- 1INRAE, Ecosystemes Méditerranéens et Risques, Aix-en-Provence, France
- 2Department of Geography, University of Idaho, Moscow, ID, United States
- 3Management of Complex Systems Department, University of California, Merced, Merced, CA, United States
- 4INRAE, Ecologie des Forêts Méditerranéennes, Avignon, France
Anthropogenic climate change is widely thought to have enhanced fire danger across parts of the world, including Mediterranean regions through increased evaporative demand and diminished precipitation during the fire season. Previous efforts have detected increases in fire danger across parts of southern Europe but a formal attribution of the role of anthropogenic climate forcing has not been undertaken. Here, we attempt to disentangle the confounding effects of anthropogenic climate change and natural variability on observed increases in fire danger in France over the past six decades, with a focus on the fire-prone Mediterranean region. Daily fire weather and fire-related drought indices were computed from a reanalyses dataset covering the 1958–2017 period. Anthropogenic signals in meteorological variables were isolated using 17 climate models and then removed from observations to form a set of counterfactual observations free of anthropogenic climate change. Our results show that anthropogenic climate change is responsible for nearly half of the long-term increases in fire weather and fire-related drought conditions across the Mediterranean region and have significantly elevated the likelihood of summers with extreme fire danger. Fire danger conditions such as those observed during the near-record breaking 2003 fire season have a <0.2% annual probability (return interval >500 years) of occurrence in the absence of anthropogenic climate change, compared to a probability of ~10% (return interval ~10 years) under today's climate accounting for anthropogenic climate change. Our approach provides modernized estimates of current fire danger levels and expected return levels of extreme fire seasons considering climate change, which may help inform fire management agencies and decision making.
1. Introduction
Fire is a major hazard throughout the Euro-Mediterranean basin threatening ecosystems, society, and taxing fire suppression resources. While most fire ignitions are due to human activities (Ganteaume et al., 2013), atmospheric variability plays a key role in the flammability of fuel and fire spread. The influence of weather and climate variability are particularly important for the occurrence of large fires. Multi-week to multi-month periods of anomalously high moisture deficits increase landscape flammability (Abatzoglou et al., 2018; Barbero et al., 2018; Ruffault et al., 2018b) though live (Pimont et al., 2019a) and dead (Boer et al., 2017) fuel dessication. Additionally, heat waves and strong gusty winds often lead to critical synoptic fire weather conditions that have been shown to facilitate fire spread across parts of Southern France (Hernandez et al., 2015; Ruffault et al., 2017; Lahaye et al., 2018). Together, the alignment of critical synoptic fire weather conditions in conjunction with longer-term fuel moisture deficit promotes the occurrence of large fires (Barbero et al., 2018). Co-occurring extremes in fuel aridity and potential fire spread rates such as those which occurred in summer 2003 (Trigo et al., 2005) contributed to near record-breaking burned area with 740,379 ha burned across Europe, including >74,000 ha in France (Trigo et al., 2006).
Climate change projections suggest widespread increase in fire danger and fire weather extremes across much of the globe over the twenty-first century (Abatzoglou et al., 2019). These trends are already evident globally in the observational record (Jolly et al., 2015), including across parts of France (Dupire et al., 2017; Fréjaville and Curt, 2017; Curt and Fréjaville, 2018). Increases in fire weather conditions have been attributed to anthropogenic global warming in portions of western North America (Yoon et al., 2015; Abatzoglou and Williams, 2016; Kirchmeier-Young et al., 2017, 2018; Tan et al., 2018; Williams et al., 2019) but the degree to which global warming has contributed to changes in fire weather danger characteristics in France, and more generally across the Euro-Mediterranean basin, has not been quantified. The region is of particular interest as climate models project both a strong warming—the so-called Mediterranean amplification—(Brogli et al., 2019) and drier summers which are expected to collectively exacerbate fire weather conditions (Turco et al., 2018; Fargeon et al., 2020).
There is increasing interest in quantifying the role of global warming on observed changes in the likelihoods of extreme events (Easterling et al., 2016; Lloyd and Oreskes, 2018; Bellprat et al., 2019; Stone et al., 2019). This is of interest both scientifically and from a hazard preparedness perspective. The latter is particularly important given that many estimates of fire danger level used by agencies for both community planning, hazard reduction, and preparedness are based on retrospective efforts. Modernized efforts that include changes in land use practices as well as changes in climate are thus essential. On the scientific front, attribution studies typically assess the relative contribution of a specific causal forcing, namely the anthropogenic climate change due to greenhouse gases, to a particular extreme event or changes in some pertinent statistic (e.g., annual maxima or frequency of daily temperatures exceeding the local 90th percentile). Such analyses are often confounded by the large internal variability in the climate system alongside known uncertainties in both the observational record and regional climatic responses to the anthropogenic forcing (Santer et al., 2019), with these issues being larger for regional-to-local attribution efforts (Angélil et al., 2018).
Additional challenges arise when attributing long-term changes in a multivariable phenomenon such as fire weather conditions (Abatzoglou et al., 2019). Fire weather indices integrate variables such as maximum temperature, precipitation, minimum relative humidity, and wind speed (Van Wagner, 1987) and the response to each of these inputs is often non-linear. Fire weather indices can thus reflect the combined influence of weather and climate extremes occurring simultaneously, such as a prolonged drought period intersecting with a heatwave (Barbero et al., 2015). Some of these inputs may be strongly influenced by anthropogenic climate forcing, some not influenced at all, and some changes may offset one another (Flannigan et al., 2016; Abatzoglou et al., 2019). In this regard, the confluence of the background warming trend with dry years across Mediterranean regions is thought to have altered the likelihood of such compound events, as seen during 2003. Quantifying the role of anthropogenic climate change in the occurrence of compound extreme events is thus a significant scientific challenge. While previous attribution efforts have focused so far on temperature extremes (Uhe et al., 2016), precipitation extremes (van Oldenborgh et al., 2017) or drought (Philip et al., 2018), the attribution framework has been sparingly applied to extreme fire weather conditions (Kirchmeier-Young et al., 2018).
This study quantifies the degree to which anthropogenic climate change has (i) contributed to observed increases in fire weather conditions over the historical record in France and in particular across the Mediterranean fire-prone region and (ii) altered the probability of compound extremes such as those that contributed to the exceptional 2003 fire season. Such analysis may help update risk assessment models and quantify the modern risk of extreme fire seasons, including the additional risk directly imposed by climate change. To answer these questions, we paired observational data alongside a set of counterfactual observations designed to reflect what we would have observed in the absence of anthropogenic climate change as deduced from climate simulations. As opposed to most previous studies using a single model or an ensemble of runs of a given model, we considered here multiple climate simulations to address the structural uncertainty inherent to climate models, which strengthens the confidence of the results.
2. Data and Methods
2.1. Fire Weather Observations
We used the daily Fire Weather Index (FWI) from the Canadian Forest Fire Danger Rating System (Van Wagner, 1987) to assess fire weather conditions. The FWI integrates both current meteorological conditions (daily maximum temperature, minimum relative humidity, wind speed, and 24-h accumulated precipitation) as well as antecedent conditions and reflects the effect of fuel moisture and potential fire spread rate on fire behavior. We used the FWI given its widespread usage globally (Di Giuseppe et al., 2016) and its well-established relationship with fire activity globally (Abatzoglou et al., 2018), including the occurrence of large fires in France (Barbero et al., 2018).
