- 1Center for Climate Physics, Institute for Basic Science, Busan, South Korea
- 2Pusan National University, Busan, South Korea
- 3Instituto de Física Interdisciplinary Sistemas Complejos, CSIC-UIB, Palma de Mallorca, Spain
- 4MTA–ELTE Theoretical Physics Research Group, Eötvös University, Budapest, Hungary
- 5Institute for Theoretical Physics, Eötvös University, Budapest, Hungary
- 6BK21 School of Earth and Environmental System, Pusan National University, Busan, South Korea
- 7Research Center for Climate Sciences, Pusan National University, Busan, South Korea
We study the forced response of the teleconnection between the El Niño–Southern Oscillation (ENSO) and the Indian summer monsoon (IM) in the Max Planck Institute Grand Ensemble, a set of Earth system ensemble simulations under historical and Representative Concentration Pathway (RCP) forcing. The forced response of the teleconnection, or a characteristic of it, is defined as the time dependence of a correlation coefficient evaluated over the ensemble. We consider the temporal variability of spatial averages and that with respect to dominant spatial modes in the sense of Maximal Covariance Analysis, Canonical Correlation Analysis and Empirical Orthogonal Function analysis across the ensemble. A further representation of the teleconnection that we define here takes the point of view of the predictability of the spatiotemporal variability of the Indian summer monsoon. We find that the strengthening of the ENSO-IM teleconnection is robustly or consistently featured in view of various teleconnection representations, whether sea surface temperature (SST) or sea level pressure (SLP) is used to characterize ENSO, and both in the historical period and under the RCP8.5 forcing scenario. It is found to be associated dominantly with the principal mode of ENSO variability. Concerning representations that involve an autonomous characterisation of the Pacific, in terms of a linear regression model, the main contributor to the strengthening is the regression coefficient, which can outcompete even a declining ENSO variability when it is represented by SLP. We also find that the forced change of the teleconnection is typically nonlinear by 1) formally rejecting the hypothesis that ergodicity holds, i.e., that expected values of temporal correlation coefficients with respect to the ensemble equal the ensemble-wise correlation coefficient itself, and also showing that 2) the trivial contributions of the forced changes in means and standard deviations are insignificant here. We also provide, in terms of the test statistics, global maps of the degree of nonlinearity/nonergodicity of the forced change of the teleconnection between local precipitation and ENSO.
1 Introduction
ENSO teleconnections are widely studied, but their changes resulting from external forcing, such as an increasing concentration of greenhouse gases, remain to be further explored and understood. Power and Delage (2018) provide a multi-model assessment of ENSO-precipitation teleconnection changes based on the fifth phase of the Coupled Model Intercomparison Project (CMIP5) archive. They consider, in particular, ENSO-driven precipitation anomalies in tropical regions around the globe, and assess them jointly with changes of mean precipitation. Haszpra et al. (2020a) have evaluated only the trend in the strength of ENSO-precipitation teleconnection, however, not in a multimodel ensemble but the so-called “single model initial condition large ensemble” (SMILE) CESM1-LE (Kay et al., 2015). Working with a SMILE has the advantages that the response to forcing is correctly represented in that model at least (Bódai and Tél, 2012; Drótos et al., 2015; Tél et al., 2019), and seeking a physical interpretation of changes is not faced with confusion at the outset, even if the physics depicted in that model is inaccurate or unrealistic.
The forced response is the time evolution of some ensemble-wise statistics, or, most generically, that of the probability measure carried by the climate snapshot attractor (Bódai and Tél, 2012; Drótos et al., 2015; Tél et al., 2019). The ensemble-wise statistics evaluated over the converged ensemble (Drótos et al., 2017) is in a one-to-one correspondence with the external forcing of the nonautonomous dynamical system, hence the term ‘forced response.’ For specific observables, this translates to the time evolution of any statistics evaluated with respect to an ensemble, e.g., that of mean values, standard deviations, correlation coefficients, empirical orthogonal functions (EOFs). Note that quantifiers of internal variability exhibit a forced response just as well as quantifiers of the “mean state.” The ensemble must have converged to the time-evolving snapshot attractor (Drótos et al., 2017); in the terminology of climate research, this means the correct representation of the full ensemble spread along with the absence of any drift. External forcing is defined as an explicit dependence on time of any parameter of the system (Ghil and Lucarini, 2020), which in historical and RCP runs corresponds to changes in the atmospheric composition (including greenhouse gases, anthropogenic aerosols and volcanic aerosols), land use, and solar activity (Meinshausen et al., 2011).
Without convergence, or considering a single realization, which may even correspond to observations, the state depends also on initial conditions, so that forced changes cannot be precisely disentangled from changes brought about by internal variability. In an attempt of doing this nevertheless, concerning a single realization, the standard practice is that a trend, linear or not (Franzke, 2014), is simply identified as a forced change, before possibly “anomalies,” i.e., (what is assumed to be) the internal variability is analyzed. Identifying the principal component (PC) of the leading or first empirical orthogonal function (EOF1) (Storch and Zwiers, 1999) obtained without detrending with the forced response (Kim and Ha, 2015; Pandey et al., 2020) is still somewhat arbitrary. It certainly leads to biases (Drótos et al., 2016). Yet, even with SMILEs available, it is very common to see that authors evaluate temporal statistics first–unnecessarily involving a subjective factor—and ensemble statistics afterward (Vega-Westhoff and Sriver, 2017; Carréric et al., 2020). The biases of all these approaches are certainly controlled by the magnitude of the climate change signal relative to the intensity of internal variability—a kind of signal-to-noise ratio. Computing the PC1 of EOF1 without detrending, as mentioned just above, is in fact meant to maximize the signal at least, and the EOF1 is referred to (Santer et al., 2019) as a “fingerprint” of the forced change—the spatial pattern that is supposed to be distorted by internal variability, in any single realization, the least. See (Timmermann, 1999) which is concerned rather with the minimization of the “noise.”
It is not pertinent to talk about “advantages” of temporal or ensemble methods over one another, because no situation arises when we need to decide between them. When an ensemble is available, the correct, conceptually sound ensemble method is to be applied if the forced response (including that of internal variability) is concerned. However, certainly we can make statements only about the given model.1 Therefore, we should speak only about limitations in the respective situations of analyzing observational or modeled ensemble data.
We should note that the finite-size estimators of some statistics of basic interest are not generally unbiased—an example to appear in this work—and there might not be a universally applicable correction to the estimator to make it unbiased [unlike, e.g., the fortunate cases of the central moments (Heffernan, 1997)]. Therefore, even an ensemble-wise approach could suffer from biases in practice with the ensemble size being always finite. Some extreme value statistics are likely other examples.
Concerning teleconnections, in particular, the forced response can be identified as the time evolution of the ensemble-wise Pearson correlation coefficient (Herein et al., 2016; Yettella et al., 2018) (in a simple linear approach) between some quantities representing anomalies. The anomaly can correspond to a simple spatial (mean of a temporal) mean (Bódai et al., 2020b), but also the PC of an EOF concerning variability across the ensemble, called a snapshot EOF (SEOF) (Haszpra et al., 2020c). Haszpra et al. (2020a) take the latter approach; however, this can be extended to obtaining anomalies observing the “mutual variability,” e.g., in the sense of Maximal Covariance Analysis (MCA) (Storch and Zwiers, 1999) or Canonical Correlation Analysis (CCA) (Storch and Zwiers, 1999; Härdle and Simar, 2007). That is, with an interest in a teleconnection and its forced response, MCA and CCA—just like EOF analysis—can also be pursued concerning the variability across the ensemble, whereby we can refer to these methods as SMCA and SCCA. This is perhaps best suited to teleconnection analyses concerning two extended, possibly disconnected, regions. See an application of SMCA to studying the forced change of the coupling of JJA 200 hPa geopotential height and September sea ice concentration in (Haszpra et al., 2020b; Topál et al., 2020).
An other important example would be the relationship of the summer monsoon precipitation on the Indian subcontinent with the ENSO phenomenon which extends over the whole of the Equatorial Pacific (Wang, 2006; Mishra et al., 2012; Wang et al., 2015). In a previous publication (Bódai et al., 2020b) we examined the forced response of the ENSO-Indian monsoon teleconnection in the Max Planck Institute Grand Ensemble (MPI-GE) (Maher et al., 2019) representing the monsoon by the average JJAS precipitation over India and the ENSO by either the gridpoint- and SLP-based SOI (Southern Oscillation Index), or the areal mean of the SST in some extended area in the Equatorial Pacific. Standard choices for the latter are the Niño3, Niño4, and Niño3.4 regions. For the first time we established, via a formal hypothesis test, that standard representations of the teleconnection2 strengthened in this model, both in the historical period and under the high-emission RCP8.5 forcing scenario, although not necessarily monotonically. In fact, using the SOI it was the historical period only when an increase could be detected, and, using the Niño3 index, it was rather the RCP8.5 scenario under which an increase could be detected. This raises the question whether the discrepancy is down more to 1) the physical difference between the SOI and Niño3 indices, or 2) rather that–one based on two distant gridpoints while the other on a limited region of the Equatorial Pacific–they “take somewhat different slices” of the whole ENSO phenomenon. This question is especially relevant when spatial characteristics of ENSO may also have a forced response besides the amplitude. In fact, using the Niño3.4 index, instead of Niño3, the result is closer to that using the SOI or the so-called box-SOI. With all these representations, one is able to detect the same nonmonotonicity: a temporary weakening around the turn of the millennium. In addition, both with Niño3.4 and the box-SOI changes are detected both under the historical and scenario forcing. On the one hand (I), this seems to rule out that using SST versus SLP makes much difference. We will see below that this is actually not precisely the case. On the other hand (II), it is still a question whether spatial characteristics of ENSO (in terms of SEOFs) or the whole teleconnection (in terms of SMCA or SCCA) would also change, or only the variance as reflected in the magnitudes of the PCs belonging to either of the said instantaneous (snapshot) spatial modes. These are the two basic questions that we set out here to investigate.
Furthermore, this study identifies, in terms of a regression model, three controlling factors driving the forced change of the ENSO-IM teleconnection strength. These are the ENSO variability, the coupling (i.e., the regression coefficient), and the intensity of other influences—which include but are not restricted to internal influences from the IM region and can be viewed as noise. We find that the changes of the ENSO-IM teleconnection are not driven only or dominantly by the change of ENSO variability. Nevertheless, it imprints its nonlinearity onto the teleconnection change, which might be an important source of biases (besides using a biased estimator). In particular, any nonlinearity prompts that the system should be nonergodic3 with respect to correlations, i.e., biases should exist (Drótos et al., 2016) in the temporal correlation coefficient evaluated in, say, multi-decadal time windows. Such a bias is something to bear in mind besides the ample statistical fluctuations of finite-size statistics even under a stationary climate (Bódai et al., 2020b). Nonlinearity is implied in our case by a nonmonotonic change in the ENSO variability: after a seemingly monotonic change, a decline follows in the second half of the 21st c. under RCP8.5.4 Given that the ensemble size is finite and not so large from the point of view of teleconnections, we develop here a statistical test whereby we can detect nonergodicity, and, subsequently, map out regions of the world where such a nonergodicity can be detected in the MPI-GE in the context of the relationship of local precipitation with ENSO.
The rest of the paper is organized as follows. In Section 2 we provide details about our methodology of analyzing the forced response of teleconnections, such as the way we pursue EOF, MCA, CCA, the definitions of a host of teleconnection representations, as well as the idea of decomposing the change of the teleconnection strength in terms of a simple regression model. In Section 3 we provide results both on spatial aspects of the forced change of the ENSO-IM teleconnections and those concerning magnitudes. Section 4 provides a comparison of the MPI-ESM with observational and reanalysis data. In Section 5 we outline our method of detecting nonergodicity and map out the world with respect to the degree of nonergodicity concerning the synoptic relationship of the local JJA precipitation with ENSO. In Section 6 we discuss and summarize our results.
2 Data and Methods
2.1 Data
The analysis is restricted to the historical and RCP8.5 simulations of the Max Planck Institute Grand Ensemble (MPI-GE, Maher et al., 2019), which is a collection of initial-condition ensemble simulations of the Max Planck Institute Earth System Model (MPI-ESM) under various forcing. The MPI-ESM is a fully coupled Earth system model, and its version 1.1.00p2, between phases 5 and 6 of the CMIP, was used to generate the MPI-GE from initial conditions that sample a long pre-industrial control run. See Maher et al. (2019) for further details. We make use of the same 63 of the 100 ensemble members as done in (Bódai et al., 2020b), due to concerns that the discarded members have not converged to the climate attractor of the model (Drótos et al., 2017).
To characterize the ENSO we make use of the 2D fields of either the Sea Level Pressure (SLP) or the Sea Surface Temperature (SST). The Indian Summer Monsoon Rain (ISMR) was calculated from the total (convective and large-scale) precipitation rate. The variable codes for SLP, SST and total precipitation rate are 134, 12 and 4, respectively. Monthly mean SLP is accessible publicly at https://esgf-data.dkrz.de/projects/mpi-ge/
under variable name “psl.” The SST can be derived from the top layer of the 3D potential temperature field, whose variable is “thetao.” Alternatively, one can use the surface air temperature variable, as e.g., the monthly mean approximates the SST very well over the ocean. Instead of the total precipitation rate [km/s], one can use the precipitation flux [kg/s/m2], whose variable name is “pr.”
