- 1School of Environmental Sciences, University of Liverpool, Liverpool, United Kingdom
- 2Alaska Volcano Observatory, U.S. Geological Survey Volcano Science Center, Anchorage, AK, United States
- 3Alaska Volcano Observatory, Geophysical Institute, University of Alaska Fairbanks, Fairbanks, AK, United States
- 4School of Geography, Politics and Sociology, Newcastle University, Newcastle upon Tyne, United Kingdom
- 5Istituto Nazionale di Geofisica e Vulcanologia, Sezione di Pisa, Pisa, Italy
- 6Departamento de Fisica Teorica y del Cosmos, University of Granada, Granada, Spain
The injection of gas and pyroclastic material from volcanic vents into the atmosphere is a prolific source of acoustic waves. Infrasound arrays offer efficient, cost-effective, and near real-time solutions to track the rate and intensity of surface activity at volcanoes. Here, we present a simple framework for the analysis of acoustic array data, based on least-squares beamforming, that allows to evaluate the direction and speed of propagation of acoustic waves between source and array. The algorithms include a new and computationally efficient approach for quantitative assessment of the uncertainty on array measurements based on error propagation theory. We apply the algorithms to new data collected by two 6-element infrasound arrays deployed at Mt. Etna during the period July–August 2019. Our results demonstrate that the use of two infrasound arrays allowed detecting and tracking acoustic sources from multiple craters and active vents associated with degassing and ash-rich explosions, vigorous and frequent Strombolian activity, opening of new eruptive fractures and emplacement of lava flows. Finally, we discuss the potential use of metrics based on infrasound array analyses to inform eruption monitoring operations and early warning at volcanoes characterized by episodic intensification of activity.
1. Introduction
Over the past two decades infrasound has become an increasingly popular tool to monitor volcanoes (e.g., Fee and Matoza, 2013; McNutt et al., 2015). Because of its ability to detect and discriminate shallow and sub-aerial volcanic activity (Matoza et al., 2019) infrasound is a desirable complement to seismology for monitoring unrest, and to detect and track the evolution of eruptions in real- or near real-time (e.g., Ripepe et al., 2009, 2018; Cannata et al., 2013; Coombs et al., 2018) that is over time scales of the order of few seconds to few minutes. Acoustic waves are generated when the atmosphere is perturbed from equilibrium (e.g., Garcés et al., 2009). At volcanoes, small and large explosions, gas-and-ash jets and plumes, sector collapses, rockfalls, pyroclastic flows, and lahars, are likely to generate infrasound over a wide range of magnitudes, frequencies, and durations (Johnson and Ripepe, 2011; Fee and Matoza, 2013; Johnson and Palma, 2015; McNutt et al., 2015; Matoza and Fee, 2018). Infrasound is recorded by band-sensitive microphones at different scales (Fee and Matoza, 2013) from local (<20 km) to regional (several 10s to few 100s of km), and even global (several 100s to 1,000s of km).
At the local scale, microphones are deployed either as individual sensors within distributed networks or as small-aperture arrays (i.e., tight clusters of three or more instruments at 50–150 m from one another) at distances of between few hundreds of meters to within 20 km of an active volcanic vent. Data from distributed networks have traditionally been used for absolute source location and event discrimination (e.g., Cannata et al., 2009), and to evaluate eruption source parameters (e.g., Caplan-Auerbach et al., 2010; Fee et al., 2017; De Angelis et al., 2019; Diaz-Moreno et al., 2019). On the other hand, the close spacing between sensors deployed as a small-aperture array allows detection of coherent infrasound even in low signal-to-noise ratio (SNR) conditions, characterization of the direction-of-arrival (DOA), and the apparent speed of propagation of acoustic waves. Notably, the use of infrasound arrays even at distances of a few tens of kilometers from active vents provides robust and efficient remote detection of eruptive activity and source discrimination (e.g., Fee et al., 2010), thus reducing risks for observatory personnel during field campaigns. Johnson (2004) and Ripepe et al. (2007) discussed applications of infrasound array methods to track degassing and eruptive activity from multiple active vents at Stromboli volcano (Italy). Ripepe et al. (2018) reported on over a decade of acoustic monitoring at Mt. Etna (Italy) with two small aperture infrasound arrays, demonstrating the ability to detect eruption onset in real-time, discriminate source position, and dispatch rapid notifications of the ongoing activity to local civil protection authorities. Matoza et al. (2007) analyzed array data during activity at Mt. St. Helens (USA) from October 2004 to March 2005; they discussed the key role of infrasound in separating surface from deeper processes and in identifying the timing and assessing the magnitude of eruptive events.
Previous studies focussing on (local scale) infrasound array applications to volcano monitoring do not provide quantitative estimates of the true uncertainty associated with their measurements. In this manuscript we tackle this issue presenting a simple framework for infrasound array processing based on least-squares beamforming (Olson and Szuberla, 2005; Haney et al., 2018) and introducing a new scheme for quantitative assessment of the uncertainty on estimates of DOA and apparent velocity. We test the method on data collected by two arrays deployed at Mt. Etna (Italy) during the summer of 2019 (Figure 1). We describe the array processing workflow, and show how infrasound data can be used to discriminate and track volcanic activity, from background degassing to individual ash-rich explosions, persistent Strombolian activity and lava effusion.
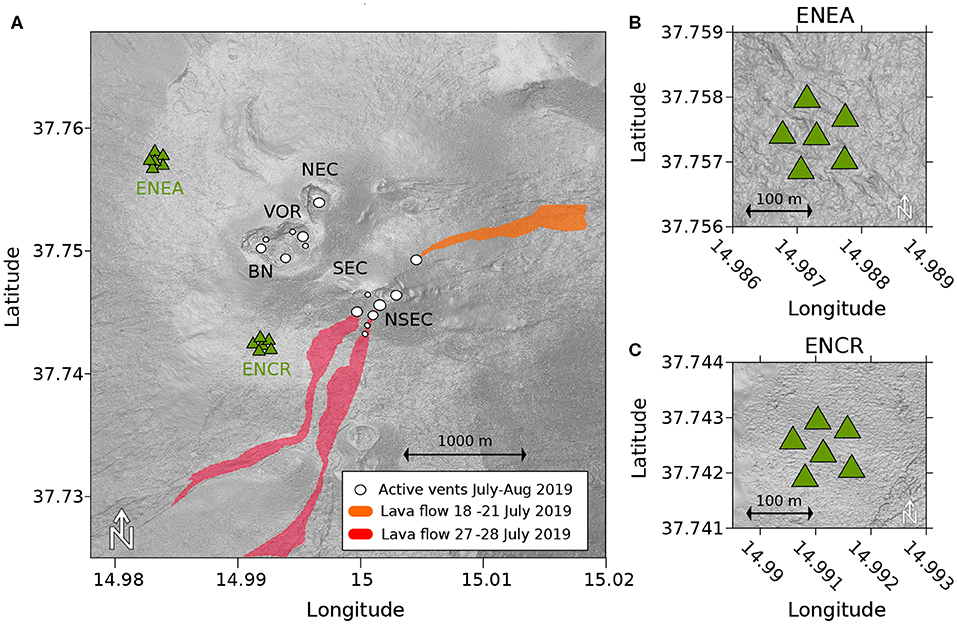
Figure 1. (A) Map showing: (1) the locations of two infrasound arrays (green triangles), ENEA and ENCR, deployed at Mt. Etna, Italy, during July–August 2019; (2) approximate locations of vents active in the summit area (white circles of variable size according to vent dimensions as qualitatively inferred from visual observations) during the deployment period; (3) active lava flows during the deployment period. (B,C) Details of the geometry of the two infrasound arrays, ENEA and ENCR.
