).
Inland Networks
The initial JMA EEW system was being operated with ∼220 JMA versatile seismometers and ∼800 Hi-net stations (Figure 1A). On March 31, 2015, JMA installed 50 new versatile seismometers mainly on the Pacific side to enhance the detection capability (JMA, 2015; Figure 1B). Deep borehole seismometers of KiK-net (15 stations in the southern Kanto region) were also added for the main PSM algorithms to obtain additional lead times for earthquakes in the Kanto region (JMA, 2015; Figures 1B, 2B). On March 22, 2018, the start date for the PLUM operation, the system incorporated JMA intensity meters (seismometers that can transmit seismic intensities only) to perform the PLUM algorithm with a denser seismic network (Kodera et al., 2018). As of December 2020, real-time seismic intensities from ∼660 JMA intensity meters are fed into PLUM (Figures 1B, 2C). The Hi-net high-sensitivity velocity meters (Figure 2D) are used only for the NYAD and Hypoon algorithms.
Offshore Networks
To enhance the detection capability for offshore earthquakes, JMA also incorporated two ocean-bottom seismometer (OBS) networks maintained by NIED (Figures 1B, 2B). One of the OBS networks is DONET (Kaneda et al., 2015; Kawaguchi et al., 2015; Aoi et al., 2020), which consists of 22 OBSs across the Kumano-Nada (DONET1) and 29 OBSs off the Kii channel (DONET2). JMA incorporated the DONET stations partially on March 31, 2015 (JMA, 2015) and fully on June 27, 2019 (JMA, 2019b; Figure 1B). The other network is S-net (Kanazawa et al., 2016; Mochizuki et al., 2016; Uehira et al., 2016; Aoi et al., 2020), 150 OBSs deployed along the Japan and Kuril trenches. 125 and 25 OBSs of S-net were introduced in the system on June 27, 2019 (JMA, 2019b) and on March 24, 2020 (JMA, 2020a), respectively (Figure 1B).
For robust magnitude estimates with OBSs, a new magnitude estimation algorithm specialized for OBSs was developed and introduced (Hayashimoto et al., 2019; Hayashimoto et al., 2021; submitted to Quarterly Journal of Seismology). For inland seismometers, the JMA EEW system calculates magnitudes from the vector sum of three-component displacements. However, for OBSs, three-component displacements could overestimate magnitudes because of horizontal site amplification caused by thick sedimentary layers (Hayashimoto and Hoshiba, 2013; Nakamura et al., 2014) and acceleration offset signal contamination by device orientation changes when strong motions hit (Hayashimoto et al., 2016; Nakamura and Hayashimoto, 2019; Takagi et al., 2019). Hayashimoto et al. (2019) found that those overestimation effects could be mitigated by using vertical-component instead of three-component displacements. JMA therefore implemented a new magnitude estimation equation based solely on vertical-component displacements for OBSs. In addition, to eliminate possible amplitude anomalies at OBSs near hypocenters, the new magnitude estimation algorithm requires three or more OBSs to calculate magnitudes (i.e., magnitude estimates are unavailable until the system has received displacement amplitudes from three or more OBSs; Hayashimoto et al., 2021, submitted).
Performance Evaluation for the Updated Japan Meteorological Agency Earthquake Early Warning System
To evaluate the performance of the updated JMA EEW system, we assessed how the IPF and PLUM implementation influenced the prediction accuracy and warning issuance performance. Also, we analyzed how the S-net incorporation contributed to the detection timeliness for earthquakes around the Japan and Kuril trenches. A part of the prediction accuracy and warning issuance performance analysis in this study is the same as what Kodera et al. (2020) conducted but with an extended analysis period.
Prediction Accuracy Changes With the Integrated Particle Filter and Propagation of Local Undamped Motion Implementation
We investigated how the prediction accuracy of the JMA EEW system changed with the IPF and PLUM implementation, using the maximum and final ground motion prediction results. We focused on the maximum ground motion predictions for the accuracy evaluation because the system issues warnings once predicted ground motions exceed the warning threshold. The final ground motion predictions were also used because their prediction errors would indicate the upper limit of prediction accuracy for target algorithms. For PLUM, the final ground motion predictions are the same as the maximum ground motion predictions. In this analysis, the prediction accuracy was evaluated for three different periods: 1) April 1, 2016 to December 14, 2016, 2) December 14, 2016 to March 22, 2018, and 3) March 22, 2018 to December 31, 2020. December 14, 2016 and March 22, 2018 are the start dates for the IPF and PLUM operation, respectively. To evaluate the system performance before the IPF and PLUM implementation, we took the analysis period from April 1, 2016. We focused only on recent EEW reports for the evaluation of the previous PSM algorithms to exclude the possible performance change due to different software versions. The analysis period was relatively short compared to the entire operation period of the previous PSM algorithms but was enough long to capture the algorithms’ characteristics because during the period the system processed many earthquakes including the 2016 Kumamoto earthquake sequence.
