- 1Department of Earth Sciences, University of Pisa, Pisa, Italy
- 2Swiss Seismological Service, Swiss Federal Institute of Technology, Zürich, Switzerland
- 3Red Sismológica Nacional y Escuela Centroamericana de Geología, Universidad de Costa Rica, San Jose, Costa Rica
We analyze the performance of a prototype earthquake early warning system deployed at the National Seismological Network of Costa Rica in collaboration with the Swiss Seismological Service by presenting the real-time performance during six earthquakes (Mw 5.1-6.4) that took place during 2018 and 2019. We observe that, despite only limited efforts to optimize the existing network of 158 stations, for EEW purposes, the network density allows fast determination of source parameters using both the Virtual Seismologist and the Finite Fault Rupture Detector algorithms. Shallow earthquakes on or near-shore are routinely identified within 11–20 s of their occurrence. The warning times for the capital city of San Jose are of 43 s for epicenters located at 220 km, like for the Mw 6.4 Armuelles earthquake. On the other hand, during the time analyzed, the EEW system did not provide positive warning times for earthquakes at distances less than 40 km from San Jose. Even though large (Mw > 7) distant historical earthquakes have not caused heavy damage in San Jose, there is potential for developing an EEW system for Costa Rica, especially for the purposes of rapid earthquake notifications, disaster response management, and seismic risk mitigation.
Introduction
Costa Rica is located at the boundary of three major tectonic plates and one microplate (Figure 1). There is a subduction zone in the Pacific side of the country, where the Cocos plate is subducting underneath the Caribbean plate and the Panama microplate at rates from 83 mm/yr in the Northern Pacific of the country to 89 mm/yr in Southeast Costa Rica (DeMets et al., 1994). Large (up to Mw 7.7) seismogenic zone earthquakes have occurred historically along this plate boundary. Intermediate-depth earthquakes (depths 40–200 km) also occur beneath most of Costa Rica (e.g., Lücke and Arroyo, 2015). In addition, shallow earthquakes are common along the central Costa Rica Deformed Belt (CCRDB) and the North Panama Deformed Belt (NPDB), which define the limit between the Caribbean plate and the Panama microplate (Montero, 2001), and along the Panama Fracture Zone (PFZ) which separates the Cocos and Nazca plates. This complex tectonic setting generates high-seismicity rates. Since 1821, the nation has faced 68 damaging earthquakes, with an average rate of one every three years (Montero, 1989; Peraldo and Montero, 1994; Linkimer and Alvarado, 2014).
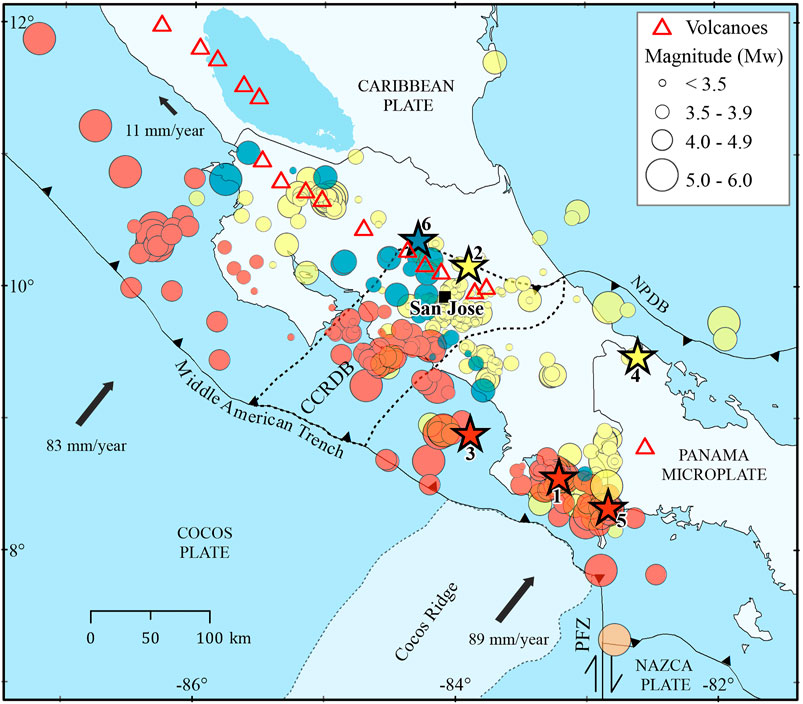
FIGURE 1. Tectonic framework of Costa Rica and felt earthquakes during 2018 and 2019, as reported to RSN. The origin of the earthquakes is differentiated by color: red interplate subduction, orange Panama Fracture Zone, yellow shallow crustal earthquakes, and blue intraslab subduction earthquakes. The region contained within the dotted lines represents the central Costa Rica Deformed Belt (CCRDB). The dashed line represents the simplified northeast boundary of the Central American Forearc Block along the Volcanic Arc Faults (VAF). The numbered stars represent the earthquakes shown in Table 1. The largest events, 1 and 5, are examined in detail. Volcanos in the region are indicated by red triangles.
The National Seismological Network of Costa Rica (RSN) is a research program at the University of Costa Rica (UCR) which includes a seismic network designed to monitor seismic and volcanic activity within the country (Linkimer et al., 2018). Recently, the RSN has focused on rapid dissemination of seismic information and within minutes after an earthquake, data are available in a website, social networks, a smartphone application, and intensity maps (e.g., Porras, 2017).
The aim of an earthquake early warning (EEW) system is to detect and quantify the effect of earthquakes as soon as possible after they have begun and, if necessary, to warn people that they are about to experience strong and potentially destructive shaking (Allen et al., 2009). Conventional EEW systems characterize the location and magnitude of an earthquake based on the rapid detection of the fastest seismic waves, the P-waves, that travel at ∼6 km/s. With the knowledge of the source, an alert can be disseminated to end users through TV, cell phone applications, radio systems, or dedicated alerting devices. In addition to alerting the public, these alerts can be used to perform automated emergency responses, such as the shutdown of critical systems, slowing and stopping of trains to prevent derailment, shutting off gas or water mains, and stopping elevators to the nearest floor and opening its door, to just name a few applications.
EEW systems have been developed in many countries. They are operational in Mexico (Cuéllar et al., 2018), Taiwan (Hsiao et al., 2009), and Japan (Hoshiba and Ozaki, 2014; Kodera et al., 2016), which are regions located in subduction tectonic environments with different potential of generating destructive interplate earthquakes, and California (Given et al., 2018). Test systems continue to run, in particular in Europe (Clinton et al., 2016), for example, in Switzerland (Massin et al., 2021), Italy (Zollo et al., 2014), Romania (Böse et al., 2007), and Turkey (Wenzel et al., 2014).
The effectiveness of EEW systems depends on many factors that include the density of the seismic network, the quality and design of the acquisition, and telemetry infrastructure as well as the data processing resources (Behr et al., 2015). There are other aspects related to the earthquake characteristics which must also be considered, such as the epicentral location, tectonic environment, depth, and fault kinematics.
Over the last decades, SED-ETH has developed EEW methodologies such as the Virtual Seismologist (VS) (Cua and Heaton, 2007), a traditional pick-based point source algorithm, and the Finite Fault Rupture Detector (FinDer) (Böse et al., 2012), an approach that uses the spatial extent of peak ground motions to infer the strike and length of the finite fault. As described in Massin et al. (2021), both these algorithms have been implemented as a set of modules that operate within the SeisComP3 system (Hanka et al., 2010). We call these modules ESE (the ETHZ-SED SeisComP EEW system). Utilizing two very different EEW approaches provide redundant and independent EEW results. VS is more suited for small and intermediate-magnitude earthquakes that are well-approximated by a point-source, while FinDer has been developed to resolve high magnitude earthquakes when source finiteness becomes significant. Their different methodologies provide different advantages and independent solutions to the same EEW system.