2. Activity at Mt. Etna: July–August 2019
Mt. Etna, Italy, is one of the most active volcanoes in the world. Several eruptive episodes have taken place in the past two decades, at its summit, from the North East (NEC), Voragine (VOR), Bocca Nuova (BN), and New South East (NSEC) craters. Since 2011, more than 50 effusive events have taken place in the area, which is visited by thousands of tourists every year (e.g., De Beni et al., 2019; Sciotto et al., 2019). During the last decade lava fountaining has frequently been observed from the NSEC, as well as shorter episodes of Strombolian activity from BN, VOR, and the NEC (Sciotto et al., 2019). Eruption at Etna occasionally evolves into episodes of more intense activity referred to as paroxysms that is, a significant increment in the rate and intensity of explosions from one or multiple active vents accompanied by emplacement of lava flows and/or generation of significant ash plumes. Activity at Mt. Etna during July–August 2019 was marked by the occurrence of two paroxysms on 18 and 27–28 July, accompanied by the emplacement of lava flows from lateral vents in the NSEC area (Figure 1). The weeks preceding each paroxysm were characterized by both sustained and explosive degassing occasionally punctuated by ash-rich explosions (Figures 2A,B). The Istituto Nazionale di Geofisica e Vulcanologia (INGV) reported intense degassing and observations of four large explosions from the NEC on 2, 3, 8, and 13 July (Figure 2B). Degassing activity from VOR was low-to-moderate, whilst BN produced deep intra-crater gas explosions with minor amounts of ash (Figure 2A). Activity at the NSEC increased gradually from vigorous degassing to ash-rich Strombolian activity throughout the first week of July; degassing-only activity resumed on 7 July and escalated again into strong and nearly continuous Strombolian explosions on 18 July. Explosion rates varied from one every 1–2 min to several per minute, eventually leading to the opening of a fracture and subsequent emplacement of a lava flow on the NE flank of the NSEC (23:09 UTC on 18 July). During the night on 19 July, Strombolian activity shifted from NSEC to NEC, and gradually decreased until it completely halted—including lava flow effusion—in the morning of 20 July.
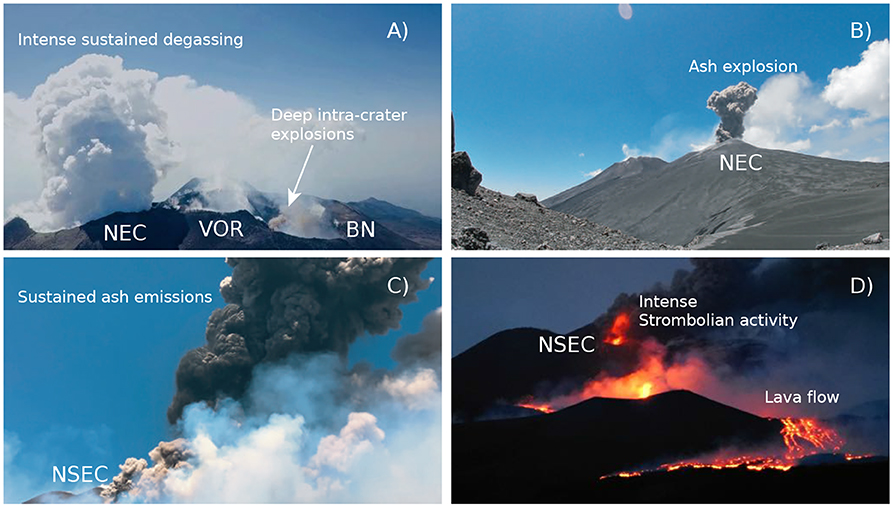
Figure 2. Images of eruptive activity at Mt. Etna, Italy, during July–August 2019: (A) Intense sustained degassing activity from the North East Crater (NEC) and deep intra-crater gas-rich explosions from Bocca Nuova (BN) recorded during a UAV flight on 5 July 2019 at 08:00 UTC (Photo credit: A. Diaz-Moreno, University of Liverpool); (B) Ash-rich explosion from NEC on 3 July 2019 at 10:06 UTC (Photo credit: A. Diaz-Moreno, University of Liverpool); (C) Explosive activity accompanied by sustained ash emissions observed on 27 July 2019 at 12:21 UTC from the New South East crater (NSEC) area (Photo credit: Giuseppe Di Stefano/Marco Restivo, Etna Walk); (D) Intense Strombolian activity and lava flow originating from a vent in the NSEC area on 27 July 2019 (Photo credit: INGV-Osservatorio Etneo).
On 25 July, a new phase of eruption began at the NSEC with the onset of Strombolian activity, transitioning into nearly continuous lava fountaining early in the morning of 27 July. This activity was accompanied by sustained ash emissions (Figures 2C,D) just a few hours before the opening of two new vents on the southern flank of the NSEC from which two new lava flows developed (Figure 1). A strong explosion occurred on July 27 at 12:21 UTC, accompanied by an ash plume that reached nearly 4 km above the vent (Figure 2C). Strombolian activity ceased on 28 July at 03:40 UTC, while lava flows continued until late that day. The remainder of our deployment period was characterized by background levels of degassing from NSEC, VOR, and BN, while NEC continued producing episodic ash explosions during August.
INGV reported no major changes in deformation during the 2 months of our temporary deployment, while daily gas emissions increased notably during 15–29 July and then returned to background levels. Seismic tremor fluctuated with marked peaks on 6 July, coincident with the increase in Strombolian activity at NSEC. Seismic tremor remained stable, at high levels, during both paroxysms, and eventually returned to background during August. Finally, infrasound locations provided by INGV successfully identified the first paroxysm on July 18, but high wind conditions on 27–28 July prevented the network from detecting the second paroxysm. The activity recorded during the summer of 2019 is typical at Mt. Etna; other eruptions with a similar fingerprint have been reported in the past (Corsaro et al., 2017; De Beni et al., 2019; Polacci et al., 2019).