We complemented the FWI analyses using the Keetch Byram Drought Index (KBDI) (Keetch and Byram, 1968), a fire-related drought metric requiring only daily maximum temperature and precipitation. The KBDI is a daily water balance describing the drying rate of the soil as a cumulative estimate of moisture deficiency and is often considered as a proxy of live fuel moisture (Ruffault et al., 2018a). The KBDI is well-correlated with fire activity across parts of the world (Dolling et al., 2005; Taufik et al., 2015; Yoon et al., 2015). Here, we used an improved version of the KBDI to minimize the structural underestimation of water loss during the summer in Mediterranean regions (Ganatsas et al., 2011). Multiple adjustments were suggested by Ganatsas et al. (2011) including a different estimation of potential evapotranspiration and threshold for canopy interception of precipitation.
We used the French reanalyses SAFRAN (Système d'Analyse Fournissant des Renseignements Atmosphèriques à la Neige; Analysis system providing data for the snow model), a quality-controlled dataset available from 1958 to 2017 on a daily basis and over an 8-km grid spanning France (Vidal et al., 2010). The SAFRAN dataset provides all meteorological variables needed to derive FWI and KBDI (namely daily maximum temperature, precipitation, wind speed, and minimum relative humidity) and has been extensively used in previous studies.
2.2. Counterfactual Observations
Long-term trends in climate may be affected by two components, namely anthropogenic climate change (external forcing) and natural variability of the climate system (internal forcing). The relative role of each component cannot be distinguished through observations as both internal and external forcing may contribute equally to a warming trend, or alternatively, the absence of long-term change may be the result of forcing of opposite signs. Attribution studies are thus usually based on expected responses to anthropogenic climate change, that are commonly estimated using General Circulation Models (GCMs). While regional climate models may provide additional nuanced spatial information, output from regional models is typically limited temporally and only available from a few models. Spatial details resolved by the combination of different regional/global models are also associated with large uncertainties as regional models are notoriously known to inherit the biases from their driving GCM. Additionally, previous observational studies based on homogenized in situ time series revealed a spatially uniform warming across France (Gibelin et al., 2014), supporting the use of GCMs to examine the signal of change (anomalies with respect to a baseline period). We thus focused on using outputs from 17 GCMs participating in the Fifth Phase of the Coupled Model Intercomparison Project (CMIP5, Table S1) given our objectives in examining long-term transient simulations from pre-industrial through present. Our approach is fairly conservative as it may avoid some of the uncertainties in the spatial manifestation of anthropogenic climate change. We additionally considered the anthropogenic climate signal separately from each of the 17 GCMs to assess intermodel uncertainty in the anthropogenic forcing signal (Fargeon et al., 2020). All model output was regridded to a common 2.5° grid and only land cells were retained as relative humidity is expected to show contrasting responses between ocean and land (Byrne and O'Gorman, 2016).
Modeled changes in maximum temperature, precipitation, and minimum relative humidity at monthly timescales were deduced from each model relative to the model average during the quasi pre-industrial 1861–1910 baseline. Wind speed remained unchanged as no systematic trend was detected in CMIP5 experiments (Abatzoglou et al., 2019). We further isolated the 50-year low-pass filtered anomalies relative to a quasi pre-industrial 1861–1910 baseline (Figure 1), with the 50-year low-pass filter designed to minimize the influence of internal multidecadal variability (Abatzoglou and Williams, 2016; Williams et al., 2019). Figure 1 illustrates traces of 50-year low-pass filtered signals of climate change for maximum temperature, precipitation, and minimum relative humidity for the month of July for a given GCM grid cell. In agreement with previous findings (Terray and Boé, 2013), most models simulate a strong summer warming alongside a decrease in precipitation and minimum relative humidity. Anomalies were treated as additive for maximum temperature and minimum relative humidity, and multiplicative for precipitation following previous studies (Abatzoglou and Williams, 2016; Williams et al., 2019). These anomalies were used to derive counterfactual observations, that is the climate we would have observed in the absence of anthropogenic climate change. Counterfactual daily maximum temperature TMAXcf and daily minimum relative humidity RMINcf were calculated as the observed daily temperature TMAXobs and RMINobs (in SAFRAN) minus the anthropogenic trend for a given month. Counterfactual daily precipitation PRCPcf was calculated as PRCPcf = PRCPobs(1−(PRCPanomaly/100)) where PRCPobs represents daily precipitation observed (in SAFRAN) and PRCPanomaly corresponds to the anthropogenic trend in monthly precipitation expressed as percent of anomalies in a given month. In addition to examining the signal separately for each GCM, we calculated an additional estimate of the anthropogenic trend by averaging the 50-year low-pass filtered time series across the 17 models. For each grid cell and each month, we thus consider a total of 18 (17 GCMs + the multimodel mean) estimates of anthropogenic trend in climate variables. The spread among the models was estimated though the inter-quartile range (75% CI) as larger ranges (i.e., 95%) may encapsulate models that are outliers.
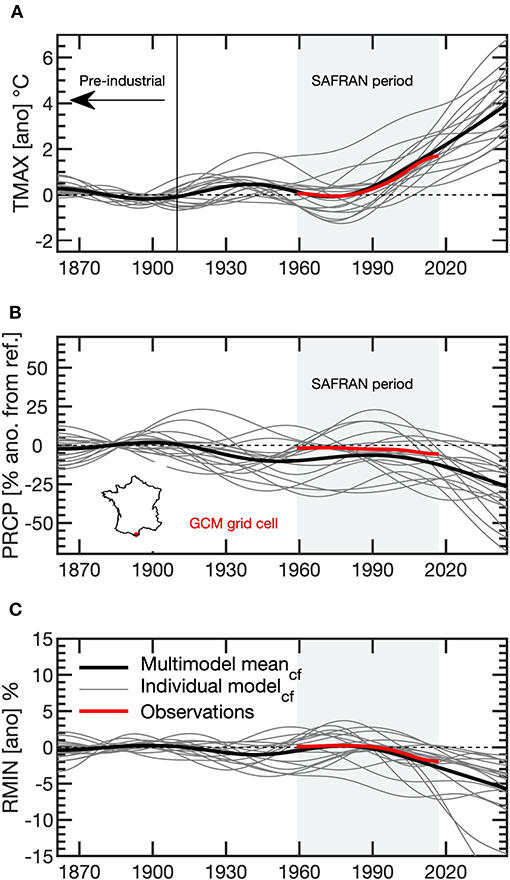
Figure 1. (A) Example of the anthropogenic trend in maximum temperature (expressed as anomalies with respect to the 1861–1910 baseline) simulated by 17 GCMs (gray lines) in a given GCM grid cell at 42.5°N-2.5°E (see insert in B) for the month of July. The anthropogenic trend is defined as the 50-year low pass filter of maximum temperature anomalies. The thick black curve shows the 50-year low pass filtered mean of the 17 GCMs. Observed anomalies in SAFRAN (1958–2017) in the corresponding grid cells are shown in red. Observed anomalies were computed with respect to counterfactual observations based on the multimodel mean (black curve). (B) Same as (A) but for precipitation (expressed as percent of anomalies with respect to the 1861–1910 baseline). (C) Same as (A) but for minimum relative humidity.
FWI and KBDI were calculated using daily observed data (FWIobs, KBDIobs) and the 18 daily counterfactual observations that exclude the anthropogenic climate signal (FWIcf, KBDIcf) reflecting what we would have observed in the absence of global warming. Linear trends in both observations and counterfactual observations were computed and the contribution of the anthropogenic forcing over the whole period was estimated as 100 × ((bobs − bcf)/bobs) where b denotes the slope of the linear trend.