Since ESE is embedded within SeisComP, EEW can readily be tested in any SeisComP environment (Massin et al., 2021). SeisComP is widely used across Central America (Massin et al., 2018). In 2016, the Swiss Seismological Service (SED-ETH) and the Nicaraguan Institute of Territorial Studies (INETER) started a joint project named “Earthquake Early Warning in Nicaragua and Central America” (EWARNICA), funded by the Swiss Development Agency to assess the feasibility of EEW in the region, starting with Nicaragua.
In the first phase of the project (2016–2018), a prototype EEW system was implemented at INETER. In a second phase (2018–2021), this system was extended to El Salvador and Costa Rica, and the RSN was able to participate during 2018–2019. Currently, the RSN is not an active partner in this project because the lack of personnel does not allow it to cope with more projects in addition to the pre-existing ones in the UCR.
In this work, we report on the performance of ESE at the RSN. We use the solutions of two earthquakes in Costa Rica and Panama (Mw 6.1 and Mw 6.4) for which the EEW system has performed optimally. In addition, we present results from four smaller earthquakes (Mw 5.1-5.4) from different parts of the country that allows us to further analyze factors such as the earthquake magnitude and the station density. The main motivation to show these results is to document the potential for the development of an EEW system in Costa Rica.
Data and Methodology
Data
The data used in this study primarily comes from the RSN Seismic Network, whose code is TC in the FDSN, the International Federation of Digital Seismograph Network (Red Sismológica Nacional de Costa Rica, 2017). The RSN is composed of 37 broadband (BB) and 121 short period (SP) sensors from which 69 have strong motion (SM) sensors incorporated. These SM sensors are early generation Sixaolas, version 3, with low resolution microelectromechanical system (MEMS) accelerometers. This is problematic for small and moderate earthquakes (Mw < 6), which are poorly resolved even in the near field. This density and quality of strong motion sensors would need to be addressed if an EEW system is to be developed for this network. The median data delay of the TC network is 3.75 s (April 2020), defined as the delay between the signal being recorded at the sensor/datalogger in the field and the arrival of the corresponding digitized waveform at the processing hub (Behr et al., 2015). Though this delay is long, it is not unexpected as the TC network is setup for earthquake and volcano monitoring, but not for EEW systems.
In addition to their own seismic stations, the RSN incorporates real-time data from other seismic networks in Nicaragua (Code NU), Panama (PA), and from the OVSICORI-UNA in Costa Rica (OV), shared directly or via the Incorporated Research Institutions for Seismology (IRIS) (Figure 2). These stations have significantly longer delays. Earthquake locations in the RSN are performed both automatically and manually by using the open-source software SeisComP3 (Hanka et al., 2010) and SeisAn (Havskov et al., 2020), respectively.
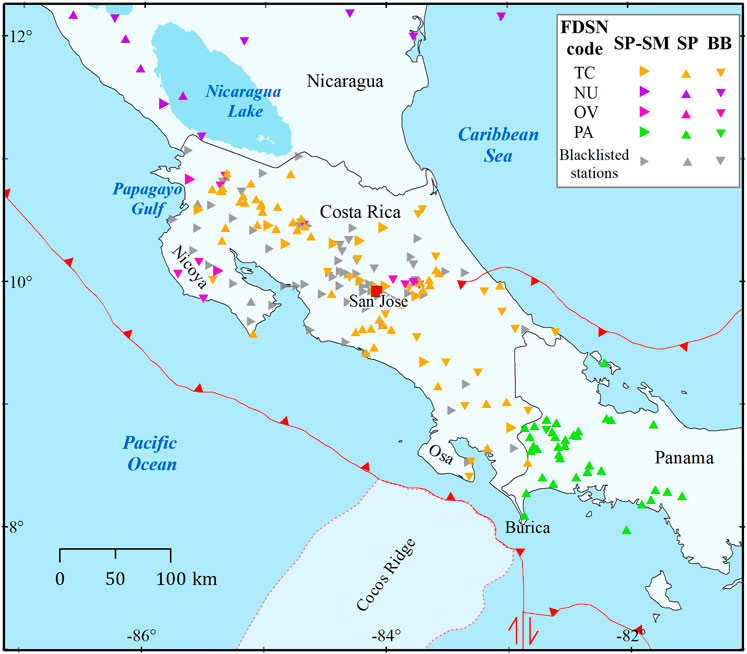
FIGURE 2. Seismic stations used in this study. BB are broadband sensors, SP are short-period geophones, and SP-SM are short period and strong motion sensors. The stations are differentiated by FDSN (International Federation of Digital Seismograph Network) web services: TC, Red Sismológica Nacional de Costa Rica (light grey); NU, Nicaraguan Seismic Network (light blue); OV, Observatorio Vulcanológico y Sismológico de Costa Rica (light red); and PA, ChiriNet, Panama (orange). The blacklisted stations are represented in black color.
Six significant earthquakes that occurred during 2018 and 2019 were selected to analyze the performance of the EEW system (Table 1 and Figure 1). The first earthquake, which we call the Golfito earthquake, happened on August 17th, 2018, 23:22:24 UTC, with a magnitude of Mw 6.2 and a depth of 21 km. This event happened in the South Pacific of Costa Rica and is associated to the subduction of the Cocos plate beneath the Caribbean plate. The solution of the focal mechanism reported by the RSN shows a thrust fault with strike: 291°, dip: 52°, and rake: 90°. This earthquake generated intensities of up to VI in the epicentral area and of IV in San Jose at a distance of 180 km (Arroyo and Linkimer, 2021).

TABLE 1. Seismic source parameters reported by the RSN for the six earthquakes used in this study. N=number, OT = origin time, Mw = moment magnitude, Lon = longitude, Lat = latitude, D. (km) = epicentral distance to San Jose, and T. (s) = time for S-wave to arrive at San Jose.
The second event, referred to as Armuelles earthquake, took place near the locality of Armuelles in Panama, few kilometers away from the border with Costa Rica. It is associated to a strike-slip fault (strike: 304°, dip: 76°, and rake: 4°) within the subducting Cocos plate. This earthquake occurred on June 26th, 2019, at 05:23:48 UTC, with a magnitude of Mw 6.4 and a depth of 29 km. Intensities of VI-VII were reported in the localities of Golfito and Paso Canoas in Costa Rica and Armuelles and David in Panama, while in San Jose, the Central Pacific, and the South Caribbean of Costa Rica, it was felt with intensities of IV-V (Red Sismológica Nacional de Costa Rica, 2019). This event nucleated 50 km SE of the Golfito event.
The other four events analyzed are intermediate-magnitude earthquakes (Mw 5.1-5.4) located in different regions of Costa Rica (Table 1 and Figure 1). One of them occurred on November 17th, 2018 (Mw 5.1), in the central part of the country, where the network density is high, with a depth of 8 km and is associated to a shallow crustal fault. The next earthquake took place on January 31st, 2019 (Mw 5.4), offshore the central Pacific of Costa Rica at a depth of 15 km and associated to the subduction process. A subsequent event took place on April 1st, 2019 (Mw 5.2), originated by a crustal fault in the southern Caribbean of Costa Rica at a depth of 10 km. The last earthquake nucleated on August 6th, 2019 (Mw 5.4), in the Wadati–Benioff zone beneath central Costa Rica, at 105 km depth.
Methodology
The deployment process of the EEW system at the RSN started with the setup of a Dell Workstation with 6 cores in the RSN laboratory; it was modified with a 500 GB solid state drive disk and 12 GB random access memory to work as a dedicated EEW server. Ubuntu server 16.04 LTS was chosen as the operating system and SeisComP3 was installed and configured to read real-time waveforms from the RSN main acquisition server via the Seedlink protocol.