2.2 Representations of the ENSO-IM Teleconnection
The scalar quantity of the ISMR, averaged over JJAS and over India, is the same as that calculated in (Bódai et al., 2020b), in order to secure a correspondence with the so-called AISMR rain gauge data set (Parthasarathy et al., 1994) (excluding some states of India; see their Figure 1). We will refer to the model ISMR corresponding to the observational AISMR region also as AISMR. Scalar quantities to represent ENSO variability are also the same as in (Bódai et al., 2020b), as follows. 1. Average SST in the standard Niño3 region represented by the box (5°S, 5°N, 210°E, 270°E); 2. average SST in the standard Niño3.4 region (5°S, 5°N, 190°E, 240°E); 3. SLP difference,
We investigate the correlation of the “near-synoptic” JJA mean of any of Niño3, Niño3.4, SOI and box-SOI with the JJAS AISMR. The correlation coefficient r is evaluated across the ensemble, as done in (Bódai et al., 2020b), which results in time series with one data point from each year. These time series are representations of the forced response of the ENSO-IM teleconnection. However, the finite ensemble size entails a relatively large sampling error, and the corresponding fluctuations in the estimated signal,
We will evaluate correlation coefficients of the AISMR also with the PCs belonging to EOFs. PCs and EOFs can be also defined and constructed with respect to (wrt.) the ensemble, as a “snapshot”6, hence the name SEOF, as proposed by Haszpra et al. (2020a,c).7 The SEOFs concerning SST are evaluated here wrt. the Equatorial Pacific box (30°S, 30°N, 150°E, 295°E), and, concerning SLP, wrt. the narrower box (10°S, 10°N, 150°E, 295°E). Traditional EOFs are decomposing spatio-temporal variability as a sum of independent standing waves, i.e., spatial patterns, orthogonal in the N-dimensional gridpoint space, modulated by arbitrary but uncorrelated temporal signals, the PCs. Snapshot EOFs are computed in the same way but different time steps are replaced by different ensemble members (i.e., realizations of the dynamics).
Besides the requirement of (“spatial”) orthogonality, each new EOF is defined such that the corresponding PC has the largest possible variance, leading to an eigen-problem. The variances of the PCs svd
as done in (Björnsson and Venegas, 1997). The ordering of the singular values wrt. magnitude,
PC1s of the SLP and the SST fields in the same box have more in common than, e.g., Niño3 and SOI, because Niño3 and SOI do not derive from a mathematical definition of dominance9; and one could think that more similar representations yield more similar results10. Therefore, firstly, we wish to see if we can establish a robust representation of the ENSO-IM teleconnection by having possibly a better match between the forced response signals belonging to the SLP and SST than between those using the classic indices 1.-4. Secondly, the teleconnection strength can possibly change because of a shift in the “center of action” of the ENSO phenomenon, something that can have a different impact on the teleconnection representations given by one of indices 1.-4. and the AISMR. Thirdly, including higher EOF modes can reveal more predictive power of the full field compared with a simple index alone. In this regard we are interested in whether the correlations to do with the higher modes are more susceptible to the anthropogenic forcing. We will only show results for the second modes. Traditionally, only the first two or three EOFs are regarded to describe ENSO11, and we should distinguish between ENSO-related and full Pacific variability, but EOFs beyond the third will be found to have negligible importance, we will thus usually drop this distinction.
The pursual of the listed three points of inquiry can be supported by MCA and CCA. MCA and CCA are similar to the EOF analysis, but consider two fields, and find paired modes recursively whose paired PCs are respectively uncorrelated with the readily determined PCs belonging to the other modes of the same kind (or “side”) and have the maximal covariance and correlation, respectively, between them12. Note that these modes do not capture in general the locally dominant modes of variability in the given regions (which may be represented e.g., by EOFs); this is the situation only in the uninteresting case: for fields that are not related at all. Instead, they highlight parts of the variability in the two regions that are interrelated the most. These are not additive “parts” of the variability for CCA though; only the MCA modes are independent being spatially orthogonal, but not the CCA modes. By comparing these patterns and their changes to those locally defined in the given regions and thus characterizing ENSO and the IM in our case, we might possibly obtain some hints about how much spatial rearrangements of the relevant areas contribute to changes in the teleconnection strength, but confirmations by further analyses would be necessary.
Similarly to ENSO, we may carry out an EOF analysis on the Indian precipitation field, as represented by M gridpoints, to identify dominant modes of variability from a local point of view, for which we can use the box of (5°S, 40°N, 65°E, 100°E). However, an arbitrarily selected box may not provide a good representation. In fact, the comparison of the time dependence of correlation coefficients belonging to teleconnection representations or characteristics involving the scalar AISMR, on the one hand, and involving the full spatio-temporal monsoon variability, on the other hand, would make most sense if the domain for the latter were the same as for AISMR. We make this choice for our main exposition, and provide the results with the choice of the box given above for comparison in the Supplementary Material, including those obtained by replacing AISMR by the box summer precipitation (BOXSR).
CCA yields the correlation coefficients between the paired PCs by definition; as for the MCA, besides the covariance of the paired PCs yielded by the definition, the correlation coefficient is straightforward to compute. The correlations of these PCs are not analogous to those between the AISMR and the PCs of EOFs of ENSO. We anticipate that the SMCA and SCCA lead to higher correlations than the SEOF analysis, stemming from their very definition. In this regard, as the fourth point of inquiry, we want to see if higher correlations are more susceptible to anthropogenic forcing. A comparison of the correlation coefficients yielded by the MCA or CCA can be made with those between the PCs of the EOFs of the same order on the two sides. Although, in contrast with MCA and CCA, PCs mismatched wrt. the EOF order will in general feature a nonzero r.
On the side where
From the point of view of predictability, in view of the regression model
where
In the case of multiple predictors,
This concept can be further generalized considering multiple scalar predictands,
In the natural case when Φn are the PCs belonging to the ENSO-side MCA modes, which is our choice for calculations, Rm reduces to be just r(Φm, Ψn), n=m. One can take the square root of TFSTVE in order to have something comparable to the correlation coefficient r associated with the one-dimensional setting of e.g., spatial averages. Nevertheless, they are not comparable from the point of view that the same fraction of AISMR variability explained as the TFSTVE provides much less information given that it overlooks the spatial part of the variability. Furthermore, concerning the detection of nonstationarity potentially by the MK-test, it is not clear to us whether the Fisher transform can be applied to TFSTVE to produce residuals described by independent identically distributed random variables—a requirement by the MK-test—the same way as it does in the case of the Pearson correlation coefficient (Fisher, 1915; Bódai et al., 2020b). We pursue this analysis in the belief that it does to a reasonable approximation.
We carry out the MCA and CCA over the same domains as the EOF analyses, whether it is the AISMR domain or a box, by applying Matlab’s svd
and canoncorr
, respectively. Before pursuing MCA and EOF analysis, we regrid the SST data using Matlab’s griddata
, mapping the irregular ocean grid onto a regular one of 2° latitude-longitude resolution. With this, a weighting by the grid cell area as proposed by Baldwin et al. (2009) is straightforward (although it makes hardly any difference in these tropical regions). Instead of the complete field, the SCCA is performed on the PCs corresponding to the first 10 SEOFs, since it is ill-defined on the full fields as a result of the number of gridpoints on any considered domain being larger than the number of ensemble members used. MCA could also be restricted to the first 10 SEOFs, presumably without much influence on the results. In fact, it turns out that on the Equatorial Pacific side, as few as 3 EOFs capture most of the variance (see Supplementary Figures S2, S3). Therefore, we can regard the TFSTVE to represent the ENSO-IM teleconnection even if we are prepared to regard only the first 3 EOFs to constitute the ENSO phenomenon. On the other side, 10 EOFs are also sufficient to represent the IM variability. We will speak about SCCA modes as the linear combination of the SEOF modes in terms of the coefficients defining the so-called canonical variables. Indeed, thanks to the orthogonality of the SEOFs, the projection of the full fields onto these modes yields the SCCA PCs whose correlations were maximized by the SCCA.
The finite size of the data set (the number of the ensemble members, or the length of the time series if evaluation is carried out wrt. time) introduces errors in the estimates of the spatial modes of the various kinds mentioned. The relative errors are increasing with the order of the mode (North et al., 1982; Quadrelli et al., 2005). In our experience, these errors seem to lead to a negative bias in estimating r involving some EOF (e.g., r(PC1, AISMR) or r(PC1, PC1)), but to a positive bias of r to do with MCA or CCA. In fact, with a high enough dimensionality of the spatial representation, given the number of ensemble members fixed to be 63,
The biases of r can be reduced by pooling data within a relatively short time window. We use a moving window of a length of 11 years. The anomaly for each data point in such a time window could be calculated by subtracting the combined temporal and ensemble mean (i.e., the mean over all the pooled data points). Alternatively, which is what we choose to rather do, one can pool data for determining only the modes more accurately. Such a smoothed MCA or CCA mode (or even an EOF) calculated from the pooled anomaly data as described is ordered to the middle of the time window. The correlation coefficient r is then estimated wrt. ensemble-wise variability only, from the subset of the pooled anomaly data belonging only to the mid-window single year. One can go further, as we do, by leaving out the mid-window data for the first part of this process of determining smoothed modes. This should lead to a negative bias, just like with r(PC1, AISMR), etc. We will refer to these estimates of r as “conservative.” From the point of view of detecting nonstationarity, however, our conclusions rely on the assumption that the bias depends only on the ensemble size, but not e.g., the true value of r. As for the simple representations involving indices like Niño3, one can also apply pooling to determine the ensemble means, and, so, anomalies, more precisely.
The statistical errors of the numerically determined modes can pose a problem in studying the forced response of the modes, however. It is so because the svd
algorithm returns the mode estimates with an arbitrary orientation. Before looking at the year-to-year time dependence, or possibly taking temporal means in time windows to compare, one needs to make sure that all the mode estimates corresponding to different years have the same orientation. We do this by picking a reference year and flip modes in other years if they do not have the same orientation as the one in the reference year. We check whether they do by checking the sign of the cross-correlation with the mode in the reference year. However, if modes are corrupted too much, then we are in fact checking the agreement of the orientation of mostly the random components, not those of the underlying true modes. In our experience, mistakes of wrongly flipping modes are made already in the case of the second modes. Nevertheless, the fewer the mistakes, the less error is made in determining the forced response. Furthermore, this problem should affect the smoothed modes to a lesser degree.
In conclusion, to represent the teleconnection, we shall use r of Niño3–AISMR, Niño3.4–AISMR, CCA-AISMR, R, and TFSTVE. We provide results separately for the constituents of R including r of PC1-AISMR and PC2-AISMR in association with EOFs on the Pacific side. Furthermore, we show in the Supplementary Material that r of CCA-AISMR and R are one and the same thing. We shall not consider as a representation any individual r(PCn,PCn) for EOF, MCA, CCA, but the latter quantities also characterize some aspect of the teleconnection and will support our analysis.
We will explain some findings about these r’s by comparing spatial patterns of different modes. Nevertheless, we show in the Supplementary Material that forced changes of spatial patterns do not necessarily have robust implications for the change of the teleconnection strength: r of CCA-AISMR may be dominated by r of EOF1–AISMR even if the spatial pattern of CCA-AISMR in the Pacific has a large weight from EOFi,
2.3 Decomposition of the Forced Change of the Teleconnection Strength
Once the
These three factors are:
• The ENSO variability
• The ENSO-IM coupling a, being the regression coefficient;
• The noise strength
Note that, like r, both
Concerning the numerical evaluation of the quantities in question, the first step is to evaluate
For a quantitative assessment of which factor dominates the change in r out of the ENSO-related variability
Note that such an attribution proposed here is not generically applicable to representations or characteristics of the teleconnection based on MCA and CCA. This is because the signal
3 The Forced Response of the ENSO-IM Teleconnection
3.1 Spatial Aspects
We start by inspecting spatial characteristics of the ENSO and IM variability by means of SEOFs and by comparing them to those corresponding to largest covariances and correlations defined through SMCA and SCCA whereby on the IM side the domain of the AISMR is considered only. We pay particular attention to the forced changes of these patterns.
Figure 1 shows the first modes of these analyses on the side of the ENSO based on the SST, averaged within four subsequent 50-year periods starting from 1900. We only show these temporal means of the smoothed modes, which were described in Section 2.2. The year-to-year changes of the modes are presented in Supplementary Videos13.
