- 1Research Institute for Geo-Hydrological Protection, Consiglio Nazionale Delle Ricerche, Bari, Italy
- 2Institute of Geography, Romanian Academy, Bucharest, Romania
- 3Department of Biosciences and Territory, University of Molise, Isernia, Italy
- 4Environmental Geography Group, Institute for Environmental Studies (IVM), VU University Amsterdam, Amsterdam, Netherlands
- 5Department of Environmental Science, University of Naples Parthenope, Naples, Italy
- 6Department of Earth and Environmental Sciences, University Aldo Moro, Bari, Italy
A high dynamic environment is typically interested by changes affecting the natural processes and their related consequences. Landslides do not only alter the landscape, but substantially affect human activities. When it comes to natural hazards, landslides have been acknowledged as one of the main causes of human casualties or damage to assets. Furthermore, economic losses to rural lands are also significant, despite often being underrated, especially in rural areas. In not densely populated territories, the main productive activities are in fact often based on the agricultural and pastoral resources. We intend to propose a methodology that helps to investigate the potential loss of value (expressed in €) of lands usually exploited for economical profit in rural areas. We test the method on two case studies, belonging to different European Countries with very different economical assets and geological, geomorphological, and environmental conditions. The first study area is located in the Southern Italian Apennines, in the Molise region, while the second area is located in Buzau County, a region belonging to the Romanian Curvature Carpathians and Subcarpathians. Our analysis is focused not only on the actual situation, represented by the past and present landslides, but also on potential future scenarios for 2050. The scenarios foresee future similar socio-economical and technological activities, with no major changes expected. The loss estimation is based on the presence of landslides affecting the rural lands, but it also considers both a present and future landslide susceptibility scenario. This procedure allowed the estimation of the economic losses in the two case-study areas, highlighting how the same natural processes might result in different economical consequences. Following our approach, the results highlight that for the Italian case study there is a loss of 10.4% for 2007 and 9.9% for 2050 of the total land value as concerns landslides susceptibility. In the Romanian case study, on the other hand, the loss corresponds to 29.6 and 29.8% for 2010 and 2050, respectively. In addition, the proposed procedure could be considered a valuable methodological approach to assess landslide-induced economic losses, and be effectively used during spatial planning activities, aimed at supporting decision makers for a more sustainable land management.
Introduction
Natural hazards, including landslides, cause severe damage to a large variety of assets (meaning structural, infrastructural, and social goods), determining losses on population activities, natural, and human environment all over the world (Zêzere et al., 2008; Petley, 2012; Promper and Glade, 2016; Salvati et al., 2018).
There is an increasing interest on studying how anthropogenic pressure on the ecosystems can determine changes in the spatial distribution of landslides (Reichenbach et al., 2014; Malek et al., 2015; Schmaltz et al., 2017; Gariano et al., 2018). It is also important to underline that changes in the socio-economical structure are going to affect also the number and the type of assets exposed to natural hazards and risks, including landslides (Pisano et al., 2017a). The interactions among exposed assets, landslides, and economic analysis of the occurred damage have therefore become object of several studies since a few decades (i.e., Schuster, 1996; Godt et al., 2000).
Estimating consequences of landslides in terms of direct and indirect damage (Fleming and Taylor, 1980; Schuster and Fleming, 1986) is imperative in the framework of reliable risk assessment and management strategies.
When dealing with natural disasters, direct and indirect losses have to be distinguished. According to the United Nations Office for Disaster Risk Reduction (UNISDR): “Direct losses refer to damage to human lives, buildings, infrastructure, and natural resources. Indirect disaster losses are declines in output or revenue, as a consequence of direct losses or owing to impacts on a supply chain” (GAR, 2015). Other types of costs are intangible costs, business interruption costs, and risk mitigation costs, as defined in Kreibich et al. (2014). The damage observed, and the related costs, may be also very different to quantify depending upon the type of natural hazards (Parise et al., 2013; Vennari et al., 2015, 2016; Salvati et al., 2018).
Furthermore, there are generally two ways to assess costs of natural hazards, namely “ex-post” are those estimates after the event, meanwhile with “ex-ante” can be named those estimated taking into account the likely future hazard occurrence. Following Donnini et al. (2017) the first is the approach most commonly addressed in the scientific literature, because it provides information relevant to the stakeholders or planners related to the compensation and economic support.
When a natural hazard occurs, there can be a great variety of elements at risk affected, but commonly the focus is on buildings and public infrastructure such as roads, railroads, and other communication routes (Alexander, 1986; Chiocchio et al., 1997; Iovine and Parise, 2002; Calcaterra et al., 2008; Calò et al., 2012; Del Soldato et al., 2017). However, when it comes to rural areas the main economic resources are strictly tied to the exploitation of agricultural, pastoral, and natural resources, therefore land damage represents a serious threat to the socio-economic development. In this case, the landslide direct damage can be identified as the partial or complete loss of agricultural production and of soil productivity in time, and/or the reduction in land value.
Indirect damages caused by landslides, on the other hand, are related to the reduction of functions derived by rural activities normally taking place in the affected land (for example harvesting, plowing, pasture), determining also the reduction in the normally produced public services.
It is quite common that the losses to rural land, caused by activity of slope movements, tend to be underrated, due to the fact that they do not constitute a practical risk to personal safety. For instance, at present there is quite relevant scientific literature available concerning loss or damage of rural lands due to natural hazards such as drought (IPCC, 2012; WMO, 2014; Lesk et al., 2016) or floods (Ward et al., 2013; Tanoue et al., 2016), but almost anything about landslides to the best of our knowledge.
Economic damage produced by soil erosion and landslide phenomena in rural environments, together with the lack of methodological approaches to assess landslide-induced damage, makes difficult to take decisions concerning land management, or to plan and develop actions to prevent and mitigate such damage. This amplifies the need to develop methods potentially contributing to a more precise and rapid evaluation of the likely economic consequences.
In this work we propose a methodology that helps to determine the loss of value suffered by those land parcel types which are exploited for economical profit in rural areas. The method is a multi-disciplinary approach, based on the integration of GIS applications, effective and easy to use because it does not require a large amount of data. To test its robustness we applied it to two different case studies, characterized by different socio-economical environments, geological and geomorphological settings, and data availability and resolution as well.
In addition, considering that future damage is often considered the essential basis of risk reduction strategies and preparedness planning in any risk analysis (Van Westen et al., 2006; Vranken et al., 2013), we further analyse the expected losses for both case studies, according to a possible Business As Usual (BAU) scenario for year 2050.
Study Areas
The study is focused on two areas located in different European Countries. The first one is the Rivo catchment in the Molise region of the Italian Southern Apennine, while the second is placed in the Curvature sector of the Romanian Carpathians and corresponds to the upper sector of the Bălăneasa catchment. Selection of these two areas lies in the fact that they are representative for the rural environment in the respective countries. In addition, they were selected also on account of the fact that we wanted to apply the methodology in two quite distinct settings from both the environmental and the socio-economical standpoints.
Rivo Catchment
The Rivo catchment study area covers an area of 82 km2 and is located along the Adriatic flank of Molise region (Southern Italy). It is a small hilly to low mountainous basin which is part of the larger valley system of the Trigno River, one of the major water courses in the region. The area is characterized by altitudes ranging from ca. 230 to 980 m above sea level. From a geological standpoint the outcropping geological formations consist mainly of marly-arenaceous and clayey deposits (from Upper Oligocene to Middle Miocene), with subordinate limestone and Middle-Lower Miocene bio-calcilutites, as reported in the sheet 393 “Trivento” of the geological map (Sgrosso and Naso, 2012).
Slope modeling processes, such as landslides and deep gully erosion, take place extensively on steep slopes modeled on clayey lithologies (mainly varicolored scaly clays; Aucelli et al., 2010).
