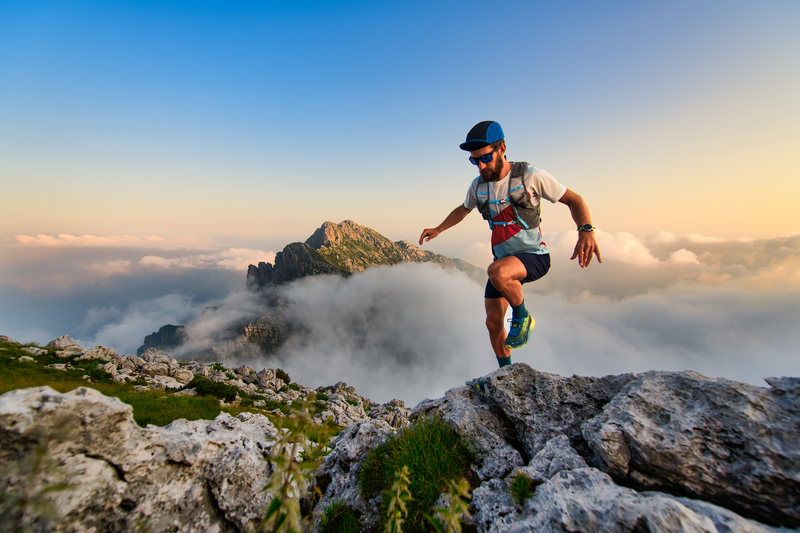
95% of researchers rate our articles as excellent or good
Learn more about the work of our research integrity team to safeguard the quality of each article we publish.
Find out more
MINI REVIEW article
Front. Dev. Psychol. , 23 September 2024
Sec. Cognitive Development
Volume 2 - 2024 | https://doi.org/10.3389/fdpys.2024.1467880
This article is part of the Research Topic Advances in Metacognition and Reflection View all 10 articles
Examining children's decisions to explore or exploit the environment provides a window into their developing metacognition and reflection capacities. Reinforcement learning, characterized by the balance between exploring new options (exploration) and utilizing known ones (exploitation), is central to this discussion. Children initially exhibit broad and intensive exploration, which gradually shifts toward exploitation as they grow. We review major theories and empirical findings, highlighting two main exploration strategies: random and directed. The former involves stochastic choices without considering information or rewards, while the latter is driven by reducing uncertainty for information gain. Behavioral tasks such as n-armed bandit, horizon, and patch foraging tasks are used to study these strategies. Findings on the n-armed bandit and horizon tasks showed mixed results on whether random exploration decreases over time. Directed exploration consistently decreases with age, but its emergence depends on task difficulty. In patch-foraging tasks, adults tend to overexploit (staying too long in one patch) and children overexplore (leaving too early), whereas adolescents display the most optimal balance. The paper also addresses open questions regarding the mechanisms supporting early exploration and the application of these strategies in real-life contexts like persistence. Future research should further investigate the relation between cognitive control, such as executive function and metacognition, and explore-exploit strategies, and examine their practical implications for adaptive learning and decision-making in children.
When a child is born, the world around them is new and unpredictable. However, they gradually learn about their environment through contingency, forming associations between their behaviors and either positive or negative consequences, and start to use these contingencies to guide their future behaviors. This type of learning is known as reinforcement learning (e.g., Nussenbaum and Hartley, 2019). For example, infants as young as 2 months old quickly increase their kicking behavior in an experiment where a ribbon is attached to their ankle and connected to a mobile hanging overhead (Rovee-Collier, 1997). This behavior occurs because they explore the object attached to their ankle and learn the associations between their leg movements and the mobile's movements. In the beginning, this kind of exploration aims at improving and expanding knowledge. However, choosing whether and when to explore is a genuinely complex decision, as more options become available, varying in value. For instance, if the infants also were given an attractive toy to grasp, they could explore the new toy for potential enjoyment or continue playing with the mobile, which already provides them with joy. As children grow, they face decisions ranging from trivial ones, such as what to eat for dinner or where to play, to more significant ones, such as whether to go to college and whom to be friends with. In such situations, they must either search for better options (explore) or utilize their known options (exploit). Developmental psychologists actively research how children balance the competing demands of exploration and exploitation when faced with two or more options, yet much is still unknown.
