- School of Geography, Earth and Environmental Sciences, University of Birmingham, Edgbaston, United Kingdom
The use of low-cost sensor networks (LCSNs) is becoming increasingly popular in the environmental sciences and the unprecedented monitoring data generated enable research across a wide spectrum of disciplines and applications. However, in particular, non-technical challenges still hinder the broader development and application of LCSNs. This paper reviews the development of LCSNs over the last 15 years, highlighting trends and future opportunities for a diverse range of environmental applications. We found air quality, meteorological and water-related networks were particularly well represented with few studies focusing on sensor networks for ecological systems. Furthermore, we identified bias toward studies that have direct links to human health, safety and livelihoods. These studies were more likely to involve downstream data analytics, visualizations, and multi-stakeholder participation through citizen science initiatives. However, there was a paucity of studies that considered sustainability factors for the development and implementation of LCSNs. Existing LCSNs are largely focused on detecting and mitigating events which have a direct impact on humans such as flooding, air pollution or geo-hazards, while these applications are important there is a need for future development of LCSNs for monitoring ecosystem structure and function. Our findings highlight three distinct opportunities for future research to unleash the full potential of LCSNs: (1) improvement of links between data collection and downstream activities; (2) the potential to broaden the scope of application systems and fields; and (3) to better integrate stakeholder engagement and sustainable operation to enable longer and greater societal impacts.
Introduction
In recent years there has been a marked increase in the use of low-cost sensor networks (LCSNs) in the environmental sciences to address both pure research questions and applied management issues (Benedetti et al., 2010; Ojha et al., 2015; Prasad, 2015). As sensor networks with low-cost components in the setup, the rise of LCSNs has been driven by a number of factors including: the reduced cost of microcontrollers, communication modules and environmental sensors (Fisher et al., 2015), and the open science movement, which has seen the research community readily sharing designs, underlying software and firmware and data (Pearce, 2013). While there are some trade-offs with regards to robustness, calibration requirements and accuracy of low cost sensors when compared to high–end commercial sensors (Castell et al., 2017), the potential for greatly increased spatial coverage will facilitate new insights into environmental process dynamics (Krause et al., 2015). In addition to the low-cost, a key advantage of open source electronics and “DIY” sensor networks is that end-users can fully customize the network applications with potential to employ adaptive monitoring or real-time feedback and control (Blaen et al., 2016). This also enables specific monitoring or research requirements to be achieved in a number of contexts, such as smart earth and smart agriculture (Hart and Martinez, 2006; Ojha et al., 2015; Bakker and Ritts, 2018).
The technical aspects of low-cost sensor network design and application are now relatively well understood, thanks to the rapid development of information and communication technologies. However, recent research suggests that remaining challenges are largely focused around non-technical factors such as stakeholder engagements, socio-economic contexts, financial and operational mechanisms (Mao et al., 2018). These non-technical issues have already started to hinder the potential benefits these sensor networks can provide society. For example, the potential for risk reduction, resilience building, and adaptive management are frequently overlooked (Paul et al., 2018). These points are salient given the potential of low-cost sensor networks to address the inadequate data coverage in low- and mid-income countries (e.g., Strigaro et al., 2019), particularly as this lack of information remains a major challenge for policy makers in these regions (UN, 2015). Hence, there is an urgent need to better understand these emerging challenges and identify possible opportunities for future research.
Given the above, this study aims to quantitatively and systematically review and synthesize the contemporary literature on environmental LCSNs, in order to analyze current research foci and identify knowledge gaps. Reviewed publications are assessed in three non-technical dimensions that are believed to be critical for successful implementation of low-cost sensor networks and maximize their societal benefits (Mao et al., 2018) – first, clear workflow from data collection to data processing and provision (Paul et al., 2018); second, consideration of stakeholder groups (e.g., end-users and operators) in designing, using or managing sensor networks; and third, sustainable and adaptive setup of the sensor network. In doing so we sought to address four specific hypotheses, namely that: (H1) studies using LCSNs have a bias toward fields that have a in situ sensor monitoring tradition, such as meteorology; (H2) the predominate focus has been on data collection, with limited effort dedicated to other downstream data activities such as data visualization, analytics or real-time control; (H3) most studies focus on technically orientated single end-users (i.e., scientists), without considering the high potential for multi-stakeholder participation; and, (H4) given H3, the focus in the field has been on the technical feasibility of sensors and networks and the importance of factors such as sustainable operating mechanisms and physical and socio-economic contexts have been neglected.
Methods
The use of systematic review procedures to identify the state of the art in a given research field is becoming increasingly popular in the physical (e.g., Bartesaghi Koc et al., 2018), medical (e.g., Hill et al., 2016) and social sciences (e.g., Karpouzoglou et al., 2016). This approach facilitates a rigorous appraisal and synthesis of the literature in a (semi)-quantitative way to address specific hypotheses or research questions (Mulrow, 1987). Furthermore, the analytical tools and search engines now available enable large databases of academic literature to be searched and results categorized in short amounts of time (Xu and Marinova, 2013). However, search criteria must be carefully selected to ensure the pool of literature used is suited to the hypotheses or questions posed. Here we adopt the approach outlined by Pickering and Byrne (2014) which attempts to identify geographical patterns, theoretical trends, and methodological gaps rather than undertake statistical analysis of evidence as is common in the meta-analyses of the medical sciences.