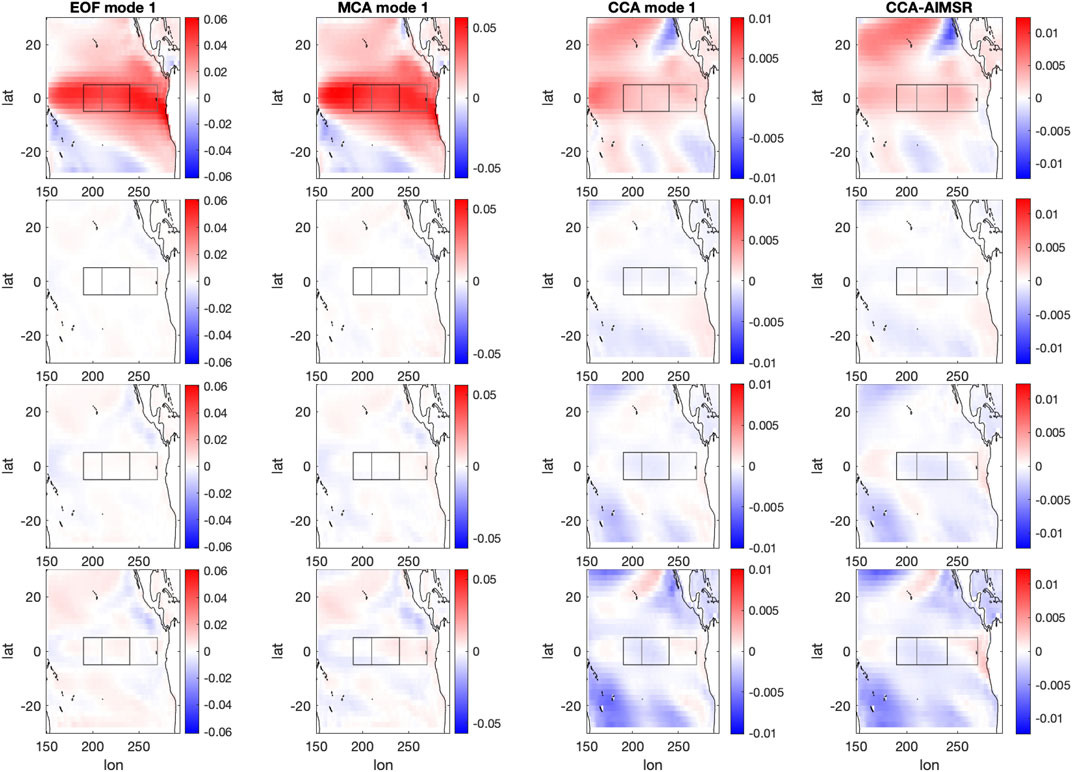
FIGURE 1. Forced change of the first modes of JJA-mean SST variability in the Equatorial Pacific. Temporal means are taken in four consecutive 50-year periods starting from 1900. The top row displays the temporal mean in the first period, and the subsequent rows display the difference with respect to that in the following periods. Leftmost column: EOF-, 2nd column: MCA-, third column: CCA first mode, last column: the only mode of CCA-AISMR. For the MCA and CCA analysis the domain on the side of the Indian monsoon is the area associated with the AISMR, as seen in Figure 2. The Niño3 and Niño3.4 boxes are marked in gray and black, respectively.
At the beginning of the simulations, EOF1, which is the most important pattern associated with ENSO variability, looks very similar to results in the literature, featuring its main “bump” in the central Pacific. Furthermore, hardly any forced change is visible in it even upon the strong RCP8.5 forcing. In comparison, Carréric et al. (2020) suggests that the maximum of the EOF1 mode shifts to the east in the CESM1-LE by showing that some center (C) mode (Takahashi et al., 2011) shifts to the east more in comparison with the westward shift of some east (E) mode14. This does not appear to be so here.
The first SST MCA mode looks practically the same when evaluated with respect to the full field and to the first three SST EOFs as seen in Supplementary Figure S2, and it is very similar to EOF1 in both cases. This suggests that MCA1 mainly reflects ENSO variability, which is further supported by the similarity in the forced changes of these patterns. We recall that these changes are minor.
In contrast to the MCA1 modes, the first CCA modes, evaluated with respect to the first 10 SST EOFs, once with the full Indian precipitation field and once with AISMR (in which case CCA1 is the only CCA mode), show considerable differences when compared to EOF1. Perhaps the main difference is a much weaker weight of the central Pacific (with more weight being concentrated to the west, perhaps because it is geographically close to India), and this might give even more importance to the observation that variability in some off-equatorial regions seems to have an opposite contribution to the teleconnection than to the main ENSO mode (EOF1). (Although, recall that the relative weights of EOFs in CCA-AISMR do not imply a similar relationship concerning the corresponding correlation coefficients.) One such region is next to South America, and another is to the east of Australia, at least at the beginning of the simulations.
The contrast in spatial features persists for the forced changes. The first CCA modes do undergo a considerable change by the late 21st century. It is even more interesting to observe this change to have a different sign in the middle of the ENSO domain (highlighted by the Niño3 and Niño3.4 boxes) compared to the sign of the minor change in the EOF1 and the MCA1 mode. On the other hand, the region to the east of Australia seems to revert its sign, finally conforming with that in EOF1.
Although each panel of Figure 1 blends 50 separate entities, the mostly monotonic emergence of the changes in consecutive rows suggests that we can see meaningful signals. Otherwise, the Supplementary Video https://youtu.be/2AVETrcfBVU shows ample year-to-year change in the form of a flickering. Given that the forcing is rather smooth and gradual (except for volcanic eruptions), this is likely just a fluctuation stemming from the error of sampling by a finite-size ensemble.
EOF2 for SST also exhibits a well-known pattern, which is practically a dipole or tripole, see Supplementary Figure S12. Its central part undergoes some degree of attenuation until 2100, except perhaps in the very middle, which can be regarded as a westward shift of one “pole” of the tripole. At the same time, areas in the north of the box gain importance. However, all changes are moderate.
CCA2, which is likely not orthogonal to CCA1, seems to be also shaped—beside EOF1—by higher-order EOFs other than EOF2 or even EOF3, which is prompted by Supplementary Figure S2. MCA2 is just in between EOF2 and CCA2, which is not surprising given that an association between MCAi and EOFi and also between MCAi and CCAi is expected. The strength of the association is determined by the regions’ own variances, on the one hand, and their interrelation, on the other.
We would like to remind though that the temporal means of the second modes are less reliable than those of the first modes, as discussed in Section 2.2, which can be seen in the Supplementary Videos https://youtu.be/EZQ7r2v7-zk and https://youtu.be/fahPv_fLgx0.
The Supplementary Figures S6, S14 suggest very similar conclusions for the first two modes in the SLP-based representations and characteristics as for SST on the side of the Pacific, except that the patterns look rather trivial for the EOFs and the MCA modes.
On the side of the IM, Figure 2 shows that the leading mode of precipitation variability (EOF1) is concentrated to the middle of the AISMR region. The MCA1 mode is somewhat different in putting more weight on the foothills of the Himalayas. This is consistent with the r map featuring the strongest correlations over land at this region; and it is epitomized by the CCA1 mode putting an overwhelming part of its weight here. CCA1 misses practically any similarity with EOF1. At the same time, the similarity of the patterns of the forced changes of the four fields shown (rows 2–4) is remarkable, even though these changes are minor over the land.
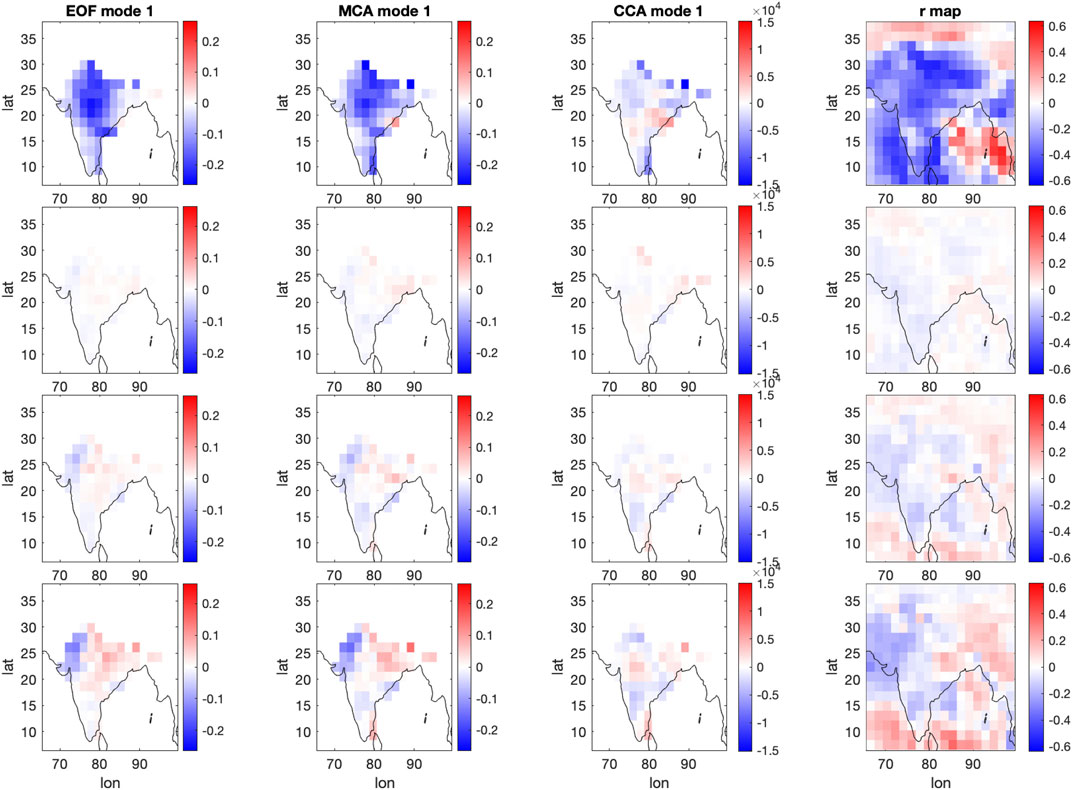
FIGURE 2. Same as Figure 1 wrt. the first three columns, but concerning the Indian summer monsoon. In the fourth column a correlation map and its changes are shown concerning the gridpoint-wise JJAS precipitation and the PC1 of the EOF1 of the SST in the box seen in Figure 1.
EOF2 for the precipitation is also very different from CCA2, see Supplementary Figure S16. EOF2 is a dipole, with an emphasis on the northeast of India but not yet at its edge. CCA2 also features important areas in the southern part of India, not coinciding with the peaks of EOF2 or EOF1. Interestingly, MCA2 is very similar to CCA2 and is thus very different from EOF2. The r map computed for the subleading mode, EOF2, of the Pacific SST field is also very different from any of these patterns. Surprisingly, it is quite similar to that computed for EOF1 (seen in Figure 2). Note that the second modes presented in Supplementary Figure S16 undergo moderate changes in time, which do not affect the conclusions of this paragraph.
Recognizing the importance of areas off the AISMR region, we repeat all computations of this section based on the precipitation field of the complete Indian box instead of the AISMR region. Surprisingly, there is hardly any alteration on the Pacific side (Supplementary Figures S5, S13). On the Indian side, as expected, CCA1 (Supplementary Figure S10) features peaks where the r maps also do, with a special emphasis on the Himalayas. Regions over the Indian Ocean lose importance with time. Interestingly, EOF1 and MCA1 (in the same figure) become similar to CCA1, although with the stronger peak appearing over the ocean (but also weakening with time).
The second modes of the complete Indian box (Supplementary Figure S18) are mainly concentrated on the sea. While CCA2 exhibits considerable similarity with the r map corresponding to EOF2 of the Pacific SST field, there are major differences with MCA2 and EOF2 in the same figure, not attenuated over time either.
So far, all joint modes presented in the analysis of the Indian patterns were based on SST on the Pacific side. Supplementary Figures S9, S11, S17, S19 illustrate that almost identical results are obtained with SLP. We thus conclude that the patterns on the Indian side are very much insensitive to the representation of variability on the Pacific side.
3.2 The Forced Evolution of Correlation Coefficients
The time evolution of the quantifiers defined in Section 2.2 is given in Figure 3 for the SST-based representations of the ENSO-side variability and applying the AISMR mask on the IM side. Concerning the affected representations involving MCA and CCA, the Mann–Kendall test statistics corresponding to the meaningful conservative r estimates are plotted for every subinterval in Figure 4, etc.
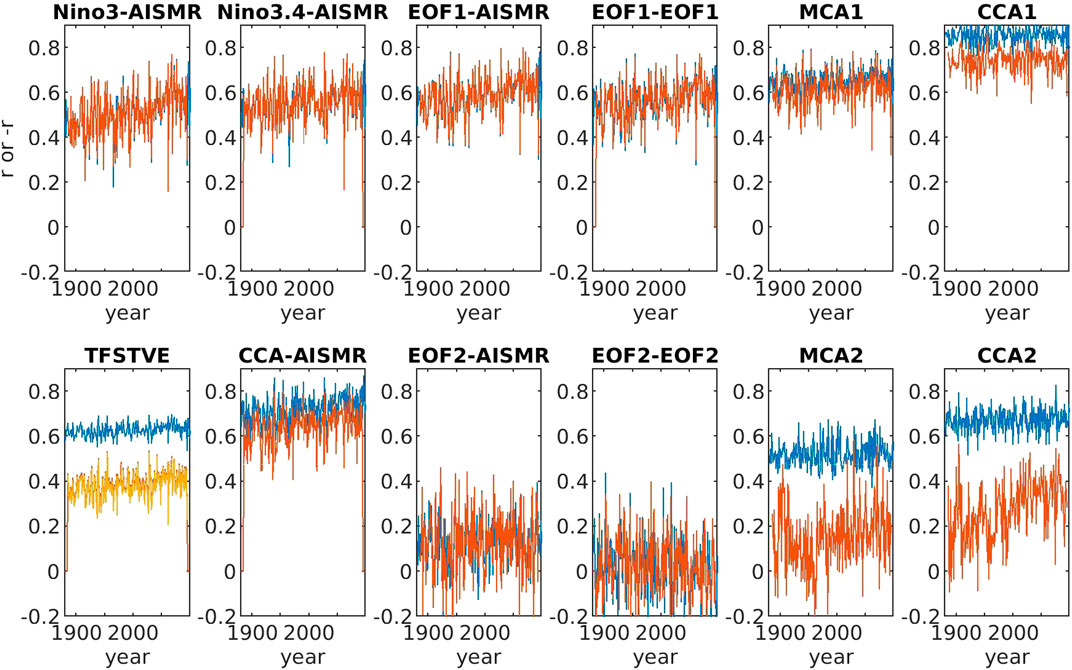
FIGURE 3. Time-evolution of the ensemble-wise correlation coefficients r associated with different representations of the ENSO-IM teleconnection or some relationship between the two domains seen in color in Figures 1, 2. In addition, in the bottom left corner we show the (square-root of the) total fraction of spatio-temporal IM variability explained, TFSTVE (Eq. 5), by PCs of the ENSO-side SMCA modes, as a further represenation of the ENSO-IM teleconnection. Blue curves show the straightforward naive estimates, and brown curves show the “conservative” estimates (see Section 2.2). In the case of TFSTVE, the yellow curve shows the estimate obtained by performing SMCA based on only 3 Equatorial Pacific SEOFs.
