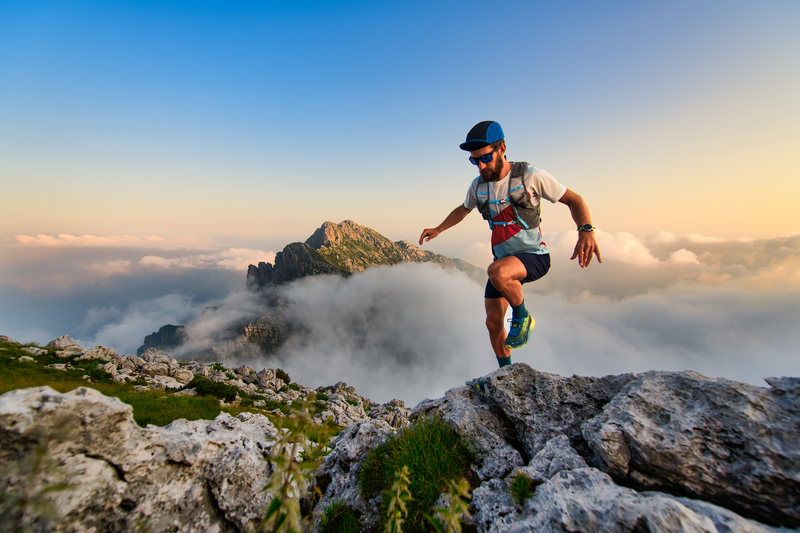
95% of researchers rate our articles as excellent or good
Learn more about the work of our research integrity team to safeguard the quality of each article we publish.
Find out more
DATA REPORT article
Front. Earth Sci. , 21 April 2021
Sec. Solid Earth Geophysics
Volume 9 - 2021 | https://doi.org/10.3389/feart.2021.653275
Ground-penetrating Radar (GPR) a geophysical method based on the propagation of electromagnetic (EM) waves in the 10 MHz to 1 GHz frequency range. An EM pulse is transmitted into the earth by a transmitter antenna. That pulse refracts and reflects at interfaces separating rocks with distinct dielectric properties and is eventually registered in a receiver antenna. GPR has a wide range of applications in geosciences, including hydrogeological (de Menezes Travassos and Menezes, 2004), environmental (Kumar et al., 2016), soil (Aranha et al., 2002), and reservoir analogs (Menezes et al., 2016) studies. A comprehensive review of the GPR acquisition, processing, and interpretation techniques can be found in Jol (2008).
Open-source GPR datasets are becoming more and more common in geosciences. There are some published examples that were collected at several distinct geological scenarios like volcanoes (Leopold and Schorghofer, 2017), river beds (Huber, 2019), wetlands (Matthew et al., 2017), soil (Romero-Ruiz et al., 2018), and test sites (Igel et al., 2019).
Here we present the open-source Itapemirin River delta GPR dataset. The GPR data herein introduced can be used for educational and research purposes, like testing new processing workflows, trying distinct geophysical attributes to be applied to GPR data, or promoting deltaic reservoirs analogs' advanced studies.
The Quaternary sedimentary plains are confined along the Espírito Santo coastline as their geological evolution is associated with sea-level fluctuations and the availability of fluvial sediments. The decrease of 3–4 m in sea level that occurred in the last 5,700 years favored the progradation of the shoreline, originating extensive plains of coastal strands and filling estuaries sandy-muddy sediments and the development of broad mangrove areas (Albino et al., 2016).
The studied area (Figure 1) is in the northern portion of the wave-dominated Itapemirim River delta. The delta shows a cuspate to arcuate geometry, with the coastal strands parallel to the nearly north-south trending shoreline and extending over 10 km. The beach ridges show a stratigraphic sequence of fine to coarse sands (Supplementary Figure 1.3) with heavy minerals lenses such as monazite, ilmenite, zircon, and rutile (CPRM, 2015).
