- 1Istituto Nazionale di Geofisica e Vulcanologia, Sezione di Bologna, Italy
- 2Istituto Nazionale di Geofisica e Vulcanologia, Osservatorio Nazionale Terremoti, Bologna, Italy
- 3Ministero dello Sviluppo Economico, Direzione Generale per le Infrastrutture e la Sicurezza dei Sistemi Energetici e Geominerari (DGISSEG), Rome, Italy
- 4Ricerca sul Sistema Energetico S.p.A (RSE), Milano, Italy
A number of oil- and gas-producing leases have been operating in Italy in the last decades, many of which are located in the surroundings of tectonically active regions. Identifying human-induced seismicity in areas with high levels of natural seismicity is a difficult task for which virtually any result can be a source of controversy. We implemented a large-scale analysis aiming at tracking significant departures of background seismicity from a stationary behavior around active oil and gas development leases in Italy. We analyzed seismicity rates before and after hydrocarbon peak production in six oil-producing and 43 gas-producing leases, and evaluate the significance of possible seismicity rate changes. In a considerable number of cases seismicity rate results stationary. None of the observed cases of seismicity rate increase after the peak production is statistically significant (at a s.l. = 0.05). Conversely, considering cases of seismicity rate decrease after peak production, our results suggest that the seismicity rate reduction is statistically significant (s.l. = 0.05) around one oil-producing lease (Val d’Agri, Basilicata) and around a cluster of gas-producing leases in Sicily. Our results put in evidence correlated changes between the rates of shallow seismicity and hydrocarbon production in these areas, which are then identified as hotspots requiring more detailed research; assessing actual causal relationships between these processes will require further physically-based modelling. If a physical causative link between these processes exists, then the observed seismicity rate reduction could either be due to increased seismicity during the progressive increase in production before reaching its maximum, or to an actual seismicity rate reduction after that peak. Considering that there is evidence of seismicity occurring before the start of hydrocarbon production, which contrasts with the evident reduction of events observed after the peak production, we think it likely that the seismicity inhibition is a plausible hypothesis. Using a simple model we also calculate Coulomb stress changes in planes optimally oriented for failure, and we show that under some conditions the inhibition of seismicity is feasible in at least one of these cases. We conclude that more efforts to study the mechanisms and the possible consequences of anthropogenically-driven seismicity inhibition are required.
1 Introduction
The complex geological setting that characterizes the Italian peninsula is the result of different geodynamical processes closely acting in time and space; consequently, today the crust in this zone is characterized by a complex stress field with tightly spaced compressional and extensional regions (Amato and Montone, 1997). The most predominant geomorphologic features in this region are the Southern Alps and the Apennines mountain chains, which are characterized by thrust-and-fold belts originated from the interaction between the European and the Adriatic-African tectonics plates (see e.g., Calamita et al., 1994; Cello and Mazzoli, 1998; D’Agostino et al., 2001; Bertello et al., 2010; Handy et al., 2010; Cazzini et al., 2015; van Hinsbergen et al., 2020). Such an active and complex tectonic setting makes of Italy a seismically active region where on average, every year, more than 2,000 seismic events with magnitude ≥2.0 are located by the Italian national seismic network (see e.g., the Bollettino Sismico Italiano, Pagliuca et al., 2020). Moreover, Italian seismic sequences are generally very complex, often characterised by the occurrence of either foreshocks, multiple mainshocks, or strong aftershocks; this feature of the seismicity in this region has a strongly influence on the seismic hazard (Guidoboni and Valensise, 2015).
In recent years, the interest in the possible influence of anthropogenic activities on seismicity has significantly grown mainly because of a generalized public concern, which has stimulated the development of independent scientific research to support objective policy making (e.g., Ellsworth, 2013; Dahm et al., 2015; van der Voort and Vanclay, 2015; Garcia–Aristizabal et al., 2020). The Italian territory is the scenario of a wide number of underground industrial activities such as oil and gas extraction, geothermal energy production, and gas storage, many of which have been suspected to have direct or indirect causal links with some seismic events located nearby; however, to date there are no unambiguously documented reports of damaging seismic events associated with anthropogenic activities in the country (see e.g., Braun et al., 2018). Probably, the only clear cases of seismicity linked to underground geo-resource development in Italy are the low-magnitude seismicity occurrences recorded in connection with wastewater reinjection at the Costa Molina 2 well in the High Val d’Agri, southern Italy (Valoroso et al., 2009; Improta et al., 2015), and the seismicity recorded near geothermal power plants in Tuscany (Evans et al., 2012).
Among all the anthropic activities having the potential to stimulate earthquakes to occur, the effects of fluid injection or extraction from the crust are probably the processes arising more concern, in particular the activities related with oil and gas production. In Italy, hydrocarbons are found in several oil and gas provinces, most of which are located in the Po plain, the northern Adriatic sea, the southern Apennines, and in Sicily (e.g., Bertello et al., 2010); as a consequence, these are the areas hosting most of the development leases in the country (Figure 1). According to data published by the Italian Ministry of Economic Development (MISE), as of 2019 there were 193 development leases in the country, 127 of which are onshore and the other 66 offshore (UNMIG, 2020).
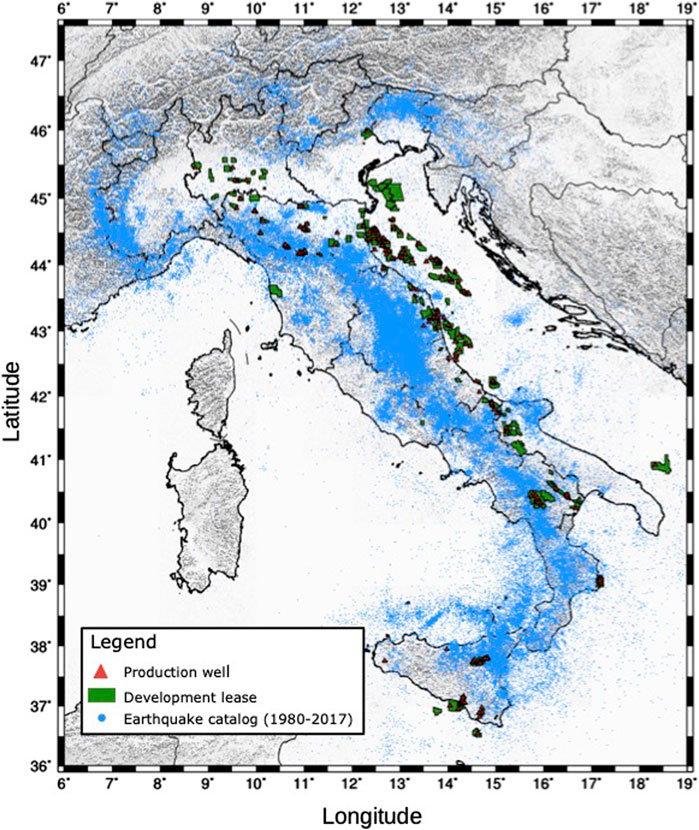
FIGURE 1. Map showing the spatial distribution of the oil and gas production leases in Italy, as well as the epicenters of the earthquakes from the HORUS catalog used in this study.
Discriminating natural from induced seismicity in seismically active regions is a particularly complex task. Early attempts to discriminate induced from natural seismicity were performed, for fluid injection operations, by Davis and Frohlich (1993), and for fluid withdrawal by Davis and Nyffenegger (1995); however, these approaches were mainly based on qualitative assessments.
More quantitative, physically-based and/or stochastic methods for discriminating natural from induced seismicity have recently been proposed in literature (a review can be found, e.g., in Grigoli et al., 2017). For example, Dahm et al. (2015) propose a quantitative probabilistic approach to discriminate induced, triggered, and natural earthquakes, calculating the probability that events have been anthropically triggered/induced from the modeling of Coulomb stress changes and a rate-and-state dependent seismicity model.
Schoenball et al. (2015) analyzed inter-event times, spatial distribution, and frequency-size distributions for natural and induced earthquakes around a geothermal field. Determining the distribution of nearest neighbor distances in a combined space-time-magnitude metric, they identify clear differences between both kinds of seismicity. For example, it is suggested that compared to natural earthquakes, induced earthquakes feature a larger population of background seismicity and nearest neighbors at large magnitude rescaled times and small magnitude rescaled distances. They argue that unlike tectonic processes, stress changes caused by anthropic underground operations occur on much smaller time scales and appear strong enough to drive small faults through several seismic cycles. As a result, it is likely to record seismicity close to previous hypocenters after short time periods.
Zhang et al. (2016) compared moment tensors of both natural and induced events in the Western Canadian Sedimentary Basin. These authors calculated full moment tensors and stress drop values for eight induced earthquakes (magnitudes between 3.2 and 4.4), as well as for a nearby M5.3 event considered as a natural earthquake. This study suggests that, first, it may be possible to discriminate between induced and natural seismicity considering region-specific attributes, as for example the focal depths (which they suggest as the most robust parameter since the induced events in their study area are significantly shallower than most of the intra-plate earthquakes in the Canadian Shield). Moreover, they found a non-negligible (>25%), non double couple component for most of the induced events studied.
Zaliapin and Ben–Zion (2016) analyzed statistical features of background and clustered subsets of earthquakes in California and in South Africa. These authors suggest that, compared to regular tectonic activity, induced seismicity in the analyzed data sets exhibit remarkable features as i) a higher rate of background events, ii) faster temporal offspring decay, iii) higher rate of repeating events (i.e., earthquakes located in the rupture area of some previous earthquakes, but that occur at times far exceeding the typical duration of an aftershock series), iv) larger proportion of small clusters, and v) larger spatial separation between parent and offspring.
Discriminating human-induced from natural seismicity is therefore a difficult problem for which virtually any solution can be a source of controversy. For this reason, the evaluation of possible interactions between seismicity and hydrocarbon production, if possible, should rely on multidisciplinary analyses such as e.g., detailed physically-based modelling complemented by sophisticated stochastic methods able to provide probabilistic assessments and to take uncertainties into account. It is worth noting however that the ways in which these interactions may occur are complex and their identification in a context characterized by high levels of naturally-occurring seismicity is not straightforward. Moreover, given the relatively high number of development leases active in Italy, performing such analyses at the national scale may be considered an intractable problem.
These reasons pushed us to explore the possibility to implement large-scale screening methods aiming at tracking measurable phenomena, such as e.g., changes in seismicity rates, that plausibly could occur if notable interactions between underground human operations and nearby seismicity sources are actually occurring in a given area. Spatial and temporal correlation between human activity and event rates is usually considered a key parameter to suspect possible relationships between seismicity and underground anthropic activity (e.g., Shapiro et al., 2007, 2010; Cesca et al., 2014; Leptokaropoulos et al., 2017; Garcia-Aristizabal, 2018; Molina et al., 2020); for example, significant changes in seismicity rates with respect to background seismicity, as well as the spatial and temporal correlation of gas injection operations and seismicity were analyzed by Cesca et al. (2014) to suggest a possible case of triggered/induced seismicity near an offshore platform used for gas storage in Spain (the Castor project).
However, underground human-induced perturbations (as e.g., pore pressure variations due to fluid injections) can produce changes at large distances and/or with large temporal delays, potentially causing earthquakes to occur several kilometers away as well as months/years after the industrial operations have stopped or reached the maximum peak (e.g., Mulargia and Bizzarri, 2014); likewise, natural seismicity may also occur within few kilometers from industrial sites. Therefore, in seismically active regions (such as in Italy), spatio-temporal correlations between industrial activity and significant changes in seismicity rates with respect to background activity by themselves usually do not provide irrefutable proofs of causal relationships between hydrocarbon production and seismic activity. Despite this, we argue that studying such correlations has a remarkable added value since it gives us the possibility of performing large-scale, systematic analyses of a huge amount of seismic and production data and, under the working hypotheses considered, to identify hotspot areas where it could be possible to perform, in a later stage, more detailed research to verify possible causal relationships.
The industrial data publicly available for this study consists of a time series of hydrocarbon production volumes; for this reason, our analyses are particularly focused on studying the possible effects on seismicity of stress perturbations caused by fluid withdrawal processes. The working hypothesis in this work therefore starts from assuming that fluid withdrawal from the crust may induce deformations in the surroundings of the host rock (especially in depleting reservoirs); the magnitude of such deformations will depend on multiple factors such as, for example, the litology, the structural geology, the volume and rate of fluid removed, the geomechanical features of the reservoir, and its behavior during the fluid withdrawal process, among others. The deformations may in turn alter the local stress field and, as a consequence, stimulate or inhibit seismicity in the surroundings.