To identify the body of literature for quantitative review we used the Web of Science database, which is the largest online database for searching peer reviewed scientific literature and the most academically orientated of the main search engines (Xu and Marinova, 2013). Our aim was to include papers from two general themes: (1) low-cost environmental sensors networks, and (2) low-cost technologies that have direct relevance to (low-cost environmental) sensor networks. To achieve this, we used the following search criteria:
TS = [(“sensor network∗”) AND (“low-cost” OR “lowcost” OR “opensource” OR “open source” OR “inexpensive”)]
(1)
where TS represents topic searching title, abstract and keywords that returned the initial pool of papers for consideration (n = 4593). The literature was then filtered to include only papers that were deemed explicitly related to environmental monitoring. To achieve this, we only included papers from Web of Science categories that were related to the geographical, environmental or earth sciences (see Supplementary Table S1 for list of categories). This step returned 218 articles from 153 journals and conferences proceedings.
These papers were then assessed in turn by examining the abstract or full manuscript to extract: (i) general information (publication year, country and study type); (ii) information on the environmental system studied (H1; i.e., Atmosphere, Hydrosphere, Earth, etc.); (iii) sensor mobility and data transmission/processing level (H2); (iv) user groups (H3); and, (v) sustainability considerations (H4). In order to consider how the existing studies utilize sensor networks, we also checked if the publications were: (1) focused on an environmental application of the technologies described; (2) describing a sensor network rather than a single sensor; or (3) focused on the measurement and collection of environmental data rather than the performance of the network per say. There were 135 publications meeting all the three criteria. More detailed information on this procedure can be found in Supplementary Table S2. All data collation and analysis was conducted using R version 3.5.1 and the Tidyverse ecosystem of packages outlined in Wickham and Grolemund (2016).
Results and Discussion
The concept of low-cost environmental sensor networks appears to have first emerged in the literature in 2004. Since this date there has been a steady increase in the number of publications per year, with the highest numbers (32 and 33) recorded in 2017 and 2018, respectively (Figure 1A). This result was expected as the increase in published studies tracks the growing trend toward open science and the rise of the “makers movement” within the wider scientific community (Baden et al., 2015). Interestingly, 2004 roughly coincides with the development and release of the Arduino board an inexpensive, consumer orientated microcontroller board1 and the increase in publications post 2012 also coincides with the release of the low cost, single board computer, Raspberry Pi2.
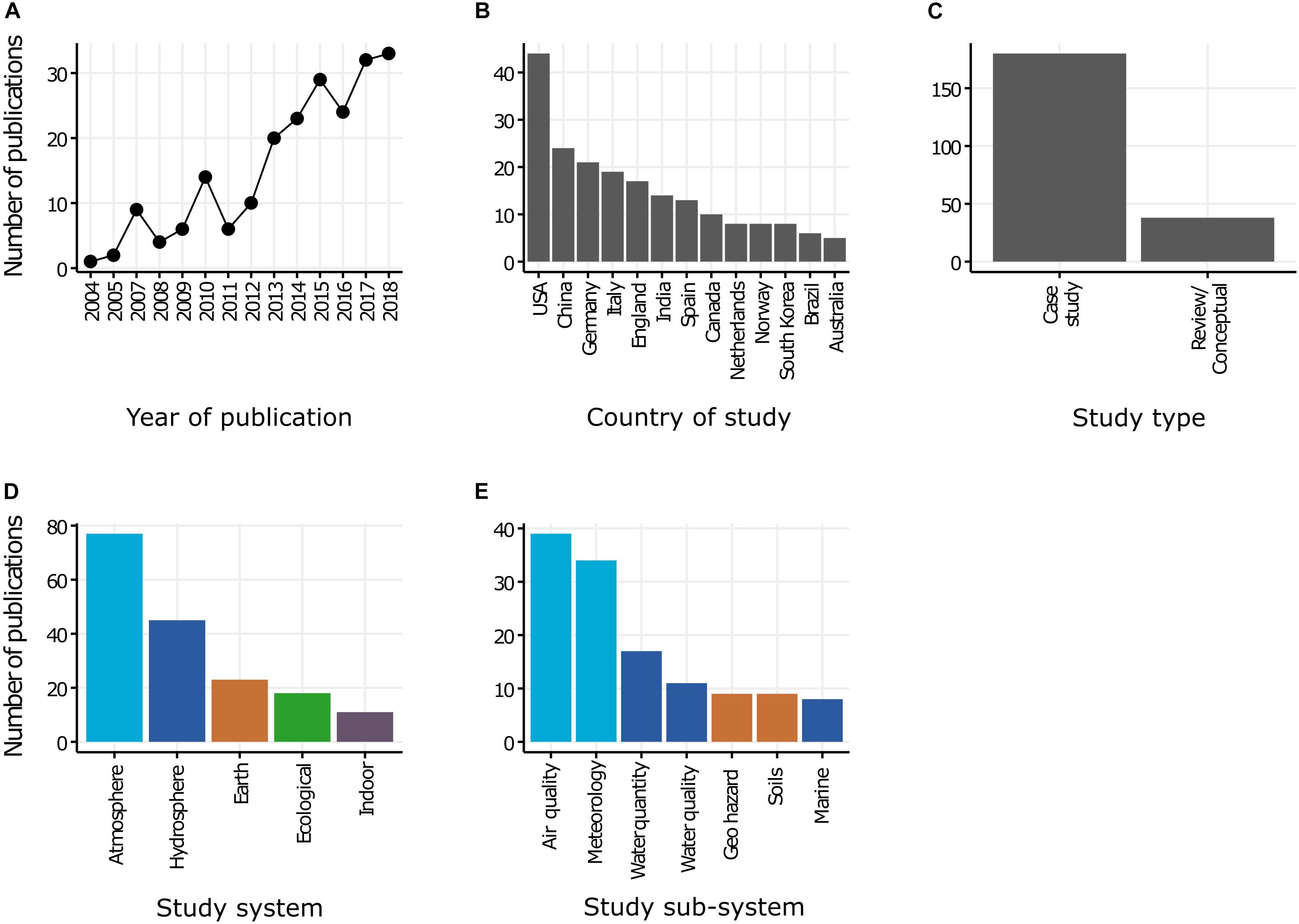
Figure 1. Number of publications as a function of: (A) year of publication, 2004–2018; (B) country in which the study was conducted; (C) the type of study or paper; (D) broad study system(s), and; (E) sub-categories identified within the air, water and earth study systems. In panel (B) only countries with >5 studies are displayed.
