- 1Istituto Nazionale di Geofisica e Vulcanologia, Rome, Italy
- 2Luxembourg Institute of Science and Technology, Esch-sur-Alzette, Luxembourg
Monitoring volcanic eruptions provides key information for hazard assessment and its time evolution. Satellite remote sensing data are nowadays essential to perform such task, thanks to their capability to survey disastrous events also in remote and under-monitored regions, with frequent revisit time and accurate spatial resolution. Even though satellite imageries are presently used to analyze several phenomena related to eruptions, automatic methods and synergic exploitation of different sensors are rarely considered. In this work, we have analyzed satellite images coming from both synthetic aperture radar (SAR) and optical sensors, to study the effusive eruption of Fogo volcano, Cape Verde, which took place between November 2014 and January 2015. In particular, we have exploited multi-sensor images from Sentinel-1, COSMO-SkyMed, Landsat-8, and Earth-Observing-1 missions, to retrieve lava flow patterns and volcanic source parameters related to the eruption. The main outcome of our work is the application of a new automatic change detection technique for estimating the lava field and its temporal evolution, combining the SAR intensity and the interferometric SAR coherence. The innovative algorithm is able to take full advantage of the Sentinel-1 mission’s 6-day repeat cycle. Such data are here used for the first time for lava mapping, thereby providing an unprecedented example of using the multi-temporal interferometric SAR (InSAR) coherence to automatically monitor lava flow evolution in emergency phase. This new technique, jointly used with optical satellite images, is capable of resolving with spatial and temporal detail the evolution of lava flows. We have also performed differential SAR interferometry (DInSAR) to map the ground deformation and retrieve the feeding dyke by inverting syn-eruptive signals. Results from source modeling show a SW-NE oriented dyke, located inside Chã das Caldeiras, SW of the Pico do Fogo. Our work highlights how multidisciplinary and satellite open data, along with innovative and automatic processing techniques, may be adopted for real-time hazard estimates in an operational environment.
Introduction
Hazards assessment in volcanic areas requires the combination and the coordination of many instruments, techniques, and expertise, in different fields such as volcanology, geology, seismology, data analysis, meteorology, human sciences, and so on. All these fields of knowledge support decision makers and authorities to address risk reduction and improve crises management capabilities when volcanic eruptions occur. Volcanic eruptions are complex natural events that involve several phenomena: lava flows, gas emission, ash dispersal and ash fall, and other secondary effects, e.g., earthquakes, pyroclastic flows, landslides, and lahars, producing a wide spectrum of impacts (e.g., Bosi and MIAVITA group, 2012). In this heterogeneous scenario, satellite remote sensing data and the associated techniques, with their intrinsic multidisciplinary capability, represent very powerful tools. Especially in the present era, many sensors, in particular optical and synthetic aperture radar (SAR), are available on several platforms and constellation missions [e.g., European Space Agency (ESA)—Sentinel missions, and Agenzia Spaziale Italiana (ASI)—COSMO-SkyMed (CSK) mission], and can provide supporting information for monitoring ongoing eruptions. However, even though satellite imageries are intensely used, automatic methods and synergic exploitation of different sensors are rarely considered. Our case study is the eruption that began on November 2014 in Fogo Island, Cape Verde. We mapped the lava flow and its evolution with time using satellite imagery. In particular, we exploited the complementarity of SAR and optical images acquired by the new SAR sensor on board of Sentinel-1mission (S1), and by optical sensors of Landsat-8 (L8) and Earth-Observing-1 (EO-1) missions (from NASA/USGS), plus the high-resolution capability of CSK SAR sensor. We tested the capability of a novel change detection algorithm, based on multiscale tiling approach, for deriving automatic surface change maps from SAR data. Secondarily, we complemented the lava flow analysis, with geodetic observations by means of differential SAR interferometry (DInSAR). DInSAR data coming from Sentinel-1 and CSK missions, have allowed mapping the ground deformation due to the lava ascent and, by inverting them, the estimation of the eruption source. We show how the synergic use of multi-sensor data gathers useful information for hazards during volcanic crisis related to both internal (i.e., the feeding dyke) and external (e.g., lava flow) processes.
The Fogo Eruption (Cape Verde)
Located in the NW Atlantic Ocean, at about 500 km far from the coast of Senegal, Cape Verde is composed of 10 main islands, some of which are inhabited. Since the first settlements in 1460, only Fogo Island experienced volcanic eruptions. Fogo Island has nearly conical shape, with a diameter of about 30 km, an area of 471 km2, and a population of about 37,000 inhabitants (Faria and Fonseca, 2014). The island is cut at about 2000 m a.s.l. by a lateral collapse (Day et al., 1999) that formed a plateau known as Chã das Caldeiras. About 700 farmers live in Chã das Caldeiras, close to cone of Pico do Fogo that with its summit, reaching 2898 m a.s.l., dominates the caldera. In the last five centuries, the eruptions occurred in the caldera, both at the summit of Pico do Fogo and, since 1785, from fissures near its base, and were characterized by an average recurring period of about 20 years, and an average duration of 2 months (Ribeiro, 1960; Day et al., 2000). The most relevant hazard in Fogo is the lava flow, in particular inside Chã das Caldeiras, and in the steep eastern coast where 30% of inhabitants live (Faria and Fonseca, 2014 and the references therein). Indeed, in 1951, the lava flow destroyed a village on the eastern coast, and in 1995 (Amelung and Day, 2002) and lastly in 2014 the villages inside Chã das Caldeiras were destroyed. In such hazardous context, the monitoring of lava flow and its evolution in time can be of crucial importance to provide useful information for planning rescue activities and save lives.
The last eruption in Fogo started on 23 November 2014 and lasted 78 days, and was characterized by an effusive activity originated from a fissure located at the base of Pico do Fogo, spreading the lava in Chã das Caldeiras. The lava flow traveled to the SW, and then was split into two main lobes toward NW and S (González et al., 2015; Cappello et al., 2016; Calvari et al., 2018).
Data and Methods
A total of 21 images were used to map the lava emplacement in Chã das Caldeiras, nine from Sentinel-1A (S1A), six from CSK, and the remaining six from multispectral sensors on board of EO-1 (three images) and L8 (three images). Table 1 shows the available dataset, divided by type and by orbit.
The optical dataset was used to perform the analysis of lava filed that occurred in Chã das Caldeiras. SAR imagery was used for a twofold objective: the automatic change detection by means of hierarchical-split-based approach (HSBA, Chini et al., 2017), to map the lava occurrence, and the retrieval of the volcanic source by taking advantage of SAR interferometry technique.
Lava Emplacement Analysis
SAR Data Exploitation
Synthetic aperture radar data occupy a privileged place as regards change detection algorithms thanks to the sensitivity of the backscattering toward differences in land covers and their quasi-all weather, day/night observation capacity. For these reasons, SAR-based change detection (SAR-CD) was developed over many years to provide useful and reliable information on land surface changes that occur across different temporal and spatial scales (Bovolo and Bruzzone, 2005). SAR-CD usually finds different domain of application, and in particular for all concerns natural hazard related to floods (Chini et al., 2013), volcanoes (Bignami et al., 2013; Valade et al., 2019), earthquakes (Pierdicca et al., 2018), and tsunamis (Chini et al., 2008). SAR-CD algorithms typically generates the difference image and then classifies it, which consists of a binary classification problem, aiming at separating the change and the no change classes (hereafter CC and NCC) (Ajadi et al., 2016). To do this, histogram thresholding is one of the most commonly used methods (Rosin, 2002), having as a critical step the selection of an adequate threshold, affecting directly the classification results. Parametric approaches usually fit the probability density function (PDF) of CC and NCC (PDFCC and PDFNCC), which are assumed Gaussian, and then set the threshold where the two PDFs intersect (Bruzzone and Prieto, 2002). The classification accuracy heavily depends on the classes proportions within the scene and the overlap between the two PDFs. When CC and NCC are strongly unbalanced, it is difficult to robustly parameterize their PDFs, while the amount of overlap between PDFs directly affects the under- and over-detection. Here, to overcome the two aforementioned drawbacks, we used an adaptive threshold approach previously developed to map floodwater (Chini et al., 2017), and also applied to map buildings (Chini et al., 2018). The approach is composed of two main steps. It first parameterizes the PDFCC and PDFNCC, and then based on the two PDFs iteratively applies thresholding and region-growing to find the best threshold for seeds (THS) and the one for stopping the region growing (THSRG). The PDFs parameterization is performed by HSBA (Chini et al., 2017), which identifies regions, or tiles, of the image where the PDFCC and PDFNCC can be fitted more reliably and accurately. The size of the tiles depends on the possibility of parameterizing the PDFs attributed to two different classes. HSBA starts with bigger tiles, which reduce successively, depending on the spatial extension of changes on the surface with respect to the entire image. HSBA starts from the entire image and then reduce the tile size following a quad-tree decomposition of the image. In the second step, in order to reduce class overlap effects on the final classification, spatial information is introduced on the selection of the best threshold (Haralick and Shapiro, 1985). The latter is done by a region-growing approach assuming that pixels constituting CC are clustered rather than randomly spread out over the entire image (Giustarini et al., 2013; Chini et al., 2017). Therefore, we first classify as CC those pixels that have high magnitude of change and then we add to CC those pixels with a lower magnitude of change but which are spatially contiguous to the first guesses. To do this, we use the region-growing algorithm, where the PDFCC and PDFNCC will drive the selection of THS and THSRG. THS selects seeds, i.e., pixels with high change of magnitude. We can set THS to the mean value of PDFCC, which are pixels with a high probability to belong to CC. In order to select THSRG, different thresholds are tested. The choice is based on the minimization of the root-mean-squared error between the PDFCC and the histogram resulting from the region growing. The two thresholds are automatically selected in those areas defined by HSBA, and then they are applied to the entire image to get the final classification. This SAR-CD algorithm is applied to the intensity difference image (hereafter IDI), which is the difference between the two log-transformed images acquired on two different instants. The IDI registers changes in the roughness and the dielectric constant of the surface. In this particular case, the images acquired after the event onset were subtracted to the images acquired before the event, and the change image was mainly detecting an increase of the backscattering on those areas where new lava was flowing from the vent down to Chã das Caldeiras. This was probably due to the increased of roughness of the surface.