For each period, we calculated the prediction score. For the score calculation, predicted intensities were compared to observed intensities on an area-by-area basis (i.e., each intensity was obtained by taking the maximum of predicted or observed intensities in a sub-prefectural area; for the definition of the sub-prefectural areas, see Figure 1C in Kodera et al. (2020)). The prediction score was defined as the ratio of areas in which the difference between predicted and observed
During the first period, which is before the IPF and PLUM operation, the prediction score was 69.7% with the maximum ground motion predictions (Figure 3A) and 86.1% with the final predictions (Figure 4A). The prediction score with the maximum predictions was low mainly due to overprediction for events of the 2016 Kumamoto earthquake sequence (Kodera et al., 2016). The system still had difficulty in distinguishing multiple simultaneous events from a large earthquake because IPF had not been implemented yet. The system overpredicted ground motions for several concurrent aftershocks, mislocating the hypocenters. The prediction score with the final predictions was higher than that with the maximum predictions, although the prediction accuracy was still affected by the overprediction for the Kumamoto earthquake sequence. The underprediction case with predicted
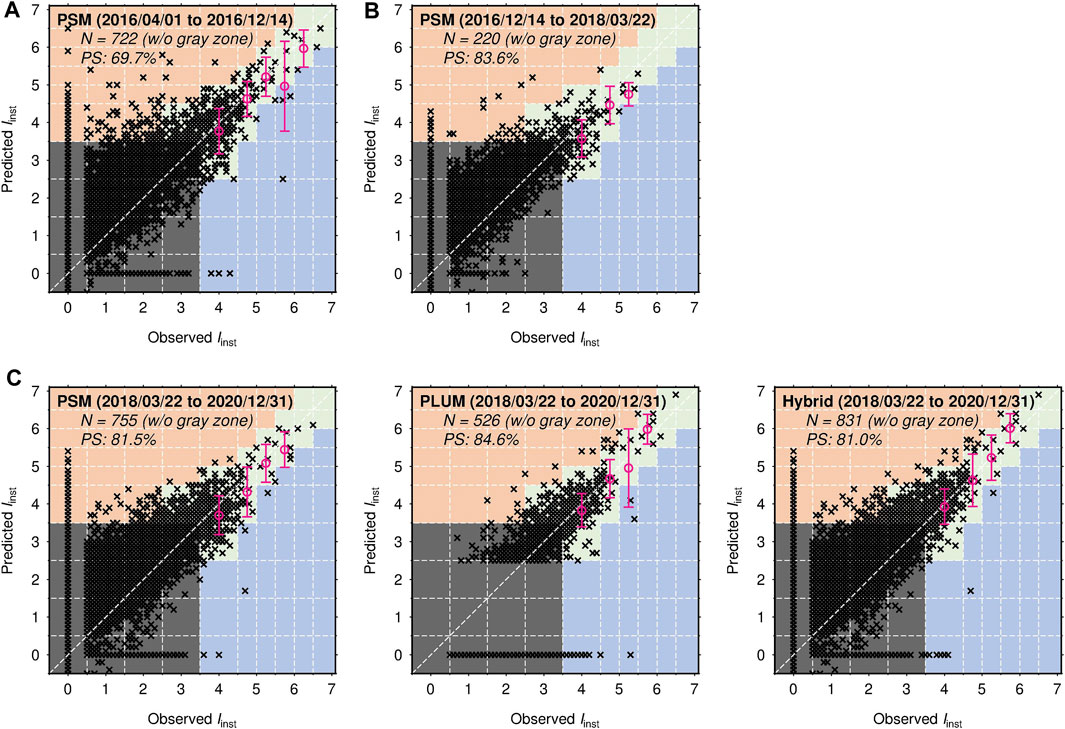
FIGURE 3. Comparisons of observed and predicted intensities using the maximum ground motion prediction results for the three periods: (A) April 1, 2016 to December 14, 2016 (the start date for the IPF operation), (B) December 14, 2016 to March 22, 2018 (the start date for the PLUM operation), and (C) March 22, 2018 to December 31, 2020. Intensities were plotted on an area-by-area basis; that is, each intensity was obtained by taking the maximum among those at all sites in a sub-prefectural area. Circles and bars in magenta indicate the mean and standard deviation of predicted intensities for each observed
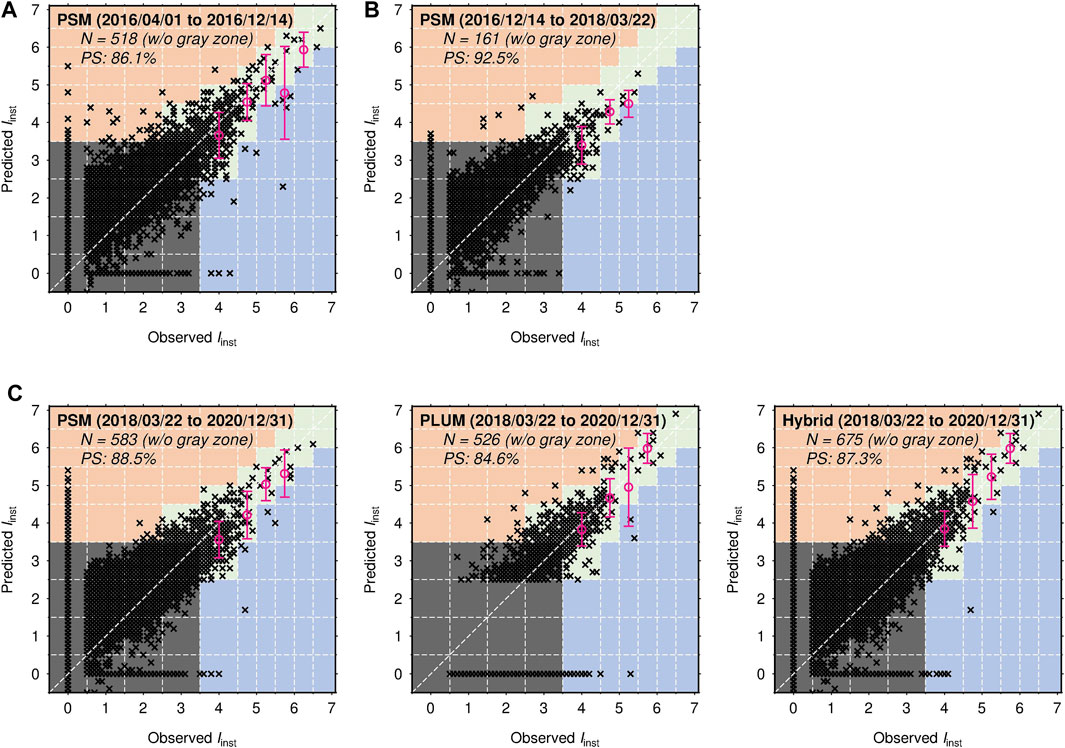
FIGURE 4. The same figure as Figure 3 but using the final ground motion prediction results.