2.3. FWI and KBDI Attributes
We examined different attributes of FWI and KBDI that have been shown to relate to fire activity. First, we examined FWI and KBDI averaged over the primary fire season from May to September (hereafter FWImean and KBDImean) as warm season conditions correlate positively with total burned area (Abatzoglou et al., 2018). Second, we examined the annual occurrence of days with high FWI and KBDI as large fires generally occur during periods of high fire danger (Barbero et al., 2018; Lahaye et al., 2018) with possible fire outbreaks below critical fuel moisture content levels (Pimont et al., 2019b). While percentile-based threshold indices (e.g., 95th percentile) typically measure the frequency of exceedance with respect to local conditions, they may not be well-suited to tracking elevated fire weather conditions in regions where the baseline climate is unfavorable to fire (typically outside the Mediterranean region). We thus examined the annual occurrence of days with FWI >20 (hereafter NFWI >20 with N denoting the number of days with FWI >20) and KBDI >35 (hereafter NKBDI>35), as a measure of critical fire danger levels. This is in agreement with previous FWI thresholds used in the Euro-Mediterranean basin ranging from FWI >15 (Moriondo et al., 2006; ) to FWI >30 (Fargeon et al., 2020) and with thresholds used in Canada to define weather conditions on days when fires grew significantly (Podur and Wotton, 2011). This also corresponds to the lower limit of conditions under which large fires develop in the French Alps (Dupire et al., 2017) and in the French Mediterranean (Barbero et al., 2018). All these analyses were conducted at the 8-km grid cell level and were then aggregated across environmental regions.
Varied fire-climate relationships exist across France (Barbero et al., 2018) ranging from typical Mediterranean fire-prone conditions in the South to more moisture-limited conditions in the North. Here, we focused mainly on the Mediterranean region (see Figure 3) where the vast majority of fires and burned area occur. Note that while a recent massive fire suppression policy has contributed to a general decline in burned area across this region, suppression has been mostly effective for smaller fires (Evin et al., 2018) occurring under lower fire weather conditions. The Mediterranean region was delimited using a European environmental stratification based on climate, topography, and geographical position (Metzger et al., 2005).
2.4. Estimating Annual Exceedance Probability
While attribution studies generally focus on extreme values such as annual maxima, such metrics are generally poorly related to fire activity and have been shown to emerge more slowly from natural variability (Abatzoglou et al., 2019). Here, we sought to maximize the signal-to-noise ratio and capture both the spatial and temporal extents of the risk that are relevant to fire suppression strategies. Hence, FWImean and KBDImean (FWI and KBDI averaged over the May-September fire season) as well as NFWI>20 and NKBDI>35 were averaged across the Mediterranean region (see section 2.3), where the vast majority of burned area occurs. The resultant time series were then fitted to an appropriate statistical distribution to strengthen the quantile estimate. FWImean and KBDImean were fitted to a normal distribution with the probability density function:
with mean μ and variance σ2 while NFWI>20 and NKBDI>35 were fitted to a Weibull distribution. The Weibull distribution has been commonly used for wind data (Curry et al., 2012), and belongs to the exponential family. We used this distribution instead of the Generalized Pareto Distribution (GPD), which is frequently used in extreme-event attribution studies, as 20 and 35 cannot be considered as extreme values for FWI and KDBI, respectively. Moreover, the use of a spatial average is a priori inconsistent with GPD. We thus opted for the Weibull distribution which may also be fitted to less extreme data. The Weibull probability density function is:
where x ≥ 0 is the variable of interest (spatially averaged NFWI>20 or NKBDI>35), A>0 is the scale parameter closely related to the mean of the distribution and k > 0 is a dimensionless shape parameter. We used the method of maximum likelihood to estimate all the model parameters (Katz et al., 2002). The goodness-of-fit was assessed using the quantile-quantile plot for FWImean and KBDImean and the Weibull probability plot for NFWI>20 or NKBDI>35. Figure S1 indicates that FWImean and KBDImean likely come from a normal distribution while NFWI>20 and NKBDI>35 likely come from a Weibull distribution.
Using the inverse cumulative distribution function, we then estimated the annual exceedance probability (AEP), which refers to the probability of exceeding a given return level in any year. For instance, a 1 in 100 year event has an AEP = 1%. The AEP was preferred over the return period concept, as return periods have been shown to obscure the intended probabilistic meaning and are often misinterpreted by users (Grounds et al., 2018).
AEP were estimated under (i) counterfactual conditions free of anthropogenic trends under the stationarity assumption (assuming that AEP do not change over time), (ii) observed conditions under the stationarity assumption and (iii) observed conditions under the non-stationarity assumption with either time or global mean surface temperature (GMST) as a covariate of either the mean parameter μ for the normal distribution or the scale parameter A for the Weibull distribution. In the former cases (i) and (ii), the parameters of the fitted distribution are constant and the AEP do not change with time. In the latter case (iii), the μ parameter of the normal distribution and the scale parameter A of the Weibull distribution change with time while keeping the other parameters constant:
with y(t) denoting a time-varying covariate and β0, β1 representing unknown parameters to be estimated. Here, y(t) is either time or the GMST in year t acquired from the National Aeronautics and Space Administration (NASA) Goddard Institute for Space Science (GISS) surface temperature analysis (Hansen et al., 2010) (see Figure S2). A 1,000-member non-parametric bootstrap procedure was used to estimate 95% confidence intervals for the fit and estimated AEP.
2.5. The 2003 Fire Weather Season
The 2003 summer was the warmest summer in Europe over the last 500 years (Luterbacher et al., 2004). A blocking pattern persisted over western Europe, partly due to the high soil moisture deficits during previous month that have enhanced the ratio of sensible to latent heat (Vautard et al., 2007). Together, anomalously dry soils and the blocking pattern resulted in large temperature anomalies across much of Europe, especially in France (Trigo et al., 2005). Attribution studies have shown that anthropogenic emissions largely contributed to this record-breaking summer (Schär et al., 2004), making the mean summer temperature across Europe twice as likely as it would have been in the absence of anthropogenic forcing (Stott et al., 2004) and increasingly likely in the future (Christidis et al., 2015). The 2003 heat wave was conducive to a fire outbreak across the continent, including near record-breaking burned area and large fire occurrences in France (Lahaye et al., 2018; Ganteaume and Barbero, 2019).
We sought to determine to what extent the odds of extreme fire weather conditions observed during the 2003 fire season have changed as a result of anthropogenic climate change. For that purpose, the levels of FWI and KBDI observed in 2003 provide benchmarks for estimating annual probabilities under actual and counterfactual climates separately, in turn allowing us to quantify how the anthropogenic forcing has changed the likelihood of such an event. For each FWI and KBDI attribute, we computed the risk ratio of the AEP corresponding to a 2003-like year observed under today's climate (2017 fit) to the AEP corresponding to a 2003-like year in each counterfactual observations.
Note that risk ratios reported here depend on the selected metric, the choice of exceedance threshold of critical fire weather conditions, time period and spatial scale on which the FWI and KBDI are aggregated. Given that increasing the spatial scale generally reduces interannual variability which in turn increases the risk ratio (Angélil et al., 2018; Leach et al., 2020; Yiou et al., 2020), the risk ratios reported here in the Mediterranean region are likely lower (larger) than those expected on broader (smaller) regions.
3. Results
FWImean (Figure 2A) and KBDImean (Figure 2C) during 2008-2017 both show a strong latitudinal gradient, with higher fire danger level in the French Mediterranean. Note that KBDImean exhibits slight differences with FWImean in the Mediterranean as the rate of moisture loss in the KBDI increases with increasing annual rainfall. Likewise, the annual frequency of critical days (e.g., NFWI>20) is the highest in the Mediterranean (Figures 2E,G) and to a lesser extent in the west. The difference between observed and counterfactual FWI (as estimated here by the multimodel mean) suggests that both mean conditions (Figures 2B,D) and critical fire weather conditions (Figures 2F,H) were exacerbated in recent years in response to anthropogenic climate change across the Mediterranean, and to a lesser extent across the Southwest.