Another import SAR-related feature frequently used as change detector is the InSAR coherence. This quantity is mostly influenced by the phase difference between radar returns, a distinctive parameter measured by a coherent sensor such as SAR, and it is particularly related to the spatial arrangement of the scatterers within the pixel and thus to their possible random displacements. Its high sensitivity to surface changes is well-documented and enables the detection of damages caused by catastrophic events such as volcano eruptions, earthquakes, and floods (e.g., Hoffmann, 2007; Chini et al., 2012; Valade et al., 2019). Compared to the SAR intensity, the coherence sensitivity to surface changes is much higher, because even a target rotation can create a temporal decorrelation, while to detect changes in the intensity, it is necessary that the roughness and the dielectric properties of surface change. We compared the IDI with an InSAR coherence image (hereafter ICI) computed using the same couple of images, and although both localize the change in the same region, in Figure 1 is possible to appreciate that the spatial extensions are not the same. The ICI (Figure 1B) is showing more changes with respect to the IDI (Figure 1A), and this could be due to the surface sliding of the lava flow without producing a substantial change to the surface roughness detectable by the intensity. It is worth noting that the change depicted by coherence includes the change detected by intensity, having a bigger extent. Moreover, the spatial transition of coherence values from low to high is quite sharp, and this is because the incoherent surface movement produced by the lava flow is quite important. Based on these evidences, we integrated the InSAR coherence information to that one provided by intensity reapplying a region growing on the ICI. In this case, the seeds are those pixels depicted as change by the intensity and the rule to stop the growing is the standard deviation of seeds plus an epsilon. The rule to use the change maps from intensity as seed for the region growing on the coherence is motivated by the fact the coherence drop-off occurs also for reasons other than lava flow, e.g., vegetated areas (Valade et al., 2019). Indeed, looking at the coherence map in Figure 1B, the effect of the vegetation is quite evident in many areas (NNE zones of the volcano). Therefore, it is necessary to circumscribe the coherence loss only to those areas where a change in the SAR intensity has also occurred. In Figure 1, the inputs, the intermediate products, and the final change map are depicted. The latter obtained as a combination of IDI and ICI features. The increase in the intensity values is shown in Figure 1C (cyan areas) and in Figure 1E (white areas), while the decrease of coherence is in Figure 1D (dark areas), where it is highlighted that the InSAR coherence is detecting more changes than the only intensity. Figure 1F shows the intermediate product resulting from the IDI, while the final map is shown in Figure 1G, where the ICI was also integrated. The overall scheme for integrating ICI and IDI is reported in Figure 2.
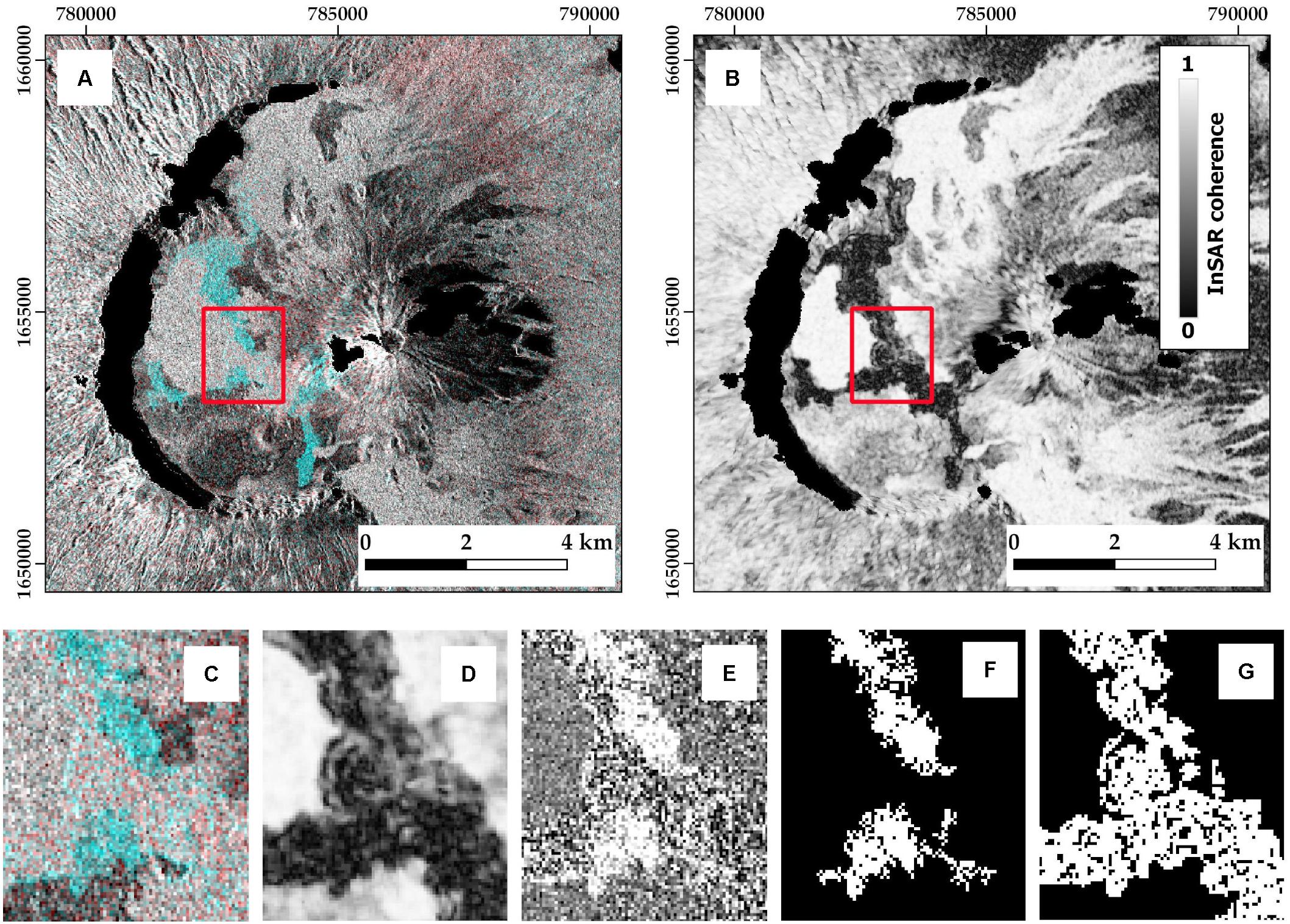
Figure 1. Inputs, intermediate products, and final change map. (A) False color combination of SAR intensity images: R = pre-eruption SAR image on 2014/11/03, G = B = post-eruption SAR image on 2015/01/02. (B) ICI. (C) Detail of false color combination in A. (D) Detail of ICI. (E) IDI. (F) Intermediate HSBA output using IDI only. (G) Final HSBA output using IDI and ICI.
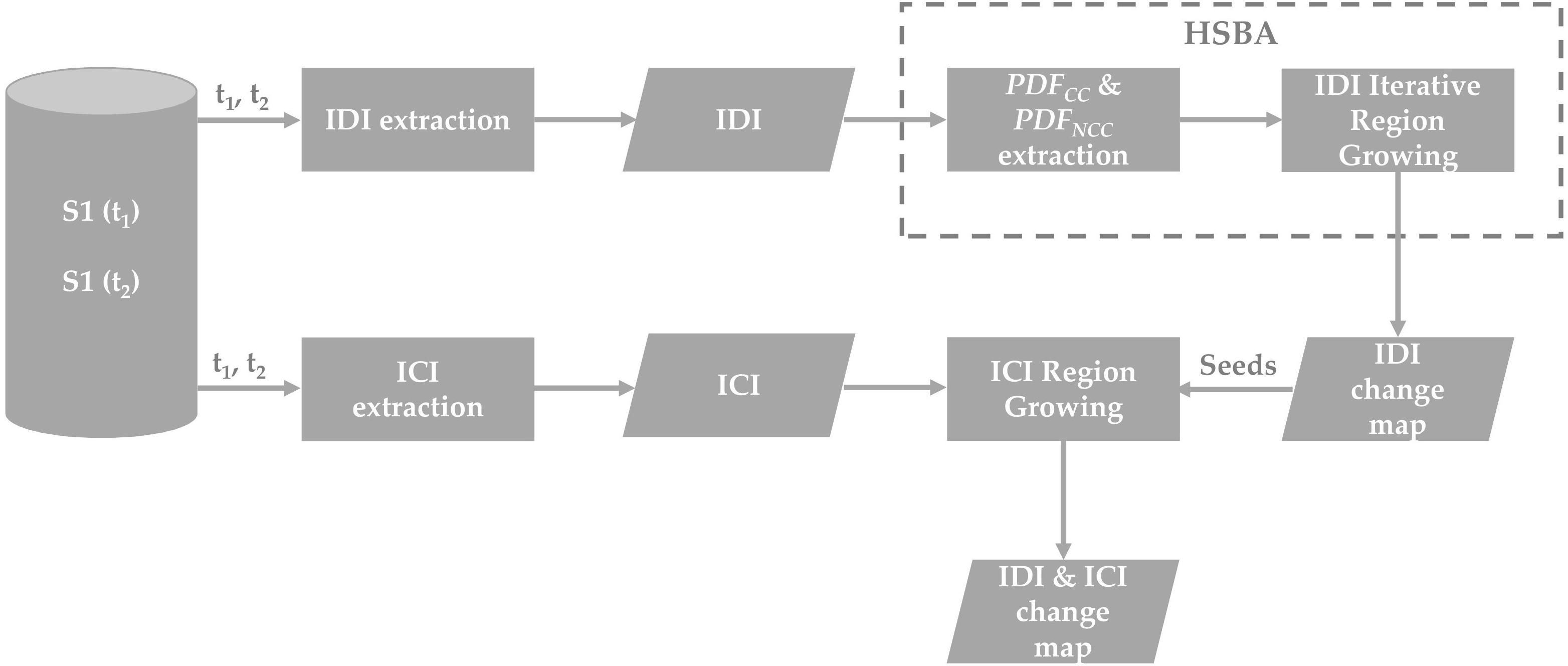
Figure 2. Workflow of the change detection algorithm based on HSBA. See main text for acronyms definition.