In this paper, we aim to review the major theories and empirical findings regarding explore-exploit strategies and how they shift across development. Indeed, young children do explore intensively and broadly, often at the cost of exploitation, and the exploration tendency decreases with age (see Gopnik, 2020, and Nussenbaum and Hartley, 2019 for review). Below, we will overview the definition of exploration and exploitation, the explore-exploit tradeoff/dilemma, and one optimal solution. Next, we will summarize exploration across development in the reinforcement learning literature. Finally, we will highlight directions for future explore-exploit developmental research, with a focus on its potential to advance our understanding of executive function, metacognition, and reflection.
While this is not a systematic review, our methodology is consistent with utilizing PsychInfo and Google Scholar as primary sources. The search was conducted using the following keywords: 1. explore-exploit; development, 2. explore-exploit; development; and task-specific terms (e.g., bandit, horizon, patch-foraging), 3. exploration; reinforcement learning, 4. exploration; reinforcement learning; and task-specific terms (e.g., bandit, horizon, patch-foraging). The search was restricted to articles published between 2010 and 2024, with exceptions made for seminal articles that introduce key concepts, focusing on studies involving human participants from infancy through early adulthood (see Table 1).
Exploration involves experimenting with various options and is typically favored under conditions of low knowledge and high uncertainty (Daw et al., 2006). Conversely, exploitation involves adhering to the most lucrative option to maximize rewards and is typically favored under conditions of high knowledge and low uncertainty. Exploration and exploitation represent endpoints along a spectrum–ranging from broad to narrow, noisy to efficient, and information-seeking to reward-seeking–rather than a strict dichotomy (Frankenhuis and Gopnik, 2023). An explore-exploit tradeoff naturally occurs in the decision-making process because choosing to seek new information (exploration) means forgoing an opportunity to choose a familiar option and secure a known reward (exploitation). This dilemma is prevalent not only in human lives but also across the animal kingdom and within society in general (Cohen et al., 2007; Hills et al., 2015; Mehlhorn et al., 2015).
One strategy used by organisms, including humans, animals, and machines to tackle the explore-exploit dilemma, is balancing exploration and exploitation. This balance refers to initially preferring exploration and gradually transitioning toward exploitation (Cohen et al., 2007; Hills et al., 2015; Mehlhorn et al., 2015). Exploration is prioritized at the onset of the learning process and diminishes over time as the agent accumulates knowledge and reduces uncertainty (Auer, 2002). This pattern is sensible for two reasons, according to Gopnik (2020). Firstly, agents cannot effectively exploit the reward structure of their environment until they have sufficiently explored it. As agents learn more, it becomes more rational to rely on existing knowledge and reduce the drive to acquire new information. Secondly, if there is a limited timeframe to solve a task, as time passes, there are fewer chances to leverage the information acquired through exploration. There is substantial empirical evidence to believe that explore-first and exploit-later strategies may be embodied in our typical developmental trajectories.
There is considerable evidence of children's increased exploration during play in their early years (e.g., Bonawitz et al., 2012; Doan et al., 2020; Golinkoff et al., 2006; Schulz and Bonawitz, 2007). However, this paper focuses specifically on reinforcement learning literature, as it provides the most compelling evidence of developmental transitions in exploration, explicitly showing adaptive decision-making with age (Table 1). Two major exploration strategies are random exploration and directed exploration. Random exploration follows a stochastic choice policy, without considering information or rewards (Giron et al., 2023; Meder et al., 2021). Directed exploration, on the other hand, is driven by a strong desire to gain information and resolve high uncertainty (Giron et al., 2023; Meder et al., 2021; Schulz et al., 2019). Although they are conceptually distinct (Wilson et al., 2014), with dissociable neural signatures (Zajkowski et al., 2017), random and directed exploration are not mutually exclusive. For example, systematic switching in random exploration appears to approximate directed exploration. Behavioral tasks used to study exploration strategies include n-armed bandit tasks (e.g., Gittins and Jones, 1979; Speekenbrink, 2022), horizon tasks (e.g., Wilson et al., 2014), and patch-foraging tasks (e.g., Charnov, 1976; Lloyd et al., 2023).