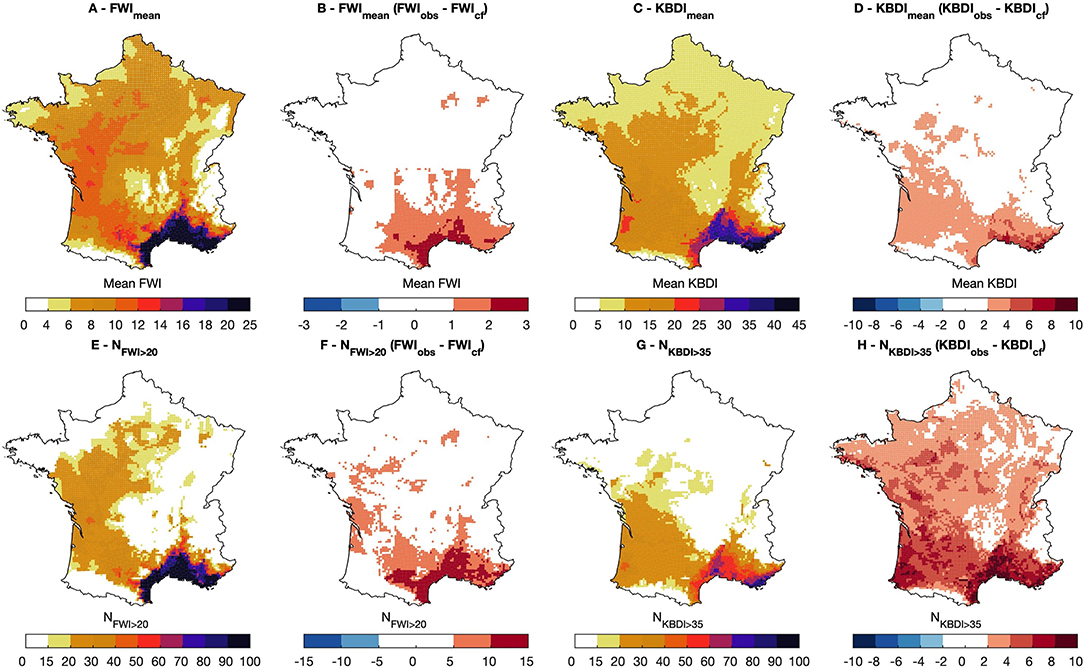
Figure 2. (A) Mean observed FWI from May-September during the last 10 years of the observational record (2008–2017). Note the non-linear color scale. (B) Difference between observations and counterfactual observations as deduced from the multimodel mean during the last 10 years of the observational record (2008–2017). (C) Same as (A) but for mean observed KBDI. (D) Same as (B) but for KBDI. (E) Average number of annual occurrence of daily FWI>20 during the last 10 years of the observational record (2008–2017). (F) Same as (B) but for the annual occurrence of daily FWI>20. (G) Same as (C) but for the annual occurrence of daily KBDI>35. (H) Same as (F) but for KBDI>35.
We then restricted our attention to the Mediterranean given the strong signal of change across the region. Figure 3 indicates that all metrics have seen a continued increase throughout the period and lie above counterfactual data as from 2000s. The anthropogenic forcing was found to contribute to about half of the linear trend in FWI metrics (47% for FWImean and 50% for NFWI>20) and even more for KBDI metrics with a contribution of 72% in NKBDI>35 probably due to the dominant role of maximum temperature in the KBDI. In both FWI and KBDI, the anthropogenic contribution seems to be stronger when considering the frequency of critical daily fire danger conditions. These changes are mostly due to a warming trend and a decrease in minimum relative humidity in more recent decades (not shown). Note that the warming rate found here is in agreement with previous studies based on homogenized in situ stations (Gibelin et al., 2014) presenting a warming rate of 0.42°C/decade during the summer period and with other large-scale observational products such as CRUTEM4 (Jones et al., 2012). By contrast, precipitation has seen a nominal decrease due to anthropogenic climate change and the signal remains largely dominated by interannual variability (not shown).
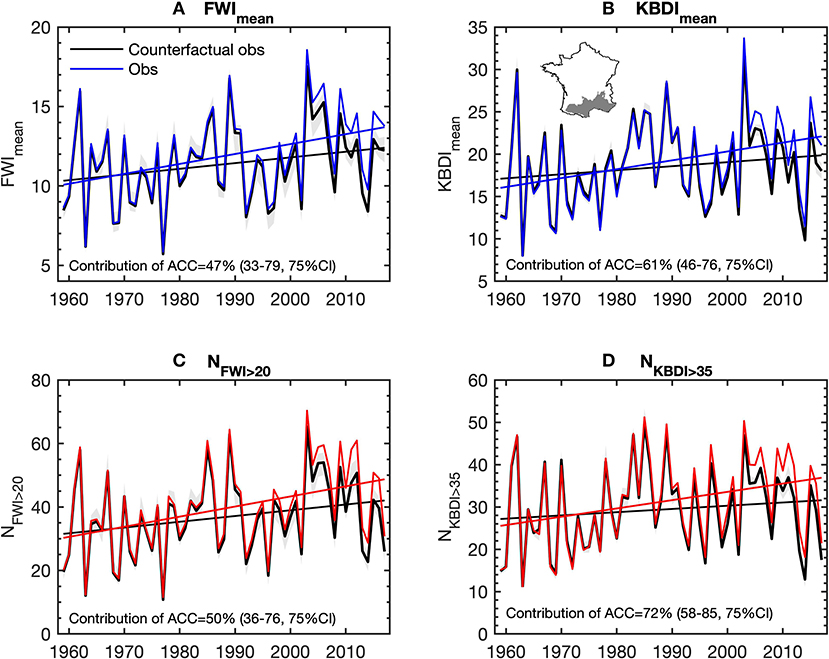
Figure 3. (A) Mean FWI from May to September averaged across the Mediterranean region (see map) using observations (color) and counterfactual observations (black) as deduced from the multimodel mean. The shaded gray area shows the 75% range of counterfactual observations as deduced from different GCMs. Linear trends are also shown as well as the fractional contribution of anthropogenic climate change (ACC) calculated as 100 × ((bobs−bcf)/bobs) where b denotes the slope of the linear trend. The mean fractional contribution across models as well as the interquartile range are indicated. (B) Same as (A) but for KBDI. (C) Same as (A) but for NFWI>20. (D) Same as (A) but for NKBDI>35.
Return levels in FWImean in counterfactual observation as deduced from the multimodel mean (gray) are much lower than those under observations (orange) (Figure 4A). The 2003 summer has an AEP <0.2% (>500-year return period) in counterfactual observations and an AEP ~ 0.6% (~ 167-year return period) in observations under the stationarity assumption (orange). Using a non-stationary distribution in actual 2017 climate (red), the AEP increases due to the underlying trend in FWImean and a 2003-like summer has now an AEP ~ 3.5% (~29-year return period). When considering NFWI>20 (Figure 4C), the AEP of a 2003-like summer is <0.2% in counterfactual observations (gray) and actual today's climate (red) suggests that the AEP has increased to ~ 10%, (~ 10-year return period). Similar results were obtained with KBDI (Figures 4B,D) with however slight differences in the AEP. Likewise, similar results were found when repeating the analysis with the GMST as a covariate in a non-stationary context (Figure S3). Overall, these results suggest that the AEP of high fire danger conditions has increased over time. The stationarity assumption would be a very conservative estimate of the current risk (based on retrospective data).
