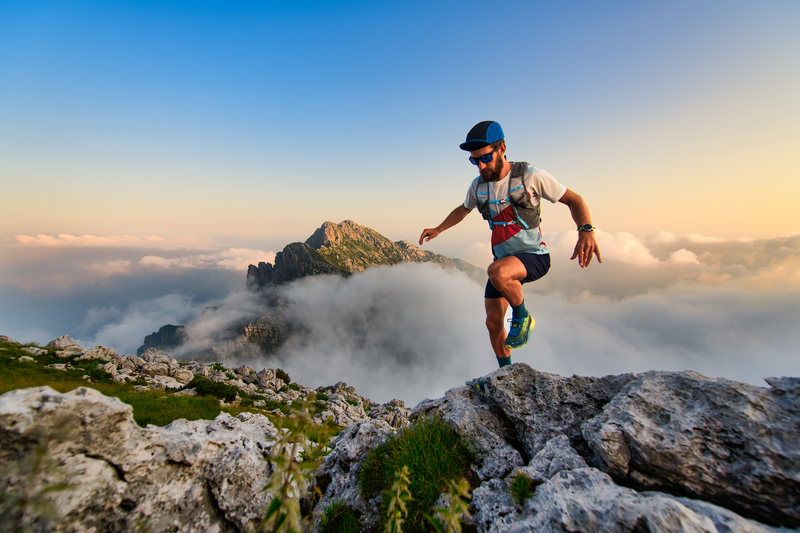
95% of researchers rate our articles as excellent or good
Learn more about the work of our research integrity team to safeguard the quality of each article we publish.
Find out more
ORIGINAL RESEARCH article
Front. Earth Sci. , 29 January 2021
Sec. Geohazards and Georisks
Volume 8 - 2020 | https://doi.org/10.3389/feart.2020.601288
This article is part of the Research Topic Geohazards and Risks in High Mountain Regions View all 9 articles
Ongoing recession of glaciers in the Himalaya in response to global climate change has far-reaching impacts on the formation and expansion of glacial lakes. The subsequent glacial lake outburst floods (GLOFs) are a significant threat to lives and livelihoods as they can cause catastrophic damage up to hundreds of kilometres downstream. Previous studies have reported the rapid expansion of glacial lakes and several notable destructive past GLOF events in the Mahalangur Himalaya, suggesting a necessity of timely and updated GLOF susceptibility assessment. Here, an updated inventory of glacial lakes across the Mahalangur Himalaya is developed based on 10-m Sentinel-2 satellite data from 2018. Additionally, the GLOF susceptibilities of glacial lakes (≥0.045 km2) are evaluated using a multi-criteria-based assessment framework where six key factors are selected and analyzed. Weight for each factor was assigned from the analytical hierarchy process. Glacial lakes are classified into very low, low, medium, high, and very high GLOF susceptibility classes depending upon their susceptibility index based on analysis of three historical GLOF events in the study area. The result shows the existence of 345 glacial lakes (>0.001 km2) with a total area of 18.80 ± 1.35 km2 across the region in 2018. Furthermore, out of the 64 glacial lakes (≥0.045 km2) assessed, seven were identified with very high GLOF susceptibility. We underline that pronounced glacier-lake interaction will likely increase the GLOF susceptibility. Regular monitoring and more detailed fieldworks for these highly susceptible glacial lakes are necessary. This will benefit in early warning and disaster risk reduction of downstream communities.
The Himalaya is characterized by a dense distribution of glacial lakes (Zhang et al., 2015; Maharjan et al., 2018), and they are rapidly expanding due to glaciers wasting in response to global climate change (Zhang et al., 2015; Nie et al., 2017). Such glacial lakes are located on the surface of glaciers (supraglacial lakes or ponds), behind the terminal or lateral moraines (proglacial lakes), and other lakes which are disconnected, but lie in the periphery to the glacier and are fed by the glacier ice and snowmelt (Salerno et al., 2012; Otto, 2019). These glacial lakes display characteristic differences in terms of formation, dam structure, lifespan, expansion, emergence, disappearance and impact upon burst (Richardson and Reynolds, 2000; Mertes et al., 2017; Nie et al., 2017). Glacial lake outburst flood (GLOF) occur when the sudden release of large water volumes from the glacial lakes appear either by dam failure or overtopping waves due to external triggering or self-destruction, such as ice/rock avalanche and extreme precipitation into the lake (Somos-Valenzuela et al., 2015; Harrison et al., 2018). GLOFs are often associated with sediments and debris flows, having the potential for catastrophic damage to downstream areas (Osti and Egashira, 2009; Liu et al., 2014). The Himalayan region has been documented as a hot spot of GLOFs primarily from moraine-dammed glacial lakes with several historical GLOF records (Carrivick and Tweed, 2016; Nie et al., 2018; Veh et al., 2019).
The formation of new glacial lakes and the rapid expansion of the existing ones increases the risk of GLOFs in the Himalaya (Wang et al., 2015; Nie et al., 2017; Khadka et al., 2018). Mahalangur Himalaya, a section of central Himalaya, is a highly glacierized area (Bajracharya and Mool, 2009). Glaciers in this region are responding to rising air temperature and reduced annual precipitation with moderate negative mass balance (Salerno et al., 2015). Consequently, an accelerated areal expansion of glacial lakes has been observed between 1987 and 2017 (Khadka et al., 2018) and lakes such as Imja Tsho and Barun Tsho have the possibility of future expansion (Watson et al., 2020). This implies that the potential threat posed by glacial lakes in this region may also be increasing, and thus a timely and updated assessment of GLOF susceptibility, the tendency of future occurrence of GLOF hazards, is urgent. In addition, about six historical GLOF events have been well-recorded in the Mahalangur region (Byers et al., 2018; Nie et al., 2018; Veh et al., 2019); for instance, notable GLOF events from Nare Lake (1977), Dig Tsho (1985), Tam Pokhari (1998) and Langmale Lake (2017) (ICIMOD, 2011; Byers et al., 2018). The avalanche triggered displacement waves in Dig Tsho resulted in an estimated peak discharge of >2,000 m3 s−1, destroying a small hydropower project, a trail, and causing the loss of life and property (Vuichard and Zimmermann, 1987; Richardson and Reynolds, 2000). Almost more than a decade later, the moraine dam of Tam Pokhari collapsed in 1998 due to avalanche/landslides (Byers et al., 2013; Lamsal et al., 2016b) with an estimated 10,000 m3 s−1 peak discharge and approximately 18 million m3 water being released (Osti and Egashira, 2009). This GLOF caused nearly US$ 2 million in economic damage and the loss of lives (Dwivedi et al., 2000; ICIMOD, 2011). A recent GLOF in 2017 from the Langmale Lake in the Barun Valley was triggered by rockfall and had a peak discharge of 4,400 ± 1,800 m3 s−1, damaging buildings, bridges, and farmlands (Byers et al., 2018). The triggering mechanisms such as ice, snow and rock avalanching, glacier calving, wave overtopping, atmospheric triggers (extreme precipitation), ice-cored moraine degradation and earthquakes are the causes of dam failure and thus GLOFs, which are considered as the components in the GLOF modeling cascade (Westoby et al., 2014).
Different methods are used to appraise the potentially dangerous glacial lakes (PDGL) across the Himalaya (e.g., Rounce et al. (2016); Worni et al. (2013); Prakash and Nagarajan (2017)), which were adopted and modified from the Swiss Alps (Huggel et al., 2004), British Columbia (McKillop and Clague, 2007), and other parts of the world (Wang et al., 2011; Emmer and Vilímek, 2013). These methods are generally different among others, with the selection of contrasting parameters for hazard and risk assessment. Rounce et al. (2016) comprehensively reviewed the existing methods and developed a robust and unified evidence-based GLOF hazard assessment framework. Based on this method, GLOF hazard assessment of glacial lakes (≥0.1 km2) in Nepalese Himalaya has been conducted and classified into four levels, where glacial lakes with steep moraine dam and susceptibility to avalanche were considered to be very high hazard level (Rounce et al., 2017b). Allen et al. (2019) assessed the GLOF risk of all glacial lakes (≥0.1 km2) in the Tibetan Plateau as a function of hazard and exposure using an automated, large-scale assessment approach that integrated lake area, upstream watershed area of each lake, the topographic potential for ice/rock avalanches and mean slope of the dam to model and ranks the hazard level of each lake. They identified the exposure of buildings to the modelled GLOF flow path for each lake to represent its subsequent downstream impacts. Wang et al. (2015) identified the PDGLs in the Chinese Himalaya by selecting four factors and using the analytical hierarchy process (AHP) to determine their weights. Moreover, eleven factors were considered by Prakash and Nagarajan (2017) for identifying the PDGLs over the western Himalaya whose precise remote measurements are difficult. Hence, the selection and use of an appropriate method and factors for GLOF susceptibility are necessary to identify the PDGLs and provide the first-order ranking of glacial lakes. Furthermore, the recent destructive GLOF events in the Nepalese (Byers et al., 2018) and Indian Himalaya (Das et al., 2015; Rafiq et al., 2019) are reported from glacial lakes <0.1 km2. Thus, we aim at lowering the size threshold to 0.045 km2 from 0.1 km2, which was mostly used by previous studies, to include more small glacial lakes in this study. The lowering of the size threshold has also been emphasized by previous studies (Nie et al., 2018; Veh et al., 2019).