We assume that in absence of other stress perturbation sources, the regional release of background seismicity is predominantly influenced by the regional tectonic stress field; in such a context, it can be expected that a steady stress field should tend to generate background seismicity with stationary rates; however, if other natural (e.g., hydrogeology: see Hainzl et al., 2006; Pintori et al., 2021) or man-made (e.g., pressurized fluid injections: see Shapiro et al., 2007; Garcia-Aristizabal, 2018) processes are able to perturb the local stress field, then it is possible that the rate at which seismicity is released in that specific area can be altered. In such a case, slight deviations from stationarity could possibly be measured.
In this work we are interested in identifying significant departures of background seismicity from a stationary behavior around productive oil and gas development leases in Italy. We are particularly interested in exploring cases in which the long-term hydrocarbon production may have left a measurable footprint on the release of background seismicity (as e.g., by processes related to reservoir depletion), and to test whether possible changes in the rate at which background seismicity is released in such areas are correlated with the main changes in the hydrocarbon production patterns.
The article is structured as follows: first we present the seismic and the hydrocarbon production data available for this study. Second, we present the methodological approach used in order to identify zones with possible anomalies in seismicity rates concomitant with significant changes in oil and gas production. Finally, we present the results and discuss the importance and limitations of these findings.
2 Data
For this study we use a national-wide seismic catalog containing earthquake locations and magnitudes, as well as the most detailed public oil and gas production data from development leases in Italy. All the used data are freely accessible from public sources (Section 7 for details).
2.1 Seismicity
We use the seismic data collected in the publicly-available HORUS catalog (Homogenized instrumental seismic catalog, Lolli et al., 2020a, b). This is an extended instrumental seismic catalog reporting earthquake locations and magnitudes since 1960 and is continuously updated as new data is processed. An outstanding feature of this catalog is the effort made to harmonize the event magnitudes in terms of an equivalent homogeneous moment magnitude, Mw.
We use the data in the time interval 1980–2017, which includes 368,258 earthquakes (Figure 1). This time interval is selected because it covers the same time window of available hydrocarbon production data; moreover, the eighties are probably the period when the national seismic network started to grow more consistently, improving as a consequence the quality of the earthquake locations and reducing the completeness magnitude (Mc). However, this process does not evolve uniformly throughout the whole country, since the spatial and temporal distribution of new seismic stations is not spatially homogeneous. For example, Figure 2 shows the temporal evolution of the Italian National Seismic Network; the stations belong to different networks deployed for seismic monitoring in Italy (e.g., the Italian Seismic Network and the Euro-Mediterranean Network, both maintained by INGV; the Italian Strong Motion Network, managed by the National Civil Protection; the networks operated by other national institutes and universities such as the Istituto Nazionale di Oceanografia e di Geofisica Sperimentale, the University of Basilicata, the University of Genoa, the University of Trieste, the University of Bari, and other organisations in the border areas).
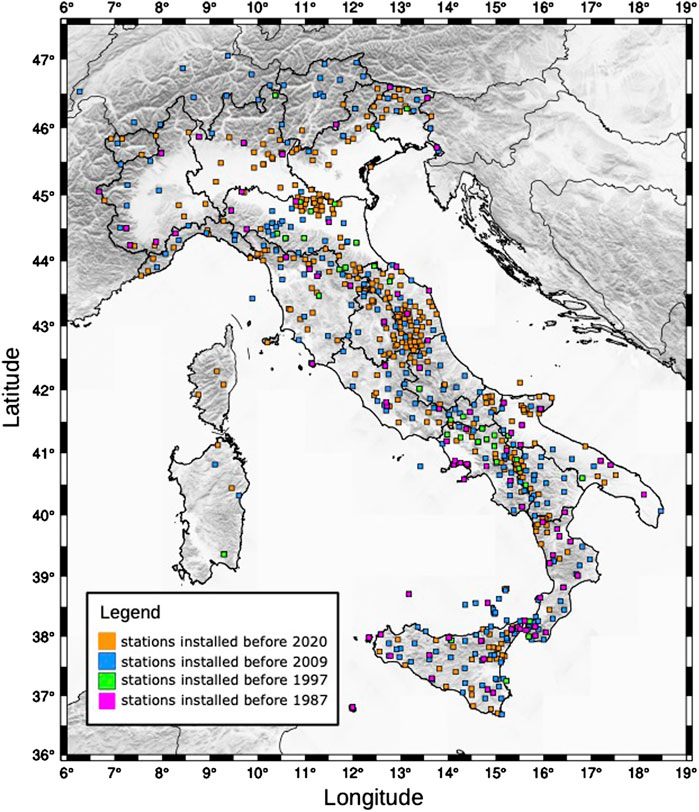
FIGURE 2. Temporal evolution of the deployment of monitoring seismic stations in Italy, maintained by various Italian and international institutes and universities (see the text for details). Substantial increases in the number of stations concurred with the occurrence of different seismic sequences.
The completeness magnitude (Mc) of this catalog therefore changes in time and in space, as a consequence of different factors as e.g., changes in the number and distribution of seismic stations available for locating events, as well as the quality of the instrumentation and of the site facilities. Therefore, identifying a single completeness magnitude (in both time and space) for the full catalog results in a very high Mc value; adopting such a choice would force us to discard an important amount of seismic data, and for this reason, the Mc is rather determined locally for each site, as discussed in the Section 3.
2.2 Oil and Gas Production Data
We collected the most detailed public information about hydrocarbon production in Italy (available from the Italian Ministry of Economic Development, MISE, Section 7 for details). Out of 109 active development permits (Figure 1), we got production data from 102 leases in which a total of 588 production wells are present. The available data set includes the annual oil and gas production since 1980 (volumes in thousands of cubic meters of oil or standard cubic meters of gas); the data is aggregated by development lease, that is, summing up the production/year from all the wells producing within the lease. For development leases in which production started before 1980, the total oil and gas production preceding 1980 is also available.
It is worth noting that besides the hydrocarbon production, a number of sites where underground waste water injection is performed are also present in Italy; nevertheless, the fluid injection-related data are not publicly available and for this reason these activities were not considered in this study.
As Figure 1 shows, the active development leases are mostly distributed along the Adriatic coast (onshore and offshore), as well as along the Po Valley (in the North), the central/southern Apennines and Sicily. In this study we consider the hydrocarbon production from 1980 up to 2017 (Figure 3). From the 102 development leases for which production data is available, 85 exclusively produce gas, one exclusively produces oil, and 16 produce both oil and gas. The development leases with the highest annual oil production in the study period are found in the Val d’Agri (Basilicata region), Villafortuna-Trecate (Piemonte region, western Po Valley), B.C 8. LF (offshore, southern Adriatic sea), and C.C 6. EO (Sicily, southern Italy) leases (Figure 3A); on the other hand, those with the highest annual gas productions in the same period are the A.C 7. AS (offshore, central Adriatic sea), D. C 1. AG (Calabria region), A.C 2. AS and A.C. 27. EA (offshore, northern Adriatic sea, Figure 3B).
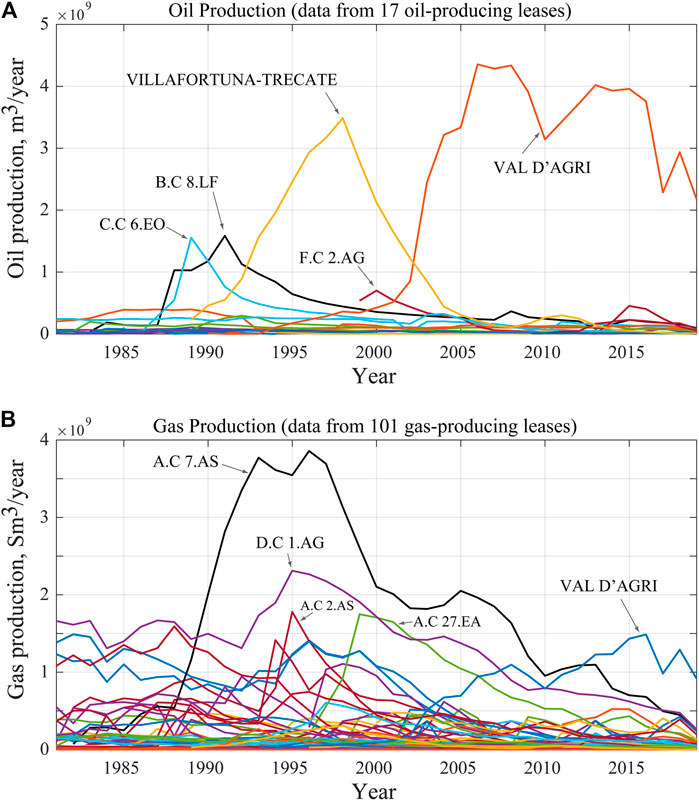
FIGURE 3. Annual oil and gas produced between 1980 and 2017 in Italy from 102 development leases in Italy: (A) Oil is produced from 17 leases (one of which exclusively produces oil); (B) Gas is produced from 101 leases (85 of which exclusively produce gas). Data available from the Italian Ministry of Economic Development (MISE).
3 Methods
We perform a large-scale screening of the behavior of background seismicity (i.e., the events considered independent, as described in Section 3.3) in areas around oil and gas production sites in Italy. Our goal is to attempt i) to identify correlated changes between seismicity rates and hydrocarbon production, and ii) to test the significance of these seismicity rate changes.
First, a pre-processing step is performed in order to identify the areas around the target leases where a reasonable amount of both seismic and production data is available. Afterwards, we proceed with the proper data analyses, namely i) identification of independent background seismicity in the lease area, ii) selection of background events located within a distance δx from the production wells, and iii) test the significance of possible seismicity rate changes correlated with changes in hydrocarbon production. These steps are explained in the following paragraphs.
3.1 Identifying Development Leases for the Analyses
If the withdrawal of fluids from the crust affects the stress field and the seismicity release in a given zone, then a possible way to identify potential traces of such effects is to look for changes in background seismicity rates before and after important changes in the rate at which fluids are extracted. Observing the time series of production data (Figure 3), it can be seen that in most of the sites, the oil production (Figure 3A) and the gas production (Figure 3B) have some outstanding features: after the production starts, it follows different paths up to a point in which it reaches maximum production. The time at which oil or gas production reaches its maximum is hereinafter called the maximum production time, tm. Afterwards, for times t > tm, the production tends to decrease (probably related to field depletion processes).
Looking in detail at the development of the time series of production data, the main change points in oil and gas production data of potential interest for the analyses in this article are the start of production and the time at which production reaches its maximum. We consider the peak of maximum hydrocarbon production as the reference point for exploring possible changes in seismicity rates (before and after the peak). Changes before and after the production start cannot be analyzed because in most of the sites the time at which production initiates precede the start of the seismic data catalog.
An essential requirement to study changes in seismicity rates consists of taking a sufficiently long time window of seismic data in the time periods preceding and following the change point in production data considered for comparing seismicity rates. In general, the longer the time window, the more representative will our data set likely be. Taking into account the time window of data available for this study (1980–2017), as well as the average seismicity rates observed around the active development leases, we consider as a requirement for including a given development lease in this analysis the availability of a complete set of seismic data for at least 10 years before and 10 years after the tm associated with the peak production in the respective development lease.
Figure 4 shows histograms summarizing the tm at which maximum oil (Figure 4A) and gas (Figure 4B) production are reached in all the development leases for which data are available. Adopting the minimum 10-years time window before and after tm, and also considering the temporal completeness identified for the seismicity around the leases (Section 3.2 for details), the sites that can be reliably analyzed in this work reduce to those for which the peak production in the available time series is reached between 1995 and 2008 (i.e., 1995 ≤ tm ≤ 2008). In this way, six oil-producing leases and 43 gas-producing comply with these conditions and are selected for further analyses.