Figure 1. The satellite photograph of the Itapemirim River delta from June 2020 displays the GPR survey location along the coastal strings. Image modified from Google Earth™. Coordinates are in UTM, zone 39S.
The GPR dataset was acquired operating a RAMAC/GPR MALA GeoScience, with the 100 MHz unshielded antennae in the broadside-perpendicular configuration. All GPR profiles were acquired in the fixed-offset mode with an antennae separation of 1.0 m and done in a stop-and-go triggering mode for a maximum coupling with the ground. Antennas were pulled along the profiles with a constant step of 0.20 m. The total time window utilized in the fixed-offset profiles comprised 350 ns. The dataset consists of five 2D lines 50 m long each (Supplementary Figure 1.4). Three lines were acquired along the east-west direction (transversal to the coastline) and two north-south (parallel to the shoreline). The spacing between parallel lines is 25 m.
In practice, when propagating in the geological environment, the effects of absorption and dispersion attenuate the GPR pulse. These effects are revealed in the radargram, mainly as loss of resolution with increasing time. As a result, the spectrum of amplitudes decreases, and displacement of the central frequency toward the lowest frequency components cause a spectral imbalance.
We carried out a set of processing steps aiming, among other aspects, to attenuate noise generated during the acquisition, compensate for the signal loss, and reduce scattered energy at diffracting points in depth. These steps' primary purpose is to produce a final GPR section that highlights the subsurface geological features, facilitating these events' interpretation.
The data processing workflow included seven steps briefly outlined in the following subsections. More details of the whole process can be found in the acquisition-processing report granted as Supplementary Material.
The data editing step included the data reorganization, data file merging, and the time-zero correction (correction of start time to match with surface position). There was no need to apply the static correction as the terrain was planar. As an example of the raw data presented, we show in Figure 2 - Upper Panel, the Profile A radargram loaded into the open-source software GPRPy (Plattner, 2020).
Figure 2. Profile A radargrams loaded in GPRPy. (Upper) edited raw data. (Lower) final processed data.
The first step is the necessary temporal filtering to remove the primary DC signal component and the very low-frequency content from the data. This step is referred to as dewowing the data. Very low-frequency contents of the data are associated with either inductive phenomena or possible instrumentation dynamic range limitations. Dewowing is an essential step as it reduces the data to a mean zero level and, therefore, allows the positive-negative color filling to be used in the recorded traces (Jol, 2008).
Radar waves are very fast attenuated as they propagate into the ground. Usually, signals from great depth are tiny and display this information simultaneously as signals from shallower depths may be unmanageable. When the wave's amplitude is optimal for shallow depth, events from greater depths may be hidden or indistinct. Balancing amplitudes by applying some time-dependent gain function compensates for the rapid fall-off in radar signals from deeper depths.
We applied the AGC (automatic gain control), a continuously adaptive gain filter. With the AGC gain, each data trace is processed, so that the average signal is computed over a time window. The aspired output value's quotient magnifies the data point at the window's center to the average signal amplitude.
Migration is a technique that compensates spurious effects introduced by wave propagation and acquisition geometry. Dipping events are misplaced, the lateral resolution is low, and a wide array of non-geological effects like diffractions influence the data to mislead interpretation. Migration concentrates the energy along hyperbolas to the actual spatial position from which the energy originated. Migration relocates dipping reflections to their proper subsurface position and collapses diffractions, thus enhancing spatial resolution. A practical limitation of the migration approach lies in the fact that our data is 2D, and out-of-plane diffractions will not be correctly dealt with within the migration process.
In this work, we used Stolt's f–k migration approach (Yilmaz, 2001). It is assumed that the GPR data can be considered as zero offset and that the average velocity can be taken as a constant background migration velocity Vm = 0.10 m/ns.