In this study, we aim to 1) map the glacial lakes across the Mahalangur Himalaya using the medium to high-resolution Landsat, and Sentinel-2 satellite images, and 2) evaluate their GLOF susceptibility selecting influencing factors and assigning their weights using the AHP method. More importantly, Nepal is ranked as one of the countries in the Himalaya with the greatest national-level socio-economic impacts from the GLOFs (Carrivick and Tweed, 2016). This study will evaluate the GLOF susceptibility of the lakes with the aim of identifying the potentially dangerous glacial lakes for detail investigations and for safeguarding life and properties downstream through the informed-decision making.
Mahalangur Himalaya (Mahalangur Himal) is the section of Himalaya covering northeastern Nepal and south-central Tibet (China) bounded on the east by the Popti La and the Arun River and on the west by the Gyabrag Glacier, the Nangpa La, Nangpa Tsangpo and Dudh Koshi (Carter, 1985). This study is focused on the southern part of the main Himalaya range encompassing the Dudh Koshi and Barun River basins, covering a total area of 3,701 km2 (Figure 1). This region includes four of the six highest mountain peaks of the world: Mt. Everest (8,849 m a.s.l., Sagarmatha in Nepali), Mt. Lhotse (8,516 m a.s.l.), Mt. Makalu (8,485 m a.s.l.), and Mt. Cho You (8,188 m a.s.l.) (Figure 1). The climate of the study area ranges from the temperate in the lower elevation regions in the south to polar tundra climate in the higher elevation regions in the north (Karki et al., 2016). The climate is influenced by South Asian monsoons in summer and westerlies in winter (Hamal et al., 2020; Sharma et al., 2020b). The climatology of this region obtained from the Pyramid weather station (5,050 m a.s.l., Figure 1A) reveals the maximum precipitation and air temperature during the summer (June–September) and minimum during the winter (December–February) (Figure 1C). The temporal analysis of maximum, minimum and mean monthly air temperature shows +1.75, –5.71, and –2.48 °C month−1 during 1994–2013, while cumulated mean precipitation of ∼436 mm year−1 during 1994–2016 (Figure 1C).
FIGURE 1. (A) Map showing the spatial distribution of glaciers, glacial lakes and historical GLOF events in the Mahalangur region. The insets show (B) the location of the study area in the Himalaya (base map from ESRI) and (C) the annual monthly distribution of air temperature (1994–2013) and precipitation (1994–2016) obtained from the high elevation pyramid weather station (5,050 m a.s.l.). The error bars represent the standard deviation. The boundary of the Himalaya is adapted from Nie et al. (2017).
The study area covers a total glacierized area of ∼740 km2 derived from an updated GAMDAM glacier inventory (Sakai, 2019). Most of the glaciers are valley glaciers with debris cover in the ablation zone that extends in broad elevation range from 4,817 to 6,732 m a.s.l. (Bajracharya and Mool, 2009; Rounce and McKinney, 2014; Salerno et al., 2015). The mean mass balance for nine large glaciers in the Dudh Koshi basin was found to be −0.58 ± 0.19 m w.e. a−1 between 2005 and 2015 (King et al., 2017). The glaciers in the southern slope of the Everest region were found to be shrinking with an overall area loss of 13 ± 3% during 1962–2011 (Thakuri et al., 2014). This region hosts the highest number of glacial lakes per square kilometer compared to other parts of Nepal (Khadka et al., 2018) and has previously experienced six GLOF events in 1977, 1985, 1998, 2015, 2016 and 2017 (Byers et al., 2018; Nie et al., 2018).
Multi-source optical satellite images from Landsat and Sentinel missions were used to delineate the glacial lake outlines across the Mahalangur Himalayan region (Table 1). Landsat images were obtained from the U.S. Geological Survey (USGS) data portal (http://earthexplorer.usgs.gov) and Sentinel-2 images were downloaded from the Copernicus open access hub of the European Space Agency (https://scihub.copernicus.eu/). The images were from the post-monsoon period (October and November) nearly free from cloud, and seasonal snow cover in the study area. Pleiades imagery was used for validation of glacial lake delineations. The 30-m Shuttle Radar Topography Mission Digital Elevation Model (SRTM DEM) used in this study was downloaded from the National Aeronautics and Space Administration (NASA) data portal (https://urs.earthdata.nasa.gov/). The latest updated glacier outlines from the Glacier Area Mapping for Discharge from the Asian Mountains (GAMDAM) inventory was used (Sakai, 2019). For the climatological study, we used daily air temperature (1994–2013) and precipitation data (1994–2016) from the high elevation Pyramid Automatic Weather Station (5,050 m) located within the study area (Figure 1). Modeled ice-thickness data was obtained from Farinotti et al. (2019) to model possible future expansion of lakes.
The glacial lake extent was delineated by consistent manual digitization from the geometrically corrected false-colour composite Landsat (30 m) and Sentinel-2 images (10 m). The alternative images of the same season were used whenever the shading and cloud cover disturbed the lake delineation. Moreover, the scene classification algorithm in Sentinel Application Platform (SNAP) (Chand and Watanabe, 2019) and Google Earth were used to remove the effect of shading in lake delineations. All lakes within 10 km buffer zone from the nearest glacier were included and classified into four types based on their positions in relation to the glaciers: supraglacial lakes on the surface of glaciers; proglacial lakes at the lateral or terminal position of the glacier; and unconnected lakes far from glaciers that were further classified into unconnected glacial-fed and non-glacial fed lakes depending upon whether the lake gets fed by glacier melt (Nie et al., 2017; Khadka et al., 2018). For glacial lake classification, each lake was assigned a unique code in ArcGIS and the lake, glacier, and lake watershed shapefiles were converted into KML files. The KML files were loaded into Google Earth and lakes were classified into different types (supraglacial, proglacial and unconnected lakes) according to visual interpretation using their respective codes (Khadka et al., 2018). In Google Earth, lakes usually far from glaciers with no glaciers in their watershed were classified as unconnected non-glacial fed (Zhang et al., 2015). The minimum size threshold of ∼0.001 km2 (10 pixels of Sentinel-2 image) was used to map glacial lakes in 2018.
We designed and applied the multi-criteria decision-based method to assess the GLOF susceptibility across the Mahalangur Himalaya. The overall processes followed in the GLOF susceptibility assessment is shown in Figure 2. Here, we identified and compiled several factors governing the mechanism of GLOF potential based on previous literature (Supplementary Table S1). Similarly, we complied and studied the details characteristics of three GLOFs in the study region (Table 2). Various triggering factors can initiate the GLOFs through either by dam overtopping or dam failure. The GLOF initiating from these two cases are determined by dam characteristics (dam type, dam freeboard, dam crest width, dam width to height ratio, slope of the dam, piping), lake and glacier characteristics (lake area, lake perimeter, area expansion rate, glacier and lake distance), lake surrounding characteristics (potential for snow/rockfall avalanches), and other characteristics (earthquake, extreme precipitation events) (Emmer and Vilímek, 2014; Prakash and Nagarajan, 2017). Here, six factors ((lake area (F1), expansion rate (F2), glacier-lake distance (F3), dam front slope gradient (F4), ice/snow avalanche potential (F5) and potential of rockfall with upstream GLOF (F6)) were selected to examine the GLOF susceptibility as they represent lake and glacier characteristics, dam characteristics and surrounding terrain characteristics (Table 3 and Supplementary Table S1). Eminently, factors F1-F6 are used to characterize the GLOF resulting from dam failure, whereas F1, F2, F5, and F6 are used for the GLOF resulting from dam overtopping (Prakash and Nagarajan, 2017). Furthermore, these factors well represent the characteristics of three well-documented past GLOF events within the study area (Table 2) and can be remotely measured from freely available datasets. Each factor was classified into alternatives based on previous literature, the potentiality for triggering a GLOF and their potential role on GLOF impact depending upon detail characteristics of three GLOF events in the region (Table 3), nevertheless, partly influenced by subjective expert judgement (refer Factor selection and GLOF susceptibility).