It is worth to recall the S1 enhanced observational capabilities which reduce the drawbacks of previous moderate resolution SAR images and potentially enable the fully exploitation of the InSAR coherence capabilities for identifying surface changes. The high repeat cycle (i.e., small temporal baseline) and the relatively narrow orbit tube (i.e., small perpendicular interferometric baseline) of S1 mission reduce the temporal and the spatial decorrelation in vegetated areas and in the presence of structures with geometrical complexity, respectively. These peculiar characteristics of S1 have recently even enabled the detection of floodwater in urban areas using InSAR coherence (Chini et al., 2019), areas so far blind at 20 m spatial resolution.
Finally, SAR images captured by S1A spacecraft have been used for the first time to map the lava field in the caldera during the Pico do Fogo eruption. The dataset is composed of images in the innovative Terrain Observation with Progressive Scans SAR—TOPSAR—acquisition mode [aka Interferometric Wide (IW) swath]. The Fogo eruption is the first volcanic event captured by S1A mission in TOPSAR mode, and it was the first case study where SAR interferometry was applied to this very new imaging scan (González et al., 2015). Both ascending and descending orbit data were processed to estimate the lava flow coverage in the period between the eruption’s onset and the last S1A image dated 2 January 2015. In addition to S1A data, we have taken advantage of CSK imageries. These data are high-resolution SAR images, at 3 m per pixel (full resolution). The TOPSAR images allowed the generation of seven change detection maps, four for the ascending data, and three for the descending ones. It is worth to note that the CSK were used to obtain some additional change maps but, unfortunately, they did not provide more information than those already provided by the S1 dataset.
The resulting change detection maps, from SAR intensity and phase coherence features, allowed the generation of the time series of lava emplacement (Figure 3). To do this, we computed the change maps using separately ascending and descending orbits, respectively. The change intensity images were all calculated with respect to the first available image of the time series (SARi–SAR0), which is the one acquired before the event started. Instead, the InSAR coherence maps were calculated using two consecutive SAR images in order to reduce the temporal baseline, then the temporal decorrelation in vegetated areas.
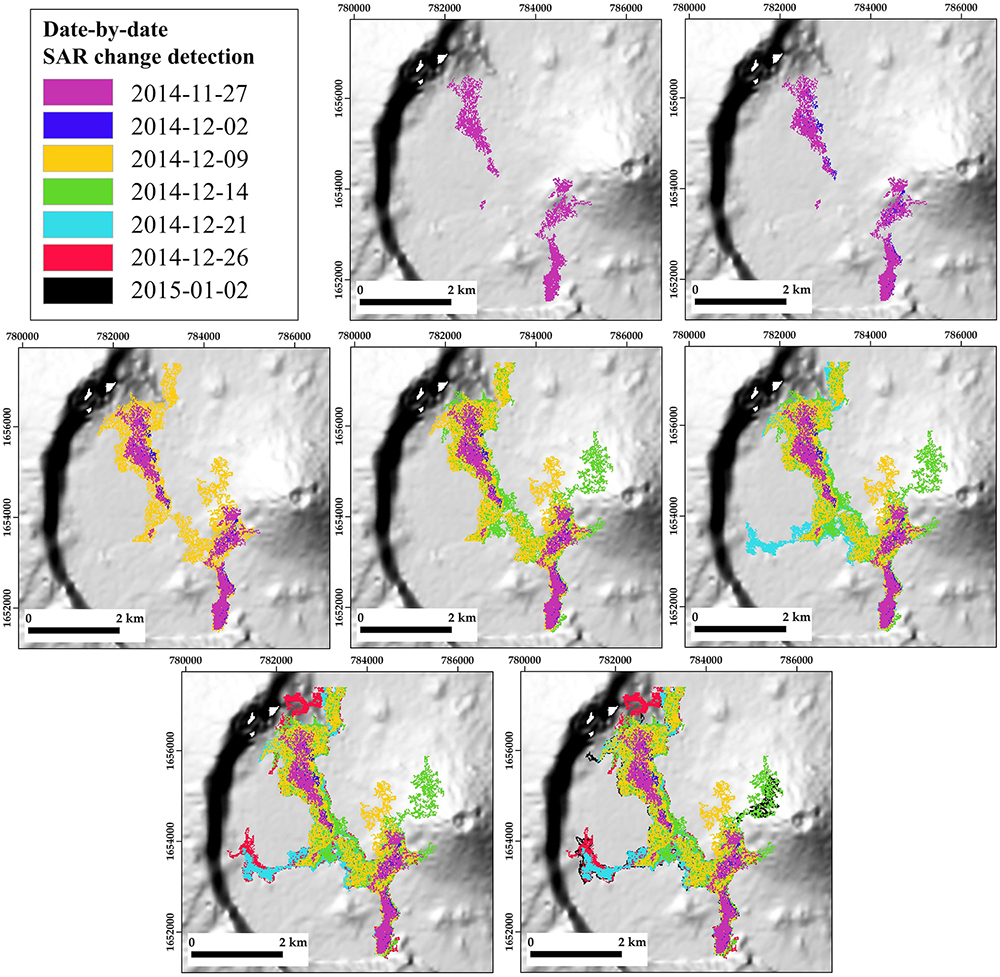
Figure 3. Temporal evolution of the deposited lava obtained by using HSBA algorithm applied to S1 images. The figure shows the area affected by erupted lava at each S1 overpass, in different colors, on ascending and descending orbit. Both intensity and phase coherence data were processed.
Optical Data Exploitation
Multispectral satellite data, at medium spatial resolution (30 m) pixel, were acquired by EO-1 and L8 satellites. The EO-1 satellite was 1-year technology validation/demonstration mission that was extended due to its successful results. EO-1 is equipped with an Advanced Land Imager (ALI) instrument to validate and demonstrate technology for the Landsat Data Continuity Mission (LDCM) and Hyperion hyperspectral sensor1. L82 is a joint initiative between NASA and USGS, and it is equipped with two push-broom instruments: the Operational Land Imager (OLI) and the Thermal Infrared Sensor (TIRS). We have employed the bands positioned in visible (VIS), near infrared (NIR), and short wave infrared (SWIR) of the electromagnetic spectrum, to map the flow-field evolution.
In particular, the SWIR (2.2 μm), VNIR (1.6 μm), VNIR-Green (0.56 μm) of both L8-OLI and EO-1-ALI data were used to create a false color composite for Red, Green, and Blue channels, respectively (Figure 4). These false color images (similar to natural colors) visually enhance some features allowing to outline lava flows which appear black and brown in the images while vegetated areas appear green. Active lava flows (hot lava) show a change in color from red to yellow as a result of an increase in the temperature of the crustal component and/or an increase in the areas of high temperature fractures (Wright et al., 2001). In addition, clouds appear white in the images, while the plumes colored in blue can be related to gases emitted by the volcano (e.g., SO2) (Flynn et al., 2000; Flynn et al., 2001; Lee et al., 2015). Figure 4B shows the active flow field on 24 November 2014, 1 day after the beginning of the eruption. Active lava flows from the eruptive fissure bifurcate originating two lava flows, one moving to the North in the direction of Portela and Bangaeira villages, and a second flow directed to South. The red haze surrounding the flows is a combined effect of smearing, i.e., high radiant pixels into adjacent pixels (Rothery et al., 1988), leading to an over representation of the size of the anomaly (Wright et al., 2001).