The global distribution of the analyzed studies displayed a distinct bias toward developed countries (particularly North America and Western Europe) with no studies from Africa and only a limited number from other developing regions (Figure 1B). This is concerning as, for example, the low number of African hydrological or meteorological monitoring stations hamper policy development and environmental management (van de Giesen et al., 2014). However, there are some projects underway such as the TAHMO project3 which aims to install 20,000 low-cost weather stations across Africa.
Most studies were single case studies with few review or conceptual articles captured by our literature search (Figure 1C). This disparity is likely to represent the relatively recent development of LCSNs as tools for environmental monitoring applications, particularly those used in peer reviewed scientific studies. The review papers were either focused on more general technological advances in environmental monitoring and not focused solely on low-cost networks (e.g., Rossiter, 2018), or provided a user group perspective on low cost sensor networks (e.g., citizen science; cf. Rai et al., 2017; Paul et al., 2018).
When considering the study system at a relatively coarse scale the literature appeared to support H1 (i.e., there was a bias toward fields with a history of in situ monitoring), with 77 publications focused on applications in the lower atmosphere and 19 on ecological systems (Figure 1D). Given the long history of sensor use for in situ atmospheric monitoring, particularly for meteorological variables, and limited use of sensors for monitoring ecological systems these results may not be surprising (Hart and Martinez, 2006). However, a larger number of the atmosphere system studies were focused on air pollution (n = 39), rather than displaying a bias toward meteorology as anticipated (n = 34) (Figure 1E). This was unexpected given the historical reliance on passive sampling and expensive laboratory equipment for analysis in air quality studies (Snyder et al., 2013). It appears public awareness of health risks (Ali et al., 2015; van Zoest et al., 2018), and the proliferation of low-cost in situ sensors (Schneider et al., 2017; Munir et al., 2019) are driving this trend. For water systems more studies focused on quantity (n = 17) as opposed to quality (n = 11). The water quantity studies were predominately focused on flooding (e.g., Horita et al., 2015; Acosta-Coll et al., 2018; Bartos et al., 2018), but studies on water resource management (e.g., Katsiri and Makropoulos, 2016) and the interface between agriculture and water resource monitoring were apparent (e.g., Kim et al., 2011; López et al., 2015). The water quality studies represented a mixture of pollution monitoring networks (e.g., Schneider et al., 2016) and agriculture focused applications (e.g., López Riquelme et al., 2009). Studies on earth systems were evenly distributed between those with a geo-hazard focus, such as landslides and earthquakes (Pumo et al., 2016; Finazzi and Fassò, 2017) and those with a focus on soil properties (e.g., Shaw et al., 2016). A further category was identified that represented studies focused on communication protocols or network architecture. An interesting trend was identified with many of these studies being pre 2012 (e.g., Bengston and Dunbabin, 2007; Walter, 2010), suggesting the field is moving beyond some of the technical aspects of wireless communication protocols and hardware with the focus now on data quality, interpretation and analysis.
When considering how existing studies collect environmental data and how they are utilized (e.g., analysis, decision-making and system control), some distinct patterns are apparent. Firstly, most sensor networks used fixed point sensors and data were transferred wirelessly either to a base station, remote server or the cloud (Figures 2A,B). The use of mobile sensors is more common for ecological systems, particularly tracking animal movement (e.g., Davis et al., 2012) and for monitoring air quality (Mead et al., 2013); however, Schneider et al. (2016) outlined a study in which sensors fitted to rafts or kayaks were used to continuously gather water quality data while moving downstream. Wired sensor networks or systems that required direct data download from local storage were associated with either: (1) scientific studies in which networks were maintained to answer a specific research objective or test a new senor type (Barnard et al., 2014; Pohl et al., 2014); or (2) monitoring networks for human infrastructure in urban environments where Ethernet connections were available (Dauwe et al., 2014; Rettig et al., 2014).