FIGURE 2. Flowchart for GLOF susceptibility evaluation. AHP represents the analytical hierarchy process. Refer to Figure 4 for the definition of factors.
In this study, glacial lakes with an area of ≥0.045 km2 were considered in the GLOF susceptibility assessment, as suggested by Nie et al. (2018). Moreover, GLOF from Dig Tsho and Tam Pokhari glacial lakes (∼0.5 km2) had caused catastrophic damage in the region; meanwhile, large lakes have the potential to generate large flood volume than smaller lakes (ICIMOD, 2011; Fujita et al., 2013). Furthermore, lakes with the large surface area are exposed to higher chances of impact from the potential mass movement from the surrounding terrain. Hence, the size threshold of ≥0.5 km2 was set as the upper limit, and the F1 class was adjusted accordingly (Table 3).
Previous studies had used the threshold of >100% expansion to determine the lake growth in 40 years period for GLOF susceptibility assessment (Bolch et al., 2011; Aggarwal et al., 2017). Following the previous study, here, the threshold value of >50% was chosen for determining the expansion rate of glacial lakes in the period of 20 years (1998–2018). Notably, glacial lakes were considered as rapidly expanding that had an expansion rate of 20% in 20 year period (Nie et al., 2013; Nie et al., 2017). Thus, the expansion rate >50% was considered as the upper limit and <20% as the lower limit to adjust the F2 class (Table 3). Distinctly, out of three, two glacial lakes had high expansion rates before their burst in the study area (Figure 3). Seven glacial lakes evolved after 1998, so their expansion rate was considered as the highest alternative.
FIGURE 3. The (A) Dig Tsho, (B) Tam Pokhari and (C) Langmale glacial lakes at different stages before and after their outburst and at present (2018). The arrow shows the v-shaped trench with debris outcrops revealing the evidence of past GLOF. The background images are from Corona (1962), Sentinel-2 (2018) and remaining from Landsat TM/OLI.
As all glacial lakes were chosen regardless of types (lakes connected or unconnected to glaciers), the glacier and lake proximity was considered as one of the factors in the GLOF susceptibility evaluation, which was determined from delineated lake polygon and glacier outline. Out of 12 Himalayan GLOF cases, nine lakes were in contact with glacier before burst, while others were far within 700 m (Supplementary Table S2). Similarly, the previously drained glacial lakes in Tibetan Plateau were reported to be at the distance of 0–800 m from the glacier, and Wang et al. (2011) used <500 m threshold determining it to be more favourable value for a lake to outburst. Moreover, those glacial lakes that have experienced GLOFs in the study area were connected to their parent glaciers (Table 2). Thus, glacial lakes in contact with glaciers were set as the upper limit and >500 m as the lower limit to adjust the F3 class (Table 3).
TABLE 3. Selected factors with their classes and index values. Rank and weight of each factor are computed using the AHP method.
Dam characteristics, such as dam geometry (dam width to height ratio, width of crest, dam distal face slope), dam material properties, ice-cored moraine and freeboard conditions govern the stability of the dam (Huggel et al., 2004; Wang et al., 2011; Prakash and Nagarajan, 2017). However, owing to the difficulty for precise remote measurements of these parameters, we used a single feature, i.e., dam front slope gradient (DFSG) to model the stability of the moraine dam. DFSG was defined as a tangent angle determined by the difference in height between the lake dam and the dam base, overall considering a horizontal distance downstream of ∼0.6–1 km as the dam base differently to all lakes (Figure 4). The DFSG was calculated along the terminal moraine and/or flow path of the glacial lake. The DFSG is reasonably similar to the average steep lakefront area slope (Fujita et al., 2013) and is mostly relevant to moraine-dammed glacial lakes, indicating possibilities of erosion, incision and failure. Thus, glacial lakes were classified according to their dam types into ice-dammed, moraine-dammed and rock-dammed (Supplementary Table S7) from visual interpretation in Google Earth following the classification scheme described by Otto (2019). Here, the glacial lakes with a steep dam (DFSG >10°) were classified as the highest alternative, and F4 has been adjusted accordingly (Table 3). The value of DFSG for bedrock dammed glacial lakes was assigned as zero as they are relatively stable when compared to moraine dams. Moreover, the GLOF in the Himalaya are most often reported as breaches from moraine dam (ICIMOD, 2011; Nie et al., 2018).
FIGURE 4. The concept of six factors considered in the GLOF susceptibility assessment: an example from South Chamlang Tsho. Here, θ represents the dam front slope gradient and the value of DFSG for rock dammed glacial lakes is zero. The background is a false-colour composite Sentinel-2 image.
The impact of the mass movement has caused more than 70% of the known GLOF events (dynamic failure) in the Himalaya, either from ice/snow avalanche, rockfall/landslide or upstream GLOF (Supplementary Figure S1). The avalanche is the major trigger of GLOFs in the Himalaya (Nie et al., 2018). Thus, this factor was ranked as the highest priority by expert judgement, and the maximum weight assigned to this factor from AHP is justifiable (Table 3). Glacial lakes susceptible to avalanche and rockfall were classified as the highest alternative (F5 and F6), while those susceptible to upstream GLOF (lakes >0.05 km2 located upstream) were classified as medium (Table 3). Within the upstream watershed of each glacial lake, ice and rock avalanche-prone areas were calculated as the sum of the pixels fulfilling the criteria of slope >30°, where the overall trajectory slope is >17° (or angle of reach; tanα = 0.25) (Allen et al., 2019). Further, glacier outlines were used to discriminate the probable ice and rock/landslide areas (Figure 4). The overall process was modelled in ArcGIS 10.5 software. Moreover, those lakes with large watersheds where the prone areas were located at horizontal distance >2 km2 were discarded based on flow direction algorithm in ArcGIS 10.5, assuming that the avalanche/rockfall does not hit the lake directly. However, in exceptional cases, mass movements from more gentle slopes and obtaining larger run-out distances are possible.
The AHP method was used to assign the weights of each factor. The AHP is a semi-quantitative decision-making approach using weights through pairwise comparison between different factors without inconsistencies (Saaty, 1990). The expert evaluation was conducted to find the relative importance of each factor against each other by establishing a comparison matrix (Supplementary Table S3). Finally, the comparison matrix was normalized to determine the rank and weight of each factor through AHP (Tables 3 and Supplementary Table S4). The consistency ratio of the computed AHP was ∼9% within an acceptable range for good consistency in the judgements and pairwise comparisons (Supplementary Table S5) (Saaty and Vargas, 2012). Three alternatives are provided with index values of 1, 0.5 and 0.25 (Prakash and Nagarajan, 2017). The final weights for each factor were computed by multiplying the factor weight (Wi) with the class index values (Ci) based on the measurement of lake factors. The final weights of each factor were summed to evaluate the outburst susceptibility of the lake (Eq. (1)).
The value of GLOF susceptibility index falls between 0.25 and 1. To display the results of GLOF susceptibility, the values obtained from GLOF susceptibility index (0.25–1) are categorized into five classes using the equal interval classification method (Figure 2). This method is representative as revealed by previous three GLOFs in the study area, which had the susceptibility index exceeding 0.85 (Table 2).
The sources of uncertainties in the GLOF susceptibility assessment can arise from the satellite data used for preparing the inventory and the DEM used for estimating the factors. The uncertainty in glacial lake delineation was calculated as a product of the perimeter of the lake and half of the resolution of data (Salerno et al., 2012). The uncertainty in lake delineation affects the input values for F1 (lake area) and F2 (lake expansion rate); however previous studies (Nie et al., 2017; Rounce et al., 2017b) have successfully used the similar dataset for preparing the inventory and computing the factors for the GLOF hazard (Rounce et al., 2017b; Allen et al., 2019), susceptibility (Prakash and Nagarajan, 2017), and risk (Wang et al., 2015) assessments. Moreover, the use of 30 m SRTM DEM in the high mountainous region has limitation to reveal the topography precisely.