For each earthquake analyzed, the waveforms were processed by using the VS and FinDer algorithms compiled within the SeisComP software in the EEW server. The VS method is a pick-based point-source approach for EEW. The location is determined using the standard SeisComP module scautoloc, requiring 6 triggered stations. The VS magnitude as implemented at the time required a minimum of 3 s of data following the P-wave arrival. This algorithm combines relationships between the final magnitude and the ratios between ground motion acceleration and displacement and specific ground motion prediction equations (GMPEs) for P- and S-waves (Cua and Heaton, 2007). Once a first solution is available, VS solutions are updated every second, each one consisting of values of magnitude, latitude, longitude, depth, and creation time.
The FinDer EEW algorithm (Böse et al., 2012; Böse et al., 2018) uses template matching to automatically provide estimates of the fault rupture extent in real-time (assuming a line-source) by estimating the current centroid position, length, and strike. Unlike VS, FinDer is not based on picks. Its approach compares the observed spatial extent of ground motion with a set of pre-calculated fixed depth templates using a combined grid-search and divide-and-conquer approach. FinDer keeps track of the evolving dimensions of a rupture in progress until peak shaking is reached. In case of a major earthquake, where the finite fault is significant, the estimates of source geometries as provided by FinDer make predicted shaking intensities more accurate for EEW (Böse et al., 2018).
In contrast to VS, the FinDer solutions presented in this study are updated irregularly, only when a new solution differs from the previous one. It is important to highlight that VS uses any sensor type for detection, and for magnitude, it can use all on-scale data, whereas FinDer can only use unsaturated BB or SM data (current configuration within ESE is to identify a waveform as saturated once it reaches with a raw amplitude (in counts) above 80% of 223; this assumes all digitizers are 24 bit). This is problematic at the RSN as the SM sensors are noisy and the majority of the streamed real-time data come from SP sensors, not used by FinDer. FinDer is also more sensitive to gross errors in sensor metadata gains, timing errors, and late arriving data.
In our study, the warning times available for each earthquake assume the strong shaking arrives with the first arriving S-waves. The S-wave velocity is based on the P-wave velocity model for Costa Rica from Quintero and Kissling (2001) and a Vp/Vs ratio of 1.75, as used by the RSN. In this study, all warnings were calculated for the capital city San Jose as target, which is also the most populated region of Costa Rica (Figure 2).
The key parameters used to evaluate EEW solutions here are the location error—the difference in km between the final RSN hypocenter and each EEW hypocenter; the magnitude error—the difference between the final RSN magnitude and each EEW magnitude; and the time difference between the origin time of each earthquake which is based on the final RSN location and the creation time for each EEW solution.
The results presented in this paper span the one year testing period of 2018–2019. Following the larger earthquakes, the performance was reviewed, and the algorithms were tuned using event playback, which favored an improvement of the EEW system over time. During this step, we optimized the configuration of the P-wave arrival detection parameters and the hypocenter location grid, and we created and managed a blacklist of problematic stations (e.g., those with high latency or excessively noisy stations). Blacklists are maintained independently for each algorithm, as they are susceptible to different issues. Crucially, EEW algorithms are very susceptible to individual timing and metadata errors, in particular in terms of gain. We performed a detailed revision of the seismic network metadata and improved the telemetry configuration and delay by decreasing the number of retransmission nodes.
Results
Tables 2 and 3 summarize the real-time performance of VS and FinDer with the first, last, and best solution, which was chosen as the solution with the smallest difference in location error when compared to the reference revised network solution (RSN). The complete list of the VS and FinDer solution updates for each earthquake is available in the supplementary material. In this analysis, we do not discuss the line-source component of the FinDer solutions (which actually is the most important output of FinDer); we treat FinDer as point-source algorithm reporting the FinDer centroid solution and the equivalent magnitude results.
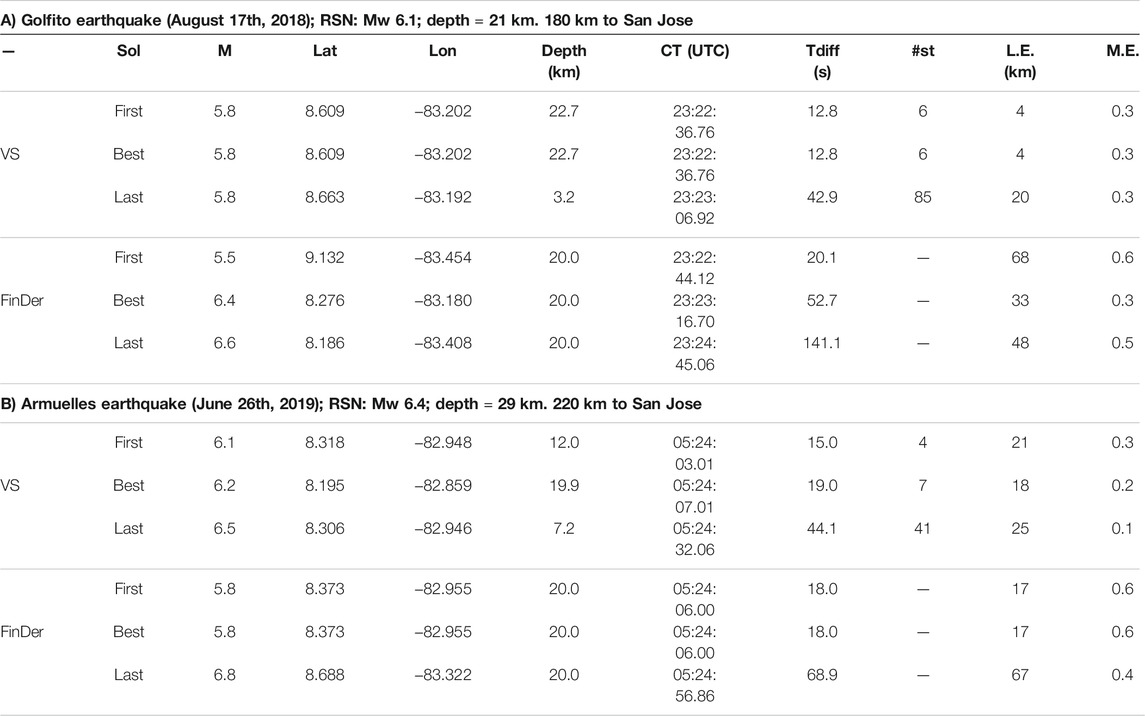
TABLE 2. Solutions of the Virtual Seismologist (VS) and Finite Fault Rupture Detector (FinDer) for the Golfito and Armuelles earthquakes. Sol = solution, M = magnitude value (VS or FinDer), Lat = latitude, Lon = longitude, CT = creation time, Tdiff (s) = time difference in seconds between CT and OT, #st = number of stations used in the VS solution, L.E. (km) = location error, and M.E. = absolute value of magnitude error. The best solutions are chosen when the smallest location differences are obtained compared to the RSN solution.