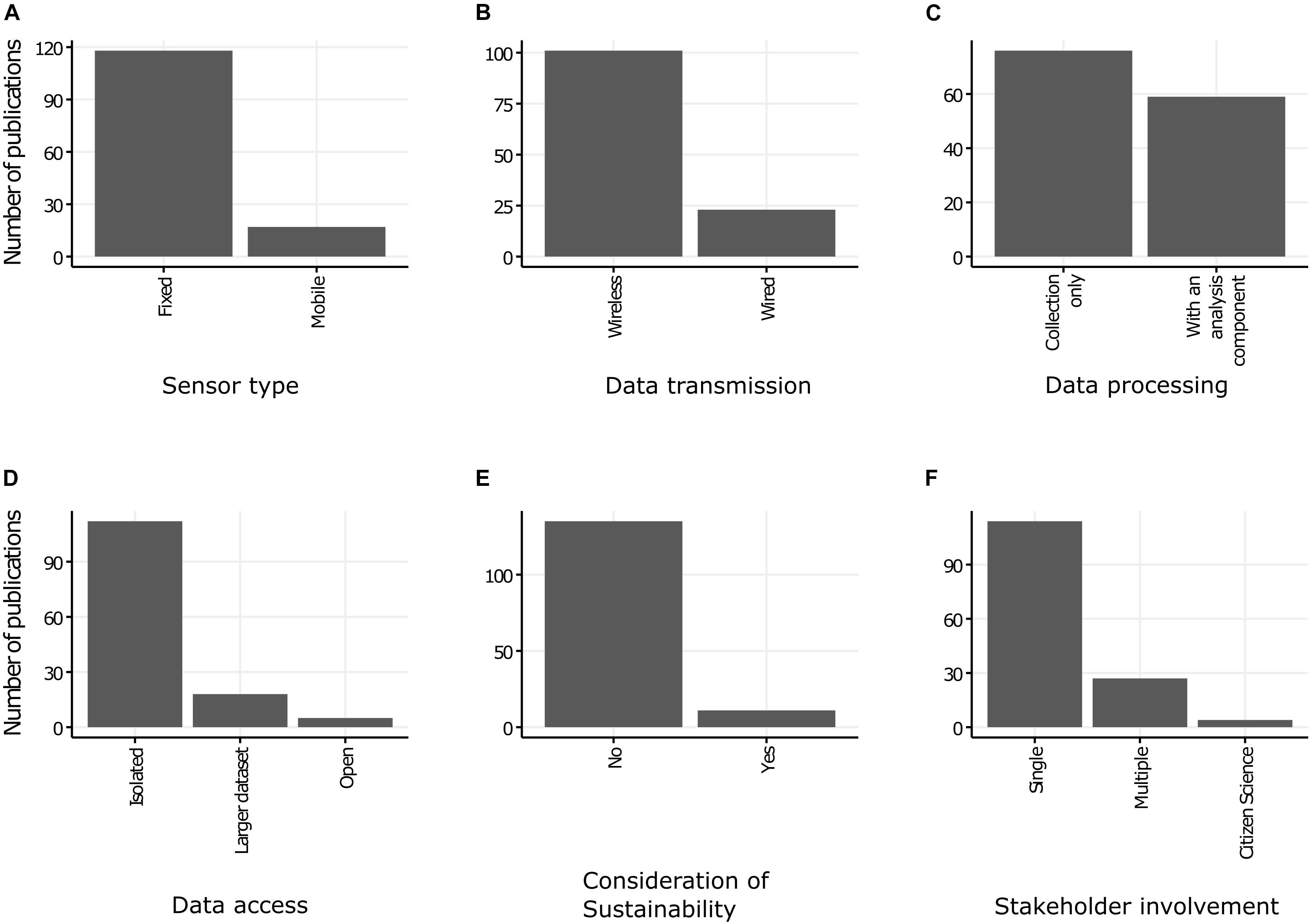
Figure 2. The distributions of publications based on sensor and data collection properties. (A) Publication number split by sensor/node deployment properties. (B) The breakdown between wireless transmission of data to a base station or cloud-based server vs. hard-wired connection including Ethernet and local storage with manual data download. (C) The number of studies which highlighted networks for collection and data storage only, as opposed to studies with a data analysis component after collection, including visualization, analytics and control. (D) Data access, here the category “Isolated” refers to a dataset only available internally or to direct stakeholders, “Open” refers to an open access data storage platform, and “Larger dataset” refers to a study that contributed to a larger dataset. (E) The number of publications that explicitly considered sustainability principles. (F) The degree of stakeholder and end-user engagement in the study.
Secondly, there was a slight bias with regards to how the data were used with more papers (n = 76) reporting just data collection and storage than with a data analysis component (n = 59) (Figure 2C). This result appears to support H2 (i.e., predominate focus is on data collection), however, there appears to be a growing trend toward the development of online analytics and visualization with 23.8% of all pre 2012 studies and 46.6% of those post 2011. Most storage-only-networks were used in scientific studies with analysis conducted offline by researchers. For example, Pohl et al. (2014) used a network of low-cost weather stations to collect information on snow depth at a high spatial-resolution to quantify the influence of landscape factors on snow accumulation. Monitoring networks with online analytics and visualization were more common in recent studies where some degree of human safety or health was related to the sensed parameters. Examples include geo-hazards (Finazzi and Fassò, 2017), flooding (Jones et al., 2015; Acosta-Coll et al., 2018; Bartos et al., 2018) or air quality (Schneider et al., 2017; Kizel et al., 2018). A further type of monitoring network with analytics and visualization was associated with agriculture (Kubicek et al., 2013) and in several studies this was advanced toward automated control of nutrient addition/irrigation to optimize resource use and yield (López et al., 2015; Srbinovska et al., 2015).