In a multi-criteria decision-based GLOF assessment, sensitivity analysis (SA) plays a key role to determine how much uncertainty of the results of the assessment are influenced by the uncertainty of its input criteria (Saltelli et al., 1999). Here, we performed the SA in two ways: 1) shifting the class/alternative and 2) modifying the GLOF susceptibility class. We checked the sensitivity of GLOF assessment by shifting the low and medium class/alternative of F1 to medium and high classes, respectively keeping other factors constant. Similarly, SA was performed for the remaining four factors (F2, F3, F5, and F6) except F4. For F4, the SA was conducted by setting the high and low alternatives to >5° and <5°, respectively, keeping other factors constant. The second SA examines the extent to which GLOF susceptibility of lakes will change when the susceptibility class (Figure 2) is modified by ±5%, i.e., ±0.05.
In 2018, a total of 345 high mountain lakes (>0.001 km2), covering a total area of 18.80 ± 1.35 km2, are distributed across the Mahalangur Himalaya (Figure 1). Among the lakes, 114 (1.38 ± 0.27 km2) are supraglacial, 113 (12.78 ± 0.68 km2) are proglacial, 87 (3.74 ± 0.31 km2) are unconnected glacier-fed, and 31 (0.89 ± 0.10 km2) are unconnected non-glacial fed lakes (Figure 5). The mean size of the glacial lakes is 0.05 km2, and the largest glacial lake in the region is the Lower Barun Tsho (1.92 ± 0.04 km2). About 83% of the total glacial lakes are below the mean size (0.05 km2); however, remaining lakes, i.e. those above the mean size constitute about 80% of the total lake area. The glacial lakes are distributed in elevation range between 3,772 and 5,592 m a.s.l. with mean elevation of 4,989 m a.s.l. The density of the glacial lakes in the study area is ∼10 glacial lake per 100 km2. The limnic ratio, the ratio of the total lake area to the total documented area where the inventory was performed, was 0.005 (Chou, 1995).
In this study, we selected 64 glacial lakes with an area ≥0.05 km2 in 2018 for GLOF susceptibility assessment. Thus, their surface area changes between 1998 and 2018 were examined (Figure 6). The results show that seven out of 64 lakes evolved after 1998, of which two were proglacial lakes, and the remaining five were supraglacial lakes. The total area of the glacial lakes in 1998 was 12.0 ± 1.6 km2, which expanded by ∼30%, reaching 15.7 ± 0.7 km2 in 2018. The three typical end-moraine proglacial lakes (Imja Tsho, Lumding Tsho and Barun Tsho) connected with their parent glaciers expanded the most in the region, contributing to ∼65% of total expansion (Figure 6, Supplementary Table S6). Besides, previous small supraglacial ponds on the surface of the glaciers grew and coalesced rapidly to form large glacial lakes that are usually dammed by the mix of ice and moraine (Figure 6B). Such characteristics may involve in developing a large proglacial lake behind the terminal moraine (Watanabe et al., 1994). Notably, most of the supraglacial lakes are dynamic, transient and often drain through the expansion of englacial conduits during summer (Benn et al., 2001; Watson et al., 2016).
FIGURE 6. Area change of glacial lakes >0.05 km2(A), the evolution of supraglacial lake (B) and expansion of proglacial lakes (C,D,E) between 1998 and 2018. Since different resolution images were used in 1998 and 2018, unchanged lakes were considered as those lakes in 1998 that did not exceed the lake area delineated in 2018 and did not reduce significantly.
The GLOF susceptibility assessment shows that out of 64 glacial lakes assessed, seven were classified with very high level of GLOF susceptibility (Table 4). Similarly, 11 glacial lakes were classified with high, 18 with medium, 24 with low and 4 with very low GLOF susceptibility (Figure 7 and Supplementary Table S7). Glacial lakes within small size class have high level of GLOF susceptibility (Figure 7B), implying that small size glacial lakes must be included in a comprehensive risk evaluation. Our method ranked notable glacial lakes like Imja Tsho as medium level and others (Lumding Tsho, Barun Tsho and South Chamlang Tsho) as very high outburst susceptibility. The details on these glacial lakes with their susceptibility classes are presented in Table 4 and Supplementary Table S7.
FIGURE 7. (A) Map showing the spatial distribution of 64 glacial lakes assessed with their GLOF susceptibility classes across the Mahalangur Himalaya. (B) The bar graph shows the GLOF susceptibility of glacial lakes in various size interval. The background is false-colour composite Landsat OLI image acquired from November 19, 2016.
Glacial lakes are indicators of climate change in the alpine environment formed due to climate-induced glacier dynamics (Otto, 2019). There are various classification schemes to classify the glacial lakes relating to glacial and non-glacial processes, their location, damming materials and structures (ICIMOD, 2011; Maharjan et al., 2018). The conventional methods for identifying glacial lakes around 10 km buffer zone from the glaciers include non-glacial fed lakes (those lakes that do not receive glacial supply); thus, studies usually use the term "high mountain or alpine lakes" and classify the types of lakes (Zheng et al., 2019). The classification of lakes helps to distinguish between glacier-fed and non-glacier fed lakes and their damming types (Bhambri et al., 2018). Non-glacier fed lakes lie in the periphery of glacial environment and are mostly fed by seasonal snowmelt and rainfall (Zhang et al., 2015; Khadka et al., 2018), while other studies classify them as unconnected glacial lakes (Salerno et al., 2016). The automatic lake delineations in the Himalaya requires substantial post corrections (Nie et al., 2017); thus, glacial lakes were consistently digitized from a single expert to explicitly produce a high-resolution inventory of glacial lakes in the study area. Comparing the delineation of 31 lakes between 10 m Sentinel-2 images and 0.5 m Pleiades imagery revealed that precise lake delineation from fine-resolution imagery yielded a slightly greater area (Supplementary Table S8). Moreover, two lakes joined by a narrow channel (strait) are visible in the fine resolution imagery featuring as a single lake, which is often misclassified as two lakes from medium to high-resolution images. The lake mapping from 10 m Sentinel-2 images yielded better results (Supplementary Table S8) with lower errors than mapped from 30 m Landsat images because the standard way of detecting an error in lake delineation (i.e., ±1/2 pixel × lake perimeter) depends upon the spatial resolution of images used (Salerno et al., 2012). A total of 103 additional lakes were mapped from high resolution Sentinel-2 images in the study area in 2018 when compared with the inventory in 2017 from Landsat imagery (Khadka et al., 2018). Furthermore, small supraglacial lakes are highly detected from Sentinel-2 imagery (Chand and Watanabe, 2019). However, the mapping depends upon the quality of image, threshold and method used. Here, the lake expansion rate was calculated utilizing different resolution images between 1998 (Landsat TM, 30 m) and 2018 (Sentinel-2, 10 m) instead of using same resolution images as the nominal differences in the expansion rates did not change the class it falls under as the current method applies three classes for lake expansion rates (Table 3).
The Himalayas have been documented as a hotspot of GLOFs and more than 50 GLOFs are recorded, among them, few are reported with details (Nie et al., 2018). The factors used in the present study might not hold for all GLOFs in the Himalaya; instead, they are focused on the study area. Moreover, the details and exact triggering factors, lake area, expansion rate and glacier-lake activity before GLOF are still difficult to be obtained for all GLOF cases. Furthermore, Nie et al. (2018) heavily rely on old documents/reports/literature to report details about GLOF, and Veh et al. (2019) has reported some unreported GLOFs without details mechanism of lake failure. Thus, it is very difficult in the current study to analyze all the factors related to previous GLOFs in the Himalayan region to select key parameters and thresholds that govern the outburst. Notably, GLOF reported with details in the Himalaya had high susceptibility score (Supplementary Table S2). We recommend for improving the methodology in future studies in other regions as GLOF mechanism, impacts and risk differs from one region to other. For instance, destructive GLOF from lateral moraine dammed Chorabari lake (∼0.05 km2) was triggered by extreme precipitation event (149 mm rainfall) in the Indian Himalaya which is very rare in our study area as precipitation analysis reveal only four extreme precipitation days (25–28 mm rainfall) during 1994–2016. In this study, we used a pre-defined lake area threshold of ≥0.045 km2 to assess GLOF susceptibility. Thus, only 19% of the lakes were considered in the study due to the size threshold. We prioritized large lakes within the highest alternative because the mean area of the lakes that previously produced GLOF was 0.4 km2 (Supplementary Table S2). This does not imply that small lakes (<0.045 km2) are not susceptible to burst. For example, 2016 GLOF from Gongbatongsha Tsho (0.017 km2) was transboundary and destructive as it destroyed infrastructures and settlements along the SINO-Nepal highway (Liu et al., 2020).