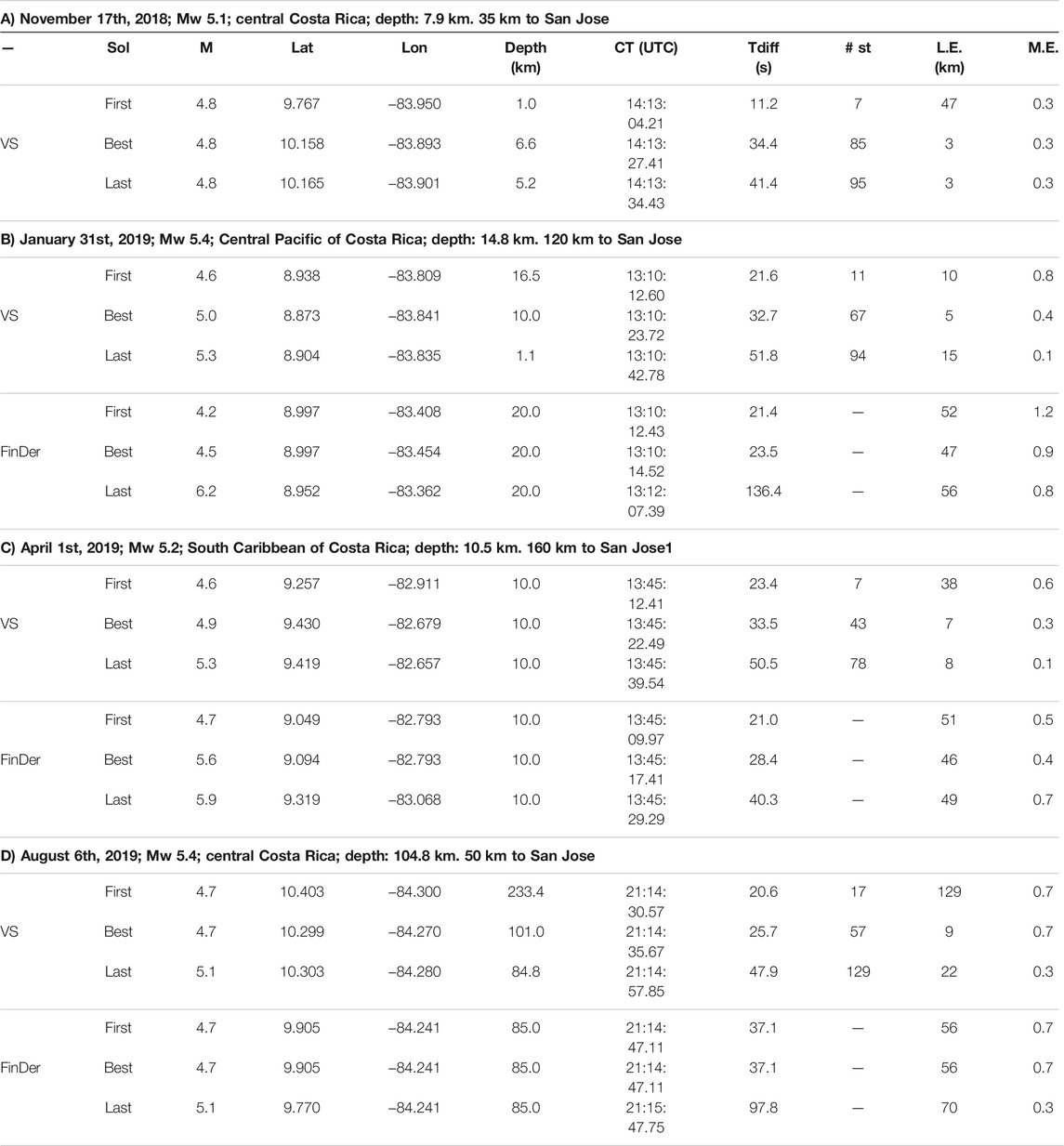
TABLE 3. Solutions of VS and FinDer for the other four earthquakes in different regions of Costa Rica. Sol = solution, M = magnitude, Lat = latitude, Lon = longitude, CT = creation time, Tdiff (s) = time difference in seconds between CT and OT, #st = number of stations used in the VS solution, L.E. (km) = location error, and M.E. = absolute value of magnitude error. The best solutions are chosen when the smallest location differences are obtained compared to the RSN solution.
Golfito Earthquake
The Mw 6.2 Golfito earthquake occurred on August 17, 2018. VS provided 31 and FinDer 20 updates in real time. The first VS and FinDer solutions were determined 12.8 and 20.1 s after the origin time (OT), with location errors of 4 and 68 km, respectively (Table 2). The blind zone of this earthquake covered a radius of ∼40 km from the epicenter (Figure 3); all VS solutions were located within 20 km of the RSN epicenter, while the FinDer solutions had location errors between 33 and 70 km (Figures 4A,B). Considering that San Jose is 181 km from the hypocenter and a S-wave velocity of 3.7 km/s, the EEW system issued a warning time of 36 s before the S-wave arrived in San Jose, where an intensity of IV was experienced.
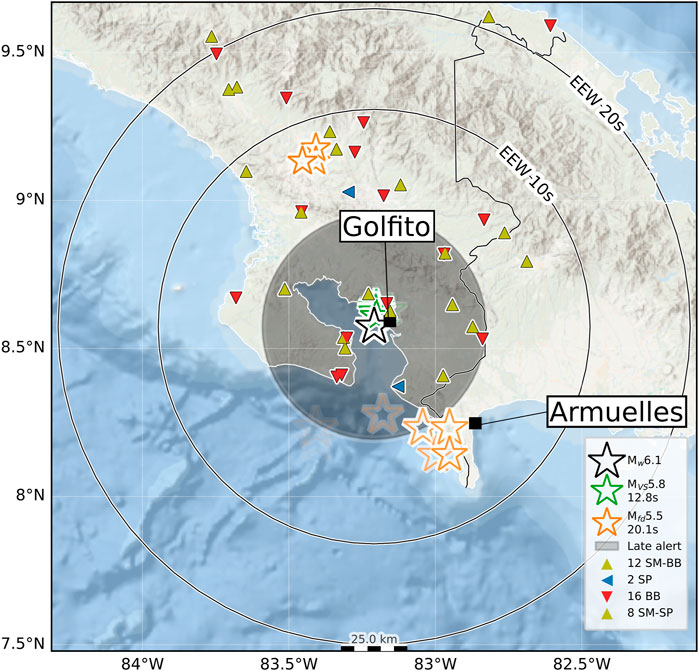
FIGURE 3. Map of the 2018 Golfito earthquake (Mw 6.1). Solutions provided by VS and FinDer are shown as green and orange stars, respectively. The RSN location is represented as a black star (Arroyo and Linkimer, 2021). Triangles represent the seismic stations and are color coded (see inset) based on the sensor type, as short period (SP), broad band (BB), and strong motion (SM). SP stations are used by VS, but not by FinDer. The blind zone is the concentric shadow area around the RSN location and represents the late alert zone. The thin black circles represent the warning time at different distances from the epicenter.
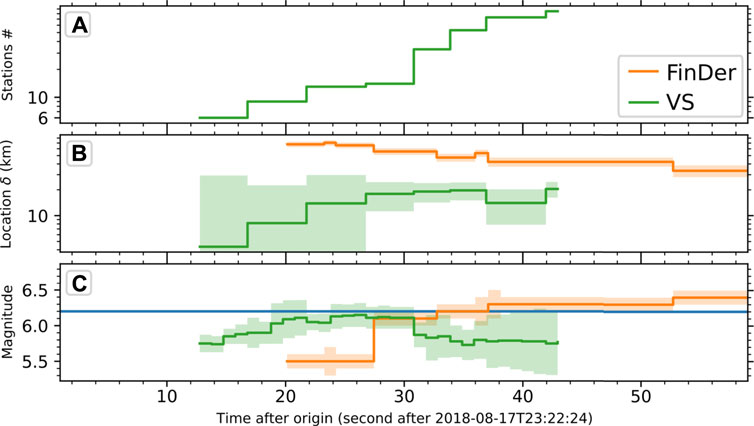
FIGURE 4. Time evolution of the (A) number of stations contributing to the location, (B) the location error (km), and (C) EEW magnitude for the 2018 Golfito earthquake (Mw 6.1).
Though both algorithms correctly and immediately recognized the event to be significant, their initial magnitudes were underestimated, with errors up to 0.5 units for both VS and FinDer (Figure 4C). They approached the Mw 6.2 reference magnitude with the incorporation of more stations. The best VS and FinDer solutions were obtained 12.8 and 52.7 s after the OT, with location errors of 4 and 33 km, respectively (Table 2).
This event occurred shortly after the collaboration began, and since alerts were sounded at RSN using the open-source software Earthquake Early Warning Display (EEWD) (Cauzzi et al., 2016), many seconds before the ground motions were felt, it was very encouraging and provided a first real-time experience of the performance of the EEW system. It was the first big event after the deployment of the EEW system, and, despite no significant effort to optimize the network for EEW, the system performed very well with a first solution 12 s after the OT and location error of 4 km.
