- 1International Centre for Integrated Mountain Development, Kathmandu, Nepal
- 2Scott Polar Research Institute, University of Cambridge, Cambridge, United Kingdom
- 3British Antarctic Survey, Natural Environment Research Council, Cambridge, United Kingdom
- 4Norwegian Water Resources and Energy Directorate, Oslo, Norway
- 5Department of Physical Geography, Utrecht University, Utrecht, Netherlands
Seasonal snow is an important component of the Himalayan hydrological system, but a lack of observations at high altitude hampers understanding and forecasting of water availability in this region. Here, we use a passive gamma ray sensor that measures snow water equivalent (SWE) and complementary meteorological instruments installed at 4962 m a.s.l. in the Nepal Himalayas to quantify the evolution of SWE and snow depth over a 2-year period. We assess the accuracy, spatial representativeness and the applicability of the SWE and snow depth measurements using time-lapse camera imagery and field observations. The instrument setup performs well for snowpacks >50 mm SWE, but caution must be applied when interpreting measurements from discontinuous, patchy snow cover or those that contain lenses of refrozen meltwater. Over their typical ∼6-month lifetime, snowpacks in this setting can attain up to 200 mm SWE, of which 10–15% consists of mixed precipitation and rain-on-snow events. Precipitation gauges significantly underrepresent the solid fraction of precipitation received at this elevation by almost 40% compared to the gamma ray sensor. The application of sub-daily time-lapse camera imagery can help to correctly interpret and increase the reliability and representativeness of snowfall measurements. Our monitoring approach provides high quality, continuous, near-real time information that is essential to develop snow models in this data scarce region. We recommend that a similar instrument setup be extended into remote Himalayan environments to facilitate widespread snowpack monitoring and further our understanding of the high-altitude water cycle.
Introduction
High Mountain Asia’s cryosphere delivers water resources to over one billion people (Barnett et al., 2005; Bookhagen and Burbank, 2010; Immerzeel et al., 2010; Bolch et al., 2012; Gardner et al., 2013; Smith T. et al., 2017). In many Himalayan river catchments, the contribution of seasonal snowmelt to mean annual runoff equals or exceeds that of glacial melt (Prasch et al., 2013; Rohrer et al., 2013; Lutz et al., 2014). Many communities, particularly those at high elevations, are dependent on seasonal snowmelt for water, hydropower generation and irrigation (Smith T. et al., 2017), whilst changes in snow conditions and extent have major ramifications for ecosystem function, avalanche threat, and climate feedbacks such as land surface albedo (Lehning et al., 1999; Sturm et al., 2010; Rohrer et al., 2013). With ongoing climate change predicted to affect the high-altitude Himalayan cryosphere (e.g., Singh and Kumar, 1997; Singh and Bengtsson, 2005; Stewart, 2009; Kraaijenbrink et al., 2017; Smith and Bookhagen, 2018), it is critical that seasonal Himalayan snowpacks are comprehensively monitored to facilitate the development of hydrological models that can be used to forecast water availability in this region (López-Moreno et al., 2013; Sexstone and Fassnacht, 2014; Saloranta et al., 2019).
The quantity of water contained within a snowpack, termed snow water equivalent (SWE), is one of the most important variables to consider when examining seasonal runoff production (Jonas et al., 2009). SWE is difficult to accurately measure and model over broad spatial areas, and large discrepancies exist between estimates derived from different measurement techniques such as reanalysis products, precipitation gauges, and satellite observations (Takala et al., 2011; Ménégoz et al., 2013). The application of Regional Circulation Models has shown potential to resolve issues associated with coarser spatial resolution Global Circulation Models in Himalayan regions (Polanski et al., 2010; Sabin et al., 2013); however, these simulations still have large biases when compared with observations due to difficulties in correctly partitioning solid and liquid phases of precipitation (Ménégoz et al., 2013). Many of the issues associated with SWE measurement and modeling are a result of the scarcity of reliable solid precipitation observations at high altitude with which to calibrate and develop SWE products (Ma et al., 2009; Ménégoz et al., 2013; Rohrer et al., 2013).
Obtaining accurate in situ SWE observations is a difficult and time-consuming process (Sturm et al., 2010). Automated methods of SWE measurement can increase the ease with which seasonal SWE patterns can be monitored and, unlike manual sampling techniques, do not invasively disturb a snowpack’s internal structure (Kinar and Pomeroy, 2015). Many automatic ground-based methods of measuring SWE exist, including weighing techniques (e.g., Serreze et al., 1999; Johnson et al., 2015), radiation-based methods (e.g., Kodama et al., 1979; Choquette et al., 2008; Martin et al., 2008; Rasmussen et al., 2012), technologies that measure the reflectance of acoustic impulses (Kinar and Pomeroy, 2007) and methods that utilize the Global Navigation Satellite System (Henkel et al., 2018; Appel et al., 2019). However, there is no ideal method of automatically measuring SWE (Egli et al., 2009), and installation and maintenance of gauging stations at elevations where the majority of Himalayan snow cover resides and melts (4000–5000 m a.s.l.) (Immerzeel et al., 2009; Tiwari et al., 2016; Gurung et al., 2017) is logistically difficult, expensive, and often dangerous in poor weather conditions. Even where present, many of the meteorological stations in the Himalayas have large data gaps (e.g., Shea et al., 2015), and rain gauges are generally not adapted to measure solid precipitation, resulting in large instrumental uncertainties which negatively impact efforts to calibrate SWE products over larger spatial scales (Lang and Barros, 2004; Ma et al., 2009; Ménégoz et al., 2013).
SWE can be estimated over greater spatial scales from airborne and satellite-based passive microwave sensors (e.g., Carroll et al., 1999; Smith and Bookhagen, 2018). These, along with other remote sensing methods, may provide useful information for downstream hydrological applications (Immerzeel et al., 2009; Bookhagen and Burbank, 2010). However, estimation of SWE from remote sensing data is associated with large uncertainties due to the topographic heterogeneity of mountainous terrain, which may result in lower than true mean SWE values (Takala et al., 2011; Tiwari et al., 2016). Furthermore, the applicability of these techniques to Himalayan settings is limited due to the substantial influence of melting and wetting in these snowpacks – both of which increase the uncertainty of microwave-based methods of SWE retrieval (Dong et al., 2005; Smith and Bookhagen, 2016). The spatial resolution of many remote sensing SWE products is also coarse when compared to the typical size of most Himalayan catchments (Stigter et al., 2017). Consequently, remotely sensed estimates of solid precipitation in the Himalayas differ widely from one product to another (Palazzi et al., 2013), and there is currently very little reliable information about changing patterns of Himalayan SWE (Lutz et al., 2015).
An alternative method of monitoring the water stored in seasonal snow is to calculate SWE as the product of snowpack depth and bulk density. The average (bulk) density of a snowpack depends on the initial density and compaction history of each constituent snow layer (Sturm et al., 2010; Schleef et al., 2014). Snowpack bulk density is influenced by many meteorological drivers and processes, including the air temperature and humidity at the time of snow crystal formation, the wind speed during fresh snow deposition, and the metamorphism, melting, refreezing and windblown packing of snow once settled (Hedstrom and Pomeroy, 1998; Meløysund et al., 2007; Bormann et al., 2013; Essery et al., 2013). As many of these drivers can vary over relatively short (sub-daily to hourly) timescales, snowpack density can exhibit substantial spatial and temporal variation, and continuous high-resolution observations of snowpack processes are necessary to understand seasonal snowpack densification (Jepsen et al., 2012; Sexstone and Fassnacht, 2014).
Many physically- and empirically-based models exist to predict snowpack properties (e.g., Brun et al., 1989, 1992; Carroll et al., 1999; Meløysund et al., 2007; Best et al., 2011; Saloranta, 2012; Essery et al., 2013; McCreight and Small, 2014). However, the physical mechanics underpinning these models are based on research conducted in high- to mid-latitude locations such as the large northern hemisphere snowfields of North America and Europe (e.g., Sturm and Holmgren, 1998; Judson and Doesken, 2000; Meløysund et al., 2007; Sturm et al., 2010; Zhong et al., 2014). Continuous observations of snow properties in high-altitude, low-latitude environments such as the Himalayas are almost non-existent and, consequently, calibrating the current generation of snow models to run in Himalayan settings is challenging.
The development, calibration, and improvement of more sophisticated SWE products in High Mountain Asia calls for a greater number of accurate, high-altitude, precipitation observations with rigorously constrained uncertainties. In this study, we present an automatic monitoring system installed at nearly 5000 m a.s.l. in the Nepal Himalayas that is capable of providing high-resolution snowpack observations in near-real time. We assess the suitability of the monitoring system to provide reliable estimates of SWE, snow depth, and solid precipitation that could be used to calibrate and develop SWE and snow models in this data-scarce region. We make recommendations about how this setup could be extended into similarly remote environments to attain greater understanding of the high-altitude Himalayan water cycle in the future.
Data and Methods
Instrument Setup
The Automatic Weather Station
An automatic weather station was installed on the southern side of the Ganja La Pass (4962 m a.s.l.), near the boundary of the Langtang Valley catchment in the Nepal Himalayas (Figure 1). The automatic weather station was installed as part of the “Snow accumulation and melt processes in a Himalayan catchment” (SnowAMP) project described in Saloranta et al. (2016). The automatic weather station consists of sensors measuring SWE, snow depth, precipitation, ambient air temperature, surface temperature, relative humidity, average and maximum hourly wind speed, wind direction, barometric pressure, and the incoming and outgoing components of shortwave and longwave solar radiation (Table 1). The sensors are installed on a 5-m high lightweight (10 kg) aluminum mast mounted on a boulder and secured with guy wires (Figure 1c). Two precipitation gauges are installed at a distance of ∼3 m from the main instrument mast (Figure 1d). A Uovision UV565 time-lapse camera installed on the weather station captures images of the surrounding site six times per day.
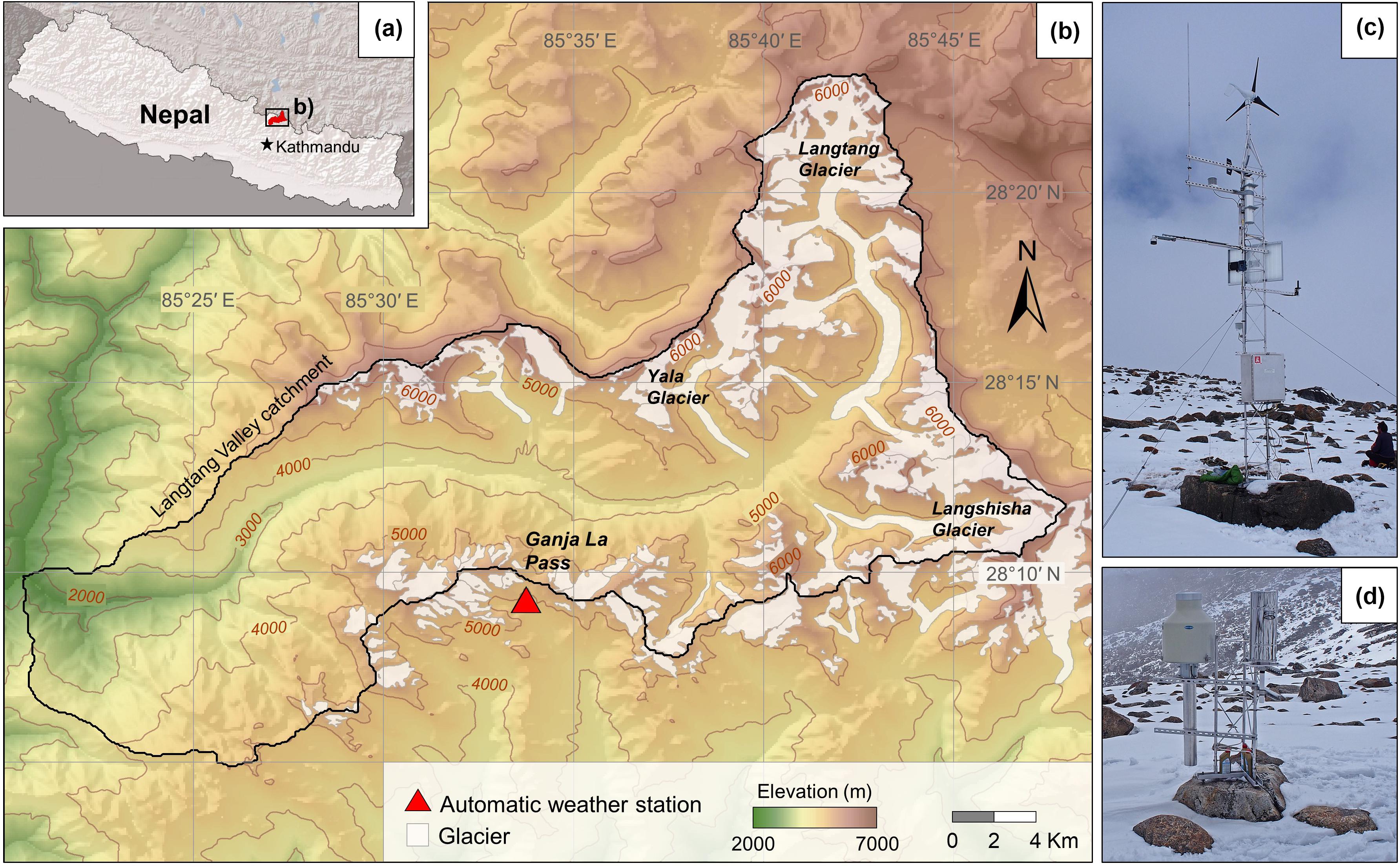
Figure 1. Study site and instruments. (a) Location of the Langtang catchment, adjacent to the study site, Nepal. (b) The automatic weather station is located on the southern side of the Ganja La Pass at 4962 m.a.s.l. Major glaciers and ice masses in Nepal are displayed (Bajracharya et al., 2014). (c) The automatic weather station and (d) the Total Precipitation Gauge at the Ganja La Pass in May 2017.