We used lake area rather than lake volume as a factor in determining the possible magnitude of a GLOF because most existing empirical formulas for volume calculation are area-dependent (Fujita et al., 2013; Sakai, 2012). The expansion rate of the lake area is also essential because the growing lake area increases the potential flood volume. Besides, the growth of the proglacial lakes such as the Imja Tsho will bring the lakes closer to areas prone to ice/rock avalanche (Linsbauer et al., 2016; Watson et al., 2020), altering the GLOF susceptibility in the future. The expansion of South Chamlang Tsho is restricted by bedrock and lateral moraines, yet it is prone to avalanches (Figure 4) (Khadka et al., 2019; Lamsal et al., 2016a). Generally, the stability of a moraine dam depends upon its geometry, geomorphology and buried ice-core (Haritashya et al., 2018). The concept of using DFSG or steep lakefront (SLA) slope to analyze the stability of moraine dam is reasonable (Fujita et al., 2013) as piping failure due to seepages and crack on dam is difficult to be perceived through remote observations (Rounce et al., 2017a). Similarly, dam crest width and lake freeboard determine the magnitude of outburst resulting from dam overtopping; in this case, DFSG alone may not represent the dam conditions. These two factors were not included in the assessment since they are challenging to analyze from remote sensing, and most GLOFs in Nepal were due to dam failure. The atmospheric trigger (such as extreme rainfall) was not considered as it is inconvenient to determine for the lakes individually. Moreover, during 2014–2016, Sharma et al. (2020a) found that the Pyramid station only recorded 15 events >10 mm/days (heavy precipitation days). Furthermore, it only recorded four R25 mm events (extreme precipitation days with >25 mm rainfall) during 1994–2016. However, atmospheric factors (heavy and extreme precipitation events) have the potentiality to trigger GLOF in two ways: directly by triggering a GLOF itself (Supplementary Figure S1) and indirectly by initiating sudden heavy snow and ice melt and triggering mass movements. This complexity is a limitation, even though the indirect trigger is captured by other factors, i.e. avalanche susceptibility used in the study. The size and topography of upstream watershed of each glacial lake determine the accumulation volume of precipitation or snowmelt that will alter the lake water level if it does not have outflow. Thus, the upstream watershed area was included in the assessment over Tibetan Plateau as a proxy to extreme precipitation events (Allen et al., 2019); however, we did not consider this factor as extreme events are low in the study area. We excluded the seismic factor which was considered by a previous study (Prakash and Nagarajan, 2017) since it is difficult to predict accurately, although the earthquake can trigger a GLOF by initiating avalanches and weakening the moraine dam. It was reported that the 2015 Nepal earthquake and its aftershock had destabilized the glacial lakes by forming new cracks and triggering landslide activities within lateral and terminal moraines (Byers et al., 2017). Nevertheless, a subsequent study in the aftermath of a devastating earthquake did not find any evidence of GLOF from 491 glacial lakes (Kargel et al., 2016). Nepal lies in a high seismic zone with the same values of peak ground acceleration from the earthquake for the study area (Rahman and Bai, 2018); thus, it will not affect the final GLOF score for individual lakes.
We used a multi-criteria-based assessment framework to prioritize glacial lakes with high and very high GLOF susceptibility. This method is straightforward, systematic, and easy to implement and repeat. It is inevitable, of course, that it has certain limitations, such as subjective expert evaluation and judgement. Additionally, the weights of each factor obtained from AHP depends upon the two-way comparison matrix and is subjective (Supplementary Table S3). However, we have maintained its compatibility and consistency ratio (Supplementary Table S5) by adding a high sampling number (n = 11) from the experts in the same field for the two-way comparison matrix (Supplementary Table S3). It should be emphasized that those glacial lakes classified as high and very high susceptibility do not necessarily indicate that they are currently in a state of outburst, but they require priority for regular monitoring and detailed ground investigation. The timing of a potential GLOF is challenging to estimate, however, some possible triggers such as snow/ice/rock avalanche and ice-cored moraine degradation will increase the risk of dam collapse (Westoby et al., 2014). The majority of recorded GLOFs in the Himalaya were produced by snow/ice/rock avalanches (Nie et al., 2018), and the probability of an avalanche or rockfall entering the lake and triggering the GLOF depends upon their volume, trajectory, velocity and positions in relation to the lake (Bolch et al., 2011; Rounce et al., 2016). For instance, the current risk of avalanches hitting into Imja Tsho is low due to the steep relief surrounding the lake is located far from the lake. In contrast, the steep hanging glaciers above Tam Pokhari glacial lake increases the susceptibility of the repeated outburst flood.
The GLOF susceptibility assessment is sensitive to change in thresholds of class/alternatives of factors. The results of the first sensitivity analysis revealed that 13–22% of the lakes (out of 64) changed their susceptibility class when the class/alternative level of F1-F3 are shifted. Similarly, 20% of lakes changed their class when high, and low class of F4 was altered to >5° and <5°, respectively keeping other factors constant. Moreover, 44% of the lakes changed their class when F5 was altered, revealing that ice/snow avalanche (F5) is the most sensitive parameter, among others. No change was observed in GLOF class of lakes when F6 was altered because almost 85% of lakes were prone to probable rockfall/landslides.
The results of the second sensitivity analysis show that if the susceptibility class (Figure 2) is decreased by 0.05 then seven high GLOF class lakes (M30, M31, M34, M45, M61, M62, M63) will be classified into very high class. Similarly, if susceptibility class is increased by 0.05, four (M20, M47, M52, M53) out of seven very high lakes (Table 4) will fall into high class. This shows that the lakes are very sensitive to the small change in GLOF class.
ICIMOD (2011) identified ten potentially dangerous glacial lakes, ranking four as first, three of each as second and third-order critical lakes highly depending upon expert judgment based on physical and socio-economic criteria. In contrast, our assessment ranked glacial lakes based on its susceptibility score. The first order Lower Barun and Lumding Tsho are classified as very high while Imja Tsho as medium GLOF susceptibility.
An assessment of GLOF hazard has been conducted across the Nepalese Himalaya to classify the hazard level of glacial lakes solely depending upon one or two parameters or a combination (Rounce et al., 2017b). For instance, glacial lakes prone to snow avalanche and with steep moraine dam were considered as very high hazardous. Glacial lakes with medium to high hazard level were considered either susceptible to avalanche/rockfall or steep moraine dam or ice-cored dam or upstream GLOF (Rounce et al., 2017b). Out of 131 glacial lakes (>0.1 km2) across the Nepalese Himalaya, 19 glacial lakes were categorized with a very high hazard (Rounce et al., 2017b). Our assessment identified high GLOF susceptible lakes of size <0.1 km2 (Figure 7B), which was excluded in previous assessment due to size threshold. Both studies have identified three common glacial lakes with a very high level of hazard (Table 4). Our study differs from their study in terms of methodology, as our method considered the collective response of different factors to classify lakes.
We used updated GAMDAM glaciers outline, considering areas >30° to know ice avalanche areas assuming that the breaking of hanging glaciers (Margreth et al., 2017) in the Himalayas can also initiate GLOFs. The uncertainty associated with DEM and GAMDAM outline affected the mass movement calculations as glacier outlines are prepared from the Landsat images of 1990–2010 (Sakai, 2019). However, since our methodology applied slope >30° to find avalanche or rockfall areas and classified into two alternatives (Table 3), the quality of the glacier outline will have no qualitative and quantitative effects on the results. Here, F5 (ice/snow avalanche) played a dominant role in the final susceptibility score because of its highest factor weight. The GLOF susceptibility classification largely depends on factors selections, quality of the data used, classification scheme, weights and scores (Bolch et al., 2011) and the alternation or adjustment of them would change the susceptibility ranking.
Any variation in the morphology of the glacial lakes (e.g., growth) can change the lake area (F1) and its expansion rate (F2), subsequently, resulting to the shift in their classes in which they fall at present. Due to such changes, GLOF susceptibility classes can vary in the future. Coupled glacier-lake interactions play a significant role in the expansion of the proglacial and the dynamics of the supraglacial lakes, while the expansion of glacial lakes promotes the retreat of glaciers (Song et al., 2017; Zhang et al., 2019). Proglacial lakes with wide calving front have high expansion rates (Haritashya et al., 2018); however, the expansion rate varies between glaciers from high to steady and diminishing rates (King et al., 2018). In the study region, the future lake expansion modeling shows that notable calving glacial lakes like Imja Tsho and Lower Barun Tsho, when expanded at current rates, would expand volumetrically to store 90 × 106 and 62 × 106 m3 of additional water by 2040 and 2060 than their estimate in 2018 (Watson et al., 2020). This expansion would increase the GLOF susceptibility in two ways: the expanding lakes may reach up to the areas prone to an avalanche, and volumetric expansion (area × depth) could increase the hydrostatic pressure to the moraine dam. Consequently, growing lake volume will increase the potential flood volume, which can cause more damage to downstream areas. In the case of Lumding Tsho, ranked as a very high GLOF susceptible level (Table 4), has the capability for future expansion when demonstrated from modelled glacier thickness (Farinotti et al., 2019) and slope derived from DEM (Figure 8). The lake, when expanded at its current rate (0.025 km2 yr−1 between 1998 and 2018) will attend the extent shown in Figure 8A by 2040s, increasing the water volume. Although its expansion rate is lower than Imja Tsho and Lower Barun Tsho (Supplementary Table S6), it needs to be monitored with a focus as given to Imja Tsho by several researchers. It is recommended that GLOF hazard assessment is needed to be improved by including the modeling of possible future lake extents and their volumetric analysis.