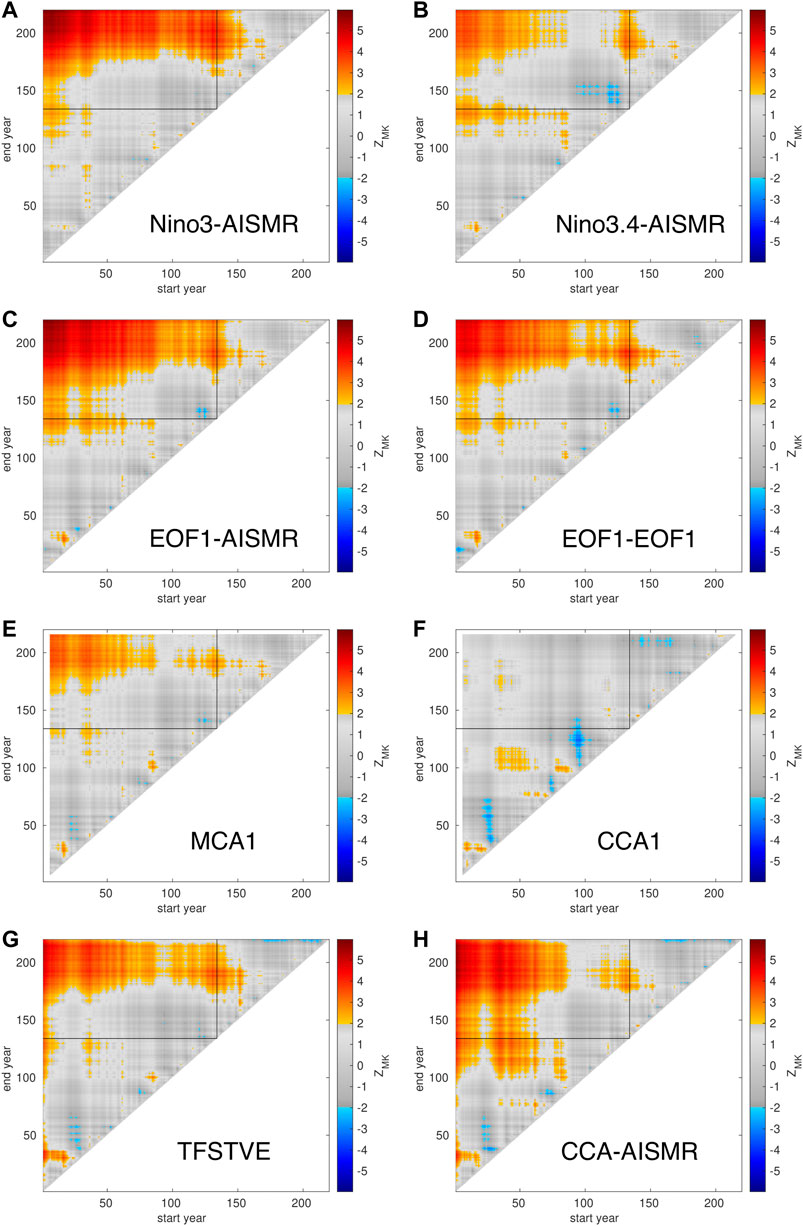
FIGURE 4. Test statistics of the Mann-Kendall test for the stationarity of (the “conservative estimates” of)
From a technical point of view, the different estimates of r featuring a positive (blue curves) and a negative bias (brown curves) are further apart for the more erroneous MCA2 and CCA2 modes in Figure 3, as can be expected. Furthermore, even their nonstationarity is likely considerably corrupted by the positive biases. From the point of view of detectability, however, the positive bias goes with smaller statistical fluctuations. It is interesting to note that the result with positive bias does not feature much nonstationarity for either CCA1 or CCA2, while it does with CCA-AISMR. As for the result with negative bias, the nonstationarity for CCA-AISMR is in between those of CCA1 and CCA2.
A very distinct separation of the results with positive and negative bias is also seen for TFSTVE which quantifies the predictability of also the spatial variation. Furthermore, performing MCA using PC1-3 of the SST field (yellow line) is hardly different from using the full fields (brown line), which underlines that TFSTVE does represent an ENSO-IM variability. This result could perhaps be expected considering the rapid decay of the variance explained by higher-order Pacific EOFs as presented in Figure 5. Furthermore, in fact, TFSTVE is dominated overwhelmingly by MCA1, which latter on the Pacific side is in turn dominated by EOF1 (Figure 1). This is prompted by the decay of the variance explained by higher-order IM EOFs, on the one hand, and those of
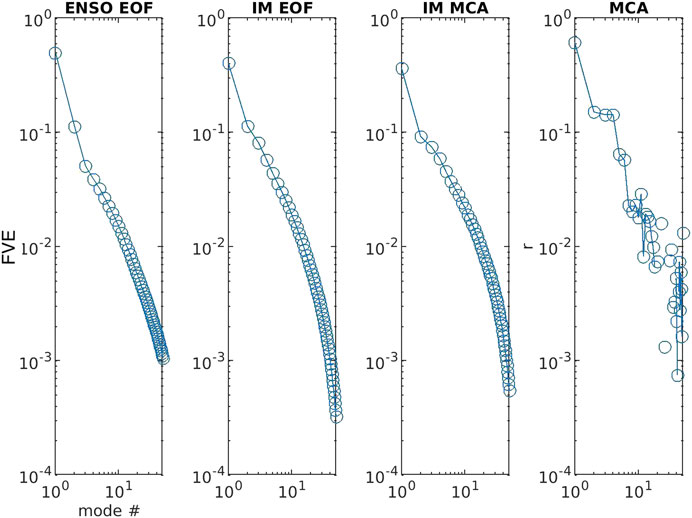
FIGURE 5. Fraction of variance explained FVE by (“raw”/“unsmoothed”) SEOF and SMCA modes. In addition, the rightmost diagram shows the “conservative” estimate of the correlation coefficients for the paired PCs of SMCA modes. Results of temporal averages for the full data span of 1880–2100 are shown in log-log diagrams.
Having said these, we conclude that TFSTVE is reasonably high to represent a strong teleconnection, and its time evolution is detected to be a long-term increase in Figure 4G. An increase is, however, not detected in shorter intervals around 1900, 2000, and in the very late 21st c.; instead, slight drops are suggested by the test statistics.
The PCs of MCA1, the leading contributor to TFSTVE, exhibit a relatively high r (MCA1 in Figure 3) with a time dependence very similar to that of TFSTVE, which carries over to the MK-test results in Figures 4E,G. r of the PCs of MCA2 is lower; in fact, it is relatively close to zero in the lower estimate (MCA2 in Figure 3). The dominant feature in its time dependence is also a long-term increase (Supplementary Figure S38C).
We recall here from the previous section that MCA modes have a similar pattern to EOFs, which may suggest that the relationship expressed by the MCA modes should be observable between the EOFs, characterizing variability of local importance, of either side. Indeed, r between the PCs of EOF1s (EOF1-EOF1 in Figure 3) is only slightly smaller than for MCA1. It undergoes a more pronounced long-term increase (Figure 4D). At the same time, r between the PCs of EOF2s (EOF2-EOF2 in Figure 3) is practically zero and is not detected to change at all (Supplementary Figure S38B). This suggests that the phenomena expressed by EOF1s are quite important for the teleconnection, but the same is not true for EOF2s.
Correlation is preserved for EOF1 and somewhat regained for EOF2 on the Pacific side when the IM side is chosen to be respresented by AISMR instead of EOFs, see EOF1-AISMR and EOF2-AISMR in Figure 3. AISMR is thus more strongly correlated with the Pacific variability than PCs of individual EOFs of the AISMR region. Temporal changes are generally also a bit more pronounced (Figure 4C; Supplementary Figure S38A).
One can then go further and take the coefficient of multiple correlation, R, of all Pacific EOFs with AISMR, which is the same as r corresponding to the single CCA-AISMR mode, a full representation of the ENSO-IM teleconnection. It is very high (see CCA-AISMR in Figure 3). It undergoes a relatively strong increase in the 20th c. with a more definite stop in the late 21st c. (Figure 4H), especially in comparison with the time evolution of TFSTVE (Figure 4G), but also with other MCA- or EOF-based characteristics (Figures 4C–E). Such differences may be related to the differences in the changes of the corresponding patterns as described in Section 3.1. Nevertheless, all main features are shared by these representations or charactersistics.
This is also true for the Niño3-AISMR and Niño3.4-AISMR representations (Figures 4A,B, both showing a high correlation in Figure 3). On the level of details, the increase of r of Niño3-AISMR extends very late to the 21st c. For r of Niño3.4-AISMR, the increase is more moderate, and a drop is undoubtedly detected after 2000, which is exceptional among the representations and characteristics discussed so far. The relative suppression of the overall increase for r of Niño3.4-AISMR may be related to the opposing changes of EOF1 and CCA-AISMR in the Niño3.4 region as discussed in Section 3.1.
CCA1 and CCA2 evaluated with the precipitation field within the AISMR region do not fit the picture outlined so far. Remember from Section 3.1 that their spatial patterns look very different from EOFs and MCA modes on the IM side and are concentrated on the very edges of the AISMR region, so that moderate importance should be associated with the corresponding results from the point of view of the teleconnection, in spite of the high correlations (CCA1 and CCA2 in Figure 3).
The shape of CCA1 and CCA2 on the IM side, together with the r maps, suggests that important areas for the teleconnection fall outside of the AISMR region and prompts to evaluate modes of spatiotemporal variability in the complete Indian box. As mentioned in Section 3.1, this brings modes of the same order similar to each other and to the r maps of the Pacific EOFs, reveals the importance of the bulk of the Himalayas and of oceanic areas, and a loss of this importance with time. This importance loss might imply that characteristics of the teleconnection might strengthen more when they are based solely on the AISMR region rather than the complete box.
We do observe in Supplementary Figures S26, S34 that results for TFSTVE, the MCA modes and the paired EOFs are very similar for the complete box as for the AISMR region except that the signal of strengthening is much weaker (and turns to a pronounced weakening for EOF2-EOF2). At the same time, CCA1 and CCA2 now exhibit strengthening (with the previously described intermittent periods), even stronger than the other characteristics. However, for characteristics for which AISMR is replaced by the mean box precipitation (BOXSR), the correlation becomes unreasonably low. These characteristics do not deserve further analysis and illustrate that applying appropriate techniques to select IM-related precipitation within the box is essential.
Using SLP instead of SST in a suitable subset of the Pacific box does not yield any difference that would be worth mentioning (Figure 6; Supplementary Figures S25, S27, S33, S35, S39, S41, except for SOI and box-SOI). This means that SLP reflects the same phenomenology as SST in the respective domains. The results with SOI and box-SOI are similar to the rest, and differences in the details are not possible to interpret without extending the currently used domain to the west.