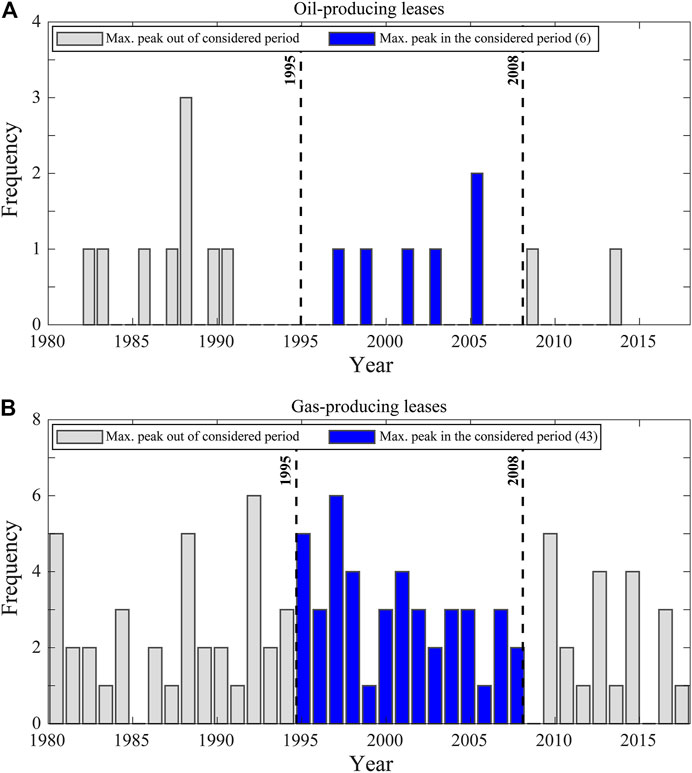
FIGURE 4. Year at which the maximum production is reached in the available data at each development lease (tm). Histograms shows the number of (A) oil- and (B) gas-producing leases in which tm is reached in the respective year; the blue bars identify the period selected for the analyses (1995–2008) according to the described criteria.
The time series of oil and gas production data from the selected development leases are shown, respectively, in Figures 5A,B. Taking the peak oil/gas production as a reference, the data follow different patterns that can be better observed normalizing the production by the maximum production reached in each lease and realigning the time series with respect to tm (Figures 5C,D). For the sake of simplicity, we identify three main patterns (Figure 5E): pattern I (the most frequent), is when the production rate steadily increases with time up to reach its maximum; afterwards the production progressively reduces with time. Patterns II and III instead refer to cases where production increases up to remain at relatively high levels for a while, before significantly decreasing again. In some cases the peak production is reached at the end (pattern II), and in other cases it is reached at the beginning (pattern III) of the high-production period.
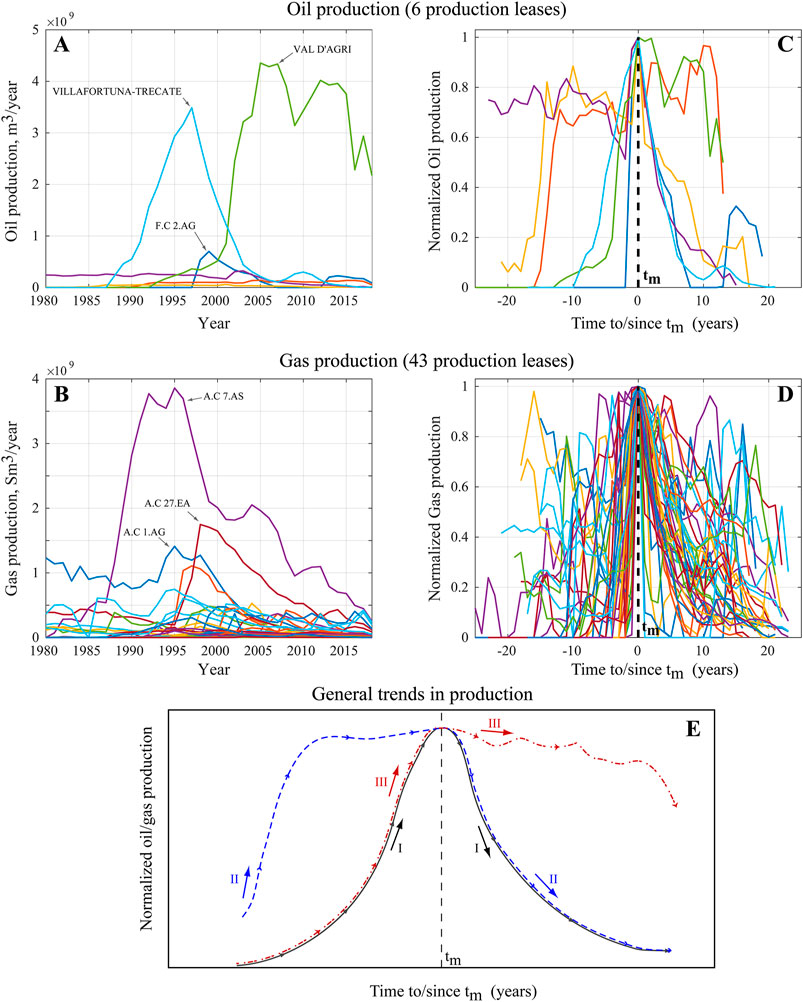
FIGURE 5. Annual production of identified sites in which it is possible to perform the proposed analyses. Annual production of (A) oil and (B) gas in the selected development leases. Production data normalized by the maximum value reached in each time series and realigned respect to peak time, tm are shown in plots (C) (oil) and (D) (gas). (E) Main temporal trends observed in production data.
3.2 Seismic Data Selection
We first calculate the epicentral distance between each earthquake in the catalog and the nearest production well in a lease. This information is used to select the whole seismicity located around each lease, paying particular attention to include all possible clusters of events in time and space observed in the surroundings (i.e., to avoid including incomplete data of seismic sequences, for example). This generally means including seismicity located within a few hundred kilometers around each lease (Figure 6A). Moreover, since it is generally observed that events induced by man-made, underground operations tend to be shallower than most natural, tectonically driven events, and that induced events often occur at depths comparable to the depth of wells (see e.g., Zhang et al., 2016; Foulger et al., 2018), then for this analysis we only consider the events with depth shallower than 15 km.
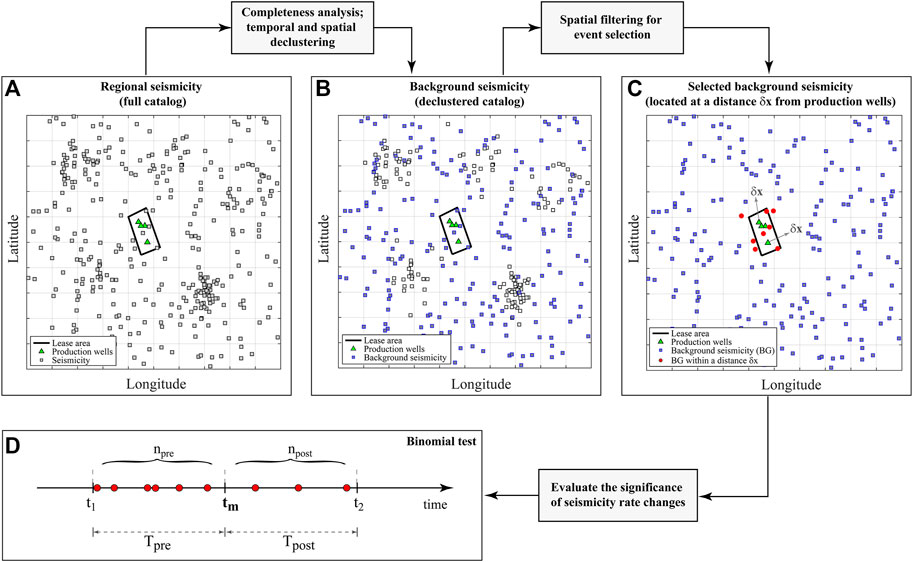
FIGURE 6. Summary of the processing for selecting seismic data around a given production lease for the correlation analysis: (A) Regional seismicity around the lease, for which the
Finally, for each resulting (local) catalog we calculate the completeness magnitude (Mc) using, comparatively, three methods [the Maximum Curvature, the Goodness of Fit (Wiemer and Wyss, 2000), and the Modified Goodness of Fit (Leptokaropoulos et al., 2013)], that are available as open tools in the EPOS (European Plate Observing System) platform for anthropogenic hazards (IS-EPOS, 2016; Orlecka-Sikora et al., 2020). In this way, for each lease we obtain a seismic catalog covering a given time interval and is complete above a given minimum magnitude Mc (Figures 6A,B).
3.3 Declustering the Seismic Catalog
Since our main target is the identification of depletion-induced effects on seismicity, to perform the analyses proposed in this work we suggest to use a seismic catalog composed as much as possible by independent events, that is, events not likely triggered by earthquake interaction processes (as e.g., aftershocks). That set of independent events is hereinafter referred to as background seismicity (see e.g., Figure 6B).
The seismic declustering is a crucial issue in statistical seismology. In general, the term “background” (or “independent”) events refers to events that are typically related to regional tectonic activity. Triggered events occur in space-time clusters, and are associated with the occurrence of previous events (e.g., stimulated by stress perturbations from previous-earthquakes); they are often referred to as “dependent events.” The distinction within a catalog between the contribution of independent and dependent earthquakes is very complex, and each method inherently contains subjectivity (Zhuang et al., 2002). This characteristic implies that applying different declustering models to the same catalog may generate catalogs of independent events that may differ. Moreover, uncertainties on the data (as e.g., earthquake locations and magnitudes) may challenge the proper performance of declustering algorithms.
In seismology, several techniques have been developed to address the problem of declustering; van Stiphout et al. (2012) provides an overview of this issue, describing the pros and cons of the most popular algorithms. In this work we use the Nearest-Neighbour (NN) Clustering Analysis technique, developed by Zaliapin and Ben-Zion (2013). An outstanding advantage of the NN algorithm is its simplicity because the link between the background events with those triggered is a metric that only concerns a distance measure in time, space and magnitude between any pair of events. The technique consists of calculating, for each earthquake ith in the catalog, the distance to any other jth earthquake subsequently occurred as:
selecting, for each earthquake i, the smallest ηij. In Equation 1, the tij = tj − ti is the difference between the two occurrence times expressed in years (with tij > 0); rij is the distance between the two hypocenters in km; df is the fractal dimension of the distribution of hypocenters, which in this work was set to 1.2 following the values suggested by Kagan (1991) and used for declustering seismicity in Italy by Stallone and Marzocchi (2019); b is the Gutenberg-Richter b-value, which is calculated for the seismicity around each lease using the method described in Marzocchi and Sandri (2003); and mi is the magnitude of the i earthquake.
Within a complete seismic catalog, the distribution of these 3-dimensional distances always shows a bi-modal pattern: the first group of earthquakes is characterized by unusually small distances and represents earthquakes that are “clustered,” whereas the second group identifies the events interpreted as “background earthquakes,” since in the considered parameter space they exhibit greater distances from each other.
3.4 Evaluating the Significance of Seismicity Rate Changes
Once the background seismicity around a given lease is obtained, we select a set of seismic events for studying possible changes in seismicity rate correlated with changes in hydrocarbon production. With this aim, we set a maximum distance from the producing wells, δx, to define the volume enclosing the events to be included in the analysis (Figure 6C). Defining δx is critical since it reflects the spatial extent where the potential deformations and stress perturbations are supposed to alter the natural occurrence of seismicity. In principle it should be carefully evaluated case by case accounting for different local factors such as, e.g., the size and depth of the reservoir, the volume of fluids withdrawn from the crust, etc. If δx is small, there is a risk of including a small, non representative sample of events; on the other hand, if δx is very large, the significance of possible local changes in seismicity rates can be hidden by a large sample of regional (and presumably stationary) background seismicity.
In tectonic earthquake interaction studies, the size of the area at which earthquake triggering is mostly expected to occur is usually mapped by static stress perturbations; if L is the mainshock source length (as derived from scaling relations, such as for example Wells and Coppersmith, 1994), a characteristic distance in the range 1–3 L is often suggested as a plausible distance within which triggered aftershock are expected to occur (e.g., Parsons and Velasco, 2009; Tahir et al., 2012). Some authors have suggested similar scaling properties for determining characteristic distances for seismicity induced by reservoir impoundments (Grasso et al., 2019) and gas reservoirs (Grasso et al., 2021); in such cases, the characteristic distance is determined as a function of the size of the reservoir. We do not have information about the dimensions of the oil and gas reservoirs from where hydrocarbons are produced in the analyzed cases; therefore, heuristically we select events located at distances within δx = 5 and 10 km from the production wells, which seems a reasonable and conservative choice for this study.