We estimated the migration velocity using a try-and-error approach. We performed the migration rounds using multiple velocities in the 0.05 to 0.15 m/ns range at 0.01 m/ns intervals. The chosen velocity, 0.10 m/ns, produced a radargram attenuated the waves' diffraction effects, reducing the hyperboles without smearing out the geological reflectors.
Temporal filtering is often used in the GPR processing for spectral balancing and downgrade high-frequency noise. Filtering can be employed before or after AGC as long as the gain's effect is understood since the AGC is a non-linear process. We applied a 30–110 MHz band-pass FFT filter to attenuate the high-frequency noise before using an AGC filter second pass. The filter was designed to prevent noise boosting in this second pass of the gain.
For stratigraphic and structural interpretation, the reflectors' continuity is more important than the information irrespective of amplitude fidelity. Therefore, we applied the second pass of AGC attempting to equalize the radar signals by using a gain inversely proportional to signal strength. As result, the difference between the raw and processed spectra is up to three times larger. In the final processed Profile A radargram (Figure 2 - Lower Panel), we can observe the enhancement of the radar reflections' sharpness and continuity compared with the raw data (Figure 2 - Upper Panel), thus making the interpretation process of the main sedimentary reflections, out of the scope in the present paper, more amenable.
In this last step, we converted the processed radar files to the standard seismic SEG-Y format and loaded the dataset in the open-source seismic interpretation software OpendTect (Huck, 2012).
Our dataset can be employed for several applications like teaching, practices with new processing methodologies or workflows, promote advances in deltaic sedimentation and reservoirs analogs studies, and experiments with different GPR interpretation attributes. To that end, we made available the raw and processed data in the Sensors and Software (.dt1) and Seg-Y format. We also provide a full Opendtect (ODT) survey project. Further details of the acquisition, data processing, and a 3D view of the ODT survey are found in the companion reports, available as additional material.
To take part in the Resource Identification Initiative, please use the corresponding catalog number and RRID in your current manuscript. For more information about the project and for steps on how to search for an RRID, please click here.
The datasets presented in this study can be found in online repositories. The names of the repository/repositories and accession number(s) can be found at: Mendeley Data Menezes (2020).
PM acquired and processed the GPR data.
The GPR survey was funded by UERJ(Universidade do Estado do Rio de Janeiro).
The author declares that the research was conducted in the absence of any commercial or financial relationships that could be construed as a potential conflict of interest.
PM acknowledges Universidade do Estado do Rio de Janeiro (UERJ) for funding the fieldwork. PM was also grateful to A. Plattner for making freely available the GPRPy processing software and dGB Earth Sciences for the seismic interpretation software OpendTect.
The Supplementary Material for this article can be found online at: https://www.frontiersin.org/articles/10.3389/feart.2021.653275/full#supplementary-material
Albino, J., Neto, N. C., and Oliveira, T. C. A. (2016). “The beaches of Espírito Santo,” in Brazilian Beach Systems, eds A. Short and A. Klein (New York, NY: Springer), 333–361. doi: 10.1007/978-3-319-30394-9_13
Aranha, P. R. A., Augustin, C. H. R. R., and Sobreira, F. G. (2002). The use of GPR for characterizing underground weathered profiles in the sub-humid tropics. J. Appl. Geophys. 49, 195–210. doi: 10.1016/S0926-9851(02)00123-4
CPRM (2015). “Geologia e recursos minerais do estado do espírito santo,” in Texto Explicativo do Mapa Geológico e de Recursos Minerais, eds V. Vieira and R.G. de Menezes (Rio de Janeiro: Companhia de Pesquisa de Recursos Minerais-CPRM/Serviço Geológico do Brasil), 289.
de Menezes Travassos, J., and Menezes, P. D. T. L. (2004). GPR exploration for groundwater in a crystalline rock terrain. J. Appl. Geophys. 55, 239–248. doi: 10.1016/j.jappgeo.2004.01.001
Huber, E. (2019). GPR Data Used to Test the efficient Deconvolution Method of Schmelzbach and Huber (2015) (Version 1.0.0) [Data set]. Zenodo. doi: 10.5281/zenodo.2586189
Huck, H. (2012). “The road to open source: sharing a ten years experience in building opendtect, the open source seismic interpretation software,” in 74th EAGE Conference and Exhibition-Workshops (Copenhagen: European Association of Geoscientists & Engineers), 295.