The automatic weather station transmits its data in real time using the Iridium satellite network using the Short Burst Data transmission protocol. Data is sent hourly to the SutronWIN acquisition and presentation service,1 and is immediately accessible. A complete data series for all instruments is available between May 2016 and June 2018, except the precipitation weighing gauge which was dysfunctional from August 2016 to September 2016 and from August 2017 to January 2018. These periods of dysfunction were due to the gauge overflowing with water. The gauge was emptied in October 2016, but severe weather conditions prevented the automatic weather station from being accessed in October 2017. After December 2017, however, the water evaporated from the instrument and measurements could be recorded again. We exclude periods of gauge overflow from our analysis.
Snow Depth Measurement
Snow depth is measured with an SR50AT that uses the return speed of an ultrasonic pulse to determine the distance to the surface of the snowpack. The instrument has a typical accuracy of ±0.01 m. The measurement is adjusted for the varying speed of sound in different air temperatures using an internal temperature sensor with an accuracy of less than ±0.75°C (Campbell, 2016). Quality values output by the sensor were used to remove erroneous readings, and time-lapse camera images were used to filter out “false” snow events produced by the noise of the sensor.
To assess the optimal smoothing interval to remove noise from the snow depth sensor in locations where time-lapse camera imagery is not available, we performed sensitivity analysis that quantifies the effect of time series smoothing on snow event detection. To achieve this, we first removed any snow depths associated with a poor data quality value, before applying a shape-preserving piecewise cubic Hermite spline to interpolate missing data gaps within the raw snow depth timeseries. This interpolation method fills in data gaps without modifying the amplitude or shape of the timeseries. We then produced multiple smoothed snow depth timeseries using moving average filters of between 2 and 12 h. Increases in snow depth, greater than the 0.01 m h-1 accuracy of the SR50AT, which occur during the intervals confirmed by the time-lapse images are classified as “true” snow events. Conversely, increases in snow depth greater than 0.01 m h-1 that are not verified by the time-lapse camera images are assumed to be “false” snow events produced by the noise of the sensor. This method permits the effect of smoothing on snow event detection to be determined.
Snow Water Equivalent Measurement
The automatic weather station is equipped with a Campbell Scientific CS725 sensor which calculates SWE by passively measuring the attenuation of gamma rays emitted from naturally occurring isotopes of Potassium (40K) and Thallium (208Tl) present in the substrate beneath the sensor (Campbell, 2015; Stranden et al., 2015). The site’s 40K and 208Tl radiation levels were measured in September 2015 and were found to be well suited for making SWE measurements. Both isotopes provide near-identical estimates of SWE (Figure 2) except for the largest SWE values [>160 mm water equivalent (w.e.)] where 40K yields higher values. The isotope associated with the higher count is generally the most reliable (Smith C.D. et al., 2017) and, consequently, this study focuses on 40K. The CS725 calculates SWE by integrating gamma-ray emissions over a 24-h period before outputting an estimate of snowpack SWE at a 6-h time resolution. The instrument can measure the SWE of snowpacks up to ∼600 mm w.e. and has a measurement accuracy of ±15 mm from 0 to 300 mm w.e. and ±15% from 300 to 600 mm w.e. (Campbell, 2015).
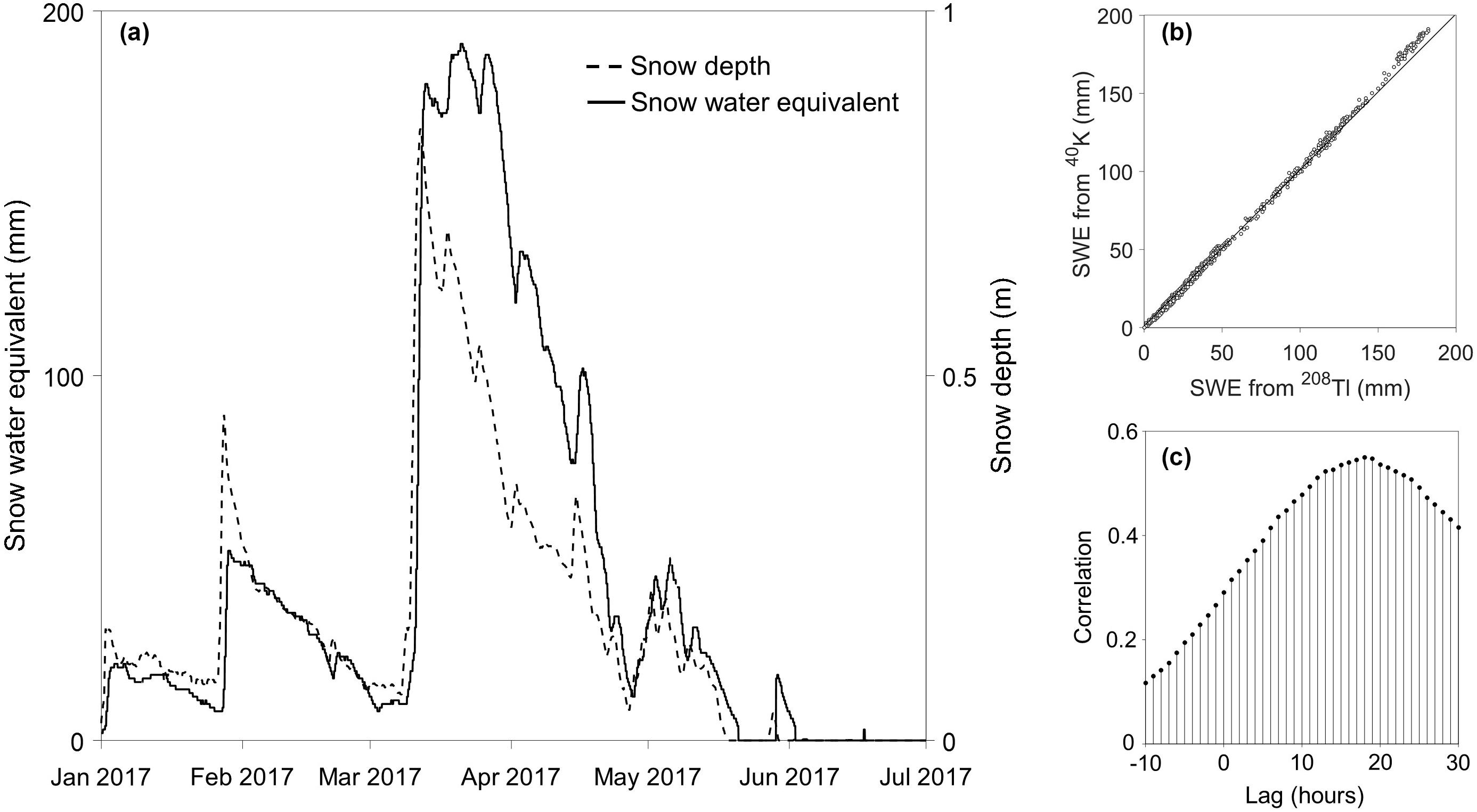
Figure 2. Time delay and isotope comparison for the CS725. (a) Example of the time delay between SWE measurements and snow depth measurements between January 2017 and July 2017. (b) SWE measurements derived from Potassium (40K) versus Thallium (208Tl) isotopes measured by the CS725. (c) Cross correlation plot between snow depth and SWE demonstrating that SWE most frequently lags behind snow depth by 18 h.
The CS725 has an effective field of view of ∼120°, allowing it to monitor the SWE of a ∼150 m2 area of snow surrounding the automatic weather station when mounted 4 m above the ground. The SWE measurement is most heavily weighted toward the snow directly beneath the sensor as gamma photon intensity is attenuated by greater travel distance through the snowpack with increasing radial distance from the automatic weather station. As the topography surrounding the sensor is relatively uniform, the instrument was installed without a collimator which reduces the surface area from which the gamma rays are sourced. Consequently, approximately 44% of incoming radiation counts are sourced from outside the sensor’s effective field of view, increasing the surface area of snow over which SWE values are derived (Campbell, 2015). Experiments in other similarly uniform and unforested sites suggest that the absence of a collimator should result in no significant difference in the measured SWE value compared to an instrument installed with a collimator in this setting (Wright, 2011).
Snowpack Density Data
On the 30th April, 2018, twelve snow pits were dug in a radial pattern around the automatic weather station, covering an area of ∼200 m2. The snow pits were arranged to systematically cover approximately the same area measured by the footprint of the CS725. All pits reached the base of the ∼0.4 m thick snowpack. A snow corer was used to measure the bulk density of the upper (0–0.2 m deep) and lower (0.2–0.4 m deep) portions of the snowpack by weighing a known volume of snow to within ±0.01 kg. The depth of the snowpack and the thickness of a basal ice layer present beneath the snowpack were measured to within ±0.005 m. The field measurements provide a snapshot of the spatial distribution of snowpack SWE, depth, bulk density, and basal ice thickness within the area measured by the weather station instruments.
Comparing CS725 SWE and Snow Depth Measurements
The suitability of the automatic weather station setup to remotely monitor Himalayan snowpack dynamics is assessed in terms of its temporal accuracy and its spatial representativeness. First, we assess the ability of the CS725 to provide timely estimates of changing snowpack SWE. Due to the 24-h moving average window employed by the CS725, it is possible that the changes in SWE detected by the sensor are delayed relative to the actual timing of fresh snow events. We use cross-correlation analysis to examine the presence of any lead or lag in the SWE estimates compared to changes in the depth of the snowpack. Secondly, we compare SWE and snow depth measurements to the spatial distribution of these variables measured in the field. Thirdly, to quantify spatial sensitivity of the sensors to changing snowpack thickness, we use time-lapse imagery to classify snow cover as either uniform or discontinuous and compare these classes to the changing SWE and snow depth values measured by the automatic weather station. Snow cover is defined as uniform when no gaps are present in the snow layer, and discontinuous when snow cover is present but patchy, with some bare ground visible. Finally, we combine the measured snowpack SWE with snow depth estimates to calculate snowpack bulk density and compare this with the snow pit measurements.
Calculation of SWE From Solid Precipitation Measurements
To examine whether precipitation gauges, which are more extensively deployed in the Himalayas than dedicated SWE sensors (Andermann et al., 2011), could be reliably used to monitor the SWE of incoming snowfall events, we compare between the CS725 and the Sutron Total Precipitation Gauge installed on the automatic weather station. The Total Precipitation Gauge is an unheated weighing bucket gauge designed to measure both solid and liquid precipitation. The gauge accumulates and weighs precipitation in a large bucket which is then differenced to derive the quantity of hourly precipitation. The instrument requires regular maintenance to empty the gauge and avoid the risk of overflowing, otherwise no additional measurements can be recorded. The precipitation gauge has a 200 mm diameter funneled orifice which is designed to prevent blockages and evaporation (Sutron, 2015). Antifreeze is added to the bucket to prevent the instrument from filling with low-density snow and to avoid damage to the bucket in sub-zero temperatures. The precipitation gauge is not windshielded.
Precipitation gauge SWE values are calculated by summing hourly precipitation within a 6-h interval in order to permit comparison to the CS725. We calculate and compare the cumulative SWE of incoming precipitation measured by each instrument for periods when a substantial snowpack (>15 mm w.e. and persisting for more than one month) was present. SWE increases recorded by the CS725 as a result of windblown snow redistribution are removed by ignoring any 6-h period of SWE without any observed precipitation in the precipitation gauge. We examine the correspondence between the CS725 and the snow depth sensor by comparing the total cumulative SWE measured by the CS725 to periods when the CS725 measurements increase simultaneously with snow depth.
There is no conclusive way to determine the phase of precipitation collected by the Total Precipitation Gauge, or the contribution to snowpack SWE made by each phase of precipitation. We therefore investigate four methods of identifying and accounting for precipitation phase using the Total Precipitation Gauge. First, we assume that solid precipitation falls when air temperatures are below –2°C, a widely employed ambient air temperature threshold for snow (e.g., Ye et al., 2004; Kochendorfer et al., 2017). We compare this to precipitation which coincides with an increase in snow depth. Third, we calculate the sum of precipitation assuming a linear transition between snowfall and rainfall that occurs between air temperatures of –2 and 2°C (e.g., Pipes and Quick, 1977; Harder and Pomeroy, 2013). Finally, we calculate the sum of any precipitation that occurs when snow cover is present in the time-lapse imagery.
Undercatch Correction of Solid Precipitation
Attempts to obtain accurate measurements with precipitation gauges are obstructed by the deflection of falling hydrometeors away from the inlet of the gauge, resulting in underestimation of the measured quantity of precipitation compared to its true value (Sevruk et al., 1991; Rasmussen et al., 2012). This precipitation “undercatch” is considered to be the most significant systematic error associated with gauge-based measurements of precipitation (Mekonnen et al., 2015). The magnitude of precipitation undercatch is dependent on the wind speed, the presence or absence of dedicated windshields, precipitation gauge design, and the size, phase and velocity of the falling hydrometeors (Sieck et al., 2007; Wolff et al., 2013; Colli et al., 2015). Measurement losses due to precipitation undercatch can amount up to 10% for rainfall and can exceed 50% for falling snow (Ye et al., 2004;Wolff et al., 2015).