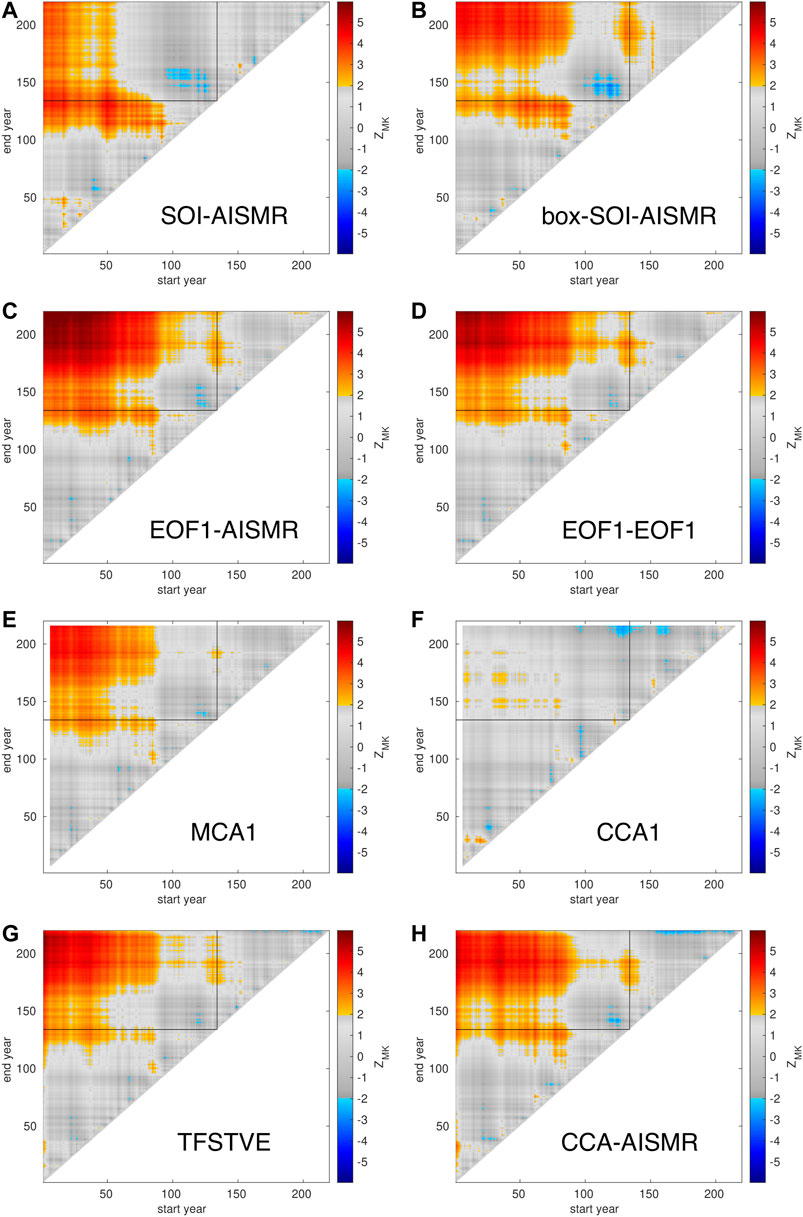
FIGURE 6. Same as Figure 4 but the ENSO side is characterized based on the SLP.
3.3 The Drivers of Changes in Correlation Coefficients
Having established the observable changes, we can now ask about their drivers in terms of the linear regression model as introduced in Section 2.3. We emphasize again that attribution in the case of characteristics involving MCA and CCA is in general not possible. Furthermore, we do not perform statistical tests here regarding these drivers. Therefore, the attribution of a change in r to different factors is not rigorous but rather tentative.
Figure 7 suggests that ENSO-side variability,
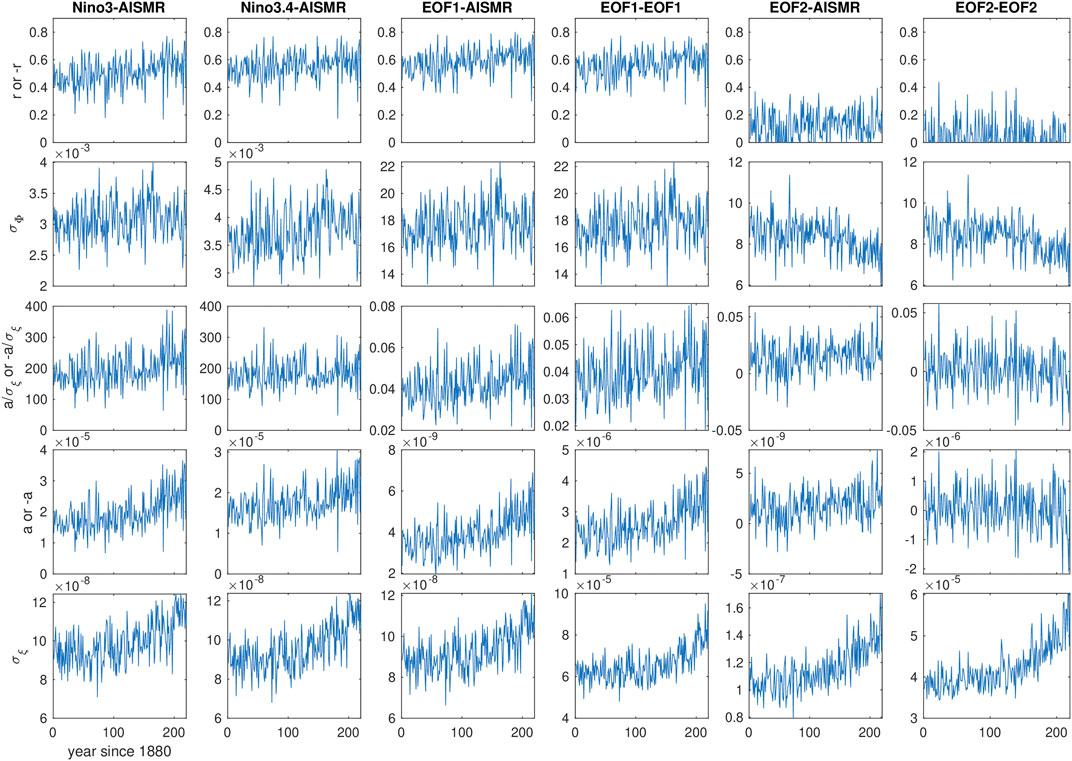
FIGURE 7. The forced evolution of correlation coefficients
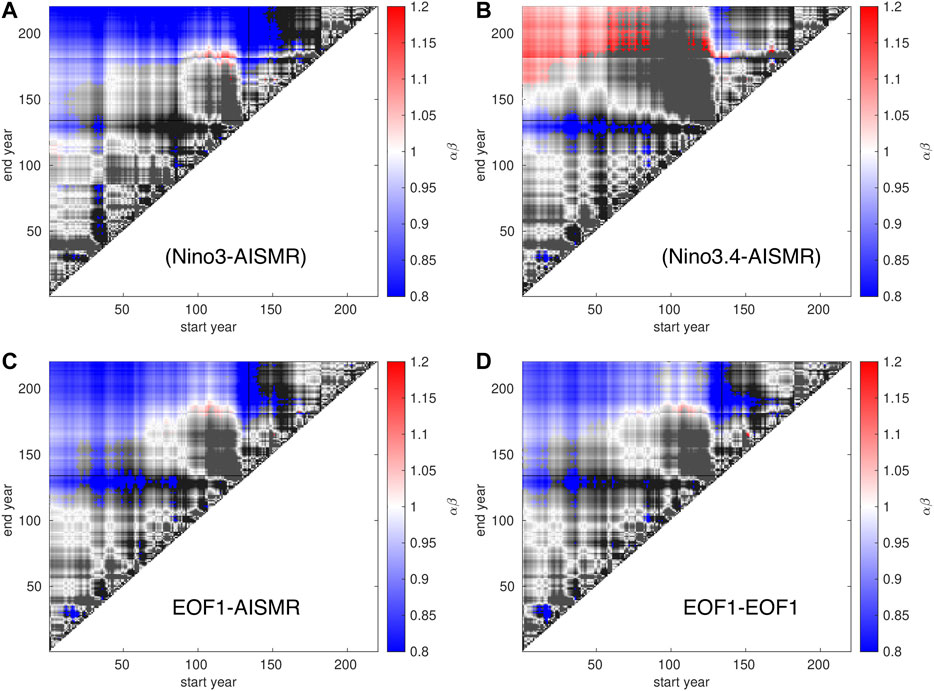
FIGURE 8. Relative importance of
Making use of the SLP mostly agrees with the findings obtained with the SST, see Figures 6, 9. The only but important exception is a decreasing ENSO-side variability in view of PC1. We note that the same domain as used for the SST is not suited for the SLP: the corresponding principal modes (whether SEOFs, SMCA and SCCA modes) are unrelated to the essence of the ENSO phenomenon, as a concentration of the high-amplitude areas on the edges of the domain indicates (Bódai et al., 2020a).
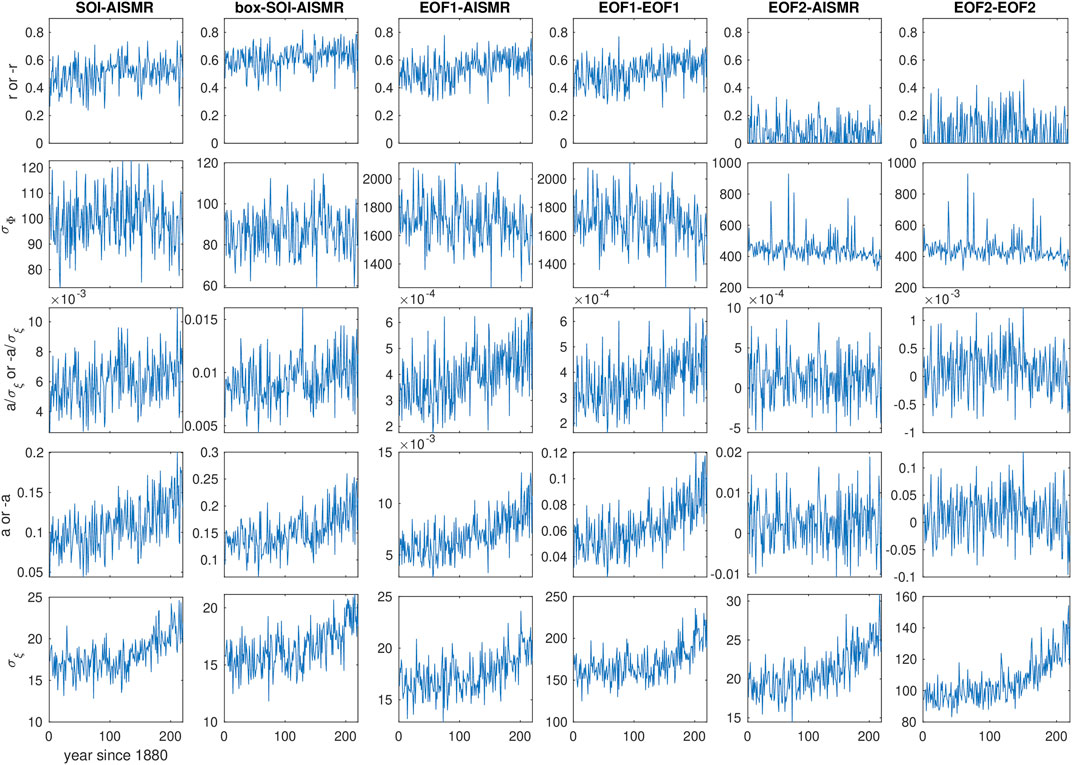
FIGURE 9. Same as Figure 7 but the ENSO side is characterized based on the SLP.
Although second modes were found to be relatively unimportant for the teleconnection, there seems to be considerable nonstationarity in
4 Comparison of the MPI-GE to Observations and Reanalysis
Results and conclusion that derive from a large ensemble apply only to the model that generated the data. However, the ultimate enquiry pertains to the actual Earth system, and, in that regard, one should perform some comparison of the model with observations. Clearly, it has its limitations given that the observational record corresponds to a single realization, and, so, it provides proportionally less information about the system. In (Bódai et al., 2020b) we compared long-term means and trends of the SST fields and precipitation in South Asia, as well as temporal correlation coefficients pertaining to areal means like e.g. the AISMR and Niño3. Our conclusion was, however, that it is not possible to detect nonstationarity from single realisations. In terms of spatial modes of variability and associated correlations, it is likewise doubtful to be able to establish the forced response from observational data. What we can compare here are modes and correlations that are in a way overall “representative of a time period.”
Calculations are carried out with respect to temporal variability, not only for observations, but also for each member of the ensemble, so as to make a comparison meaningful. In turn, the different realisations for the model will provide some statistics for the finite-size temporal estimates. As proper anomalies cannot be obtained for observations, we instead feed the calculations with data that represent “high-frequency variability.” It is obtained by subtracting a low-frequency component from every data point, which is approximately a 21 years moving window mean. We use the same method as in (Bódai et al., 2020b), applying a Savitzky–Golay filter of order 3 to the running window means. We obtain conservative estimates e.g. for r associated with PCs belonging to modes by parting the data into two consecutive periods of equal length, computing the modes from the first part, and computing PCs by projecting the fields given by the second part onto those modes.
The data representing observations are obtained from the 20th century CERA 20C reanalysis product (Laloyaux et al., 2018) for Pacific SST, and the CRU PRE v4.03 gridded precipitation data (Harris, 2019a; Harris, 2019b) for the Indian summer monsoon rain. We analyze the time period of 1901–2010. Precipitation variables are not available for CERA 20C, hence, we can make a comparison with the model only with respect to the representation where the AISMR domain is used. This is not a problem from the point of view that only land areas have a practical relevance. The precipitation data of the 20th Century Reanalysis V2 data (Compo et al., 2011) is not so faithful to reality; it does not reproduce the AISMR time series (Parthasarathy et al., 1994) well, and yields only an r(Niño3, AISMR) = –0.2.
The results for the SST principal modes are displayed in Figure 10. The ensemble means of the temporal modes in the top row are hard to distinguish from the temporal means of the snapshot modes as seen in Figure 1. In the second row the ensemble standard deviations reveal that the sampling error is much bigger to do with the CCA compared to the MCA and EOF. The observational EOF1 is more concentrated on the Eastern Equatorial Pacific, or, at least, it does not feature a second peak in the Western Equatorial Pacific like the model does. The weight of the observational MCA1 mode is somewhat shifted to the west wrt. EOF1. The observational CCA1 and CCA-AISMR modes are similar to one another, just like those of the models, but the respective observational modes are much less similar to the model modes as compared to EOF1 and MCA1. The observational CCA1 and CCA-AISMR modes are concentrated to the middle of the Equatorial Pacific while the model modes on the westernmost of the Equatorial Pacific and even on off-equatorial areas. Yet, the associated correlation coefficients match very closely (Figure 11F).