3. Data
In this study, we used data recorded by two 6-element infrasound arrays (ENEA and ENCR, Figure 1), deployed at Mt. Etna between 2 July and 25 August 2019. Changes in eruptive activity during this period, summarized in section 2 of this manuscript, are reported in the INGV activity bulletins and were confirmed by both Unmanned Aerial Vehicle (UAV) imagery (Figure 2A) and ground-based visual observations (Figures 2B–D) gathered during the deployment by the authors. The ENEA and ENCR arrays were installed at distances of between 1 and 1.8 km from the active vents (Figure 1). Each had similar configurations, deployed with an ~100 m aperture (i.e., the largest distance between any two elements within an array) pentagon shape with a central element. Care was taken, considering the constraints imposed by topography, that the difference in elevation between any two microphones within each array was small; for both arrays, this difference did not exceed ~30 m (see Methods section for additional details). The arrays were designed to have apertures large enough to discriminate acoustic arrivals and small enough to record coherent infrasound across all microphones (Ripepe et al., 2007). Data from both arrays were recorded on-site with a sampling frequency of 100 Hz at 24-bit resolution using DiGOS Data-cube digitizers (https://digos.eu/seismology-and-cubes/); ENEA was equipped with Chaparral Model 60 Ultra High Pressure microphones (full-scale range of 2000 Pa peak-to-peak, flat response between 0.03 and 245 Hz), and ENCR with IST2018 sensors (full-scale range of 480 Pa peak-to-peak, flat response between 0.1 and 40 Hz, as described in Grangeon and Lesage, 2019). Pressure amplitudes recorded by the two arrays ranged from few Pa for signals associated with both sustained and explosive degassing, to several tens of Pa during intense and persistent Strombolian activity. Examples of multi-channel data recorded by both ENEA and ENCR are shown in Figures 3A–E; these include an ash-rich explosion from NEC (Figure 3C) and repeated deep intra-crater explosions from BN (Figure 3D) on 2 July 2019, as well as Strombolian activity from NSEC on 18 July 2019 (Figure 3E). It is worth noting how infrasound waveforms in Figures 3B,E exhibit a marked asymmetry, although the investigation of this intriguing feature falls beyond the scopes of this manuscript.
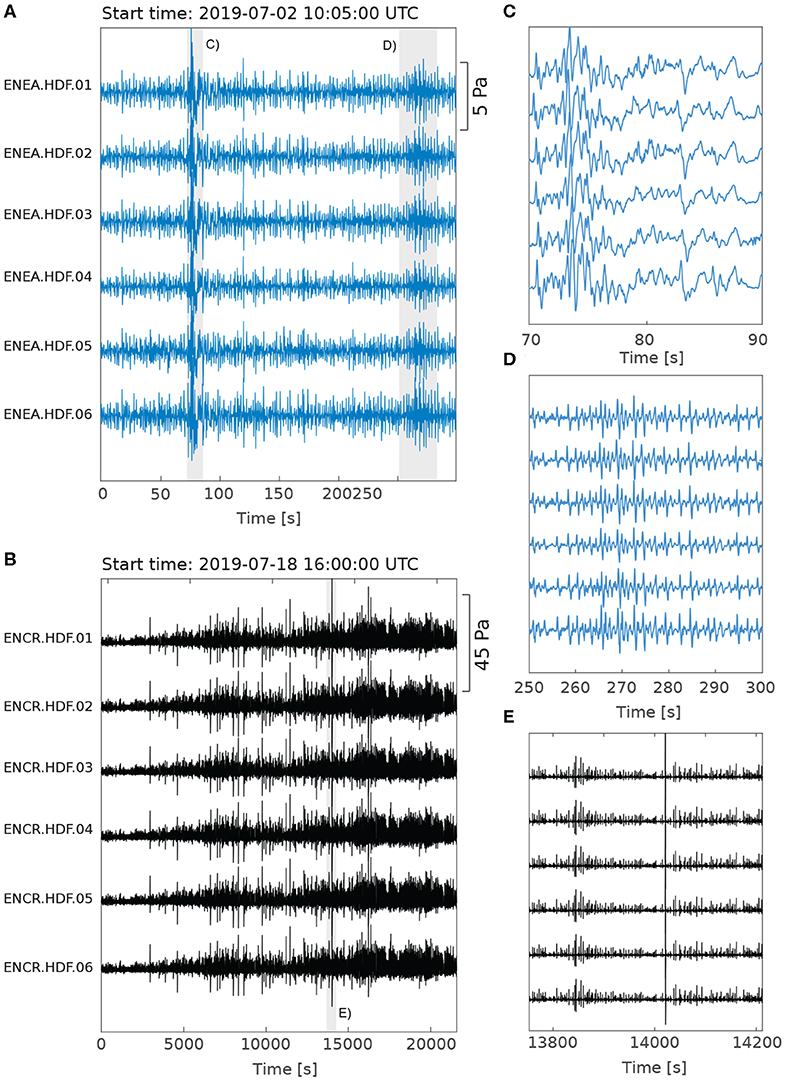
Figure 3. Infrasound data recorded by the ENEA (blue) and ENCR (black) arrays during July 2019: (A) 5 min of continuous infrasound activity recorded by ENEA on 2 July 2019; (B) 6 h of intense Strombolian activity recorded by ENCR on 18 July 2019; (C,D) Detail (gray shaded areas in A) of ENEA infrasound associated with an ash-rich explosion from the NEC and deep intra-crater gas explosions from BN, respectively; (E) Detail (gray shaded area in B) of ENCR infrasound recorded during vigorous Strombolian activity on 18 July 2019. Note the difference in infrasound amplitudes between signals in (A,B).
4. Methods
Here we discuss an array processing workflow to derive estimates of DOA and horizontal velocity from volcano infrasound array data. The method solves, via least-squares inversion, the problem of fitting a plane wave arrival traveling from an azimuth θ with horizontal velocity v to a vector of measured time delays between traces across the array (e.g., Claerbout, 1986; Olson and Szuberla, 2005; Haney et al., 2018). We also introduce a new method to quantify errors on the measurements of θ and v using standard propagation of error. For an array of n sensors, a data vector d = (δt1, δt2, …, δtN) of N = n(n − 1)/2 delay times between the elements of the array can be estimated, for instance using waveform cross-correlation; a linear relationship exists between d and a model vector, of slowness (defined as the inverse of velocity) in the East-West (sx) and North-South (sy) directions:
where G is a Nx2 matrix of horizontal distances between all pairs of array elements. When the measurements of delay times are affected by error, Equation (1) can be re-written to explicitly include it as:
where ϵ is the vector of errors in the estimates of time delays. These errors can be assumed to be normally distributed, with zero mean and variance (Olson and Szuberla, 2005). The solution to (2) for m is then found by minimizing the sum of squared errors:
that is:
Finally, DOA and apparent trace velocity across the array can be estimated from the solution vector m as:
It is important to note that the beamforming analysis in (1–5) is carried out in the horizontal plane (Edwards and Green, 2012), that is under the assumption that the contribution of differences in sensor elevations to the time delay measurements can be neglected; for this reason it is crucial that such differences are small.