The purpose of this study is not to conduct a carefully controlled undercatch quantification test (e.g., MacDonald and Pomeroy, 2007; Wolff et al., 2015) which would be needed to derive a site-specific undercatch correction function for the precipitation measurements. Instead, we estimate the undercatch of the precipitation gauge by comparing the accumulated precipitation to the cumulative SWE observed by the CS725. The percentage of precipitation measured by the Total Precipitation Gauge compared to the CS725 is termed “catch efficiency.” We compare our catch efficiency results to the theoretical catch efficiency ratio calculated from Kochendorfer et al. (2017), derived from the results of the World Meteorological Organization Solid Precipitation Intercomparison Experiment (WMO-SPICE). This correction function was chosen since it is currently the most comprehensive evaluation of precipitation undercatch due to being calculated from a large precipitation dataset gathered from multiple climatic regions including lowland and mountainous areas of Europe and North America. The correction function also incorporates both wind and air temperature parameters, and was derived using precipitation gauges similar to the Total Precipitation Gauge deployed at the Ganja La Pass as a reference, among others. The function calculates the catch efficiency ratio, CE, of the precipitation gauge using wind speed, Uh (m s-1), average air temperature, Tair (°C), and three empirically derived constants (a, b, and c), which vary according to the presence or absence of a windshield (see Kochendorfer et al., 2017):
Average and maximum wind speeds were downscaled from the height of the anemometer to the lower height of the precipitation gauge orifice using a logarithmic wind profile that assumes neutral atmospheric stability (Yang et al., 1998), accounting for relative changes in instrument height due to changing snow depth.
Results
Seasonal Meteorology and Snow Water Equivalent Patterns
Two years of hourly meteorological data from the Ganja La automatic weather station, recorded and transmitted in near-real time, are displayed in Figure 3. For comparison, Supplementary Figure 1 displays the daily values of the same data. Ganja La is characterized by cyclic, seasonally varying patterns of precipitation, relative humidity, wind speed, wind direction, surface temperature, and air temperature. The annual sum of precipitation exceeds 2000 mm, the majority of which falls during the monsoon season between July and October. Both air temperature and humidity exhibit cyclic seasonal variation, progressing from colder, but highly variable (–20 to ∼5°C), air temperatures in the winter (January–March), pre- (March–July) and post-monsoon (October–January) periods to warmer (≥5°C), less variable, air temperatures during the monsoon. Similarly, relative humidity progresses steadily from ∼5 to >50% in the winter months to ∼90–100% during the monsoon season, including highly variable relative humidities between 10 and 90% in the pre- and post-monsoon seasons. Surface temperatures exhibit large diurnal fluctuations of up to 30°C day-1 when the surface is not snow covered. Presence of snow cover is associated with reduced diurnal surface temperature variation, with temperatures typically between -5 and 0°C. Strong diurnal variations in incoming and outgoing shortwave and longwave radiation are also present. Net shortwave and longwave radiation fluxes are relatively consistent during the monsoon season. Measured shortwave and longwave radiation fluxes increase and decrease, respectively, once a snowpack forms in the post-monsoon season. The prevailing wind speed and direction is seasonally variable with gentle (<2 m s-1) southerly winds dominating during the monsoon season. Winds become stronger (>3 m s-1) and switch to a northerly prevailing direction prior to and following the monsoon season.
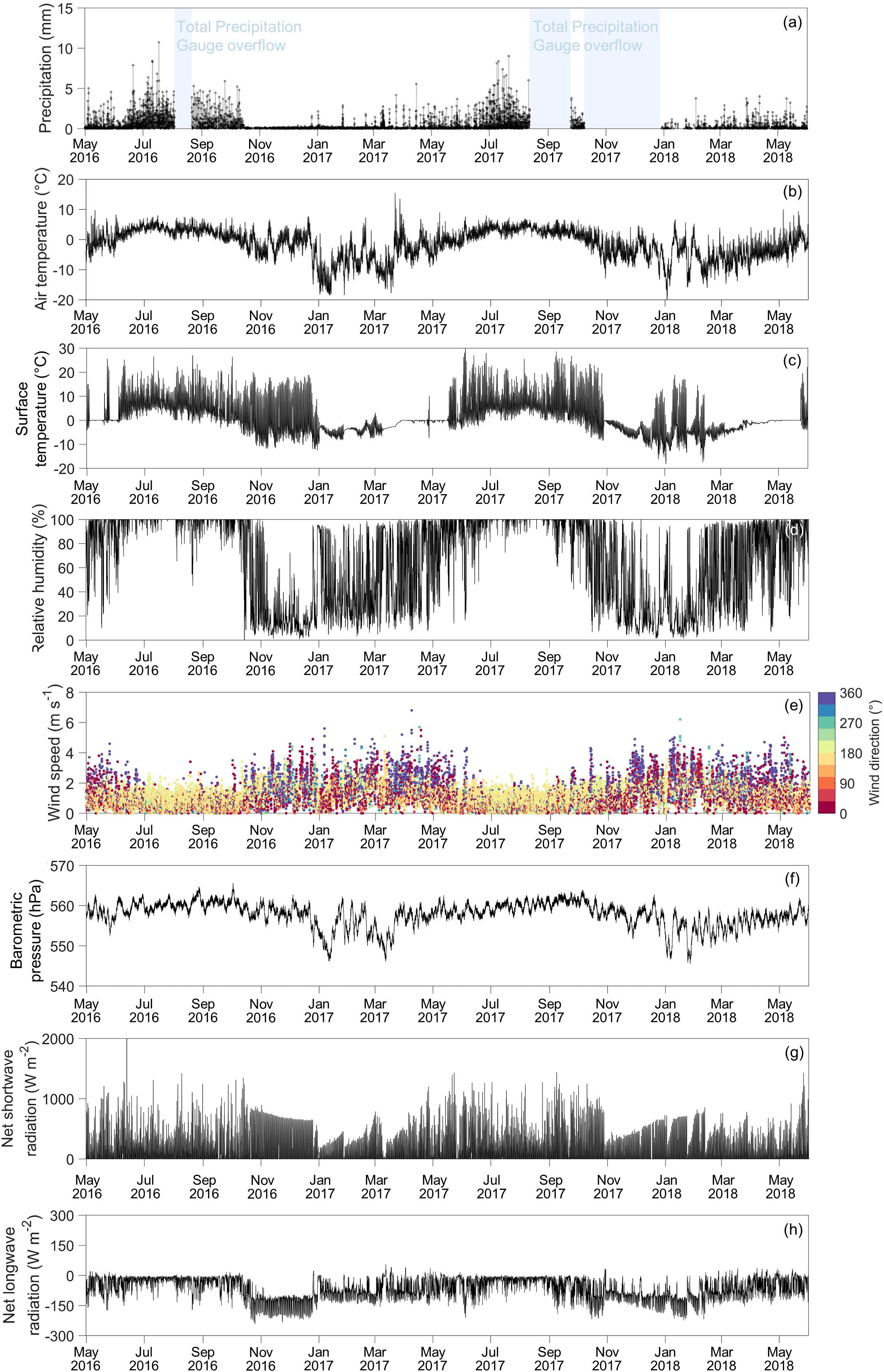
Figure 3. Hourly meteorological data measured by the automatic weather station between May 2016 and June 2018. (a) Precipitation from the Total Precipitation Gauge, (b) air temperature, (c) surface temperature, (d) relative humidity, (e) wind speed and direction, (f) barometric pressure, (g) net shortwave radiation and (h) net longwave radiation.
Three snowpacks with a SWE exceeding 15 mm w.e. and persisting for longer than one month were present between May 2016 and June 2018. Cross correlation analysis demonstrates that the response of the CS725 to rapid changes in SWE generally lags behind instruments that provide immediate “snapshot” responses to changes in the snowpack, such as the SR50AT snow depth sensor (Figure 2c). The range of most common lags for the SWE measurement is between 15 and 23 h for both 40K and 208Tl, and the mean time lag for the entire SWE timeseries is 18 h. An 18-h time lag is therefore used to correct the observed SWE timeseries.
The seasonal evolution of SWE for the three snowpacks present between May 2016 and June 2018 is displayed in Figure 4a. The 6-hourly sum and phase of the precipitation recorded in the Total Precipitation Gauge is also displayed, split into solid, liquid, and mixed precipitation phases using ambient air temperature thresholds of < -2°C for snow, >2°C for rain and -2 to 2°C for mixed precipitation. Although the majority of the precipitation that falls at the beginning of the accumulation period (September–February) is snow, a snowpack often does not persist until the advent of more frequent precipitation events from March through to May. Once established, snowpacks at Ganja La contain up to 200 mm w.e. Single, sustained snowfall events contribute to large proportions of the total SWE, in some instances increasing snowpack SWE by 100–150 mm w.e. over the course of several days (e.g., March 2017 in Figure 4). Established snowpacks have a longevity of 5–6 months before SWE fully disappears. The phase of incoming precipitation becomes increasingly mixed during the last 2 months of the snowpack’s lifespan.
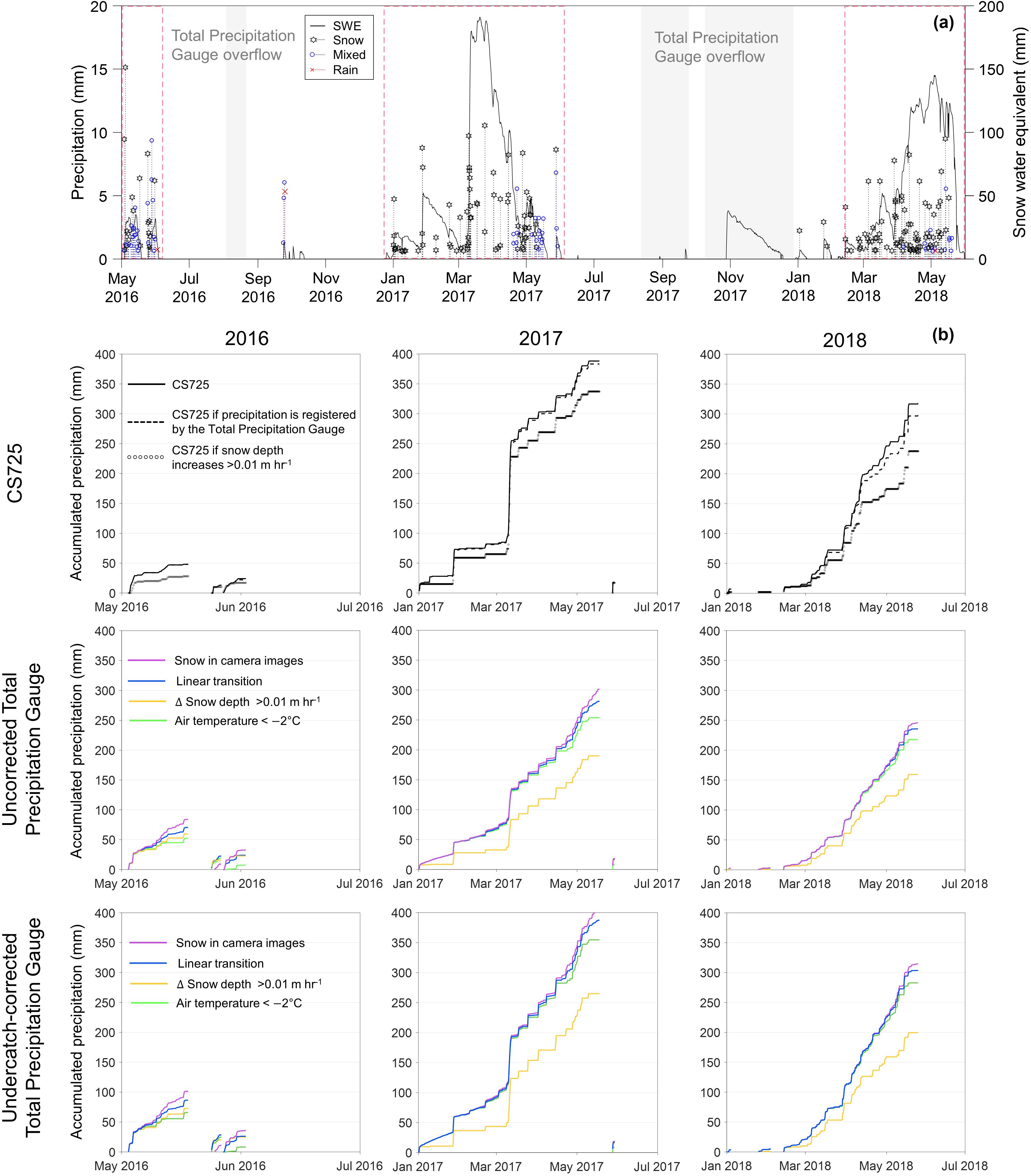
Figure 4. Cumulative SWE patterns for the CS725 and the Total Precipitation Gauge when substantial snow cover is present. (a) SWE measured by the CS725 and measured precipitation, split into solid, mixed and liquid phases using temperature thresholds. The three periods studied in panel (b) are highlighted in red dashed boxes. (b) Cumulative precipitation sums for the CS725 and the raw and undercatch corrected Total Precipitation Gauge using snow cover, air temperature, snow depth and a linear phase transition to determine when solid precipitation falls.