An n-armed bandit task is like a slot machine with multiple levers. In a 4-armed bandit task, individuals choose from four options, receive feedback on the reward, and make the next selection (Daw et al., 2006). They must balance between exploiting the highest-value option and exploring others to confirm that the known highest-value option remains the best choice. The reward probability can stay constant or change over time (Speekenbrink, 2022). Studies using n-armed bandit tasks have mixed results on whether the randomness of choices decreases over time. Using a spatially correlated multi-armed bandit task (where rewards of different options are correlated to their spatial proximity, meaning that close-distance options have similar reward probabilities), a study comparing 6- and 8-year-olds found high levels of random exploration only in the 6-year-olds group, suggesting a decline in random exploration by middle childhood (Meder et al., 2021). Similar results were observed in a broad age range of participants from 5 to 55 years old, showing a decrease in random exploration with age (Giron et al., 2023). These findings support the “cooling off” theory (Gopnik, 2020), drawing an analogy from statistical physics (Kirkpatrick et al., 1983). Random exploration is likened to a “higher-temperature” (noisier) search, and the “cooling off” process is likened to a simulated annealing algorithm. Just as heating and cooling metal strengthens its structure, children—naïve learners—begin with broad, “high temperature” exploration to avoid local optima and gradually shift to narrow, “low temperature” exploitation by reducing randomness. However, other studies reported no significant differences between children and adults in the amount of random exploration (Schulz et al., 2019) or found that children's exploration is even “systematic” from a young age. In a simplified 4-armed bandit task, Blanco and Sloutsky (2020, 2021, 2024) found that 3–4-year-old children frequently switched their responses and specifically prioritized choosing options they had visited the least recently, making their exploration pattern systematic. These findings may suggest that children are engaging in uncertainty-based directed exploration.
Unlike random exploration, there is more consensus that directed exploration decreases across ages. Relative to adults, children have a bias toward directed exploration and sample options with an intrinsic goal of maximizing the information gain. In a simplified 4-armed bandit task, 4-year-old children preferred options with hidden rewards over visually explicit ones, although there was significant variability within the group (Blanco and Sloutsky, 2021). Using a spatially correlated multi-armed bandit task, studies with children ages 4 to 11 showed higher levels of directed exploration than adults (Meder et al., 2021; Schulz et al., 2019; Wu et al., 2018). For individuals implementing directed exploration, obtaining information is inherently rewarding, and the exploration is encouraged by an information bonus (Auer, 2002).
It is important to note, however, that in n-armed bandit tasks and similar explore-exploit tasks, there is a reward-information confound, making it hard to distinguish between random and directed exploration. Participants only receive feedback on their chosen options and often select the rewarding options to maximize their rewards. This results in an abundance of information about rewarding options, obscuring whether participants' choices were random or aimed at reducing uncertainty. To address this concern, novel tasks like the horizon task have been developed (Wilson et al., 2014). A horizon task is a 2-armed bandit task that includes initial forced-choice trials revealing information about one bandit, followed by free-choice trials where participants choose between two bandits. This design clearly parses between random and directed exploration by removing reward-information confounds in forced-choice trials and manipulating the number of free-choice trials with varying time horizons (e.g., one free-choice trial for a short horizon vs. six for a long horizon).
Several studies have used horizon tasks to investigate how individuals strategically use random and directed exploration. In strategic learning, individuals should select the option with lower means of rewards across trials and the uncertain option more often in the long horizon than in the short horizon. This is because, on the long horizon, individuals have more opportunities to utilize the rewards they explored and learned.
Concerning how this strategic use matures with age, the existing literature does not clearly indicate when children start to show the adult level of mature adaptation to the time horizon or strategic uses of random and directed exploration based on the utility of the environment. Adults increased both directed exploration (by choosing the uncertain option) and random exploration (by choosing the lower-mean option) in the long horizon relative to the short horizon (Wilson et al., 2014). However, adolescents were less flexible in guiding their exploration based on the horizon length, often choosing less uncertain options in the long horizon and preferring high-mean options instead (Somerville et al., 2017). This behavior suggests adolescents value immediate rewards more than new information that holds potential long-term benefits. No age-related changes in random exploration were observed. While Somerville et al. (2017) reported 12-year-olds did not exhibit mature adaptation like adults, another study using a simplified horizon task found that adult-like adaptation can be acquired by ages 11–12, but not at ages 5–6 years old (Zhuang et al., 2023).