During the Golfito earthquake, all available stations were used by the EEW system, and, without being optimized, the main limitations on the algorithms were that the FinDer location error was always above 30 km, which is expected as this earthquake is located at the edge of the network. Furthermore, the two closest stations (TC.EDAD. and TC.EDS2) had huge delays during the Golfito earthquake, and a number of close-by stations (FITO, JIME, NELY, and PANO) had been blacklisted for FinDer, because of previous issues. Despite that, the FinDer magnitude was stable, and the line-source strike was estimated as 330o, which is close to the RSN moment tensor solution (286o) (Arroyo and Linkimer, 2021). In contrast, the VS location errors remained always below 20 km; however, the VS magnitude dropped significantly after 30 s when P-wave energy from the many stations in the central valley near San Jose began to be incorporated.
After this event, we identified several issues to improve. The PA stations in Figure 2 were not incorporated into the RSN network, thus leaving a big gap towards Panama. Changes in the configuration of the EEW system needed to be made including the blacklisting of stations with recurrent false trigger, adjustment of the STA/LTA detection and AIC re-picker filters, incorporation of the UCR velocity model, and optimization of the location grid used by SeisComP.
Armuelles Earthquake
Nearly one year later, the largest event during the testing period, the Armuelles earthquake, occurred 50 km to the SE of the Golfito earthquake. At that time, the PA stations were already incorporated into the RSN network (though with higher latency) and the algorithms had been optimized as described before. At this stage, the blacklist includes 82 stations.
VS provided 30 and FinDer 14 solutions during this event. At that time, VS had been modified to produce a solution with only four stations instead of six, resulting in the first two solutions provided 15.0 and 16.0 s after the OT with location errors of 21 km, while the last VS solution was estimated with 41 readings 44.1 s after the OT. On the other hand, the first and last FinDer solutions were provided 18 and 68.9 s after the OT (Table 2). The blind zone of this event covers a radius of 50 km from the epicenter and considering its hypocenter at 222 km from San Jose (Figure 5) and an S-wave velocity of 3.9 km/s resulted in 43 s of warning time for the Armuelles earthquake.
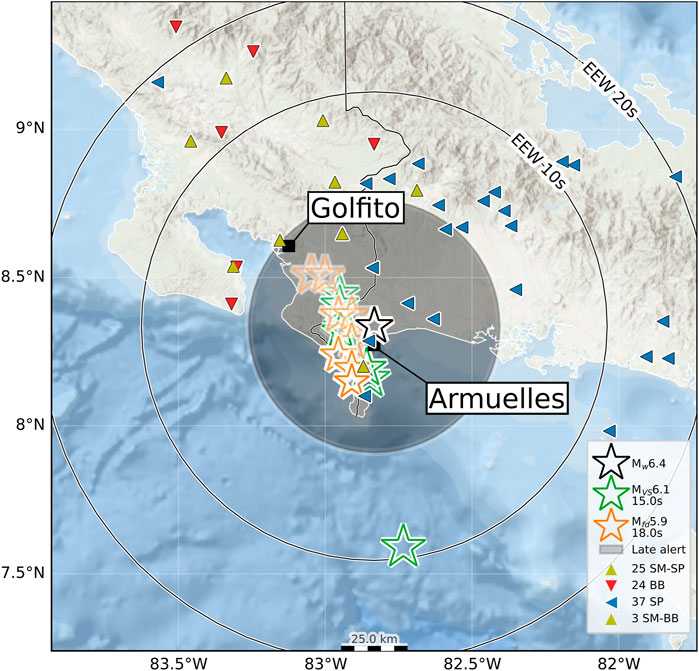
FIGURE 5. Map of the 2019 Armuelles earthquake (Mw 6.4). Solutions provided by VS and FinDer are shown as green and orange stars, respectively. The RSN location is represented as a black star. Triangles represent the seismic stations and are color coded (see inset) based on the sensor type, as short period (SP), broad band (BB), and strong motion (SM). SP stations are used by VS, but not by FinDer. The blind zone is the concentric shadow area around the RSN location and represents the late alert zone. The thin black circles represent the warning time at different distances from the epicenter.
The VS location errors ranged between 18 and 84 km with 7 and 5 stations, respectively, while for FinDer, the errors were between 17 and 67 km, respectively (Figures 6A,B). At 17.0 s after the OT, a false pick increased dramatically the VS location error by placing the solution 83 km south of the RSN location; however, two seconds later, the error decreased to 18 km after incorporating two more stations into the VS solution. The FinDer locations for the Armuelles earthquake were more stable than those provided by VS, with errors of less than 30 km during the first 31 s along an alignment marked by the solution updates, which might give insights into the evolution of the earthquake rupture during the updating process.
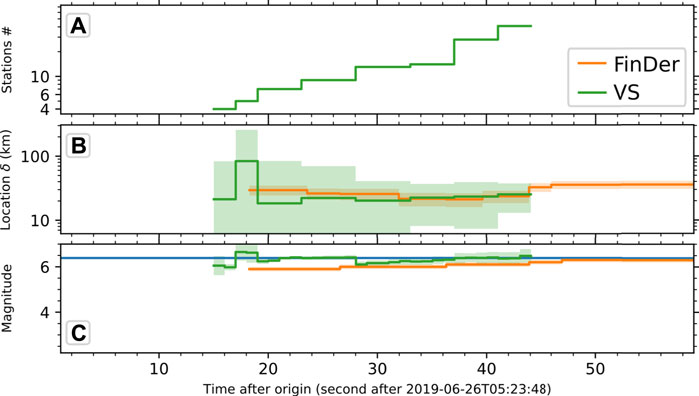
FIGURE 6. Time evolution of the (A) number of stations contributing to the location, (B) the location error (km), and the (C) EEW magnitude for the 2019 Armuelles earthquake (Mw 6.4).
The magnitude variation for both algorithms is much more stable than the magnitude performance of the Golfito earthquake (Figure 6C), with errors of less than one unit. The fact that the earliest solution was available 15 s after the OT, that is, 3 s later than the first solution of the Golfito earthquake, can be explained by the increased depth of the event (7 km deeper) and the location further south and across the border, further away from the denser parts of the network. The additional stations in Panama, despite their higher latency and only available to VS, contributed positively to obtain stable magnitude and location results. At the time of the Armuelles earthquake, we had a more robust EEW system.
Intermediate-Magnitude Earthquakes
In addition to the two significant earthquakes described before, the EEW solutions for four intermediate-magnitude (Mw 5.1-5.4) seismic events in different regions of Costa Rica are reported to observe the effect of the seismic network density and the geographical variation of earthquakes in the EEW performance (Figure 7).
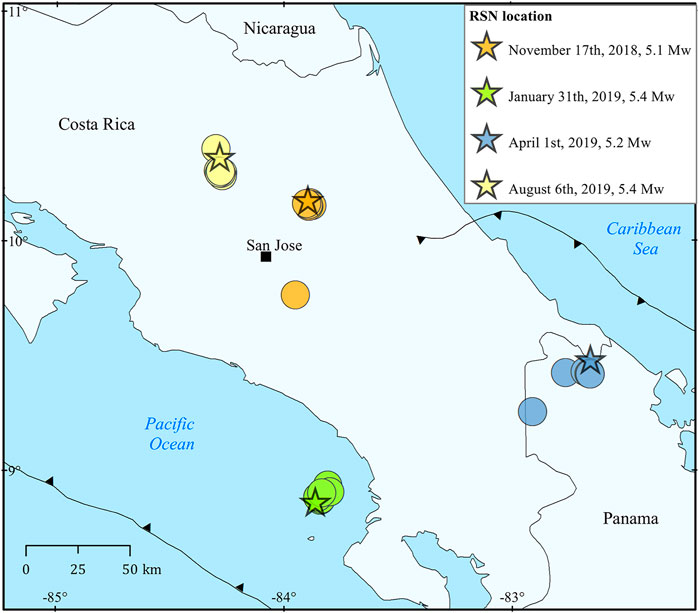
FIGURE 7. Solutions for four events selected in different regions of Costa Rica (Table 3). The stars represent the RSN locations, and the circles represent the VS solutions. The colors correspond to the date of the earthquake, as shown in the inset.