To assess the prevalence of snow redistribution by wind, we examine the number of SWE and snow depth increases that do not coincide with a corresponding precipitation measurement in the precipitation gauge. Approximately 19% of the CS725 increases in SWE and 9% of the increases in snow depth were not associated with a precipitation gauge measurement, possibly resulting from windblown snow redistribution. Using outgoing longwave radiation to calculate the snow surface temperature, assuming a snow emissivity of ∼0.98 (Hori et al., 2006), and measured changes in snow depth and SWE, we assess the lowering and loss of SWE from the snowpack in relation to snow surface temperature. This analysis reveals that, prior to April, the majority of surface lowering occurs when snow surface temperatures are below 0°C. Of this sub-zero lowering, 80% coincides with a loss of SWE from the snowpack. After April, the majority of surface lowering is associated with above-zero surface temperatures; although a diurnal cycle is present where the snowpack surface often continues to lower in the presence of sub-zero temperatures at night. When snow surface temperatures exceed 0°C, 84% of surface lowering is associated with a loss of SWE, with the remaining 16% possibly being related to snow redistribution, compaction, or refreezing within the snowpack within the footprint of the CS725.
During periods when snow height fluctuations occur in the absence of a change in SWE, mean wind speeds are typically low, ranging between 0.5 and 4 m s-1, although maximum wind speeds of up to 12 m s-1 were achieved during the hour of measurement. Evidence of wind scouring of the snowpack is also present in the time-lapse imagery for these periods. No significant difference is present between the wind speeds experienced during periods where snow depths fluctuate without a change in SWE, compared to periods when snow depth fluctuations are associated with a change in SWE.
Comparison Between Methods of Measuring Solid Precipitation
The cumulative SWE measured by the CS725 and the Total Precipitation Gauge for the three substantial snowpacks present between May 2016 and June 2018 is displayed in Figure 4b. The CS725 generally reports a higher cumulative SWE than the precipitation gauge, especially when the latter is not corrected for undercatch. Increases in SWE that were recorded by the CS725 but not the precipitation gauge caused the total cumulative SWE measured by the CS725 to differ from its raw value by between 4 mm and 20 mm by the end of the three periods.
When uncorrected for undercatch, the Total Precipitation Gauge captures 62% of the solid precipitation measured by the CS725 on average. In most cases, the precipitation gauge measures more similar quantities of solid precipitation to the CS725 when wind speeds are lower (<2.5 m s-1), air temperatures are higher (>-10°C) and snowfall is more intense. The observed catch efficiency of the Total Precipitation Gauge (compared to the CS725) is lower than that derived from the results of WMO-SPICE (Eq. 1), which calculates that the instrument should capture between 70 and 93% of incoming solid precipitation (95% confidence interval), with a mean catch efficiency of 81% (Figure 4b). These relatively high predicted catch efficiencies result from low downscaled mean hourly wind speeds, which typically do not exceed 2 m s-1 during snowfall events.
The catch efficiency performance of the Total Precipitation Gauge decreases substantially during an exceptionally large snow event that occurred between the 8th and 12th of March 2017 in which the SWE measured by the CS725 increased by 168 mm in 104 h. During this time period, the Total Precipitation Gauge measures only one third of the SWE gain quantified by the CS725. A theoretical catch efficiency of ∼75% is calculated for this time period, implying that an additional factor, such as the blockage of the precipitation gauge orifice by intense snowfall, resulted in this discrepancy and the poor performance of the instrument during this snowfall event.
When corrected for undercatch using Eq. 1, the Total Precipitation Gauge produces a better agreement with the CS725 (Figure 4b), although cumulative SWE is slightly overpredicted in comparison to the CS725 on average. The exception to this bias is in 2017 where the large March snowfall event falsely skews the accumulated precipitation toward the SWE measured by the CS725. The sum of precipitation measured by the undercatch-corrected Total Precipitation Gauge that fell when snow cover was present in the time-lapse images, compared to the sum that fell when air temperatures were below –2°C provides a maximum estimate for the contribution of mixed and rain-on-snow events to snowpack SWE. The majority of the precipitation that contributed to snowpack SWE occurred when air temperatures were below –2°C, with mixed precipitation and rain-on-snow events combined contributing 55 and 32 mm w.e. to the total snowpack SWE in 2017 and 2018, respectively. This equates to approximately 10–15% of the accumulated snowpack SWE. In this location, mixed precipitation and rain-on-snow events do not contribute SWE to the snowpack until late April/early May, when the average air temperature at the station shifts from -6.6°C ± 3.8°C to -1.8°C ± 2.7°C. Using increases in snow depth to determine when SWE was contributed to the snowpack results in the poorest comparison to the CS725, excluding approximately a quarter (118–145 mm w.e.) of the total SWE measured by the CS725. This pattern likely reflects a combination of snow depth increases that occur below the 0.01 m accuracy threshold of the SR50AT and the occurrence of mixed and rain-on-snow precipitation events. The use of a linear phase transition for precipitation results in excellent agreement with the CS725, reproducing the accumulated precipitation to within ∼6 mm of the CS725 in 2017 and 2018.
Representativeness of Automatic Weather Station Measurements
Uniformity of Snow Cover
The temporal variation in snow cover uniformity, assessed using time-lapse camera images of the area surrounding the automatic weather station, is displayed in Figure 5. Snowpacks are typically uniform during the accumulation period regardless of the snow depth (Figures 5a,b). Uniform snow cover is associated with an albedo between 0.46 and 0.84 (mean = 0.65), whilst the albedo of discontinuous snow cover ranges between 0.10 and 0.60, with a mean of 0.30 (95% confidence interval) (Supplementary Figure 2). Figures 5c,d display histograms of the SWE and snow depth values measured when discontinuous snow cover is present. The majority of discontinuous snow cover periods are associated with SWE values that are less than the 15 mm w.e. accuracy of the CS725; however, in some instances, SWE values of up to 50 mm are measured before the snowpack becomes uniform. Snow depths of less than 0.10 m tend to be associated with patchy snow cover, although depths of up to 0.18 m are infrequently recorded during periods in which snow cover is not uniform.
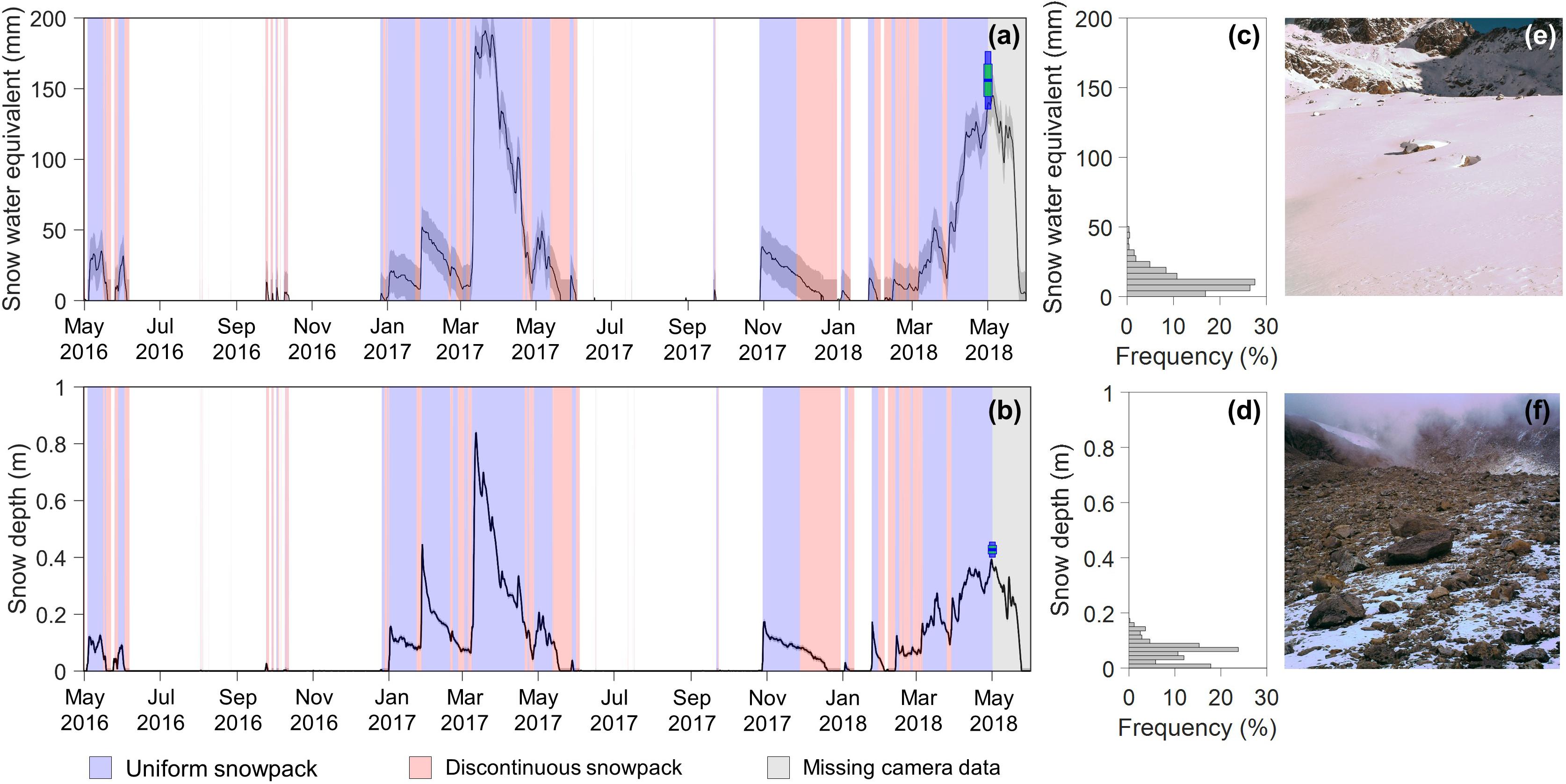
Figure 5. Temporal variation in SWE and snow cover continuity. (a,b) Snow cover classes for SWE and snow depth. Periods where a uniform snowpack is present are highlighted in purple and times when a discontinuous snowpack is present are shown in pink. Instrument uncertainties are displayed as a shaded gray band. Boxplots display the variation in snow pit SWE and depth measured on the 30th April, 2018. (c,d) The magnitude and proportion of SWE values and snow depths that occur while there is discontinuous snow cover. (e,f) Examples of uniform (e) and discontinuous (f) snow cover from time-lapse imagery close to the automatic weather station.
The number of “true” and “false” snowfall events detected by the SR50AT for different smoothing window lengths is shown in Figure 6. The raw data series detects 377 periods in which snow depth increases above the instrument’s 0.01 m h-1 accuracy threshold. Comparison with the time-lapse images reveals that only 58% of these detected increases in snow depth are real, meaning that 157 periods of instrument noise would be misclassified as snowfall if the raw data was used without a filter. Smoothing the snow depth timeseries using even a 2-h moving average filter dramatically reduces the number of “false” events that are classified as snow to only 6% of the total. Increasing the smoothing window length to 7 h removes all false events completely; however, this comes at the cost of a loss of ∼75% of the true events detected in the raw dataset. Window lengths greater than 7 h result in almost no further differences in the number of real events detected. The 2-h filter retains 79% of the “true” events detected in the raw dataset. Based on these results, it is preferable to use a 2-h smoothing filter to retain the maximum number of “true” snow events, and validate these results with the 7-h smoothed timeseries to ensure that any artifacts produced by the remaining 6% of events which relate to instrument noise do not influence the analysis.
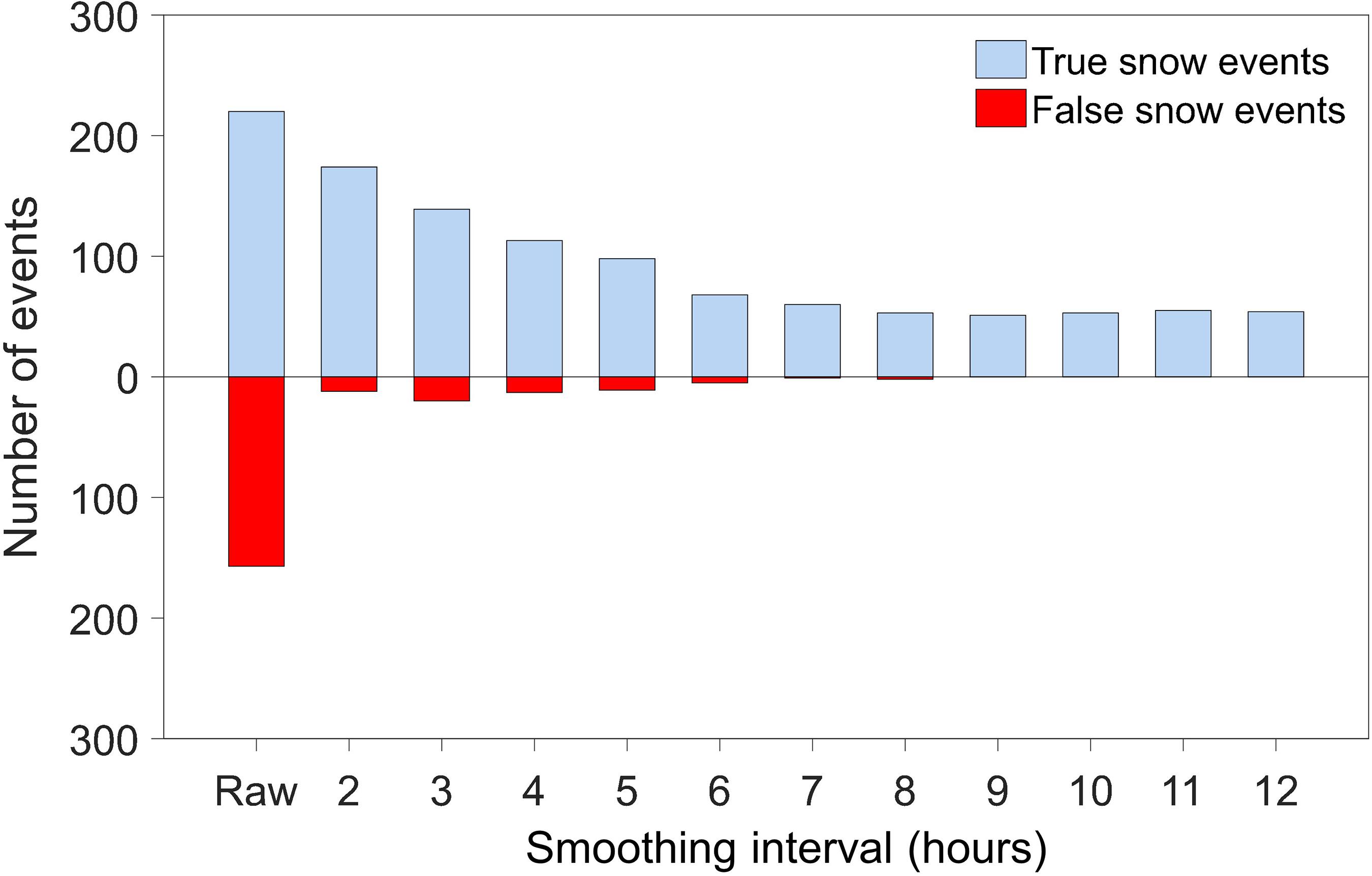
Figure 6. The effect of moving average smoothing interval on the number of true and false snow events detected by the snow depth sensor, verified by time-lapse camera images.