Landslides belong to the categories of earthflows and complex earthslide-earthflows, and are primarily triggered by rainfall (Rosskopf and Aucelli, 2007; Borgomeo et al., 2014). Most of the phenomena are dormant and relatively superficial (depth ≤ 4 m); they are typically found along fluvial slopes directly bordered by stream incisions, and thus severely affected by river undercutting (Aucelli et al., 2001, 2004).
The area lies within a prevalently rural context with low population density. The prevailing land use is represented by crops (55.4%), forest lands (32.87%), and pasture (4.7%). The most common population sustenance methods are agricultural, pastoral, and crafting activities, and small enterprises.
Bălăneasa Catchment
The second study area is situated in the Curvature sector of the Romanian Carpathians, at the limit between the Buzău Carpathians and Subcarpathians (see Figure 1), an area considered representative for the two main morpho-structural units in respect to the landslide typology—land cover categories relationship. Overlaying the upper catchment of Bălăneasa River (main tributary of Buzau), the area extends from 1,100 m to 300 m a.s.l., and can be divided in two almost equal sub-sectors: an upper mountainous zone, and a lower hilly zone. The northern mountainous area (Buzău Carpathians) features low-altitude summits (1,000–800 m a.s.l.) and elongated, NE-SW-oriented, smooth ridges alternating with large valleys carved in Mid-Upper Palaeogene flysch deposits (Eocene schistose sandstone flysch, Oligocene sandstone flysch with schistose intercalations, bitumen and conglomerates). The lithological features, together with the highly folded and faulted structures, constitute the main predisposing factors of the active morphodynamics. The slopes are modeled by large, deep-seated landslides (debris/rock slumps, rock block slides), triggered by snowmelt or long-lasting rains, with a high reactivation potential, especially in the form of shallow and medium-seated earth/debris slides. The southern hilly sector corresponding to the Buzău Subcarpathians consists of an alternation of low-to-high rounded hills with enlarged valleys as a result of differentiated erosion exerted on the different-consistence molasse deposits of Low Neogene age (Miocene schistose clays and schists, alternating with thin sandstone, gypsum or conglomerate layers). Developed between 800 and 300 m a.s.l., the Subcarpathian sector is marked by intense landslide activity, conditioned by the lithology and enhanced by human activity (road cuts). The landslide typology consists of shallow and medium-seated earth/debris slides and numerous earthflows, triggered by early spring showers or torrential summer rains. Throughout both sectors, slope processes are strongly correlated with the river network, both at the level of large valleys and smaller tributaries, so that in numerous cases landslide deposits cause river blockages and temporary landslide dams. The human activities are leaving strong imprints on the landscape. Even if it is a rural area, social, and political-economical frameworks marked changes in the land-cover patterns. Partial collective agriculture until 1990, followed by a transition period and by Romania's EU joining procedures (2007) induced different management procedures, in which recent deforestations, property fragmentation or abandonment strongly influenced the correlation between slope processes (landslides and erosion) and land coverage/usage (Micu, 2008). The broad-leaved forests are covering the majority of the land in the Carpathian sector, being replaced in the Subcarpathians by orchards and hayfields. The settlements are usually clustered along valleys, while seldom households could be encountered scattered across the upper slopes and used mainly during the harvest periods (of both fruits and hay). The subsistence agriculture prevails, while arable lands are limited in extension and used mainly for maize or potato crops.
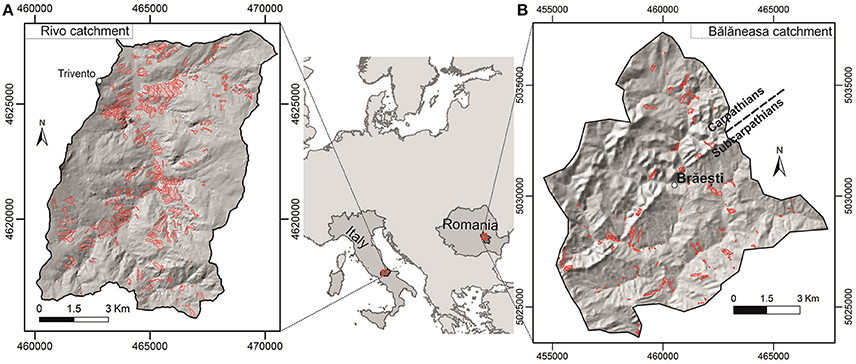
Figure 1. General overview of the study areas locations (in A Rivo catchment; in B Balaneasa catchment) and landslides bodies (reported in red) used for the analysis.
Data Preparation and Methods
Estimation of the damage suffered by rural lands lying on slopes prone to landslides is built through the interaction of two features, namely: (i) land use/cover type and associated economic value, and (ii) landslide susceptibility. These two are overlapped by using GIS software in order to obtain the potential degree of loss suffered by rural and agricultural lands due to different levels of landslides susceptibility. To this aim, we completed the landslide susceptibility analyses for the two case studies, and collected information related to the average land values of forested areas, pastures, cultivated land, bareland, and shrubland for both the case studies. Landslides susceptibility classes and land values were eventually intersected by means of a matrix that, after the application of specific indicators, provided a net land value reduced by the estimated loss, called the “real” value.
This section illustrates the data and the methods used to perform the analysis, whilst land use data and the future 2050 scenario were developed by Pisano et al. (2017b,c). Here the output related to the 2007 land cover, and the so-called 2050 trend scenarios, together with the associated susceptibility, were used as input data in order to calculate the potential loss of economic value of the rural lands due to susceptibility. For the Bălăneasa catchment case study, we performed the land cover scenario and the associated susceptibility (for years 2010 and 2050) by adopting the same methodology used for the Rivo catchment. In the following sections we present data and methods related, in both case studies, to the land cover and landslide susceptibility, the economic value of the rural lands and its loss due to landslide susceptibility, an overall overview of the methodology adopted is given in the flowchart provided as the Supplementary Materials (Supplementary Figure 1).
Land Cover and Land Cover Scenarios
Land cover maps were designed in the framework of a multi-temporal analysis aimed at evaluating land cover changes in a 50 year period (Pisano et al., 2017b,c). The multi-temporal land cover mapping was performed in GIS environment by using ortho-rectified and co-registered aerial photos. The aerial image interpretation was performed using a scale of 1:5,000, organized in the following seven classes: settlements, cultivated area, forested land, pasture, shrubland, wetland, and bareland.
In these works, we considered only those land cover classes exploited as agricultural, pastoral, and natural resources in less populated territories where rural activities are the main source of income. In detail, they are forested areas, pastures, cultivated land, bareland, and shrubland.
For the Bălăneasa case study we used the land cover maps developed by Malek et al. (2014) starting by classifying the forest cover through Landsat images for the years 1989, 2000, and 2010. To map settlements and cropland, we combined the Landsat data with most recent high resolution Google Earth images. More detailed topographic maps and spatial plans obtained from local authorities were also used to help with manual digitization of settlements and cropland. The final land cover map had a 89% overall accuracy. More information on the land cover classification and the underlying data are available in Malek et al. (2014).
Regarding the scenario modeling, we projected future land use changes in both study areas for the year 2050 by simulating future changes based on the observed historical trends, which we assumed to continue in the future. To spatially allocate future land cover change, we developed a model using Dinamica EGO. Dinamica EGO is a GIS, particularly suitable for spatial land cover change simulation on a high spatial and temporal resolution (Soares-Filho et al., 2002).