For the second period, just after the IPF implementation, the prediction score was 83.6% with the maximum ground motion predictions and 92.5% with the final predictions, higher than those during the first period (Figures 3B, 4B). IPF did not provide mislocated hypocenters for multiple simultaneous events, and therefore the prediction accuracy was improved compared to that before the IPF implementation. However, there was still an overprediction case in which ground motions were overpredicted with errors of
During the third period, after the PLUM implementation, the prediction score of PSM was 81.5 and 88.5% with the maximum and final ground motion predictions, respectively (Figures 3C, 4C). As during the second period, IPF provided accurate source parameter estimates without hypocenter mislocation for multiple simultaneous earthquakes. However, an overprediction issue occurred again on July 30, 2020 (JMA, 2020b). For a M6.0 earthquake that occurred near the Torishima island (the epicenter was ∼500 km southward of the Japan mainland), the system issued an overpredicted warning, estimating a M7.3 earthquake whose location was ∼440 km northward of the actual epicenter. The overprediction was significant because no felt ground shaking was observed although EEW users received the warning. The Hypoon algorithm provided a mislocated hypocenter estimate, and the system used the source estimate because the location estimation errors (calculated by Hypoon) were lower than the rejection criteria. The magnitude was calculated with a displacement amplitude observed at a seismometer in the Hahajima island, ∼800 km southward of the estimated source location. After the overprediction issue, as a tentative measure, JMA modified the magnitude calculation logic, imposing the condition that seismometers used for the magnitude estimation must be located within 700 km from the estimated epicenter. Without the M6.0 near-Torishima event, the prediction score of the PSM algorithms would have been 85.5 and 94.3% with the maximum and final ground motion predictions, respectively (Supplementary Figures S1C, S2C). For the maximum ground motion predictions, there were several overprediction cases in which
During the second and third periods, the PSM algorithms tended to underpredict ground motions especially for strong shakings of
During the third period, the PLUM prediction score was 84.6% (Figures 3C, 4C; the maximum and final ground motion predictions are the same for PLUM). The prediction score for PLUM was influenced by prediction errors for inland earthquakes. The assumption of unattenuated wave incidence did not hold for sites near epicenters of shallow inland earthquakes; PLUM therefore overpredicted ground motions for several inland events. In addition, PLUM missed ground motions for three inland earthquakes with observed
The prediction score for the hybrid algorithm was 81.0 and 87.3% with the maximum and final predictions, respectively (Figures 3C, 4C). Without the M6.0 near-Torishima earthquake, the score would be 84.7 and 92.2% (Supplementary Figures S1C, S2C). The prediction score for the hybrid algorithm was similar to but slightly smaller than that for PSM, influenced by PLUM ground motion predictions. This indicates that the hybrid algorithm inherited the characteristics of both PSM and PLUM.
Warning Issuance Performance Changes With the Integrated Particle Filter and Propagation of Local Undamped Motion Implementation
We also evaluated how the warning issuance performance was changed by the IPF and PLUM implementation. We investigated which earthquakes with
During the first period, the JMA EEW system issued warnings for large earthquakes with a high detection rate (Figure 5A). Especially, warnings were issued for all earthquakes with observed
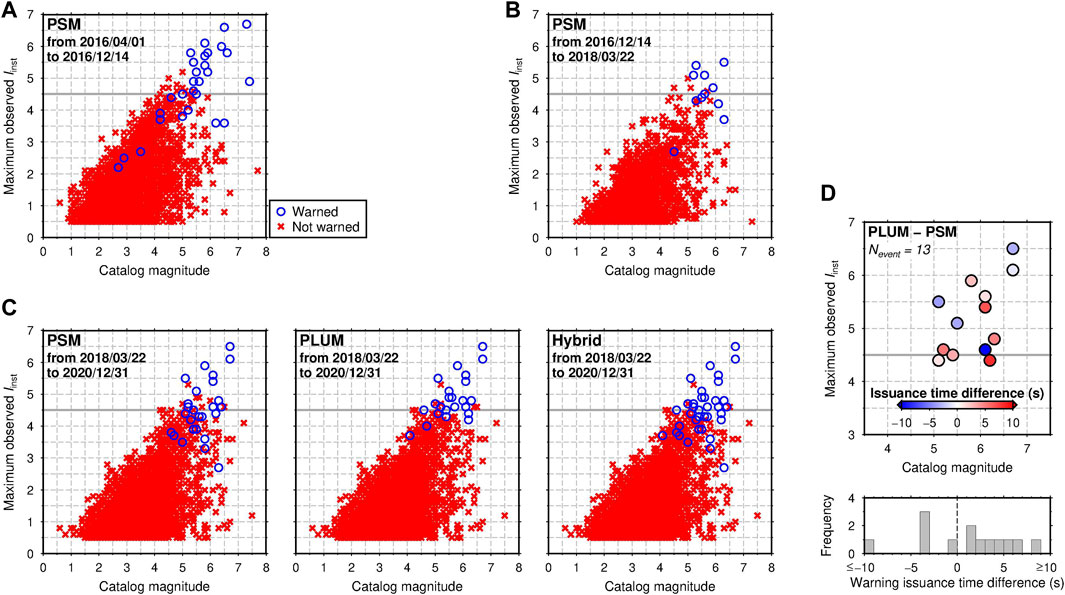
FIGURE 5. (A–C) Earthquakes with a warning issued by the PSM, PLUM, and hybrid algorithms (blue circle) and earthquakes without a warning issued (red cross). In this analysis, earthquakes with
During the second period, the system issued warnings with a similar detection rate to that during the first period for earthquakes with observed
During the third period, PSM and PLUM detected warning events with a low missing rate for observed
To assess the warning timeliness, we evaluated warning issuance time differences between PSM and PLUM, using the 13 earthquakes warned by both of PSM and PLUM during the third period (Figure 5D). For five out of the 13 earthquakes, the warning issuance times of PLUM were earlier than those of PSM. The median time difference was ∼3.4 s. The warning timeliness was improved for those events because PLUM was less likely to underpredict strong ground motions than PSM, and PLUM used the denser JMA seismic network than IPF.