Comparison to Snow Pits
Figure 7 compares measured bulk density, measured snow depth and calculated SWE from the twelve snow pits on the 30th April, 2018, to the values derived from the automatic weather station instruments. The effective footprint of the SR50AT is 1.4 m2, whilst the CS725 has an effective footprint of ∼150 m2 (Figure 7a). Figure 7b displays the location and calculated SWE of the twelve snow pits dug around the automatic weather station. SWE values across the surface where no measurements were taken are estimated by linearly interpolating between the snow pit measurements. Calculated SWE values exhibit considerable spatial variation within the ∼200 m2 area covered by the snow pits, ranging between 122 mm and 196 mm w.e., with a mean of 156 mm w.e., resulting in a coefficient of variation of 13%. The interpolated average SWE value within the 1.4 m2 SR50AT footprint (146 mm w.e.) is representative of the value measured by the CS725 at this time (140 ± 15 mm w.e.) (Figure 7c).
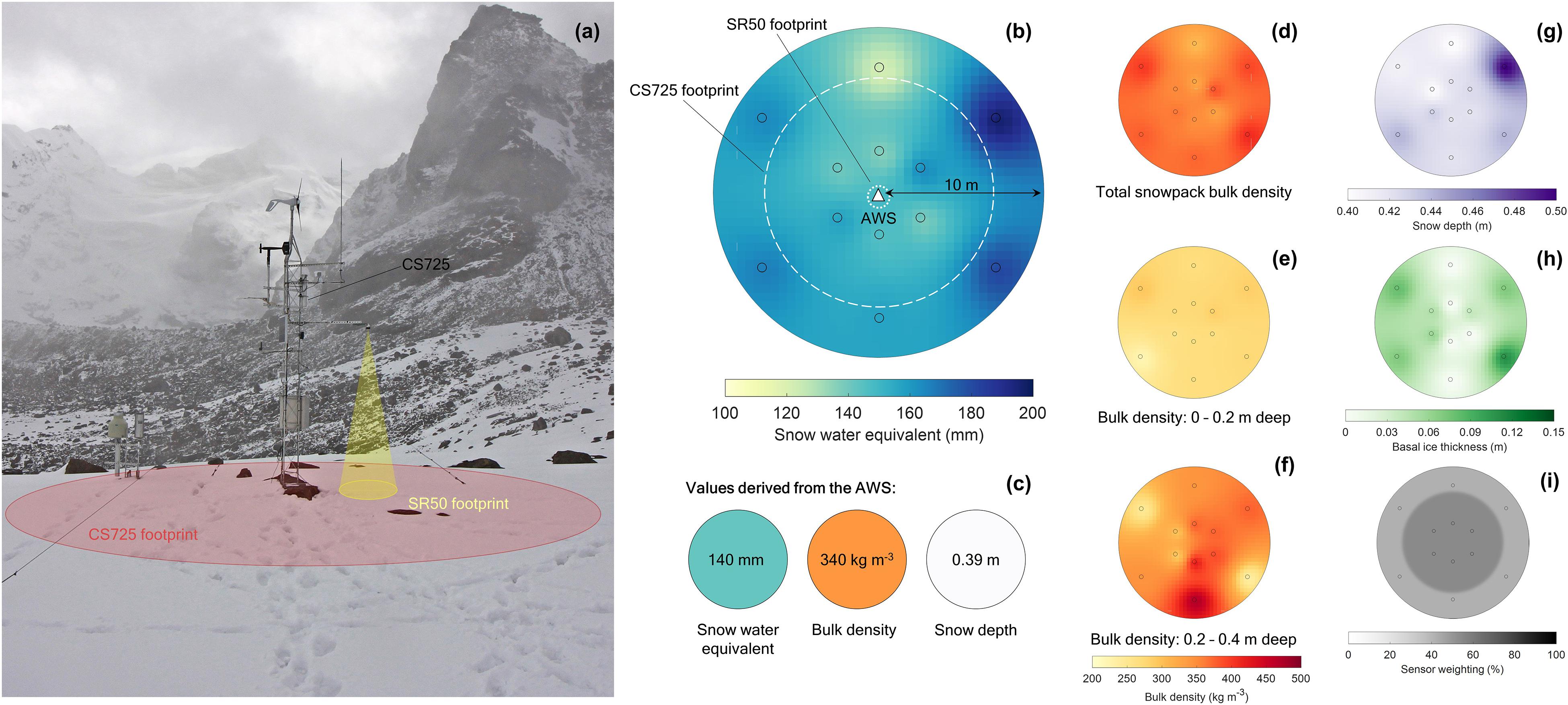
Figure 7. Comparison between snow pit SWE, bulk density and snow depth values and those derived from the CS725 and the snow depth sensor. (a) The automatic weather station displaying the effective footprints of the CS725 and the SR50AT snow depth sensor. (b) Spatial distribution of SWE derived from snow pit density and snow depth measurements around the automatic weather station (AWS). (c) SWE, bulk density and snow depth derived from the CS725 and the SR50AT. (d–f) Snowpack bulk density for: (d) the entire snowpack, (e) 0–0.2 m deep, (f) 0.2–0.4 m deep, (g) snow depth, (h) basal ice thickness, and (i) Spatial weighting of the CS725 SWE measurements.
Figures 7d–f present the bulk density of the whole snowpack (including basal ice), the upper 0–0.2 m section and the lower 0.2–0.4 m section of the snowpack, respectively, measured in the snow pits. The average bulk density of the entire snowpack is 364 ± 32 kg m-3 (±1 σ) and has a coefficient of variation of less than 9%. The average density of the upper 0–0.2 m portion of the snowpack is 270 ± 18 kg m-3 whist the lower 0.2–0.4 m section is approximately 1.3 times denser at 348 ± 69 kg m-3. The bulk density of the snowpack calculated from the automatic weather station measurements (by taking the quotient of SWE and snow depth) was 340 kg m-3 at the time when the snow pit measurements were made.
With the exception of one snow pit, the depth of the snowpack is relatively uniform, ranging between 0.40 and 0.50 m, with a mean value of 0.43 m and a coefficient of variation of 6%. The snow pits are 0.03 m deeper than the value measured by the SR50AT on average. Figure 7h displays the thickness of the basal ice layer situated between the base of the snowpack and the underlying substrate. The ice layer thickness is spatially variable, is not present in all snow pits and is up to 0.11 m thick. When averaged over the ∼150 m2 CS725 footprint, the basal ice is ∼0.03 m thick. Assuming an ice density of 910 kg m-3, this refrozen water corresponds to ∼30 mm of SWE, equivalent to ∼22% of the value measured by the CS725 for the overlapping 6-h time period. The presence of the thicker ice layers strongly influences the bulk density measurements which, when ice is present, are 30–46 kg m-3 greater than the average snowpack bulk density.
Discussion
Instrument Performance and Errors
The differences between the radiation-based and weighing-based methods of measuring SWE (Figure 4b) demonstrates some of the challenges and variability associated with obtaining reliable in situ measurements of solid precipitation in high-altitude environments (Sieck et al., 2007). In its raw form, the Total Precipitation Gauge captures 62% of the solid precipitation measured by the CS725 on average. For years in which a substantial snowpack was established, the Total Precipitation Gauge tends to record more similar quantities of precipitation to the CS725 when air temperatures are warmer and wind speeds are lower. Warmer air temperatures are commonly associated with higher fresh snow densities that are less easily disrupted by winds which, when combined with lower wind speeds, will increase the catch efficiency of precipitation gauges (Hedstrom and Pomeroy, 1998; Meløysund et al., 2007; Kochendorfer et al., 2017). The tendency for the Total Precipitation Gauge to overflow without regular maintenance at the end of the monsoon season resulted in the loss of useful data in the last three months of 2017.
The CS725 captures more solid precipitation than the uncorrected Total Precipitation Gauge, implying either that undercatch results in a reduction in the precipitation entering this instrument, or that the CS725 overestimates the SWE of the snowpack. Precipitation gauge undercatch can exceed 50% for snowfall when gauges are not windshielded (Sevruk et al., 1991; Ye et al., 2004; Rasmussen et al., 2012; Mekonnen et al., 2015; Wolff et al., 2015), and we estimate that between 19 and 46% of incoming solid precipitation is not recorded in the Total Precipitation Gauge on average (Kochendorfer et al., 2017). This difference agrees well with the 38% discrepancy present between the Total Precipitation Gauge and the CS725. However, the CS725 has previously been found to overestimate SWE by between 18 and 35% in boreal climates in Finland, Switzerland, and Canada (Choquette et al., 2008; Smith C.D. et al., 2017). This error has been attributed to the infiltration and retention of snowmelt into soils prior to freeze up which alters the soil moisture content compared to the reference conditions that the CS725 is calibrated for. Water stored in the soil will continue to attenuate gamma radiation, resulting in a false overestimation of SWE (Gray et al., 2001; Smith C.D. et al., 2017).
In this particular region, however, we suggest that the monsoon-dominated climate may minimize the prevalence of this issue. The automatic weather station’s surface temperature sensor demonstrates that unfrozen soil conditions begin around June in the presence of snow-free conditions and continue until the establishment of a snowpack in November to December (Figure 3c). The precipitation regime of this region is monsoon dominated (Immerzeel et al., 2009, 2014), with ∼80% of the total annual precipitation recorded at Ganja La falling onto unfrozen ground during the summer monsoon. Assuming a temperature threshold of 2°C (Ye et al., 2004; Kochendorfer et al., 2017), liquid precipitation is observed to fall up until the advent of frozen ground conditions, shortly after which a snowpack typically forms. The short transition between the last of the liquid precipitation events and the onset of substrate freezing implies that any soil beneath the CS725 will be at or near to saturation when the ground freezes. As monsoon precipitation occurs consistently from year to year, the ground moisture content when new snowpacks form is likely to be similar to when the CS725 was first calibrated. Thus, the sensor may not be as prone to overestimation as previously recorded in other climatic settings. This conclusion is supported by our snow pit SWE estimates which, at an average of 156 mm w.e., are comparable with or even slightly higher, than the 140 ± 15 mm w.e. measured by the CS725, 2.5 years after the instrument was first calibrated. Accordingly, we suggest that the 38% precipitation discrepancy between the uncorrected Total Precipitation Gauge data and the CS725 is likely related to precipitation undercatch rather than an overestimation of SWE by the CS725.
The Ganja La automatic weather station is situated in the 4000–5000 m a.s.l. elevation band that is highly important for water storage and meltwater generation in this high mountain region (Immerzeel et al., 2009; Tiwari et al., 2016; Gurung et al., 2017). At this location, very little (10–15%) of the annual SWE falls as mixed precipitation or rain-on-snow events, emphasizing the importance of the need to obtain reliable estimates of solid precipitation in Himalayan environments. Despite its failure to successfully replicate the snowfall that occurred in March 2017, the undercatch-corrected Total Precipitation Gauge timeseries generally corresponds closely to that of the CS725, especially during periods when no exceptionally large snowfall events occurred (Figure 4). This result demonstrates that the undercatch correction function derived from Kochendorfer et al. (2017), despite having a tendency toward slightly overpredicting the quantity of SWE, generally performs well in this high-altitude environment and could potentially be used to correct similar precipitation gauges deployed in other Himalayan regions.