Thirdly, the majority of studies (82.9%) involved networks that collected data and were isolated in nature (i.e., not part of a wider dataset or larger network) (Figure 2D). These data were then only available to or used by direct stakeholders, for example technicians/scientists (e.g., Pohl et al., 2014) or farmers involved in crop production (e.g., López et al., 2015). More recent studies have collected data to complement existing monitoring efforts (i.e., or have been operating as a sub network within a larger national network). These were in many cases associated with human health (Rogulski, 2018) or climate impacts (Shusterman et al., 2018; Šećerov et al., 2019) or had direct economic implications, for example through flooding (Horita et al., 2015) or fishing livelihoods (Wada et al., 2007). It should be noted that very few studies embraced the principles of open science and open data more generally (however see Rettig et al., 2014; Jones et al., 2015).
Despite stakeholder engagement, especially citizen science, being one of the most significant “innovative” approaches associated with LCSNs there was a paucity of such studies identified in the literature (Figure 2F). Given the relatively small number of studies with multiple stakeholders (21.2%) there appears to be strong support for H3 (i.e., most studies focus on technically orientated single end-users). However, there are some interesting examples of multiple stakeholder participation (e.g., Ali et al., 2015; Finazzi and Fassò, 2017). The involvement of citizen scientists can improve the functionalities and impacts of low-cost sensor networks by supporting its operation, enhancing adaptation, information provisioning and resilience building (Horita et al., 2015; Paul et al., 2018). In return, some sensor network applications have tailored designs to improve the user experience of citizen scientists (Schneider et al., 2016).
The application of low-cost sensor networks has been highlighted as a key area that can transform environmental governance, yet long-term environmental governance requires sustainable and long-term operations of low-cost sensor networks (Bakker and Ritts, 2018; Paul et al., 2018). Despite this, most studies identified in this review do not explicitly consider sustainability (92.5%; Figure 2E) and thus support of H4 is strong (i.e., sustainable operating mechanisms and physical and socio-economic contexts have been neglected). One possible explanation for this could be that most studies are from developed regions with significant resources and infrastructure (cf. Figure 1B). Sustainability can be achieved through either technical improvements via means such as optimization of energy efficiency (Gleonec et al., 2017; Mazinani and Davarzani, 2017), or innovative soft management/incentive-based approaches (Bakker and Ritts, 2018). Most of the reviewed studies identified with a sustainability element were associated with explicit and direct human benefits, such as monitoring a particular resource (e.g., Wada et al., 2007), protecting property or livelihoods (e.g., Lopes Pereira et al., 2014) or were agricultural in nature and focused on resource use to maximize yields (e.g., Geipel et al., 2015).
Conclusion and Future Opportunities
To summarize, LCSNs are increasing in popularity but there is still a distinct bias toward developed countries, particularly Western Europe and North America, and certain study systems (e.g., atmosphere and hydrosphere). From this systematic literature review, three key challenges and opportunities were identified, which can also guide future technical development of LCSNs. Firstly, data outputs from LCSNs need to be processed and presented to benefit multiple stakeholders including scientists, the general public and policy makers. While there is still a paucity of examples with studies exploring down-stream data activities such as analysis, decision-making and system control examples exist for certain study purposes (e.g., geo-hazards) from which lessons can be learned for other purposes. Secondly, there is a clear need to improve data integration and sharing. This will involve a move away from isolated datasets to closer alignment with existing monitoring systems to create larger, richer datasets and a concerted effort to make data more open. While this has begun the idea needs to be at the core of future networks to improve system understanding and avoid duplication of effort. Thirdly, the design of LCSNs needs to better integrate stakeholder engagement and sustainable operation to enable longer term and greater societal impacts and environmental benefits.
Author Contributions
FM conceived of the presented idea and designed the research framework with support from KK, JC, SK, and DH. FM and KK reviewed the literature and drafted the manuscript. KK led the data analysis and interpreted the results together with FM. SK and DH provided critical feedback and constructive comments. All authors were involved in the discussion of the results.
Funding
This work was supported by United Kingdom Natural Environment Research Council (NERC) - United Kingdom Economic and Social Research Council - United Kingdom Department for International Development, Grant/Award Number: project NE/K010239/1 (Mountain-EVO); NERC and DFID - Science for Humanitarian Emergencies and Resilience (SHEAR) program, Grant/Award Number: project NE/P000452/1 (Landslide EVO). This research has also been funded by the European Union’s Horizon 2020 Research and Innovation Programme under the Marie Skłodowska-Curie Grant Agreement No. 734317 (HiFreq). Funds for open access publication fees were received from the University of Birmingham.
Conflict of Interest Statement
The authors declare that the research was conducted in the absence of any commercial or financial relationships that could be construed as a potential conflict of interest.
Supplementary Material
The Supplementary Material for this article can be found online at: https://www.frontiersin.org/articles/10.3389/feart.2019.00221/full#supplementary-material
Footnotes
References
Acosta-Coll, M., Ballester-Merelo, F., and Martínez-Peiró, M. (2018). Early warning system for detection of urban pluvial flooding hazard levels in an ungauged basin. Nat. Hazards 92, 1237–1265. doi: 10.1007/s11069-018-3249-4
Ali, H., Soe, J. K., and Weller, S. R. (2015). “A real-time ambient air quality monitoring wireless sensor network for schools in smart cities,” in Proceedings of the 2015 IEEE First International Smart Cities Conference (ISC2), Guadalajara. 2, 5–10.