FIGURE 8. (A) Possible future expansion of Lumding Tsho derived from the slope (B) and modeled glacier ice thickness of its parent glacier (Farinotti et al., 2019).
This study was conducted in the Mahalangur section of the Himalaya to investigate the glacial lakes, and GLOF susceptibility as this region includes the largest concentration of glacial lakes, high elevation glaciers, and several previously recorded GLOF events. A high-resolution inventory of glacial lakes from Sentinel-2 images shows 345 lakes with a total area of 18.80 ± 1.35 km2 in 2018 across the study area. The multi-criteria decision-based analytical hierarchy process was used to evaluate the degree of GLOF susceptibility from glacial lakes in the region discussing the several factors that regulate the probability of an outburst. With the eminent GLOF events from small size glacial lakes (<0.1 km2), the size threshold was reduced to 0.05 km2, and a GLOF susceptibility assessment was conducted with the basis of six factors and detail characteristics of three past GLOF events in the study area. The results show that out of 64 glacial lakes (≥0.05 km2) in the region, seven were assessed to have very high outburst susceptibility while the remaining ranged from high to very low, providing more detail insights into GLOF susceptibility in the region. The very high GLOF susceptible lakes must be prioritized for detail investigation and studies. The methodology is replicable for basin level or regional level studies and can be improved with the detailed characteristics of past GLOF and associated parameters. The results are significant to the decision-makers and scientists as it has implications for integrated disaster risk reduction. This study emphasizes that the GLOF susceptibility increases as glacier-lake interaction becomes pronounced, adding to the risk of GLOFs. Furthermore, the application of high-resolution data, with robust methodologies combined with in-situ studies, will help in improving GLOF susceptibility assessment.
Publicly available datasets were analyzed in this study. This data can be found here: Landsat images were obtained from the U.S. Geological Survey (USGS) data portal (http://earthexplorer.usgs.gov) and Sentinel-2 images were downloaded from the Copernicus open access hub of the European Space Agency (https://scihub.copernicus.eu/).
Conceptualization: NK, XC and ST; Data Curation: NK, ST and GXZ; Methodology, analysis, and original draft: NK; Writing, Reviewing, and editing: XC, YN, ST, GXZ, GZ, NK; Supervision, project administration and funding: XC, YN and ST.
This study was financially supported by the International Partnership Program of Chinese Academy of Sciences (Grant No. 131551KYSB20180042) and the Natural Science Foundation of China (21661132003). NK is supported by the Chinese Academy of Science and The World Academy of Science (CAS-TWAS) President's Fellowship. XC is supported by The National Science Fund for Distinguished Young Scholars. ST acknowledges the research support under the Tribhuvan University (TU)- HERP DLI-7B.
Frontiers Media SA remains neutral with regard to jurisdictional claims in published maps and institutional affiliations.
The authors declare that the research was conducted in the absence of any commercial or financial relationships that could be construed as a potential conflict of interest.
Authors would like to acknowledge the experts from different countries for conducting the comparison matrix. NASA and ESA are acknowledged for producing freely available satellite images. The Ev-K2-CNR Association (Pyramid station) is also acknowledged for providing the rain-gauge precipitation datasets. PLEIADES images were received under the Specific Cooperation agreement between CNES and TU for the cryospheric study. NK acknowledges Shankar Sharma for technical help.
The Supplementary Material for this article can be found online at: https://www.frontiersin.org/articles/10.3389/feart.2020.601288/full#supplementary-material.
Aggarwal, S., Rai, S. C., Thakur, P. K., and Emmer, A. (2017). Inventory and recently increasing GLOF susceptibility of glacial lakes in Sikkim, Eastern Himalaya. Geomorphology. 295, 39–54. doi:10.1016/j.geomorph.2017.06.014
Allen, S. K., Zhang, G., Wang, W., Yao, T., and Bolch, T. (2019). Potentially dangerous glacial lakes across the Tibetan Plateau revealed using a large-scale automated assessment approach. Science Bulletin. 64, 435–445. doi:10.1016/j.scib.2019.03.011
Bajracharya, S. R., and Mool, P. (2009). Glaciers, glacial lakes and glacial lake outburst floods in the Mount Everest region, Nepal. Ann. Glaciol. 50, 81–86. doi:10.3189/172756410790595895
Benn, D. I., Wiseman, S., and Hands, K. A. (2001). Growth and drainage of supraglacial lakes on debris-mantled Ngozumpa Glacier, Khumbu Himal, Nepal. J. Glaciol. 47, 626–638. doi:10.3189/172756501781831729
Bhambri, R., Misra, A., Kumar, A., Gupta, A. K., Verma, A., and Tiwari, S. K. (2018). Glacier lake inventory of Himachal Pradesh.Himal. Geol. 39, 1–32.|
Bolch, T., Peters, J., Yegorov, A., Pradhan, B., Buchroithner, M., and Blagoveshchensky, V. (2011). Identification of potentially dangerous glacial lakes in the northern Tien Shan. Nat. Hazards. 59, 1691–1714. doi:10.1007/s11069-011-9860-2
Byers, A.C., Byers, E.A., McKinney, D.C., and Rounce, D.R. (2017). A field-based study of impacts of the 2015 earthquake on potentially dangerous Glacial Lakes in Nepal. J. Assoc. Nepal Himal. Studies. 37 (2), 7. |
Byers, A. C., McKinney, D. C., Somos-Valenzuela, M., Watanabe, T., and Lamsal, D. (2013). Glacial lakes of the hinku and hongu valleys, makalu Barun national park and buffer zone, Nepal. Nat. Hazards. 69, 115–139. doi:10.1007/s11069-013-0689-8
Byers, A. C., Rounce, D. R., Shugar, D. H., Lala, J. M., Byers, E. A., and Regmi, D. (2018). A rockfall-induced glacial lake outburst flood, Upper Barun Valley, Nepal. Landslides, 1–17.
Carrivick, J. L., and Tweed, F. S. (2016). A global assessment of the societal impacts of glacier outburst floods. Global and Planetary Change. 144, 1–16. doi:10.1016/j.gloplacha.2016.07.001
Chand, M. B., and Watanabe, T. (2019). Development of supraglacial ponds in the everest region, Nepal, between 1989 and 2018. Remote Sensing. 11, 1058. doi:10.3390/rs11091058
Chou, L. (1995). Physics and chemistry of lakes. in The lakes handbook, Lake Restoration and Rehabilitation. E. B. P. O'Sullivan, and C. S. Reynolds (Berlin: Springer-Verlag).
Das, S., Kar, N. S., and Bandyopadhyay, S. (2015). Glacial lake outburst flood at Kedarnath, Indian Himalaya: a study using digital elevation models and satellite images. Nat. Hazards. 77, 769–786. doi:10.1007/s11069-015-1629-6
Dwivedi, S., Acharya, M., and Simard, R. (2000). The Tam Pokhari glacier lake outburst flood of 3 September 1998. J. Nepal Geol. Soc. 22, 539–546.
