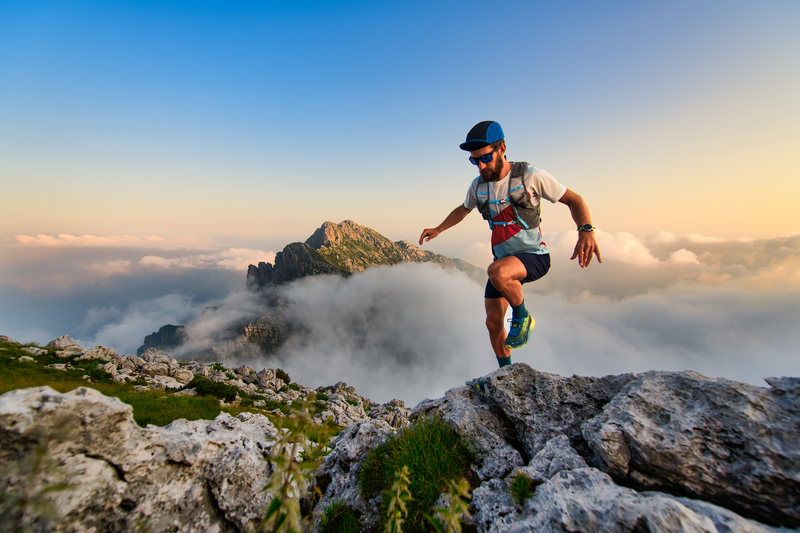
94% of researchers rate our articles as excellent or good
Learn more about the work of our research integrity team to safeguard the quality of each article we publish.
Find out more
ORIGINAL RESEARCH article
Front. Dev. Psychol. , 18 September 2024
Sec. Cognitive Development
Volume 2 - 2024 | https://doi.org/10.3389/fdpys.2024.1371500
Introduction: Self-regulation is important for social as well as academic success, and research shows that it is also shaped by social interactions. At the same time, peers shape children's development in many domains. Linking these two concepts, it can be predicted that children who are surrounded by peers with a high level of self-regulation later show higher self-regulation themselves compared to those who are surrounded by peers with a low level of self-regulation.
Methods: To test this assumption, a longitudinal sample with N = 1,658 participants in 181 classrooms (μage = 8.37) was analyzed. The self-regulation facets of working memory updating, inhibition, and flexibility were measured using an age-appropriate version of the Stroop test, the repeat-digits-backward test, and the Wisconsin card-sorting task. Based on this data, a score for both the individual and class level was calculated.
Results: The results show that the classmates‘ level of self-regulation predicted later individual self-regulation above the individual self-regulation score. While neither age nor gender moderated this relationship, there was a significant cross-level interaction: Especially individuals with a low level of cognitive flexibility gained from being surrounded by peers with a high level of cognitive flexibility.
Discussion: While the results show that peers constitute an important social context for the development of self-regulation, this fact is often only a minor point in modern self-regulation theories.
Peers are often seen as important socialization agents in late childhood and early adolescence. Therefore, it is not surprising that peers influence a child's development across a range of multiple outcomes, such as aggressive behavior (Busching and Krahé, 2015), prosocial behavior (Sullivan et al., 2022), and eating behavior (Salvy et al., 2012). This paper aims to extend the knowledge about peers' influence to include another crucial concept regarding individual development: self-regulation. Self-regulation can be defined as a set of “processes aimed at adjusting mental and physiological state adaptively to context” (Nigg, 2017, p. 364) and encompasses cognitive, affective, and behavioral components. Self-regulation is crucial for academic success, maintaining good social relations, and physical as well as psychological health (Munakata and Michaelson, 2021; Robson et al., 2020). Therefore, it is important to understand which contexts foster a positive development of self-regulation. Despite the fact that peers are an important social context during childhood and adolescence, the role of peers has received little attention in previous research, and no studies investigating self-regulation were included in a recent meta-analysis on peer influences during this age period (Giletta et al., 2021).
Self-regulation is a term in psychology with many different and contradictory definitions (Burman et al., 2015). In this paper, self-regulation is defined as the ability to suppress dominant impulses in order to modify thoughts, feelings, or behavior (Robson et al., 2020). Self-regulation is considered as a concept with a hierarchical structure including both a basal level and a complex level (Nigg, 2017). The basal level is often regarded as crucial for a person to be able to show successful self-regulation. The executive functions can be seen as forming the core of this basal level, which are also required for more complex self-regulation skills, such as planning (Warschburger et al., 2023).
