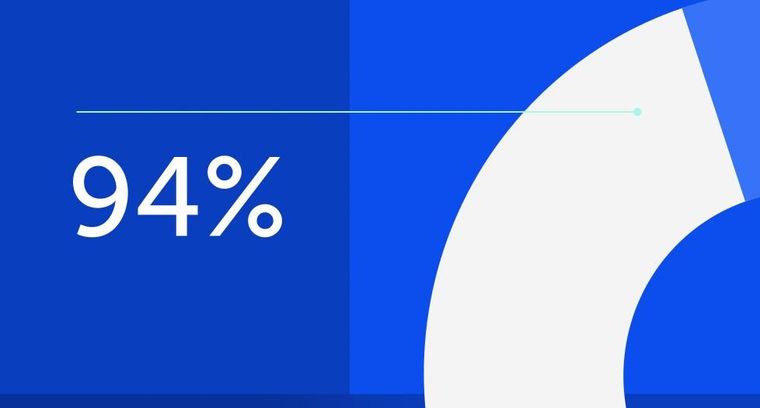
94% of researchers rate our articles as excellent or good
Learn more about the work of our research integrity team to safeguard the quality of each article we publish.
Find out more
ORIGINAL RESEARCH article
Front. Earth Sci., 06 August 2020
Sec. Solid Earth Geophysics
Volume 8 - 2020 | https://doi.org/10.3389/feart.2020.00321
This article is part of the Research TopicThe Power of Citizen Seismology: Science and Social ImpactsView all 16 articles
In this citizen science project, we ask citizens to listen to relevant sections of seismograms that are converted to audible frequencies. Citizen scientists helped identify local seismic events whose recorded signals are much smaller than those associated with the surface waves that have triggered these local events. The local events include small earthquakes as well as tectonic tremor. While progress has been made in understanding how these events might be triggered by surface waves from large teleseismic earthquakes around the world, there is no consensus on its physical mechanism. The aim of our project is to engage the help of citizen scientists to increase general knowledge of triggered seismic events that may or may not occur during transient strain changes, such as from propagating surface waves. A better understanding of triggered seismic events is expected to provide important clues toward a fundamental understanding of how earthquakes nucleate and the physical mechanisms that connect different earthquakes and other slip events. From the volunteers’ classifications we determined that citizen scientists achieve a higher reliability in detecting earthquakes and noise than in detecting tremor or other signals and that citizen scientists more accurately identify earthquake signals than a trained machine-learning algorithm. For tremor classifications we currently depend entirely on humans as no machine has yet learned to detect triggered tremor.
Surface waves generally have the longest duration and largest displacement of all seismic waves. When they pass through a seismically active region, surface waves from distant earthquakes may locally trigger an earthquake or tremor (Miyazawa and Mori, 2005; Gomberg et al., 2008; Rubinstein et al., 2009; Chao et al., 2012; Ide, 2012). Determining the frequency and conditions under which triggered seismic events occur will lead to a better understanding of the dynamic triggering of earthquakes (Peng and Gomberg, 2010; Brodsky and van der Elst, 2014). Seismometers continuously record ground motion at stations around the world, including seismic waves of small events which may be detected at one or at multiple instrument locations. Due to the large number of seismometers, the available seismograms are too numerous to be examined by seismologists (Liang et al., 2016). With Earthquake Detective, we utilize the Zooniverse platform to engage citizen scientists in an experiment to test if many human ears and eyes can replace the process of a professional seismologist in identifying dynamically triggered seismic events. We focus on data from seismic stations in Alaska, including USArray stations of EarthScope. Our approach has three advantages: (1) The human ear naturally performs a time-frequency analysis and is capable of discerning a wide range of different signals (Zwicker, 1961), (2) many human ears listening to the same data provides statistics that rank seismograms in order of their likelihood to contain a recording of a local event, which is helpful to researchers’ analysis of this data (Kilb et al., 2012), and (3) part of the citizen scientists’ responses can be compared to the results of a machine-learning algorithm to assess their performance.