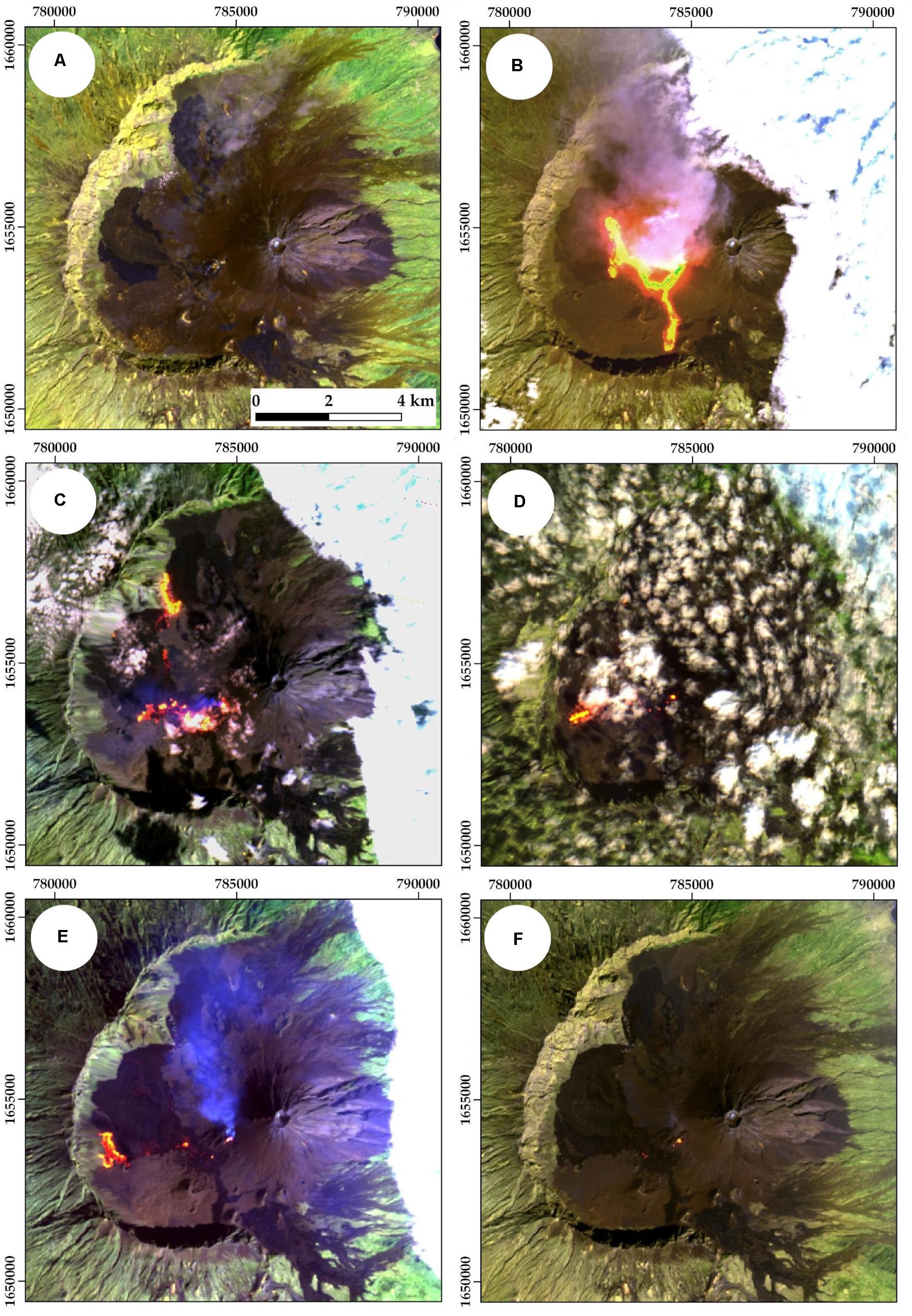
Figure 4. False color representations of optical dataset (see main text for RGB assignment). (A) pre-eruptive (23 October 2014) image acquired by L8. (B) L8 acquired on 24 November 2014: it shows two active fresh lava flows. Bright yellow-orange pixels illustrate areas of very high temperature of crustal component and/or an increase in the areas of high temperature fractures and bright green pixels within the lava flow are related to saturated signal. (C) EO-1 image acquired on 16 December 2014; shows the advancement of the lava flow on west and north. (D) EO-1 acquired on 18 December 2014. (E) EO-1 acquired on 24 December 2014. (F) L8 image acquired on 11 January 2015.
Lava flow mapping by using multispectral sensors relies generally on near-IR bands (1.6–2.2 μm) to map hot lava flow, and on Thermal IR bands (10–11 μm) to map cooling lava which tends to form lava tubes (Flynn et al., 1994). In this context, because of ALI sensor has not thermal infrared bands and L8-TIRS bands have a spatial resolution of 100 m per pixel, we have used two different approaches to map active and cooling lava flows. L8 and ALI images were synergically used to produce the chrono-contour of active lava flow (Figure 5) by visually inspecting the images at their best zoom (Flynn et al., 2001). The change detection was produced to explore the possibility to derive additional information on cooling flow-fields besides hot lava characterization obtained by the optical multi-sensors time series.
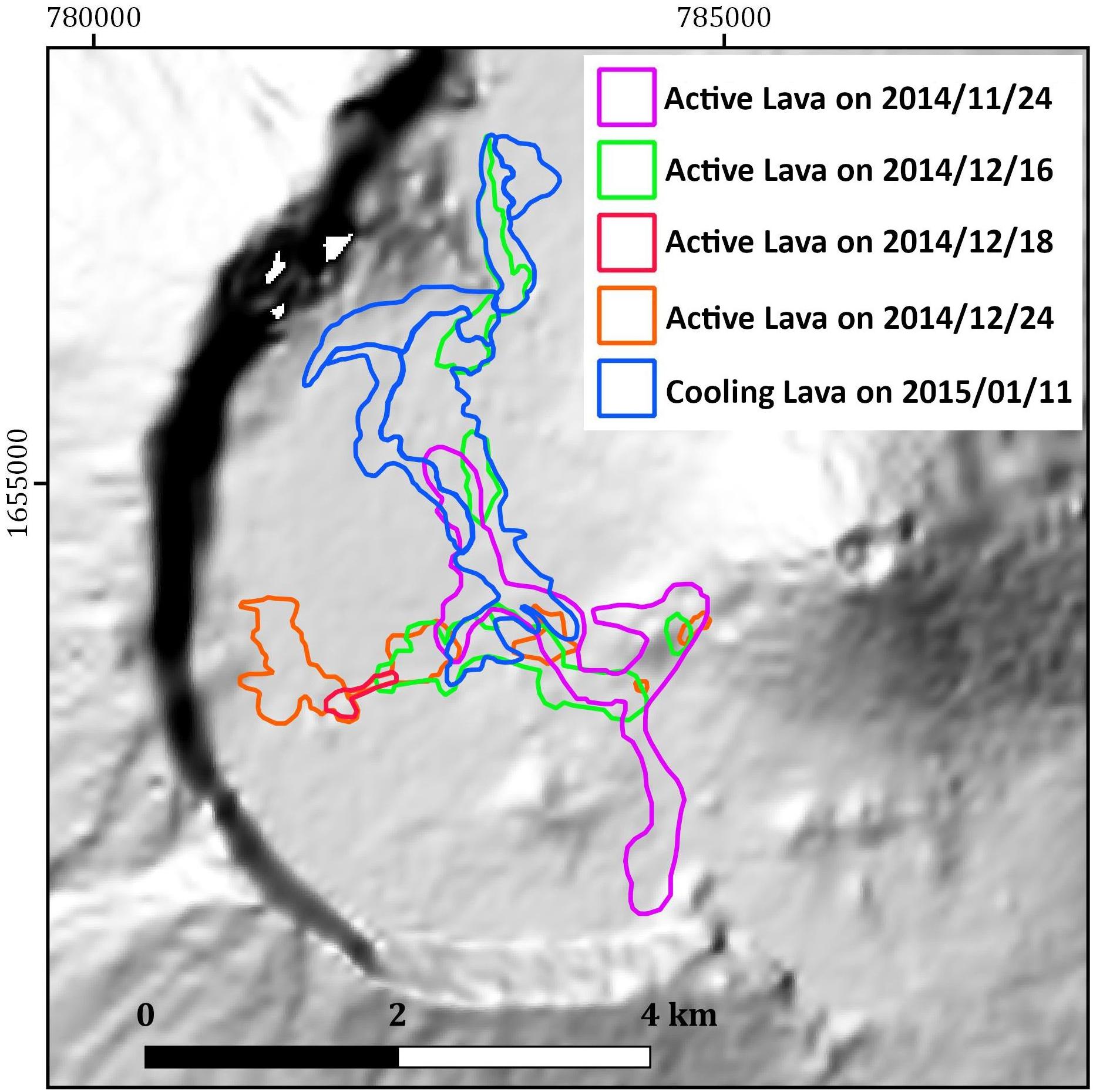
Figure 5. Active lava perimeters derived by visual delineation of L8-OLI and EO1-ALI SWIR bands and change image from October 2014–January 2015 L8 data provide the flow fields evolution.
In order to implement the change detection, the images were previously converted into reflectance, and to improve the spatial resolution of multispectral channels, we adopted the pan-sharpening Graham–Smidth method implemented in ENVI© software for resampling the bands originally at 30 m resolution into the 15 m resolution of L8-OLI panchromatic channel (band 8).
The pan-sharpened SWIR channel of L8-OLI was used to calculate a change detection map between the images captured on 23 October 2014 and 11 January 2015 (Figure 6) using the automatic co-registration option offered by ENVI©. Several bands were tested and best result was obtained by using the band 7. The change detection map highlights in gray scale the subsequent lava flows. The color of lava flow from light gray pixels to dark pixel can indicate a combined effect of lava thickness, composition and cooling areas. The thermal L8-TIRS band, at 10 μm and with a spatial resolution of 100 m, acquired on 11 January 2015 was used to support the interpretation regarding the cooling effect, although the TIR imagery is affected by reflectance component being acquired at daytime (see Figure 6 inset).