The demands of future land cover were based on observed trends. In this way, we simulated how the future land cover might look like if socio-economic conditions, current agricultural, and forestry practices, as well as spatial planning will continue with the business as usual (BAU) scenario. In both areas, we however modified the observed trends, to allow the continuation of the most significant processes. For example, forest expansion was observed as the prevailing process in the landscape. To allow the continuation of the forest expansion trends, we therefore had to modify the change rates of other processes, that occurred on a smaller spatial extent. This did not influence the final results, as we only limited cropland and urban expansion change rates to exclude expansion on the account of forests. Moreover, agricultural abandonment and subsequent overgrowth have been identified as a major land use process in European mountain areas (van Vliet et al., 2015), a trend likely to continue.
The trend scenarios were different for the two areas: whereas the Italian area witnessed cropland and urban expansion, that in Romania was characterized by forest expansion and to some extent small scale deforestation, as indicated by forest management trends at the regional level (Malek et al., 2015, 2018).
In future scenarios particular land cover types, such as forest or cropland, are in our study areas more likely to be found near or adjacent to existing forests or croplands. We therefore mostly modeled expansion of particular land cover, instead of forming new isolated patches in a landscape. We still allowed the formation of new patches of specific land cover, based on the observed size and variation of observed past land cover changes. In this way, we aimed to capture more realistic spatial patterns of future land cover change specifically for each of the study areas.
Landslide Susceptibility and Associated Scenario
To quantify the potential loss of value of rural lands due to landslides we considered the landslide susceptibility of the two analyzed case studies, starting from the landslide susceptibility analysis already available for the Rivo catchment (Pisano et al., 2017c). We carried out the susceptibility using the Spatial Multi Criteria Evaluation (SMCE) (Alkema, 2007; Boerboom and Alan, 2012; Pourghasemi et al., 2014) in GIS environment using ILWIS software (ILWIS™ Academic 3.3, 2012)1 and taking into account seven predisposing factors (slope angle, slope aspect, altitude, internal relief, land cover, soils, and ancient landslide deposits), for which the frequency ratio (FR) was calculated (Pradhan and Lee, 2010; Yalcin et al., 2011; Persichillo et al., 2017). FR was calculated exploiting the landslide dataset points extracted in the source zone, in order to represent the probability of occurrence from the initiation area. To the variables were assigned weights representing their relative importance; namely, we gave the highest weights to slope declivity and land cover (0.19), followed by slope orientation, large old landslide deposits (0.14), internal relief (0.13), soil (0.11), and altitude (0.09). The dataset was divided in two parts, allowing to have two independent dataset, one of which representing the 10% of the total to be used for validation. This latter was operated applying the receiver operating characteristic (ROC) curve (Pisano et al., 2017c).
In the SMCE the FR was used to relate the factor classes weights to the landslide density standardized using the maximum linear function; meanwhile the factors standardization was performed using the direct method based on expert judgment (Pisano et al., 2017c). The obtained map (Figure 2) for 2007 has been re-classified using five intervals from very low to very high, and showed a ROC curve of 0.814 (Pisano et al., 2017c). The map identifies the highest susceptibility in the central areas in correspondence of the most clayey formations, i.e., Varicoloured Scaly Clays, that showed high Frequency Ratio values (Pisano et al., 2017c), and is known to be highly affected by landslides in the Southern Apennines of Italy (Fiorillo et al., 1996; Parise and Wasowski, 1999; Rosskopf and Aucelli, 2007).
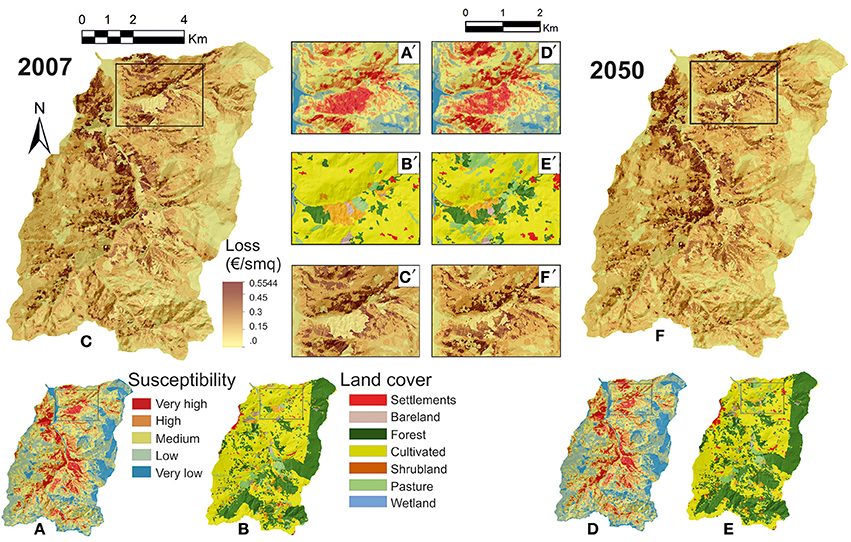
Figure 2. Rivo catchment landslide susceptibility (A), land cover (B), and damage (C) maps for 2007 and 2050 (respectively, D–F). In the zoomed squares (A′-C′ for 2007 and D′-F′ for 2050) some sectors of the related maps are highlighted.
Following the same approach, we carried out the susceptibility analysis for the Bălăneasa catchment. Based on a wide knowledge of the geological and geomorphological characters and of the main hydro-geological processes occurring in the area, we prepared the conditional factors maps useful for the SMCE method application. Further, in doing so, several previous studies focusing on landslides occurrence and spatial distribution in proximal areas were considered in the selection of the conditional factors (i.e., Damen et al., 2014; Zumpano et al., 2014; Hussin et al., 2016). Therefore, we sorted out the following parameters: slope declivity, slope aspect, altitude, internal relief, land cover, soil, and ancient landslides deposits.
The geomorphological parameters were derived in GIS environment from a DEM (25 m cell size) extracted from a topographical map produced by the Military Topographic Direction (scale 1:25,000); the soil map (scale 1:100,000) was available from the Institute of Geography Archives, and the land cover map was derived from digital aerial photo interpretation. We decided to take in consideration also old large landslides areas among the conditional factors, due to the fact that in the region very often new landslides develop within old, large, landslide bodies (Micu et al., 2014). Old landslides areas were extracted from archive inventory data from the Institute of Geography of the Romanian Academy, and from previous studies (Damen et al., 2014). For the SMCE, guided by the tool available in ILWIS software, we standardized the factors using the expert judgment and conversely to what was done before for the Rivo catchment, we weighted the factors classes by integrating the FR values with the expert judgment. This was given by the fact that a preliminary analysis carried out using only the FR values turned out in unreliable results, probably because the landslides database is compiled starting from civil protection reports and field activity, resulting in a non-uniform dataset, partially incomplete, across the area.
The validity of the analysis' performance was evaluated by using the ROC curve, which for this map showed a value of 0.811. Finally, we run the susceptibility analysis scenario adopting the land cover 2050 scenario among the input factor replacing the land cover 2010 in both models. We maintained the same factors weights and the same standardization approach, the resulting map being presented in Figure 3.