Detection Capability Changes With the S-Net Incorporation
Focusing on the S-net incorporation, we investigated how the new observation facilities changed the detection capability of the JMA EEW system. The timeliness of the first EEW report was evaluated for earthquakes around the Japan and Kuril trenches, where S-net has been deployed. Here, we use the term “detection time” as the time when the system issued the first EEW report.
The target area for the detection time analysis is shown in Figure 6A. For earthquakes inside the area, we calculated the cumulative distribution functions (CDFs) of detection times before and after June 27, 2019, the start date for the S-net incorporation (Figure 6B). The CDF after the S-net incorporation took smaller values for all detection times than the CDF before the incorporation, indicating that S-net successfully improved the detection timeliness for earthquakes around the Japan and Kuril trenches. Especially, after the S-net incorporation, the system issued the first EEW report within 21.78 and 30.14 s from the origin time for 50 and 75% of earthquakes inside the area, respectively, which were 3.90 and 8.83 s earlier than before the incorporation.
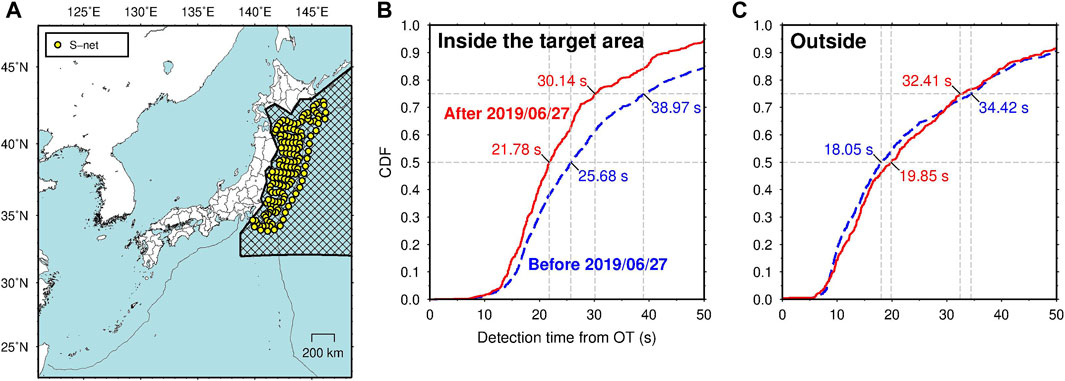
FIGURE 6. (A) The target area for the detection time analysis for S-net (shaded area) and the locations of S-net stations. (B) the CDFs of detection times for earthquakes inside the target area before and after June 27, 2019, the start date for the S-net incorporation (that is, from April 1, 2016 to June 27, 2019 and from June 27, 2019 to December 31, 2020; blue broken and red solid lines, respectively). The detection time means the time when the JMA EEW system issued the first EEW report. The detection times for CDF values of 0.50 and 0.75 are shown. OT, origin time. (C) The same figure as (B) but for earthquakes outside the target area.
In addition, we also calculated the CDFs for earthquakes outside the target area (Figure 6C). The CDF values did not change drastically before and after the S-net introduction. The detection time difference was less than ∼2.0 s, implying that the detection time variability due to temporal seismicity change was within ∼2.0 s. The detection time reduction for earthquakes inside the area was larger than ∼2.0 s; therefore, the timeliness improvement by S-net was significant.
Discussion
IPF has been implemented to overcome the overprediction issue caused by the mislocation of multiple simultaneous earthquakes. Before the IPF operation, the prediction score for the PSM algorithms was 69.7% with the maximum ground motion predictions; after the IPF implementation, the prediction score increased to more than 80% (83.6% from December 14, 2016 to March 22, 2018 and 81.6% from March 22, 2018 to December 31, 2020). After the IPF operation, the PSM algorithms caused significant ground motion overpredictions for the M4.5 and M4.0 earthquakes in January 2018 and for the M6.0 near-Torishima earthquake in July 2020, which were not due to IPF but due to the event identification process and the Hypoon algorithm. Without the two overprediction cases, the prediction score would have been 89.8% from December 14, 2016 to March 22, 2018 and 85.5% from March 22, 2018 to December 31, 2020. Those results indicate that IPF provided source parameter estimates with a higher accuracy than the previous PSM algorithms. The warning performance analysis showed that, before the IPF implementation, the system issued overpredicted warnings for M2–3-class earthquakes because of mislocated source estimates provided by the previous PSM algorithms. On the other hand, IPF did not cause overpredicted warnings for earthquakes with observed
The prediction score for PSM using the final EEW reports was ∼90% after the IPF operation; however, even with the final ground motion predictions, the PSM algorithms were likely to underpredict strong shakings caused by large inland earthquakes, because of GMPE errors and/or minor magnitude underestimation. Especially, the underprediction due to GMPE errors underscored the prediction accuracy limitation of the source-based approach; that is, correct hypocenter location and magnitude estimates do not always provide accurate ground motions because of GMPE errors (Hoshiba et al., 2010).