The last explore-exploit behavior task is a patch foraging task (e.g., Orchard task in Constantino and Daw, 2015; Harms et al., 2024; Lloyd et al., 2021), which simulates the animal foraging scenario where an individual must decide how long to exploit a resource patch (e.g., a bush with apples) before exploring a new one (Lloyd et al., 2023). As time spent in a patch increases, the resources (apples) become scarcer. Moving to a new patch incurs time costs, and so during the limited time, the best strategy is to optimize harvest per patch. The marginal value theorem (MVT) suggests that the optimal time to explore new patches is when the expected rewards from the current patch drop below the background reward rate, or the average reward rate of the environment.
In patch foraging tasks, exploration decreases from childhood through adulthood (Lloyd et al., 2023). As children grow, they become adept at adjusting their foraging behavior to the environment's richness, aligning with MVT (Lloyd et al., 2023). Adolescents and adults explore more in richer environments and exploit patches more in poorer ones (Lloyd et al., 2023). In some foraging tasks, mature “leaving” even emerges as early as age 6, indicating the early development of optimal threshold identification (de Liaño et al., 2022). However, in classic patch foraging tasks like the Orchard task, middle adolescence seems to be the peak period for optimal foraging behavior. Early adolescents around 11 years old and young adults aged 19 displayed more exploration by leaving earlier than was optimal for reward maximization (Harms et al., 2024). In contrast, using a similar task, 16–17-year-old middle adolescents explored more than adults aged 30 (Lloyd et al., 2021), whereas adults tended to overexploit patches, showing suboptimal performance (Constantino and Daw, 2015). Middle adolescents' optimal-like foraging, garnering more rewards compared to adults, contrasts with the “cooling off” theory, which posits that adults should be more effective at acquiring rewards. Researchers attribute adults' overexploitation to their risk sensitivity, placing too much value on immediate rewards (Constantino and Daw, 2015). Adolescents' reduced aversion to ambiguity may explain their greater exploration and faster adaptation to new environments (Conley and Baskin-Sommers, 2023).
We have reviewed key literature on the dynamics of exploration and exploitation from the preschool period through adulthood. In this section, we highlight two significant questions that remain underexplored and suggest directions for future research.
The first question is: How can explore-exploit strategies be studied in relation to more real-life contexts characterized by uncertainty, complex reward structures, and constraints on time, money, and effort? One relevant context is persistence. Traditionally, the persistence literature has focused on whether individuals persist by repeating the same action until achieving a goal or quitting (e.g., Leonard et al., 2017, 2020, 2021). Recent studies have begun to view persistence as a dynamic process, incorporating the temporal-behavioral aspects of persistence (Lucca et al., 2020; Oeri et al., 2020, 2024; Wang and Bonawitz, 2022). For example, Wang and Bonawitz (2022) found that preschoolers quit difficult tasks, especially when the likelihood of reward is low, suggesting that they strategically use explore-exploit strategies by considering task difficulty and reward probabilities, when they adjust their persistence. In our own work, Kim et al. (2024) investigated explore-exploit strategies in a novel persistence task that was age-appropriate but challenging to achieve the goal (catching pretend fish in ponds with diminishing rewards). Using latent class analysis, we found that children aged 3–7 used three different strategies when persisting toward a goal: exploration-dominant, exploitation-dominant, and balanced. The ability to balance exploration and exploitation did not emerge until around age 6. The balanced approach was interpreted as the most adaptive strategy, revealed by this more dynamic approach to task analysis as opposed to simply capturing persisting vs. quitting. Incorporating explore-exploit strategies in studying persistence dynamics is promising, and more research is anticipated in this area.
The second question is: What are the underlying mechanisms that support young children's intensive and broad exploration in their early lives and their shift to more strategic exploration? One possible mechanism is children's intrinsic motivation to explore. A study by Liquin and Gopnik (2022) supports the idea that children's heightened exploration tendencies are primarily driven by their strong motivation to explore. The authors tested whether the differences in exploration between children and adults were due to differences in their initial beliefs about the environment—assumptions about which options will be rewarding or costly—or motivational differences. Their findings showed no significant differences in initial beliefs between children and adults, indicating that the differences in exploration were derived from motivation. In a follow-up study, when the same hints about the environment were given, both children and adults made similar inferences, further supporting the motivational account.