For the most part, studies that apply array processing to volcano infrasound data either provide qualitative statements on the uncertainty associated with estimates of DOA and trace velocity or discuss the theoretical azimuthal resolution of the array in terms of its aperture in relation to the wavelength of the signals analyzed (e.g., Ripepe et al., 2018). The statistical confidence in the estimates of θ and v are rarely discussed (e.g., Szuberla and Olson, 2004); precise estimates of DOA and trace velocity are made difficult by the ubiquitous presence of noise in the data and the complex propagation of the acoustic wavefield, which affect measurements of time delays across arrays. Under the assumption that errors on the measurements of time delays across an array are normally distributed, with zero mean and variance , the deviation of m from its expected value, due to errors in the estimates of time delays, is described by the model covariance matrix, ℂ(m), given by:
The matrix ℂ(⋗) is symmetrical and can be written in terms of the variances (, ) and covariance () of sx and sy:
Equations (6) and (7) provide a link between the variances and covariance of sx and sy, and the variance of time delay measurements, that is:
We are interested in error estimates on DOA and trace velocity, which are non-linear functions of sx and sy as shown in (5). In order to estimate the variances for θ and v ( and , respectively) we apply standard propagation of errors theory (e.g., Vardeman and Jobe, 2001) to (5). Neglecting high-order terms we obtain:
The terms in (9) are obtained by simply differentiating (5) and evaluating them at the values for sx and sy given by the solution of (4), that is:
Finally, the standard deviations of trace velocity and DOA, σv and σθ, are obtained by taking the square root of (10).
5. Results
We applied the array processing workflow described in section 4 of this manuscript to continuous infrasound data recorded at Mt. Etna between 2 July 2019 and 25 August 2019. Data collected by two infrasound arrays, ENEA and ENCR, were pre-processed by applying a band-pass (2-pole, zero-phase) filter within the frequency band of interest, which for the activity recorded during our experiment was between 0.7 and 15 Hz. The delay times between pairs of sensors across each array were determined using cross-correlation with sub-sample accuracy (e.g., Haney et al., 2018) within 10-s sliding windows overlapping by 5 s. DOA and trace velocity were calculated for all data windows by least-squares inversions according to (1–5), and their variances were calculated according to (7–9). The mean of the normalized cross-correlation maxima (MCCM) within each data window reflects the level of coherence between signals across the array; only values of DOA and trace velocity corresponding to MCCM > 0.5 were considered to ensure that inversion was performed for coherent signals across the arrays.
Examples of estimates of DOA and trace velocity, along with their uncertainties, are shown in Figures 4, 5. In Figure 4 we show data recorded at ENEA on 2 July 2019 (the only array installed at this time). Figure 5 illustrates results from both ENEA and ENCR for just over 2 days of activity starting on 18 July 2019. These examples are representative of the variable activity, during July–August 2019, from multiple vents (Figure 1) and across all summit craters at Mt. Etna. Figure 4 shows stable locations in the direction of BN associated with deep intra-crater explosions (Figures 2A, 3D) and a sharp change in DOA at about 10:06 UTC, which corresponds to a larger ash-rich explosion from the NEC (Figures 2B, 3C); trace velocities are of the order of 330–335 m/s with standard deviations of 5–15 m/s, and uncertainties on DOA estimates of 0.5–2° (≈10–40 m at the distance between ENEA and the vent). Locations in Figure 5 are dominated by activity at NSEC, in particular between 18:00 and 24:00 on 18 July and during 12:00–19:00 on 19 July, when frequent and intense Strombolian activity was observed at this crater (Figures 3B,E). Values of trace velocities at ENEA during this period are of the order of 310–335 m/s, with uncertainties of 5–15 m/s and standard deviations on DOA between 1 and 2° (≈30–60 m); at ENCR, trace velocities are in 330–335 m/s range with uncertainties of 2–8 m/s, and uncertainties on DOA of 0.5–2° (≈10–40 m). Notably, both arrays detected a shift in activity in the direction of the NEC, also reported by INGV at about 23:00 on 19 July (section 2).
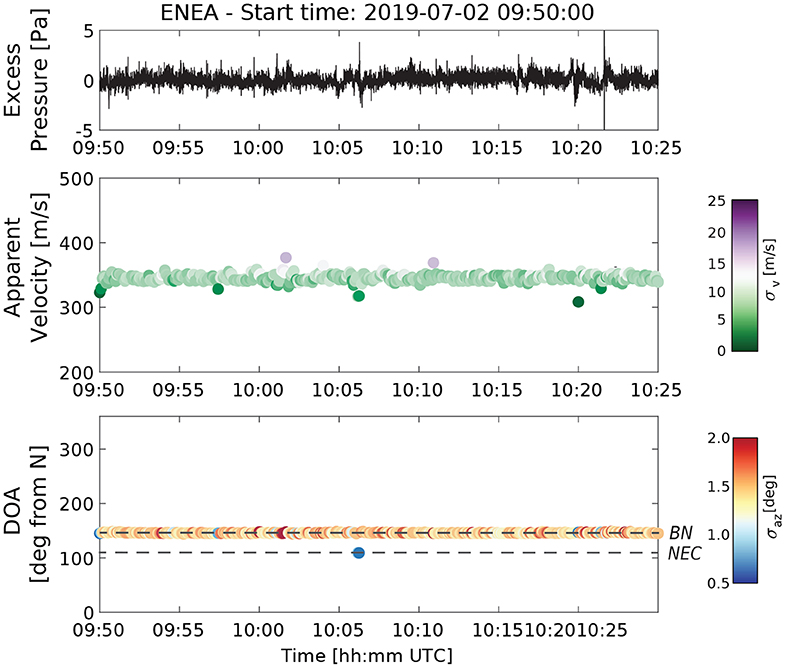
Figure 4. Array processing of 35 min of data recorded at ENEA on 2 July 2019 between 9:50 and 10:25 UTC. Note (bottom panel) consistent detection of deep intra-crater activity from BN briefly interrupted by a larger ash-rich explosion from NEC (see section 2).