After the IPF operation, significant ground motion overpredictions occurred for the M4.5 and M4.0 earthquakes in January 2018 and for the M6.0 near-Torishima earthquake in July 2020. The first case was due to incorrect event association in the event identification process. The other case was because of a mislocated hypocenter estimate from the Hypoon algorithm. Those overprediction cases indicate that there are still technical challenges that need to be addressed to attain more robust source-based ground motion predictions. A possible solution for the technical issues is to skip the event identification process by integrating the different PSM algorithms into a single algorithm. The integration may be possible by feeding all available seismic data into IPF, although the current IPF algorithm does not incorporate Hi-net high-sensitivity velocity meters. Several previous studies suggested that the Hi-net velocity sensors could be used for a PSM algorithm in combination with accelerometers if the instrumental response was corrected (Yamada et al., 2014; Noguchi et al., 2020; Yamada et al., 2021).
PLUM has been introduced to provide robust ground motion predictions for complex earthquake scenarios such as a large earthquake with a finite fault and an intense earthquake sequence. The prediction score for PLUM was 84.6%, which was affected by prediction errors for shallow inland earthquakes. On the other hand, PLUM predicted strong ground motions caused by large inland earthquakes with smaller underprediction errors than PSM. The warning performance analysis showed that, for earthquakes near the warning threshold (i.e., the peak observed intensity was 4.5
Owing to the PLUM implementation, the JMA EEW system attained robust and timely ground motion predictions for earthquakes with high observed intensities. At the same time, the system had a higher possibility of overprediction for shallow inland earthquakes than before. Although the PLUM implementation caused an adverse effect on the system prediction accuracy, the prediction accuracy analysis for the hybrid algorithm indicates that the adverse impact was not very large. The prediction score for the hybrid algorithm was 81.0% with the maximum ground motion predictions and 87.3% with the final predictions. Those percentages were lower than those for PSM; however, the differences were limited to within ∼1%, implying that the prediction errors due to PLUM were acceptable.
Two large-scale OBS networks, DONET and S-net, have been incorporated into the JMA EEW system to improve the detection capability for offshore earthquakes. The detection capability analysis for S-net showed that S-net shortened detection times for earthquakes around the Japan and Kuril trenches substantially. This indicates that the current JMA EEW system would issue public warnings with longer lead times than the system in 2011 if large earthquakes occur again around the Japan trench. In the western region of the Nankai trough, along which a M9-class megathrust earthquake is anticipated, NIED is planning to construct a new large-scale OBS network, N-net (Aoi et al., 2020). The N-net observation data will be transmitted to the JMA EEW system in real time. N-net would improve the detection timeliness for earthquakes around the network as well as S-net.
Conclusion
The 2011
To evaluate the prediction accuracy changes with the IPF and PLUM implementation, we calculated a prediction score, defined as the ratio of sub-prefectural areas for which ground motions are predicted within intensity errors of one
The PLUM implementation made the system less likely to underpredict strong ground motions caused by large inland earthquakes, which was due to PLUM’s ground motion prediction approach using direct observation of ongoing ground shakings. PLUM also enabled the system to issue warnings with a low missing rate for earthquakes whose observed intensities were just above the warning threshold. The warning timeliness was improved by PLUM for five out of the 13 earthquakes warned by both of PSM and PLUM. The median of the improved warning times was ∼3.4 s.
The detection time analysis for S-net showed that the incorporation of S-net shortened 50th and 75th percentile detection times (times required to issue the first EEW report) by 3.90 and 8.83 s, respectively, for offshore earthquakes around the Japan and Kuril trenches.
Those findings indicate that, over the 10 years after the Tohoku-Oki earthquake, the JMA EEW system has made substantial progress both on software and hardware aspects. At the same time, there is still room for further improvements of the system on the ground motion prediction algorithms and observation facilities. The JMA EEW system in the next 10 years will achieve more accurate, timely, and robust EEW issuance for possible future large earthquakes.
Data Availability Statement
EEW reports issued by the JMA EEW system were obtained from an internal database of JMA. Earthquake locations and observed JMA intensities can be obtained from the JMA website at https://www.data.jma.go.jp/svd/eqev/data/bulletin/index_e.html (last accessed June 2021).
Author Contributions
YK designed the study, performed the analyses, and drafted the article. NH, KN, KM, RT, MM, and KO maintained the JMA EEW system and made the database of EEW reports. KT developed the IPF algorithm. YK and MH proposed the PLUM algorithm. NH, KN, and MH developed the algorithms to process OBS data. All authors read and approved the final article.
Conflict of Interest
The authors declare that the research was conducted in the absence of any commercial or financial relationships that could be construed as a potential conflict of interest.
Publisher’s Note
All claims expressed in this article are solely those of the authors and do not necessarily represent those of their affiliated organizations, or those of the publisher, the editors, and the reviewers. Any product that may be evaluated in this article, or claim that may be made by its manufacturer, is not guaranteed or endorsed by the publisher.
Acknowledgments
The authors would like to thank the handling editor and two reviewers for their useful comments and suggestions that improved the article. The JMA EEW system has been developed based on joint research of JMA and the Railway Technical Research Institute, technological achievements by NIED, and research led by Kyoto University that was supported by funding for Next Generation World-Leading Researchers from the Cabinet Office of Japan. The JMA EEW system uses seismic data obtained from observational facilities of NIED, in addition to those of JMA (as of December 2020). Figures were drawn using Generic Mapping Tools (Wessel and Smith, 1998).