Igel, J., Günther, T., Müller-Petke, M., Dlugosch, R., and Jiang, C. (2019). Hydrogeophysical Data Schillerslage Test Site, Joint MRT ERT GPR (Version 1.0) [Data set]. Zenodo. doi: 10.5281/zenodo.2686824
Kumar, V. S., Dhakate, R., Amarender, B., and Sankaran, S. (2016). Application of ERT and GPR for demarcating the saline water intrusion in coastal aquifers of southern india. Environ. Earth Sci. 75:393. doi: 10.1007/s12665-015-5207-8
Leopold, M., and Schorghofer, N. (2017). Mauna Kea Permafrost Survey, Geophysical Data [Data set]. Zenodo. doi: 10.5281/zenodo.896963
Matthew, M., Xavier, C., Brian, B., Ross, H., and David, S. (2017). Estimating Belowground Carbon Stocks in Isolated Wetlands of the Northern Everglades Watershed, Central Florida, Using Ground Penetrating Radar (GPR) and Aerial Imagery [Data set]. Zenodo. doi: 10.5281/zenodo.848862
Menezes, P. (2020). Itapemirim River Delta GPR Dataset. Mendeley Data, V1. doi: 10.17632/zfhrs9bkv8.1
Menezes, P. T. L., Travassos, J. M., Medeiros, M. A. M., and Takayama, P. (2016). High-resolution facies modeling of presalt lacustrine carbonates reservoir analog: Morro do Chaves Formation example, Sergipe-Alagoas Basin, Brazil. Interpretation 4, SE63–SE74. doi: 10.1190/INT-2014-0213.1
Plattner, A. M. (2020). Gprpy: open-source ground-penetrating radar processing and visualization software. Leading Edge 39, 332–337. doi: 10.1190/tle39050332.1
Romero-Ruiz, A., Linde, N., Keller, T., and Or, D. (2018). Geophysical Data From: “A Review of Geophysical Methods for Soil Structure Characterization” [Data set]. Zenodo. doi: 10.5281/zenodo.1451685
Keywords: ground penetrating radar, reservoir analogs, deltaic sedimentation, processing workflow, open-source dataset
Citation: Menezes PTL (2021) The Itapemirim River Delta GPR Dataset. Front. Earth Sci. 9:653275. doi: 10.3389/feart.2021.653275
Received: 14 January 2021; Accepted: 26 March 2021;
Published: 21 April 2021.
Edited by:
Pier Paolo Bruno, University of Naples Federico II, ItalyReviewed by:
Vincenzo Di Fiore, Consiglio Nazionale delle Ricerche (CNR), ItalyCopyright © 2021 Menezes. This is an open-access article distributed under the terms of the Creative Commons Attribution License (CC BY). The use, distribution or reproduction in other forums is permitted, provided the original author(s) and the copyright owner(s) are credited and that the original publication in this journal is cited, in accordance with accepted academic practice. No use, distribution or reproduction is permitted which does not comply with these terms.
*Correspondence: Paulo T. L. Menezes, cGF1bG8ubWVuZXplc0B1ZXJqLmJy
Disclaimer: All claims expressed in this article are solely those of the authors and do not necessarily represent those of their affiliated organizations, or those of the publisher, the editors and the reviewers. Any product that may be evaluated in this article or claim that may be made by its manufacturer is not guaranteed or endorsed by the publisher.
Research integrity at Frontiers
Learn more about the work of our research integrity team to safeguard the quality of each article we publish.