Rating scales and psychological tests often either correlate weakly or even not at all (Diamond, 2013). Toplak et al. (2013) explain that based on repeated findings, psychological tests measure the extent to which an individual is capable of self-regulating, whereas ratings measure the extent to which self-regulation is demonstrated in real life. As these two concepts also draw on different theoretical frameworks (Eisenberg et al., 2019), it does not make sense to blend these two aspects together but rather to investigate them separately. This paper thus focuses on the use of psychological tests to investigate whether an individual is able to show self-regulation.
Also, the construct of executive functions is not one-dimensional, but often encompasses different interrelated facets (Friedman and Miyake, 2017; Nigg, 2017). This paper analyzes three interrelated constructs central to the basal level of self-regulation (Warschburger et al., 2023). The first construct is inhibition. Inhibition refers to the ability “to control one's attention, behavior, thoughts, and/or emotions to override a strong internal predisposition or external lure” (Diamond, 2013, p. 136). Without this ability, it would be impossible to focus on a simple task or maintain a behavior over an extended period of time. One example would be responses in the Stroop task, where a dominant response must be inhibited to enable a non-dominant one (Stroop, 1935).
Working memory updating is the second important construct. Working memory is necessary “to hold and update information in mind” (Diamond, 2013, p. 141). This is needed for academic tasks, where information has to be stored and updated (e.g., math, translations into a different language, etc.), as well as interpersonal situations where a person has to follow a conversation and merge new information with information already given. A commonly used way to measure this construct is to present a list of numbers and ask the participant to repeat the presented numbers in reverse order (Petermann and Petermann, 2007).
Cognitive flexibility is the third construct involved in top-down regulation. Cognitive flexibility refers to the ability to “adjust to changed demands” (Diamond, 2013, p. 147) in a multitude of ways. This can be on the task level by trying a different approach to solving a problem or on the interpersonal level by adopting a different perspective. A common way to measure cognitive flexibility using experimental approaches is to assign participants one simple main task of ordering stimuli and then sometimes giving them a switch command, so that the participants have to sort according to a different rule (Zelazo, 2006).
While it is well-established that self-regulation is influenced by genetics (Friedman and Miyake, 2017; Willems et al., 2019), studies show that self-regulation is also shaped throughout one's life. For example, either explicitly targeted in interventions (Murray et al., 2016) or implicitly through parental behavior (Valcan et al., 2018), the social context shapes self-regulation. Similar results are reported for children in kindergarten: If they are surrounded by peers with a high level of self-regulation they will also show a later increase in self-regulation (Montroy et al., 2016; Korucu et al., 2023). Basic learning processes such as modeling are possible theoretical explanations for the influence of others on the development of self-regulation (Miller-Slough and Dunsmore, 2016). For instance, observing another person demonstrate a high level of self-regulation can lead to an increase in self-regulation (Orange, 1999).
Munakata and Michaelson (2021) argue that social norms play an important role in explaining whether or not self-regulation is shown in school settings. The degree of self-regulation functions depends on whether they are rewarded (Adam and Vogel, 2016; Munakata and Michaelson, 2021). For children and adolescents, their peers' reaction often shape their behavior (Rohlf et al., 2016), since the behavior and reactions work as a reward (Henry et al., 2000). It is reasonable to assume that peers' behavior works also as a reward for self-regulation.
Peers form an important social context for children's development, as the majority of children and adolescents spend most of their non-sleeping time with their peers (Rubin et al., 2015). This often includes different, but overlapping social contexts, such as sports clubs, friends, and other extracurricular activities. However, one particular social context stands out in two ways: the classroom. (1) In Germany, while parents are free to choose their children's school, they typically have no say in which of the classrooms in those schools the children are placed. Since the students are assigned by their respective school administration, it is possible to investigate socializing effects while minimizing selection effects in a natural setting. (2) The German school system is structured so that during school, students spend nearly all their time with the same students in the same classroom. This often extends into breaks, where students primarily interact only with students from their own classrooms. Further, classrooms are normally stable over several years. Students changing classrooms within a school is a rare exception. This high degree of temporal stability offers the opportunity to investigate peer influences that unfold over a long time in a largely constant social environment with very limited selection effects.