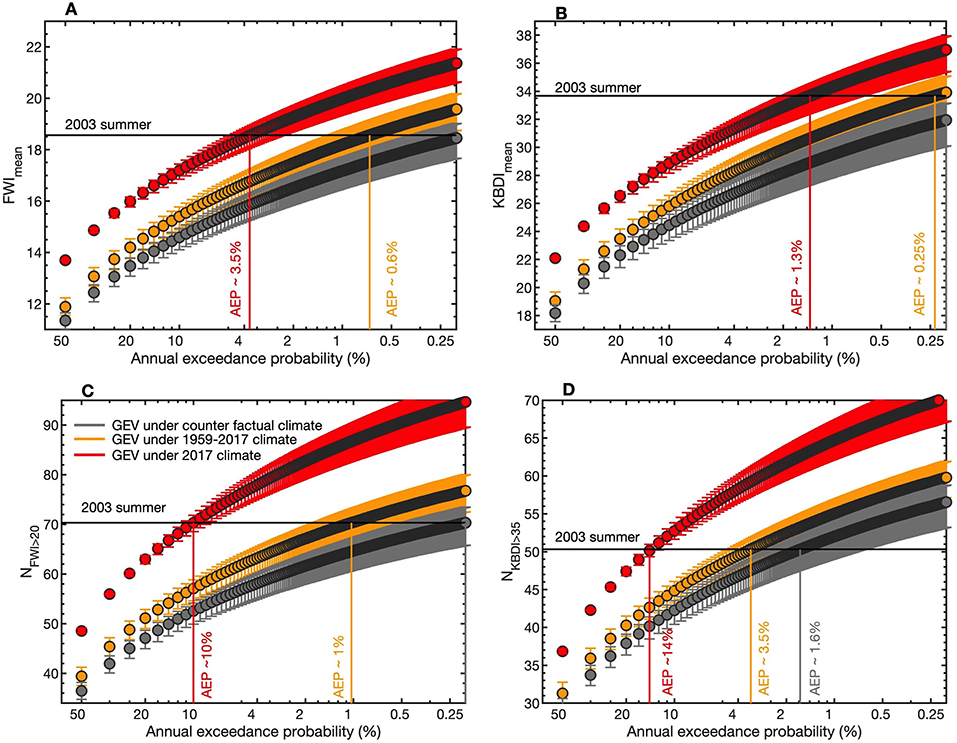
Figure 4. (A) Return levels in the mean FWI from May to September averaged across the Mediterranean region for different annual exceedance probabilities (AEP) ranging from 50% (2-year return period) to 0.2% (500-year return period) estimated with a normal distribution using counterfactual observations as deduced from the multimodel mean (gray), observations under the stationarity assumption (orange), and observations under the non-stationarity assumption with the fitted trend to 2017 (red). The 95% confidence intervals were estimated using a bootstrapping approach. The black horizontal line denotes the level observed in 2003 and the vertical lines indicate the AEP in different fits (best estimate). (B) Same as (A) but for KBDI. (C) Same as (A) but for the annual number of occurrence of daily FWI>20 averaged across the Mediterranean region. In this case, the AEP has been estimated with a Weibull distribution. (D) Same as (C) but for the annual number of occurrence of daily KBDI>35.
Finally, we reported on changes in the probability of occurrence of a 2003-like year between counterfactual observations and observations with the non-stationary fit to 2017 (Figure 5). The different values summarized in boxplots were obtained using individual GCMs to estimate the counterfactual observations that allows for a more complete assessment of model uncertainty (see Figure S4), instead of the multimodel mean as done previously. We find that the risk ratio of fire weather metrics increased dramatically through the inclusion of anthropogenic forcing. Anthropogenic climate change has made a 2003-like year about 25 (15–200, 75% CI) times more likely in 2017 when considering NFWI>20 and 9 (6–23, 75% CI) times more likely when considering NKBDI>35.
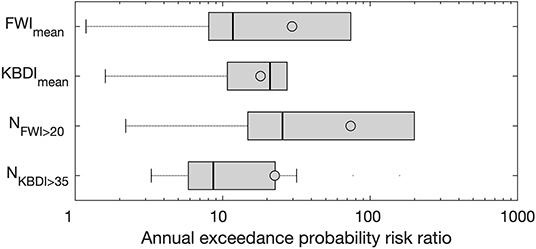
Figure 5. Annual exceedance probability risk ratio of a 2003-like year across the Mediterranean region between observations with the fitted trend to 2017 and counterfactual observations for different FWI and KBDI attributes. The boxplots indicate the range of changes obtained from different counterfactual observations as deduced from different GCMs. This is slightly different from Figure 4 where counterfactual observations were deduced from the multimodel mean of the 17 GCMs. Boxes indicate the inter-quartile range, vertical thick lines indicate the median and circles indicate the mean.
4. Conclusion and Discussion
Previous observational studies have reported on increase in fire weather conditions globally (Jolly et al., 2015) and regionally across portions of Europe (Turco et al., 2019). Here, we disentangled the anthropogenic forcing from natural variability and showed that anthropogenic climate change has increased mean fire weather conditions across France alongside the frequency of critical days as viewed through the lens of two different fire weather indices, elevating the probability of occurrence of a 2003-like fire weather season by orders of magnitude under today's climate. Based on the likelihood scale of the risk ratio provided in Lewis et al. (2019), we conclude that conditions observed in 2003 have become very much more likely due to climate change. Although comparison with previous studies examining the impact of anthropogenic climate change on heat waves is confounded by methodological and data differences, or the way an event is defined in space and time, our results are in line with Christidis et al. (2015) who showed that the 2003 heat wave has become increasingly more probable with global warming. Further studies are needed to compare relative changes in fire weather metrics with respect to heat extremes. The exceptional character of extreme events such as 2003 is hypothesized to be amplified when examined through the lens of fire weather indices rather than heat alone, particularly in regions experiencing decreased precipitation during the fire season.
About half of the long-term increases in fire weather conditions over the last 60 years was accounted for by anthropogenic climate change, with larger contribution in the frequency of critical days. Yet, this leaves a considerable part of the variability which is not explained by anthropogenic climate change. It should be kept in mind that this number was estimated through a simple linear regression spanning a period prior to 1980s with lower anthropogenic emissions. The anthropogenic contribution is thus likely to increase when restricting the analysis to more recent years. Using piecewise linear fitting, polynomial or other non-linear fitting may also describe more accurately historical changes. A potential source of underestimation of the anthropogenic forcing may also arise from a late and/or weak simulated warming over France in some GCMs with respect to observations due to the combination of natural variability and anthropogenic aeorosols cooling effect in 1950–1970s seen in a number of GCMs (Wilcox et al., 2013). Aerosols have been shown to strongly modulate multi-decadal trends in CMIP5 simulations and are often considered as one of the main sources of inter-models uncertainty on such timescales (Rotstayn et al., 2015). Uncertainty may also arise from the climate reanalysis. Gridded reanalyses such as SAFRAN provide a uniform spatial coverage but long-term trends in climate variables may differ from in situ time series (Vidal et al., 2010). Although the warming rate found here is in agreement with that reported in Gibelin et al. (2014) based on direct temperature measurements, other variables such as precipitation may exhibit different signals from in situ data. Further studies in other Euro-Mediterranean countries utilizing different observational products may help validate our results across broader scales.