Emmer, A., and Vilímek, V. (2013). Review Article: Lake and breach hazard assessment for moraine-dammed lakes: an example from the Cordillera Blanca (Peru). Nat. Hazards Earth Syst. Sci. 13, 1551. doi:10.5194/nhess-13-1551-2013
Emmer, A., and Vilímek, V. (2014). New method for assessing the susceptibility of glacial lakes to outburst floods in the Cordillera Blanca, Peru. Hydrol. Earth Syst. Sci. 18, 3461–3479. doi:10.5194/hess-18-3461-2014
Farinotti, D., Huss, M., Fürst, J. J., Landmann, J., Machguth, H., Maussion, F., et al. (2019). A consensus estimate for the ice thickness distribution of all glaciers on Earth. Nat. Geosci. 12, 168–173. doi:10.1038/s41561-019-0300-3
Fujita, K., Sakai, A., Takenaka, S., Nuimura, T., Surazakov, A. B., Sawagaki, T., et al. (2013). Potential flood volume of Himalayan glacial lakes. Nat. Hazards Earth Syst. Sci. 13, 1827. doi:10.5194/nhess-13-1827-2013
Hamal, K., Sharma, S., Baniya, B., Khadka, N., and Zhou, X. (2020). Inter-annual variability of winter precipitation over Nepal coupled with ocean-atmospheric patterns during 1987–2015. Front. Earth Sci. 8, 161. doi:10.3389/feart.2020.00161
Haritashya, U., Kargel, J., Shugar, D., Leonard, G., Strattman, K., Watson, C., et al. (2018). Evolution and controls of large glacial lakes in the Nepal Himalaya. Remote Sensing. 10, 798. doi:10.3390/rs10050798
Harrison, S., Kargel, J. S., Huggel, C., Reynolds, J., Shugar, D. H., Betts, R. A., et al. (2018). Climate change and the global pattern of moraine-dammed glacial lake outburst floods. The Cryosphere. 12, 1195. doi:10.5194/tc-12-1195-2018
Huggel, C., Haeberli, W., Kääb, A., Bieri, D., and Richardson, S. (2004). An assessment procedure for glacial hazards in the Swiss Alps. Can. Geotech. J. 41, 1068–1083. doi:10.1139/t04-053
ICIMOD (2011). Glacial lakes and glacial lake outbursts floods in Nepal kathmandu. Kathmandu: International Centre for Integrated Mountain Development (ICIMOD).
Kargel, J., Leonard, G., Shugar, D.H., Haritashya, U., Bevington, A., Fielding, E., et al. (2016). Geomorphic and geologic controls of geohazards induced by Nepal's 2015 Gorkha earthquake. Science. 351 (6269).
Karki, R., Talchabhadel, R., Aalto, J., and Baidya, S. K. (2016). New climatic classification of Nepal. Theor. Appl. Climatol. 125, 799–808. doi:10.1007/s00704-015-1549-0
Khadka, N., Zhang, G., and Thakuri, S. (2018). Glacial Lakes in the Nepal Himalaya: Inventory and Decadal Dynamics (1977-2017). Remote Sensing. 10, 1913. doi:10.3390/rs10121913
Khadka, N., Zhang, G., and Chen, W. (2019). The state of six dangerous glacial lakes in the Nepalese Himalaya. Terr. Atmos. Ocean. Sci. 30, 63–72. doi:10.3319/tao.2018.09.28.03
King, O., Quincey, D. J., Carrivick, J. L., and Rowan, A. V. (2017). Spatial variability in mass loss of glaciers in the Everest region, central Himalayas, between 2000 and 2015. The Cryosphere. 11, 407–426. doi:10.5194/tc-11-407-2017
King, O., Dehecq, A., Quincey, D., and Carrivick, J. (2018). Contrasting geometric and dynamic evolution of lake and land-terminating glaciers in the central Himalaya. Global and planetary change. 167, 46–60. doi:10.1016/j.gloplacha.2018.05.006
Lamsal, D., Sawagaki, T., Watanabe, T., and Byers, A. C. (2016a). Assessment of glacial lake development and prospects of outburst susceptibility: Chamlang South Glacier, eastern Nepal Himalaya. Geomatics, Natural Hazards and Risk. 7, 403–423. doi:10.1080/19475705.2014.931306
Lamsal, D., Sawagaki, T., Watanabe, T., Byers, A. C., and McKinney, D. C. (2016b). An assessment of conditions before and after the 1998 Tam Pokhari outburst in the Nepal Himalaya and an evaluation of the future outburst hazard. Hydrol. Process. 30, 676–691. doi:10.1002/hyp.10636
Linsbauer, A., Frey, H., Haeberli, W., Machguth, H., Azam, M. F., and Allen, S. (2016). Modelling glacier-bed overdeepenings and possible future lakes for the glaciers in the Himalaya-Karakoram region. Ann. Glaciol. 57, 119–130. doi:10.3189/2016aog71a627
Liu, J.-J., Cheng, Z.-L., and Li, Y. (2014). The 1988 glacial lake outburst flood in Guangxieco Lake, Tibet, China. Nat. Hazards Earth Syst. Sci. 14, 3065. doi:10.5194/nhess-14-3065-2014
Liu, M., Chen, N., Zhang, Y., and Deng, M. (2020). Glacial lake inventory and lake outburst flood/debris flow hazard assessment after the gorkha earthquake in the bhote Koshi basin. Water. 12, 464. doi:10.3390/w12020464
Maharjan, S. B., Mool, P. K., Lizong, W., Xiao, G., Shrestha, F., Shrestha, R. B., et al. (2018). The status of glacial lakes in the Hindu Kush Himalaya. Kathmandu: International Centre for Integrated Mountain Development (ICIMOD).
Margreth, S., Funk, M., Tobler, D., Dalban, P., Meier, L., and Lauper, J. (2017). Analysis of the hazard caused by ice avalanches from the hanging glacier on the Eiger west face. Cold Regions Science and Technology. 144, 63–72. doi:10.1016/j.coldregions.2017.05.012
McKillop, R. J., and Clague, J. J. (2007). Statistical, remote sensing-based approach for estimating the probability of catastrophic drainage from moraine-dammed lakes in southwestern British Columbia. Global and Planetary Change. 56, 153–171. doi:10.1016/j.gloplacha.2006.07.004
Mertes, J. R., Thompson, S. S., Booth, A. D., Gulley, J. D., and Benn, D. I. (2017). A conceptual model of supra-glacial lake formation on debris-covered glaciers based on GPR facies analysis. Earth Surf. Process. Landforms. 42, 903–914. doi:10.1002/esp.4068
Nie, Y, Liu, Q, and Liu, S (2013). Glacial lake expansion in the central Himalayas by Landsat images, 1990-2010. PloS One. 8, e83973. doi:10.1371/journal.pone.0083973 |
Nie, Y., Sheng, Y., Liu, Q., Liu, L., Liu, S., Zhang, Y., et al. (2017). A regional-scale assessment of Himalayan glacial lake changes using satellite observations from 1990 to 2015. Remote Sensing of Environment. 189, 1–13. doi:10.1016/j.rse.2016.11.008
Nie, Y., Liu, Q., Wang, J., Zhang, Y., Sheng, Y., and Liu, S. (2018). An inventory of historical glacial lake outburst floods in the Himalayas based on remote sensing observations and geomorphological analysis. Geomorphology. 308, 91–106. doi:10.1016/j.geomorph.2018.02.002
Osti, R., and Egashira, S. (2009). Hydrodynamic characteristics of the Tam Pokhari glacial lake outburst flood in the Mt. Everest region, Nepal. Hydrol. Process. 23, 2943–2955. doi:10.1002/hyp.7405
Otto, J.-C. (2019). “Proglacial lakes in high mountain environments,” in Geomorphology of proglacial systems. New York: Springer.
Prakash, C., and Nagarajan, R. (2017). Outburst susceptibility assessment of moraine‐dammed lakes in Western Himalaya using an analytic hierarchy process. Earth surface processes and landforms. 42, 2306–2321. doi:10.1002/esp.4185
Rafiq, M., Romshoo, S. A., Mishra, A. K., and Jalal, F. (2019). Modelling Chorabari lake outburst flood, kedarnath, India. J. Mt. Sci. 16, 64–76. doi:10.1007/s11629-018-4972-8
Rahman, M. M., and Bai, L. (2018). Probabilistic seismic hazard assessment of Nepal using multiple seismic source models. Earth Plan. Phy. 2, 327–341. |
Richardson, S. D., and Reynolds, J. M. (2000). An overview of glacial hazards in the Himalayas. Quaternary International. 65–66, 31–47. doi:10.1016/s1040-6182(99)00035-x |
Rounce, D., and McKinney, D. C. (2014). Debris thickness of glaciers in the Everest area (Nepal Himalaya) derived from satellite imagery using a nonlinear energy balance model. Cryosphere. 8, 1317–1329. doi:10.5194/tc-8-1317-2014
Rounce, D. R., McKinney, D. C., Lala, J. M., Byers, A. C., and Watson, C. S. (2016). A new remote hazard and risk assessment framework for glacial lakes in the Nepal Himalaya. Hydrol. Earth Syst. Sci. 20, 3455. doi:10.5194/hess-20-3455-2016
Rounce, D. R., Byers, A. C., Byers, E. A., and McKinney, D. C. (2017a). Brief communication: observations of a glacier outburst flood from Lhotse Glacier, Everest area, Nepal. The Cryosphere. 11, 443–449. doi:10.5194/tc-11-443-2017
Rounce, D., Watson, C., and McKinney, D. (2017b). Identification of Hazard and Risk for Glacial Lakes in the Nepal Himalaya Using Satellite Imagery from 2000-2015. Remote Sensing. 9, 654. doi:10.3390/rs9070654
Saaty, T. L., and Vargas, L. G. (2012). Models, methods, concepts & applications of the analytic hierarchy process. New York: Springer Science & Business Media.