Supplementary Material
The Supplementary Material for this article can be found online at: https://www.frontiersin.org/articles/10.3389/feart.2021.726045/full#supplementary-material
References
Aoi, S., Asano, Y., Kunugi, T., Kimura, T., Uehira, K., Takahashi, N., et al. (2020). MOWLAS: NIED Observation Network for Earthquake, Tsunami and Volcano. Earth Planets Space 72, 126. doi:10.1186/s40623-020-01250-x
Böse, M., Allen, R., Brown, H., Gua, G., Fischer, M., Hauksson, E., et al. (2014). “CISN ShakeAlert: An Earthquake Early Warning Demonstration System for California,” in Early Warning for Geological Disasters—Scientific Methods and Current Practice. Editors J. Zschau, and F. Wenzel (Berlin, Germany: Springer), 49–69. doi:10.1007/978-3-642-12233-0_3
Chen, D. Y., Hsiao, N. C., and Wu, Y. M. (2015). The Earthworm Based Earthquake Alarm Reporting System in Taiwan. Bull. Seismological Soc. America 105 (2A), 568–579. doi:10.1785/0120140147
Clinton, J., Zollo, A., Marmureanu, A., Zulfikar, C., and Parolai, S. (2016). State-of-the Art and Future of Earthquake Early Warning in the European Region. Bull. Earthquake Eng. 14 (9), 2441–2458. doi:10.1007/s10518-016-9922-7
Doi, K. (2011). The Operation and Performance of Earthquake Early Warnings by the Japan Meteorological Agency. Soil Dyn. Earthquake Eng. 31 (2), 119–126. doi:10.1016/j.soildyn.2010.06.009
Espinosa-Aranda, J. M., Cuellar, A., Garcia, A., Ibarrola, G., Islas, R., Maldonado, S., et al. (2009). Evolution of the Mexican Seismic Alert System (SASMEX). Seismological Res. Lett. 80 (5), 694–706. doi:10.1785/gssrl.80.5.694
Hayashimoto, N., and Hoshiba, M. (2013). Examination of Travel Time Correction and Magnitude Correction of Tonankai Ocean Bottom Seismographs for Earthquake Early Warning. Q. J. Seismology 76, 69–81.
Hayashimoto, N., Nakamura, T., and Hoshiba, M. (2019). A Technique for Estimating the UD-Component Displacement Magnitude for Earthquake Early Warnings that Can Be Applied to Various Seismic Networks Including Ocean Bottom Seismographs. Q. J. Seismology 83, 1.
Hayashimoto, N., Nakamura, T., and Hoshiba, M. (2016). Stability of Ocean Bottom Seismograph Data Exposed to strong Shaking: Efforts for Utilizing OBS for Earthquake Early Warning. ECGS ESC/EAEE Jt. Workshop Proc. 31, 41–49.
Horiuchi, S., Negishi, H., Abe, K., Kamimura, A., and Fujinawa, Y. (2005). An Automatic Processing System for Broadcasting Earthquake Alarms. Bull. Seismological Soc. America 95 (2), 708–718. doi:10.1785/0120030133
Hoshiba, M., and Aoki, S. (2015). Numerical Shake Prediction for Earthquake Early Warning: Data Assimilation, Real‐Time Shake Mapping, and Simulation of Wave Propagation. Bull. Seismological Soc. America 105 (3), 1324–1338. doi:10.1785/0120140280
Hoshiba, M., Iwakiri, K., Hayashimoto, N., Shimoyama, T., Hirano, K., Yamada, Y., et al. (2011). Outline of the 2011 off the Pacific Coast of Tohoku Earthquake (M W 9.0) -Earthquake Early Warning and Observed Seismic Intensity-. Earth Planet. Sp 63, 547–551. doi:10.5047/eps.2011.05.031
Hoshiba, M., Kamigaichi, O., Saito, M., Tsukada, S. y., and Hamada, N. (2008). Earthquake Early Warning Starts Nationwide in Japan. Eos Trans. AGU 89, 73–74. doi:10.1029/2008EO080001
Hoshiba, M., Ohtake, K., Iwakiri, K., Aketagawa, T., Nakamura, H., and Yamamoto, S. (2010). How Precisely Can We Anticipate Seismic Intensities? A Study of Uncertainty of Anticipated Seismic Intensities for the Earthquake Early Warning Method in Japan. Earth Planet. Sp 62 (8), 611–620. doi:10.5047/eps.2010.07.013
Hoshiba, M. (2013). Real-time Prediction of Ground Motion by Kirchhoff-Fresnel Boundary Integral Equation Method: Extended Front Detection Method for Earthquake Early Warning. J. Geophys. Res. Solid Earth 118, 1038–1050. doi:10.1002/jgrb.50119
Iwakiri, K., Hoshiba, M., Nakamura, K., and Morikawa, N. (2011). Improvement in the Accuracy of Expected Seismic Intensities for Earthquake Early Warning in Japan Using Empirically Estimated Site Amplification Factors. Earth Planet. Sp 63 (2), 57–69. doi:10.5047/eps.2010.12.002
Japan Meteorological Agency JMA (2020b). Press release: On the implementation of an urgent improvement of earthquake early warning. Retrieved from: https://www.jma.go.jp/jma/press/2008/07a/kaizen_20200807.pdf (last accessed June, , 2021).
Japan Meteorological Agency JMA (2014). Document No. 1: On the Dissemination History and Accuracy Evaluation of Recent Earthquake Early Waning reportsThe Fifth Meeting of the Committee of Evaluation and Improvement of Earthquake Early Warning. Retrieved from: https://www.data.jma.go.jp/svd/eqev/data/study-panel/eew-hyoka/05/shiryou1.pdf (last accessed June, 2021).
Japan Meteorological Agency JMA (2019a). Document No. 2: Document of the Ninth Meeting of the Technical Panel of the Committee of Evaluation and Improvement of Earthquake Early Warning. The Ninth Meeting of the Technical Panel of the Committee of Evaluation and Improvement of Earthquake Early Warning. Retrieved from: http://www.data.jma.go.jp/svd/eqev/data/study-panel/eew-hyoka/t09/20190305_siryou2.pdf (last accessed June, 2021).
Japan Meteorological Agency JMA (2016). Press Release: On a Technical Improvement of Earthquake Early Warning (The IPF Algorithm) and Measure for the False Earthquake Early Warning Report on August 1, 2016. Retrieved from: https://www.jma.go.jp/jma/press/1612/13a/EEW_kaizen_201612.pdf (last accessed June, 2021).