There are currently only a few studies that focus on the impact of classmates on the development of self-regulation during late childhood. The results of these studies are mixed. While Reid and Ready (2022), Barnes et al. (2022), and Wei et al. (2021) reported that higher class levels of self-regulation predicted higher individual levels of self-regulation later, Zakszeski et al. (2020) found no significant relationship between the class level of self-regulation and individual self-regulation. The results of Finch et al. (2019) were also mixed. While being surrounded by classmates with a higher level of self-regulation did predict lower reaction times in self-regulation tasks, no similar results were found for accuracy in the same self-regulation tasks. However, most of the studies focused only on working memory updating or used aggregated values across different self-regulation scales and did not include inhibition. With the exception of Wei et al. (2021), who collected data in Brazil, all data was collected in the United States. Therefore, further research is needed to test the robustness of the findings across different facets of self-regulation, as well as their different operationalizations and cultures.
Another important issue is the identification of potential moderators. Even very old theories explaining the influence of social context on individual development do not predict that everybody will be affected by the social context in the same way. On the contrary, they suggest that the impact of the social context often depends on individual characteristics (e.g., Bronfenbrenner, 1979; Newcomb and Charters, 1950). However, precisely which individual characteristics shape this relationship is still under discussion and is rarely empirically tested. As all the previous studies were conducted on different samples, it can be argued that the divergent results are due to different sample characteristics. Therefore, the second part of this paper investigates whether the relationship between class-level self-regulation and individual self-regulation over time is moderated by individual characteristics. Based on theoretical considerations, age, gender, and the initial level of self-regulation skills will be taken into account.
Both the overall level and the structure of self-regulation change during late childhood and early adolescence. As the overall level of self-regulation increases, the number of different self-regulation facets also increases, which leads to a much more complex structure (Nigg, 2017). The age range in previously presented research is between 5.7 and 9.9 years. These ages are particularly sensitive to peer influences, as well as to the development of self-regulation. One important developmental process during this period is that peers become more important, while parents and other members of the immediate family become less important (Walper et al., 2018). Since learning theories suggest that especially important models have a higher influence on individual behavior, age could be a moderator. On the other hand, however, a meta-analysis of peer influence during adolescence for a range of different behaviors does not show that age is a moderator (Giletta et al., 2021).
Another possible moderator of interest is gender. Although all the above-mentioned studies included gender as a control variable, none investigated systematically whether girls or boys were more affected by their peers. However, friendships are qualitatively different between girls and boys (Rose et al., 2021), and for other behavior domains peer influence seems to be moderated by gender (for example, deviant behavior; Busching and Krahé, 2018). Since peer interactions are structured differently in these two gender groups, learning experiences in these interactions could also be different, which would lead to moderating effects of gender.
Finally, an important individual-level moderator is the person's initial level of self-regulation. One can argue that individuals with a low level of self-regulation gain more than their peers, as they have a greater possibility to increase their level. Conversely, individuals with higher self-regulation levels may find learning new things easier, as suggested by Robson et al. (2020), and thus could benefit more from being among peers with similarly high self-regulation levels. This implies that students with higher self-regulation may particularly benefit from being in such an environment. To test these opposing viewpoints, it is crucial to consider initial self-regulation as a potential moderating factor.
Self-regulation is central to academic achievement in school, as well as to social interactions with peers and teachers (Robson et al., 2020). Therefore, it is important to investigate which factors influence the development of self-regulation. School can be characterized as a social setting where most children and adolescents spend a considerable amount of time interacting with their peers. Therefore, it seems fruitful to examine how this social context shapes the development of self-regulation. From a learning theory perspective, it can be assumed that an environment that offers a variety of opportunities to observe a certain behavior, and where that behavior is also associated with positive effects, will contribute to the behavior being more frequently exhibited by members of that social environment (Bandura, 2000). If this theoretical assumption holds, we should find evidence to support the following hypothesis:
H1: The level of classmates' self-regulation prospectively predicts individual self-regulation.