The event of November 17th, 2018 (Mw 5.1), in the central part of the country where the network density is high occurs within 35 km of San Jose and was the closest event to the capital from the earthquakes analyzed. The first VS solution was available just 11.2 s after the OT with seven stations and a location error of 47 km due to single false pick, which was corrected after two seconds, leading to a more reasonable error of 12 km that is dominated by an error in depth. The best solution was obtained with a location error of 3 km, 85 stations, and 34.4 s after the OT (Table 3). The magnitude estimation was very stable, varying between 4.7 and 4.8. FinDer was not running at that time. Regardless of its fast first solution 11 s after the OT, the alert arrived one second after the S-wave arrival in San Jose due to its vicinity to the target.
The first solution for the earthquake on January 31st, 2019, in the Central Pacific of Costa Rica (Mw 5.4) came from FinDer 21.4 s after the OT with a location error of 52 km. This event was outside of the network coverage, and we obtained a warning time of 12 s for San Jose. The high location error was corrected to 10 km with the first VS solution 0.2 s later with 11 stations. The VS location error decreased up to 5 km 32.7 s after the OT with 67 stations (Table 3).
For the event that took place on April 1st, 2019, in the South Caribbean of Costa Rica with Mw 5.2, the first solution came also from FinDer 21.0 s after the OT with a location error of 51 km and a magnitude of 4.7. The first VS solution was available two seconds later, with seven stations and a location error of 38 km. A first alert provided a warning of 21 s for San Jose. The VS location error decreased to 15 km 30 s after the OT with 23 stations and the best VS solution was obtained 33.5 s after the OT with 43 stations and a location error of 7 km (Table 3). Recalling that FinDer uses only data from BB and SM sensors to match ground motion templates, its performance for the middle-magnitude events in the Central Pacific and South Caribbean of Costa Rica was affected by the few (<4) BB triggered stations and the blacklisted low resolution MEMS SM sensors.
Another earthquake analyzed occurred on August 6th, 2019 (Mw 5.4), in the Wadati–Benioff zone beneath central Costa Rica, at 105 km depth. The significant depth of this event resulted in a first VS solution obtained 20.6 s after the OT, with a location error of 129 km by using 17 stations, which decreased rapidly to an error of 31 km just two seconds later with 37 stations and to 9 km after 3 s with 57 stations. The high density of stations in central Costa Rica contributed to rapidly improve the VS source parameters. On the contrary, FinDer was limited by the few BB and SM sensors EEW capable, obtaining the first solution 37.1 s after the OT with location error of 56 km (Table 3). Even though the epicenter was located in a high station density region, we obtained only 4 s of warning due to the significant depth of this event.
Discussion
The RSN seismic network, composed mainly of SP sensors with low resolution SM MEMS and with a high median data delay of 3.75 s, is not designed for EEW systems since RSN is oriented towards earthquake and volcano monitoring using pick-based algorithms. With this in mind, the EEW system was deployed at RSN to assess its potential in Costa Rica with the existing infrastructure.
Evaluating the EEW performance over time with different events becomes difficult, as the number of stations monitored by the seismic network, their EEW performance, and the configuration of the EEW system were not constant over the test period. Also, the performance of VS and FinDer are compared in terms of point-source characteristics, even though FinDer is also capable of tracking the current rupture size (which is actually the main output of FinDer).
The first EEW solutions for the Golfito (12.8 s) and Armuelles (15 s) earthquakes are similarly fast considering differences in event depth, but subsequent EEW updates for the Armuelles earthquake are more stable. This is attributed to the optimization of the EEW system, for which the main changes included the incorporation of 25 SP stations from the PA network after the Golfito earthquake (which help improving the VS estimates), the blacklisting of 82 stations which are not EEW operational (due to low resolution data, poor timing, or high latency) and other changes in configuration, such as the STA/LTA detector, re-picker filters, and the customization of the location grid. It is noted that once the network has been optimized, FinDer approaches and at times exceeds the speeds of the VS alerts.
The aforementioned customization of the EEW system provided a much more robust FinDer performance during the Armuelles earthquake, with a much lower FinDer location error than the one for the Golfito earthquake and a low magnitude fluctuation for both algorithms. These results show that the EEW system at RSN already operated satisfactorily without any customization for VS, and with relatively minor efforts, we were able to improve the FinDer speed and stability. A number of critical issues to FinDer, such as the handling of latent data and faster magnitude convergence, have been addressed in the latest FinDer release in 2021.
The results of the intermediate-magnitude earthquakes indicate that VS performs better than FinDer in the RSN network since it is composed mainly of SP sensors and the VS system can use these for location and also for magnitude, when on-scale. The VS magnitudes are generally robust even using few stations. Thus, VS has an advantage especially in the regions of higher station density including different sensor types, such as central Costa Rica.
Limitations related to the seismic network have been revealed. These include the high median data delay of 3.75 s that would need to be reduced for EEW. Other station issues observed are GPS timing problems and low resolution of the strong motion MEMS sensors (Figure 8A); these problems led to a large proportion of the network, 82 stations, being blacklisted, significantly reducing the number of operational EEW stations at RSN. A final challenge observed was the lack of network resilience during strong motions. At numerous near-field stations, the signal was lost shortly after the P-wave arrival, most likely due to failure of communication or mains power (Figure 8B). Redundancy here should be considered. The efforts for network optimization outlined here have to be taken if EEW is to become a product at RSN (Massin et al., 2020).
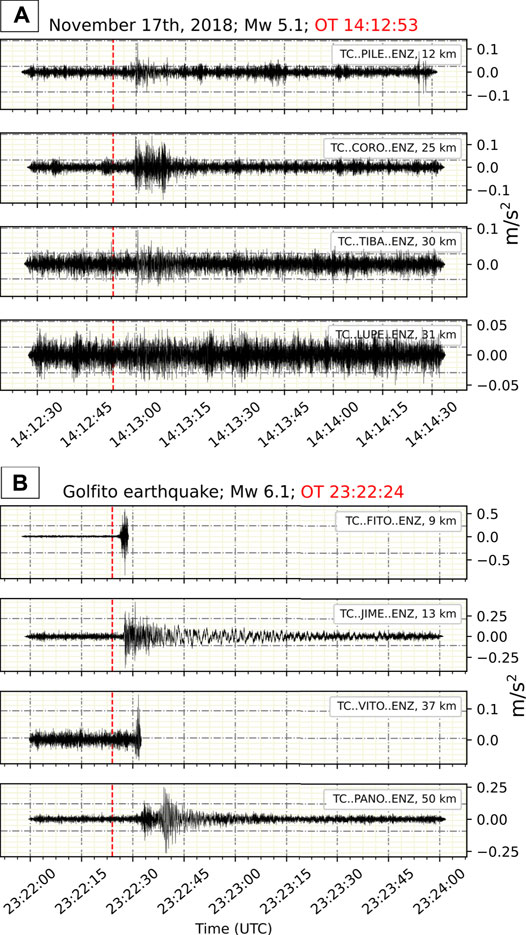
FIGURE 8. Closest four vertical SM recordings of the (A) November 17th, 2018 (Mw 5.1), and (B) Golfito (Mw 6.1) earthquakes. The vertical red lines indicate the origin times. Left vertical axis is in counts and the corresponding acceleration value is indicated in the right vertical axis. The epicentral distance of each station is indicated in the labels after the station name. (A) The low resolution of the strong motion MEMS sensors and (B) the lost signals shortly after the P-wave arrival during the Golfito earthquake, most likely due to failure of communication or mains power.