Japan Meteorological Agency JMA (2018). Press Release: On an Improvement of Earthquake Early Warning—The Prediction Accuracy of Seismic Intensity when Large Earthquakes Occur Is Improved. Retrieved from: https://www.jma.go.jp/jma/press/1803/08c/EEW_kaizen_201803.pdf (last accessed June, 2021).
Japan Meteorological Agency JMA (2020a). Press Release: On the Additional Use of New Ocean Bottom Seismometers for Earthquake Early Warning. Retrieved from: https://www.jma.go.jp/jma/press/2003/19a/20200319_eewkatsuyouS6.pdf (last accessed June, 2021).
Japan Meteorological Agency JMA (2015). Press Release: On the Start Date for Using New Observation Data for Earthquake Early Warning. Retrieved from: https://www.jma.go.jp/jma/press/1503/24a/eewkatsuyou20150324.pdf (last accessed June, 2021).
Japan Meteorological Agency JMA (2019b). Press Release: On the Use of New Ocean-Bottom Seismic Observation Data for Earthquake Early Warning. Retrieved from: https://www.jma.go.jp/jma/press/1906/21a/eewkatsuyou20190621.pdf (last accessed June 2021).
Kamigaichi, O. (2004). JMA Earthquake Early Warning. J. JAEE 4 (3), 134–137. doi:10.5610/jaee.4.3_134
Kamigaichi, O., Saito, M., Doi, K., Matsumori, T., Tsukada, S., Takeda, K., et al. (2009). Earthquake Early Warning in Japan: Warning the General Public and Future Prospects. Seismological Res. Lett. 80 (5), 717–726. doi:10.1785/gssrl.80.5.717
Kanazawa, T., Uehira, K., Mochizuki, M., Shinbo, T., Fujimoto, H., Noguchi, S., et al. (2016). S-net Project, Cabled Observation Network for Earthquakes and Tsunamis. SubOptic. WE2B–3.
Kaneda, Y., Kawaguchi, K., Araki, E., Matsumoto, H., Nakamura, T., Kamiya, S., et al. (2015). “Development and Application of an Advanced Ocean Floor Network System for Megathrust Earthquakes and Tsunamis,” in Seafloor Observatories. (Berlin, Germany: Springer), 643–662. doi:10.1007/978-3-642-11374-1_25
Kawaguchi, K., Kaneko, S., Nishida, T., and Komine, T. (2015). “Construction of the DONET Real-Time Seafloor Observatory for Earthquakes and Tsunami Monitoring,” in Seafloor Observatories. (Berlin, Germany: Springer), 211–228. doi:10.1007/978-3-642-11374-1_10
Kilb, D., Bunn, J. J., Saunders, J. K., Cochran, E. S., Minson, S. E., Baltay, A., et al. (2021). The PLUM Earthquake Early Warning Algorithm: A Retrospective Case Study of West Coast, USA, Data. J. Geophys. Res. Solid Earth 126, e2020JB021053. doi:10.1029/2020JB021053
Kodera, Y. (2019). An Earthquake Early Warning Method Based on Huygens Principle: Robust Ground Motion Prediction Using Various Localized Distance‐Attenuation Models. J. Geophys. Res. Solid Earth 124 (12), 12981–12996. doi:10.1029/2019JB017862
Kodera, Y., Hayashimoto, N., Moriwaki, K., Noguchi, K., Saito, J., Akutagawa, J., et al. (2020). First-year Performance of a Nationwide Earthquake Early Warning System Using a Wavefield-Based Ground-Motion Prediction Algorithm in Japan. Seismological Res. Lett. 91 (2A), 826–834. doi:10.1785/0220190263
Kodera, Y. (2018). Real-time Detection of Rupture Development: Earthquake Early Warning Using P Waves from Growing Ruptures. Geophys. Res. Lett. 45, 156–165. doi:10.1002/2017GL076118
Kodera, Y., Saitou, J., Hayashimoto, N., Adachi, S., Morimoto, M., Nishimae, Y., et al. (2016). Earthquake Early Warning for the 2016 Kumamoto Earthquake: Performance Evaluation of the Current System and the Next-Generation Methods of the Japan Meteorological Agency. Earth Planets Space 68 (1), 202. doi:10.1186/s40623-016-0567-1
Kodera, Y., Yamada, Y., Hirano, K., Tamaribuchi, K., Adachi, S., Hayashimoto, N., et al. (2018). The Propagation of Local Undamped Motion (PLUM) Method: A Simple and Robust Seismic Wavefield Estimation Approach for Earthquake Early Warning. Bull. Seismological Soc. America 108 (2), 983–1003. doi:10.1785/0120170085
Kunugi, T., Aoi, S., Nakamura, H., Suzuki, W., Morikawa, N., and Fujiwara, H. (2013). An Improved Approximating Filter for Real-Time Calculation of Seismic Intensity. Jssj 65 (3), 223–230. doi:10.4294/zisin.65.223
Liu, A., and Yamada, M. (2014). Bayesian Approach for Identification of Multiple Events in an Early Warning System. Bull. Seismological Soc. America 104 (3), 1111–1121. doi:10.1785/0120130208
Matsuoka, M., and Midorikawa, S. (1994). The Digital National Land Information and Seismic Microzoning. in Proceeding of the 22nd Symposium of Earthquake Ground Motion, AIJ, Tokyo, Japan, 23–34.
Mochizuki, M., Kanazawa, T., Uehira, K., Shimbo, T., Shiomi, K., Kunugi, T., et al. (2016). S-net Project: Construction of Large Scale Seafloor Observatory Network for Tsunamis and Earthquakes in Japan. AGU Fall Meeting, San Francisco, United States. NH43B–1840.