An inherent limitation of the methodology here is the use of climate simulations that do not explicitly distinguish changes in the climate system driven by anthropogenic emissions from purely natural variability. The low-pass filter signal from GCMs ideally removes interannual-to-decadal natural variability to better isolate the anthropogenic signal, but natural variability may still persist for individual ensemble members. Additionally, inflating the amount of precipitation during wet days in regions where climate models simulate precipitation decreases may provide a reasonable estimate of monthly precipitation without anthropogenic emissions, but this approach fails to account for the effect of climate change on the frequency of wet days. This is of particular importance as both KBDI and FWI exclude precipitation amount below a given threshold. New climate models, such as those submitted to the Climate of the twentieth Century Plus Detection and Attribution project (C20C + D&A) (Stone et al., 2019) now simulate the present-day climate with and without anthropogenic emissions. Such simulations may provide a more realistic estimate of the effect of anthropogenic climate change on fire weather, albeit with a limited number of climate simulations.
Additional global warming is projected to foster fire weather conditions across the region into the twenty-first century (Fargeon et al., 2020). Further compound analyses that consider the covariance structure of KBDI and FWI may resolve future changes to fuel moisture contents and fire weather. The co-occurrence of such extremes is likely to continue in the future and may have implications for fire activity as the climate-fire relationship involves non-linear mechanisms (Williams et al., 2019), possibly in response to the moisture-fire relationship (Pimont et al., 2019a), such that subtle increases in fire weather conditions may translate into disproportionate increase in fire activity. These findings have implications for fire management strategies that may necessitate adaptation measures to reduce societal risk.
Further studies are required to better understand the impact of anthropogenic climate change not only on fire weather conditions but also on fire activity. The influence of the weather and climate forcing on fire activity is now well-understood and to some extent, well-reproduced by probabilistic models (Barbero et al., 2018). Feeding such models with both observations and counterfactual observations may provide insights on the contribution of anthropogenic emissions in fire activity during extreme seasons such as 2003 and would help bridge the gap between attribution studies and climate-fire modeling studies. Finally, further analysis is also required to disentangle the relative contribution of the climate forcing and human activities such as suppression policies that have been shown to obscure the functional climate-fire relationship (Ruffault and Mouillot, 2015; Curt and Fréjaville, 2018). Regardless, fire weather conditions have become increasingly unfavorable to fire suppression and future conditions are likely to overwhelm current fire management capacity.
Data Availability Statement
The datasets generated for this study are available on request to the corresponding author.
Author Contributions
RB carried out the analysis. RB and JA contributed to the design of the methodology. RB, JA, FP, JR, and TC discussed the results and contributed to writing the paper.
Funding
JA was partially supported by NSF grant DMS-1520873.
Conflict of Interest
The authors declare that the research was conducted in the absence of any commercial or financial relationships that could be construed as a potential conflict of interest.
The handling Editor declared a past co-authorship with one of the authors RB.
Acknowledgments
The authors appreciate the constructive reviews by MT and XW who helped improve the quality of this manuscript and provided valuable insights on the statistical aspects of this study.
Supplementary Material
The Supplementary Material for this article can be found online at: https://www.frontiersin.org/articles/10.3389/feart.2020.00104/full#supplementary-material
References
Abatzoglou, J. T., Williams, A., Boschetti, L., Zubkova, M., and Kolden, C. A. (2018). Global patterns of interannual climate-fire relationships. Global Change Biol. 24, 5164–5175. doi: 10.1111/gcb.14405
Abatzoglou, J. T., and Williams, A. P. (2016). Impact of anthropogenic climate change on wildfire across western US forests. Proc. Natl. Acad. Sci. U.S.A. 113, 11770–11775. doi: 10.1073/pnas.1607171113
Abatzoglou, J. T., Williams, A. P., and Barbero, R. (2019). Global emergence of anthropogenic climate change in fire weather indices. Geophys. Res. Lett. doi: 10.1029/2018GL080959
Angelil, O., Stone, D., Perkins-Kirkpatrick, S., Alexander, L. V., Wehner, M., Shiogama, H., et al. (2018). On the nonlinearity of spatial scales in extreme weather attribution statements. Clim. Dyn. 50, 2739–2752. doi: 10.1007/s00382-017-3768-9
Barbero, R., Abatzoglou, J., Kolden, C., Hegewisch, K., Larkin, N., and Podschwit, H. (2015). Multi-scalar influence of weather and climate on very large-fires in the Eastern United States. Int. J. Climatol. 35, 2180–2186. doi: 10.1002/joc.4090
Barbero, R., Curt, T., Ganteaume, A., Maillé, E., Jappiot, M., and Bellet, A. (2018). Simulating the effects of weather and climate on large wildfires in France. Nat. Hazards Earth Syst. Sci. 19, 441–454. doi: 10.5194/nhess-2018-283
Bellprat, O., Guemas, V., Doblas-Reyes, F., and Donat, M. G. (2019). Towards reliable extreme weather and climate event attribution. Nat. Commun. 10:1732. doi: 10.1038/s41467-019-09729-2
Boer, M. M., Nolan, R. H., Resco De Dios, V., Clarke, H., Price, O. F., and Bradstock, R. A. (2017). Changing weather extremes call for early warning of potential for catastrophic fire. Earth Fut. 5, 1196–1202. doi: 10.1002/2017EF000657
Brogli, R., Kroner, N., Sorland, S. L., Luthi, D., and Schar, C. (2019). The role of Hadley circulation and lapse-rate changes for the future European summer climate. J. Clim. 32, 385–404. doi: 10.1175/JCLI-D-18-0431.1
Byrne, M. P., and O'Gorman, P. A. (2016). Understanding decreases in land relative humidity with global warming: Conceptual model and GCM simulations. J. Clim. 29, 9045–9061. doi: 10.1175/JCLI-D-16-0351.1
Chatry, C., Le Quentrec, M., Laurens, D., Le Gallou, J.-Y., Lafitte, J.-J., and Creuchet, B. (2010). Rapport de la Mission Interministérielle 'Changement Climatique et Extension des Zones Sensibles aux Feux de Forêts. CGAER- CGEDD-IGA, Juillet 2010. 89.