We look for significant seismicity rate changes before and after tm using the binomial test proposed by Leptokaropoulos et al. (2017). Let Tpre = [t1, tm] be the time interval identified before the maximum production peak (with tm − t1 ≥ 10 years, as defined before), and Tpost = [tm, t2] the time interval identified after the maximum production peak (with t2 − tm ≥ 10 years as well, see Figure 6D for reference). Let npre be the number of events that occurred in the period Tpre, and npost the number of events that occurred in the period Tpost. The total number of events in both periods is therefore N = npre + npost (Figure 6D). If the seismicity rate exhibit changes that are correlated with changes in the production trend before and after the time at which the maximum production is reached (tm), that is, if the seismicity rate during Tpre is significantly different from the seismicity rate during Tpost, then the actual division of the total number of events N in both periods into npre and npost should be significantly different from the division which could be attained at random. Therefore, if we hypothesize stationary seismicity, the proposed null hypothesis, H0, states that:
H0 : npostcan be obtained at random fromNunder probabilityP, where P is related to the time partitions as follows:
This hypothesis is tested by means of the binomial test (e.g., Wonnacott and Wonnacott, 1977). If N events occur randomly in the interval [t1, t2], this test provides i) the probability p1 that the number of events in the interval [tm, t2] is less than or equal to npost,
or ii) the probability p2 that the number of events in the interval [tm, t2] is greater than or equal to npost,
The binomial test assumes that each event is independent, with equal probability of occurrence in the interval [t1, t2]; the null hypothesis (H0) is evaluated at a given significance level (e.g., s.l. = 0.05), so that if p1 (or p2) <s.l., we conclude that there is evidence, with a given significance s.l., that rate in the interval [tm, t2] decreased (or increased) with respect to the rate in the interval [t1, tm].
4 Results
One of the main issues faced to perform the data analysis was to concentrate our efforts in areas where both seismic and production data were sufficiently representative to avoid, as much as possible, data-driven biases in our results. For this reason, and given the time span covered by the seismic and production data available for this study, we avoided analyzing any region in which the minimum data requirements defined in the Methods section were not accomplished. In particular, the minimum length of the time window of seismic data before and after tm, the time at which the maximum production is reached, becomes one of the main constraints, forcing us to not considering about 56% of the leases for which production data are actually available (that is, 57 out of the 102 leases). In such cases, the peak production occurred too close to the end or the beginning of the seismic catalog, which prevents an accurate estimation of the pre– and post– tm seismicity rate. On the remaining 45 leases (39 produce gas only, two produce oil only, and four produce both gas and oil), we applied the analyses described in Section 3. The spatial distribution of the analyzed leases cover different areas of the country, including the western Po plain, the northern and central Adriatic sea, southern Apennines and Sicily.
The completeness magnitudes and b values determined for the regional seismicity around each lease are summarized in Table 1 (for the oil-producing leases) and Table 2 (for the gas-producing leases). It is worth noting that, in general, the completeness magnitudes tend to be relatively high due to our interest in having seismic data sets as long as possible in time; in fact, the Mc has been selected for the seismicity around each lease so that completeness is ensured, at least, since 1985. The seismic catalogs, composed of nearby regional events with magnitudes above the Mc specifically determined for each study area, are then declustered using the NN clustering analysis technique (Section 3.3) to obtain a sample of background seismicity composed of independent events at regional scale.
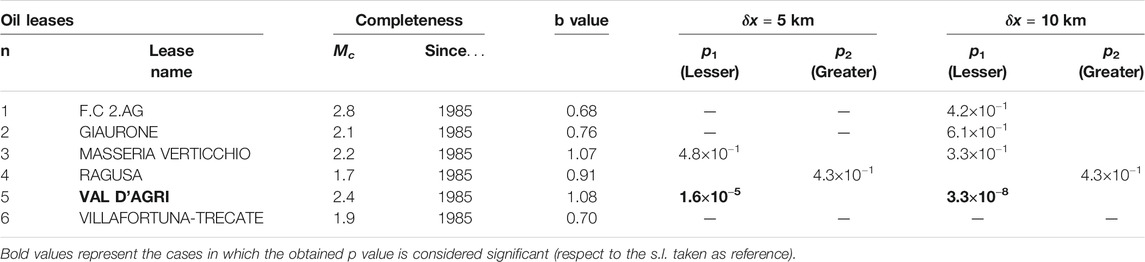
TABLE 1. Oil-producing leases analyzed in this study. For each lease we present the estimated completeness magnitude, the b value, as well as the p values (p1 and p2) of the binomial test performed considering the seismicity located within a distance δx = 5 and δx = 10 km (the symbol—indicates cases in which both the seismicity rate in both the pre- and the post- tm time windows are zero).
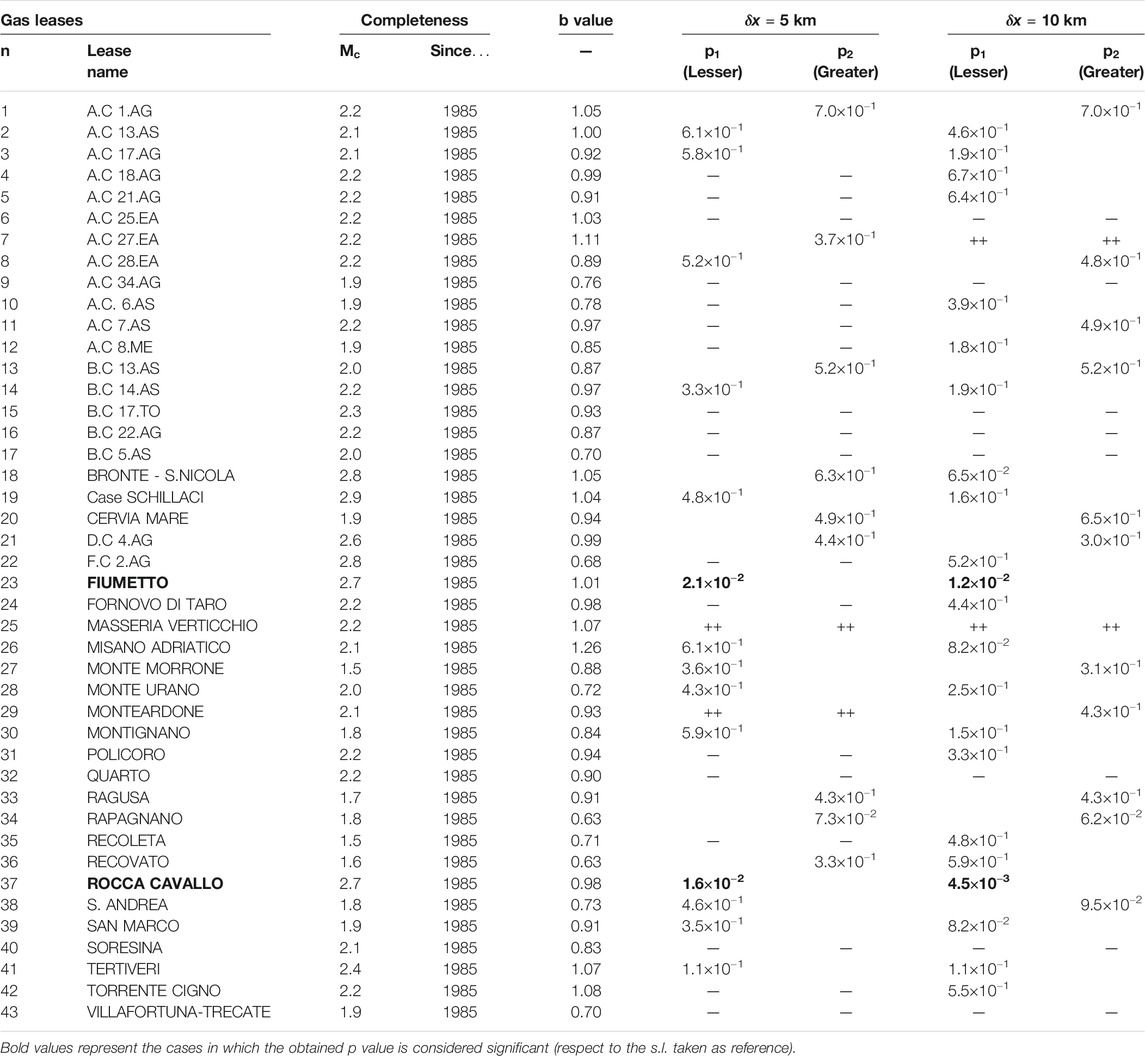
TABLE 2. Gas-producing leases analyzed in this study. For each lease we present the estimated completeness magnitude, the b value, as well as the p values (p1 and p2) of the binomial test performed considering the seismicity located within a distance δx = 5 and δx = 10 km (the symbol ++ indicate cases with equal seismicity rates in both the pre- and the post-tm time windows, whereas the symbol—indicates cases in which both rates are zero).
It is worth noting that the declustering step deserves some specific considerations. We argue that in the context of this work, declustering is an important step given our particular interest in detecting seismicity rate changes induced by eventual stress changes caused by depleting reservoirs located in areas where regional stresses are assumed to generate stationary background seismicity. Therefore, the proposed approach can hardly be applied to cases where induced seismicity tends to be strongly clusterized in time and space, as for example cases of seismicity induced by pressurized fluid injections. Moreover, uncertainties related to the effects of declustering could overshadow the reliability of the seismicity rate variations identified with the proposed approach; to mitigate this risk, we also discuss the results that can be obtained by analyzing the full complete catalog (that is, considering events above Mc without declustering); such results, along with the data sets (both the full and the declustered catalogs) are available in the Supplementary Material.
After declustering, we identify background events located within δx = 5 and δx = 10 km from the production wells in a given lease, and select the events before and after the respective tm. In this way we calculate the number of events before (npre) and after (npost) the peak production time, tm, as well as the respective time window lengths Tpre and Tpost (see e.g., Figure 6D for reference). The same procedure is performed using the full complete catalog (Supplementary Material) to compare the results obtained with and without the declustering processing.
Using these data, we first calculate the seismicity rate (in terms of average number of events/year) observed before and after tm for events located within a distance δx from the producing wells. Comparing the seismicity rates before and after the peak production, it is possible to highlight the areas with seismicity rate variations. For example, Figure 7A and Figure 8A show the location and estimate of seismicity rate variations around, respectively, the six oil-producing and 43 gas-producing leases analyzed in this study when considering seismicity within δx = 5 km from production wells; colors indicate the behavior of the seismicity rate (i.e., increase, decrease, unchanged) before and after tm. Regarding the oil-producing leases, the seismicity rate around three sites results null and unchanged before and after tm (green squares in Figure 7A; names can be seen in Table 1), in 2 cases (Masseria Verticchio and Val d’Agri) the seismicity rate before the peak production results higher than the seismicity rate in the time window after tm (blue squares in Figure 7A), whereas in one case (Ragusa) we observe the opposite situation (that is, the seismicity rate before is lower than the seismicity rate after tm, red squares in Figure 7A). Regarding the gas-producing leases, we observe that in 20 sites the seismicity rate does not change (18 of which have zero events before and after tm). These sites are represented as green squares in Figure 8A. In the remaining 23 leases (names can be seen in Table 2), a seismicity rate variation has been detected: nine sites exhibit an increase (red squares in Figure 8A), whereas 14 sites exhibit a decrease in the rate (blue squares in Figure 8A). When considering δx = 10 km, one oil-producing and 10 gas-producing leases do not exhibit seismicity rate changes, in 4 and 22 leases (oil and gas, respectively) the seismicity rate before tm results higher than the seismicity rate after the peak production, whereas the opposite behavior is observed in one oil- and 11 gas-producing leases.