Different seismic events can be classified by citizen scientists when listening to the audio data alongside the visual graphs. When sufficient data is classified, seismologists and data scientists can use it to train a machine-learning algorithm (an example of artificial intelligence) to automate the classification of seismograms (Xing et al., 2003; Perol et al., 2018; Tang et al., 2020). From there, seismic models for how, where, when, and why earthquakes happen may be refined by seismologists. The work citizen scientists put into this project contributes to the fundamental understanding of our planet that will allow a more sustainable society by allowing professionals to better assess hazards from future seismic events. An electronic supplement provides details on interface diagrams of the project and portions of data utilized.
Far-field surface waves of large magnitude earthquakes can dynamically trigger seismic events such as small, local earthquakes (Prejean et al., 2004) and tectonic tremor (Peng and Gomberg, 2010). Here, we address results from the citizen scientists’ classifications of data from USArray (TA) and the Alaska Regional Network (AK), which were recorded in the US from 2013 to 2018 (see section “Acknowledgments and Data” for details). The seismic waveforms presented to citizen scientists are downloaded from the IRIS (Incorporated Research Institutions for Seismology) Data Management System (DMS) (see section “Acknowledgments and Data”). The downloaded waveforms (Figure 1A) have a start time of 60 minutes before and an end time of 180 minutes after the origin times of selected large earthquakes with moment magnitude (Mw) greater than 7.5 (Table 1; Aiken and Peng, 2014; Chao and Obara, 2016). Waveforms were converted to ground velocity by deconvolving the instrument response from the recorded waveforms, and rotated to radial, transverse and vertical components (Figure 1B). The waveforms are then band-pass filtered between 2 and 8 Hz (Figure 1C) to remove Rayleigh waves from the radial and vertical components and Love waves from the transverse component. After determining the beginning of the surface-wave window for each station based on its distance from the epicenter and using a group velocity of 4.5 km/s, we selected the first 2000 s of the time series after this start time (Figure 1D). We generated audio files by speeding up the time series by a factor of 800 and applying an arctangent function to the amplitudes for dynamic-range compression (Figure 1E). This provides improved audibility for signals with smaller amplitude while preventing events with larger amplitude signals from excessive loudness. Waveforms with either gaps in the time series, calibrations or re-centering signals, or other glitches were discarded before presenting the data to citizen scientists on the largest people-powered research platform, “Zooniverse” (Supplementary Figures S1–S3). With this platform, we were able to provide tutorial and practice sessions for training our citizen scientists to identify “earthquakes,” “tremor,” and “noise” signals. Citizen scientists are asked to choose “none of the above” when the seismic signals do not clearly fall in one of the other categories or more than one different signal is present in the data (Supplementary Figures S4, S5).
Figure 1. Data processing. (A) Raw seismogram from MPM station following the February 27, 2010 Chile earthquake with Mw8.8. (B) Seismogram converted to velocity by removing the instrument response. (C) Seismogram after applying a filter from 2 to 8 Hz. (D) Seismogram is cut for the surface-wave interval. (E) Generating audio files by speeding up the time series and scaling the amplification.
Seismic waves that are caused by the displacement of tectonic plates along a fault are known as earthquake signals. They are caused by the sudden release of seismic energy, making them short in duration and resembling the sound of a slamming door. Tremors have a longer duration and are generated by a slow release of acoustic and seismic energy. Sped up to audible frequencies, tremor can sound like a train darting over railroad tracks.
The Earth is in constant motion under the influence of forces from atmosphere, hydrosphere (e.g., ocean currents and waves), and biosphere, including anthropogenic activity, generated by traffic or industry, for example. Therefore, every seismogram contains relatively steady noise, even in the absence of seismic signals or distinct noise events, which converts to a slowly varying, white noise “baseline” for the sound file. These noise signals sound like whistling wind, crinkling aluminum foil, or radio static.