Baden, T., Chagas, A. M., Gage, G., Marzullo, T., Prieto-Godino, L. L., and Euler, T. (2015). Open labware: 3-d printing your own lab equipment. PLoS Biol. 13:e1002175. doi: 10.1371/journal.pbio.1002086
Bakker, K., and Ritts, M. (2018). Smart earth: a meta-review and implications for environmental governance. Glob. Environ. Change 52, 201–211. doi: 10.1016/j.gloenvcha.2018.07.011
Barnard, H. R., Findley, M. C., and Csavina, J. (2014). PARduino: a simple and inexpensive device for logging photosynthetically active radiation. Tree Physiol. 34, 640–645. doi: 10.1093/treephys/tpu044
Bartesaghi Koc, C., Osmond, P., and Peters, A. (2018). Evaluating the cooling effects of green infrastructure: a systematic review of methods, indicators and data sources. Sol. Energy 166, 486–508. doi: 10.1016/j.solener.2018.03.008
Bartos, M., Wong, B., and Kerkez, B. (2018). Open storm: a complete framework for sensing and control of urban watersheds. Environ. Sci. Water Res. Technol. 4, 346–358. doi: 10.1039/c7ew00374a
Benedetti, M., Ioriatti, L., Martinelli, M., and Viani, F. (2010). Wireless sensor network: a pervasive technology for earth observation. IEEE J. Sel. Top. Appl. Earth Obs. Remote Sens. 3, 488–496. doi: 10.1109/JSTARS.2010.2052917
Bengston, K. J., and Dunbabin, M. D. (2007). “Design & performance of a networked Ad-Hoc acoustic communications system using inexpensive commercial CDMA modems,” in Proceedings of the Oceans 2007-Europe, (Aberdeen: IEEE), 6.
Blaen, P. J., Khamis, K., Lloyd, C. E. M., Bradley, C., Hannah, D., and Krause, S. (2016). Real-time monitoring of nutrients and dissolved organic matter in rivers: capturing event dynamics, technological opportunities and future directions. Sci. Total Environ. 569–570, 647–660. doi: 10.1016/j.scitotenv.2016.06.116
Castell, N., Dauge, F. R., Schneider, P., Vogt, M., Lerner, U., Fishbain, B., et al. (2017). Can commercial low-cost sensor platforms contribute to air quality monitoring and exposure estimates? Environ. Int. 99, 293–302. doi: 10.1016/j.envint.2016.12.007
Dauwe, S., Oldoni, D., De Baets, B., Van Renterghem, T., Botteldooren, D., and Dhoedt, B. (2014). Multi-criteria anomaly detection in urban noise sensor networks. Environ. Sci. Process. Impacts 16, 2249–2258. doi: 10.1039/c4em00273c
Davis, M. J., Thokala, S., Xing, X., Thompson Hobbs, N., Walsh, D. P., Han, R. Y., et al. (2012). Developing a data-transfer model for a novel Wildlife-tracking network. Wildl. Soc. Bull. 36, 820–827. doi: 10.1002/wsb.188
Finazzi, F., and Fassò, A. (2017). A statistical approach to crowdsourced smartphone-based earthquake early warning systems. Stoch. Environ. Res. Risk Assess. 31, 1649–1658. doi: 10.1007/s00477-016-1240-8
Fisher, R., Ledwaba, L., Hancke, G., and Kruger, C. (2015). Open hardware: a role to play in wireless sensor networks? Sensors 15, 6818–6844. doi: 10.3390/s150306818
Geipel, J., Jackenkroll, M., Weis, M., and Claupein, W. (2015). A sensor web-enabled infrastructure for precision farming. ISPRS Int. J. Geo Inform. 4, 385–399. doi: 10.3390/ijgi4010385
Gleonec, P. D., Ardouin, J., Gautier, M., and Berder, O. (2017). “Architecture exploration of multi-source energy harvester for IOT nodes,” in Proceedings of the 2016 IEEE Online Conference on Green Communications (OnlineGreenComm), Piscataway, NJ, 27–32. doi: 10.1109/OnlineGreenCom.2016.7805402
Hart, J. K., and Martinez, K. (2006). Environmental sensor networks: a revolution in the earth system science? Earth Sci. Rev. 78, 177–191. doi: 10.1016/j.earscirev.2006.05.001
Hill, N. R., Fatoba, S. T., Oke, J. L., Hirst, J. A., Callaghan, A. O., Lasserson, D. S., et al. (2016). Global prevalence of chronic kidney disease – a systematic review and meta-analysis. PLoS One 11:e0158765. doi: 10.5061/dryad.3s7rd
Horita, F. E. A., Albuquerque, J. P., de Degrossi, L. C., Mendiondo, E. M., and Ueyama, J. (2015). Development of a spatial decision support system for flood risk management in Brazil that combines volunteered geographic information with wireless sensor networks. Comput. Geosci. 80, 84–94. doi: 10.1016/j.cageo.2015.04.001