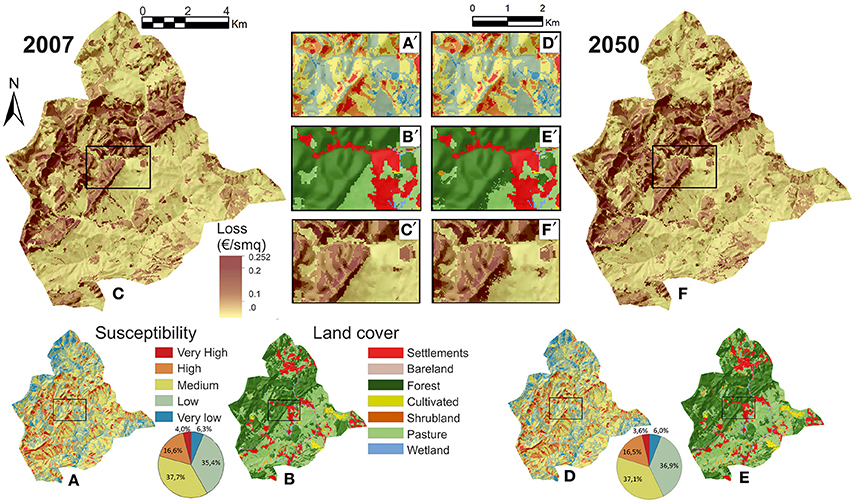
Figure 3. Bălăneasa catchment landslide susceptibility (A), land cover (B), and damage (C) maps for 2010 and 2050 (respectively, D–F). In the zoomed squares (A′-C′ for 2010, and D′-F′ for 2050) some sectors of the related maps are highlighted. The pie charts represent the percentage area of the susceptibility classes related to the susceptibility maps (A,D).
Land Value and Estimated Economic Loss
The second phase of the data preparation focused on the collection of the information related to the economic land values for the two case studies.
The average land values for the Rivo catchment were extracted from the regional dataset available at the Italian Income Revenue Agency of the Ministry of the Economy and Finance website (http://wwwt.agenziaentrate.gov.it/mt/Osservatorio%20immobiliare/valori_agricoli_medi/molise/CB_2007.pdf). The dataset organized for regions provides values in €/ha at the provincial level for all the agricultural land types falling within the province. As reported in the website, the numbers represent the weighted averages of agricultural values within the region based on the indemnity to be paid to the owner of an agricultural land expropriated for public use, according to the actual cultivation practiced. From the database we collected the values for the Campobasso province and correlated them to the Rivo land cover classes, afterward we transformed the values to €/m2 (Table 1).
For the Bălăneasa catchment the data related to the land values were collected through an accurate survey of both the formal legal documents recommending values and procedures of selling/buying land parcels according to the Romanian law (LG 17/2014), but also taking into account informal transactions documents. This duplex approach was demanded by the fact that in terms of land value based on its coverage/usage, there are major differences between informal (suggested benchmarks) and official transaction values. Prices vary a lot when taking into account the relief (morphometry, accessibility) and the economic and social requirements (village location inside different relief units, imposing different requirements for agricultural land). In addition, they depend also on its quality as a reflection of relief units and settlements' presence relationship, but also on the size of the land parcels, since in the study area there are not many properties exceeding 5-10 ha in surface. For this application we decided to take into consideration an average of the prices provided as guides for the real-estate market by the Romanian National Association of Authorized Evaluators (Table 2); however, one should take in consideration this ambiguity when looking at the value loss. Finally, values were converted into €/m2, and correlated to the land cover classes object of interest in this study.
For both case studies we decided to use also the present economic values for the future land cover scenario. This was due to the fact that considering forecasted economic variables to predict future prices for the scenarios would have made the calculation very complex and difficult to manage, in addition producing final outcomes difficult to be compared (Mela et al., 2012).
To establish the economic damage in terms of loss value we decided to set up a rapid and feasible approach based on three parameters, namely the average land value in €/m2 (LV), the susceptibility class, ranging from very low to very high (S), and a specific land cover damage index (DI), according to the formula:
The first parameter (S) was ranked ascribing, based on expert judgment, proportionally higher values from 0 to 0.9 to the five susceptibility classes (from very low to very high; Table 3). We decided not to use 1 for the highest value, given the fact that we are estimating here a probability or proneness, thus not having the certainty of the landslide occurrence. For the DI parameter we have attributed qualitatively a value from 0 to 1 based on a qualitative estimation conceived to represent the resilience of the land parcel to reconstitute its potential use after a landslide event.
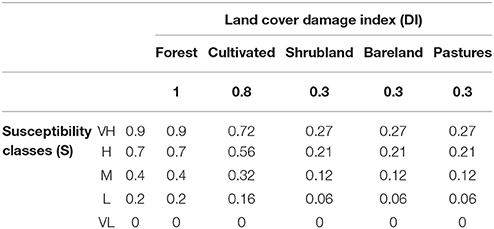
Table 3. Matrix used to correlate the land cover damage index (DI) and the susceptibility classes indexes (S).
We choose to combine the susceptibility classes (S) and the landslide-damage index (DI) by multiplying them in a matrix (Table 3) from which we obtained a value that, multiplied to the land value (LV), gives the value loss (LOSS).
Finally we calculated also a net land value that considers the estimated loss, which can be intended as the real economic land value.
The matrix was used for both present and future scenarios, and the final results were compared in order to examine how landslide susceptibility affects, and might affect in the future, the land values.
Further, with our application we investigate the extent of this potential loss considering future land cover changes anticipated by a business as usual (BAU) scenario in the two considered European Countries, highlighting how the same natural process might result in quite different economic consequences.
Results
To quantify the changes in the economic values for the land cover categories considered relevant for the rural economic activity of the study areas, we started to evaluate the variations in the total land value exclusively due to land cover changes for the period 2007–2050 in the Rivo catchment, and 2010–2050 for Balaneasa (section Variations in the Land Values Due to Land Cover Changes and Scenario); afterwards, we present the results obtained for the estimated loss due to susceptibility (section Landslide Susceptibility and Scenario) for the past situation and for the future 2050 scenario. Eventually, we compare the loss in the two case studies considering the respective land cover, the differences in the land prices, the degree of landslide susceptibility and the scenarios.
Variations in the Land Values Due to Land Cover Changes and Scenario
By means of the application of the proposed method implemented in GIS, we observed the changes in the total land values by comparing the actual and the future 2050 extent for each class in the Rivo and the Bălăneasa catchments (Tables 4, 5).
As shown in the tables, there is a difference in the associated values for the classes for both case studies. For the Rivo area some classes such as cultivated, shrub and bareland are decreasing their extent (Pisano et al., 2017c). Due to the fact that cultivated land has the highest value, its variation pays the highest contribution to the value of the total area. This loss is partially balanced primarily by the increase of forest extension (see Table 4). As a consequence, there is a decrease in the total rural land value of ~2 Mln€ (representing 3.6%, with respect to the initial value).
In the Bălăneasa catchment, the forest expansion due to abandonment of cropland and pastures was identified as the prevailing process. In this area the changes are not so straightforward, and forest and shrubland expansion are about 2% of the total area. On the other hand, pasture is the class showing the most significant decrease. The highest forest value with respect to the other classes determines an increase of the total land value of around 290,000 €, corresponding to 2% of the total land value.
Landslide Susceptibility and Scenario
The values related to each land cover class, presented in the previous section, must be considered as gross values, given that they do not take into any consideration the possible land depreciation given by landslides spatial probability of occurrence that might affect them. For this reason we used the landslide susceptibility organized in five classes, interpolated with the land cover maps to understand the degree of loss that can affect the rural land. This procedure was applied by assigning also a coefficient that considers the resilience of the land according to its use type.
The results carried out for the present and for future scenario are presented in Figures 2, 3.
Landslide susceptibility in the Rivo catchment, as described in Pisano et al. (2017c), shows a decrease in the highest susceptibility classes, primarily linked to the transformation of pasture, bareland, and shrubland into forest and cultivated area. In fact, the highest susceptibility classes vary from 26.8% in 2007 to 25.2% in 2050.
As a first approach, we estimated the loss due to susceptibility in the year 2007. By following the approach described in section Land Value and Estimated Economic Loss and summarized with the matrix (Table 3) we obtained the loss of land value for the examined areas, as displayed in Figures 2, 4.