Approximately 20% of the snow depth changes measured by the SR50AT are not associated with a change in SWE and likely reflect a combination of snow redistribution by wind within the footprint of the CS725, internal refreezing of meltwater, and surface compaction. The fact that wind-related processes appear to play an important role in even the relatively sheltered Ganja La site where windspeeds are generally low (Figure 3e), highlights the value of having a SWE sensor with a large footprint as this removes the issue of undercatch altogether and avoids many of the spatial variability issues that are associated with point measurements of SWE (Osterhuber et al., 1998; Wright, 2013; Kinar and Pomeroy, 2015; Smith C.D. et al., 2017). Large proportions of surface lowering occur in sub-zero temperatures, particularly in the winter and early pre-monsoon seasons when relative humidities are low and wind speeds are comparatively high. These periods of SWE loss and surface lowering likely correspond to wind scouring of surface snow and the loss of SWE directly to the atmosphere through sublimation. Low atmospheric pressures, high wind speeds, dry air, and low surface vapor pressures make sublimation an important process at high-altitude locations (Strasser et al., 2008; Wagnon et al., 2013). Relatively high average sublimation rates of 1.0 mm w.e. per day, or 21% of annual snowfall, have been reported for the nearby Yala Glacier in the Langtang Catchment (Stigter et al., 2018) and Saloranta et al. (2019) report that sublimation accounts for 17% of all SWE losses at Ganja La. The importance of sublimation as a process in high-altitude environments demonstrates the value of applying a dedicated SWE sensor, along with complementary meteorological instruments, that can distinguish SWE losses from redistribution within the footprint of the sensor and quantify the loss of SWE under different meteorological conditions.
Due to the sensor’s 24-h integration of gamma radiation, the larger monitoring area of the CS725 comes at the cost of the loss of near-instantaneous monitoring capacity. Measurements from the CS725 must also be carefully interpreted due to its averaging of spatially heterogeneous SWE, which is influenced by the location of refrozen meltwater (Figure 7). Furthermore, the uniformity of the snowpack must be considered when interpreting results derived from this method as patchy snow cover can contain up to 50 mm SWE without being a substantial and uniform snowpack (Figure 5). The sensor will generally underestimate SWE during non-uniform snow cover conditions as gamma photon intensity decreases exponentially with greater snowpack water content. This issue can be exemplified using an extreme scenario where an area consists of 50% bare ground (0 mm w.e.) and 50% thick snow cover (600 mm w.e.). In this situation, although gamma radiation from the snow-covered portion of ground would be almost completely absorbed, the average intensity of gamma radiation reported by the instrument will be equal to half of the radiation emitted from the completely snow-free influence area. Simplified, this equates to approximately 50 mm of SWE, whereas the actual average SWE of the area would be 300 mm. Thus, radiation-based methods of SWE measurement will always have a bias toward the lower SWE sub-regions of the measured area during non-uniform snow cover conditions, although for the maximum SWE values observed here under patchy snow cover (50 mm), this effect would only amount to a ∼4 mm underestimation of SWE.
The bulk density calculated using the automatic weather station data exhibits excellent agreement with the range of densities measured in the snow pits on the 30th April, 2018 (Figure 7c). Although the snow pits represent only one point in time and are invariably not without error due to the difficulty of taking manual snow cores containing ice layers at high altitude in sub-zero temperatures (Smith C.D. et al., 2017), the pits capture a valuable snapshot of the spatial variability of SWE, snow depth and bulk density in this location. Calculating snowpack bulk density from continuous automatic weather station observations of SWE and snow depth comes with the caveat that this measure is only reliable when the footprints of the SR50AT and the CS725 are representative of the average values of the whole snowpack. As demonstrated by the time-lapse images and the low coefficients of variation associated with our snow pit SWE and depth measurements (13 and 6%, respectively), snow cover tends to be uniform during periods of snowpack accumulation (Figure 5), suggesting little snow depth variability within the CS725 footprint. Accordingly, when snow cover is uniform, the quotient of the CS725 and the SR50AT can be used to estimate snowpack bulk density. However, this method will be less accurate for periods of discontinuous snow cover when the snowpack is ablating.
Recommendations for Remote, High-Altitude Snowpack Monitoring
In this section, we use our assessment of instrument performance to postulate about the optimal setup for an automatic weather station that could be installed in a remote, high-altitude location and left without the need for maintenance for several years. Such a setup could provide input to achieve near-real time hydrological forecasting in a region where seasonal snow resources are important for millions of people.
The transmission of data using the Iridium satellite constellation is a robust and convenient method for achieving near-real time monitoring of snow conditions in high mountain environments. Variables measured and transmitted using this technique can be used to force near-real time simulations of the timing and magnitude of snowmelt into the catchment hydrological system and forecast avalanche risk (Saloranta et al., 2019). In order for these efforts to be worthwhile in the long term, the setup should require minimal maintenance whilst providing representative estimates of their measured variables of interest.
Reliable estimates of precipitation are some of the most important measurements to collect when attempting to monitor seasonal water resources (Jonas et al., 2009; Rohrer et al., 2013). Precipitation gauges are more extensively deployed in the Himalayas than dedicated SWE sensors (Andermann et al., 2011). Although it is preferable to install a windshield on these instruments to reduce the magnitude of precipitation undercatch, our results demonstrate that, under most conditions, unshielded precipitation weighing gauges can be corrected for undercatch using simple wind speed and temperature inputs to within 20% of an independent SWE sensor. The accuracy of undercatch-corrected precipitation gauges can be improved by using these instruments in combination with time-lapse camera images to verify snow events, or by applying linear precipitation phase transitions to the data collected (Figure 4b; Harder and Pomeroy, 2013). However, the possibility of precipitation gauge blockage under exceptional snow conditions remains a serious issue when attempting to accurately monitor the total quantity of precipitation stored as seasonal snow. This issue is very difficult to detect without reference to an independent SWE sensor or a camera pointed at the precipitation gauge. Taking the event which occurred between the 8th and 12th of March 2017 as an example, the loss of over two thirds of this SWE due to instrument blockage would seriously affect understanding of avalanche risk or the quantity of snowpack water storage predicted by a snow model and consequently alter the strategic efforts to mitigate the risk of these hazards.
This study has shown through the comparison of two SWE sensors that when corrected for undercatch, weighing gauge systems such as the Total Precipitation Gauge provide a relatively accurate method of measuring solid precipitation in Himalayan environments. However, the need to manually empty this type of gauge remains problematic in remote locations that cannot be accessed recurrently. Although self-emptying versions of gauges similar to the Total Precipitation Gauge are available, antifreeze is still required to be prevent damage to the instrument which requires a field team to visit the site regularly. Similar issues exist for snow pillows or weighing scales, as the former requires large quantities of antifreeze and the latter requires the transportation of heavy material to the study site, which is impractical in rugged high-altitude mountain terrain. Furthermore, snow pillows and weighing scales are prone to snow bridging produced by the refreezing of hard snow crusts that partially support the weight of the snowpack (Engeset et al., 2000; Smith C.D. et al., 2017) and, in the case of snow pillows, must be corrected for measurement errors produced by the differential compression of the instrument (Johnson, 2004; Johnson and Marks, 2004).
Although typically several times more expensive than weighing gauge systems, radiation-based methods of SWE monitoring such as the CS725 are comparatively well suited for remote high-altitude environments as they are portable, provide consistent measurement performance regardless of snow type, snowpack structure, or properties, are not affected by snow bridging, precipitation undercatch or adverse weather conditions, and their passive measurements do not disturb the internal structure of the snowpack (Osterhuber et al., 1998; Wright, 2013; Campbell, 2015; Stranden et al., 2015). Furthermore, this type of instrument is not affected by extremely cold temperatures, requires no antifreeze, and can typically be left in the field for 7 years without maintenance (Wright, 2013; Stranden et al., 2015). Radiation-based methods of SWE measurement also continue to measure the water content of the snowpack regardless of the phase that the water is stored in, reducing the scope to misinterpret the melting and refreezing of water within the snowpack as snowmelt and runoff.
The CS725 is capable of monitoring snowpacks up to approximately 600 mm w.e. Above this threshold, gamma radiation is attenuated to below the detection threshold of the instrument, although in general greater snow depths are beneficial as the radioactivity signal to noise ratio decreases as snow depth increases, yielding more accurate measurements (Kinar and Pomeroy, 2015). The applicability of radiation-based methods of SWE measurement may therefore be restricted in some locations by the need for installation sites to have a minimum background level of naturally occurring radioactive isotopes present in the substrate or in areas where particularly large snowpacks are present (Campbell, 2015; Stranden et al., 2015). Average winter SWE values are generally below 140 mm w.e. in High Mountain Asia, although higher snowpack SWE can exist in some high-elevation areas (Ménégoz et al., 2013; Tiwari et al., 2015, 2016; Smith and Bookhagen, 2018). The majority of snow cover in the Langtang catchment is less than 600 mm w.e. (Stigter et al., 2017; Saloranta et al., 2019). Locations where SWE exceeds 600 mm w.e. generally occur below very steep slopes in avalanche-prone terrain where the installation and long-term sustainability of a SWE sensor would be difficult to achieve. Thus, in most instances, the upper limit of SWE detection should not be a significant problem when siting the instrument in high mountain environments. The delayed integration of the CS725 may be problematic for uses that require instantaneous knowledge of SWE; however, with the exception of extreme snowfall events, using the SWE value of the previous day is likely to be sufficient for most monitoring purposes.
A snow depth sensor such as the SR50AT may be combined with a SWE sensor to provide calculated estimates of changing snowpack bulk density dynamics with which to develop and test numerical snow models in this region. However, the small footprint of the snow depth sensor may result in measurements being unrepresentative over larger areas due to the spatial heterogeneity commonly associated with snow depth (López-Moreno et al., 2013; Grünewald and Lehning, 2015). Additionally, the accuracy of the SR50AT can also be affected by a skewed sensor orientation, poor sound reflectance from extremely low-density snow, and rough or uneven surfaces (Campbell, 2016). This study has demonstrated that the installation of a time-lapse camera provides valuable imagery with which to cross-check the validity of snowfall events, optimizing the spatial resolution and continuity of snow depth and SWE measurements made remotely in the field. Unfortunately, this imagery is currently unable to be transmitted in near real-time. Cameras that transmit photos through a cellular network are becoming increasingly available on the market, but these would need to be adapted for the lack of phone signal in high-altitude Himalayan environments.
Overall, the large footprint, low maintenance setup and reliable performance of radiation-based measurements of SWE make this type of instrument suitable for monitoring remote high-altitude snow cover. SWE measurement accuracy and reliability can be improved by correcting instruments for time lag and wind-related issues using sensors that provide instantaneous measurements of snow depth and precipitation, or by validating SWE measurements in context using time-lapse camera imagery. Previous research has demonstrated that this region is characterized by significant and seasonally varying gradients of precipitation and temperature which need to be considered when attempting to spatially distribute automatic weather station measurements over a larger spatial scale (Immerzeel et al., 2014). If several stations, similar to the automatic weather station presented here, were installed along an elevational transect, precipitation and temperature gradients could be estimated in near real-time across a catchment, permitting snow properties to be modeled and estimated at the catchment scale or beyond (Saloranta et al., 2019). When combined with a reliable satellite-based transmission service, this instrument setup is capable of delivering valuable near real-time information about seasonal water resources stored as snow in high-altitude locations.
Conclusion
In this study we have presented a unique meteorological dataset comprising 2 years of continuous SWE, snow depth, precipitation, air temperature, relative humidity, surface temperature, wind speed, wind direction, barometric pressure, and shortwave and longwave solar radiation data from a high-altitude weather station at 4962 m a.s.l. in the Nepal Himalayas. The automatic weather station measurements are transmitted hourly via the Iridium satellite constellation, and are immediately available. We have assessed the accuracy and spatial representativeness of SWE and snow depth measurements using snow pits and time-lapse camera imagery. Our results show that although measurement errors become greater when snow cover thins, the automatic weather station measurements are spatially and temporally representative and are capable of providing valuable insights into the timing and processes governing the high altitude water cycle. The automatic monitoring system is capable of providing continuous SWE, snow depth, and snowpack bulk density measurements that could be used to develop and calibrate density and SWE models in this poorly documented region.
Wind scouring and redistribution of snow produces prominent measurement artifacts even in sheltered locations and care must be taken when interpreting precipitation measurements with narrow footprints and orifices. The application of sub-daily time-lapse camera imagery can help to correctly interpret and increase the reliability and representativeness of snow surface measurements. Over 85% of incoming SWE at the elevation of the automatic weather station fell as solid precipitation, emphasizing the importance of obtaining reliable estimates of snowfall in Himalayan environments. Comparison between an independent SWE sensor and a precipitation gauge has shown that precipitation gauges significantly underrepresent the magnitude of solid precipitation received in high elevation regions by almost 40%; however, this bias can largely be corrected using simple wind speed and temperature inputs.
Widespread deployment of similarly robust, relatively maintenance free snow measurement stations that transmit data remotely using satellite connection across other Himalayan regions would permit widespread near-real time monitoring of seasonal Himalayan snowpacks. This monitoring setup may then permit snow condition assessment, hydrological forecasting, and evaluation of avalanche threats to be made at a temporal resolution that is currently unavailable in this region of the world.
Author Contributions
JK, IK, ML, and TS designed the study with inputs from ES and WI. JK conducted the analysis together with IK, ML, and TS, and wrote the initial version of the manuscript. KnM designed the measurement station setup. AT, KnM, KjM, and ML participated in initial automatic weather station installation in Langtang in 2015. All authors participated in fieldwork to collect the data, and setup and maintain the automatic weather station between 2015 and 2018, and contributed to data interpretation and writing of the final version of the manuscript.