Nakamura, T., and Hayashimoto, N. (2019). Rotation Motions of Cabled Ocean-Bottom Seismic Stations during the 2011 Tohoku Earthquake and Their Effects on Magnitude Estimation for Early Warnings. Geophys. J. Int. 216 (2), 1413–1427. doi:10.1093/gji/ggy502
Nakamura, T., Nakano, M., Hayashimoto, N., Takahashi, N., Takenaka, H., Okamoto, T., et al. (2014). Anomalously Large Seismic Amplifications in the Seafloor Area off the Kii peninsula. Mar. Geophys. Res. 35, 255–270. doi:10.1007/s11001-014-9211-2
Nakamura, Y. (1988). On the Urgent Earthquake Detection and Alarm System (UrEDAS). in Proceedings of Ninth World Conference on Earthquake Engineering, Tokyo and Kyoto, Japan, August 2–9, 1988, 7, 673–678.
Nof, R. N., and Kurzon, I. (2021). TRUAA-earthquake Early Warning System for Israel: Implementation and Current Status. Seismological Res. Lett. 92 (1), 325–341. doi:10.1785/0220200176
Noguchi, K., Hayashimoto, N., Tamaribuchi, K., and Kodera, Y. (2020). Improvement of IPF Method with Utilization of Hi-Net. SSJ Fall Meet. 2020, S15–S06.
Odaka, T., Ashiya, K., Tsukada, S., Sato, S., Ohtake, K., and Nozaka, D. (2003). A New Method of Quickly Estimating Epicentral Distance and Magnitude from a Single Seismic Record. Bull. Seismological Soc. America 93 (1), 526–532. doi:10.1785/0120020008
Okada, Y., Kasahara, K., Hori, S., Obara, K., Sekiguchi, S., Fujiwara, H., et al. (2004). Recent Progress of Seismic Observation Networks in Japan -Hi-Net, F-Net, K-NET and KiK-Net-. Earth Planet. Sp 56, xv–xxviii. doi:10.1186/BF03353076
Sheen, D. H., Park, J. H., Chi, H. C., Hwang, E. H., Lim, I. S., Seong, Y. J., et al. (2017). The First Stage of an Earthquake Early Warning System in South Korea. Seismological Res. Lett. 88 (6), 1491–1498. doi:10.1785/0220170062
Si, H., and Midorikawa, S. (1999). New Attenuation Relationships for Peak Ground Acceleration and Velocity Considering Effects of Fault Type and Site Condition. Nihon Kenchiku Gakkai Kozokei Ronbunshu 64 (523), 63–70. doi:10.3130/aijs.64.63_2
Takagi, R., Uchida, N., Nakayama, T., Azuma, R., Ishigami, A., Okada, T., et al. (2019). Estimation of the Orientations of the S‐net Cabled Ocean‐Bottom Sensors. Seismological Res. Lett. 90 (6), 2175–2187. doi:10.1785/0220190093
Tamaribuchi, K., Yamada, M., and Wu, S. (2014). A New Approach to Identify Multiple Concurrent Events for Improvement of Earthquake Early Warning. Jssj 67 (67), 41–55. doi:10.4294/zisin.67.41
Tsukada, S., Odaka, T., Ashiya, K., Ohtake, K., and Nozaka, D. (2004). Analysis of the Envelope Waveform of the Initial Part of P-Waves and its Application to Quickly Estimating the Epicentral Distance and Magnitude. Jssj 56 (56), 351–361. doi:10.4294/zisin1948.56.4_351
Uehira, K., Kanazawa, T., Mochizuki, M., Fujimoto, H., Noguchi, S., Shinbo, T., et al. (2016). Outline of Seafloor Observation Network for Earthquakes and Tsunamis along the Japan Trench (S-Net). EGU General Assembly 2016, Vienna, Austria, EGU2016–13832.
Wessel, P., and Smith, W. H. F. (1998). New, Improved Version of Generic Mapping Tools Released. Eos Trans. AGU 79 (47), 579. doi:10.1029/98EO00426
Wu, S., Yamada, M., Tamaribuchi, K., and Beck, J. L. (2015). Multi-events Earthquake Early Warning Algorithm Using a Bayesian Approach. Geophys. J. Int. 200 (2), 791–808. doi:10.1093/gji/ggu437
Yamada, M., Tamaribuchi, K., and Wu, S. (2014). Faster and More Accurate Earthquake Early Warning System. J. JAEE 14 (4), 21–24. doi:10.5610/jaee.14.4_21
Yamada, M., Tamaribuchi, K., and Wu, S. (2021). The Extended Integrated Particle Filter Method (IPFx) as a High-Performance Earthquake Early Warning System. Bull. Seismological Soc. America 111 (3), 1263–1272. doi:10.1785/0120210008
Keywords: earthquake early warning, ground motion prediction, real-time analysis, hypocenter determination, ocean bottom seismometer, 2011 Tohoku-Oki earthquake
Citation: Kodera Y, Hayashimoto N, Tamaribuchi K, Noguchi K, Moriwaki K, Takahashi R, Morimoto M, Okamoto K and Hoshiba M (2021) Developments of the Nationwide Earthquake Early Warning System in Japan After the 2011
Received: 16 June 2021; Accepted: 17 September 2021;
Published: 04 October 2021.
Edited by:
Katsuichiro Goda, Western University , CanadaReviewed by:
Jindong Song, Institute of Engineering Mechanics, China Earthquake Administration, ChinaGemma Cremen, University College London, United Kingdom
Copyright © 2021 Kodera, Hayashimoto, Tamaribuchi, Noguchi, Moriwaki, Takahashi, Morimoto, Okamoto and Hoshiba. This is an open-access article distributed under the terms of the Creative Commons Attribution License (CC BY). The use, distribution or reproduction in other forums is permitted, provided the original author(s) and the copyright owner(s) are credited and that the original publication in this journal is cited, in accordance with accepted academic practice. No use, distribution or reproduction is permitted which does not comply with these terms.
*Correspondence: Yuki Kodera, y_kodera@mri-jma.go.jp