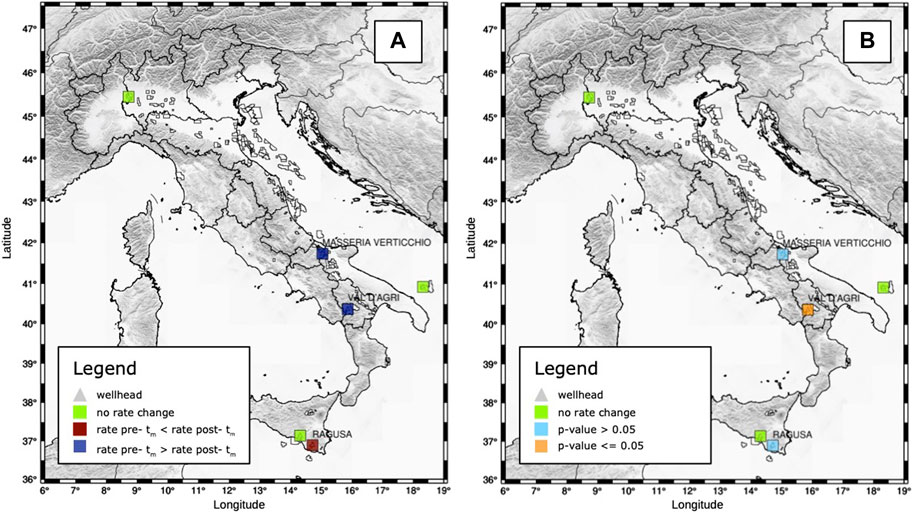
FIGURE 7. Map of the pre– and post– tm seismicity rate changes (A) and p values (B) for the six oil-producing leases analyzed in this work considering the seismicity located within δx = 5 km from producing wells.
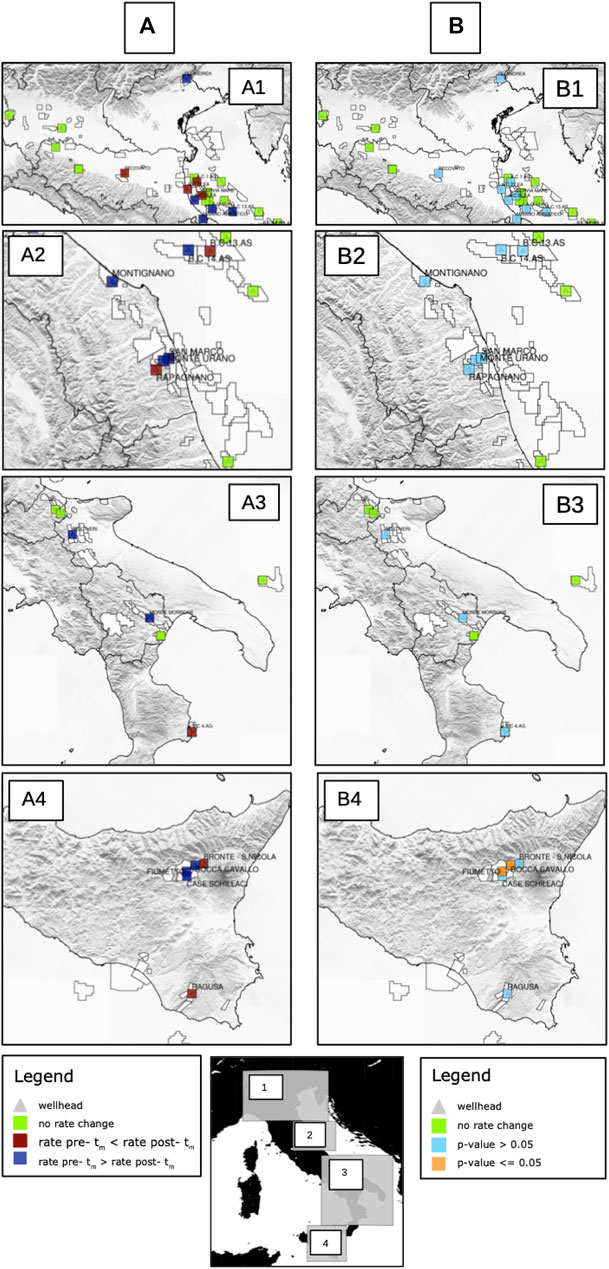
FIGURE 8. Map of the pre– and post–tm seismicity rate changes (A) and p values (B) for the 43 gas-producing leases analyzed in this work considering the seismicity located with-in δx = 5 km from producing wells.
We then evaluate the significance of these seismicity rate variations using the binomial test described in Section 3.4. For the areas exhibiting a reduction in seismicity rate after tm, we calculate p1 (Eq. 3) to evaluate the significance of the observed seismicity rate reduction in the time interval Tpost = [tm, t2] with respect to the rate observed in the interval Tpre = [t1, tm]. Likewise, for the areas exhibiting an increase in seismicity rate after tm we calculate p2 (Eq. 4) to evaluate the significance of the observed increase in the time interval Tpost with respect to the rate observed in the interval Tpre. The results of these calculations, considering seismicity located within both
The resulting probability values (p1 and p2) provide an indication about how likely it is to observe the number of events npost under the null hypothesis (which basically reflects what would be expected in case of stationary seismicity in the whole interval [t1, t2], see Figure 6D for reference). Therefore, the lower the p value, the more unlikely is to observe npost under such hypothesis (and therefore the more evidence in favor of a significant seismicity rate change). Considering for example a significance level s.l. = 0.05 we find that, on the one hand, none of the cases in which an increase in seismicity rate was observed after tm is statistically significant (considering seismicity located within both 5 and 10 km from production wells). On the other hand, regarding the cases exhibiting a seismicity rate decrease after tm, our results suggest that the observed seismicity rate change is statistically significant for one oil-producing lease (the Val d’Agri) and two gas-producing leases (Fiumetto and Rocca Cavallo). The location of all the analyzed leases, classified by the calculated p values considering as reference a s.l. = 0.05, are shown in Figure 7B for the oil-producing and Figure 8B for the gas-producing leases. The Val d’Agri lease is located in the Basilicata region, in the Southern Appenines, whereas Fiumetto and Rocca Cavallo leases are located in Sicily, near Etna Volcano.
The leases for which the seismicity rate change is considered statistically significant are identified using events within both δx = 5 and δx = 10 km (see Tables 1 and 2). It is worth to note that in most of the cases the rate change (i.e., increase or decrease) is coherent for seismicity selected considering both δx values. However, in a few cases of data selected around some gas-producing leases (5 sites out of 43, namely A.C 28. EA, Bronte-S. Nicola, Monte Morrone, Recovato, and S. Andrea), there is a change in the observed trend of seismicity rate variation (for example, a decrease observed for the seismicity located within 5 km contrasts with an increase observed when selecting events within a 10 km distance); discrepancies in such areas are due to instabilities mainly caused by either low seismic activity (such as, e.g., in the Po plain or offshore in the Adriatic sea) or sites close to seismically active sources (such as, e.g., very active tectonic or volcanic areas). It is worth noting however that in all these cases, as well as for cases in which no events are identified within the first 5 km, the observed rate changes are in any case not statistically significant.
In order to compare these results with the results that would be obtained if we do not use the background events only, the same analyses were performed using the full, complete catalog (i.e., considering events above Mc). It is worth noting that using the full catalog may somehow violate the methodological assumption considered for the statistical test (that is, assuming that in the absence of external stress perturbations, seismicity is expected to be stationary). Nevertheless, in this case such comparison is useful to demonstrate that the significant seismicity rate changes found in this work are not artificially created by the declustering procedure. These results are reported in Supplementary Tables S1 and S2 and in Supplementary Figures S1 and S2 in the Supplementary Material. When using the full catalog, we still find evidence of significant seismicity rate changes in the same three leases described in the previous paragraph (i.e., Val d’Agri, Fiumetto, and Rocca Cavallo). Nevertheless, it is worth observing that using the full catalog a few additional zones show some evidence of significant rate changes, especially when considering seismicity located at distances within 10 km from production wells; we consider however that such results are unreliable (for example, in the same area the rate may change e.g., from rate increase to rate decrease– when considering events at different distances, see for instance Tables S1 and S2 in the Supplementary Material); such unstable results are most probably due to the presence of clusters of aftershock events randomly located around some leases.
5 Discussion
In this work we monitor significant departures of background seismicity from a stationary behavior around active oil and gas development leases in Italy. We collected oil and gas production data from 102 leases in the period 1980–2017, and we used the seismic data from the HORUS catalog (Lolli et al., 2020b). After a close and conservative inspection of the available data, it has been possible to implement the proposed analyses in six oil-producing and 43 gas-producing leases in the country (including about 44% of the leases from which production data was available). We identify statistically significant seismicity rate changes (considering a s.l. = 0.05) concomitant with outstanding changes in hydrocarbon production (i.e., before and after peak production) in one oil-producing lease, Val d’Agri (Figure 7B), and two gas-producing leases, Fiumetto and Rocca Cavallo (Figure 8B). In all these cases, the seismicity rate after the peak production results significantly lower with respect to the seismicity rate observed before it.
It is worth to remind that spatial and temporal correlations between background seismicity rates and industrial activity, as the one highlighted in these areas, do not constitute an absolute proof to establish a causal relationship between hydrocarbon production and seismic activity. Therefore, we stress that the main value of this finding is to highlight these areas as hotspot zones deserving further detailed analyses to verify (or discard) possible causal relationships. If the oil or gas production in the Val d’Agri, Fiumetto and Rocca Cavallo leases played a role to alter shallow earthquake occurrences in these areas, then more sophisticated, physically-based studies are required to understand if the observed changes in seismicity rates are actually an observable consequence of physical mechanisms able to generate such changes. However, such analyses require access to detailed, geological, structural and geomechanical information.
Val d’Agri, located in the Basilicata region, is a particularly interesting case (Figure 9). This area hosts the largest onshore oil and gas field in Europe, where possibly-induced seismicity has been detected in connection with wastewater reinjection at the Costa Molina 2 well (e.g., Stabile et al., 2014b; Improta et al., 2015; Buttinelli et al., 2016; Improta et al., 2017). Moreover, some authors reported clustered seismicity located to the south of the nearby Pertusillo artificial water reservoir, whose origin has been suggested to be induced by rapid water level changes of the Pertusillo impoundment (Valoroso et al., 2009, 2011; Stabile et al., 2014a).
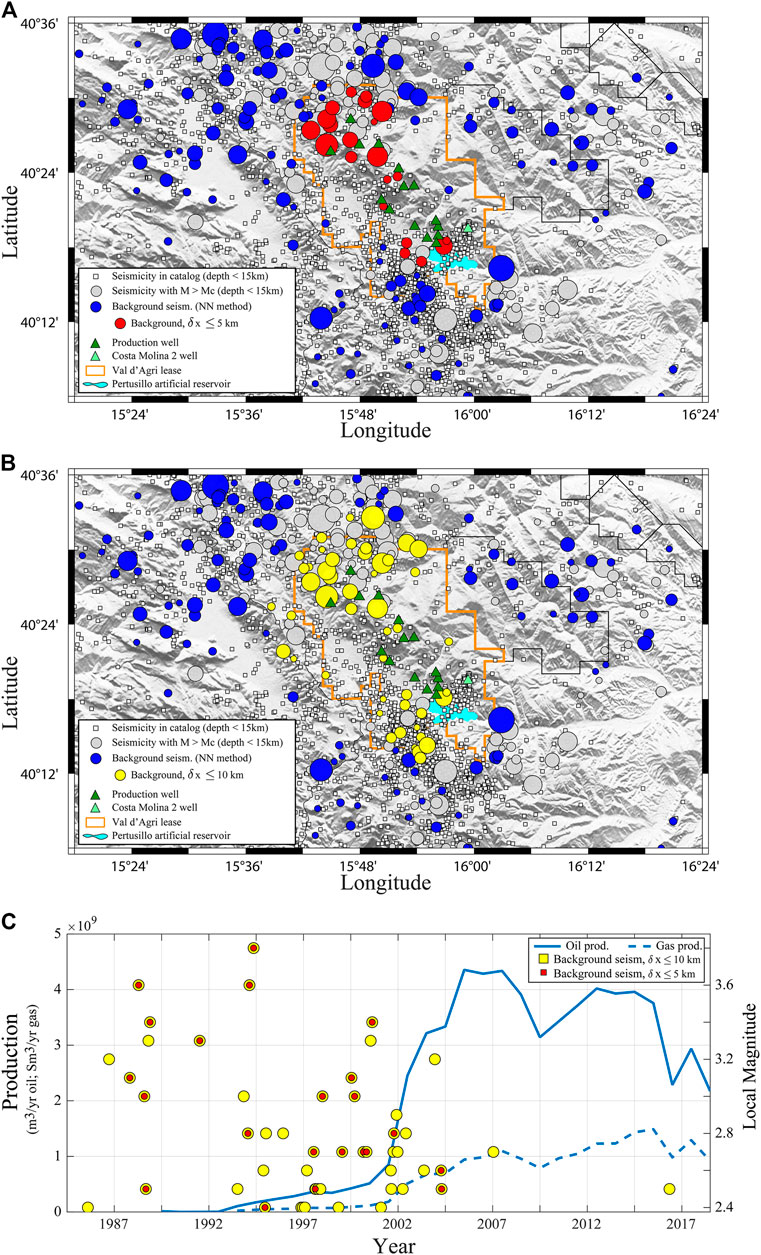
FIGURE 9. Seismic and production data used in the analysis for the Val d’Agri lease. Panels (A,B) show the event locations: squares represent the full catalog, and circles represent events above Mc; earthquakes identified as background seismicity are presented as colored circles, highlighting in particular the events located at distances δx = 5 (panel (A), red circles) and 10 km (panel (B), yellow circles) from the production wells. (C) Time series of production data and plot of the temporal occurrence and magnitude of seismic events
Figure 9 shows the location of the Val d’Agri lease, the production wells, as well as the regional seismicity in the area (gray squares), the events with magnitude above Mc (all circles), the background seismicity identified using the NN clustering analysis technique (colored circles), and the selected background seismicity located within a distance δx = 5 km (Figure 9A, red circles) and δx = 10 km (Figure 9B, yellow circles) from production wells. Only a few selected events are located in the southern part of the lease, close to the cluster of seismicity located south of the Pertusillo lake. Figure 9C shows the time series of annual oil and gas production from the Val d’Agri, and the plot of event times and magnitudes of selected seismicity. What we actually observe is a clear reduction in the number of shallow (z ≤ 15 km) background seismic events just after the oil production in Val d’Agri reached its maximum in 2005.