Two additional unanswered research questions will also be addressed in this paper. So far, most studies investigating this link have either focused on one measure of self-regulation or combined multiple measures of self-regulation, and therefore have not considered the potentially different effects of the individual measures. While the theory suggests that comparable effects should be found across the different facets of self-regulation, this relationship has not been systematically tested to date. On the other hand, the relationships between variables after aggregation over the multiple sub-constructs of self-regulation are stronger (e.g., Valcan et al., 2018; Friedman and Miyake, 2017), which is often done since the psychometric properties of the psychological tests employed are not ideal. Aggregating over the different facets gives a more reliable score, making it possible to identify weaker effects, and offers the possibility to compare the results with other published results.
RQ1: Does this relationship hold for different facets and the total score?
Therefore, we use a two-pronged approach. We investigate the single facets to test for differential effects, as well as the combination of all facets, to yield a total score which gives a more reliable measure and allows us to compare the current results with those reported in the literature.
As discussed above, existing findings are highly diverse, and neglect of potential moderators could be the reason for such diverse results. Therefore, this paper will test the moderating influence of individual differences, namely age, gender, and individual self-regulation scores at T1, to answer the second research question.
RQ2: Do age, gender, and individual self-regulation moderate the link between class-level self-regulation and later individual self-regulation?
To address this research gap, the current study uses a large longitudinal data set where it is possible to investigate the effects of the different facets simultaneously. Using non-experimental data always comes with the challenge of controlling for possible confounders. One approach to do this is longitudinal modeling, where all cofounders that are constant over the investigated time period are automatically controlled (Singer and Willett, 2003). Since all participants were recruited together with their classmates, multilevel modeling can be used to investigate the classroom effects (Hox et al., 2017).
A total of 1,657 students participated in this longitudinal study. At T1, they belonged to 181 different classes in 33 public elementary schools in the federal state of Brandenburg.1 To obtain a representative sample, schools in urban, suburban, and rural areas were recruited. This means that each class was represented, on average, by 9.17 students (SD = 4.13). At T1, the mean age of the sample was 8.36 years (SD = 0.95), and 794 (48%) of the participants identified themselves as female. It was not possible to collect data about skin color or ethnicity; therefore, we collected as a proxy the language spoken at home. Most of the participants reported speaking German at home (93%). The mean time difference between the first measurement (T1) and the second (T2) was 8.95 months. At T2, 1,612 children were still participating in this study and only 45 (3%) dropped out. A more detailed description of the sampling and recruitment process can be found in Warschburger et al. (2023). Nearly no one changed the social context between T1 and T2. The coefficient of contingency between T1 class-membership and T2 class-membership indicated a very high stability was c = 0.997.
Self-regulation was operationalized by three measures of executive functioning established in the literature.
To assess inhibition, an age-appropriate paper-pencil version of the Stroop test was used (Röthlisberger et al., 2010; Roebers et al., 2011), whereby the participants had to name the colors of different fruits. The first page showed colored rectangles, the second page correctly colored fruits and vegetables (e.g., a yellow banana), the third page fruits and vegetables colored gray, and the last page showed the fruits and vegetables colored incorrectly (e.g., a blue banana). For pages 1 and 2, participants were asked to name the color. For pages 3 and 4, participants were asked to name the colors the objects normally have. For each page, the number of correctly named objects was recorded. The interference score was calculated according to the following formula: time page 4 – ((time page 1 * time page 3)/(time page 1 + time page 3). Additionally, the score was reversed, so that a higher score indicated a higher level of inhibition; the direction of this measure is in line with the other self-regulation measures. While it was not possible to calculate reliabilities for the sample of this study, the authors of the original English version (Archibald and Kerns, 1999) report very good retest reliabilities (r was between 0.87 and 0.93). For the German adaptation, a CFA supported the one-dimensional structure (Röthlisberger et al., 2010).
To measure working memory updating, the participants had to repeat digits backward (Petermann and Petermann, 2007). The number of digits was increased until two consecutive trials could not be repeated successfully. As a score, the total number of correct answers was calculated, where a higher score indicated a higher level of working memory updating. While we could not assess internal consistency, other studies report a high retest reliability (r = 0.71; Müller et al., 2012).
Flexibility was assessed using an age-modified version of the Wisconsin card-sorting task (Röthlisberger et al., 2010). The participants saw two differently colored families of fish on a screen and were instructed to feed them alternately. To feed them, the participants had to press a key according to the position of the fish on the screen. In most trials, each family was on its own side of the screen, but in some trials the fish switched places, so that the participants also had to change their reactions. As a measure, the number of correct responses in the switch trials was calculated. Higher scores indicated a higher level of cognitive flexibility. The reported split-half reliability of this test was also high (r = 0.70 Röthlisberger et al., 2010).