Saaty, T. L. (1990). Multicriteria decision making: the analytic hierarchy process: planning, priority setting resource allocation, 287 pp, 2nd ed., RWS Publications, Pittsburgh, Pa. USA.
Sakai, A. (2012). Glacial lakes in the Himalayas: a review on formation and expansion processes. Global Environ. Res. 16, 23–30.
Sakai, A. (2019). Brief communication: updated GAMDAM glacier inventory over high-mountain Asia. The Cryosphere. 13, 2043–2049. doi:10.5194/tc-13-2043-2019
Salerno, F., Thakuri, S., D’Agata, C., Smiraglia, C., Manfredi, E. C., Viviano, G., et al. (2012). Glacial lake distribution in the Mount Everest region: uncertainty of measurement and conditions of formation. Global and Planetary Change. 92–93, 30–39. doi:10.1016/j.gloplacha.2012.04.001 |
Salerno, F., Guyennon, N., Thakuri, S., Viviano, G., Romano, E., Vuillermoz, E., et al. (2015). Weak precipitation, warm winters and springs impact glaciers of south slopes of Mt. Everest (central Himalaya) in the last 2 decades (1994-2013). The Cryosphere. 9, 1229–1247. doi:10.5194/tc-9-1229-2015
Salerno, F., Thakuri, S., Guyennon, N., Viviano, G., and Tartari, G. (2016). Glacier melting and precipitation trends detected by surface area changes in Himalayan ponds. The Cryosphere. 10, 1433–1448. doi:10.5194/tc-10-1433-2016
Saltelli, A., Tarantola, S., and Chan, K. (1999). A role for sensitivity analysis in presenting the results from MCDA studies to decision makers. J. Multi-Crit. Decis. Anal. 8, 139–145. doi:10.1002/(sici)1099-1360(199905)8:3<139::aid-mcda239>3.0.co;2-c
Sharma, S., Chen, Y., Zhou, X., Yang, K., Li, X., Niu, X., et al. (2020a). Evaluation of GPM-era satellite precipitation products on the southern slopes of the central Himalayas against rain gauge data. Remote Sensing. 12, 1836. doi:10.3390/rs12111836
Sharma, S., Khadka, N., Hamal, K., Baniya, B., Luintel, N., and Joshi, B. B. (2020b). Spatial and temporal analysis of precipitation and its extremities in seven provinces of Nepal (2001-2016). Appl. Ecol. Environ. Sci. 8, 64–73. doi:10.12691/aees-8-2-4
Somos-Valenzuela, M. A., McKinney, D. C., Byers, A. C., Rounce, D. R., Portocarrero, C., and Lamsal, D. (2015). Assessing downstream flood impacts due to a potential GLOF from Imja Tsho in Nepal. Hydrol. Earth Syst. Sci. 19, 1401–1412. doi:10.5194/hess-19-1401-2015
Song, C., Sheng, Y., Wang, J., Ke, L., Madson, A., and Nie, Y. (2017). Heterogeneous glacial lake changes and links of lake expansions to the rapid thinning of adjacent glacier termini in the Himalayas. Geomorphology. 280, 30–38. doi:10.1016/j.geomorph.2016.12.002
Thakuri, S., Salerno, F., Smiraglia, C., Bolch, T., D'Agata, C., Viviano, G., et al. (2014). Tracing glacier changes since the 1960s on the south slope of Mt. Everest (central Southern Himalaya) using optical satellite imagery. The Cryosphere. 8, 1297–1315. doi:10.5194/tc-8-1297-2014
Veh, G., Korup, O., von Specht, S., Roessner, S., and Walz, A. (2019). Unchanged frequency of moraine-dammed glacial lake outburst floods in the Himalaya. Nat. Clim. Change. 9, 379. doi:10.1038/s41558-019-0437-5
Vuichard, D., and Zimmermann, M. (1987). The 1985 catastrophic drainage of a moraine-dammed lake, Khumbu Himal, Nepal: cause and consequences. Mountain Res. Develop. 9, 91–110. doi:10.2307/3673305
Wang, W., Yao, T., Gao, Y., Yang, X., and Kattel, D. B. (2011). A first-order method to identify potentially dangerous glacial lakes in a region of the southeastern Tibetan Plateau. Mountain Res. Develop. 31, 122–130. doi:10.1659/mrd-journal-d-10-00059.1
Wang, S., Qin, D., and Xiao, C. (2015). Moraine-dammed lake distribution and outburst flood risk in the Chinese Himalaya. J. Glaciol. 61, 115–126. doi:10.3189/2015JoG14J097
Watanabe, T., Ives, J. D., and Hammond, J. E. (1994). Rapid growth of a glacial lake in Khumbu Himal, Himalaya: prospects for a catastrophic flood. Mountain Res. Develop. 14, 329–340. doi:10.2307/3673729
Watson, C. S., Quincey, D. J., Carrivick, J. L., and Smith, M. W. (2016). The dynamics of supraglacial ponds in the Everest region, central Himalaya. Global and Planetary Change. 142, 14–27. doi:10.1016/j.gloplacha.2016.04.008
Watson, C. S., Kargel, J. S., Shugar, D. H., Haritashya, U. K., Schiassi, E., and Furfaro, R. (2020). Mass loss from calving in Himalayan proglacial lakes. Front. Earth Sci. 7, 342. doi:10.3389/feart.2019.00342
Westoby, M. J., Glasser, N. F., Brasington, J., Hambrey, M. J., Quincey, D. J., and Reynolds, J. M. (2014). Modelling outburst floods from moraine-dammed glacial lakes. Earth-Science Reviews. 134, 137–159. doi:10.1016/j.earscirev.2014.03.009
Worni, R, Huggel, C, and Stoffel, M (2013). Glacial lakes in the Indian Himalayas--from an area-wide glacial lake inventory to on-site and modeling based risk assessment of critical glacial lakes. Sci. Total Environ. 468–469, S71–S84. doi:10.1016/j.scitotenv.2012.11.043 |
Zhang, G., Yao, T., Xie, H., Wang, W., and Yang, W. (2015). An inventory of glacial lakes in the Third Pole region and their changes in response to global warming. Global and Planetary Change. 131, 148–157. doi:10.1016/j.gloplacha.2015.05.013
Zhang, G., Bolch, T., Allen, S., Linsbauer, A., Chen, W., and Wang, W. (2019). Glacial lake evolution and glacier-lake interactions in the Poiqu River basin, central Himalaya, 1964-2017. J. Glaciol. 65, 347–365. doi:10.1017/jog.2019.13
Keywords: glacial lake outburst flood, susceptibility, analytical hierarchy process, Sentinel-2, Mahalangur Himalaya
Citation: Khadka N, Chen X, Nie Y, Thakuri S, Zheng G and Zhang G (2021) Evaluation of Glacial Lake Outburst Flood Susceptibility Using Multi-Criteria Assessment Framework in Mahalangur Himalaya. Front. Earth Sci. 8:601288. doi: 10.3389/feart.2020.601288
Received: 31 August 2020; Accepted: 28 December 2020;
Published: 29 January 2021.
Edited by:
Adam Emmer, University of Graz, AustriaReviewed by:
Georg Veh, University of Potsdam, GermanyCopyright © 2021 Khadka, Chen, Nie, Thakuri, Zheng and Zhang. This is an open-access article distributed under the terms of the Creative Commons Attribution License (CC BY). The use, distribution or reproduction in other forums is permitted, provided the original author(s) and the copyright owner(s) are credited and that the original publication in this journal is cited, in accordance with accepted academic practice. No use, distribution or reproduction is permitted which does not comply with these terms.
*Correspondence: Xioaqing Chen, eHFjaGVuQGltZGUuYWMuY24=
Disclaimer: All claims expressed in this article are solely those of the authors and do not necessarily represent those of their affiliated organizations, or those of the publisher, the editors and the reviewers. Any product that may be evaluated in this article or claim that may be made by its manufacturer is not guaranteed or endorsed by the publisher.
Research integrity at Frontiers
Learn more about the work of our research integrity team to safeguard the quality of each article we publish.