Earthquakes and tremors as well as natural and anthropogenic sources generate seismic signals that may or may not exceed the baseline noise level of a seismogram. These different sources can be distinguished by the sound of their signals.
Of 2467 seismograms recorded by the AK network, 1103 seismograms were classified as earthquakes by citizen scientists, 141 as tremor, 770 as noise, and 228 were labeled as to pertaining to none of these categories. The distribution of classifications in the four categories (Figure 2) indicates that earthquakes (74% of all classifications on seismograms identified as earthquakes are made for this category) and noise (66%) were identified with more certainty by citizen scientists than tremor (50%) and other, unclear events (51%). Hence, citizen scientists were able to classify earthquakes and noise more consistently than tremor and other events.
Figure 2. Histograms for each classified category. The four categories contain seismograms which have received a majority classification for one category and show the average of the classifications made for the seismograms contained in this category.
For one Mw7.5 earthquake on December 5, 2018, seismologists independently classified the seismograms for which 7 of 10 citizen scientists agreed, in order to assess the accuracy of the project volunteers. For comparison, we applied a machine-learning (ML) algorithm, trained to detect earthquake signals only (Tang et al., 2020), and compared its output with our expert labels as well. Assuming that the expert labels are “true,” citizen scientists’ labels were 85% accurate in classifying earthquakes and did not mislabel any seismogram without earthquakes though 23% of all earthquakes remained undetected by citizen scientists (Figure 3). Figure 4 shows results from ML as projections into two-dimensional spaces via the PCA (Principal Component Analysis) of 10-dimensional embeddings. PCA is a non-parametric statistical technique (George and Vidyapeetham, 2012) used for dimensionality reduction in machine learning and the principal components are the coefficients of orthogonal linear combinations of the variables in the dataset. Contours indicate the distributions of the training dataset and the symbols represent the testing dataset. The machine-learning algorithm achieved only 76.2% accuracy in classifying earthquakes in the same dataset, a score nearly 10% lower than citizen scientists (Figure 4).
Figure 3. Confusion matrices for citizen scientists’ and the machine-learning algorithm’s classifications.
Figure 4. The convolutional neural network for classifying the training dataset (contour maps) and the test dataset (symbols) from the December 5, 2018 Mw7.5 teleseimic earthquake. Shown in red are seismograms with an earthquake, seismograms without earthquakes are shown in blue.
Seismograms are retired after having been classified by 10 different users on Zooniverse. Of 2467 seismograms, 2242 have received a conclusive label, meaning that the number of classifications made for one category is not reached for any other category as shown by a narrow distribution. There was a larger level of agreement between volunteers when the seismographs contained either earthquakes or noise (Figure 2). Citizen scientists agree less on which seismograms contain tremor and other signals as shown by a wider distribution of classifications (Figure 5). We assume that the degree of agreement of classifications between citizen scientists reflects the collective confidence of citizen scientists in identifying the seismic signals. We found that earthquakes and noise have characteristic waveforms and associated audio signals that make it easy to distinguish them from other seismic signals. Citizen scientists are directed to classify seismic signals as not pertaining to any of the other categories when the seismograms contain several different signals or have unclear waveforms or audio signals. In these situations the seismograms are often classified as an earthquake or tremor. It is therefore unsurprising that the agreement of classifications made on seismograms in the category “none of the above” is lower than on seismograms in the other categories.
Figure 5. Histograms for classifications made on seismograms without conclusive label. The seismograms contained in this category have received the same amount of classifications for at least two different categories with less classifications for the other categories.
The 225 seismograms which have not received a conclusive label (Figure 5), meaning that the highest amount of classifications has been reached for more than one category, amount to only 9% of all seismograms. Unsurprisingly, the distribution of classifications made on these seismograms shows no clear preference for any of the categories. However, it stands out that classifications for tremor and “none of the above” are more numerous than for earthquakes and noise, reflecting that these seismic signals are more difficult to identify and confirming the affirmations made for seismograms with a conclusive label. This may bias citizen scientists (Hart et al., 2009; Swanson et al., 2016) to classify seismograms with “none of the above” events as earthquakes, tremor or noise. These “none of the above” events reflect that seismograms within the surface wave intervals may contain instrument signals, and signals of anthropogenic and natural sources (Smith and Tape, 2019).