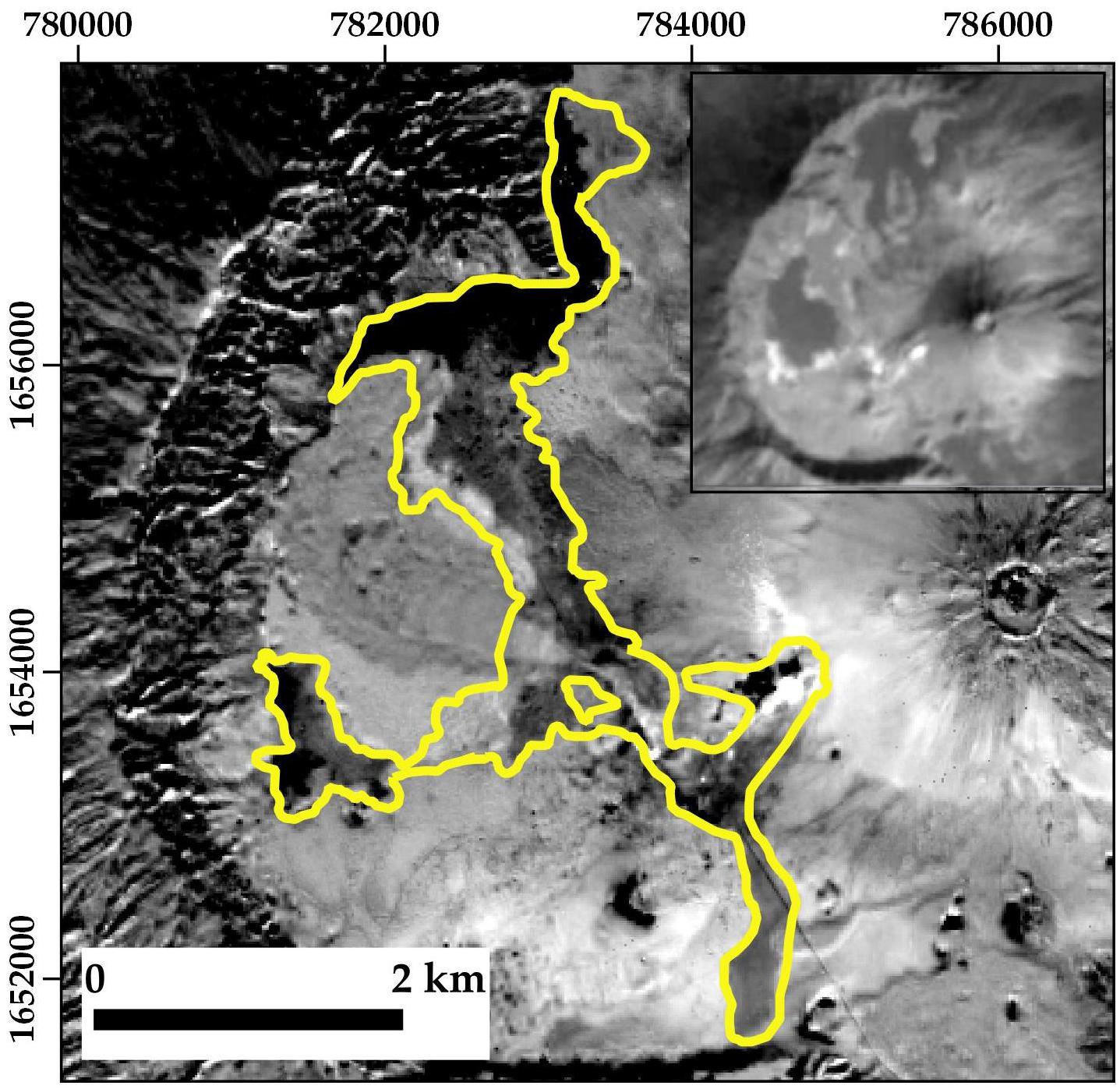
Figure 6. The results of SWIR pan-sharp change detection between pre- (October 2014) and post-eruption (January 2015) data. The inset (top right) shows the L8-TIRS thermal band at 10 μm (January 2015). The variation of the gray tones mainly results by chronology, and cooling phases. The yellow polygon was drawn to highlight the detected whole change by visual inspection.
Lava Field Emplacement Evolution
The results about emitted lava estimated by SAR and optical images were compared in order to assess their capability to monitor the temporal evolution of the event. The plot reported in Figure 7 shows four lines indicating the lava coverage in square km at each satellite time acquisition. The green and yellow lines refer to SAR data results, the blue line is the analysis performed with optical dataset, and the red one corresponds to the evaluation done by the Emergency Mapping Service (EMS) of Copernicus3 by exploiting satellite images acquired by very high resolution sensors from many space missions.
Optical analysis has a very similar trend with respect to EMS data, showing a very close effusion rate (0.10 km2/day for our data and 0.083 km2/day for EMS) and few square km of bias (about 1 km2) at the early stage of the effusion. These small discrepancies can be attributed to the ground resolution of the images used for the two estimates, i.e., medium resolution sensors in our dataset vs. very high resolution sensors in EMS study.
Synthetic aperture radar intensity only estimates the superficial effusion rate and lava total area smaller with respect to the one estimated from the other two datasets (green curve in Figure 7). The lava flow is a complex surface scenario, which cannot be explained with only change in roughness of the surface, which is the ground parameter that strongly affects SAR intensity signal. We think that the new emitted lava does not completely change the roughness of the soils, because the area of Chã das Caldeiras was already covered by lava (from previous eruptions) that can be characterized by similar texture. This can partially explain why the SAR intensity is underestimating it. Considering the SAR intensity and coherence results (yellow trend in Figure 7), two different temporal phases on the evolution of the surface changes are highlighted. The first section concerns the coverage estimation before the third SAR image taken on 9 December 2014. In this phase, SAR change detection underestimates the coverage with respect to the EMS maps. Actually, these set of data do not take into account the InSAR coherence information because the first two change coherence maps are related to SAR pairs that have a high temporal baseline, i.e., 24 days of separation (either ascending or descending pairs). For these maps, the coherence loss is not only due to changes in surface scattering because of lava emplacement, but also because of the effects of temporal decorrelation due to changes in the vegetation cover (Zebker and Villasenor, 1992). Moreover, in this first phase, the volcanic cloud was still present, and it represents another source of coherence loss (Jung et al., 2016). Therefore, we omitted the first two coherence maps because the decrease of coherence was also due to reasons other than lava within the caldera of Fogo Island (see Supplementary Figure S1). The second section of the trend (after 9 December 2014) concerns the merged information coming from both SAR intensity and coherence change maps. In this part, SAR results overestimate EMS results, and the total area coverage is 4 km2 larger than the one measured by SAR intensity only (green line). Maps reported in Figure 3 show where the overestimation occurred, and probably it is due to the InSAR coherence feature. The pictures highlight that the algorithm identifies some changes on the Pico do Fogo flanks (north sector) and few “false alarm” pixels in the northwest of Chã das Caldeiras (see lava coverage for dates 9 and 14 December 2014, in Figure 3). We calculated, by manually delineating “false alarms” areas, that the surface extension of these areas is about 2 km2, which basically corresponds to the overestimation with respect to EMS maps and optical analysis. These changes, which were detected by S1 InSAR coherence drop off, are not mapped using other datasets. It is worth considering that here a “real ground truth” is not available, hence, in case we assume that S1 intensity and coherence method overestimate the surface effusion rate, a possible reason for coherence loss is still the presence of the volcanic cloud4 (multispectral images in Figure 4), as discussed by Jung et al. (2016). Although SAR coherence seems to overestimate the lava emplacement, the information carried out by such feature is extremely useful to compensate the systematic underestimation of SAR intensity data only. This is clearly reported in Figure 7, where the line related to the SAR intensity only (in green) is constantly below the estimates from optical and EMS data. It is also important to point out that the results from S1 data are obtained using a completely automatic procedure and with lower resolution data, with respect to those from other datasets. Results clearly show the synergic role of SAR intensity and SAR InSAR coherence for lava mapping.
Volcanic Source Modeling
Beside the change detection approach, S1 and CSK images were also exploited to map the ground deformation caused by the eruption. The master–slave images for each sensor and orbit are evidenced in Table 1, and they are all syn-eruptive. For this purpose, we processed the data by means of classical DInSAR (Zebker et al., 1994), and we calculated four interferograms. The SRTM DEM (Farr et al., 2007) was used to remove the topographic phase contribution, and the multi-looking technique was applied to reduce noise and to obtain products sampled at a square pixel size of 20 × 20 and 15 × 15 m2 for S1 and CSK, respectively. Even though the two SAR systems operate with different bands (C and X band) and with different incidence angles (S1 at about 43.5° on ascending orbit and 35° on descending orbit; CSK at about 20° for both ascending and descending images), the patterns of the ground displacement are coherent (Figure 8). Indeed, inside the caldera, two lobes of deformation are visible on both S1 and CSK ascending interferograms. It is also worth to note the fringe pattern that is present on the south flank of Pico do Fogo on both S1 and CSK descending data. Of course, the fringe rate is different because of the different wavelengths.