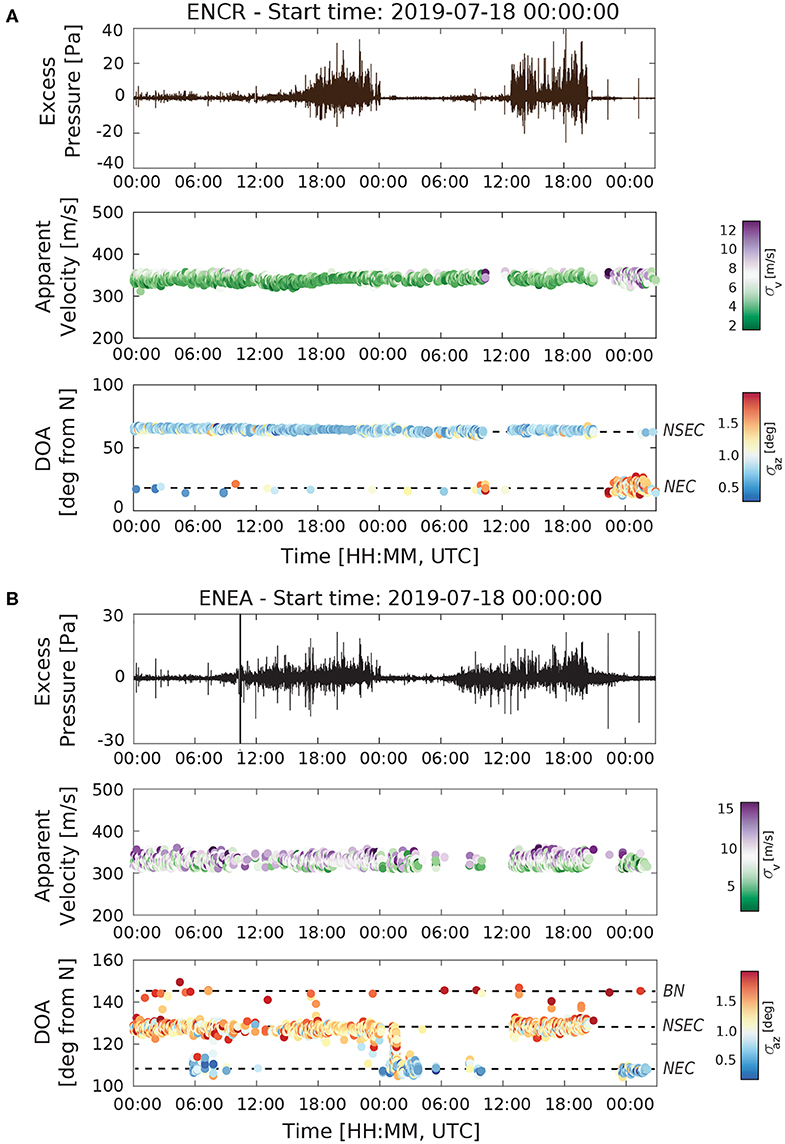
Figure 5. Array processing of 51 h of data recorded at ENEA and ENCR, starting on 18 July 2019, 00:00 UTC: (A) ENCR shows stable detections during Strombolian activity (18:00–24:00 on 18 July and 12:00–22:00 on 19 July) as well as during a period of lava effusion from the NE flank of NSEC (23:09 on 18 July, see section 2 in this manuscript); (B) ENEA captures the most intense Strombolian activity from the NSEC but shows larger fluctuations in DOA (direction of arrival) than ENCR. This is due to less favorable signal-to-noise ratio for activity at the NSEC as well as its proximity to secondary sources within the BN crater. Both arrays detect a clear shift in activity from NSEC to NEC late during the night between 19 and 20 July. Note how uncertainties and scatter in locations are consistently smaller at the array closer to the active vent.
The two arrays were installed to provide the best coverage of all active craters at Mt. Etna (within limitations imposed by site access and safety). Owing to their positions relative to the active vents ENEA was ideally located to independently discriminate activity from NEC, whereas ENCR was positioned to optimize detection of activity from the NSEC area (Figure 1). In Figure S1, we have provided an additional example of array locations showing the stability and low uncertainties associated with detections at ENCR during a period of Strombolian activity, lava fountaining and sustained ash emissions at the NSEC on 27–28 July 2019 (see section 2 in this manuscript, Figures 2C,D).
6. Discussion and Conclusions
We have presented an algorithm for the inversion of infrasound array data that allows estimating DOA with apparent sound velocity, and their associated uncertainties. We have applied the workflow to data collected by two 6-element infrasound arrays deployed at Mt. Etna during the summer of 2019; continuous detections from the two arrays tracked the activity observed at the volcano, including shifts in degassing and eruptions across multiple summit craters and vents. The results presented in Figures 4, 5, suggest that: (i) array location relative to a complex system of active vents is key to allow discrimination of variable activity across multiple craters, and (ii) the quality of detection and thus the final estimates of uncertainties are crucially influenced by SNR levels. Figure 5, for instance, shows higher quality (i.e., lower uncertainties) detections from ENEA for activity located at the NEC, while ENCR seems better suited to track eruptions in the NSEC area; ENEA is also able, albeit with comparatively large uncertainties, to track small intra-crater explosions from BN (Figure 5B) even during periods of elevated Strombolian activity at the NSEC. At the first order, this observation arguably reflects variable SNR at the two sites, resulting from the interplay between array proximity to the source (NEC and BN closer to ENEA; NSEC closer to ENCR) and wind noise levels (qualitatively observed to be consistently higher at ENEA). Wind strength and direction are factors that can potentially introduce a bias in the estimates of DOA and trace velocity from infrasound arrays (e.g., Schwaiger et al., 2020). At large source-receiver distances time reversal location of infrasound sources, propagating the acoustic wavefield in a windy atmosphere, has been used to assess the misfit between known source backazimuths and DOA estimates (e.g., Lonzaga, 2016). At the local scale, Johnson et al. (2012) demonstrated how temporal variations in acoustic parameters, such as infrasound travel times over short distances—<20 km—can be exploited to infer atmospheric conditions, including the strength of wind in a vertically stratified atmosphere. In theory, appropriate corrections for the effect of wind on infrasound measurements could be introduced in array inversion workflows; the main challenge for this lies in the fact that wind measurements or models with the required temporal and spatial resolution for the local scale are generally not available. In addition to wind noise, uncertainty on estimates of DOA and sound velocity are further linked to array configuration and its position relative to the sources; these factors control the degree to which the measured time delays across the array correspond to a physically realizable set of delays associated with the propagation of a plane wave across the array (Szuberla and Olson, 2004). Qualitatively, the plane wave approximation is considered valid at source-to-array distances much greater than the aperture of the array (e.g., Almendros et al., 1997), a condition met in our study for all source-array combinations. Finally, effects from topography, such as diffraction can affect acoustic propagation and introduce additional bias in array estimates of DOA and sound speed. At the local scale Fee et al. (2019) recently investigated the effects of topography on back-projection locations of volcano infrasound sources integrating improved travel time calculations from finite difference modeling of the acoustic wavefield. At Mt. Etna, specifically, Diaz-Moreno et al. (2019) performed 3D finite difference simulation of acoustic wavefield propagation; their results suggest that the effects of topography on acoustic propagation from sources in the area of the summit craters to the locations of the arrays used in this study are minor.