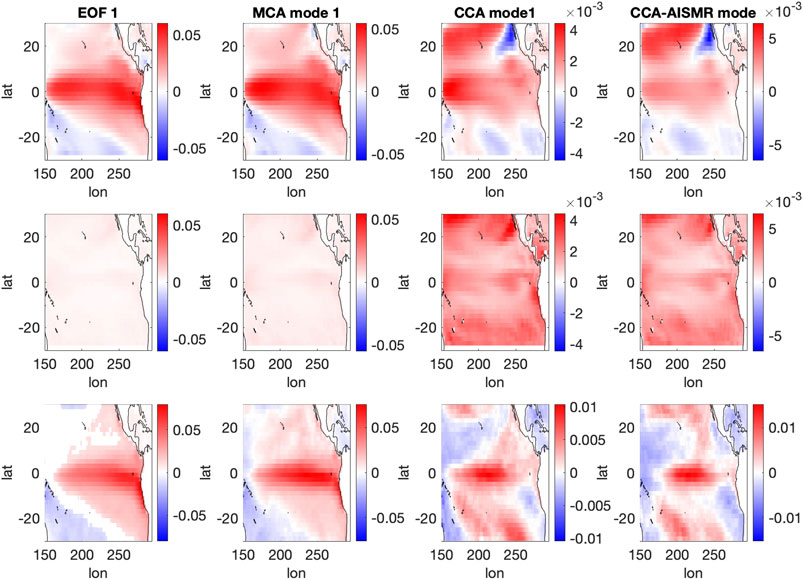
FIGURE 10. Comparison of principal SST modes obtained by temporal EOF analysis, MCA, CCA (the columns corresponding to those of Figure 1) between the MPI-ESM (top row) and observation (bottom row). In the middle row the ensemble-wise standard deviations are shown in the same color range as the modes themselves.
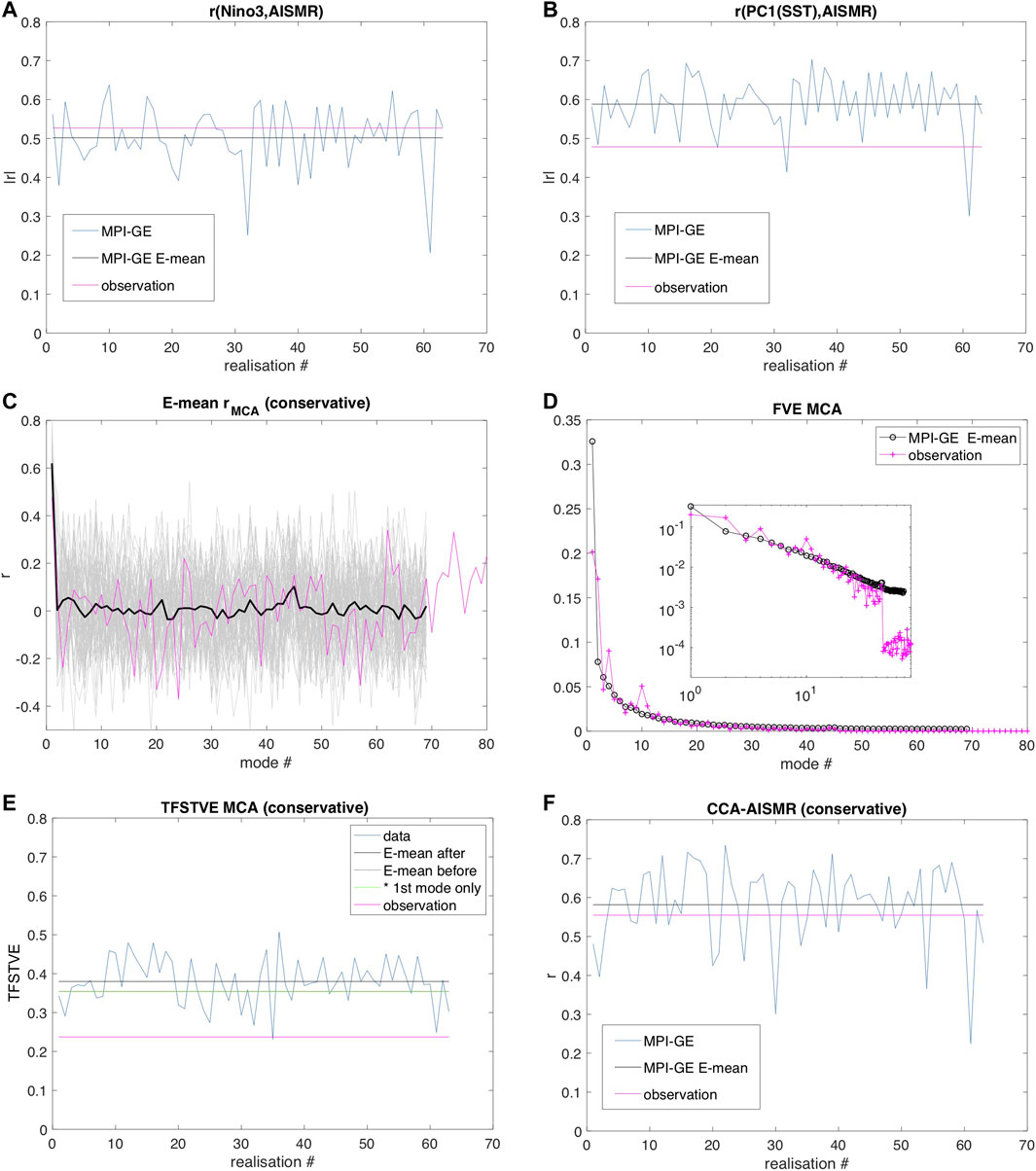
FIGURE 11. Comparison of temporal correlation coefficients r(A-C,F), FVE (D), TFSTVE (E), between the MPI-ESM (blue, black, gray, green curves) and observation (magenta curve) for various representations of the ENSO-IM teleconnection. Panel (E) indicates that the systematic error for the conservative estimate of TFSTVE (brown curve) in Figure 3 is about 0.02.
As for the IM side, the modes are shown in Figure 12. Again, regarding the model, the resemblance of the ensemble means of the temporal modes in the top row and the temporal means of the snapshot modes as seen in Figure 2 is uncanny. It is rather surprising that the CCA1 modes on the IM side match rather well between observation and model. Otherwise, so do the r maps and the MCA1 modes match rather well. The observational EOF1, however, does not appear to closely resemble the observational MCA1 mode, unlike for the model, which might have only to do with the fact that the model does not resolve the very wet climatology over the Western Ghats. The fact that the observational MCA1 mode does not have a strong weight over the Western Ghats despite the high resolution should have to do with the weak correlations with PC1 of the Equatorial Pacific SST in this area, as shown by the r maps.
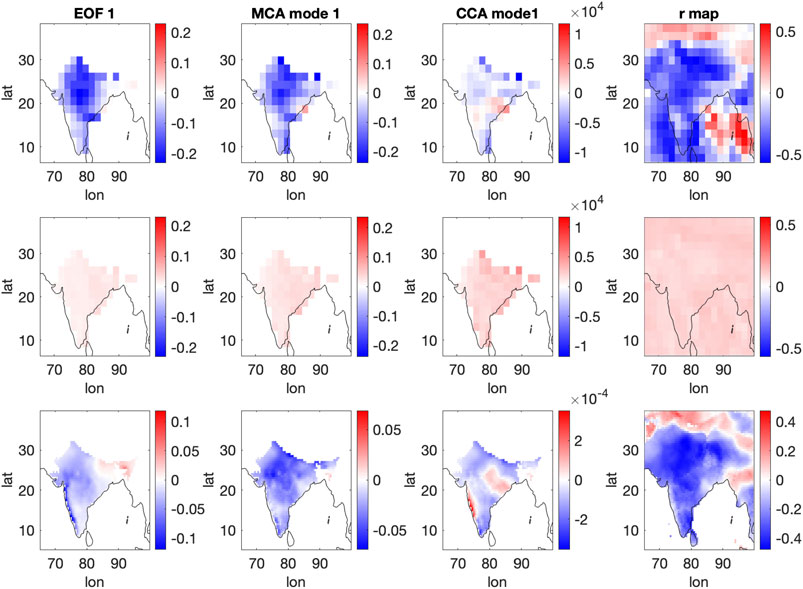
FIGURE 12. Same as Figure 10, but for the Indian summer monsoon precipitation (the columns corresponding to those of Figure 2).
Some of these observations apply also to the second modes. It is particularly surprising how the observational and model CCA2 modes both on the Pacific (Supplementary Figure S42) and IM side (Supplementary Figure S43) resemble one another, even despite the large sampling fluctuations as shown in the middle rows.
As for the correlation coefficients r and TFSTVE, seen in Figure 11, the estimate from observations is always smaller, except for the Ninõ3-AISMR representation [in panel (A)]. It is not clear how this exception could be explained by the restriction of the observational EOF1 to the east (Figure 10, bottom left), if it could be at all. It is the CCA-AISMR representation (F) that yields the largest (conservative estimate of) r also for observations, very slightly below the figure for the model. In contrast, the TFSTVE (E) is considerably smaller for observations, which is prompted by both r (C) and FVE (D) being smaller for the observational MCA1 mode. Nevertheless, these diagrams suggest that this can be just a statistical fluctuation of the single observational realization. Note also that r for the higher-order MCA modes is practically zero; that is, only MCA1 contributes to the TFSTVE.
We emphasize once again that the above comparison cannot confirm confidence that the MPI-GE correctly features a strengthening ENSO-IM teleconnection. Neither can it undermine the confidence thanks to the fairly close match in most aspects, the observational results falling seemingly within the ensemble-wise variation of the MPI-GE estimates.
5 Nonergodicity
Whenever the forced change is nonlinear, whether due to a nonlinear progression of the forcing or a nonlinear response characteristic, an estimation of the momentary ensemble-mean climatology by a temporal mean in a finite window should be biased (Drótos et al., 2016). That is, the ensemble mean of the temporal mean would not be equal to the ensemble mean itself (say, at the middle of the time window), which is termed nonergodicity. Higher-order statistics should be biased even in the unlikely case that the forced change of the ensemble mean is linear and the internal variability would not feature a forced change. The linear Pearson correlation coefficient is no exception, i.e., its time-based evaluation (the traditional choice) will be biased even if the ensemble means of the correlated quantities exhibit a temporally linear forced response. Notwithstanding, a linear time evolution of the Pearson correlation coefficient
In fact,
To the end of constructing a suitable test, we observe that the Fisher transform
at any time does not match the theoretical value
i.e., nonergodicity.
We have four issues to consider. First, z is not available because of the finite N ensemble size. Therefore, we cannot evaluate nonergodicity at any time (every year). Instead, we can consider nonergodicity overall in an interval of length T calculating
Second, had
Third,
As a fourth issue, there might be a low-frequency influence on the teleconnection, say, via a time-dependent a (Gershunov and Barnett, 1998; Torrence and Webster, 1999; Krishnamurthy and Goswami, 2000; Krishnamurthy and Krishnamurthy, 2014; Watanabe and Yamazaki, 2014). This should increase the width of the distribution of the sample temporal correlation coefficient, i.e., it should be larger than
Turning to our application, the test statistics is found to be 756 for the ENSO-IM teleconnection representation given by the Niño3-AISMR pair. That is, it is well above the 0.95 quantile, corresponding to a minuscule p-value, so that the hypothesis of ergodicity can be rejected with extremely high confidence. We also evaluate the test statistics corresponding to the global correlation maps seen in Supplementary Figure S1. The result of this can be presented as a global map, too (Figure 13), in which a contour for the 0.95 quantile, 670, encloses regions where ergodicity can be rejected at the usual significance level of 0.05. We can see such regions not just in the tropics or in the Equatorial Pacific, but all over the world. Nevertheless, the highest levels of bias/nonergodicity is indeed found at the center of ENSO variability and elsewhere in the Equatorial Pacific.
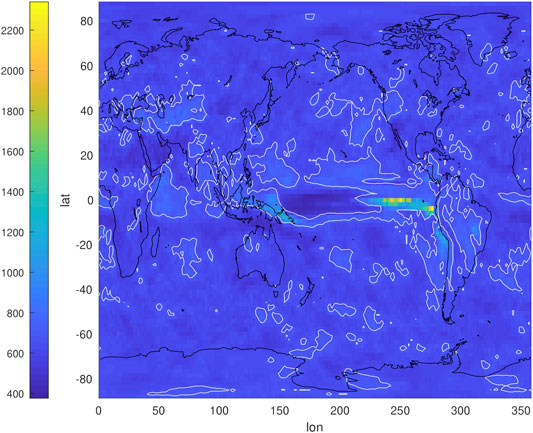
FIGURE 13. Test statistics of our ad hoc test of nonergodicity regarding the Pearson correlation coefficient. The contours mark the 0.95 quantile. Here, ENSO is represented by PC1 of EOF1.