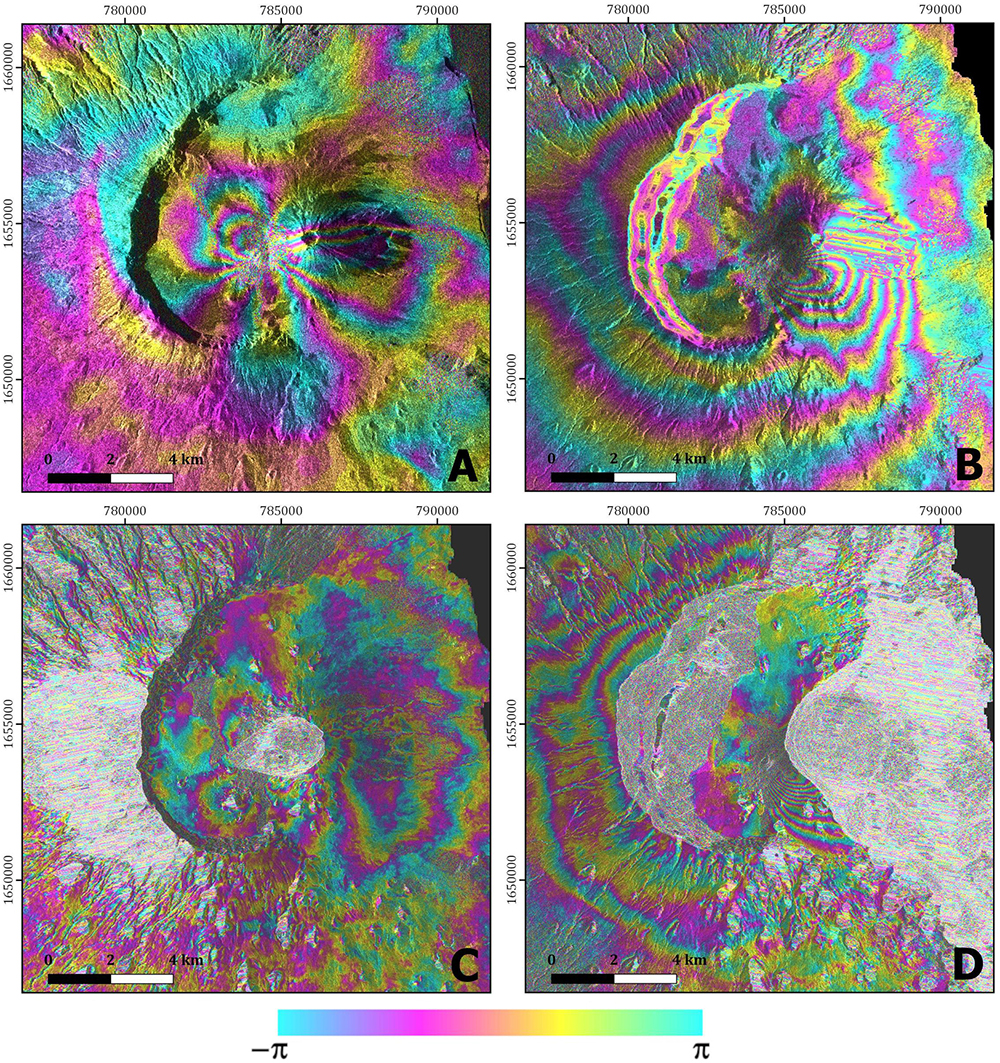
Figure 8. Wrapped interferograms obtained from S1 ascending (A), S1 descending (B), CSK ascending (C), and CSK descending (D) images. Two lobes of deformation are visible on both S1 and CSK ascending interferograms within the caldera. The south flank of Pico do Fogo is characterized by a very similar fringe arrangement, on S1 and CSK descending data.
These ground deformation estimates were exploited to retrieve the volcanic source modeling and to constrain the syn-eruptive feeding dyke. The four interferograms are subsampled with a step of 180 m in the inner caldera of Fogo, and 540 m outside, for a total of about 13,000 datapoints. The inversions were performed by means of the Volcano and Seismic source Modeling (VSM) tool (Trasatti, 2019). The code allows considering several analytical models of volcanic source, whose parameters are retrieved by non-linear inversion. The inversion is then followed by an appraisal stage based on a Bayesian approach aimed at finding the most probable parameters (instead the single best-fit model), obtaining posterior PDF (Sambridge, 1999). Several attempts were computed in order to find the most suitable geometrical source to reproduce the observed data. We find that pressurized sources such as a sphere or a spheroid are not suitable since they are unable to reproduce the wide negative line of sight (LOS) area in the eastern sector of the volcano. Instead, an opening dyke (Okada, 1992) reproduces the highest LOS values reaching 9–12 cm (opposite signs in the ascending/descending orbits, being the movement horizontal) and the corresponding opposite lobes (Figure 9 and Supplementary Figure S2 for CSK data). The misfit obtained, based on the chi-squared function, amounts to 14.8, while the null solution relating data to their uncertainties is 25.5. The dyke, whose trace is represented with the black line in Figure 9, is SW-NE oriented, and it is located inside Chã das Caldeiras, SW of the Pico do Fogo. Our results show that it slightly dips of 86° toward SE, and opens about 60 cm, for a total volume change of 2.0 ± 0.6 106 m3 in the observation time span (i.e., one month baseline and up to 8 days after the start of the eruption). The mean parameters’ values are reported in Table 2, while the posterior PDFs are reported in Supplementary Figure S3. The top depth of the dyke was fixed at 100 m below the Fogo’s mean altitude. Figure 9 also shows the agreement between the constrained lava flow and the surface projection of the feeding dyke retrieved by an independent technique.
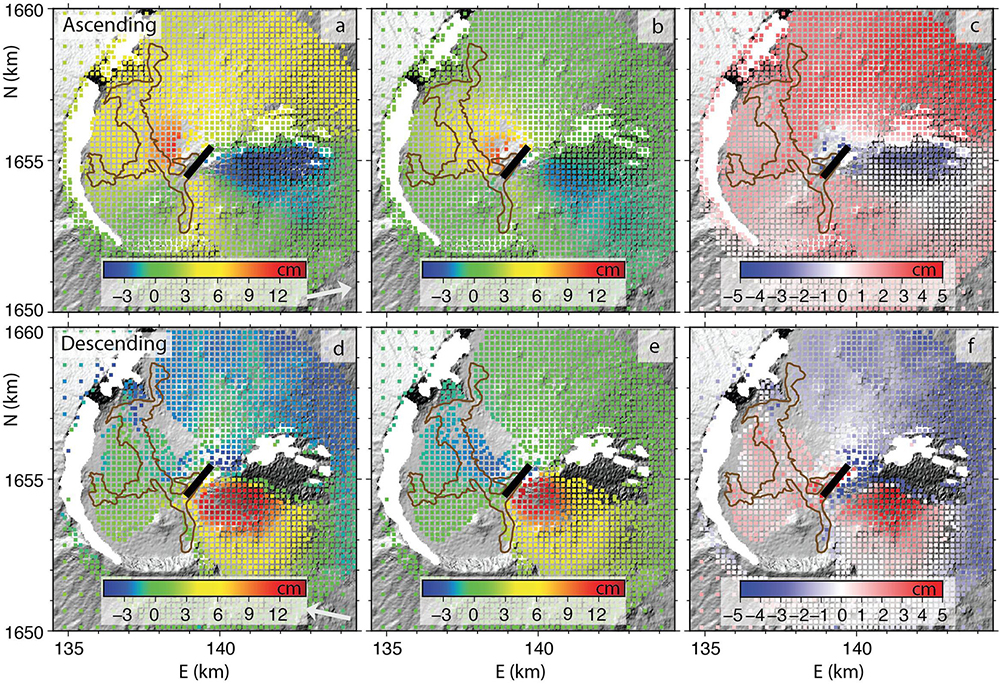
Figure 9. Results of the VSM inversion. Comparison between observed (a,d) and modeled (b,e) data, and residuals (c,f) in ascending and descending orbits of S1 satellites. The black line is the surface projection of the feeding dyke. E and N are UTM projection, zone 27. The brownish transparency is the lava from SWIR pan-sharp change detection. Similar results for CSK mission are shown in Supplementary Figure S2.