A quantitative estimate of how well the measured time delays correspond to a plane wave crossing the array is directly provided by the a priori variance of the time delay measurements, which is given by (Szuberla and Olson, 2004):
where (following the same notation of Equations 1–10) , with 𝕀 being the identity matrix and R = G[(GTG)−1 − 1]GT; N is the number of station pairs and r is the rank of R. We calculated for periods with high MCMM > 0.5; values were of the order of 10−2 s, that is ≈ 1/fs (with fs being the data sampling frequency) as also reported in other studies (e.g., Szuberla and Olson, 2004). This gives confidence that the plane wave assumption does not introduce significant bias on the results of our array analysis. On the other hand for data with low MCMM, that is no coherent signal traveling across the array, the values of were very high, up to a few seconds.
In our workflow, uncertainties on apparent velocity and DOA are evaluated using the theory of error propagation. While the variances estimated using this method are a first order approximation to the true uncertainty, this solution is easy to implement with very low computational overhead, an obvious advantage for deployment within real-time volcano monitoring systems. An exact solution for the statistical confidence in the estimates of DOA and signal velocity for planar arrays (i.e., without significant variations in elevation across the array) is discussed in Szuberla and Olson (2004). We benchmarked the results of our workflow against this solution and found good agreement between the two approaches (Figure S2).
Our study also reveals that combining estimates of DOA from multiple arrays improves the ability to discriminate multiple active vents. The ENEA and ENCR arrays were ideally positioned to independently discriminate sources within the NEC and NSEC, respectively (Figure 1). In the absence of complementary observations, however, resolving activity from variable vents within the other two summit craters (BN and VOR) frequently requires joint measurements from two arrays; combining the DOAs obtained from both arrays, a source location could be uniquely identified (Figure S3). Finally, we note that our workflow implicitly assumes a single arrival within each signal window analyzed. At volcanoes with multiple vents separated by a small distance different sources could be simultaneously active, and thus, produce multiple arrivals within a signal analysis window. This is discussed, for example, by Yamakawa et al. (2018) at Stromboli; the authors demonstrate how the MUSIC algorithm can help to resolve multiple active sources. We find that when active vent are separated by comparatively large distances and have different characteristic dimensions, such as Mt. Etna, appropriate selection of the signal analysis window and filters still allows effective source discrimination.
A key advantage of using infrasound arrays over individual microphones is that with a single data processing step multi-element arrays can provide information on source location, as well as the levels of volcanic activity in terms of both signal amplitude and rates of detection. This is an ideal scenario to inform early warning and assessment of alert levels during period of unrest accompanied by elevated surface activity at volcanoes. Ripepe et al. (2018) proposed an efficient way to combine the output of infrasound array processing into a single metric, the Infrasound Parameter, calculated as the product IP = Ap · Nd between the mean infrasound amplitude, Ap, and the number of array detections per minute, Nd. They calculated IP at Mt. Etna for a continuous period of about 8 years, and discussed the implementation of an alert color code used to dispatch early warnings of impending paroxysmal activity to the local civil protection authority. Here, we estimated a modified IP during July-August 2019 by taking the product between the mean of the maximum signal amplitude within each array detection window over a minute and the number of detections per minute. For this calculation we selected only high-quality detection windows, corresponding to MCMM > 0.5. The results for both arrays during the entire deployment period are shown in Figures 6A,B. Figures 6C,D show details of IP during 17–21 July 2019, when Strombolian activity escalated at the NSEC from one explosion every 1–2 min to several events within a minute, eventually leading to the opening of a fracture and emplacement of a lava flow on the NE flank of the NSEC late during the night on 18 July. The temporal evolution of the IP parameter represents changes in surface activity; Ripepe et al. (2018) reported that this behavior, typical of eruptions at Mt. Etna, reflects the transition from rapid Strombolian explosions, driven by the repeated ascent of gas slugs in the shallow conduit, to a churn flow regime when gas discharge increases and the eruption becomes sustained. The pattern observed in Figures 6C,D suggests that IP, when appropriately calibrated, may provide a valuable metric to monitor escalating surface activity, inform changes in alert color codes, and issue early warnings. While its use has been, thus far, limited to monitor activity at Mt. Etna, it may also be applicable at other basaltic volcanoes characterized by periodic occurrence of paroxysms.
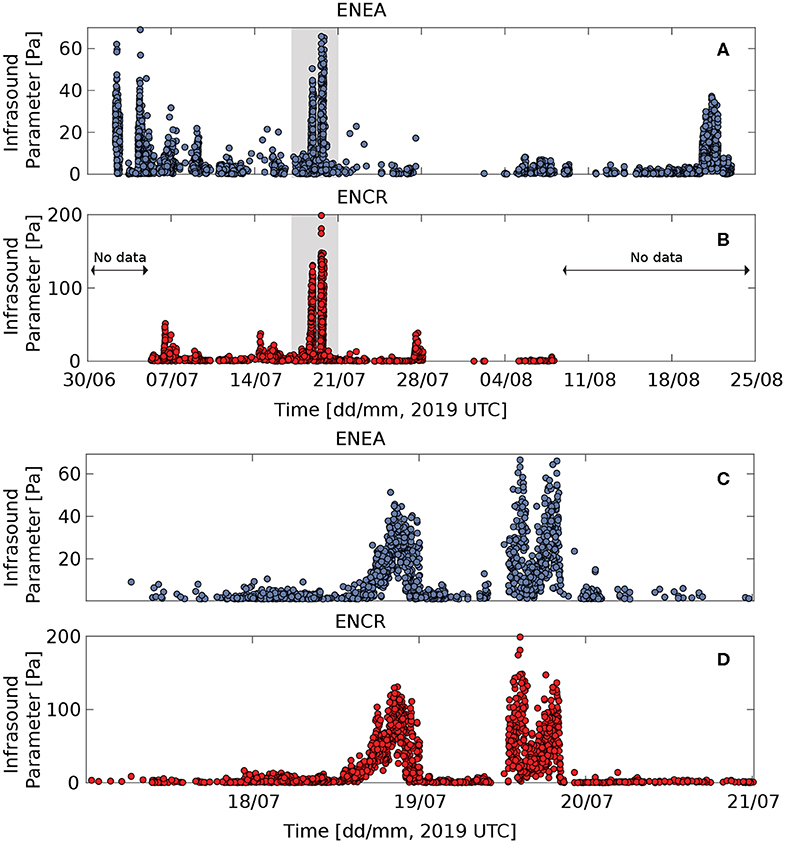
Figure 6. One-minute Infrasound Parameter during July–August 2019 for arrays ENEA (A) and ENCR (B); periods when no data were available are also indicated. Detail (zoom in of gray areas in A,B) of Infrasound Parameter values at ENEA (C) and ENCR (D) for the period 17–21 July 2019. The figure shows an obvious increase in both occurrence (i.e., increasing array detections per minute) and intensity (increasing signal amplitude) of Strombolian activity; the Infrasound Parameter peaks in coincidence with the opening of a new fracture and emplacement of a lava flow on the NE flank of the NSEC, late during the night on 18 July (see sections 2 and 6).