Christidis, N., Jones, G. S., and Stott, P. A. (2015). Dramatically increasing chance of extremely hot summers since the 2003 European heatwave. Nat. Clim. Change 5, 46–50. doi: 10.1038/nclimate2468
Curry, C. L., van der Kamp, D., and Monahan, A. H. (2012). Statistical downscaling of historical monthly mean winds over a coastal region of complex terrain. I. Predicting wind speed. Clim. Dyn. 38, 1281–1299. doi: 10.1007/s00382-011-1173-3
Curt, T., and Fréjaville, T. (2018). Wildfire policy in mediterranean France: how far is it efficient and sustainable? Risk Anal. 38, 472–488. doi: 10.1111/risa.12855
Di Giuseppe, F., Pappenberger, F., Wetterhall, F., Krzeminski, B., Camia, A., Liberta, G., et al. (2016). The potential predictability of fire danger provided by numerical weather prediction. J. Appl. Meteorol. Climatol. 55, 2469–2491. doi: 10.1175/JAMC-D-15-0297.1
Dolling, K., Chu, P. S., and Fujioka, F. (2005). A climatological study of the Keetch/Byram drought index and fire activity in the Hawaiian Islands. Agric. Forest Meteorol. 133, 17–27. doi: 10.1016/j.agrformet.2005.07.016
Dupire, S., Curt, T., and Bigot, S. (2017). Spatio-temporal trends in fire weather in the French Alps. Sci. Tot. Environ. 595, 801–817. doi: 10.1016/j.scitotenv.2017.04.027
Easterling, D. R., Kunkel, K. E., Wehner, M. F., and Sun, L. (2016). Detection and attribution of climate extremes in the observed record. Weather Clim. Extremes 11, 17–27. doi: 10.1016/j.wace.2016.01.001
Evin, G., Curt, T., and Eckert, N. (2018). Has fire policy decreased the return period of the largest wildfire events in France? A Bayesian assessment based on extreme value theory. Nat. Hazards Earth Syst. Sci. 18, 2641–2651. doi: 10.5194/nhess-18-2641-2018
Fargeon, H., Pimont, F., Martin-StPaul, N., De Caceres, M., Ruffault, J., Barbero, R., et al. (2020). Projections of fire danger under climate change over France: where do the greatest uncertainties lie? Clim. Change. doi: 10.1007/s10584-019-02629-w
Flannigan, M. D., Wotton, B. M., Marshall, G. A., de Groot, W. J., Johnston, J., Jurko, N., et al. (2016). Fuel moisture sensitivity to temperature and precipitation: climate change implications. Clim. Change 134, 59–71. doi: 10.1007/s10584-015-1521-0
Frejaville, T., and Curt, T. (2017). Seasonal changes in the human alteration of fire regimes beyond the climate forcing. Environ. Res. Lett. 12. doi: 10.1088/1748-9326/aa5d23
Ganatsas, P., Antonis, M., and Marianthi, T. (2011). Development of an adapted empirical drought index to the Mediterranean conditions for use in forestry. Agric. Forest Meteorol. 151, 241–250. doi: 10.1016/j.agrformet.2010.10.011
Ganteaume, A., and Barbero, R. (2019). Contrasting large fire activity in the French Mediterranean. Nat. Hazards Earth Syst. Sci. 19, 1055–1066. doi: 10.5194/nhess-19-1055-2019
Ganteaume, A., Camia, A., Jappiot, M., San-Miguel-Ayanz, J., Long-Fournel, M., and Lampin, C. (2013). A review of the main driving factors of forest fire ignition over Europe. Environ. Manage. 51, 651–662. doi: 10.1007/s00267-012-9961-z
Gibelin, A.-L., Dubuisson, B., Corre, L., Deaux, N., Jourdain, J., Laval, L., et al. (2014). Evolution de la température en France depuis les années 1950. La Météorologie no. 87, 45–53. doi: 10.4267/2042/54336
Grounds, M. A., Leclerc, J. E., and Joslyn, S. (2018). Expressing flood likelihood: return period versus probability. Weather Clim. Soc. 10, 5–17. doi: 10.1175/WCAS-D-16-0107.1
Hansen, J., Ruedy, R., Sato, M., and Lo, K. (2010). Global surface temperature change. Rev. Geophys. 48:RG4004. doi: 10.1029/2010RG000345
Hernandez, C., Drobinski, P., and Turquety, S. (2015). How much does weather control fire size and intensity in the Mediterranean region? Ann. Geophys. 33, 931–939. doi: 10.5194/angeo-33-931-2015
Jolly, W. M., Cochrane, M. A., Freeborn, P. H., Holden, Z. A., Brown, T. J., Williamson, G. J., et al. (2015). Climate-induced variations in global wildfire danger from 1979 to 2013. Nat. Commun. 6:7537. doi: 10.1038/ncomms8537
Jones, P. D., Lister, D. H., Osborn, T. J., Harpham, C., Salmon, M., and Morice, C. P. (2012). Hemispheric and large-scale land-surface air temperature variations: an extensive revision and an update to 2010. J. Geophys. Res. Atmos. 117:D05127 doi: 10.1029/2011JD017139
Katz, R. W., Parlange, M. B., and Naveau, P. (2002). Statistics of extremes in hydrology. Adv. Water Resour. 25, 1287–1304. doi: 10.1016/S0309-1708(02)00056-8
Keetch, J. J., and Byram, G. M. (1968). A Drought Index for Forest Fire Control. Res. Paper SE-38. Asheville, NC: U.S. Department of Agriculture; Forest Service; Southeastern Forest Experiment Station, 32.
Kirchmeier-Young, M. C., Gillett, N. P., Zwiers, F. W., Cannon, A. J., and Anslow, F. S. (2018). Attribution of the influence of human-induced climate change on an extreme fire season. Earths Future 7, 2–10. doi: 10.1029/2018EF001050
Kirchmeier-Young, M. C., Zwiers, F. W., Gillett, N. P., and Cannon, A. J. (2017). Attributing extreme fire risk in Western Canada to human emissions. Clim. Change 144, 365–379. doi: 10.1007/s10584-017-2030-0
Lahaye, S., Curt, T., Fréjaville, T., Sharples, J., Paradis, L., and Hély, C. (2018). What are the drivers of dangerous fires in Mediterranean France? Int. J. Wildl. Fire 27, 155–163. doi: 10.1071/WF17087
Leach, N. J., Li, S., Sparrow, S., Van Oldenborgh, G. J., Lott, F. C., Weisheimer, A., et al. (2020). Anthropogenic influence on the 2018 summer warm spell in Europe: the impact of different spatio-temporal scales. Bull. Am. Meteorol. Soc. 101, S41–S46. doi: 10.1175/BAMS-D-19-0201.1
Lewis, S. C., King, A. D., Perkins-Kirkpatrick, S. E., and Wehner, M. F. (2019). Toward calibrated language for effectively communicating the results of extreme event attribution studies. Earths Future 7, 1020–1026. doi: 10.1029/2019EF001273
Lloyd, E. A., and Oreskes, N. (2018). Climate change attribution: when is it appropriate to accept new methods? Earths Future 6, 311–325. doi: 10.1002/2017EF000665
Luterbacher, J., Dietrich, D., Xoplaki, E., Grosjean, M., and Wanner, H. (2004). European seasonal and annual temperature variability, trends, and extremes since 1500. Science 303, 1499–1503. doi: 10.1126/science.1093877
Metzger, M. J., Bunce, R. G., Jongman, R. H., Mücher, C. A., and Watkins, J. W. (2005). A climatic stratification of the environment of Europe. Glob. Ecol. Biogeogr. 14, 549–563. doi: 10.1111/j.1466-822X.2005.00190.x
Moriondo, M., Good, P., Durao, R., Bindi, M., Giannakopoulos, C., and Corte-Real, J. (2006). Potential impact of climate change on fire risk in the Mediterranean area. Clim. Res. 31, 85–95. doi: 10.3354/cr031085
Philip, S., Kew, S. F., Jan van Oldenborgh, G., Otto, F., O'Keefe, S., Haustein, K., et al. (2018). Attribution analysis of the Ethiopian drought of 2015. J. Clim. 31, 2465–2486. doi: 10.1175/JCLI-D-17-0274.1
Pimont, F., Ruffault, J., Martin-StPaul, N. K., and Dupuy, J.-L. (2019a). A cautionary note regarding the use of cumulative burnt areas for the determination of fire danger index breakpoints. Int. J. Wildland Fire. 28, 254–258. doi: 10.1071/WF18056