Another mechanism could be the development of cognitive control, including executive function and metacognition skills, which are essential for problem-solving (Marulis and Nelson, 2021). Exploration is often described as a complex process, as it demands several situational factors that individuals need to take into account prior to exploration, such as ambiguity, expected value of options, and information gains (Lapidow and Bonawitz, 2023; Le Heron et al., 2020). Optimizing exploration requires integrating cognitive processes, such as causal learning (Bonawitz et al., 2012, 2014), reward-based learning (Wittmann et al., 2023), and executive function/metacognition (Badre et al., 2012; Lee and Carlson, 2015; Otto et al., 2013). The protracted development of explore-exploit strategies, with a late shift from predominant exploration to goal-directed decision-making with more exploitation, may be due to the prolonged maturation of executive function and metacognition (O'Leary and Sloutsky, 2017; Roebers, 2017; Zelazo and Carlson, 2012). However, researchers found that even young children (ages 3–4) can show systematic exploration despite immature top-down regulation, which may be possible via bottom-up regulation of broad attention distribution (Blanco and Sloutsky, 2020, 2021). One study even reported no associations between proactive control and strategic exploration adapted to time horizons (Zhuang et al., 2023). In contrast, in the persistence study mentioned earlier, we found that children aged 3–7 with better executive function skills and metacognitive awareness in post-task interviews tended to balance their exploration and exploitation strategies more effectively in the context of diminishing rewards, even after controlling for age (Kim et al., 2024). We reasoned that children who reflected on the task as it unfolded were better able to monitor and control their strong urge to explore novel options. Since persistence aims at achieving a goal, future studies could examine how to foster younger children's adaptive persistence decision-making by helping them reflect upon their performance and learn flexibility in their thinking process, determining when to keep going and when to change their goals or strategies. As current findings are mixed, however, more research is needed to investigate the relations between explore-exploit strategies and cognitive control.
In conclusion, the dynamics of exploration and exploitation throughout child development is a complex interplay between the desire to seek new information and the need to take advantage of known rewards. Children's exploration is influenced by their intrinsic motivation to explore, and they become more balanced in strategy use with age and the development of cognitive control skills. Understanding these developmental trajectories not only deepens our knowledge but also has practical implications for parenting and educational interventions aimed at fostering adaptive learning and decision-making skills. Future research should continue to examine the underlying mechanisms that support children's exploration and drive the transitions with ages and examine how explore-exploit strategies can be applied to real-life situations, ultimately helping children achieve their goals effectively.
SK: Writing – original draft, Writing – review & editing. SC: Writing – review & editing.
The author(s) declare that no financial support was received for the research, authorship, and/or publication of this article.
The authors declare that the research was conducted in the absence of any commercial or financial relationships that could be construed as a potential conflict of interest.
The author(s) declared that they were an editorial board member of Frontiers, at the time of submission. This had no impact on the peer review process and the final decision.
All claims expressed in this article are solely those of the authors and do not necessarily represent those of their affiliated organizations, or those of the publisher, the editors and the reviewers. Any product that may be evaluated in this article, or claim that may be made by its manufacturer, is not guaranteed or endorsed by the publisher.
Auer, P. (2002). Using confidence bounds for exploitation-exploration trade-offs. J. Mach. Learn. Res. 3, 397–422.