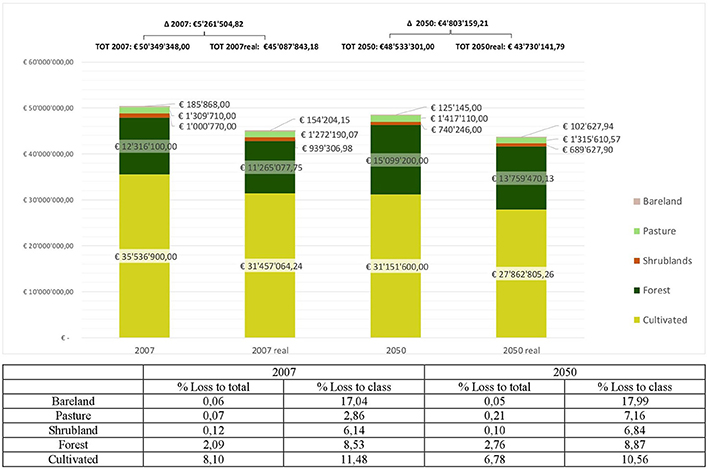
Figure 4. Histogram of the Rivo land monetary values for 2007 and 2050, differentiated between the official standard values and those diminished by the interaction with landslide susceptibility (real). In the bottom table the loss percentages with respect to the total area (% Loss to the total), and to the class official value (% Loss to the class) are listed.
In the Rivo catchment the loss values for 2007 and 2050 have been analyzed. These range from a minimum of 0, where the susceptibility is very low, to a maximum of 0.55 €/m2. The highest loss is concentrated where the susceptibility is the highest and corresponds to the cultivated land cover class, that is indeed the most valuable. The zoomed areas reported in the figure highlight how the land cover changes according to our method play a major control on the susceptibility spatial distribution and intensity, and consequently the land price.
The results obtained show how the most affected areas are placed in the central-western sector where there is the higher landslide susceptibility. Information related to each land cover class values changes are reported in Figure 4. The histograms in Figures 4, 5 indicate for both case studies a quantification of the loss of value for land cover types after considering the susceptibility for the present and the scenario (2007/2010 real and 2050 real).
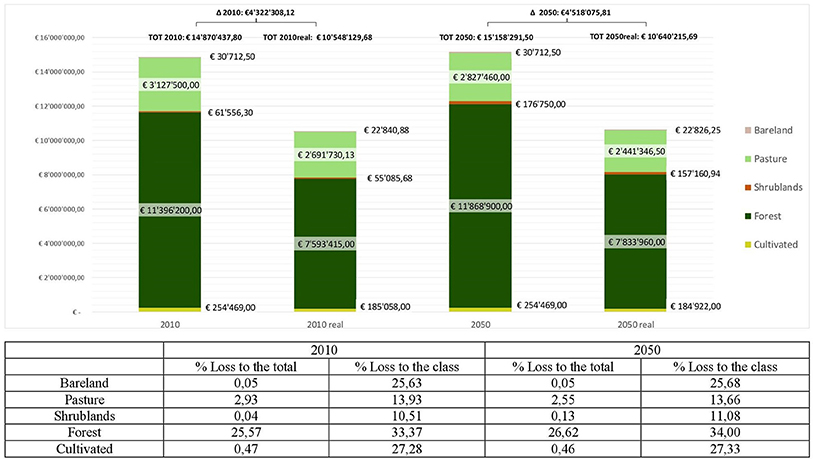
Figure 5. Histogram of the Bălăneasa land monetary values for 2010 and 2050 differentiated between the official standard values and those diminished by the interaction with landslide susceptibility (real). In the bottom table the loss percentages with respect to the total area (% Loss to the total), and to the class official value (% Loss to the class) are listed.
In the tables associated to the histograms (Figures 4, 5) the percentage of loss is calculated as the difference between the standard value and the net land value that considers the estimated loss. The percentage values are calculated based on the total land value (1st and 3rd columns), and on the single class (2nd and 4th columns). These numbers are the results of the product of land value, susceptibility and damage coefficient. In the Rivo catchment the outcome resembles very well the fact that even under similar conditions of susceptibility, the most valuable classes (i.e., cultivated and forest) present higher loss, given by the highest economic values and higher damage index. The total loss for the study area is estimated in about 5 Mln€ for 2007 and 4.8 Mln€ for 2050 (corresponding to 10.45 and 9.90% of the total, respectively).
Cultivated areas, together with barelands, are the classes giving the highest contribution to the total area land value loss, with the first one being the class with the highest value loss, followed by the forest.
The highest percentage loss for the single class due to landslide susceptibility occurs mainly in the cultivated, bareland, and forest classes. Nevertheless, the economic loss for bareland is substantially low when compared to the cultivated and forest classes. Namely, bareland losses around 30,000 € for 2007 and 20,000 € for 2050, meanwhile forest loses around 1 Mln€ for 2007 and 2050, and cultivated loses 4 Mln€ for 2007 and around 3.3 Mln€ for 2050. This is attributable to the extension of the single class in the area and even more to the initial economic value.
For the Bălăneasa catchment the susceptibility analysis was applied for the first time, even though similar applications have been run in nearby areas (Damen et al., 2014; Zumpano et al., 2014; Hussin et al., 2016; Malek et al., 2018). The resulting maps for 2010 and 2050 are presented in Figures 3A,D. For both years, the highest susceptibility is distributed at the contact between the Carpathians and the Subcarpathians, where we find high energy relief, with strong rock heterogeneity and deformation. At a greater detail, the spatial distribution of the most landslide prone areas is mostly controlled by the relief and in particular by slope and aspect. Further, a significant influence seems to be played by the ancient landslides deposits too, in correspondence of which the highest susceptibility classes are reported. The five susceptibility classes from very low to very high cover have, respectively for 2010 and 2050, the following areal percentages: VL 6.3–6%; L 35.4–36.9%; M 37.7–37.1%; H 16.6–16.5%; and VH 4–3.6% (cfr. diagram in Figure 4A). The difference in the spatial distribution and in the percentage of classes between 2010 and 2050 is attributable to the estimated changes in the land cover, namely due to the forest enlargement at the expense of cropland and pastures.
Moving to the loss due to susceptibility (Figures 3C,F), for both years the highest value corresponding to the highest susceptibility classes is 0.252 €/m2. This value is concentrated where also the most valuable land cover classes such as forest and, secondarily, cultivated areas meet the highest susceptibility classes.
The histogram in Figure 5 gives an insight about the loss in each land cover type in the two considered years, also allowing useful comparison. The total loss for year 2010 is 4.3 and 4.5 Mln€ for 2050, that correspond to the 29.6 and 29.81% with respect to the initial value.
The class giving the highest contribution to the total loss is forest (25.57%). Forest and cultivated are also the classes with the highest differences between the standard and the real value, namely 33.37 and 25.63% (highest percentage loss to the class, table in Figure 5), corresponding to about 3.8 Mln€ and 400,000 €, respectively.
In addition, given that cultivated areas are not widespread at the site, they contribute poorly to the total loss, contrarily to what happens with forest, even though this latter is not characterized by very high susceptibility.
For the scenario 2050, the total loss is 4.5 Mln€, and similar considerations can be drawn, given that they show similar figures.
Discussion and Conclusion
In this work we have introduced a semi-quantitative approach applicable at the regional scale to estimate the loss of value suffered by rural lands and, in a local way and to a lesser degree, by agricultural lands due to landslide susceptibility. The approach is mostly expert-based and quantifies the susceptibility and the land resilience through indexes that, once interpolated with the land cover values, allow to estimate the loss.
We produced two sets of landslide susceptibility maps related to two time frames (2007/2010 and 2050), in order to evaluate possible values changes and trends related to land cover variations. It is known, in fact, that landslide susceptibility is influenced by land cover, which changes might determine variations in the spatial distribution of the highest landslide susceptibility classes (Vanacker et al., 2003; Wasowski et al., 2010, 2014; Promper et al., 2014; Reichenbach et al., 2014; Pisano et al., 2017b,c).