The classifications made by citizen scientists of Zooniverse make it possible to locate the stations with additional seismic signals that occurred during the passage of surface waves of teleseismic earthquakes in the AK network (Figures 6–8). Surface waves from the earthquake on December 5, 2018 with Mw 7.5 southeast of the Loyalty Islands triggered local earthquakes within 300 km north of Anchorage, (Figure 6). During the passage of surface waves from the September 8, 2017 Mw 8.2 Mexico earthquake, tremor occurred in central Alaska (Figure 7). The signals recorded during the passage of surface waves from the September 28, 2018 Mw 7.5 Sulawesi earthquake (Figure 8) show a random mix of classifications by citizen scientists, implying that signals are present, but are ambiguous in nature.
Figure 6. Map of stations showing the location of recordings of triggered earthquake for different teleseismic earthquakes.
Figure 7. Map of stations showing the locations of recordings of triggered tremor for different teleseismic earthquakes.
Figure 8. Map of stations showing the locations of recordings where no triggered seismicity (noise) occurred for different teleseismic earthquakes.
The focus on this study has been on harnessing the intelligence of citizen scientists to identify triggered seismic events. In the subtask of detecting triggered earthquakes, we compared the results of citizen scientists to an existing machine-learning algorithm (Tang et al., 2020). The confusion matrices in Figure 3 show that the machine-learning algorithm misidentified 11 of the expert-labeled non-earthquake signals as earthquake signals and missed 9 of the expert-labeled earthquakes, while correctly labeling 47 earthquake and 17 non-earthquake signals. On the other hand, citizen scientists correctly identified 43 earthquakes and missed 13 earthquake signals, while correctly labeling 28 non-earthquake signals. From the above results, both methods can successfully identify triggered earthquakes, but citizen scientists can detect non-earthquake signals better than the machine-learning algorithm. Citizen scientists are more successful at identifying non-earthquake signals because we encourage them to classify seismograms without clear earthquake signals as “none of above,” and the same standard used by seismologists to label the data. However, the machine-learning algorithm may identify triggered earthquakes hidden by high background noise as positive examples (Figure 9). Hence, the algorithm detects 11 more earthquake signals than seismologists.
Over 2000 citizen scientists helped classify more than 2000 seismograms from 30 large worldwide earthquakes with magnitudes over 7.5 in the citizen science project “Earthquake Detective” on Zooniverse. Citizen scientists generally agree more with each other when identifying (1) seismograms with earthquake signals and (2) the absence of distinct signals (noise) than when identifying tremor or other signals. A subset of data we also classified by experts (seismologists among the authors) and a machine-learning algorithm trained to detect triggered earthquakes (Tang et al., 2020). We compared these classifications from a machine-learning algorithm, citizen scientists and seismologists with each other and with the earthquake classifications of citizen scientists. We found that citizen scientists did not misidentify seismograms without an earthquake (no false positives) but missed 13 earthquake signals in seismograms (false negatives), while correctly labeling 43 earthquake and 28 non-earthquake signals. The machine-learning algorithm misidentified 11 non-earthquake signals and failed to detect 9 earthquake signals in seismograms, while correctly labeling 47 earthquake and 17 non-earthquake signals. Both the citizen scientists and the machine-learning algorithm perform well in identifying earthquakes, but the citizen scientists outperformed the machine-learning algorithm in labeling non-earthquake signals. Earthquake Detectives and a machine-learning algorithm experience similar degrees of difficulties for example in identifying other seismic signals, which are more challenging and requires more intelligence than identifying earthquakes, even though citizen scientists are currently better at both.