A similar significant change in the seismicity rate has been detected in the surroundings of Fiumetto and Rocca Cavallo leases, which are located in Sicily, near Etna Volcano (Figure 10). These two leases are located in an area where other five gas-producing leases operate (namely Bronte-S. Nicola, Case Schillaci, Gagliano A, Gagliano B, and Samperi), forming a “cluster” of production leases distributed in a relatively small area (about 400 km2, see e.g., Figure 10A). The results of the correlation analysis for Bronte-S. Nicola and Case Schillaci are also reported in Table 2 and Figure 8, whereas the last three leases were not included in this study because they did not meet the data requirements defined in Section 3.1. For completeness, we also reproduced the study using the full catalog of events (that is, without removing the most likely aftershock sequences identified using a declustering approach). Such a test allowed us to demonstrate that the observed reduction in seismicity rate after hydrocarbon peak production in the three production leases outlined in this study is not artificially introduced by the declustering procedure.
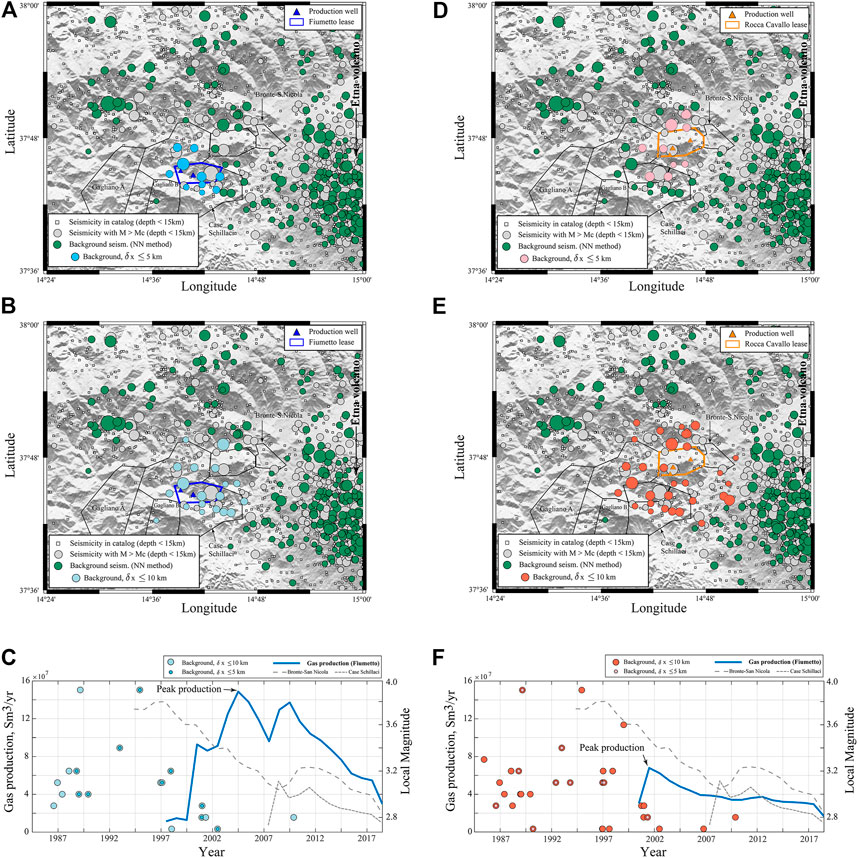
FIGURE 10. Seismic and production data used in the analysis for Fiumetto (A,B,C) and Rocca Cavallo (D,E,F) leases. For the event locations: squares represent the full catalog, whereas the circles show all the events above Mc; colored circles show events identified as background seismicity. Top: map showing background seismicity, highlighting all the events located at distances δx = 5 from the production wells. Middle: the same as the top, but highlighting events at δx = 10 km. Bottom: Time series of production data and plot of the temporal occurrence and magnitude of seismic events. Production data and location of the nearby Bronte-S. Nicola and Case Schillaci leases are also shown for reference.
Figure 10 shows the geographical location and gas production for Fiumetto and Rocca Cavallo leases, and the background seismicity identified for this zone. We also plotted the location of all the other leases in the area, as well as the gas production of the other two cases included in this study (Bronte-S.Nicola and Case Schillaci). It is worth noting that Gagliano has been producing gas for much more longer time with respect to the other four, but it was not possible to include it in the analysis since the peak production precedes the time interval considered in this study. In these plots we highlight all the background events located within 5 and 10 km from the production wells in Fiumetto (Figures 10A,B) and Rocca Cavallo (Figures 10D,E) leases.
In this context, a correlation analysis between seismicity and hydrocarbon production for this area is particularly complex, probably requiring an integrated analysis considering all the leases together. Analyzing the results obtained considering each lease independently, similar to what is observed in Val d’Agri, we observe a decrease in the rate of shallow seismicity after the peak gas production in both Fiumetto and Rocca Cavallo (Figures 10C,F). A similar behavior is observed in the Case Schillaci site as well, but the p value in this case is not statistically significant at the s.l. adopted (neither considering δx = 5 km nor δx = 10 km). Finally, and as mentioned before, the results for Bronte-S. Nicola are contrasting when considering different δx values, as a probable effect of intense, shallow seismicity related to activity at the nearby Etna volcano. It is worth noting that the higher peaks in gas production in this area (in the time interval considered in this study) are reached in Fiumetto, Rocca Cavallo and Bronte-S. Nicola between ∼1997 and ∼2005, a period in which the reduction in the number of events starts to be evident in the zone. However, it should be kept in mind that this area is a particularly complex case due to different factors, such as the closeness of different active leases and the proximity of a seismically active volcano, therefore any further analysis probably requires taking into account the whole cluster of leases together (considering also the influence in this particular area of possible stress perturbations caused by activity at the nearby Etna volcano).
In the areas highlighted by low p values in this study we observe that the seismicity after tm occurs at a lower rate with respect to the seismicity occurring before the peak production. In the case of a link with fluid withdrawal, such observation could result from either an increase in seismicity rate associated with the phase preceding tm, when the fluid withdrawal rate had an increasing trend (see e.g., Figure 5E for reference), or because seismicity is somehow inhibited as the fluid withdrawal process goes on (which results particularly evident after tm). An analysis of the seismicity rate before and after the start of fluid withdrawal operations would be useful to understand which of the two scenarios dominates; however, the seismic data for times preceding the start of operations is too scarce for reliable and systematic analyses. Looking however at the seismicity and production data in Figures 9, 10, it is interesting to note that background seismic events are anyhow present before the start of production in the highlighted cases, and this observation contrasts with the apparent seismicity rate drop observed after the peak production. Moreover, some authors have reported evidences of reservoir depletion processes in the Val d’Agri field; for instance, Improta et al. (2017) reported lacking seismicity correlated with low Vp/Vs zones and interpreted these zones as possible depleted sectors of the Val d’Agri production reservoir. They conclude that these observations agree with increased frictional resistance on preexisting faults within the hydrocarbon reservoir caused by the increase of the effective normal stress resulting from pore pressure depletion induced by significant fluid withdrawal (Improta et al., 2017). These observations lead us to think that the change in rate would primarily be due to a decrease in the number of earthquakes after the peak.
In an attempt to better understand if deformative phenomena associated with fluid withdrawal from the crust may explain an apparent inhibition of shallow seismicity, we analyzed a hypothetical case of deformation related to reservoir depletion and calculate the Coulomb stress changes on fault planes optimally oriented for failure (see e.g., Lin and Stein, 2004; Toda et al., 2005). In practice, we assume the contraction of a sub-horizontal dike in an elastic halfspace. For this theoretical exercise we use approximate geomechanical properties of the Val d’Agri zone taken from literature. The modeled dike has an area that roughly covers the area outlined by the production wells active in the Val d’Agri lease (that we assume as an approximate proxy of the actual areal distribution of the reservoir), and is located at a depth of ∼4 km below surface (information about the depth of the reservoir in Val d’Agri can be found in e.g., Stabile et al., 2014b; Improta et al., 2017).
In agreement with the regional stress field inferred for this region, we assume an extensional tectonic environment (characteristic of normal faulting regimes) with
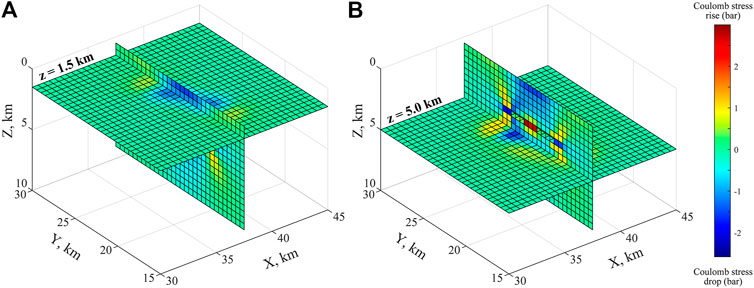
FIGURE 11. Coulomb stress change (bar) calculated on fault panes optimally oriented for failure, considering as the source of deformation the contraction of a sub-horizontal dike located at ∼4 km depth. Both plots show a vertical cross section in the NS direction, crossed by horizontal layers located at (A) 1.5 km and (B) 5.0 km below the surface. (Stress field generated with Coulomb3, Lin and Stein, 2004; Toda et al., 2005) using the following parameter values: i) Poisson ratio 0.25; ii) Young’s modulus = 8.0 × 105 bar; iii) friction coefficient = 0.7; iv) regional stress tensor:
6 Conclusion
In areas characterized by high levels of natural seismicity, the identification of human-induced seismicity is a difficult task for which, virtually, any result can be a source of controversy. In Italy, a relatively high number of oil- and gas-producing leases have been operating in the last decades, many of which are located in the surroundings of seismically active regions (e.g., Sicily, and the central and southern Apennines). Besides hydrocarbon production, underground waste water injection is also performed in different areas of the country, but these activities were not considered in this study because injection-related data are not publicly available.
Therefore, our analyses are focused on areas where fluids (oil and gas) are withdrawn from the crust. Performing detailed, physically-based analyses at the national scale to identity cases of anthropogenic seismicity in Italy may be considered an intractable problem. Consequently, we implemented a large-scale screening procedure aiming at tracking measurable phenomena, such as, e.g., changes in seismicity rates, that plausibly could occur if notable interactions between fluid withdrawal from the crust and nearby seismicity sources are actually occurring in a given area. We stress however that spatial-temporal correlations between proxies of industrial activity and significant changes in seismicity rates do not provide an absolute proof of causal relationships between hydrocarbon production and seismic activity; rather, studying such correlations gives us the possibility of performing large-scale, systematic analyses of a huge amount of seismic and production data, and to identify in this way hotspot areas where to focus more detailed research to verify, in a later stage, possible causal relationships.