Jones, A. S., Horsburgh, J. S., Reeder, S. L., Ramírez, M., and Caraballo, J. (2015). A data management and publication workflow for a large-scale, heterogeneous sensor network. Environ. Monit. Assess. 187:348. doi: 10.1007/s10661-015-4594-3
Karpouzoglou, T., Dewulf, A., and Clark, J. (2016). Advancing adaptive governance of social-ecological systems through theoretical multiplicity. Environ. Sci. Policy 57, 1–9. doi: 10.1016/j.envsci.2015.11.011
Katsiri, E., and Makropoulos, C. (2016). An ontology framework for decentralized water management and analytics using wireless sensor networks. Desalin. Water Treat. 57, 26355–26368. doi: 10.1080/19443994.2016.1202144
Kim, Y., Jabro, J. D., and Evans, R. G. (2011). Wireless lysimeters for real-time online soil water monitoring. Irrig. Sci. 29, 423–430. doi: 10.1007/s00271-010-0249-x
Kizel, F., Etzion, Y., Shafran-Nathan, R., Levy, I., Fishbain, B., Bartonova, A., et al. (2018). Node-to-node field calibration of wireless distributed air pollution sensor network. Environ. Pollut. 233, 900–909. doi: 10.1016/j.envpol.2017.09.042
Krause, S., Lewandowski, J., Dahm, C. N., and Tockner, K. (2015). Frontiers in real-time ecohydrology - a paradigm shift in understanding complex environmental systems. Ecohydrology 8, 529–537. doi: 10.1002/eco.1646
Kubicek, P., Kozel, J., Stampach, R., and Lukas, V. (2013). Prototyping the visualization of geographic and sensor data for agriculture. Comput. Electron. Agric. 97, 83–91. doi: 10.1016/j.compag.2013.07.007
Lopes Pereira, R., Trindade, J., Goncalves, F., Suresh, L., Barbosa, D., and Vazão, T. (2014). A wireless sensor network for monitoring volcano-seismic signals. Nat. Hazards Earth Syst. Sci. 14, 3123–3142. doi: 10.5194/nhess-14-3123-2014
López, J. A., Navarro, H., Soto, F., Pavón, N., Suardíaz, J., and Torres, R. (2015). GAIA2: a multifunctional wireless device for enhancing crop management. Agric. Water Manag. 151, 75–86. doi: 10.1016/j.agwat.2014.10.023
López Riquelme, J. A., Soto, F., Suardíaz, J., Sánchez, P., Iborra, A., and Vera, J. A. (2009). Wireless Sensor Networks for precision horticulture in Southern Spain. Comput. Electron. Agric. 68, 25–35. doi: 10.1016/j.compag.2009.04.006
Mao, F., Clark, J., Buytaert, W., Krause, S., and Hannah, D. M. (2018). Water sensor network applications: time to move beyond the technical? Hydrol. Process. 32, 2612–2615. doi: 10.1002/hyp.13179
Mazinani, S. M., and Davarzani, S. (2017). Presenting an optimal algorithm based on firefly algorithm with specific parameters to select the cluster head in wireless sensor networks in order to reduce energy consumption. QUID Investig. Cienc. Tecnol. 1, 1936–1941.
Mead, M. I., Popoola, O. A. M., Stewart, G. B., Landshoff, P., Calleja, M., Hayes, M., et al. (2013). The use of electrochemical sensors for monitoring urban air quality in low-cost, high-density networks. Atmos. Environ. 70, 186–203. doi: 10.1016/j.atmosenv.2012.11.060
Mulrow, C. (1987). The medical review article: state of the science. Ann. Intern. Med. 106, 485–488.
Munir, S., Mayfield, M., Coca, D., Jubb, S. A., and Osammor, O. (2019). Analysing the performance of low-cost air quality sensors, their drivers, relative benefits and calibration in cities—a case study in Sheffield. Environ. Monit. Assess. 191:94. doi: 10.1007/s10661-019-7231-8
Ojha, T., Misra, S., and Raghuwanshi, N. S. (2015). Wireless sensor networks for agriculture: the state-of-the-art in practice and future challenges. Comput. Electron. Agric. 118, 66–84. doi: 10.1016/j.compag.2015.08.011
Paul, J. D., Buytaert, W., Allen, S., Ballesteros-Cánovas, J. A., Bhusal, J., Cieslik, K., et al. (2018). Citizen science for hydrological risk reduction and resilience building. Wiley Interdiscip. Rev. Water 5:e1262. doi: 10.1002/wat2.1262
Pearce, J. (2013). Open-Source Lab: How to Build Your Own Hardware and Reduce Research Costs. Amsterdam: Elsevier.