The total score was calculated from the individual task scores by averaging the standardized flexibility, inhibition, and working memory updating for each individual. A Bayesian multilevel CFA was conducted to check whether all indicators loaded on the same factor for T1. The PPP = 0.519 and the CI was [−16.246; 16.150], which indicated a good fit. Similar results were obtained for T2 [PPP =0.52; CI = [−17.3; 19.65]]. Evidence for strong measurement invariance was also found.
Gender and age were reported by the participants. Information about participants' classroom membership was provided by the school administration.
The sample was recruited using a clustered sampling approach. Schools were contacted by the study group, which then contacted individual teachers. The teachers distributed information to the participants and their parents. Parents had to give written consent, students had to give assent. All tests were administered individually by trained research assistants. While most participants were assessed in school, some participants were assessed at home or at the University of Potsdam. Since the above-described tests were part of a larger data collection procedure each participant was contacted twice to ensure that each session did not took more than 1 h. To control for possible order effects the order of the tests was randomized between participants. The procedure was approved by the ethics committee of the University of Potsdam, as well as the Ministry of Education, Youth, and Sport of the Federal State of Brandenburg, Germany. A complete description of the data collection process can be found in Warschburger et al. (2023).
As a first step, all test data was z-standardized using the pooled mean and standard deviation across the two time points. No group mean centering was conducted. This was to ensure that the different measures were comparable but the time effects could still be observed. As a measure for the classmates' self-regulation, the mean level of each classroom for flexibility, working memory updating, and inhibition was calculated. This approach would yield reliable and valid scores for classes even if only a small number from each class participated (Lüdtke et al., 2008). Although a minimum number of students per class was not set in the reported analyses, the robustness of the results was verified by setting a minimum threshold of five students per class, confirming the consistency of the outcomes. Since only 3% of the participants were missing at T2, we used a complete case analysis. This approach results in unbiased regression coefficients given data Missing at Random (MAR) as well as Missing Completely at Random (MCAR; Little, 2024), which are the focus of this paper.
The models were estimated using the lme4 package in R (Bates et al., 2012). Since the Shapiro-Wilk's test was significant for all variables and the kurtosis exceeded three, residual bootstrapping was used to estimate the confidence intervals as implemented in lmeresampler (Loy et al., 2022). This approach does not require a normal distribution and yields robust results. To assess significance, the 95% confidence interval was inspected. Since the data is highly skewed, all figures include both the raw values and the percentile scores.
A power analysis was conducted using the R-package mlmpower (Keller, 2023). For effect sizes >0.1, the power consistently exceeded >0.95 for within-subject effects, between-subject effects, and cross-level interactions. However, the simulations also revealed that, due to the low variance at the class level attributed to the small intraclass correlation coefficient (ICC), there was a high likelihood of negative variance occurring, especially after adding additional predictors.
The descriptive results at the individual level are displayed in Table 1. Across all three different self-regulation facets, mean scores increased from T1 to T2. This pattern can be attributed to the higher age at T2 indicating an increase in self-regulation with age, which is in line with the positive correlation of the self-regulation facets and age. The correlations among the three different facets of self-regulation are medium in size and always positive, as expected.
For the class level, comparable information is displayed in Table 2, where the descriptives for the class-level scores are depicted. The variance is slightly lower compared to the individual level, whereas the correlations are slightly higher compared to the individual level. Additionally, the intraclass correlations (ICCs) are included in Table 2. ICCs give a relative explanation of the total variance by individual characteristics and by the class-level information. For all six variables, the confidence interval for the variances is >0, showing that the class level adds to the explanation of self-regulation and should be taken into account. Their size is between 0.10 and 0.20, which is normally expected for variables collected in school settings (Hedges and Hedberg, 2007).
The first hypothesis was investigated using a multilevel model that predicted each of the self-regulation facets, as well as the total score at T2. The respective individual and classroom scores were used as predictors. Additionally, age and gender were included as control variables, and classes were allowed to differ in their mean scores. The results are shown in Table 3.
Table 3. Predicting later individual executive functions by individual and class-level score, controlling for age and gender.
