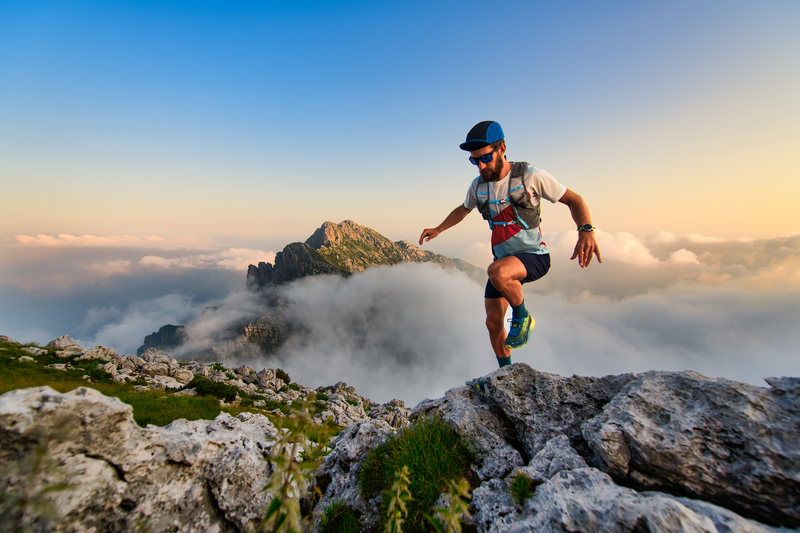
95% of researchers rate our articles as excellent or good
Learn more about the work of our research integrity team to safeguard the quality of each article we publish.
Find out more
EDITORIAL article
Front. Earth Sci. , 17 May 2021
Sec. Hydrosphere
Volume 9 - 2021 | https://doi.org/10.3389/feart.2021.698925
This article is part of the Research Topic Stochastic Modeling in Hydrogeology View all 11 articles
Editorial on the Research Topic
Stochastic Modeling in Hydrogeology
The call for this research topic asked for papers that would support the use of stochastic modeling in Hydrogeology, mainly focusing on proved applications of these techniques. We succeeded in attracting many expressions of interest that finally materialized in the collection of ten papers that makes up this section. Not all ten articles show real applications of stochastic Hydrogeology, but they all highlight the importance of uncertainty quantification in groundwater flow and mass transport modeling, and the need to use stochastic techniques to do it in an appropriate, systematic, and traceable manner.
If you were to read just one paper on this research topic, we suggest you the one by White et al. since it meets all the target objectives. It stresses the importance of parameter estimation (PE) and uncertainty quantification (UQ) and demonstrates it with a fully worked-out example in the Edwards aquifer, Texas, United States. The authors not only guide the reader on the different steps to perform PE and UQ in an aquifer that is complex to model but also provide all the scripts used so that anyone can inspire from their work and apply them to other cases.
Of the other papers demonstrating the importance of adequately handling heterogeneity in aquifer modeling, two focus on facies heterogeneity since this heterogeneity is more critical than the intrinsic heterogeneity of hydraulic conductivity within facies. The large contrasts in hydraulic conductivity are not due to its inherent spatial variability but to hydrofacies heterogeneity. Consequently, there is a need for robust methods for the generation of categorical realizations resembling the geological aquifer architecture. Both Carle and Fogg and Jorreto-Zaguirre et al. propose ways of handling soft or uncertain data in categorical simulation, each one using a different categorical simulation technique. Carle and Fogg demonstrate their findings in the Savanah River, South Carolina, United States and the Llagas basin, California, United States, and Jorreto-Zaguirre et al. in the Andrax Delta, Spain. Of particular interest is the discussion by Carle and Fogg of current methods and the outlook about the future.
Another interesting application of stochastic Hydrogeology to a real case is the paper by Colombo et al. who demonstrate the applicability of backward tracking to identify pollutant sources in the metropolitan area of Milano.
There are two more papers with real case applications. The first one, by Hemmings et al. discusses the importance of early uncertainty quantification aimed to maximize the efficiency of modeling in the context of decision support. The authors discuss the importance of identifying whether the cost of expensive history matching is worth it given the available data. They propose a decision support modeling workflow and demonstrate it in the Wairarapa Valley, New Zealand. The second one, by Cromwell et al. presents a clever use of deep neural networks to estimate hydraulic conductivities in a catchment using integrated surface-subsurface modeling and demonstrates its application in Rock Creek, Colorado, United States.
The last application by Allgeier et al. addresses an optimization problem under uncertainty: the optimal selection of a monitoring network for the delineation of groundwater divides. The authors use the Preposterior Data Impact Assessor as their optimal experimental design method and demonstrate it to delineate the groundwater divide between the Ammer and Neckar river catchments in Germany.
There are three more papers that explore different facets of stochastic Hydrogeology in very different environments. Jeong et al. address the general problem of optimization under uncertainty and compare three different ensemble-based stochastic gradient methods for the optimal well placement for brine extraction in a synthetic carbon storage reservoir. In their conclusions, they provide recommendations on when and how to use each one of the three methods analyzed. Lam et al. discuss the application of an ensemble smoother for stochastic inverse modeling of groundwater flow parameters using transient hydraulic heads and flow rates as data. They limit their analysis to multi-Gaussian distributions for the flow parameters and use a synthetic case, which resembles the French Underground Research Laboratory site, to demonstrate the performance of the approach. Their final results are clearly influenced by the uneven coverage of the model domain by the observation locations. Finally, Williams et al. focus on a very specific problem related to the loss of resolution in the velocity field computed on an equivalent porous media derived from a fractured domain. The authors demonstrate the problem and propose a downscaling approach to recover the effects of subgrid heterogeneity in the context of radionuclide transport through fractured media. They demonstrate their approach on a synthetic brittle fault zone model.
The more than 13,000 views, in the first four months after the first paper was accepted, proves the interest of the scientific community in stochastic modeling in Hydrogeology. We hope that you enjoy reading this collection.
All authors listed have made a substantial, direct, and intellectual contribution to the work and approved it for publication.
AA was employed by HydroGeoModels AG.
The remaining authors declare that the research was conducted in the absence of any commercial or financial relationships that could be construed as a potential conflict of interest.
Keywords: heterogeneity, uncertainty, Groundwater, simulation, hydrology
Citation: Gómez-Hernández JJ, Li L, Xu T and Alcolea A (2021) Editorial: Stochastic Modeling in Hydrogeology. Front. Earth Sci. 9:698925. doi: 10.3389/feart.2021.698925
Received: 22 April 2021; Accepted: 03 May 2021;
Published: 17 May 2021.
Edited by and reviewed by:
Nick Van De Giesen, Delft University of Technology, NetherlandsCopyright © 2021 Gómez-Hernández, Li, Xu and Alcolea. This is an open-access article distributed under the terms of the Creative Commons Attribution License (CC BY). The use, distribution or reproduction in other forums is permitted, provided the original author(s) and the copyright owner(s) are credited and that the original publication in this journal is cited, in accordance with accepted academic practice. No use, distribution or reproduction is permitted which does not comply with these terms.
*Correspondence: J. Jaime Gómez-Hernández, amdvbWV6QHVwdi5lcw==
Disclaimer: All claims expressed in this article are solely those of the authors and do not necessarily represent those of their affiliated organizations, or those of the publisher, the editors and the reviewers. Any product that may be evaluated in this article or claim that may be made by its manufacturer is not guaranteed or endorsed by the publisher.
Research integrity at Frontiers
Learn more about the work of our research integrity team to safeguard the quality of each article we publish.