We tested our method for the evaluation of the economic loss in two different study areas placed in different countries, with different socio-economic and environmental settings.
The main findings show how land value may suffer changes due land cover variations through time, or due to landslide susceptibility that interferes with the land itself.
In particular for the Rivo catchment we observed a change in the total land value consisting in about 2 Mln€, representing 5% of the entire area land value, that is due mainly to the decrease of cultivated areas according to the modeled scenario. On the contrary, for the susceptibility the loss is estimated to be 5 Mln€ for 2007 and 4.8 Mln€ for 2050, with the highest loss values in the cultivated areas and forest. However the class with the highest percentage loss with respect to the initial class value is bareland, that is the most susceptible class in the area, even though it is the one with the lowest economical value.
In the Bălăneasa area the land cover changes observed in the trend scenario are few, with a slight increase in the economic values (+0.29 Mln€) ascribable mostly to the changes from forest to pasture. As regards the depreciation due to susceptibility there is a loss of 29.6% and 29.81% with respect to the initial total land value for 2010 and 2050, respectively. The class suffering the highest loss is forest, given that it is also the most valuable in the area; in addition, it gives the highest contribution to the total land value, due to the fact it is the main class, covering almost 45%. As for the other classes, bareland and cultivated areas are subject to high losses in 2010 and 2050, being the most susceptible classes in the area; nevertheless, they do not contribute predominantly to the total loss, as a result of their reduced areal extension, and low economic values as regards bareland.
Looking at numbers, it appears that the total losses obtained for the Italian case study are higher. Actually, these outcomes are biased by the initial land values, which are not comparable, and present higher numbers for the Rivo catchment.
The results represent a first example of the estimation of loss values in rural environments, which in the literature are often not taken into any account, since evaluation of the loss typically is focused on buildings and infrastructures. The present work ranks therefore among the very few scientific publications devoted to present a methodology aimed at evaluating this type of loss. Being a first attempt in using such an approach, it might contain some uncertainties, and could certainly be improved in the near future. However, the method has proven to be reasonable and easily applicable. The amount of data needed is acceptable and quite easy to find, which allows an application even in contexts where the data are incomplete, or when there is the need to run a quick estimate as a pre-analysis before a following, more detailed, study. Furthermore, our approach even if simple takes in consideration both factors influencing the land values, namely the land cover variation and the landslide proneness, besides the fact it allows to estimate the potential loss before the occurrence of the landslide event.
By applying this approach, it is possible to obtain results that could be exploited for land use planning, given that landslides occurrence threatens the productive activities and hence might have a severe impact on the involved communities.
In conclusions, considering the growing attention toward global changes, especially by decision makers increasingly worried about geo-hydrological consequences (Gariano and Guzzetti, 2016), we believe that applying the method exploiting future scenarios could provide useful information for a more sound and reasonable land management in territories with high susceptibility to landslides. Using these information as a hint to plan future land uses, without excluding the possibility of occurrence of landslides, could result in a decrease of the losses of land value and land productivity. At the same time, a conscious land planning, taking into account the distribution and activity of slope movements, could in turn contribute to mitigate the risk associated to occurrence of landslide phenomena.
Data Availability
The raw data supporting the conclusion of this manuscript will be made available by the authors, without undue reservation, to any qualified researcher.
Author Contributions
VZ, LP, MP, and PPCA were responsible for the conceptual design of the work. LP and VZ mapped the landslides for Rivo area and LP mapped the land cover for the same area. MM and VZ collected the landslide data for Bălăneasa catchment. ŽM mapped past land cover for Bălăneasa and performed all the analysis for the land cover scenario for both case studies. VZ, LP, and MP carried out calculation and data interpretation. MM and DB provided all the other information regarding the Romanian case study. VZ and LP lead writing and compilation of figures and tables. MP, ŽM, and MM contributed significantly to improve the manuscript. PPCA and CR helped in the conceptualization of the work and final overview.
Funding
Part of the data acquisition and elaboration were carried out in the framework of the CHANGES project (Changing Hydro-meteormological Risks—as Analyzed by a New Generation of European Scientists), a Marie Curie Initial Training Network, funded by the European Community's 7th Framework Programme FP7/2007-2013, Grant Agreement No. 263953.
Conflict of Interest Statement
The authors declare that the research was conducted in the absence of any commercial or financial relationships that could be construed as a potential conflict of interest.
Supplementary Material
The Supplementary Material for this article can be found online at: https://www.frontiersin.org/articles/10.3389/feart.2018.00097/full#supplementary-material
Footnotes
1. ^ILWIS (2012). Academic 3.3. Available online at: http://www.ilwis.org/open_source_gis_ilwis_download.htm
References
Alexander, D. (1986). Landslide damage to buildings. Environ. Geol. Water Sci. 8, 147–151. doi: 10.1007/BF02509902
Alkema, D. (2007). Simulating Floods; on the Application of a 2D-Flood Model for Hazard and Risk Assessment. Ph.D. thesis, University of Utrecht, Utrecht.
Aucelli, P. P. C., Balteanu, D., Izzo, M., and Rosskopf, C. M. (2004). “Types and mechanism of slope failure due to the rainfall event on January 2003 in Molise region (S. Italy),” in Geomorphological Environment, eds H. S. Savindra Singh, Kr. De. Sharma, and Sunil (Kolkata: ACB Publications), 125–142.
Aucelli, P. P. C., Cinque, A., and Rosskopf, C. M. (2001). Geomorphological map of the Trigno basin (Italy): explanatory notes. Geografia Fisica e Dinamica Quaternaria 24, 3–12.
Aucelli, P. P. C., Robustelli, G., Rosskopf, C. M., Scarciglia, F., Di Paola, G., and Lucá, F. (2010). Geomorphological map of the area between Frosolone and Trivento (Molise, Italy). J. Maps 6, 423–434. doi: 10.4113/jom.2010.1039
Boerboom, L. G. J., and Alan, Ö. O. (2012). Implementation, challenges and future directions of integrating services from the gis and decision science domains: a case of distributed spatial multi-criteria evaluation. OSGeo J. 10, 49–54.
Borgomeo, E., Hebditch, K. V., Whittaker, A. C., and Lonergan, L. (2014). Characterising the spatial distribution, frequency and geomorphic controls on landslide occurrence, Molise, Italy. Geomorphology 226, 148–161. doi: 10.1016/j.geomorph.2014.08.004
Calò, F., Calcaterra, D., Iodice, A., Parise, M., and Ramondini, M. (2012). Assessing the activity of a large landslide in southern Italy by ground-monitoring and SAR interferometric techniques. Int. J. Remote Sens. 33, 3512–3530. doi: 10.1080/01431161.2011.630331
Calcaterra, D., Ramondini, M., Calò, F., Longobardi, V., Parise, M., and Galzerano, C. M. (2008). “DInSAR techniques for monitoring slow-moving landslides. in Landslides and Engineered Slopes,” in Proceedings of the 10th International Symposium on Landslides, eds Z. Cheng, J. Zhang, Z. Li, F. Wu, and K. Ho (Xi'an), 1095–1101.
Chiocchio, C., Iovine, G., and Parise, M. (1997). “A proposal for surveying and classifying landslide damage to buildings in urban areas,” in Proceedings of the 10th International Symposium on Engineering Geology and the Environment (Athens) 23–27.
Damen, M., Micu, M., Zumpano, V., van Westen, C. J., Sijmons, K., and Balteanu, D. (2014). “Landslide mapping and interpretation: implications for landslide susceptibility analysis in discontinuous data environment,” in Proceedings of the International Conference Analysis and Management of Changing Risks for Natural Hazards (Padua), 18–19.