All seismic data were downloaded through the IRIS web service (https://service.iris.edu/irisws/) using ObsPy (https://docs.obspy.org), including the following seismic networks (http://ds.iris.edu/mda): USArray (2003) and Alaska Earthquake Center (1987). The ANSS (Advanced National Seismic System) earthquake catalog can be accessed at https://earthquake.usgs.gov/data/comcat/.
The datasets generated for this study are available on request to the corresponding author.
Ethical review and approval was not required for the study on human participants in accordance with the local legislation and institutional requirements. Written informed consent from the participants’ legal guardian/next of kin was not required to participate in this study in accordance with the national legislation and the institutional requirements.
VT, BR, and SL conceived and designed the project. VT organized the database of seismic data. VT, BR, JN, and SL developed the project. JT and MP contributed miscellaneous support for project development, management, presentation, and strategy. KC contributed to data collection and critical discussions of triggered seismic events. VT, BR, and SL wrote the first draft of the manuscript and figures. All authors contributed to the revision of the manuscript, read and approved the submitted version.
The authors declare that the research was conducted in the absence of any commercial or financial relationships that could be construed as a potential conflict of interest.
This research was funded by the Integrated Data-Driven Discovery in Earth and Astrophysical Sciences (ID3EAS) program under National Science Foundation grant NSF-NRT 1450006.
We thank Zhigang Peng and Laura Trouille for critical discussions. We also sincerely thank over 2,000 citizen scientists for important contributions to Earthquake Detective on Zooniverse.
The Supplementary Material for this article can be found online at: https://www.frontiersin.org/articles/10.3389/feart.2020.00321/full#supplementary-material
Aiken, C., and Peng, Z. (2014). Dynamic triggering of microearthquakes in three geothermal/volcanic regions of California. J. Geophys. Res. Solid Earth 119, 6992–7009. doi: 10.1002/2014jb011218
Brodsky, E. E., and van der Elst, N. J. (2014). The uses of dynamic earthquake triggering. Annu. Rev. Earth Planet. Sci. 42, 317–339. doi: 10.1146/annurev-earth-060313-054648
Chao, K., and Obara, K. (2016). Triggered tectonic tremor in various types of fault systems of Japan following the 2012 Mw8. 6 Sumatra Earthquake. J. Geophys. Res. Solid Earth 121, 170–187. doi: 10.1002/2015jb012566
Chao, K., Peng, Z., Fabian, A., and Ojha, L. (2012). Comparisons of triggered tremor in California. Bull. Seismol. Soc. Am. 102, 900–908. doi: 10.1785/0120110151
George, A., and Vidyapeetham, A. V. (2012). Anomaly detection based on machine learning: dimensionality reduction using PCA and classification using SVM. Int. J. Comput. Appl. 47, 5–8. doi: 10.5120/7470-0475
Gomberg, J., Rubinstein, J. L., Peng, Z., Creager, K. C., Vidale, J. E., and Bodin, P. (2008). Widespread triggering of nonvolcanic tremor in California. Science 319, 173–173. doi: 10.1126/science.1149164
Hart, W., Albarracín, D., Eagly, A. H., Brechan, I., Lindberg, M. J., and Merrill, L. (2009). Feeling validated versus being correct: a meta-analysis of selective exposure to information. Psychol. Bull. 135, 555–588. doi: 10.1037/a0015701
Ide, S. (2012). Variety and spatial heterogeneity of tectonic tremor worldwide. J. Geophys. Res. Solid Earth 117:B03303.
Kilb, D., Peng, Z., Simpson, D., Michael, A., Fisher, M., and Rohrlick, D. (2012). Listen, watch, learn: SeisSound video products. Seismol. Res. Lett. 83, 281–286. doi: 10.1785/gssrl.83.2.281
Liang, W. T., Chen, K. H., Wu, Y. F., Yen, E., and Chang, C. Y. (2016). Earthquake school in the cloud: citizen seismologists in Taiwan. Seismol. Res. Lett. 87, 177–185. doi: 10.1785/0220150061
Miyazawa, M., and Mori, J. (2005). Detection of triggered deep low-frequency events from the 2003 Tokachi-oki earthquake. Geophys. Res. Lett. 32:L10307.