Funding
This work was supported by the International Centre for Integrated Mountain Development’s Cryosphere Initiative funded by Norway, and by core funds contributed by the Governments of Afghanistan, Australia, Austria, Bangladesh, Bhutan, China, India, Myanmar, Nepal, Norway, Pakistan, Sweden, and Switzerland. Research was also financially supported by the European Research Council under the European Union’s Horizon 2020 Research and Innovation Program (Grant Agreement No. 676819) and the Netherlands Organization for Scientific Research (NWO) under the Innovational Research Incentives Scheme VIDI (Grant Agreement No. 016.181.308).
Conflict of Interest Statement
The authors declare that the research was conducted in the absence of any commercial or financial relationships that could be construed as a potential conflict of interest.
Acknowledgments
The automatic weather station was established in October 2015 as part of the Snow accumulation and melt processes in a Himalayan catchment project developed by the International Centre for Integrated Mountain Development, the Norwegian Water Resources and Energy Directorate, and the Department of Hydrology and Meteorology Nepal. The views and interpretations in this publication are those of the authors and they are not necessarily attributable to their organizations. We would like to thank all who supported us during fieldwork or provided the equipment that was required. We thank the Kathmandu University and the Department of National Parks and Wildlife Conservation for facilitation of our research permits. We also thank Anna Sinisalo and Graham Sexstone for their helpful thoughts and suggestions during manuscript preparation, and two reviewers for their comments and ideas that helped to improve the manuscript.
Supplementary Material
The Supplementary Material for this article can be found online at: https://www.frontiersin.org/articles/10.3389/feart.2019.00177/full#supplementary-material
The raw meteorological data used in this study is available in Supplementary Data Sheets 1, 2.
Footnotes
References
Andermann, C., Bonnet, S., and Gloaguen, R. (2011). Evaluation of precipitation data sets along the Himalayan front. Geochem. Geophys. Geosyst. 12:Q07023. doi: 10.1029/2011GC003513
Appel, F., Koch, F., Rösel, A., Klug, P., Henkel, P., Lamm, M., et al. (2019). Advances in snow hydrology using a combined approach of GNSS in situ stations, hydrological modelling and earth observation—a case study in Canada. Geosciences 9:44. doi: 10.3390/geosciences9010044
Bajracharya, S. R., Maharjan, S. B., Shrestha, F., Bajracharya, O. R., and Baidya, S. (2014). Glacier Status in Nepal and Decadal Change from 1980 to 2010 Based on Landsat Data. Kathmandu: International Centre for Integrated Mountain Development (ICIMOD).
Barnett, T. P., Adam, J. C., and Lettenmaier, D. P. (2005). Potential impacts of a warming climate on water availability in snow-dominated regions. Nature 438:303. doi: 10.1038/nature04141
Best, M. J., Pryor, M., Clark, D. B., Rooney, G. G., Essery, R. L. H., Ménard, C. B., et al. (2011). The Joint UK Land Environment Simulator (JULES), model description. Part 1: energy and water fluxes. Geosci. Model Dev. 4, 677–699. doi: 10.5194/gmd-4-677-2011
Bolch, T., Kulkarni, A., Kääb, A., Huggel, C., Paul, F., Cogley, J. G., et al. (2012). The state and fate of Himalayan glaciers. Science 336, 310–314. doi: 10.1126/science.1215828
Bookhagen, B., and Burbank, D. W. (2010). Toward a complete Himalayan hydrological budget: Spatiotemporal distribution of snowmelt and rainfall and their impact on river discharge. J. Geophys. Res. Earth Surf. 115:F03019. doi: 10.1029/2009JF001426
Bormann, K. J., Westra, S., Evans, J. P., and McCabe, M. F. (2013). Spatial and temporal variability in seasonal snow density. J. Hydrol. 484, 63–73. doi: 10.1016/j.jhydrol.2013.01.032
Brun, E., David, P., Sudul, M., and Brunot, G. (1992). A numerical model to simulate snow-cover stratigraphy for operational avalanche forecasting. J. Glaciol. 38, 13–22. doi: 10.3189/S0022143000009552
Brun, E., Martin, E, Simon, V., Gendre, C., and Coleou, C. (1989). An energy and mass model of snow cover suitable for operational avalanche forecasting. J. Glaciol. 35, 333–342. doi: 10.3189/S0022143000009254
Campbell (2015). CS725 Snow Water Equivalent Sensor Instruction Manual. Edmonton, AB: Campbell Scientific Corp.
Campbell (2016). SR50AT-316SS-SR50ATH Sensor Instruction Manual. Edmonton, AB: Campbell Scientific Corp.
Carroll, S. S., Carroll, T. R., and Poston, R. W. (1999). Spatial modeling and prediction of snow-water equivalent using ground-based, airborne, and satellite snow data. J. Geophys. Res. Atmos. 104, 19623–19629. doi: 10.1029/1999JD900093
Choquette, Y., Lavigne, P., Nadeau, M., Ducharme, P., Martin, J. P., Houdayer, A., et al. (2008). “GMON, a new sensor for snow water equivalent via gamma monitoring,” in Proceedings Whistler 2008 International Snow Science Workshop, Whistler, BC.
Colli, M., Rasmussen, R., Thériault, J. M., Lanza, L. G., Baker, C. B., and Kochendorfer, J. (2015). An improved trajectory model to evaluate the collection performance of snow gauges. J. Appl. Meteorol. Climatol. 54, 1826–1836. doi: 10.1175/JAMC-D-15-0035.1
Dong, J., Walker, J. P., and Houser, P. R. (2005). Factors affecting remotely sensed snow water equivalent uncertainty. Remote Sens. Environ. 97, 68–82. doi: 10.1016/j.rse.2005.04.010
Egli, L., Jonas, T., and Meister, R. (2009). Comparison of different automatic methods for estimating snow water equivalent. Cold Regions Sci. Technol. 57, 107–115. doi: 10.1016/j.coldregions.2009.02.008
Engeset, R., Sorteberg, H., and Udnaes, H. (2000). “Snow pillows: Use and verification,” in Proceedings of the Fourth International Conference on Snow Engineering, Snow Engineering: Recent Advances and Developments, Trondheim.
Essery, R., Morin, S., Lejeune, Y., and Ménard, C. B. (2013). A comparison of 1701 snow models using observations from an alpine site. Adv. Water Res. 55, 131–148. doi: 10.1016/j.advwatres.2012.07.013
Gardner, A. S., Moholdt, G., Cogley, J. G., Wouters, B., Arendt, A. A., Wahr, J., et al. (2013). A reconciled estimate of glacier contributions to sea level rise: 2003 to 2009. Science 340, 852–857. doi: 10.1126/science.1234532
Gray, D. M., Toth, B., Zhao, L., Pomeroy, J. W., and Granger, R. J. (2001). Estimating areal snowmelt infiltration into frozen soils. Hydrol. Process. 15, 3095–3111. doi: 10.1002/hyp.320
Grünewald, T., and Lehning, M. (2015). Are flat-field snow depth measurements representative? A comparison of selected index sites with areal snow depth measurements at the small catchment scale. Hydrol. Process. 29, 1717–1728. doi: 10.1002/hyp.10295
Gurung, D. R., Maharjan, S. B., Shrestha, A. B., Shrestha, M. S., Bajracharya, S. R., and Murthy, M. S. R. (2017). Climate and topographic controls on snow cover dynamics in the Hindu Kush Himalaya. Int. J. Climatol. 37, 3873–3882. doi: 10.1002/joc.4961
Harder, P., and Pomeroy, J. (2013). Estimating precipitation phase using a psychrometric energy balance method. Hydrol. Process. 27, 1901–1914. doi: 10.1002/hyp.9799
Hedstrom, N. R., and Pomeroy, J. W. (1998). Measurements and modelling of snow interception in the boreal forest. Hydrol. Process. 12, 1611–1625. doi: 10.1002/(sici)1099-1085(199808/09)12:10/11<1611::aid-hyp684>3.0.co;2-4
Henkel, P., Koch, F., Appel, F., Bach, H., Prasch, M., Schmid, L., et al. (2018). Snow water equivalent of dry snow derived from GNSS carrier phases. IEEE Trans. Geosci. Remote Sens. 56, 3561–3572. doi: 10.1109/TGRS.2018.2802494
Hori, M., Aoki, T., Tanikawa, T., Motoyoshi, H., Hachikubo, A., Sugiura, K., et al. (2006). In-situ measured spectral directional emissivity of snow and ice in the 8-14 m atmospheric window. Remote Sens. Environ. 100, 486–502. doi: 10.1016/j.rse.2005.11.001
Immerzeel, W. W., Droogers, P., De Jong, S. M., and Bierkens, M. F. P. (2009). Large-scale monitoring of snow cover and runoff simulation in Himalayan river basins using remote sensing. Remote Sens. Environ. 113, 40–49. doi: 10.1016/j.rse.2008.08.010
Immerzeel, W. W., Petersen, L., Ragettli, S., and Pellicciotti, F. (2014). The importance of observed gradients of air temperature and precipitation for modeling runoff from a glacierized watershed in the Nepalese Himalayas. Water Res. Res. 50, 2212–2226. doi: 10.1002/2013WR014506
Immerzeel, W. W., Van Beek, L. P. H., and Bierkens, M. F. P. (2010). Climate change will affect the Asian water towers. Science 328, 1382–1385. doi: 10.1126/science.1183188
Jepsen, S. M., Molotch, N. P., Williams, M. W., Rittger, K. E., and Sickman, J. O. (2012). Interannual variability of snowmelt in the Sierra Nevada and Rocky Mountains, United States: examples from two alpine watersheds. Water Res. Res. 48:W02529. doi: 10.1029/2011WR011006
Johnson, J. B. (2004). A theory of pressure sensor performance in snow. Hydrol. Process. 18, 53–64. doi: 10.1002/hyp.1310
Johnson, J. B., Gelvin, A. B., Duvoy, P., Schaefer, G. L., Poole, G., and Horton, G. D. (2015). Performance characteristics of a new electronic snow water equivalent sensor in different climates. Hydrol. Process. 29, 1418–1433. doi: 10.1002/hyp.10211
Johnson, J. B., and Marks, D. (2004). The detection and correction of snow water equivalent pressure sensor errors. Hydrol. Process. 18, 3513–3525. doi: 10.1002/hyp.5795
Jonas, T., Marty, C., and Magnusson, J. (2009). Estimating the snow water equivalent from snow depth measurements in the Swiss Alps. J. Hydrol. 378, 161–167. doi: 10.1016/j.jhydrol.2009.09.021
Judson, A., and Doesken, N. (2000). Density of freshly fallen snow in the central Rocky Mountains. Bull. Am. Meteorol. Soc. 81, 1577–1587. doi: 10.1175/1520-0477(2000)081<1577:doffsi>2.3.co;2
Kinar, N. J., and Pomeroy, J. W. (2007). Determining snow water equivalent by acoustic sounding. Hydrol. Process. Int. J. 21, 2623–2640. doi: 10.1002/hyp.6793
Kinar, N. J., and Pomeroy, J. W. (2015). Measurement of the physical properties of the snowpack. Rev. Geophys. 53, 481–544. doi: 10.1002/2015RG000481
Kochendorfer, J., Nitu, R., Wolff, M., Mekis, E., Rasmussen, R., Baker, B., et al. (2017). Analysis of single-Alter-shielded and unshielded measurements of mixed and solid precipitation from WMO-SPICE. Hydrol. Earth Syst. Sci. 21, 3525–3542. doi: 10.5194/hess-21-3525-2017
Kodama, M., Nakai, K., Kawasaki, S., and Wada, M. (1979). An application of cosmic-ray neutron measurements to the determination of the snow-water equivalent. J. Hydrol. 41, 85–92. doi: 10.1016/0022-1694(79)90107-0
Kraaijenbrink, P. D. A., Bierkens, M. F. P., Lutz, A. F., and Immerzeel, W. W. (2017). Impact of a 1.5°C global temperature rise on Asia’s glaciers. Nature 549, 257–260. doi: 10.1038/nature23878
Lang, T., and Barros, A. P. (2004). Winter Storms in the central Himalayas. J. Meteorol. Soc. Japan 82, 829–844. doi: 10.2151/jmsj.2004.829
Lehning, M., Bartelt, P., Brown, B., Russi, T., Stöckli, U., and Zimmerli, M. (1999). SNOWPACK model calculations for avalanche warning based upon a new network of weather and snow stations. Cold Regions Sci. Technol. 30, 145–157. doi: 10.1016/s0165-232x(99)00022-1
López-Moreno, J. I., Fassnacht, S. R., Heath, J. T., Musselman, K. N., Revuelto, J., Latron, J., et al. (2013). Small scale spatial variability of snow density and depth over complex alpine terrain: Implications for estimating snow water equivalent. Adv Water Res. 55, 40–52. doi: 10.1016/j.advwatres.2012.08.010
Lutz, A. F., Immerzeel, W. W., Litt, M., Bajracharya, S., and Shrestha, A. B. (2015). Comprehensive Review of Climate Change and the Impacts on Cryosphere, Hydrological Regimes and Glacier Lakes. Available at: http://lib.icimod.org/record/32006 (accessed December 2015).
Lutz, A. F., Immerzeel, W. W., Shrestha, A. B., and Bierkens, M. F. P. (2014). Consistent increase in High Asia’s runoff due to increasing glacier melt and precipitation. Nat. Clim. Change 4, 587–592. doi: 10.1038/nclimate2237
Ma, L., Zhang, T., Frauenfeld, O. W., Ye, B., Yang, D., and Qin, D. (2009). Evaluation of precipitation from the ERA-40, NCEP-1, and NCEP-2 reanalyses and CMAP-1, CMAP-2, and GPCP-2 with ground-based measurements in China. J. Geophys. Res. 114:D09105. doi: 10.1029/2008JD011178
MacDonald, J., and Pomeroy, J. W. (2007). “Gauge undercatch of two common snowfall gauges in a prairie environment,” in Proceedings of the 64th Eastern Snow Conference, St. John’s, NL.