Pimont, F., Ruffault, J., Martin-StPaul, N. K., and Dupuy, J.-L. (2019b). Why is the effect of live fuel moisture content on fire rate of spread underestimated in field experiments in shrublands? Int. J Wildland Fire. 28, 127–137. doi: 10.1071/WF18091
Podur, J., and Wotton, B. M. (2011). Defining fire spread event days for fire-growth modelling. Int. J. Wildland Fire. 20, 497–507. doi: 10.1071/WF09001
Rotstayn, L. D., Collier, M. A., Shindell, D. T., and Boucher, O. (2015). Why does aerosol forcing control historical global-mean surface temperature change in CMIP5 models? J. Clim. 28, 6608–6625. doi: 10.1175/JCLI-D-14-00712.1
Ruffault, J., Curt, T., Martin St-Paul, N. K., Moron, V., and Trigo, R. M. (2018b). Extreme wildfire occurrence in response to global change type droughts in the northern Mediterranean. Nat. Hazards Earth Syst. Sci. 18, 847–856. doi: 10.5194/nhess-18-847-2018
Ruffault, J., Martin-StPaul, N., Pimont, F., and Dupuy, J.-L. (2018a). How well do meteorological drought indices predict live fuel moisture content (LFMC)? An assessment for wildfire research and operations in Mediterranean ecosystems. Agric. Forest Meteorol. 262, 391–401. doi: 10.1016/j.agrformet.2018.07.031
Ruffault, J., Moron, V., Trigo, R. M., and Curt, T. (2017). Daily synoptic conditions associated with large fire occurrence in Mediterranean France: evidence for a wind-driven fire regime. Int. J. Climatol. 37, 524–533. doi: 10.1002/joc.4680
Ruffault, J., and Mouillot, F. (2015). How a new fire-suppression policy can abruptly reshape the fire-weather relationship. Ecosphere 6:art199. doi: 10.1890/ES15-00182.1
Santer, B. D., Fyfe, J. C., Solomon, S., Painter, J. F., Pallotta, G., and Zelinka, M. D. (2019). Quantifying stochastic uncertainty in detection time of human-caused climate signals. Proc. Natl. Acad. Sci. U.S.A. 116, 19821–19827. doi: 10.1073/pnas.1904586116
Schär, C., Vidale, P. L., Lüthi, D., Frei, C., Häberli, C., Liniger, M. A., et al. (2004). The role of increasing temperature variability in European summer heatwaves. Nature 427, 332–336. doi: 10.1038/nature02300
Stone, D. A., Christidis, N., Folland, C., Perkins-Kirkpatrick, S., Perlwitz, J., Shiogama, H., et al. (2019). Experiment design of the international CLIVAR C20C+ detection and attribution project. Weather Clim. Extremes 24:100206 doi: 10.1016/j.wace.2019.100206
Stott, P. A., Stone, D. A., and Allen, M. R. (2004). Human contribution to the European heatwave of 2003. Nature 432, 610–614. doi: 10.1038/nature03089
Tan, X., Chen, S., Yew, T., Liu, B., and Chen, X. (2018). Dynamic and thermodynamic changes conducive to the increased occurrence of extreme spring fire weather over western Canada under possible anthropogenic climate change. Agric. Forest Meteorol. 265, 269–279. doi: 10.1016/j.agrformet.2018.11.026
Taufik, M., Setiawan, B. I., and van Lanen, H. A. (2015). Modification of a fire drought index for tropical wetland ecosystems by including water table depth. Agric. Forest Meteorol. 203, 1–10. doi: 10.1016/j.agrformet.2014.12.006
Terray, L., and Boé, J. (2013). Quantifying 21st-century France climate change and related uncertainties. Comptes Rendus Geosci. 345, 136–149. doi: 10.1016/j.crte.2013.02.003
Trigo, R. M., García-Herrera, R., Díaz, J., Trigo, I. F., and Valente, M. A. (2005). How exceptional was the early August 2003 heatwave in France? Geophys. Res. Lett. 32:L10701. doi: 10.1029/2005GL022410
Trigo, R. M., Pereira, J. M., Pereira, M. G., Mota, B., Calado, T. J., Dacamara, C. C., et al. (2006). Atmospheric conditions associated with the exceptional fire season of 2003 in Portugal. Int. J. Climatol. 26, 1741–1757. doi: 10.1002/joc.1333
Turco, M., Jerez, S., Augusto, S., Tarín-Carrasco, P., Ratola, N., Jiménez-Guerrero, P., et al. (2019). Climate drivers of the 2017 devastating fires in Portugal. Sci. Rep. 9:13886. doi: 10.1038/s41598-019-50281-2
Turco, M., Rosa-Cánovas, J. J., Bedia, J., Jerez, S., Pedro Montávez, J., Llasat, M. C., et al. (2018). Exacerbated fires in Mediterranean Europe due to anthropogenic warming projected with non-stationary climate-fire models. Nat. Commun. 9:3821. doi: 10.1038/s41467-018-06358-z
Uhe, P., Otto, F. E., Haustein, K., van Oldenborgh, G. J., King, A. D., Wallom, D. C., et al. (2016). Comparison of methods: Attributing the 2014 record European temperatures to human influences. Geophys. Res. Lett. 43, 8685–8693. doi: 10.1002/2016GL069568
van Oldenborgh, G. J., van der Wiel, K., Sebastian, A., Singh, R., Arrighi, J., Otto, F., et al. (2017). Attribution of extreme rainfall from Hurricane Harvey, August 2017. Environ. Res. Lett. 12:124009. doi: 10.1088/1748-9326/aa9ef2
Van Wagner, C. E. (1987). Development and structure of the Canadian forest fire weather index system. Forestry Technical Report 35. Ottawa: Canadian Forestry Servic, 35.
Vautard, R., Yiou, P., D'Andrea, F., de Noblet, N., Viovy, N., Cassou, C., et al. (2007). Summertime European heat and drought waves induced by wintertime Mediterranean rainfall deficit. Geophys. Res. Lett. 34:L07711. doi: 10.1029/2006GL028001
Vidal, J. P., Martin, E., Franchistéguy, L., Baillon, M., and Soubeyroux, J. M. (2010). A 50-year high-resolution atmospheric reanalysis over France with the Safran system. Int. J. Climatol. 30, 1627–1644. doi: 10.1002/joc.2003
Wilcox, L. J., Highwood, E. J., and Dunstone, N. J. (2013). The influence of anthropogenic aerosol on multi-decadal variations of historical global climate. Environ. Res. Lett. 8:024033. doi: 10.1088/1748-9326/8/2/024033
Williams, A. P., Abatzoglou, J. T., Gershunov, A., Guzman-Morales, J., Bishop, D. A., Balch, J. K., et al. (2019). Observed impacts of anthropogenic climate change on wildfire in California. Earths Future. 77, 892–910. doi: 10.1029/2019EF001210
Yiou, P., Cattiaux, J., Faranda, D., Kadygrov, N., Jézéquel, A., Naveau, P., et al. (2020). Analyses of the Northern European summer heatwave of 2018. Bull. Amer. Meteor. Soc. 101, S35–S40. doi: 10.1175/BAMS-D-19-0170.1
Keywords: climate change, fire weather index, detection and attribution, Mediterranean, France
Citation: Barbero R, Abatzoglou JT, Pimont F, Ruffault J and Curt T (2020) Attributing Increases in Fire Weather to Anthropogenic Climate Change Over France. Front. Earth Sci. 8:104. doi: 10.3389/feart.2020.00104
Received: 17 January 2020; Accepted: 23 March 2020;
Published: 17 April 2020.
Edited by:
Vimal Mishra, Indian Institute of Technology Gandhinagar, IndiaReviewed by:
Xander Wang, University of Prince Edward Island, CanadaMari Rachel Tye, National Center for Atmospheric Research (UCAR), United States
Copyright © 2020 Barbero, Abatzoglou, Pimont, Ruffault and Curt. This is an open-access article distributed under the terms of the Creative Commons Attribution License (CC BY). The use, distribution or reproduction in other forums is permitted, provided the original author(s) and the copyright owner(s) are credited and that the original publication in this journal is cited, in accordance with accepted academic practice. No use, distribution or reproduction is permitted which does not comply with these terms.
*Correspondence: Renaud Barbero, cmVuYXVkLmJhcmJlcm9AaW5yYWUuZnI=