Del Soldato, M., Bianchini, S., Calcaterra, D., De Vita, P., Di Martire, D., Tomás, R., et al. (2017). A new approach for landslide-induced damage assessment. Geomat. Nat. Hazards Risk 8, 1524–1537. doi: 10.1080/19475705.2017.1347896
Donnini, M., Napolitano, E., Salvati, P., Ardizzone, F., Bucci, F., Fiorucci, F., et al. (2017). Impact of event landslides on road networks: a statistical analysis of two Italian case studies. Landslides 14, 1521–1535. doi: 10.1007/s10346-017-0829-4
Fiorillo, F., Parise, M., and Wasowski, J. (1996). “Slope instability in the Bisaccia area (Southern Apennines, Italy),” in Proceedings of the 7th International Symposium on Landslides (Trondheim), 965–970.
Fleming, R. W., and Taylor, F. A. (1980). Estimating the Costs of Landslide Damage in the United States. U.S. Geol. Surv. Circ. 832:21.
Gariano, S. L., and Guzzetti, F. (2016). Landslides in a changing climate. Earth Sci. Rev. 162, 227–252. doi: 10.1016/j.earscirev.2016.08.011
Gariano, S. L., Petrucci, O., Rianna, G., Santini, M., and Guzzetti, F. (2018). Impacts of past and future land changes on landslides in southern Italy. Reg. Environ. Chang. 18, 437–449. doi: 10.1007/s10113-017-1210-9
Global Assessment Report on Disaster Risk Reduction (GAR) (2015). United Nations Office for Disaster Risk Reduction (UNISDR). Available online at: https://www.unisdr.org/we/inform/gar
Godt, J. W., Coe, J. A., and Savage, W. Z. (2000). “Relation between cost of damaging landslides and construction age, El Niño winter storm season, 1997-98,” in Proceedings of the 8th International Symposium on Landslides (Alameda County, CA), 26–30.
Hussin, H. Y., Zumpano, V., Reichenbach, P., Sterlacchini, S., Micu, M., van Westen, C., et al. (2016). Different landslide sampling strategies in a grid-based bi-variate statistical susceptibility model. Geomorphology 253, 508–523. doi: 10.1016/j.geomorph.2015.10.030
Intergovernmental Panel on Climate Change (IPCC) (2012). “Summary for policymakers: managing the risks of extreme events and disasters to advance climate change adaptation,” in A Special Report of Working Groups I and II of the Intergovernmental Panel on Climate Change, eds C. B. Field, V. Barros, T. F. Stocker, D. Qin, D. J. Dokken, K. L. Ebi, M. D. Mastrandrea, K. J. Mach, G.-K. Plattner, S. K. Allen, M. Tignor, and P. M. Midgley (Cambridge, UK; New York, NY: Cambridge University Press)
Iovine, G., and Parise, M. (2002). Schema classificativo per il rilievo dei danni da frana in aree urbane. Mem. Soc. Geol. Ital. 57, 595–603.
Kreibich, H., van den Bergh, J. C. J. M., Bouwer, L. M., Bubeck, P., Ciavola, P., Green, C., et al. (2014). Costing natural hazards. Nat. Clim. Chang. 4, 303–306. doi: 10.1038/nclimate2182
Lesk, C., Rowhani, P., and Ramankutty, N. (2016). Influence of extreme weather disasters on global crop production. Nature 529, 84–87. doi: 10.1038/nature16467
Malek, Ž., Scolobig, A., and Schröter, D. (2014). Understanding land cover changes in the Italian Alps and Romanian Carpathians combining remote sensing and stakeholder interviews. Land 3, 52–73. doi: 10.3390/land3010052
Malek, Ž., Zumpano, V., and Hussin, H. (2018). Forest management and future changes to ecosystem services in the Romanian Carpathians. Environ. Dev. Sustain. 20, 1275–1291. doi: 10.1007/s10668-017-9938-4
Malek, Ž., Zumpano, V., Schröter, D., Glade, T., Balteanu, D., and Micu, M. (2015). “Scenarios of land cover change and landslide susceptibility: an example from the Buzau Subcarpathians, Romania,” in Engineering Geology for Society and Territory Vol 5, eds G. Lollino, A. Manconi, F. Guzzetti, M. Culshaw, P. Bobrowsky, and F. Luino (Switzerland: Springer International Publishing), 743–746.
Mela, G., Longhitano, D., and Povellato, A. (2012). “The evolution of land values in italy. does the influence of agricultural prices really matter ?” in 123rd Eaae Seminar - Price Volatility and Farm Income Stabilisation: Modelling Outcomes and Assessing Market and Policy Based Responses (Dublin). Available online at: http://purl.umn.edu/122479
Micu, M. (2008). Landslide Hazard Assessment in the Subcarpathians Between Teleajen and Buzau Rivers. Ph.D Thesis, Institute of Geography, Bucharest.
Micu, M., Jurchescu, M., Micu, D., Zarea, R., Zumpano, V., and Bălteanu, D. (2014). A morphogenetic insight into a multi-hazard analysis: Bâsca Mare landslide dam. Landslides 11, 1131–1139. doi: 10.1007/s10346-014-0519-4
Parise, M., Vennari, C., Guzzetti, F., Marchesini, I., and Salvati, P. (2013). Preliminary outcomes from a catalogue of natural and anthropogenic sinkholes in Italy, and analysis of the related damage. Rendiconti Online della Società Geologica Italiana 24, 225–227. doi: 10.1038/504196a
Parise, M., and Wasowski, J. (1999). Landslide activity maps for the evaluation of landslide hazard: three case studies from Southern Italy. Nat. Hazards 20, 159–183. doi: 10.1023/A:1008045127240
Persichillo, M. G., Bordoni, M., and Meisina, C. (2017). The role of land use changes in the distribution of shallow landslides. Sci. Total Environ. 574, 924–937. doi: 10.1016/j.scitotenv.2016.09.125
Petley, D. (2012). Global patterns of loss of life from landslides. Geology 40, 927–930. doi: 10.1130/G33217.1
Pisano, L., Zumpano, V., Dragone, V., and Parise, M. (2017a). “Built-up area exposure to landslides and related social impacts in Molise (Italy) - advancing culture of Living with Landslides,” in Advances in Landslide Science Vol 2, eds M. Mikos, B. Tiwari, Y. Yin, and K. Sassa (Cham: Springer International Publishing), 837–845.
Pisano, L., Zumpano, V., Malek, Ž., Micu, M., Rosskopf, C. M., and Parise, M. (2017b). “Multi-temporal landslide susceptibility maps and future scenarios for expected land cover changes (Southern Apennines, Italy) - advancing culture of living with landslides: Vol 5,” in Landslides in Different Environments, eds M. Mikoš, V. Vilímek, Y. Yin, and K. Sassa (Cham: Springer International Publishing), 379–387.