As with all EEW systems, we can expect alerts to arrive only after the onset of strong shaking for earthquakes close to the target. With the examples presented here, where alerts have been provided within 11 s of event initiation, we can expect late alerts for all shallow crustal events within 40 km of the capital. Unfortunately, if the present network delivers EEW, this would mean late alerts for repeats of some historically destructive events near San Jose, such as the Cartago earthquake (M 6.4) in 1910 (Montero and Miyamura, 1981) and the Cinchona earthquake (Mw 6.1) in 2009 (Linkimer and Alvarado, 2014), which were originated by local faults with epicenters at 20 and 30 km of the city, respectively.
The alert time is modest (<5 s) for seismic events close to San Jose originated in the subduction zone, such as the 1990 Cobano (Mw 7.0) and 1999 Quepos (Mw 6.9) earthquakes, and at intermediate depth in the subducting slab, like the 1992 Naranjo earthquake (Mw 6.5). Nevertheless, warnings could be sent for the public in coordination with local authorities. Like in other nations, automated procedures for heavily-exposed industrial partners can also be considered, including actions mentioned before. It is also worth noting that even if alerts are late, EEW can provide earthquake parameters and shaking information concurrent with the arrival of ground motions, which can be effectively used to inform geoscientists, civil authorities, and the wider public before the onset of cascading failures of communications and power infrastructures that may occur.
In summary, it is clear that even with the performance presented here, the EEW can be useful for providing rapid earthquake notification for San Jose. During the testing period, a small crustal earthquake (May 9th, 2019, Mw 3.3) near San Jose was detected by VS after only 5.8 s. As the event was small and only very locally felt, it was not detailed in this study. If results like this could be replicated by using an improved seismic network, the epicentral region that receives late alerts may be reduced significantly.
Conclusions
With the existing RSN network, which has not been optimized for EEW systems, and using the SED-developed ESE EEW system for selected earthquake of 2018–2019, we observe warning times for San Jose of 36–43 s for distant (180–220 km) earthquakes. Epicenters at less than 40 km do not allow positive warning times for the metropolitan area of San Jose. FinDer has performed well for the M > 6 earthquakes; however, it is limited for the intermediate-magnitude (Mw 5.1–5.4) events since the majority of stations in the RSN network include SP sensors not used by FinDer. Additionally, VS, trigger-based and capable of using any seismic sensor, has proved to be successful for the six earthquakes studied here.
We have identified limitations in the EEW system related to noisy or high-latency stations which reduced the number of operational EEW stations. Despite that, the current RSN network has the potential to provide alerts for large (M > 6) earthquakes occurring at distances larger than 40 km by using FinDer and VS. This could be further improved by increasing the density of operational EEW stations in the country and reducing data delays. We also demonstrated the feasibility of rapid earthquake notification from the current RSN network in all studied cases, potentially providing alerts for disaster response management and seismic risk mitigation.
Data Availability Statement
The original contributions presented in the study are included in the article/Supplementary Material; further inquiries can be directed to the corresponding author.
Author Contributions
JP: former researcher at the RSN; contributed to the implementation and testing of the EEW system and analysis and interpretation of data, writing, and some of the figures. FM: responsible for the implementation of the EEW system; trainer of the staff of the seismic networks in Central America in EEW related topics; contributed to the design of the work, technical information, analysis of data, critical revision, and some of the figures. MA-S: researcher at the RSN; contributed to monitor the EEW system during the testing period; provided substantial contributions to the interpretation and analysis of data, technical information, and critical revision and prepared some of the figures. IA: current coordinator of the RSN; contributed to the data interpretation and writing. LL: coordinated the RSN during a portion of the EEW testing period and contributed to the data interpretation and writing. MB: researcher at the SED; responsible for the development of FinDer; provided substantial contributions to the design of the work, technical information, interpretation and analysis of data, and critical revision. JC: director of seismic networks at SED; responsible for the EWARNICA project; provided substantial contributions to the design of the work, technical information, interpretation and analysis of data, and critical revision.
Funding
This work was mainly supported by the Swiss Development Agency and partially by the projects 113-B5-704 and 113-B9-911 of the University of Costa Rica.
Conflict of Interest
The authors declare that the research was conducted in the absence of any commercial or financial relationships that could be construed as a potential conflict of interest.
Publisher’s Note
All claims expressed in this article are solely those of the authors and do not necessarily represent those of their affiliated organizations, or those of the publisher, the editors, and the reviewers. Any product that may be evaluated in this article, or claim that may be made by its manufacturer, is not guaranteed or endorsed by the publisher.
Acknowledgments
The authors thank the Swiss Development Agency for providing financial support to the EWARNICA project, which serves as framework for this study, and for supporting the training in the SeisComP software for technical staff of the RSN and other seismic networks in Central America. The authors also thank the Nicaraguan Seismic Network (NU, doi: 10.7914/SN/NU), the Observatorio Vulcanológico y Sismológico de Costa Rica (OV, doi: 10.7914/SN/OV), and the ChiriNet Panama seismic network (PA, doi: 10.7914/SN/PA) for sharing some of their stations in real-time with the RSN and reinforcing the seismic network coverage in Costa Rica.
Supplementary Material
The Supplementary Material for this article can be found online at: https://www.frontiersin.org/articles/10.3389/feart.2021.700843/full#supplementary-material
References
Allen, R. M., Gasparini, P., Kamigaichi, O., and Böse, M. (2009). The Status of Earthquake Early Warning Around the World: An Introductory Overview. Seismol. Res. Lett. 80 (5), 682–693. doi:10.1785/gssrl.80.5.682
Arroyo, I. G., and Linkimer, L. (2021). Geometría de la zona sismogénica interplacas en el Sureste de Costa Rica a la luz de la secuencia de Golfito del 2018. GeofInt 60 (1), 51–75. doi:10.22201/igeof.00167169p.2021.60.1.2026
Behr, Y., Clinton, J., Kästli, P., Cauzzi, C., Racine, R., and Meier, M.-A. (2015). Anatomy of an Earthquake Early Warning (EEW) Alert: Predicting Time Delays for an End-To-End EEW System. Seismol. Res. Lett. 86 (3), 830–840. doi:10.1785/0220140179
Böse, M., Ionescu, C., and Wenzel, F. (2007). Earthquake Early Warning for Bucharest, Romania: Novel and Revised Scaling Relations. Geophys. Res. Lett. 34 (7), 1–6. doi:10.1029/2007GL029396
Böse, M., Heaton, T. H., and Hauksson, E. (2012). Real-time Finite Fault Rupture Detector (FinDer) for Large Earthquakes. Geophys. Jour. Int. 191 (2), 803–812. doi:10.1111/j.1365-246X.2012.05657.x
Böse, M., Smith, D. E., Felizardo, C., Meier, M.-A., Heaton, T. H., and Clinton, J. F. (2018). FinDer v.2: Improved Real-Time Ground-Motion Predictions for M2-M9 with Seismic Finite-Source Characterization. Geophys. J. Int. 212, 725–742. doi:10.1093/gji/ggx430
Cauzzi, C., Behr, Y., Clinton, J., Kästli, P., Elia, L., and Zollo, A. (2016). An Open‐Source Earthquake Early Warning Display. Seism. Res. Lett. Online 87 (3), 737–742. doi:10.1785/0220150284
Clinton, J., Zollo, A., Marmureanu, A., Zulfikar, C., and Parolai, S. (2016). State-of-the Art and Future of Earthquake Early Warning in the European Region. Bull. Earthquake Eng. 14, 2441–2458. doi:10.1007/s10518-016-9922-7
Cua, G., and Heaton, T. (2007). “The Virtual Seismologist (VS) Method: A Bayesian Approach to Earthquake Early Warning,” in Earthquake Early Warning Systems (Berlin, Heidelberg: Springer), 97–132.