In conclusion, we have presented a framework for the inversion of infrasound array data to provide rapid estimates of source location and apparent sound velocity during periods of elevated volcanic activity. Our algorithms include a new, and computationally efficient, procedure for quantitative assessment of the uncertainties on array measurements, which is particularly well-suited for real-time implementation. We applied the proposed workflow to data gathered at Mt. Etna during July–August 2019. Our results demonstrate that infrasound arrays allowed detection and tracking of variable activity from multiple active vents at Mt. Etna. Owing to the fact that infrasound propagates efficiently over large distances (e.g., Fee and Matoza, 2013), we suggest that this data analysis framework may also hold potential to monitor eruptive activity at the regional scale (i.e., source-array distances of up to several hundreds of km), in particular in remote areas where local monitoring of individual volcanoes is not viable (Fee et al., 2019). We have further discussed a simple metric derived from infrasound array analyses that may be suitable to inform monitoring operations and form the basis to issue early warnings of impending paroxysms at basaltic volcanoes. We surmise that infrasound offers a simple and effective tool to track the temporal evolution of volcanic activity and to assist with real-time volcano monitoring, as well as the potential to inform models of the processes that control degassing and eruption at volcanoes.
Data Availability Statement
The data analyzed for this study are available via direct request to the authors or from the Natural Environment Research Council Geoscience Data Centre (https://www.bgs.ac.uk/services/ngdc/accessions/index.html?). A software implementation of the algorithms presented in this paper, along with example data, can be downloaded from: https://github.com/silvioda/Infrasound-Array-Processing-Matlab.
Author Contributions
SD, AD-M, and LZ conducted the field experiment. SD, MH, AW, JL, and DF developed the workflow for inversion and uncertainty calculations. AD-M produced the map in Figure 1 based on activity bulletins from INGV. SD produced all other figures with input from all co-authors and wrote the manuscript with input from all co-authors. All authors initiated the study and guided the investigation.
Funding
AD-M was supported by NERC grant NE/P00105X/1. LZ has also received funding from the European Union's Horizon 2020 research and innovation programme under the Marie Sklodowska-Curie grant agreement No. 798480. SD was partly supported by a Geoscientists without Borders grant from the Society of Exploration Geophysics.
Conflict of Interest
The authors declare that the research was conducted in the absence of any commercial or financial relationships that could be construed as a potential conflict of interest.
Acknowledgments
SD and LZ acknowledge the support from the EUROVOLC project under the European Union's Horizon 2020 research and innovation programme (grant agreement No. 731070). The authors thank the staff of the Istituto Nazionale di Geofisica e Vulcanologia Sezione di Catania, in particular Salvo Rapisarda, Daniele Pellegrino, Mario Pulvirenti, and Danilo Contrafatto for their valuable support in the field.
Supplementary Material
The Supplementary Material for this article can be found online at: https://www.frontiersin.org/articles/10.3389/feart.2020.00169/full#supplementary-material
References
Almendros, J., Ibanez, J. M., Alguacil, G., Del Pezzo, E., and Ortiz, R. (1997). Array tracking of the volcanic tremor source at deception island, antarctica. Geophys. Res. Lett. 24, 3069–3072. doi: 10.1029/97GL03096
Cannata, A., Di Grazia, G., Aliotta, M., Cassisi, C., Montalto, P., and Patané, D. (2013). Monitoring seismo-volcanic and infrasonic signals at volcanoes: Mt. Etna case study. Pure Appl. Geophys. 170, 1751–1771. doi: 10.1007/s00024-012-0634-x
Cannata, A., Montalto, P., Privitera, E., and Russo, G. (2009). Characterization and location of infrasonic sources in active volcanoes: Mount Etna, September–November 2007. J. Geophys. Res. 114:B08308. doi: 10.1029/2008JB006007
Caplan-Auerbach, J., Bellesiles, A., and Fernandes, J. K. (2010). Estimates of eruption velocity and plume height from infrasonic recordings of the 2006 eruption of Augustine Volcano, Alaska. J. Volcanol. Geotherm. Res. 189, 12–18. doi: 10.1016/j.jvolgeores.2009.10.002
Claerbout, J. F. (1986). Fundamentals of geophysical data processing, 2nd edn. Geophys. J. R. Astron. Soc. 86, 217–219. doi: 10.1111/j.1365-246X.1986.tb01085.x
Coombs, M. L., Wech, A. G., Haney, M. M., Lyons, J. J., Schneider, D. J., Schwaiger, H. F., et al. (2018). Short-term forecasting and detection of explosions during the 2016–2017 eruption of Bogoslof Volcano, Alaska. Front. Earth Sci. 6:122. doi: 10.3389/feart.2018.00122
Corsaro, R. A., Andronico, D., Behncke, B., Branca, S., Caltabiano, T., Ciancitto, F., et al. (2017). Monitoring the December 2015 summit eruptions of Mt. Etna (Italy): implications on eruptive dynamics. J. Volcanol. Geotherm. Res. 341, 53–69. doi: 10.1016/j.jvolgeores.2017.04.018
De Angelis, S., Diaz-Moreno, A., and Zuccarello, L. (2019). Recent developments and applications of acoustic infrasound to monitor volcanic emissions. Remote Sens. 11, 1–18. doi: 10.3390/rs11111302
De Beni, E., Cantarero, M., and Messina, A. (2019). UAVs for volcano monitoring: a new approach applied on an active lava flow on Mt. Etna (Italy), during the 27 February–02 March 2017 eruption. J. Volcanol. Geotherm. Res. 369, 250–262. doi: 10.1016/j.jvolgeores.2018.12.001
Diaz-Moreno, A., Iezzi, A., Lamb, O., Fee, D., Kim, K., Zuccarello, L., et al. (2019). Volume flow rate estimation for small explosions at Mt. Etna, Italy, from acoustic waveform inversion. Geophys. Res. Lett. 46, 11071–11079. doi: 10.1029/2019GL084598
Edwards, W. N., and Green, D. N. (2012). Effect of interarray elevation differences on infrasound beamforming. Geophys. J. Int. 190, 335–346. doi: 10.1111/j.1365-246X.2012.05465.x
Fee, D., Garces, M., and Steffke, A. (2010). Infrasound from tungurahua volcano 2006–2008: Strombolian to Plinian eruptive activity. J. Volcanol. Geotherm. Res. 193, 67–81. doi: 10.1016/j.jvolgeores.2010.03.006
Fee, D., Izbekov, P., Kim, K., Yokoo, A., Lopez, T., Prata, F., et al. (2017). Eruption mass estimation using infrasound waveform inversion and ash and gas measurements: evaluation at Sakurajima Volcano, Japan. Earth Planet. Sci. Lett. 480, 42–52. doi: 10.1016/j.epsl.2017.09.043
Fee, D., and Matoza, R. S. (2013). An overview of volcano infrasound: from Hawaiian to Plinian, local to global. J. Volcanol. Geotherm. Res. 249, 123–139. doi: 10.1016/j.jvolgeores.2012.09.002
Fee, D., Toney, L. D., Matoza, R. S., Sanderson, R. W., Haney, M. M., Lyons, J. J., et al. (2019). “A framework for improved infrasound back-projection on local and regional scales,” in AGU Fall Meeting Abstracts, Vol. 2019, S41E-0577 (San Francisco).