We note that even if
6 Discussion and Conclusion
We have re-examined the forced response of the ENSO-Indian monsoon (IM) teleconnection as conveyed by the MPI-GE data. One main increment taken by the new analysis is the consideration of spatial aspects of any forced change. This was achieved by determining empirical orthogonal functions (EOFs) and modes of Maximum Covariance Analysis (MCA) and Canonical Correlation Analysis (CCA), and considering various characteristics and representations of the teleconnection defined through these tools. Beyond individual correlation coefficients, we defined the total fraction of the spatio-temporal IM variability explained (TFSTVE) by the Pacific variability, and interpreted results in the spirit of the coefficient of multiple correlation, R. We found that both TFSTVE and R are dominated by the first-order modes, both wrt. their magnitude and forced changes. Characterizing different aspects of the relationship between the variability of the two regions allowed us to build a robust picture of forced changes.
We have found almost all characteristics and representations to convey a picture of a strengthening teleconnection in terms of a statistical test, confirming earlier findings. One or two slight drops around the turn of the 20th–21st centuries have also been detected, and the increase in strength has been found to slow down in the late-21st c. The latter aspect is more prominent when spatial variability is taken into account on a wider region around India, including the Himalayas and surrounding oceanic areas. We have found indications that using such a region may be more suited to analyzing and understanding the teleconnection between ENSO and the IM, but aspects specific to the traditional observational product of all-Indian summer monsoon rainfall (AISMR) may be underrepresented and overlooked in this way.
The late-21st c. slowdown mostly has to do with a curious nonmonotonicity in the change of the ENSO variability: in most representations of ENSO variability, about midway in the 21st c. rather suddenly it starts to decline. In terms of a linear regression model that can be associated with evaluating the (linear) Pearson correlation coefficient, the typically increasing regression coefficient—which can be viewed as an ENSO-IM coupling strength—also plays a strong role. Although the model’s noise strength undergoes a similar increase, which has an opposing role, the change of the coupling is found to dominate. The latter turns out to be the central piece of the robust or consistent picture of the strengthening ENSO-IM teleconnection. We conjecture that the temporary drop or drops in the teleconnection strength at the turn of the 20th–21st centuries are also related to corresponding changes in the coupling strength.
We leave it for future work to attribute these features to physical effects. In any case, the change of both the coupling and noise strength should involve both thermodynamic and dynamic factors.
Some differences between different characterstics and representations are due to changes in the relevant spatial patterns. The most interesting finding is that the pattern of maximal ENSO variability and that of maximal correlation with the IM precipitation undergo opposing trends in the middle of the ENSO domain: the middle becomes more important for ENSO itself, while it loses importance for the teleconnection. On the side of the IM, the AISMR region gains relative importance for local variability and even more for the teleconnection with ENSO.
It is essential to note that based on observations the ENSO-IM teleconnection appears to have weakened since about 1980 (Kumar et al., 1999; Bódai et al., 2020b). In (Bódai et al., 2020b) we argued that several reasons could be responsible for this mismatch. Among them one is that the MPI-ESM associated with the MPI-GE is not consistent with the observations of the 20th c. Indian Ocean (IO) warming and IM precipitation decline: in terms of the long-term trend, the ranges of simulated realisations, wrt. both the IO temperature and ISMR, do not contain the observations as respective single realisations–by far [see Figures 12, 13 of (Bódai et al., 2020b)]. See (Aneesh and Sijikumar, 2018), for example, for the role of the IO warming regarding the decline of ISMR, concerning La Niña years, in particular. However, it is a question yet to be answered if the decline of precipitation does actually translate into a weakening of the ENSO-IM teleconnection in the precise sense of a forced change.
Another possibility for the reason for the said mismatch was posited in (Bódai et al., 2020b) to be the very considerable fluctuations of temporal correlation coefficients—the larger in magnitude the shorter the time window—even under stationary unforced conditions, and even without decadal variability of the correlated signals. Evaluating temporal correlations is troubled by further problems when there is a forced change, namely, that 1) when the forced change is nonlinear, the temporal correlations are biased (Section 5), and that 2) the forced signal is not known, and, therefore, the anomalies that are meant to be correlated cannot even be constructed. A detrending, say, removing a linear slope in a time window, does not seem to be a good fix, as even under stationary conditions one would find spurious trends due merely to internal variability, and, so, we would remove a signal that should not be removed.
When an ensemble is available, concerning a single model at least, the biases to do with point 1) above can be detected and, to a certain extent, quantified. We have developed an ad hoc statistical test to do just that, and applied it to the ENSO-IM teleconnection as well as the relationship of global precipitation and ENSO. We have found many regions of the world where a bias could be detected. The reasons for the nonlinearities that such biases imply can be manifold, not only driven by the nonlinearity of the change of ENSO variability, and it should be carefully examined in the future.
Our methodology indeed ensures that the detected biases imply nonlinearity (i.e., not only nonlinearity implies biases, but also the other way round). In our context of the historical and scenario change of ENSO-precipitation teleconnections, robust nonlinearity has been found. Given that nonlinearity implies (presupposes) a forced change, we turn out to have on hand a statistical test for the nonstationarity of teleconnections (linear correlations). This can be seen to complement the Mann-Kendall test (in the special case of the nonstationarity of the correlation coefficient), because the latter takes the alternative hypothesis of a monotonic change, while our test includes nonmonotonic changes too.
Data Availability Statement
The data generated and analysed for this study are available upon reasonable request. For reproducing purposes, one can make use of the MPI-GE data available at https://esgf-data.dkrz.de/projects/mpi-ge/, as suggested in Section 2. Observational/reanalysis data sets analysed in Section 4 are available at the webpages whose URL's are included in the bibliographic entries.
Author Contributions
TB developed any new code, including those implementing SMCA and SCCA, carried out all the numerical analyses and created all the figures for the paper. GD and subsequently TB originated the ideas of SMCA and SCCA, respectively. TB proved the equivalence of the correlation coefficient of CCA with a scalar target and the coefficient of multiple correlation. TB proposed the breaking down of the change of the correlation coefficients into three constituents according to the linear regression model. TB devised the statistical test for nonergodicity of correlations motivated by Axel Timmermann’s enquiry. GD, J-YL, E-SC suggested to calculate the differences as e.g., in Figure 1; and to evaluate the relative importance of ENSO variability as seen in Figure 8 developed and implemented by TB. TB and GD lead the interpretation of results and the writing of the manuscript. K-JH, J-YL, E-SC contributed to the manuscript.
Funding
TB was supported by the Institute for Basic Science (IBS), under IBS-R028-D1. G.D. acknowledges financial support from the Spanish State Research Agency through the María de Maeztu Program for Units of Excellence in R&D under grant no. MDM-2017-0711, from the National Research, Development and Innovation Office (NKFIH, Hungary) under grant nos. K125171 and FK-124256, and from the European Social Fund through the fellowship no. PD/020/2018 under the postdoctoral program of the Government of the Balearic Islands (CAIB, Spain). Support for the Twentieth Century Reanalysis Project dataset is provided by the U.S. Department of Energy, Office of Science Innovative and Novel Computational Impact on Theory and Experiment (DOE INCITE) program, and Office of Biological and Environmental Research (BER), by the National Oceanic and Atmospheric Administration Climate Program Office, and by the National Oceanic and Atmospheric Administration Climate Program Office, and by the NOAA Physical Sciences Laboratory.
Conflict of Interest
The authors declare that the research was conducted in the absence of any commercial or financial relationships that could be construed as a potential conflict of interest.
Acknowledgments
TB thanks Tímea Haszpra, Malte Stuecker, Kyung-Sook Yun for useful discussions regarding applying spatial weighting according to Baldwin et al. (2009), an appropriate domain for modal analysis using the SLP, using gridded observational precipitation data (CRU PRE) only but not unreliable reanalysis data, respectively, and Axel Timmermann for his interest in nonergodicity including a discussion on whether nonergodicity implies the nonlinear change of correlations and vice versa. GD thanks Claudia E. Wieners for useful discussions about the relationship between ENSO and the total variability of the Equatorial Pacific. TB thanks Keith Rodgers for informing him about the volume of Variations that includes (Haszpra et al., 2020b). GD is thankful to B. Stevens, T. Mauritsen, Y. Takano, and N. Maher for providing access to the output of the MPI-ESM ensembles. 20th Century Reanalysis V2 data provided by the NOAA/OAR/ESRL PSL, Boulder, Colorado, United States, from their Web site at https://psl.noaa.gov/. Free access to the CRU PRE data and the CERA 20C reanalysis product is gratefully acknowledged. The preprint of this paper (Bódai et al., 2020a) can be found at https://arxiv.org/abs/2009.02155. The authors acknowledge the constructive comments by the two reviewers, one of whom prompted the definition of TFSTVE.
Supplementary Material
The Supplementary Material for this article can be found online at: https://www.frontiersin.org/articles/10.3389/feart.2020.599785/full#supplementary-material.
Footnotes
1It is, therefore, instructive to study a multimodel ensemble of SMILEs.
2We do not regard the co-evolution of the ensemble means of e.g., Niño3 and the Indian summer monsoon rain to be part of the teleconnection, unlike Pandey et al. (2020), as these responses could be largely unrelated. Yet, using an infinite ensemble, the rank correlation coefficient with respect to the temporal variability, i.e., evolution, of the ensemble means is trivially 1. The prediction or projection of the Indian summer monsoon rain does not even need to rely on the Equatorial Pacific SST, but could be just facilitated by e.g., some extrapolation of the observed response of its own. That is, if there was reason to believe that we have a reliable estimate of the forced response.
3We emphasize that the bias that defines “nonergodicity” is based on the ideal ensemble mean, not a sample mean for a finite-size ensemble.
4Such a feature is absent in the CESM1-LE (Haszpra et al., 2020a).
5The case of the standard deviation, a statistic of basic interest giving a primary representation of internal variability, is already nontrivial, as nonstationarity would imply heteroscedasticity, in which case the reliable detection of nonstationarity is not straightforward. Assuming homoscedasticity wrongly, the estimates of standard errors could be either negatively or positively biased, and, therefore, a type II error can be made: not rejecting stationarity at a certain confidence level when the process is actually nonstationary.
6The quotation marks are used because the concept of a “snapshot” makes the best sense concerning a time-continuous evolution, whereas here we consider the discrete-time annual progression of a seasonal mean.
7Ensemble-wise EOFs, SEOFs, were used earlier in the context of probabilistic ensemble forecasting in e.g., (Zheng et al., 2013; Zheng et al., 2017; Zheng et al., 2019), in which case the concern was certainly not the forced response.
8It is also common to redefine PCs to be normalized and EOFs to be multiplied by the corresponding standard deviations.
9Although, the rationale behind defining simple indices is such that we want to work with a simple-to-compute quantity (e.g., an areal average or difference between two locations) that correlates very strongly with the dominant variability in terms of EOFs, which latter can be seen to represent a more natural method of decomposing the variability of a field.
10If the same wider box is used with the SLP as with the SST, the “weight” of the EOF1 is concentrated on parts of the perimeter of the box. Despite the dissimilarity of the EOF1s with the SLP in the two different boxes, the time dependence of the corresponding correlation coefficients r(PC1,AISMR) are actually very similar.
11Strictly speaking, each mode should be tested if it can be distinguished from noise and if it features characteristics associated with ENSO.
12As for the MCA, maximizing the unexplained covariance implies that the PCs are uncorrelated as reflected by the SVD decomposition.
13SST mask mode 1: https://youtu.be/2AVETrcfBVU
SST mask mode 2: https://youtu.be/EZQ7r2v7-zk
SST box mode 1: https://youtu.be/C62BKGVDQvQ
SST box mode 2: https://youtu.be/QgLQCbS3ZRU
SLP mask mode 1: https://youtu.be/HbxJjOz1RUA
SLP mask mode 2: https://youtu.be/fahPv_fLgx0
SLP box mode 1: https://youtu.be/PQHv7B8B2sw.
SLP box mode 2: https://youtu.be/74_ltkeOiqg.
14situation is not shown conclusively in (Haszpra et al., 2020a) because only three snapshots are shown belonging to single years, and it is not clear how much statistical error is associated with them, leaving an uncertainty about the locations of the peaks.
15This approximation assumes the correlated variables to follow a Gaussian distribution, but non-Gaussianity has been checked to have a farly negligible effect for some basic ENSO-quantifiers and AISMR in (Bódai et al., 2020b).
References
Aneesh, S., and Sijikumar, S. (2018). Changes in the La Niña teleconnection to the Indian summer monsoon during recent period. J. Atmos. Sol. Terr. Phys. 167, 74–79. doi:10.1016/j.jastp.2017.11.009
Baldwin, M. P., Stephenson, D. B., and Jolliffe, I. T. (2009). Spatial weighting and iterative projection methods for EOFs. J. Clim. 22, 234–243. doi:10.1175/2008JCLI2147.1
Björnsson, H., and Venegas, S. A. (1997). A manual for EOF and SVD analyses of climatic data. Tech. rep. 97 (1), 112–134.