In this context, we analyzed seismicity rates before and after peak production in six oil-producing and 43 gas-producing leases, and evaluate the significance of potential seismicity rate changes. Such cases are about the 44% of the development leases active in Italy (the other 56% were not analyzed due to data limitation constraints). The main findings resulting from this study can be summarized as follows:
• When considering the background seismicity located within 5 km from the production wells, about 50% of the oil-producing and 46% of the gas-producing leases analyzed in this study do not show any change in seismicity rate before and after the time at which the peak production is reached; in most of these cases the seismicity rate is zero (basically due to the short distance considered). The percentage of oil- and gas-producing leases with no observed change in the seismicity rate reduces respectively to about 17 and 23% when considering seismicity located within 10 km from production wells.
• None of the observed cases of seismicity rate increase after the hydrocarbon peak production is statistically significant (at a s.l. = 0.05); such cases include about 17% of the oil- and 21–25% of the gas-producing leases analyzed in this study (depending on δx). This result is obtained selecting background seismicity within both 5 and 10 km from production wells.
• Regarding the cases exhibiting a seismicity rate decrease after the hydrocarbon peak production, our results suggest that the observed seismicity rate change is statistically significant (s.l. = 0.05) for one oil-producing lease (the Val d’Agri, in Basilicata) and two gas-producing leases (Fiumetto and Rocca Cavallo, in Sicily). These three leases, which basically correspond with two geographical areas since the later two are adjacent to each other (and to other active leases as Bronte S. Nicola, Case Schillaci, and Gagliano, all clusterized in a relatively small area) can be identified as hotspots deserving future research to study whether there may exist a causal relationship between the hydrocarbon production and the observed reduction in seismicity rate following the peak production.
In conclusion, our analyses highlight areas near some oil- and gas-producing leases in Italy where the seismicity rate reduces after peak production is reached (as compared to the seismicity rate preceding it). We emphasize however that our results just put in evidence a correlated change between the rates of shallow seismicity and hydrocarbon production in these areas, and that assessing actual causal relationships between these two processes will require further detailed, physically-based research. Despite this, we argue that should a physical link exist between these processes, the observed seismicity rate reduction could either be due to increased seismicity during the progressive increase in production rate before tm, or to actual seismicity rate reduction after tm. This second scenario would put in evidence possible processes of seismicity inhibition. Considering that the occurrence of seismicity before the start of hydrocarbon production in the hotspot areas contrasts with the reduction of events observed after the peak production, we suspect that the seismicity inhibition is a plausible hypothesis in these cases. With a simple theoretical exercise based on modelling Coulomb stress changes we showed that, at least under some simplified conditions, inhibition of seismicity is actually possible; nevertheless, more accurate models (e.g., using poroelastic theory) are required for better understanding the nature of such processes.
Our observations draw attention to an interesting research problem: the characteristics and implications of increased seismicity caused by anthropogenic activities (e.g., pressurized fluid injection) have so far had a prominent role in research on induced seismicity; the implications, instead, of anthropic processes potentially capable of inhibiting seismicity—in particular, on seismic hazard in seismically active regions—have received less attention, or have been neglected. We consider that more efforts to study the mechanisms and the possible consequences of anthropogenically-driven seismicity inhibition, as well as to document possible cases of such phenomenon, are required.
Data Availability Statement
The seismic data set analyzed in this study can be accessed through the HORUS (HOmogenized instRUmental Seismic catalog) website: http://horus.bo.ingv.it/ (Lolli et al., 2020a, b). The hydrocarbon production data are publicly available in the website of the Direzione Generale per le Infrastrutture e la Sicurezza dei Sistemi Energetici e Geominerari (DGISSEG), Ufficio Nazionale Minerario per gli Idrocarburi e le Georisorse (UNMIG)'', from the Italian Ministry of Economic Development (MISE): https://unmig.mise.gov.it/index.php/it/dati. The ViDEPI project-Visibilità dei dati afferenti all'attività di esplorazione petrolifera in Italia (Visibility of data related to mineral exploration activities in Italy, in Italian), is available at https://www.videpi.com/ (all sites, last accessed: February 2021).
Author Contributions
AG and LF conceived the experiment, implemented the methods used to analyze the data, and interpreted the achieved results. LF performed the spatial and temporal declustering of the seismic catalog. AG performed the correlation analyses and the Coulomb stress calculations. AM contributed in the discussion of results and in writing. IA collected the industrial data and created the database of hydrocarbon production used in the study. AG and LF wrote the bulk of the text, with inputs from all the co-authors. All the authors have read and approved the manuscript.
Funding
This study was performed with the support of Clypea, the Innovation Network for Future Energy financed by the Italian Ministry of Economic Development, Direzione Generale per le Infrastrutture e la Sicurezza dei Sistemi Energetici e Geominerari (MISE—DGISSEG).
Conflict of Interest
The authors declare that the research was conducted in the absence of any commercial or financial relationships that could be construed as a potential conflict of interest.
Acknowledgments
The authors thank the invaluable support from the “Direzione Generale per le Infrastrutture e la Sicurezza dei Sistemi Energetici e Geominerari (DGISSEG), Ufficio Nazionale Minerario per gli Idrocarburi e le Georisorse (UNMIG),” from the Italian Ministry of Economic Development (MISE), as well as their Innovation Network for Future Energy (Clypea). Maps were plotted using Generic Mapping Tools (GMTs) (Wessel et al., 2019, https://www.generic-mapping-tools.org/, last accessed January 2021). The authors thank also Anna Nardi, Antonio Rossi and Patrizia Battelli for sharing data on the history of the Italian seismic monitoring network. Any use of trade, firm, or product names is for descriptive purposes only. The results and conclusions of this work do not necessarily represent the position of MISE - DGISSEG.
Supplementary Material
The Supplementary Material for this article can be found online at: https://www.frontiersin.org/articles/10.3389/feart.2021.673124/full#supplementary-material
References
Amato, A., and Montone, P. (1997). Present-day Stress Field and Active Tectonics in Southern Peninsular Italy. Geophys. J. Int. 130, 519–534. doi:10.1111/j.1365-246X.1997.tb05666.x
Bertello, F., Fantoni, R., Franciosi, R., Gatti, V., Ghielmi, M., and Pugliese, A. (2010). From Thrust-and-fold belt to Foreland: Hydrocarbon Occurrences in italy. Pet. Geology Conf. Ser. 7, 113–126. doi:10.1144/0070113
Braun, T., Cesca, S., Kühn, D., Martirosian-Janssen, A., and Dahm, T. (2018). Anthropogenic Seismicity in italy and its Relation to Tectonics: State of the Art and Perspectives. Anthropocene 21, 80–94. doi:10.1016/j.ancene.2018.02.001
Buttinelli, M., Improta, L., Bagh, S., and Chiarabba, C. (2016). Inversion of Inherited Thrusts by Wastewater Injection Induced Seismicity at the Val d'Agri Oilfield (Italy). Sci. Rep. 6, 37165. doi:10.1038/srep37165
Calamita, F., Cello, G., Deiana, G., and Paltrinieri, W. (1994). Structural Styles, Chronology Rates of Deformation, and Time-Space Relationships in the Umbria-Marche Thrust System (central Apennines, Italy). Tectonics 13, 873–881. doi:10.1029/94TC00276
Cazzini, F., Zotto, O. D., Fantoni, R., Ghielmi, M., Ronchi, P., and Scotti, P. (2015). Oil and Gas in the Adriatic Foreland, italy. J. Pet. Geology 38, 255–279. doi:10.1111/jpg.12610
Cello, G., and Mazzoli, S. (1998). Apennine Tectonics in Southern Italy: A Review. J. Geodynamics 27, 191–211. doi:10.1016/S0264-3707(97)00072-0
Cesca, S., Grigoli, F., Heimann, S., González, Á., Buforn, E., Maghsoudi, S., et al. (2014). The 2013 September-October Seismic Sequence Offshore Spain: a Case of Seismicity Triggered by Gas Injection? Geophys. J. Int. 198, 941–953. doi:10.1093/gji/ggu172
Cucci, L., Pondrelli, S., Frepoli, A., Mariucci, M. T., and Moro, M. (2004). Local Pattern of Stress Field and Seismogenic Sources in the Pergola-Melandro basin and the Agri valley (Southern Italy). Geophys. J. Int. 156, 575–583. doi:10.1111/j.1365-246X.2004.02161.x
D'Agostino, N., Giuliani, R., Mattone, M., and Bonci, L. (2001). Active Crustal Extension in the central Apennines (Italy) Inferred from GPS Measurements in the Interval 1994-1999. Geophys. Res. Lett. 28, 2121–2124. doi:10.1029/2000GL012462
Dahm, T., Cesca, S., Hainzl, S., Braun, T., and Krüger, F. (2015). Discrimination between Induced, Triggered, and Natural Earthquakes Close to Hydrocarbon Reservoirs: A Probabilistic Approach Based on the Modeling of Depletion-Induced Stress Changes and Seismological Source Parameters. J. Geophys. Res. Solid Earth 120, 2491–2509. doi:10.1002/2014JB011778
Davis, S. D., and Frohlich, C. (1993). Did (Or Will) Fluid Injection Cause Earthquakes? - Criteria for a Rational Assessment. Seismological Res. Lett. 64, 207–224. doi:10.1785/gssrl.64.3-4.207
Davis, S. D., and Nyffenegger, P. A. (1995). The 9 April 1993 Earthquake in South-central texas: Was it Induced by Fluid Withdrawal? Bull. Seismological Soc. America 85, 1888–1895.