Cuéllar, A., Suárez, G., and Espinosa‐Aranda, J. M. (2018). A Fast Earthquake Early Warning Algorithm Based on the First 3 S of the P‐Wave Coda. Bull. Seismol. Soc. Am. 108 (4), 2068–2079. doi:10.1785/0120180079
DeMets, C., Gordon, R. G., Argus, D. F., and Stein, S. (1994). Effect of Recent Revisions to the Geomagnetic Reversal Time Scale on Estimates of Current Plate Motions. Geophys. Res. Lett. 21 (20), 2191–2194. doi:10.1029/94gl02118
Given, D., Allen, R. M., Baltay, A. S., Bodin, P., Cochran, E. S., Creager, K., et al. (2018). Implementation Plan for the ShakeAlert System-An Earthquake Early Warning System for the West Coast of the United States. Pasadena, CA: US Geological Survey (USGS) Open-File Report, 1155. Available at: https://pubs.usgs.gov/of/2018/1155/ofr20181155.pdf.
Hanka, W., Saul, J., Weber, B., Becker, J., and Harjadi, P. (2010). Real-time Earthquake Monitoring for Tsunami Warning in the Indian Ocean and beyond. Nat. Hazards Earth Syst. Sci. 10 (12), 2611–2622. doi:10.5194/nhess-10-2611-2010
Havskov, J., Voss, P. H., and Ottemöller, L. (2020). Seismological Observatory Software: 30 Yr of SEISAN. Seismol. Res. Lett. 91 (3), 1846–1852. doi:10.1785/0220190313
Hoshiba, M., and Ozaki, T. (2014). “Earthquake Early Warning and Tsunami Warning of the Japan Meteorological Agency, and Their Performance in the 2011 off the Pacific Coast of Tohoku Earthquake (Mw 9.0),” in Early Warning for Geological Disasters (Berlin, Heidelberg: Springer), 1–28. doi:10.1007/978-3-642-12233-0_1
Hsiao, N.-C., Wu, Y.-M., Shin, T.-C., Zhao, L., and Teng, T.-L. (2009). Development of Earthquake Early Warning System in Taiwan. Geophys. Res. Lett. 36 (5), 3–7. doi:10.1029/2008GL036596
Kodera, Y., Saitou, J., Hayashimoto, N., Adachi, S., Morimoto, M., Nishimae, Y., et al. (2016). Earthquake Early Warning for the 2016 Kumamoto Earthquake: Performance Evaluation of the Current System and the Next-Generation Methods of the Japan Meteorological Agency. Earth Planets Space 68 (1), 202. doi:10.1186/s40623-016-0567-1
Linkimer, L., and Alvarado, G. E. (2014). Distribución espacio-temporal de la sismicidad sentida en Costa Rica (1976-2013) en el marco histórico del 30 aniversario (1982-2012) de la Red Sismológica Nacional (RSN: UCR-ICE). Rev. Geol. Amér. Cent. 30, 45–71. doi:10.15517/rgac.v0i0.16569
Linkimer, L., Arroyo, I. G., Alvarado, G. E., Arroyo, M., and Bakkar, H. (2018). The National Seismological Network of Costa Rica (RSN): An Overview and Recent Developments. Seismol. Res. Lett. 89 (2A), 392–398. doi:10.1785/0220170166
Lücke, O. H., and Arroyo, I. G. (2015). Density Structure and Geometry of the Costa Rican Subduction Zone from 3-D Gravity Modeling and Local Earthquake Data. Solid Earth 6, 1169–1183. doi:10.5194/se-6-1169-2015
Massin, F., Strauch, W., Andrews, C. J., Böse, M., and Ramirez, J. (2018). Building EEW in Nicaragua: Performance and Perspectives. Seismol. Res. Lett. 89 (2B), 717–966. doi:10.1785/0220180082
Massin, F., Clinton, J., Racine, R., Böse, M., Rossi, Y., Marroquin, G., et al. (2020). “The Future strong Motion National Seismic Networks in Central America Designed for Earthquake Early Warning,” in EGU General Assembly Conference Abstracts, Vienna, Austria, 19437. doi:10.1785/0220200043
Massin, F., Clinton, J. F., and Böse, M. (2021). Status of Earthquake Early Warning in Switzerland. Lausanne, Switzerland. Front. Earth Sci. 9, 707654. doi:10.3389/feart.2021.707654
Montero, W., and Miyamura, S. (1981). Distribución de intensidades y estimación de los parámetros focales de los terremotos de Cartago de 1910, Costa Rica, América Central. Rev. Inst. Geogr. Nacional. Julio-diciembre 2, 9–34.
Montero Pohly, W. (1989). Sismicidad Histórica De Costa Rica. GeofInt 28 (3), 531–559. doi:10.22201/igeof.00167169p.1989.28.3.623
Montero, W. (2001). Neotectónica de la región central de Costa Rica: frontera oeste de la microplaca de Panamá. Rev. Geol. Amér. Cent. 24, 29–56.
Peraldo, G., and Montero, W. (1994). Los temblores del periodo colonial de Costa Rica. San Jose, Costa Rica: Edit. Tecnol. Costa Rica.
Porras, J. L. (2017). Atenuación sísmica en Costa Rica a partir de intensidades y Coda Q. [license’s thesis]. San Jose, Costa Rica: University of Costa Rica.
Quintero, R., and Kissling, E. (2001). An Improved P-Wave Velocity Reference Model for Costa Rica. GeofInt 40 (1), 3–19. doi:10.22201/igeof.00167169p.2001.40.1.416
Red Sismológica Nacional de Costa Rica (2017), Información de la Red Sismológica Nacional de Costa Rica,” in The Costa Rica National Seismological Network Catalog during 1975-2017. Universidad de Costa Rica. doi:10.15517/TC
Red Sismológica Nacional de Costa Rica (2019). Informe preliminar sobre el sismo de Armuelles, 25 de junio de 2019. Available at: https://rsn.ucr.ac.cr/actividad-sismica/reportes-sismicos/12963-informe-preliminar-sobre-el-sismo-de-armuelles-25-de-junio-de-2019 (Accessed October 15, 2020).
Wenzel, F., Erdik, M., Köhler, N., Zschau, J., Milkereit, C., Picozzi, M., et al. (2014). “EDIM: Earthquake Disaster Information System for the Marmara Region, Turkey,” in Early Warning for Geological Disasters: Scientific Methods and Current Practice (Heidelberg: Springer-Verlag), 103–116. doi:10.1007/978-3-642-12233-0_6
Zollo, A., Colombelli, S., Elia, L., Emolo, A., Festa, G., Iannaccone, G., et al. (2014). “An Integrated Regional and On-Site Earthquake Early Warning System for Southern Italy: Concepts, Methodologies and Performances,” in Early Warning for Geological Disasters: Scientific Methods and Current Practice (Heidelberg: Springer-Verlag), 117–137. doi:10.1007/978-3-642-12233-0_7
Keywords: Virtual Seismologist, Finite Fault Rupture Detector, seismic network, open-source software, seismic risk mitigation
Citation: Porras J, Massin F, Arroyo-Solórzano M, Arroyo I, Linkimer L, Böse M and Clinton J (2021) Preliminary Results of an Earthquake Early Warning System in Costa Rica. Front. Earth Sci. 9:700843. doi: 10.3389/feart.2021.700843
Received: 26 April 2021; Accepted: 18 August 2021;
Published: 23 September 2021.
Edited by:
Mourad Bezzeghoud, Universidade de Évora, PortugalReviewed by:
Gerardo Suarez, National Autonomous University of Mexico, MexicoSimone Cesca, German Research Centre for Geosciences, Germany
Copyright © 2021 Porras, Massin, Arroyo-Solórzano, Arroyo, Linkimer, Böse and Clinton. This is an open-access article distributed under the terms of the Creative Commons Attribution License (CC BY). The use, distribution or reproduction in other forums is permitted, provided the original author(s) and the copyright owner(s) are credited and that the original publication in this journal is cited, in accordance with accepted academic practice. No use, distribution or reproduction is permitted which does not comply with these terms.
*Correspondence: Juan Porras, ai5wb3JyYXNsb3JpYUBzdHVkZW50aS51bmlwaS5pdA==