Pisano, L., Zumpano, V., Malek, Ž., Rosskopf, C. M., and Parise, M. (2017c). Variations in the susceptibility to landslides, as a consequence of land cover changes: a look to the past, and another towards the future. Sci. Total Environ. 601, 1147–1159. doi: 10.1016/j.scitotenv.2017.05.231
Pourghasemi, H. R., Moradi, H. R., Fatemi Aghda, S. M., Gokceoglu, C., and Pradhan, B. (2014). GIS based landslide susceptibility mapping with probabilistic likelihood ratio and spatial multi-criteria evaluation models (North of Tehran, Iran). Arab. J. Geosci. 7, 1857–1878. doi: 10.1007/s12517-012-0825-x
Pradhan, B., and Lee, S. (2010). Delineation of landslide hazard areas on Penang Island, Malaysia, by using frequency ratio, logistic regression, and artificial neural network models. Environ. Earth Sci. 60, 1037–1054. doi: 10.1007/s12665-009-0245-8
Promper, C., and Glade, T. (2016). Multilayer-exposure maps as a basis for a regional vulnerability assessment for landslides: applied in Waidhofen/Ybbs, Austria. Nat. Hazards 82, 111–127. doi: 10.1007/s11069-016-2311-3
Promper, C., Puissant, A., Malet, J.-P., and Glade, T. (2014). Analysis of land cover changes in the past and the future as contribution to landslide risk scenarios. Appl. Geogr. 53, 11–19. doi: 10.1016/j.apgeog.2014.05.020
Reichenbach, P., Busca, C., Mondini, A. C., and Rossi, M. (2014). The influence of land use change on landslide susceptibility zonation: the briga catchment test site (Messina, Italy). Environ. Manage. 54, 1372–1384. doi: 10.1007/s00267-014-0357-0
Rosskopf, C. M., and Aucelli, P. P. C. (2007). “Analisi del dissesto da frana in Molise,” in Rapporto Sulle Frane in Italia. Il Progetto IFFI–Metodologia, Risultati e Rapporti Regionali 78, ed A. Trigila (Rapporti APAT), 493–508.
Salvati, P., Petrucci, O., Rossi, M., Bianchi, C., Pasqua, A. A., and Guzzetti, F. (2018). Gender, age and circumstances analysis of flood and landslide fatalities in Italy. Sci. Total Environ. 610–611, 867–879. doi: 10.1016/j.scitotenv.2017.08.064
Schmaltz, E. M., Steger, S., and Glade, T. (2017). The influence of forest cover on landslide occurrence explored with spatio-temporal information. Geomorphology 290, 250–264. doi: 10.1016/j.geomorph.2017.04.024
Schuster, R. L. (1996). “Socio-economic significance of landslides,” in Landslides Investigation and Mitigation: Transportation Research Board, Vol. 247, eds A. K. Turner and R. L. Schuster (Washington, DC: National Academy Press), 12–35.
Schuster, R. L., and Fleming, R. W. (1986). Economic losses and fatalities due to landslides. Bull. Ass. Eng. Geol. 23, 11–28. doi: 10.2113/gseegeosci.xxiii.1.11
Sgrosso, I., and Naso, G. (2012). Note Illustrative del Foglio 393 “Trivento” della Carta Geologica d'Italia alla scala 1:50000. Roma: ISPRA.
Soares-Filho, B. S., Coutinho Cerqueira, G., and Lopes Pennachin, C. (2002). DINAMICA - a stochastic cellular automata model designed to simulate the landscape dynamics in an Amazonian colonization. Front. Ecol. Model. 154, 217–235. doi: 10.1016/S0304-3800(02)00059-5
Tanoue, M., Hirabayashi, Y., and Ikeuchi, H. (2016). Global-scale river flood vulnerability in the last 50 years. Sci. Rep. 6:36021. doi: 10.1038/srep36021
Vanacker, V., Vanderschaeghe, M., Govers, G., Willems, E., Poesen, J., Deckers, J., et al. (2003). Linking hydrological, infinite slope stability and land-use change models through GIS for assessing the impact of deforestation on slope stability in high Andean watersheds. Geomorphology 52, 299–315. doi: 10.1016/S0169-555X(02)00263-5
van Vliet, J., de Groot, H. L., Rietveld, P., and Verburg, P. H. (2015). Manifestations and underlying drivers of agricultural land use change in Europe. Landsc. Urban Plan. 133, 24–36. doi: 10.1016/j.landurbplan.2014.09.001
Van Westen, C. J., Asch, T. W. J., and Soeters, R. (2006). Landslide hazard and risk zonation—why is it still so difficult? Bull. Eng. Geol. Environ. 65, 167–184. doi: 10.1007/s10064-005-0023-0
Vennari, C., Santangelo, N., Santo, A., and Parise, M. (2015). A database on flood and debris-flow processes in alluvial fans: a preliminary analysis aimed at evaluation of the damage. Geophys. Res. Abstracts 17, 528.
Vennari, C., Santangelo, N., Santo, A., and Parise, M. (2016). A database on flash flood events in Campania, southern Italy, with an evaluation of their spatial and temporal distribution. Nat. Hazards Earth Syst. Sci. 16, 2485–2500. doi: 10.5194/nhess-16-2485-2016
Vranken, L., Van Turnhout, P., Van Den Eeckhaut, M., Vandekerckhove, L., and Poesen, J. (2013). Economic valuation of landslide damage in hilly regions: a case study from Flanders, Belgium. Sci. Total Environ. 447, 323–336. doi: 10.1016/j.scitotenv.2013.01.025
Ward, P. J., Jongman, B., Weiland, F. S., Bouwman, A., Van Beek, R., Bierkens, M. F. P., et al. (2013). Assessing flood risk at the global scale: model setup, results, and sensitivity. Environ. Res. Lett. 8, 1–10. doi: 10.1088/1748-9326/8/4/044019
Wasowski, J., Lagreca, M. D., and Lamanna, C. (2014). “Land-use change and shallow landsliding: a case history from the Apennine Mountains, Italy,” in Landslide Science for a Safer Geoenvironment, The International Programme on Landslides (IPL) Vol. 1. eds K. Sassa, P. Canuti, Y. Yin (Cham, Springer International Publishing), 267–272.
Wasowski, J., Lamanna, C., and Casarano, D. (2010). Influence of land-use change and precipitation patterns on landslide activity in the Daunia Apennines, Italy. Q. J. Eng. Geol. Hydrogeol. 43, 387–401. doi: 10.1144/1470-9236/08-101
World Meterological Organization (WMO) (2014). Atlas of Mortality and Economic Losses from Weather, Climate and Water Extremes (1970–2012). World Meterological Organization.
Yalcin, A., Reis, S., Aydinoglu, A. C., and Yomralioglu, T. (2011). A GIS-based comparative study of frequency ratio, analytical hierarchy process, bivariate statistics and logistics regression methods for landslide susceptibility mapping in Trabzon, NE Turkey. Catena 85, 274–287. doi: 10.1016/j.catena.2011.01.014
Zêzere, J. L., Garcia, R. A. C., Oliveira, S. C., and Reis, E. (2008). Probabilistic landslide risk analysis considering direct costs in the area north of Lisbon (Portugal). Geomorphology 94, 467–495. doi: 10.1016/j.geomorph.2006.10.040
Keywords: landslides, land cover changes, economic loss, susceptibility, future scenario
Citation: Zumpano V, Pisano L, Malek Ž, Micu M, Aucelli PPC, Rosskopf CM, Balteanu D and Parise M (2018) Economic Losses for Rural Land Value Due to Landslides. Front. Earth Sci. 6:97. doi: 10.3389/feart.2018.00097
Received: 28 February 2018; Accepted: 29 June 2018;
Published: 25 July 2018.
Edited by:
Davide Tiranti, Agenzia Regionale per la Protezione Ambientale (ARPA), ItalyReviewed by:
José Darrozes, UMR5563 Géosciences Environnement Toulouse (GET), FranceClaudia Meisina, University of Pavia, Italy
Copyright © 2018 Zumpano, Pisano, Malek, Micu, Aucelli, Rosskopf, Balteanu and Parise. This is an open-access article distributed under the terms of the Creative Commons Attribution License (CC BY). The use, distribution or reproduction in other forums is permitted, provided the original author(s) and the copyright owner(s) are credited and that the original publication in this journal is cited, in accordance with accepted academic practice. No use, distribution or reproduction is permitted which does not comply with these terms.
*Correspondence: Luca Pisano, bC5waXNhbm9AYmEuaXJwaS5jbnIuaXQ=