Ellsworth, W. L. (2013). Injection-induced Earthquakes. Science 341, 1225942. doi:10.1126/science.1225942
Evans, K. F., Zappone, A., Kraft, T., Deichmann, N., and Moia, F. (2012). A Survey of the Induced Seismic Responses to Fluid Injection in Geothermal and CO2 Reservoirs in Europe. Geothermics 41, 30–54. doi:10.1016/j.geothermics.2011.08.002
Foulger, G. R., Wilson, M. P., Gluyas, J. G., Julian, B. R., and Davies, R. J. (2018). Global Review of Human-Induced Earthquakes. Earth-Science Rev. 178, 438–514. doi:10.1016/j.earscirev.2017.07.008
Garcia-Aristizabal, A., Danesi, S., Braun, T., Anselmi, M., Zaccarelli, L., Famiani, D., et al. (2020). Epistemic Uncertainties in Local Earthquake Locations and Implications for Managing Induced Seismicity. Bull. Seismological Soc. America 110, 2423–2440. doi:10.1785/0120200100
Garcia-Aristizabal, A. (2018). Modelling Fluid-Induced Seismicity Rates Associated with Fluid Injections: Examples Related to Fracture Stimulations in Geothermal Areas. Geophys. J. Int. 215, 471–493. doi:10.1093/gji/ggy284
Grasso, J.-R., Amorese, D., and Karimov, A. (2019). Anthropogenic Seismicity as Aftershocks for Geo-Resource Production? Implications for Mmax Estimates (Reservoir Impoundment Cases). Geophys. J. Int. 219, 958–967. doi:10.1093/gji/ggz337
Grasso, J.-R., Amorese, D., and Karimov, A. (2021). Did Wastewater Disposal Drive the Longest Seismic Swarm Triggered by Fluid Manipulations? Lacq, France, 1969-2016. Bull. Seismological Soc. America. doi:10.1785/0120200359
Grigoli, F., Cesca, S., Priolo, E., Rinaldi, A. P., Clinton, J. F., Stabile, T. A., et al. (2017). Current Challenges in Monitoring, Discrimination, and Management of Induced Seismicity Related to Underground Industrial Activities: A European Perspective. Rev. Geophys. 55, 310–340. doi:10.1002/2016RG000542
Guidoboni, E., and Valensise, G. (2015). On the Complexity of Earthquake Sequences: a Historical Seismology Perspective Based on the L’Aquila Seismicity (Abruzzo, Central Italy), 1315-1915. Earthquakes and Structures 8, 153–184. doi:10.12989/eas.2015.8.1.153
Hainzl, S., Kraft, T., Wassermann, J., Igel, H., and Schmedes, E. (2006). Evidence for Rainfall-Triggered Earthquake Activity. Geophys. Res. Lett. 33. doi:10.1029/2006GL027642
Handy, M. R., M. Schmid, S. S., Bousquet, R., Kissling, E., and Bernoulli, D. (2010). Reconciling Plate-Tectonic Reconstructions of Alpine Tethys with the Geological-Geophysical Record of Spreading and Subduction in the Alps. Earth-Science Rev. 102, 121–158. doi:10.1016/j.earscirev.2010.06.002
Improta, L., Bagh, S., De Gori, P., Valoroso, L., Pastori, M., Piccinini, D., et al. (2017). Reservoir Structure and Wastewater-Induced Seismicity at the Val d'Agri Oilfield (Italy) Shown by Three-DimensionalVpandVp/VsLocal Earthquake Tomography. J. Geophys. Res. Solid Earth 122, 9050–9082. doi:10.1002/2017JB014725
Improta, L., Valoroso, L., Piccinini, D., and Chiarabba, C. (2015). A Detailed Analysis of Wastewater-Induced Seismicity in the Val d'Agri Oil Field (Italy). Geophys. Res. Lett. 42, 2682–2690. doi:10.1002/2015GL063369
IS-EPOS (2016). Completeness Magnitude Estimation [web Application/source Code] – https://tcs.ah-epos.eu/
Kagan, Y. Y. (1991). Fractal Dimension of Brittle Fracture. J. Nonlinear Sci. 1, 1–16. doi:10.1007/BF01209146
Leptokaropoulos, K. M., Karakostas, V. G., Papadimitriou, E. E., Adamaki, A. K., Tan, O., and Inan, S. (2013). A Homogeneous Earthquake Catalog for Western turkey and Magnitude of Completeness Determination. Bull. Seismological Soc. America 103, 2739–2751. doi:10.1785/0120120174
Leptokaropoulos, K., Staszek, M., Lasocki, S., Martínez-Garzón, P., and Kwiatek, G. (2017). Evolution of Seismicity in Relation to Fluid Injection in the North-Western Part of the Geysers Geothermal Field. Geophys. J. Int. 212, 1157–1166. doi:10.1093/gji/ggx481
Lin, J., and Stein, R. S. (2004). Stress Triggering in Thrust and Subduction Earthquakes and Stress Interaction between the Southern san andreas and Nearby Thrust and Strike-Slip Faults. J. Geophys. Res. 109. doi:10.1029/2003JB002607
Lolli, B., Randazzo, D., Vannucci, G., and Gasperini, P. (2020b). Horus - Homogenized Instrumental Seismic Catalog – http://horus.bo.ingv.it/doi:10.13127/HORUS
Lolli, B., Randazzo, D., Vannucci, G., and Gasperini, P. (2020a). The Homogenized Instrumental Seismic Catalog (HORUS) of Italy from 1960 to Present. Seismological Res. Lett. 91, 3208–3222. doi:10.1785/0220200148
Marzocchi, W., and Sandri, L. (2003). A Review and New Insights on the Estimation of the B-Valueand its Uncertainty. Ann. Geophys. 46 (6), 1271–1282. doi:10.4401/ag-3472
Molina, I., Velásquez, J. S., Rubinstein, J. L., Garcia-Aristizabal, A., and Dionicio, V. (2020). Seismicity Induced by Massive Wastewater Injection Near Puerto Gaitán, Colombia. Geophys. J. Int. 223, 777–791. doi:10.1093/gji/ggaa326
Montone, P., Mariucci, M. T., and Pierdominici, S. (2012). The Italian Present-Day Stress Map. Geophys. J. Int. 189, 705–716. doi:10.1111/j.1365-246X.2012.05391.x
Mulargia, F., and Bizzarri, A. (2014). Anthropogenic Triggering of Large Earthquakes. Sci. Rep. 4, 6100–6107. doi:10.1038/srep06100
Orlecka-Sikora, B., Lasocki, S., Kocot, J., Szepieniec, T., Grasso, J. R., Garcia-Aristizabal, A., et al. (2020). An Open Data Infrastructure for the Study of Anthropogenic Hazards Linked to Georesource Exploitation. Sci. Data 7, 89. doi:10.1038/s41597-020-0429-3
Pagliuca, N. M., Battelli, P., Berardi, M., Modica, G., Castellano, C., Melorio, C., et al. (2020). Bollettino Sismico Italiano (BSI), III Quadrimestre 2019. Istituto Nazionale di Geofisica e Vulcanologia (INGV). doi:10.13127/BSI/201903
Parsons, T., and Velasco, A. A. (2009). On Near-Source Earthquake Triggering. J. Geophys. Res. 114 (B10), B10307, 1–14. doi:10.1029/2008JB006277
Pintori, F., Serpelloni, E., Longuevergne, L., Garcia, A., Faenza, L., D'Alberto, L., et al. (2021). Mechanical Response of Shallow Crust to Groundwater Storage Variations: Inferences from Deformation and Seismic Observations in the Eastern Southern Alps, italy. J. Geophys. Res. Solid Earth 126, e2020JB020586. doi:10.1029/2020JB020586
Schoenball, M., Davatzes, N. C., and Glen, J. M. G. (2015). Differentiating Induced and Natural Seismicity Using Space-Time-Magnitude Statistics Applied to the Coso Geothermal Field. Geophys. Res. Lett. 42, 6221–6228. doi:10.1002/2015GL064772
Segall, P. (1989). Earthquakes Triggered by Fluid Extraction. Geol. 17, 942–946. doi:10.1130/0091-7613(1989)017<0942:ETBFE>2.3.CO;2
Segall, P., Grasso, J.-R., and Mossop, A. (1994). Poroelastic Stressing and Induced Seismicity Near the Lacq Gas Field, Southwestern france. J. Geophys. Res. 99, 15423–15438. doi:10.1029/94JB00989
Shapiro, S. A., Dinske, C., and Kummerow, J. (2007). Probability of a Given-Magnitude Earthquake Induced by a Fluid Injection. Geophys. Res. Lett. 34, 1–5. doi:10.1029/2007GL031615
Shapiro, S. A., Dinske, C., Langenbruch, C., and Wenzel, F. (2010). Seismogenic index and Magnitude Probability of Earthquakes Induced during Reservoir Fluid Stimulations. The Leading Edge 29, 304–309. doi:10.1190/1.3353727
Stabile, T. A., Giocoli, A., Lapenna, V., Perrone, A., Piscitelli, S., and Telesca, L. (2014a). Evidence of Low-Magnitude Continued Reservoir-Induced Seismicity Associated with the Pertusillo Artificial Lake (Southern Italy). Bull. Seismological Soc. America 104, 1820–1828. doi:10.1785/0120130333
Stabile, T. A., Giocoli, A., Perrone, A., Piscitelli, S., and Lapenna, V. (2014b). Fluid Injection Induced Seismicity Reveals a Ne Dipping Fault in the southeastern Sector of the High Agri valley (Southern italy). Geophys. Res. Lett. 41, 5847–5854. doi:10.1002/2014GL060948
Stallone, A., and Marzocchi, W. (2019). Empirical Evaluation of the Magnitude-independence assumption. Geophys. J. Int. 216, 820–839. doi:10.1093/gji/ggy459
Tahir, M., Grasso, J.-R., and Amorèse, D. (2012). The Largest Aftershock: How strong, How Far Away, How Delayed? Geophys. Res. Lett. 39, 1–5. doi:10.1029/2011GL050604
Toda, S., Stein, R. S., Richards-Dinger, K., and Bozkurt, S. B. (2005). Forecasting the Evolution of Seismicity in Southern california: Animations Built on Earthquake Stress Transfer. J. Geophys. Res. 110, 1–17. doi:10.1029/2004JB003415
UNMIG (2020). Unmig databook 2020. ministero dello sviluppo economico, direzione generale per le infrastrutture e la sicurezza dei sistemi energetici e geominerari, ufficio nazionale minerario per gli idrocarburi e le georisorse. link: https://unmig.mise.gov.it/images/stat/databook-2020.pdf (last accessed November, 2020).
Valoroso, L., Improta, L., Chiaraluce, L., Di Stefano, R., Ferranti, L., Govoni, A., et al. (2009). Active Faults and Induced Seismicity in the Val d'Agri Area (Southern Apennines, Italy). Geophys. J. Int. 178, 488–502. doi:10.1111/j.1365-246X.2009.04166.x
Valoroso, L., Improta, L., De Gori, P., and Chiarabba, C. (2011). Upper Crustal Structure, Seismicity and Pore Pressure Variations in an Extensional Seismic belt through 3-D and 4-D VPand VP/VSmodels: The Example of the Val d'Agri Area (Southern Italy). J. Geophys. Res. 116, 1–21. doi:10.1029/2010JB007661
van der Voort, N., and Vanclay, F. (2015). Social Impacts of Earthquakes Caused by Gas Extraction in the Province of Groningen, the netherlands. Environ. Impact Assess. Rev. 50, 1–15. doi:10.1016/j.eiar.2014.08.008
van Hinsbergen, D. J. J., Torsvik, T. H., Schmid, S. M., Maţenco, L. C., Maffione, M., Vissers, R. L. M., et al. (2020). Orogenic Architecture of the Mediterranean Region and Kinematic Reconstruction of its Tectonic Evolution since the Triassic. Gondwana Res. 81, 79–229. doi:10.1016/j.gr.2019.07.009
van Stiphout, T., Zhuang, J., and Marsan, D. (2012). Seismicity Declustering, Community Online Resource for Statistical Seismicity Analysis, available at http://www.corssa.orgdoi:10.5078/corssa-52382934
Wells, D. L., and Coppersmith, K. J. (1994). New Empirical Relationships Among Magnitude, Rupture Length, Rupture Width, Rupture Area, and Surface Displacement. Bull. Seismological Soc. America 84, 974–1002.
Wessel, P., Luis, J. F., Uieda, L., Scharroo, R., Wobbe, F., Smith, W. H. F., et al. (2019). The Generic Mapping Tools Version 6. Geochem. Geophys. Geosyst. 20, 5556–5564. doi:10.1029/2019GC008515
Wiemer, S., and Wyss, M. (2000). Minimum Magnitude of Completeness in Earthquake Catalogs: Examples from alaska, the Western united states, and japan. Bull. Seismological Soc. America 90, 859–869. doi:10.1785/0119990114
Wonnacott, T., and Wonnacott, R. J. (1977). Introductory Statistics. 3rd Edn. New York, NY: John Wiley & Sons, Inc.
Zaliapin, I., and Ben‐Zion, Y. (2016). Discriminating Characteristics of Tectonic and Human‐Induced Seismicity. Bull. Seismological Soc. America 106, 846–859. doi:10.1785/0120150211
Zaliapin, I., and Ben-Zion, Y. (2013). Earthquake Clusters in Southern california I: Identification and Stability. J. Geophys. Res. Solid Earth 118, 2847–2864. doi:10.1002/jgrb.50179
Zhang, H., Eaton, D. W., Li, G., Liu, Y., and Harrington, R. M. (2016). Discriminating Induced Seismicity from Natural Earthquakes Using Moment Tensors and Source Spectra. J. Geophys. Res. Solid Earth 121, 972–993. doi:10.1002/2015JB012603
Keywords: regional seismicity, hydrocarbon production, correlation analysis, seismicity rate changes, Italy, anthropogenic hazards
Citation: Garcia A, Faenza L, Morelli A and Antoncecchi I (2021) Can Hydrocarbon Extraction From the Crust Enhance or Inhibit Seismicity in Tectonically Active Regions? A Statistical Study in Italy. Front. Earth Sci. 9:673124. doi: 10.3389/feart.2021.673124
Received: 26 February 2021; Accepted: 07 June 2021;
Published: 02 July 2021.
Edited by:
Rebecca M. Harrington, Ruhr University Bochum, GermanyReviewed by:
Agnes Helmstetter, Université Grenoble Alpes, FrancePiero Poli, Université Grenoble Alpes, France
Copyright © 2021 Garcia, Faenza, Morelli and Antoncecchi. This is an open-access article distributed under the terms of the Creative Commons Attribution License (CC BY). The use, distribution or reproduction in other forums is permitted, provided the original author(s) and the copyright owner(s) are credited and that the original publication in this journal is cited, in accordance with accepted academic practice. No use, distribution or reproduction is permitted which does not comply with these terms.
*Correspondence: Alexander Garcia, alexander.garcia@ingv.it