Badre, D., Doll, B. B., Long, N. M., and Frank, M. J. (2012). Rostrolateral prefrontal cortex and individual differences in uncertainty-driven exploration. Neuron 73, 595–607. doi: 10.1016/j.neuron.2011.12.025
Blanco, N. J., and Sloutsky, V. M. (2020). Attentional mechanisms drive systematic exploration in young children. Cognition 202:104327. doi: 10.1016/j.cognition.2020.104327
Blanco, N. J., and Sloutsky, V. M. (2021). Systematic exploration and uncertainty dominate young children's choices. Dev. Sci. 24:e13026. doi: 10.1111/desc.13026
Blanco, N. J., and Sloutsky, V. M. (2024). Exploration, exploitation, and development: Developmental shifts in decision-making. Child Dev. 95, 1287–1298. doi: 10.1111/cdev.14070
Bonawitz, E., Denison, S., Gopnik, A., and Griffiths, T. L. (2014). Win-Stay, Lose-Sample: A simple sequential algorithm for approximating Bayesian inference. Cogn. Psychol. 74, 35–65. doi: 10.1016/j.cogpsych.2014.06.003
Bonawitz, E. B., van Schijndel, T. J., Friel, D., and Schulz, L. (2012). Children balance theories and evidence in exploration, explanation, and learning. Cogn. Psychol. 64, 215–234. doi: 10.1016/j.cogpsych.2011.12.002
Charnov, E. L. (1976). Optimal foraging, the marginal value theorem. Theor. Popul. Biol. 9, 129–136. doi: 10.1016/0040-5809(76)90040-X
Cohen, J. D., McClure, S. M., and Yu, A. J. (2007). Should I stay or should I go? How the human brain manages the trade-off between exploitation and exploration. Philos. Trans. R. Soc. Lond. B Biol. Sci. 362, 933–942. doi: 10.1098/rstb.2007.2098
Conley, M. I., and Baskin-Sommers, A. (2023). Development in uncertain contexts: An ecologically informed approach to understanding decision-making during adolescence. Cogn Affect Behav Neurosci. 23, 739–745. doi: 10.3758/s13415-023-01067-7
Constantino, S. M., and Daw, N. D. (2015). Learning the opportunity cost of time in a patch- foraging task. Cogn Affect Behav Neurosci. 15, 837–853. doi: 10.3758/s13415-015-0350-y
Daw, N. D., O'Doherty, J. P., Dayan, P., Seymour, B., and Dolan, R. J. (2006). Cortical substrates for exploratory decisions in humans. Nature 441, 876–879. doi: 10.1038/nature04766
de Liaño, B. G. G., Munoz-Garcia, A., Pérez-Hernández, E., and Wolfe, J. M. (2022). Quitting rules in hybrid foraging search: From early childhood to early adulthood. Cogn. Dev. 64:101232. doi: 10.1016/j.cogdev.2022.101232
Doan, T., Castro, A., Bonawitz, E., and Denison, S. (2020). “Wow, I did it!”: Unexpected success increases preschoolers' exploratory play on a later task. Cogn. Dev. 55:100925. doi: 10.1016/j.cogdev.2020.100925
Frankenhuis, W. E., and Gopnik, A. (2023). Early adversity and the development of explore–exploit tradeoffs. Trends Cogn. Sci. 27, 616–630. doi: 10.1016/j.tics.2023.04.001
Giron, A. P., Ciranka, S., Schulz, E., van den Bos, W., Ruggeri, A., Meder, B., et al. (2023). Developmental changes in exploration resemble stochastic optimization. Nat. Human Behav. 7, 1955–1967. doi: 10.1038/s41562-023-01662-1
Gittins, J. C., and Jones, D. M. (1979). A Dynamic Allocation Index for the Discounted Multiarmed Bandit Problem. Biometrika 66, 561–565. doi: 10.1093/biomet/66.3.561
Golinkoff, R. M., Hirsh-Pasek, K., and Singer, D. G. (2006). “Why play = learning: A challenge for parents and educators,” in Play = Learning: How Play Motivates and Enhances Children's Cognitive and Social-Emotional Growth, eds. D. G. Singer, R. M. Golinkoff, and K. Hirsh-Pasek (Oxford: Oxford University Press), 3–12.
Gopnik, A. (2020). Childhood as a solution to explore–exploit tensions. Philos. Trans. R. Soc. Lond. B Biol. Sci. 375:20190502. doi: 10.1098/rstb.2019.0502
Harms, M. B., Xu, Y., Green, C. S., Woodard, K., Wilson, R., and Pollak, S. D. (2024). The structure and development of explore-exploit decision making. Cogn. Psychol. 150:101650. doi: 10.1016/j.cogpsych.2024.101650
Hills, T. T., Todd, P. M., Lazer, D., Redish, A. D., and Couzin, I. D. (2015). Exploration versus exploitation in space, mind, and society. Trends Cogn. Sci. 19, 46–54. doi: 10.1016/j.tics.2014.10.004
Kim, S., Berry, D., and Carlson, S. M. (2024). Should I stay or should I go? Children's persistence in the context of diminishing rewards. Dev. Sci. (Minor revision under review).