Discussion
We have presented the results of a novel automatic technique to estimate the lava flow propagation during the effusive eruption of Fogo, adopting a multi-sensor approach. The area coverage obtained by visual analysis of medium resolution imagery (L8 and EO-1) is estimated equal to 4.97 km2, in line with the results of EMS and by Cappello et al. (2016). This value is very close to the one estimated with more sophisticated techniques that require more computational efforts or in situ measurements, such as differential DEM (Bagnardi et al., 2016), and Terrestrial Laser Scanner (TLS) combined with structure from motion data (Richter et al., 2016). Bagnardi et al. (2016) and Richter et al. (2016) estimate a lava coverage for this eruption of 4.8 and 4.85 km2, respectively, i.e., about 0.1 km2 of difference with respect our results. This demonstrates that optical moderate resolution data are quite informative for this kind of application and are able to provide a cinematic estimate of lava emplacement. The temporal evolution information provided by the proposed approach can be delivered during an emergency phase. On the contrary, techniques such as DEM difference that require stereoscopic optical images, or TLS, that is based on field campaigns, are often prevented when an eruption is ongoing (e.g., with volcanic clouds and active lava flows). SAR data, on the other side, play a key role in such context, when prompt information is important for human rescue purposes, because of their all-weather and day-night capabilities, and their intrinsic suitability for a full automatic generation of change detection product. The HSBA presented in our work is more than a proof of concept of an operational tool, as in the case of flood mapping (Chini et al., 2017). The accuracy of SAR intensity and InSAR coherence lava maps seems to be lower than other approaches, giving under- and over-estimation of the total lava, at the beginning and at the end of the eruption (about 2 km2), respectively. Of course, results from other methods based on earth observation data too are affected by errors as well. Moreover, it is worth to note that S1 mission was not fully operational at the time of Fogo eruption. Indeed, only one satellite was orbiting at that time, so that the temporal baseline between two consecutive acquisitions was twelve days (with two satellites it is reduced to 6 days). This latter is an important parameter for reducing false alarms caused by vegetation when InSAR coherence is used. The opportunity offered by SAR constellation missions, as in the case of ESA’s S1, with weekly delivered acquisitions (or even more frequent as in the case of the CSK mission) was shown. Still some efforts have to be put in place to improve the results, correcting false alarms, and tuning algorithms, considering that volcanic areas during an eruption phase are quite challenging scenarios since different phenomena occur (e.g., ash and gas emissions). In synthesis, the comparison between the lava mapping results obtained from optical and SAR data suggests their possible automatic integration for supporting a crisis phase. Indeed, more complex approaches, such as DEM difference or TLS, even though they are expected to be more accurate (e.g., giving volume estimates too) cannot be applied easily or automatically when an eruption is ongoing. Despite some discrepancies are present in the analysis shown above, the synergic use of optical and SAR data could provide high-rate (almost daily) temporal information about lava evolution, thanks to the complementarity of such sensors, and automation of data processing.
The feeding dyke constrained by the geodetic inversion is located SW of the Pico do Fogo summit, in accordance with the area of the lava flow mapped by SAR change detection and optical images. The eruptive vent location follows the favorable calculated post-collapse stress field within the local crust (Maccaferri et al., 2017). The retrieved dyke shares position and dimension with previous analyses (González et al., 2015; Bagnardi et al., 2016) of the 2014–2015 eruption, and of the 1995 eruption (Amelung and Day, 2002). The volumes of the retrieved inflating dykes are comparable within uncertainty, being 3 ± 2 106 m3 that inferred by González et al. (2015). The syn-eruptive dyke is referred for the 3–8 days of the eruption, being the SAR slave images taken on the 27th, 29th November, and 2nd December (Table 1), while the eruption lasted for 78 days. Even considering the area and volumes related to the first days of the eruption (one to few tens of 106 m3, Bagnardi et al., 2016; Cappello et al., 2016; Calvari et al., 2018), the magma volume intruded is still only a small fraction of the erupted volume. This suggests that the shallow dyke inferred from SAR data is the lateral, sub-vertical pathway of the magma to be erupted and it is not representative of the volume actually extruded. From one hand, this is confirmed by the lack of pre-eruptive deformation and deflation after the end of the eruption (González et al., 2015), and from other hand, by the development of tubes (Calvari et al., 2018). We can conclude that the lava flow extension and volume, and the retrieved feeding dyke volume are not directly connected. Instead, we demonstrate that the full exploitation of SAR data allows quantifying multi-hazards in volcanic areas during eruptions.
As a final remark, a deeper magmatic source feeding the eruption was not retrieved both for the 1995 and 2014–2015 events (Amelung and Day, 2002; González et al., 2015). The eruptions at Fogo are fed by mantle-lithospheric source, according to petrological and geochemical data, assumed to be located below 16 km depth (Hildner et al., 2011; Calvari et al., 2018).
Conclusion
The present work is a multidisciplinary and multi-sensor study of the main hazards related to the 2014–2015 Fogo Island effusive eruption. In particular, we propose an innovative and automatic method to exploit SAR data, and their joint use with optical imageries to map the lava field emplacement. For these purposes, we have used the images acquired by the ESA’s S1, ASI’s CSK, and NASA/USGS L8 and EO-1 missions. The comparative and synergic use of this multi-sensor dataset has allowed estimating the temporal evolution of lava coverage in the Chã das Caldeiras by applying visual inspection of optical imagery and by testing the capability of a novel automatic change detection algorithm using SAR data. Our improved change detection algorithm is based on multiscale tiling approach to identify changes occurred on the SAR intensity and it combines information from the InSAR coherence to detect changes which are not directly related to changes on surface roughness. The approach is completely automatic and adaptive, showing high capability to detect different extent of lava flows. The lava flow analysis was then complemented by the geodetic observations. Indeed, thanks to DInSAR we have mapped the whole ground deformation due to the lava ascent. By inverting them, we have also identified the dyke feeding the eruption. The achieved results are in agreement with previous findings, even if based on different dataset and methods. We demonstrate how open access multi-sensor satellite imagery can be used in synergy to provide hazard information in an operational environment, when volcanic activity limits the use of single sensor data and/or on field measurements.
Data Availability Statement
The Sentinel-1, Earth-Observing-1, and Landsat-8 datasets for this study can be found in the ESA (https://scihub.copernicus.eu/dhus/#/home) and USGS (https://earthexplorer.usgs.gov). CSK images are not publicly available because were provided by means of a direct agreement between ASI and INGV.
Author Contributions
CB led the conception of the manuscript and processed SAR data for deformation mapping. MC worked on the SAR change detection analysis by developing and running the hierarchical approach. ET retrieved the volcanic source by inverting DInSAR deformation data. SA processed the satellite data to estimate change maps from Optical sensors. All authors contributed to the manuscript preparation and interdisciplinary analysis.
Funding
MC contribution was supported by the Luxembourg National Research Fund through the MOSQUITO project (Grant CORE C15/SR/10380137).
Conflict of Interest
The authors declare that the research was conducted in the absence of any commercial or financial relationships that could be construed as a potential conflict of interest.
Acknowledgments
The authors thank space agencies for data provision: ESA for Sentinel-1 dataset and ASI for the kind delivery of COSMO-SkyMed images, USGS for Landsat-8 and EO-1 set. Useful discussions with G. Ventura and M. Bagnardi are acknowledged.
Supplementary Material
The Supplementary Material for this article can be found online at: https://www.frontiersin.org/articles/10.3389/feart.2020.00022/full#supplementary-material
Footnotes
- ^ https://eo1.gsfc.nasa.gov/
- ^ http://landsat.gsfc.nasa.gov/landsat-8
- ^ https://emergency.copernicus.eu/
- ^ https://volcano.si.edu/volcano.cfm?vn=384010
References
Ajadi, O., Meyer, F., and Webley, P. (2016). Change detection in synthetic aperture radar images using a multiscale-driven approach. Remote Sens. 8:482. doi: 10.3390/rs8060482
Amelung, F., and Day, S. (2002). InSAR observations of the 1995 Fogo, cape verde, eruption: implications for the effects of collapse events upon island volcanoes. Geophys. Res. Lett. 2:1606. doi: 10.1029/2001GL013760
Bagnardi, M., González, P. J., and Hooper, A. (2016). High-resolution digital elevation model from tri-stereo Pleiades-1 satellite imagery for lava flow volume estimates at Fogo Volcano. Geophys. Res. Lett. 43, 6267–6275. doi: 10.1002/2016GL069457
Bignami, C., Ruch, J., Chini, M., Neri, M., Buongiorno, M. F., Hidayati, S., et al. (2013). Pyroclastic density current volume estimation after the 2010 Merapi volcano eruption using X-band SAR. J. Volcanol. Geoth. Res. 261, 236–243. doi: 10.1016/j.jvolgeores.2013.03.023
Bosi, V. MIAVITA group, (2012). Handbook for Volcanic Risk Management: Prevention, Crisis Management and Resilience. France: BRGM Orleans.