Garcés, M. A., Fee, D., and Matoza, R. (2009). Volcano acoustics. Model. Volcan. Process. Phys. Math. Volcan. 9780521895439, 359–383. doi: 10.1017/CBO9781139021562.016
Grangeon, J., and Lesage, P. (2019). A robust, low-cost and well-calibrated infrasound sensor for volcano monitoring. J. Volcanol. Geotherm. Res. 387:106668. doi: 10.1016/j.jvolgeores.2019.106668
Haney, M. M., Van Eaton, A. R., Lyons, J. J., Kramer, R. L., Fee, D., and Iezzi, A. M. (2018). Volcanic thunder from explosive eruptions at Bogoslof Volcano, Alaska. Geophys. Res. Lett. 45, 3429–3435. doi: 10.1002/2017GL076911
Johnson, J. B. (2004). Source location variability and volcanic vent mapping with a small-aperture infrasound array at Stromboli volcano, Italy. Bull. Volcanol. 67, 1–14. doi: 10.1007/s00445-004-0356-8
Johnson, J. B., Anderson, J., Marcillo, O., and Arrowsmith, S. (2012). Probing local wind and temperature structure using infrasound from Volcan Villarrica (Chile). J. Geophy. Res. Atmos. 117:D17107. doi: 10.1029/2012JD017694
Johnson, J. B., and Palma, J. L. (2015). Lahar infrasound associated with Volcán Villarrica's 3 March 2015 eruption. Geophys. Res. Lett. 42, 6324–6331. doi: 10.1002/2015GL065024
Johnson, J. B., and Ripepe, M. (2011). Volcano infrasound: a review. J. Volcanol. Geotherm. Res. 206, 61–69. doi: 10.1016/j.jvolgeores.2011.06.006
Lonzaga, J. B. (2016). Time reversal for localization of sources of infrasound signals in a windy stratified atmosphere. J. Acoust. Soc. Am. 139, 3053–3062. doi: 10.1121/1.4953017
Matoza, R., and Fee, D. (2018). The inaudible rumble of volcanic eruptions. Acoust. Today 14, 17–25. Available online at: https://acousticstoday.org/inaudible-rumble-volcanic-eruptions/
Matoza, R. S., Arciniega-Ceballos, A., Sanderson, R. W., Mendo-Pérez, G., Rosado-Fuentes, A., and Chouet, B. A. (2019). High-broadband seismoacoustic signature of vulcanian explosions at popocatépetl volcano, mexico. Geophys. Res. Lett. 46, 148–157. doi: 10.1029/2018GL080802
Matoza, R. S., Hedlin, M. A., and Garcés, M. A. (2007). An infrasound array study of Mount St. Helens. J. Volcanol. Geotherm. Res. 160, 249–262. doi: 10.1016/j.jvolgeores.2006.10.006
McNutt, S. R., Thompson, G., Johnson, J., Angelis, S. D., and Fee, D. (2015). Seismic and infrasonic monitoring. Encycl. Volcan. 2015, 1071–1099. doi: 10.1016/B978-0-12-385938-9.00063-8
Olson, J. V., and Szuberla, C. A. L. (2005). Distribution of wave packet sizes in microbarom wave trains observed in Alaska. J. Acoust. Soc. Am. 117, 1032–1037. doi: 10.1121/1.1854651
Polacci, M., Andronico, D., de' Michieli Vitturi, M., Taddeucci, J., and Cristaldi, A. (2019). Mechanisms of ash generation at basaltic volcanoes: the case of Mount Etna, Italy. Front. Earth Sci. 7:193. doi: 10.3389/feart.2019.00193
Ripepe, M., De Angelis, S., Lacanna, G., Poggi, P., Williams, C., Marchetti, E., et al. (2009). Tracking pyroclastic flows at soufriére hills volcano. EOS 90, 229–230. doi: 10.1029/2009EO270001
Ripepe, M., Marchetti, E., Delle Donne, D., Genco, R., Innocenti, L., Lacanna, G., et al. (2018). Infrasonic early warning system for explosive eruptions. J. Geophys. Res. Solid Earth 123, 9570–9585. doi: 10.1029/2018JB015561
Ripepe, M., Marchetti, E., and Ulivieri, G. (2007). Infrasonic monitoring at stromboli volcano during the 2003 effusive eruption: insights on the explosive and degassing process of an open conduit system. J. Geophys. Res. Solid Earth 112:B09207. doi: 10.1029/2006JB004613
Schwaiger, H., Lyons, J., Iezzi, A., Fee, D., and Haney, M. (2020). Evolving infrasound detections from Bogoslof volcano, alaska: insights from atmospheric propagation modeling. Bull. Volcanol. 82:27. doi: 10.1007/s00445-020-1360-3
Sciotto, M., Cannata, A., Prestifilippo, M., Scollo, S., Fee, D., and Privitera, E. (2019). Unravelling the links between seismo-acoustic signals and eruptive parameters: Etna lava fountain case study. Sci. Rep. 9:16417. doi: 10.1038/s41598-019-52576-w
Szuberla, C. A. L., and Olson, J. V. (2004). Uncertainties associated with parameter estimation in atmospheric infrasound arrays. J. Acoust. Soc. Am. 115, 253–258. doi: 10.1121/1.1635407
Vardeman, S., and Jobe, J. (2001). Basic Engineering Data Collection and Analysis. Statistics Series. Cengage Learning.
Keywords: volcano infrasound, infrasound arrays, volcanic degassing, ash explosions, lava flow, Mt. Etna
Citation: De Angelis S, Haney MM, Lyons JJ, Wech A, Fee D, Diaz-Moreno A and Zuccarello L (2020) Uncertainty in Detection of Volcanic Activity Using Infrasound Arrays: Examples From Mt. Etna, Italy. Front. Earth Sci. 8:169. doi: 10.3389/feart.2020.00169
Received: 13 February 2020; Accepted: 01 May 2020;
Published: 28 May 2020.
Edited by:
Andrea Cannata, University of Catania, ItalyReviewed by:
Stephen Arrowsmith, Southern Methodist University, United StatesStephen R. McNutt, University of South Florida, United States
Copyright © 2020 De Angelis, Haney, Lyons, Wech, Fee, Diaz-Moreno and Zuccarello. This is an open-access article distributed under the terms of the Creative Commons Attribution License (CC BY). The use, distribution or reproduction in other forums is permitted, provided the original author(s) and the copyright owner(s) are credited and that the original publication in this journal is cited, in accordance with accepted academic practice. No use, distribution or reproduction is permitted which does not comply with these terms.
*Correspondence: Silvio De Angelis, silvioda@liverpool.ac.uk