Kirkpatrick, S., Gelatt Jr, C. D., and Vecchi, M. P. (1983). Optimization by simulated annealing. Science 220, 671–680. doi: 10.1126/science.220.4598.671
Lapidow, E., and Bonawitz, E. (2023). What's in the box? Preschoolers consider ambiguity, expected value, and information for future decisions in explore-exploit tasks. Open Mind 7, 855–878. doi: 10.1162/opmi_a_00110
Le Heron, C., Kolling, N., Plant, O., Kienast, A., Janska, R., Ang, Y. S., et al. (2020). Dopamine modulates dynamic decision-making during foraging. J. Neurosci. 40, 5273–5282. doi: 10.1523/JNEUROSCI.2586-19.2020
Lee, W. S. C., and Carlson, S. M. (2015). Knowing when to be “rational:” Economic decision-making and executive function in preschool children. Child Dev. 86, 1434–1448. doi: 10.1111/cdev.12401
Leonard, J. A., Garcia, A., and Schulz, L. E. (2020). How adults' actions, outcomes, and testimony affect preschoolers' persistence. Child Dev. 91, 1254–1271. doi: 10.1111/cdev.13305
Leonard, J. A., Lee, Y., and Schulz, L. E. (2017). Infants make more attempts to achieve a goal when they see adults persist. Science 357, 1290–1294. doi: 10.1126/science.aan2317
Leonard, J. A., Martinez, D. N., Dashineau, S. C., Park, A. T., and Mackey, A. P. (2021). Children persist less when adults take over. Child Dev. 92, 1325–1336. doi: 10.1111/cdev.13492
Liquin, E. G., and Gopnik, A. (2022). Children are more exploratory and learn more than adults in an approach-avoid task. Cognition 218:104940. doi: 10.1016/j.cognition.2021.104940
Lloyd, A., McKay, R., Sebastian, C. L., and Balsters, J. H. (2021). Are adolescents more optimal decision-makers in novel environments? Examining the benefits of heightened exploration in a patch foraging paradigm. Dev. Sci. 24:e13075. doi: 10.1111/desc.13075
Lloyd, A., Viding, E., McKay, R., and Furl, N. (2023). Understanding patch foraging strategies across development. Trends Cogni. Sci. 27, 1085–1098. doi: 10.1016/j.tics.2023.07.004
Lucca, K., Horton, R., and Sommerville, J. A. (2020). Infants rationally decide when and how to deploy effort. Nat. Human Behav. 4, 372–379. doi: 10.1038/s41562-019-0814-0
Marulis, L. M., and Nelson, L. J. (2021). Metacognitive processes and associations to executive function and motivation during a problem-solving task in 3-5 year olds. Metacogni. Learn. 16, 207–231. doi: 10.1007/s11409-020-09244-6
Meder, B., Wu, C. M., Schulz, E., and Ruggeri, A. (2021). Development of directed and random exploration in children. Dev. Sci. 24:e13095. doi: 10.1111/desc.13095
Mehlhorn, K., Newell, B. R., Todd, P. M., Lee, M. D., Morgan, K., Braithwaite, V. A., et al. (2015). Unpacking the exploration-exploitation tradeoff: a synthesis of human and animal literatures. Decision 2, 191–215. doi: 10.1037/dec0000033
Nussenbaum, K., and Hartley, C. A. (2019). Reinforcement learning across development: what insights can we draw from a decade of research? Dev. Cogn. Neurosci. 40:100733. doi: 10.1016/j.dcn.2019.100733
Oeri, N., Kälin, S., and Buttelmann, D. (2020). The role of executive functions in kindergarteners' persistent and non-persistent behaviour. Br. J. Dev. Psychol. 38, 337–343. doi: 10.1111/bjdp.12317
Oeri, N., Kunz, N. T., and Kälin, S. (2024). Task persistence through a dynamic lens: Understanding temporal-behavioral dynamics in kindergarten children. J. Appl. Dev. Psychol. 92, 101642. doi: 10.1016/j.appdev.2024.101642
O'Leary, A. P., and Sloutsky, V. M. (2017). Carving metacognition at its joints: PROTRACTED development of component processes. Child Dev. 88, 1015–1032. doi: 10.1111/cdev.12644
Otto, A. R., Gershman, S. J., Markman, A. B., and Daw, N. D. (2013). The curse of planning: dissecting multiple reinforcement-learning systems by taxing the central executive. Psychol. Sci. 24, 751–761. doi: 10.1177/0956797612463080
Roebers, C. M. (2017). Executive function and metacognition: Towards a unifying framework of cognitive self-regulation. Dev. Rev. 45, 31–51. doi: 10.1016/j.dr.2017.04.001