Martin, J. P., Houdayer, A., Lebel, C., Choquette, Y., Lavigne, P., and Ducharme, P. (2008). “An unattended gamma monitor for the determination of snow water equivalent (SWE) using the natural ground gamma radiation,” in Proceedings of the Nuclear Science Symposium Conference Record, 2008. NSS’08. IEEE, (Piscataway, NJ: IEEE), 983–988.
McCreight, J. L., and Small, E. E. (2014). Modeling bulk density and snow water equivalent using daily snow depth observations. Cryosphere 8, 521–536. doi: 10.5194/tc-8-521-2014
Mekonnen, G. B., Matula, S., Doležal, F., and Fišák, J. (2015). Adjustment to rainfall measurement undercatch with a tipping-bucket rain gauge using ground-level manual gauges. Meteorol. Atmos. Phys. 127, 241–256. doi: 10.1007/s00703-014-0355-z
Meløysund, V., Leira, B., Høiseth, K. V., and Lisø, K. R. (2007). Predicting snow density using meteorological data. Meteorol. Appl. 14, 413–423. doi: 10.1002/met.40
Ménégoz, M., Gallée, H., and Jacobi, H. W. (2013). Precipitation and snow cover in the Himalaya: from reanalysis to regional climate simulations. Hydrol. Earth Syst. Sci. 17, 3921–3936. doi: 10.5194/hess-17-3921-2013
Osterhuber, R., Gehrke, F., and Condreva, K. (1998). “Snowpack snow water equivalent measurement using the attenuation of cosmic gamma radiation,” in Proceedings Western Snow Conference, Snowbird.
Palazzi, E., Von Hardenberg, J., and Provenzale, A., (2013). Precipitation in the Hindu-Kush Karakoram Himalaya: observations and future scenarios. J. Geophys. Res. Atmos. 118, 85–100. doi: 10.1029/2012JD018697
Pipes, A., and Quick, M. (1977). UBC Watershed Model Users Guide. Vancouver: Department of Civil Engineering, University of British Columbia.
Polanski, S., Rinke, A., and Dethloff, K. (2010). Validation of the HIRHAM-simulated Indian summer monsoon circulation. Adv. Meteorol. 2010:415632. doi: 10.1155/2010/415632
Prasch, M., Mauser, W., and Weber, M. (2013). Quantifying present and future glacier melt-water contribution to runoff in a central Himalayan river basin. Cryosphere 7, 889–904. doi: 10.5194/tc-7-889-2013
Rasmussen, R., Baker, B., Kochendorfer, J., Meyers, T., Landolt, S., Fischer, A. P., et al. (2012). How well are we measuring snow: the NOAA/FAA/NCAR winter precipitation test bed. Bull. Am. Meteorol. Soc. 93, 811–829. doi: 10.1175/BAMS-D-11-00052.1
Rohrer, M., Salzmann, N., Stoffel, M., and Kulkarni, A. V. (2013). Missing (in-situ) snow cover data hampers climate change and runoff studies in the Greater Himalayas. Sci. Total Environ. 468, S60–S70. doi: 10.1016/j.scitotenv.2013.09.056
Sabin, T. P., Raghavan, K., Ghattas, J., Denvil, S., Dufresne, J.-L., Hourdin, F., et al. (2013). High resolution simulation of the South Asian monsoon using a variable resolution global climate model. Clim. Dyn. 41, 173–194. doi: 10.1007/s00382-012-1658-8
Saloranta, T., Litt, M., and Melvold, K. (2016). Measuring and Modelling Snow Cover and Melt in a Himalayan Catchment: Instrumentation and model code setup in the Langtang catchment, Nepal. Lessons learned from the SnowAMP Project, nve Working Paper 2016/10. Kathmandu: International Centre for Integrated Mountain Development.
Saloranta, T., Thapa, A., Kirkham, J. D., Koch, I., Stigter, E. E., Melvold, K., et al. (2019). A model setup for mapping snow conditions in High-Mountain Himalaya. Front. Earth Sci. 7:129. doi: 10.3389/feart.2019.00129
Saloranta, T. M. (2012). Simulating snow maps for Norway: description and statistical evaluation of the seNorge snow model. Cryosphere 6, 1323–1337. doi: 10.5194/tc-6-1323-2012
Schleef, S., Löwe, H., and Schneebeli, M. (2014). Influence of stress, temperature and crystal morphology on isothermal densification and specific surface area decrease of new snow. Cryosphere 8, 1825–1838. doi: 10.5194/tc-8-1825-2014
Serreze, M. C., Clark, M. P., Armstrong, R. L., McGinnis, D. A., and Pulwarty, R. S. (1999). Characteristics of the western United States snowpack from snowpack telemetry (SNOTEL) data. Water Res. Res. 35, 2145–2160. doi: 10.1029/1999WR900090
Sevruk, B., Hertig, J. A., and Spiess, R. (1991). The effect of a precipitation gauge orifice rim on the wind field deformation as investigated in a wind tunnel. Atmos. Environ. Part A Gen. Top. 25, 1173–1179. doi: 10.1016/0960-1686(91)90228-Y
Sexstone, G. A., and Fassnacht, S. R. (2014). What drives basin scale spatial variability of snowpack properties in northern Colorado? Cryosphere 8, 329–344. doi: 10.5194/tc-8-329-2014
Shea, J. M., Wagnon, P., Immerzeel, W. W., Biron, R., Brun, F., and Pellicciotti, F. (2015). A comparative high-altitude meteorological analysis from three catchments in the Nepalese Himalaya. Int. J. Water Resour. Dev. 31, 174–200. doi: 10.1080/07900627.2015.1020417
Sieck, L. C., Burges, S. J., and Steiner, M. (2007). Challenges in obtaining reliable measurements of point rainfall. Water Resour. Res. 43:W01420. doi: 10.1029/2005WR004519
Singh, P., and Bengtsson, L. (2005). Impact of warmer climate on melt and evaporation for the rainfed, snowfed and glacierfed basins in the Himalayan region. J. Hydrol. 300, 140–154. doi: 10.1016/j.jhydrol.2004.06.005
Singh, P., and Kumar, N. (1997). Impact assessment of climate change on the hydrological response of a snow and glacier melt runoff dominated Himalayan river. J. Hydrol. 193, 316–350. doi: 10.1016/S0022-1694(96)03142-3
Smith, T., and Bookhagen, B. (2016). Assessing uncertainty and sensor biases in passive microwave data across High Mountain Asia. Remote Sens. Environ. 181, 174–185. doi: 10.1016/j.rse.2016.03.037
Smith, T., and Bookhagen, B. (2018). Changes in seasonal snow water equivalent distribution in High Mountain Asia (1987 to 2009). Sci. Adv. 4:e1701550. doi: 10.1126/sciadv.1701550
Smith, T., Bookhagen, B., and Rheinwalt, A. (2017). Spatiotemporal patterns of High Mountain Asia’s snowmelt season identified with an automated snowmelt detection algorithm, 1987–2016. Cryosphere 11, 2329–2343. doi: 10.5194/tc-11-2329-2017
Smith, C. D., Kontu, A., Laffin, R., and Pomeroy, J. W. (2017). An assessment of two automated snow water equivalent instruments during the WMO solid precipitation intercomparison experiment. Cryosphere 11, 101–116. doi: 10.5194/tc-11-101-2017
Stewart, I. T. (2009). Changes in snowpack and snowmelt runoff for key mountain regions. Hydrol. Process. 23, 78–94. doi: 10.1002/hyp.7128
Stigter, E. E., Litt, M., Steiner, J. F., Bonekamp, P. N. J., Shea, J. M., Bierkens, M. F. P., et al. (2018). The importance of snow sublimation on a Himalayan Glacier. Front. Earth Sci. 6:108. doi: 10.3389/feart.2018.00108
Stigter, E. E., Wanders, N., Saloranta, T. M., Shea, J. M., Bierkens, M. F., and Immerzeel, W. W. (2017). Assimilation of snow cover and snow depth into a snow model to estimate snow water equivalent and snowmelt runoff in a Himalayan catchment. Cryosphere 11, 1647–1664. doi: 10.5194/tc-11-1647-2017
Stranden, H. B., Ree, B. L., and Møen, K. M. (2015). Recommendations for Automatic Measurements of Snow Water Equivalent in NVE. Majorstua: Norges vassdrags- og energidirektorat.
Strasser, U., Bernhardt, M., Weber, M., Liston, G. E., and Mauser, W. (2008). Is sublimation important for alpine water balance? Cryosphere 2, 53–66. doi: 10.5194/tc-2-53-2008
Sturm, M., and Holmgren, J. (1998). Differences in compaction behavior of three climate classes of snow. Ann. Glaciol. 26, 125–130. doi: 10.1017/S0260305500014683
Sturm, M., Taras, B., Liston, G. E., Derksen, C., Jonas, T., and Lea, J. (2010). Estimating snow water equivalent using snow depth data and climate classes. J. Hydrometeorol. 11, 1380–1394. doi: 10.1175/2010JHM1202.1
Sutron (2015). Total Precip Gauge (TPG), TPG-0001-1, TPG-0003-1 Operations & Maintenance Manual. Available at: http://www.sutron.com/product/total-precipitation-gauge-tpg/ (accessed March 2011).
Takala, M., Luojus, K., Pulliainen, J., Derksen, C., Lemmetyinen, J., Karna, J. P., et al. (2011). Estimating northern hemisphere snow water equivalent for climate research through assimilation of space-borne radiometer data and groundbased measurements. Remote Sens. Environ. 115, 3517–3529. doi: 10.1016/j.rse.2011.08.014
Tiwari, S., Kar, S. C., and Bhatla, R. (2015). Snowfall and snowmelt variability over Himalayan region in inter-annual timescale. Aquat. Preced. 4, 942–949. doi: 10.1016/j.aqpro.2015.02.118
Tiwari, S., Kar, S. C., and Bhatla, R. (2016). Examination of snowmelt over Western Himalayas using remote sensing data. Theor. Appl. Climatol. 125, 227–239. doi: 10.1007/s00704-015-1506-y
Wagnon, P., Vincent, C., Arnaud, Y., Berthier, E., Vuillermoz, E., Gruber, S., et al. (2013). Seasonal and annual mass balances of Mera and Pokalde glaciers (Nepal Himalaya) since 2007. Cryosphere 7, 1769–1786. doi: 10.5194/tc-7-1769-2013
Wolff, M., Isaksen, K., Brækkan, R., Alfnes, E., Petersen-Øverleir, A., and Ruud, E. (2013). Measurements of wind-induced loss of solid precipitation: description of a Norwegian field study. Hydrol. Res. 44, 35–43. doi: 10.2166/nh.2012.166
Wolff, M. A., Isaksen, K., Petersen-Øverleir, A., Ødemark, K., Reitan, T., and Brækkan, R. (2015). Derivation of a new continuous adjustment function for correcting wind-induced loss of solid precipitation: results of a Norwegian field study. Hydrol. Earth Syst. Sci. 19, 951–967. doi: 10.5194/hess-19-951-2015
Wright, M. (2011). Performance Analysis of CS725 Snow Water Equivalent Sensor. Edmonton, AB: Campbell Scientific Corp.
Wright, M. (2013). CS725 Frozen Potential: The Ability to Predict Snow Water Equivalent is Essential. Edmonton, AB: Campbell Scientific Corp.
Yang, D., Goodison, B. E., Metcalfe, J. R., Golubev, V. S., Bates, R., Pangburn, T., et al. (1998). Accuracy of NWS 8” standard nonrecording precipitation gauge: results and application of WMO Intercomparison. J. Atmos. Ocean. Technol. 15, 54–68. doi: 10.1175/1520-0426(1998)015<0054:aonsnp>2.0.co;2
Ye, B., Yang, D., Ding, Y., Han, T., and Koike, T. (2004). A bias-corrected precipitation climatology for China. J. Hydrometeorol. 5, 1147–1160. doi: 10.1175/JHM-366.1
Keywords: snow water equivalent, high altitude, Himalaya, near real time, gamma radiation, snow
Citation: Kirkham JD, Koch I, Saloranta TM, Litt M, Stigter EE, Møen K, Thapa A, Melvold K and Immerzeel WW (2019) Near Real-Time Measurement of Snow Water Equivalent in the Nepal Himalayas. Front. Earth Sci. 7:177. doi: 10.3389/feart.2019.00177
Received: 23 January 2019; Accepted: 21 June 2019;
Published: 16 July 2019.
Edited by:
Anthony Arendt, University of Washington, United StatesReviewed by:
Ward van Pelt, Uppsala University, SwedenSteven Margulis, University of California, Los Angeles, United States
Copyright © 2019 Kirkham, Koch, Saloranta, Litt, Stigter, Møen, Thapa, Melvold and Immerzeel. This is an open-access article distributed under the terms of the Creative Commons Attribution License (CC BY). The use, distribution or reproduction in other forums is permitted, provided the original author(s) and the copyright owner(s) are credited and that the original publication in this journal is cited, in accordance with accepted academic practice. No use, distribution or reproduction is permitted which does not comply with these terms.
*Correspondence: James D. Kirkham, ams2NzVAY2FtLmFjLnVr