Bovolo, F., and Bruzzone, L. (2005). A detail-preserving scale-driven approach to change detection in multitemporal SAR images. IEEE Trans. Geosci. Remote Sens. 43, 2963–2972. doi: 10.1109/TGRS.2005.857987
Bruzzone, L., and Prieto, D. F. (2002). An adaptive semiparametric and context-based approach to unsupervised change detection in multitemporal remote-sensing images. IEEE Trans. Image Process. 11, 452–466. doi: 10.1109/TIP.2002.999678
Calvari, S., Ganci, G., Victória, S., Hernandez, P., Perez, N., Barrancos, J., et al. (2018). Satellite and ground remote sensing techniques to trace the hidden growth of a lava flow field: the 2014–2015 effusive eruption at fogo volcano (Cape Verde). Remote Sens. 10:1115. doi: 10.3390/rs10071115
Cappello, A., Ganci, G., Calvari, S., Pérez, N. M., Hernández, P. A., Silva, S. V., et al. (2016). Lava flow hazard modeling during the 2014-2015 Fogo eruption. Cape Verde. J. Geophys. Res.: Solid Earth 121, 2290–2303. doi: 10.1002/2015JB012666
Chini, M., Bignami, C., Stramondo, S., and Pierdicca, N. (2008). Uplift and subsidence due to the 26 December 2004 Indonesian earthquake detected by SAR data. Int. J. Remote Sens. 29, 3891–3910. doi: 10.1080/01431160701871112
Chini, M., Hostache, R., Giustarini, L., and Matgen, P. (2017). A Hierarchical split-based approach for parametric thresholding of SAR images: flood inundation as a test case. IEEE Trans. Geosci. Remote Sens. 55, 6975–6988. doi: 10.1109/TGRS.2017.2737664
Chini, M., Pelich, R., Hostache, R., Matgen, P., and Lopez-Martinez, C. (2018). Towards a 20 m global building map from sentinel-1 SAR data. Remote Sens. 10:1833. doi: 10.3390/rs10111833
Chini, M., Pelich, R., Pulvirenti, L., Pierdicca, N., Hostache, R., and Matgen, P. (2019). Sentinel-1 InSAR coherence to detect floodwater in urban areas: houston and hurricane harvey as a test case. Remote Sens. 11:107. doi: 10.3390/rs11020107
Chini, M., Piscini, A., Cinti, F. R., Amici, S., Nappi, R., and DeMartini, P. M. (2013). The 2011 Tohoku (Japan) tsunami inundation and liquefaction investigated through optical. thermal, and SAR data. IEEE Geosci. Remote Sens. Lett. 10, 347–351. doi: 10.1109/LGRS.2012.2205661
Chini, M., Pulvirenti, L., and Pierdicca, N. (2012). Analysis and Interpretation of the COSMO-SkyMed Observations of the 2011 Japan Tsunami. IEEE Geosci. Remote Sens. Lett. 9, 467–471. doi: 10.1109/LGRS.2011.2182495
Day, S., Carracedo, J. C., Gillou, H., Fonseca, J. F. B. D., Heleno, S. I. N., Pais, J., et al. (2000). Comparison and cross-checking of evidence for the location and type of historical eruptions in ocean island volcanoes. in: the archaeology of geological catastrophes; McGuire, W., volcanoes, earthquakes and archeology. special publication. Geol. Soc. Lon. 171, 281–306. doi: 10.1144/gsl.sp.2000.171.01.21
Day, S. J., Heleno da Silva, S. I. N., and Fonseca, J. F. B. D. (1999). A past giant lateral collapse and present-day flank instability of Fogo. Cape Verde Islands. J. Volcanol. Geothermal Res. 94, 191–218. doi: 10.1016/S0377-0273(99)00103-101
Faria, B., and Fonseca, J. F. B. D. (2014). Investigating volcanic hazard in Cape Verde Islands through geophysical monitoring: network description and first results. Nat. Hazards Earth Syst. Sci. 14, 485–499. doi: 10.5194/nhess-14-485-2014
Farr, T. G., Rosen, P. A., Caro, E., Crippen, R., Duren, R., Hensley, S., et al. (2007). The shuttle radar topography mission. Rev. Geophys. 45, 1–33. doi: 10.1029/2005RG000183
Flynn, L. P., Harris, A. J. L., Rothery, R. D. A., and Oppenheimer, C. (2000). High-Spatial resolution thermal remote sensing of active volcanic features using Landsat and hyperspectral data. Remote Sens. Active Volcanism AGU Geophys. Monogr. Ser. 116, 161–177. doi: 10.1029/gm116p0161
Flynn, L. P., Harris, A. J. L., and Wright, R. (2001). Improved identification of volcanic features using Landsat 7 ETM+. Remote Sens. Environ. 78, 180–193. doi: 10.1016/S0034-4257(01)00258-259
Flynn, L. P., Mouginis-Mark, P. J., and Horton, K. A. (1994). Distribution of thermal areas on an active lava flow field: landsat observations of Kilauea, Hawaii, July 1991. Bull.Volcanol. 56, 284–296. doi: 10.1007/BF00302081
Giustarini, L., Hostache, R., Matgen, P., Schumann, G. J.-P., Bates, P. D., and Mason, D. C. (2013). A change detection approach to flood mapping in urban areas using terraSAR-X. IEEE Trans. Geosci. Remote Sens. 51, 2417–2430. doi: 10.1109/TGRS.2012.2210901
González, P. J., Bagnardi, M., Hooper, A. J., Larsen, Y., Marinkovic, P., Samsonov, S. V., et al. (2015). The 2014-2015 eruption of fogo volcano: geodetic modeling of Sentinel-1 TOPS interferometry. Geophys. Res. Lett. 42, 9239–9246. doi: 10.1002/2015GL066003
Haralick, R. M., and Shapiro, L. G. (1985). Image segmentation techniques. Comput. Vis. Graph. Image Process. 29, 100–132. doi: 10.1016/S0734-189X(85)90153-90157
Hildner, E., Klügel, A., and Hauff, F. (2011). Magma storage and ascent during the 1995 eruption of Fogo, Cape Verde Archipelago. Contrib. Mineral. Petr. 162, 751–772. doi: 10.1007/s00410-011-0623-6
Hoffmann, J. (2007). Mapping damage during the Bam (Iran) earthquake using interferometric coherence. Int. J. Remote Sens. 28, 1199–1216. doi: 10.1080/01431160600928567
Jung, J., Kim, D., Lavalle, M., and Yun, S.-H. (2016). Coherent change detection using InSAR Temporal decorrelation model: a case study for volcanic ash detection. IEEE Trans. Geosci. Remote Sens. 54, 5765–5775. doi: 10.1109/TGRS.2016.2572166
Lee, S.-K., Lee, C.-W., and Lee, S. (2015). A comparison of the Landsat image and LAHARZ-simulated lahar inundation hazard zone by the 2010 Merapi eruption. Bull. Volcanol. 77:46. doi: 10.1007/s00445-015-0920-924
Maccaferri, F., Richter, N., and Walter, T. R. (2017). The effect of giant lateral collapses on magmapathways and the location of volcanism. Nat. Communi. 8:1097. doi: 10.1038/s41467-017-01256-1252
Okada, Y. (1992). Internal deformation due to shear and tensile faults in a half-space. B Seismol. Soc. Am. 82, 1018–1040.
Pierdicca, N., Anniballe, R., Noto, F., Bignami, C., Chini, M., Martinelli, A., et al. (2018). Triple collocation to assess classification accuracy without a ground truth in case of earthquake damage assessment. IEEE Trans. Geosci. Remote Sens. 56, 485–496. doi: 10.1109/TGRS.2017.2750770
Ribeiro, O. (1960). A ilha do Fogo e as Suas Erupções; Memórias, Série Geográfica, 12th Edn. Lisbon: Junta de Investigações do Ultramar.
Richter, N., Favalli, M., de Zeeuw-van Dalfsen, E., Fornaciai, A., da Silva Fernandes, R. M., Pérez, N. M., et al. (2016). Lava flow hazard at fogo volcano, cabo verde, before and after the 2014-2015 eruption. Nat. Hazards Earth Syst. Sci. 16, 1925–1951. doi: 10.5194/nhess-16-1925-2016
Rosin, P. L. (2002). Thresholding for change detection. Comput. Vis. Image Underst. 86, 79–95. doi: 10.1006/cviu.2002.0960
Rothery, D. A., Francis, P. W., and Wood, C. A. (1988). Volcano monitoring using short wavelength infrared data from satellites. J. Geophys. Res. 93:7993. doi: 10.1029/JB093iB07p07993
Sambridge, M. (1999). Geophysical inversion with a neighbourhood algorithm - II. Appraising the ensemble. Geophys. J. Int. 138, 727–746. doi: 10.1046/j.1365-246x.1999.00900.x
Trasatti, E. (2019). Volcano and Seismic source Modelling – VSM. Avaliable at: http://www.rohub.org/rodetails/volcano_source_modelling_vsm-release/overview (accessed January 2020).
Valade, S., Ley, A., Massimetti, F., D’Hondt, O., Laiolo, M., Coppola, D., et al. (2019). Towards global volcano monitoring using multisensor sentinel missions and artificial intelligence: the MOUNTS monitoring system. Remote Sens. 11:1528. doi: 10.3390/rs11131528
Wright, R., Flynn, L., and Harris, A. (2001). Evolution of lava flow-fields at mount etna, 27-28 October 1999, observed by Landsat 7 ETM+. Bull. Volcanol. 63, 1–7. doi: 10.1007/s004450100124
Zebker, H. A., Rosen, P. A., Goldstein, R. M., Gabriel, A., and Werner, C. L. (1994). On the derivation of coseismic displacement fields using differential radar interferometry: the landers earthquake. J. Geophys Res.: Solid Earth 99, 19617–19634. doi: 10.1029/94JB01179
Keywords: lava, volcanic source modeling, synthetic aperture radar, optical images, change detection, hierarchical-split-based approach, DInSAR coherence, Fogo volcano
Citation: Bignami C, Chini M, Amici S and Trasatti E (2020) Synergic Use of Multi-Sensor Satellite Data for Volcanic Hazards Monitoring: The Fogo (Cape Verde) 2014–2015 Effusive Eruption. Front. Earth Sci. 8:22. doi: 10.3389/feart.2020.00022
Received: 02 September 2019; Accepted: 24 January 2020;
Published: 11 February 2020.
Edited by:
Nico Fournier, GNS Science, New ZealandReviewed by:
Thomas R. Walter, German Research Centre for Geosciences, Helmholtz Centre Potsdam, GermanyEisuke Fujita, National Research Institute for Earth Science and Disaster Resilience (NIED), Japan
Copyright © 2020 Bignami, Chini, Amici and Trasatti. This is an open-access article distributed under the terms of the Creative Commons Attribution License (CC BY). The use, distribution or reproduction in other forums is permitted, provided the original author(s) and the copyright owner(s) are credited and that the original publication in this journal is cited, in accordance with accepted academic practice. No use, distribution or reproduction is permitted which does not comply with these terms.
*Correspondence: Christian Bignami, christian.bignami@ingv.it