Rovee-Collier, C. (1997). Dissociations in infant memory: rethinking the development of implicit and explicit memory. Psychol. Rev. 104, 467–498. doi: 10.1037/0033-295X.104.3.467
Schulz, E., Wu, C. M., Ruggeri, A., and Meder, B. (2019). Searching for rewards like a child means less generalization and more directed exploration. Psychol. Sci. 30, 1561–1572. doi: 10.1177/0956797619863663
Schulz, L. E., and Bonawitz, E. B. (2007). Serious fun: preschoolers engage in more exploratory play when evidence is confounded. Dev. Psychol. 43, 1045–1050. doi: 10.1037/0012-1649.43.4.1045
Somerville, L. H., Sasse, S. F., Garrad, M. C., Drysdale, A. T., Abi Akar, N., Insel, C., et al. (2017). Charting the expansion of strategic exploratory behavior during adolescence. J. Exp. Psychol. 146, 155–164. doi: 10.1037/xge0000250
Speekenbrink, M. (2022). Chasing unknown bandits: Uncertainty guidance in learning and decision making. Curr. Dir. Psychol. Sci. 31, 419–427. doi: 10.1177/09637214221105051
Wang, J., and Bonawitz, E. (2022). Children's sensitivity to difficulty and reward probability when deciding to take on a task. J. Cogn. Dev. 24, 341–353. doi: 10.1080/15248372.2022.2152032
Wilson, R. C., Geana, A., White, J. M., Ludvig, E. A., and Cohen, J. D. (2014). Humans use directed and random exploration to solve the explore-exploit dilemma. J. Exp. Psychol. Gen. 143, 2074–2081. doi: 10.1037/a0038199
Wittmann, M. K., Scheuplein, M., Gibbons, S. G., and Noonan, M. P. (2023). Local and global reward learning in the lateral frontal cortex show differential development during human adolescence. PLoS Biol. 21:e3002010. doi: 10.1371/journal.pbio.3002010
Wu, C. M., Schulz, E., Speekenbrink, M., Nelson, J. D., and Meder, B. (2018). Generalization guides human exploration in vast decision spaces. Nat. Human Behav. 2, 915–924. doi: 10.1038/s41562-018-0467-4
Zajkowski, W. K., Kossut, M., and Wilson, R. C. (2017). A causal role for right frontopolar cortex in directed, but not random, exploration. Elife 6:e27430. doi: 10.7554/eLife.27430.016
Zelazo, P. D., and Carlson, S. M. (2012). Hot and cool executive function in childhood and adolescence: Development and plasticity. Child Dev. Perspect. 6, 354–360. doi: 10.1111/j.1750-8606.2012.00246.x
Keywords: reinforcement learning, explore-exploit dynamics, executive function, metacognition, child development
Citation: Kim S and Carlson SM (2024) Understanding explore-exploit dynamics in child development: current insights and future directions. Front. Dev. Psychol. 2:1467880. doi: 10.3389/fdpys.2024.1467880
Received: 21 July 2024; Accepted: 26 August 2024;
Published: 23 September 2024.
Edited by:
Catherine Sandhofer, University of California, Los Angeles, United StatesReviewed by:
Elena Escolano-Pérez, University of Zaragoza, SpainCopyright © 2024 Kim and Carlson. This is an open-access article distributed under the terms of the Creative Commons Attribution License (CC BY). The use, distribution or reproduction in other forums is permitted, provided the original author(s) and the copyright owner(s) are credited and that the original publication in this journal is cited, in accordance with accepted academic practice. No use, distribution or reproduction is permitted which does not comply with these terms.
*Correspondence: Seokyung Kim, a2ltMDE0MjZAdW1uLmVkdQ==
Disclaimer: All claims expressed in this article are solely those of the authors and do not necessarily represent those of their affiliated organizations, or those of the publisher, the editors and the reviewers. Any product that may be evaluated in this article or claim that may be made by its manufacturer is not guaranteed or endorsed by the publisher.
Research integrity at Frontiers
Learn more about the work of our research integrity team to safeguard the quality of each article we publish.