Bódai, T., Drótos, G., Ha, K.-J., Lee, J.-Y., Haszpra, T., and Chung, E.-S. (2020a). Nonlinear forced change and nonergodicity: the case of ENSO-Indian monsoon and global precipitation teleconnections arXiv.2009.02155. Atmos. Oceanic Phys. Available at: https://arxiv.org/abs/2009.02155
Bódai, T., Drótos, G., Herein, M., Lunkeit, F., and Lucarini, V. (2020b). The forced response of the El niño–southern oscillation–Indian monsoon teleconnection in ensembles of earth system models. J. Clim. 33, 2163–2182. doi:10.1175/JCLI-D-19-0341.1
Bódai, T., and Tél, T. (2012). Annual variability in a conceptual climate model: snapshot attractors, hysteresis in extreme events, and climate sensitivity. Chaos: An Interdisciplinary J. Nonlinear Sci. 22, 023110. doi:10.1063/1.3697984
Carréric, A., Dewitte, B., Cai, W., Capotondi, A., Takahashi, K., Yeh, S.-W., et al. (2020). Change in strong Eastern Pacific El Niño events dynamics in the warming climate. Clim. Dynam. 54, 901–918. doi:10.1007/s00382-019-05036-0
Cochran, W. G. (1934). The distribution of quadratic forms in a normal system, with applications to the analysis of covariance. Math. Proc. Camb. Phil. Soc. 30, 178–191. doi:10.1017/S0305004100016595
Compo, G. P., Whitaker, J. S., Sardeshmukh, P. D., Matsui, N., Allan, R. J., Yin, X., et al. (2011). The Twentieth century reanalysis Project. Q. J. R. Meteorol. Soc. 137, 1–28. doi:10.1002/qj.776
Drótos, G., Bódai, T., and Tél, T. (2015). Probabilistic concepts in a changing climate: a snapshot attractor picture. J. Clim. 28, 3275–3288. doi:10.1175/JCLI-D-14-00459.1
Drótos, G., Bódai, T., and Tél, T. (2016). Quantifying nonergodicity in nonautonomous dissipative dynamical systems: an application to climate change. Phys. Rev. E 94, 022214. doi:10.1103/PhysRevE.94.022214
Drótos, G., Bódai, T., and Tél, T. (2017). On the importance of the convergence to climate attractors. Eur. Phys. J. Spec. Top. 226, 2031–2038. doi:10.1140/epjst/e2017-70045-7
Fisher, R. A. (1915). Frequency distribution of the values of the correlation coefficient in samples from an indefinitely large population. Biometrika 10, 507–521.
Franzke, C. L. E. (2014). Nonlinear climate change. Nat. Clim. Change 4, 423–424. doi:10.1038/nclimate2245
Gershunov, A., and Barnett, T. P. (1998). Interdecadal modulation of ENSO teleconnections. Bull. Am. Meteorol. Soc. 79, 2715–2726. doi:10.1175/1520-0477(1998)079<2715:IMOET>2.0.CO;2
Gershunov, A., Schneider, N., and Barnett, T. (2001). Low-frequency modulation of the ENSO–Indian monsoon rainfall relationship: signal or noise? J. Clim. 14, 2486–2492. doi:10.1175/1520-0442(2001)014<2486:LFMOTE>2.0.CO;2
Ghil, M., and Lucarini, V. (2020). The physics of climate variability and climate change. Rev. Mod. Phys. 92, 035002. doi:10.1103/RevModPhys.92.035002
Härdle, W., and Simar, L. (2007). Canonical correlation analysis. Berlin, Heidelberg: Springer Berlin Heidelberg, 321–330. doi:10.1007/978-3-540-72244-1_14
Harris, I. (2019a). [Dataset]: CRUTS v4.03 data variables: PRE. https://crudata.uea.ac.uk/cru/data/hrg/cru_ts_4.03/cruts.1905011326.Ωv4.03/pre/
Harris, I. (2019b). [Dataset]: Release notes for CRU TS v4.03. https://crudata.uea.ac.uk/cru/data/Ωhrg/cru_ts_4.03/Release_Notes_CRU_TS4.03.txt
Haszpra, T., Herein, M., and Bódai, T. (2020a). Investigating ENSO and its teleconnections under climate change in an ensemble view—a new perspective. Earth Syst Dyn. 11, 267–280. doi:10.5194/esd-11-267-2020
Haszpra, T., Topál, D., and Herein, M. (2020b). “Detecting forced changes in internal variability using Large Ensembles: on the use of methods based on the “snapshot view.” New research on climate variability and change using initial-condition Large Ensembles. Variations. Editors C. Deser, and K. Rodgers (Washington: UCAR), Vol. 18, 36–43.
Haszpra, T., Topál, D., and Herein, M. (2020c). On the time evolution of the arctic oscillation and related wintertime phenomena under different forcing scenarios in an ensemble approach. J. Clim. 33, 3107–3124. doi:10.1175/JCLI-D-19-0004.1
Heffernan, P. M. (1997). Unbiased estimation of central moments by using U-statistics. J. Roy. Stat. Soc. B 59, 861–863.
Herein, M., Márfy, J., Drótos, G., and Tél, T. (2016). Probabilistic concepts in intermediate-complexity climate models: a snapshot attractor picture. J. Clim. 29, 259–272. doi:10.1175/JCLI-D-15-0353.1
Kay, J. E., Deser, C., Phillips, A., Mai, A., Hannay, C., Strand, G., et al. (2015). The community Earth system model (CESM) large ensemble project: a community resource for studying climate change in the presence of internal climate variability. Bull. Am. Meteorol. Soc. 96, 1333–1349. doi:10.1175/BAMS-D-13-00255.1
Kim, B.-H., and Ha, K.-J. (2015). Observed changes of global and western Pacific precipitation associated with global warming SST mode and mega-ENSO SST mode. Clim. Dynam. 45, 3067–3075. doi:10.1007/s00382-015-2524-2
Krishnamurthy, L., and Krishnamurthy, V. (2014). Influence of PDO on South Asian summer monsoon and monsoon–ENSO relation. Clim. Dynam. 42, 2397–2410. doi:10.1007/s00382-013-1856-z
Krishnamurthy, V., and Goswami, B. N. (2000). Indian monsoon–ENSO relationship on interdecadal timescale. J. Clim. 13, 579–595. doi:10.1175/1520-0442(2000)013<0579:IMEROI>2.0.CO;2
Kumar, K. K., Rajagopalan, B., and Cane, M. A. (1999). On the weakening relationship between the Indian monsoon and ENSO. Science 284, 2156–2159. doi:10.1126/science.284.5423.2156
Laloyaux, P., de Boisseson, E., Balmaseda, M., Bidlot, J. R., Broennimann, S., Buizza, R., et al. (2018). CERA-20C: a coupled reanalysis of the twentieth century. J. Adv. Model. Earth Sys. 10 (5), 1172–1195. doi:10.1029/2018MS001273
Maher, N., Milinski, S., Suarez-Gutierrez, L., Botzet, M., Dobrynin, M., Kornblueh, L., et al. (2019). The Max Planck Institute Grand ensemble: enabling the exploration of climate system variability. J. Adv. Model. Earth Syst. 11, 2050–2069. doi:10.1029/2019MS001639
Meinshausen, M., Smith, S. J., Calvin, K., Daniel, J. S., Kainuma, M. L. T., Lamarque, J.-F., et al. (2011). The RCP greenhouse gas concentrations and their extensions from 1765 to 2300. Climatic Change 109, 213. doi:10.1007/s10584-011-0156-z
Mishra, V., Smoliak, B. V., Lettenmaier, D. P., and Wallace, J. M. (2012). A prominent pattern of year-to-year variability in Indian Summer Monsoon Rainfall. Proc. Natl. Acad. Sci. Unit. States Am. 109, 7213–7217. doi:10.1073/pnas.1119150109
North, G. R., Bell, T. L., Cahalan, R. F., and Moeng, F. J. (1982). Sampling errors in the estimation of empirical orthogonal functions. Mon. Weather Rev. 110, 699–706. doi:10.1175/1520-0493(1982)110<0699:SEITEO>2.0.CO;2
Pandey, P., Dwivedi, S., Goswami, B. N., and Kucharski, F. (2020). A new perspective on ENSO-Indian summer monsoon rainfall relationship in a warming environment. Clim. Dynam. 55, 3307–3326. doi:10.1007/s00382-020-05452-7
Parthasarathy, B., Munot, A. A., and Kothawale, D. R. (1994). All-India monthly and seasonal rainfall series: 1871–1993. Theor. Appl. Climatol. 49, 217–224. doi:10.1007/BF00867461
Power, S. B., and Delage, F. P. D. (2018). El Niño–Southern Oscillation and associated climatic conditions around the world during the latter half of the twenty-first century. J. Clim. 31, 6189–6207. doi:10.1175/JCLI-D-18-0138.1
Quadrelli, R., Bretherton, C. S., and Wallace, J. M. (2005). On sampling errors in empirical orthogonal functions. J. Clim. 18, 3704–3710. doi:10.1175/JCLI3500.1
Santer, B. D., Fyfe, J. C., Solomon, S., Painter, J. F., Bonfils, C., Pallotta, G., et al. (2019). Quantifying stochastic uncertainty in detection time of human-caused climate signals. Proc. Natl. Acad. Sci. Unit. States Am. 116, 19821–19827. doi:10.1073/pnas.1904586116
Storch, H. V., and Zwiers, F. W. (1999). Statistical analysis in climate research. Cambridge, United Kingdom: Cambridge University Press. doi:10.1017/CBO9780511612336
Takahashi, K., Montecinos, A., Goubanova, K., and Dewitte, B. (2011). ENSO regimes: reinterpreting the canonical and modoki El Niño. Geophys. Res. Lett. 38. doi:10.1029/2011GL047364
Tél, T., Bódai, T., Drótos, G., Haszpra, T., Herein, M., Kaszás, B., et al. (2019). The theory of parallel climate realizations. J. Stat. Phys. 179 (5–6), 1496–1530. doi:10.1007/s10955-019-02445-7
Timmermann, A. (1999). Detecting the nonstationary response of ENSO to greenhouse warming. J. Atmos. Sci. 56, 2313–2325. doi:10.1175/1520-0469(1999)056<2313:DTNROE>2.0.CO;2
Topál, D., Ding, Q., Mitchell, J., Baxter, I., Herein, M., Haszpra, T., et al. (2020). An internal atmospheric process determining summertime arctic sea ice melting in the next three decades: lessons learned from five large ensembles and multiple CMIP5 climate simulations. J. Clim. 33, 7431–7454. doi:10.1175/JCLI-D-19-0803.1
Torrence, C., and Webster, P. J. (1999). Interdecadal changes in the ENSO–monsoon system. J. Clim. 12, 2679–2690. doi:10.1175/1520-0442(1999)012<2679:ICITEM>2.0.CO;2
Vega-Westhoff, B., and Sriver, R. L. (2017). Analysis of ENSO’s response to unforced variability and anthropogenic forcing using CESM. Sci. Rep. 7, 18047. doi:10.1038/s41598-017-18459-8
Wang, B., Xiang, B., Li, J., Webster, P. J., Rajeevan, M. N., Liu, J., et al. (2015). Rethinking Indian monsoon rainfall prediction in the context of recent global warming. Nat. Commun. 6, 7154. doi:10.1038/ncomms8154
Watanabe, T., and Yamazaki, K. (2014). Decadal-scale variation of South Asian summer monsoon onset and its relationship with the pacific decadal oscillation. J. Clim. 27, 5163–5173. doi:10.1175/JCLI-D-13-00541.1
Yettella, V., Weiss, J. B., Kay, J. E., and Pendergrass, A. G. (2018). An ensemble covariance framework for quantifying forced climate variability and its time of emergence. J. Clim. 31, 4117–4133. doi:10.1175/JCLI-D-17-0719.1
Yun, K.-S., and Timmermann, A. (2018). Decadal monsoon-ENSO relationships reexamined. Geophys. Res. Lett. 45, 2014–2021. doi:10.1002/2017GL076912
Zheng, M., Chang, E. K. M., and Colle, B. A. (2013). Ensemble sensitivity tools for assessing extratropical cyclone intensity and track predictability. Weather Forecast. 28, 1133–1156. doi:10.1175/WAF-D-12-00132.1
Zheng, M., Chang, E. K. M., Colle, B. A., Luo, Y., and Zhu, Y. (2017). Applying fuzzy clustering to a multimodel ensemble for U.S. East coast winter storms: scenario identification and forecast verification. Weather Forecast. 32, 881–903. doi:10.1175/WAF-D-16-0112.1
Keywords: ENSO-Indian monsoon teleconnection, forced response, ensemble, ergodicity, snapshot attractor, maximum covariance analysis, canonical correclation analysis
Citation: Bódai T, Drótos G, Ha K-J, Lee J-Y and Chung E-S (2021) Nonlinear Forced Change and Nonergodicity: The Case of ENSO-Indian Monsoon and Global Precipitation Teleconnections. Front. Earth Sci. 8:599785. doi: 10.3389/feart.2020.599785
Received: 28 August 2020; Accepted: 22 December 2020;
Published: 05 April 2021.
Edited by:
Wen Chen, Institute of Atmospheric Physics (CAS), ChinaReviewed by:
Debashis Nath, Sun Yat-sen University, ChinaEduardo Zorita, Helmholtz Centre for Materials and Coastal Research (HZG), Germany
Copyright © 2021 Bódai, Drótos, Ha, Lee and Chung. This is an open-access article distributed under the terms of the Creative Commons Attribution License (CC BY). The use, distribution or reproduction in other forums is permitted, provided the original author(s) and the copyright owner(s) are credited and that the original publication in this journal is cited, in accordance with accepted academic practice. No use, distribution or reproduction is permitted which does not comply with these terms.
*Correspondence: Tamás Bódai, Ym9kYWlAcHVzYW4uYWMua3I=