Peng, Z., and Gomberg, J. (2010). An integrated perspective of the continuum between earthquakes and slow-slip phenomena. Nat. Geosci. 3, 599–607. doi: 10.1038/ngeo940
Perol, T., Gharbi, M., and Denolle, M. (2018). Convolutional neural network for earthquake detection and location. Sciencances 3:e1700578. doi: 10.1126/sciadv.1700578
Prejean, S. G., Hill, D. P., Brodsky, E. E., Hough, S. E., Johnston, M. J. S., and Malone, S. D. (2004). Remotely triggered seismicity on the United States west coast following the Mw 7.9 Denali fault earthquake. Bull. Seismol. Soc. Am. 94, S348–S359.
Rubinstein, J. L., Gomberg, J., Vidale, J. E., Wech, A. G., Kao, H., Creager, K. C., et al. (2009). Seismic wave triggering of nonvolcanic tremor, episodic tremor and slip, and earthquakes on Vancouver Island. J. Geophysics. Res. 114:B00A01.
Smith, K., and Tape, C. (2019). Seismic noise in central Alaska and influences from rivers, wind, and sedimentary basins. J. Geophys. Res. Solid Earth 124, 11678–11704. doi: 10.1029/2019jb017695
Swanson, A., Kosmala, M., Lintott, C., and Packer, C. (2016). A generalized approach for producing, quantifying, and validating citizen science data from wildlife images. Conserv. Biol. 30, 520–531. doi: 10.1111/cobi.12695
Tang, V., Seetharaman, P., Chao, K., Pardo, B. A., and van der Lee, S. (2020). Automating the detection of dynamically triggered earthquakes via a deep metric learning algorithm. Seismol. Res. Lett. 91, 901–912.
Xing, E. P., Jordan, M. I., Russell, S. J., and Ng, A. Y. (2003). “Distance metric learning with application to clustering with side-information,” in Advances in Neural Information Processing Systems, 521–528.
Keywords: earthquake detective, citizen sciences, triggered seismic events, machine-learning algorithm, audible pitches, Alaska
Citation: Tang V, Rösler B, Nelson J, Thompson J, van der Lee S, Chao K and Paulsen M (2020) Citizen Scientists Help Detect and Classify Dynamically Triggered Seismic Activity in Alaska. Front. Earth Sci. 8:321. doi: 10.3389/feart.2020.00321
Received: 12 February 2020; Accepted: 09 July 2020;
Published: 06 August 2020.
Edited by:
Kate Huihsuan Chen, National Taiwan Normal University, TaiwanReviewed by:
Chi-Chia Tang, China University of Geosciences, ChinaCopyright © 2020 Tang, Rösler, Nelson, Thompson, van der Lee, Chao and Paulsen. This is an open-access article distributed under the terms of the Creative Commons Attribution License (CC BY). The use, distribution or reproduction in other forums is permitted, provided the original author(s) and the copyright owner(s) are credited and that the original publication in this journal is cited, in accordance with accepted academic practice. No use, distribution or reproduction is permitted which does not comply with these terms.
*Correspondence: Vivian Tang, dml2aWFuQGVhcnRoLm5vcnRod2VzdGVybi5lZHU=; dml2aWFudGFuZzIwMTVAdS5ub3J0aHdlc3Rlcm4uZWR1
Disclaimer: All claims expressed in this article are solely those of the authors and do not necessarily represent those of their affiliated organizations, or those of the publisher, the editors and the reviewers. Any product that may be evaluated in this article or claim that may be made by its manufacturer is not guaranteed or endorsed by the publisher.
Research integrity at Frontiers
Learn more about the work of our research integrity team to safeguard the quality of each article we publish.