Pickering, C., and Byrne, J. (2014). The benefits of publishing systematic quantitative literature reviews for PhD candidates and other early-career researchers. High. Educ. Res. Dev. 33, 534–548. doi: 10.1080/07294360.2013.841651
Pohl, S., Garvelmann, J., Wawerla, J., and Weiler, M. (2014). Potential of a low-cost sensor network to understand the spatial and temporal dynamics of a mountain snow cover. Water Resour. Res. 50, 2533–2550. doi: 10.1002/2013WR014594
Prasad, P. (2015). Recent trend in wireless sensor network and its applications: a survey. Sens. Rev. 35, 229–236. doi: 10.1108/SR-08-2014-683
Pumo, D., Francipane, A., Lo Conti, F., Arnone, E., Bitonto, P., Viola, F., et al. (2016). The SESAMO early warning system for rainfall-triggered landslides. J. Hydroinform. 18, 256–276. doi: 10.2166/hydro.2015.060
Rai, A. C., Kumar, P., Pilla, F., Skouloudis, A. N., Di Sabatino, S., Ratti, C., et al. (2017). End-user perspective of low-cost sensors for outdoor air pollution monitoring. Sci. Total Environ. 607–608, 691–705. doi: 10.1016/j.scitotenv.2017.06.266
Rettig, A. J., Khanna, S., Heintzelman, D., and Beck, R. A. (2014). An open source software approach to geospatial sensor network standardization for urban runoff. Comput. Environ. Urban Syst. 48, 28–34. doi: 10.1016/j.compenvurbsys.2014.05.003
Rogulski, M. (2018). Using low-cost PM monitors to detect local changes of air quality. Polish J. Environ. Stud. 27, 1699–1706. doi: 10.15244/pjoes/77075
Rossiter, D. G. (2018). Past, present & future of information technology in pedometrics. Geoderma 324, 131–137. doi: 10.1016/j.geoderma.2018.03.009
Schneider, J., Schultz, L. E., Mancha, S., Hicks, E., and Smith, R. N. (2016). “Development of a portable water quality sensor for river monitoring from small rafts,” in Proceedings of the OCEANS 2016 MTS/IEEE Monterey, (Monterey, CA: IEEE), 1–10. doi: 10.1109/OCEANS.2016.7761392
Schneider, P., Castell, N., Vogt, M., Dauge, F. R., Lahoz, W. A., and Bartonova, A. (2017). Mapping urban air quality in near real-time using observations from low-cost sensors and model information. Environ. Int. 106, 234–247. doi: 10.1016/j.envint.2017.05.005
Šećerov, I. B., Savić, S. M., Milošević, D. D., Arsenović, D. M., Dolinaj, D. M., and Popov, S. B. (2019). Progressing urban climate research using a high-density monitoring network system. Environ. Monit. Assess. 191:89. doi: 10.1007/s10661-019-7210-0
Shaw, R., Lark, R. M., Williams, A. P., Chadwick, D. R., and Jones, D. L. (2016). Characterising the within-field scale spatial variation of nitrogen in a grassland soil to inform the efficient design of in-situ nitrogen sensor networks for precision agriculture. Agric. Ecosyst. Environ. 230, 294–306. doi: 10.1016/j.agee.2016.06.004
Shusterman, A. A., Kim, J., Lieschke, K. J., Newman, C., Wooldridge, P. J., and Cohen, R. C. (2018). Observing local CO2 sources using low-cost, near-surface urban monitors. Atmos. Chem. Phys. 18, 13773–13785. doi: 10.5194/acp-18-13773-2018
Snyder, E. G., Watkins, T. H., Solomon, P. A., Thoma, E. D., Williams, R. W., Hagler, G. S. W., et al. (2013). The changing paradigm of air pollution monitoring. Environ. Sci. Technol. 47, 11369–11377. doi: 10.1021/es4022602
Srbinovska, M., Gavrovski, C., Dimcev, V., Krkoleva, A., and Borozan, V. (2015). Environmental parameters monitoring in precision agriculture using wireless sensor networks. J. Clean. Prod. 88, 297–307. doi: 10.1016/j.jclepro.2014.04.036
Strigaro, D., Cannata, M., and Antonovic, M. (2019). Boosting a weather monitoring system in low income economies using open and non-conventional systems: data quality analysis. Sensors 19:1185. doi: 10.3390/s19051185
van de Giesen, N., Hut, R., and Selker, J. (2014). The trans-african hydro-meteorological observatory (TAHMO). Wiley Interdiscip. Rev. Water 1, 341–348. doi: 10.1002/wat2.1034
van Zoest, V. M., Stein, A., and Hoek, G. (2018). Outlier detection in urban air quality sensor networks. Water. Air. Soil Pollut. 229:111. doi: 10.1007/s11270-018-3756-7
Wada, M., Hatanaka, K., and Toda, M. (2007). “Practical use of personal buoy system for fishery using sensor network technologies,” in Proceedings of the Ocean 2007-Europe, (Vancouver, BC: IEEE), 1–6.
Walter, K. (2010). Development of an early warning information infrastructure using spatial web services technology. Int. J. Digit. Earth 3, 384–394. doi: 10.1080/17538947.2010.486871
Wickham, H., and Grolemund, G. (2016). R for Data Science: Import, Tidy, Transform, Visualize, and Model Data, 1st Edn. Newton, MA: O’Reilly Media.
Keywords: sensor network, low-cost, environment, monitoring, internet of thing, information and communication technology
Citation: Mao F, Khamis K, Krause S, Clark J and Hannah DM (2019) Low-Cost Environmental Sensor Networks: Recent Advances and Future Directions. Front. Earth Sci. 7:221. doi: 10.3389/feart.2019.00221
Received: 19 March 2019; Accepted: 13 August 2019;
Published: 11 September 2019.
Edited by:
Rolf Hut, Delft University of Technology, NetherlandsReviewed by:
Lutz Breuer, University of Giessen, GermanyPeter M. Marchetto, University of Minnesota Twin Cities, United States
Chet Udell, Oregon State University, United States
Copyright © 2019 Mao, Khamis, Krause, Clark and Hannah. This is an open-access article distributed under the terms of the Creative Commons Attribution License (CC BY). The use, distribution or reproduction in other forums is permitted, provided the original author(s) and the copyright owner(s) are credited and that the original publication in this journal is cited, in accordance with accepted academic practice. No use, distribution or reproduction is permitted which does not comply with these terms.
*Correspondence: Feng Mao, Zi5tYW9AYmhhbS5hYy51aw==