For flexibility and working memory updating, a higher class mean at T1 predicted a higher level of individual self-regulation, controlling for the individual score at T1. No significant association with the class-level score at T1 and the individual scores at T2 was found for inhibition. These findings lend qualified support to Hypothesis 1. Additionally, participants with a higher level of individual self-regulation at T1 also reported a higher level of self-regulation at T2 for all individual facets. While girls and older children reported a higher level of inhibition at T2, these two control variables did not significantly predict the T2 scores for flexibility and working memory updating.
To answer the first research question, additionally the total score was calculated. The findings were comparable. The individual score at T1 predicted the one at T2, but neither age nor gender predicted T2. However, the key finding was that students who were surrounded by peers with a higher level of self-regulation skills did show a better performance at T2, 8 months later. This underscores that, besides the individual level of self-regulation, the average level in the classroom is predictive too.
The second research question focused on whether the above results hold for everyone or are affected by individual variables. To accomplish this, the models in the prior paths were extended by adding cross-level interaction terms. The entire tables are available in the Supplementary Tables S1–S3.
The first moderator of interest was gender. The relationship between class-level self-regulation and later individual self-regulation was not moderated by participant gender for any of the three facets or the total score. This means that the classmates' influence seemed similar for boys and girls. A similar picture was found for age. Also, this moderator did not influence the relationship between class-level self-regulation at T1 and self-regulation at T2, since none of the interaction effects with age turned out to be significant.
However, for the T1 score of individual self-regulation, a slightly different picture emerged. While no significant interaction was found for inhibition, working memory updating, or the total score, the interaction between individual flexibility at T1 and class-level flexibility at T1 significantly predicted later individual flexibility. This interaction is plotted in Figure 1. It shows that for participants with a low level of individual flexibility the two lines for classroom flexibility differ, while for participants with a high level of individual flexibility the two lines meet at the same point. This means that students with a low individual level of flexibility gained from being surrounded by peers with a high level of flexibility, while students with a high level of flexibility did not seem to be affected by their peers. To provide a more comprehensive view, a Johnson-Neyman (JN) chart (McCabe et al., 2018) was created in addition to the simple interaction plots, which only visualize interaction terms over two or three discrete values, which allows to visualize the interaction term over the complete range of both variables. In Figure 2 on the x-axis the individual level flexibility is plotted, while on the y-axis the magnitude of the regression-coefficient of class-level flexibility is plotted. The idea behind this visualization, is that an interaction can be conceptualized, that the impact of one variable depends on the value of a second one. This figure shows that the higher the individual cognitive flexibility, the smaller is the impact their class mates. The classmates had a significant impact for the lower 60% of the sample, while above this threshold the coefficient was still positive, but not significant.
Figure 1. Interaction between individual and class-level flexibility at T1 predicting flexibility at T2.
The process of developing self-regulation is multifaceted, being shaped by a confluence of genetic factors and social interactions. Notably, during the critical phases for the development of self-regulation in late childhood and early adolescence, peers emerge as significant agents of influence. This article is dedicated to exploring the extent to which peers' self-regulatory behaviors predict subsequent self-regulation in children. Additionally, it aims to discern whether specific subgroups of children derive greater benefits from being in the presence of individuals exhibiting high levels of self-regulation. It was hypothesized that class-level self-regulation would predict later individual-level self-regulation, controlling for individual stability as well as age and gender as control variables that might influence self-regulation. Except for inhibition, the results did support the prediction. For inhibition, no relationship between the class-level and later individual level-scores was found.
The theoretical basis of the central hypothesis was the assumption that peer effects are a result of observational learning (Bandura, 2000). Except for inhibition, the result pattern supports this theoretical basis. This emphasizes that self-regulation is also shaped by social interactions during late childhood. Currently, many theories focusing on self-regulation see the social context as playing only a minor role (e.g., Nigg, 2017; Carver and Scheier, 2016). However, the results show that social interactions should be incorporated into theories focusing on the development of self-regulation. Extending these theories could enable the formulation of clearer hypotheses and the prediction of which variables might act as moderators. At the moment, the explanation of moderators is still mostly on a one by one basis and the reasoning behind the moderators is not connected.
The fact that no significant results for inhibition were found is an important deviation from this overall pattern, since there is a lack of previous studies investigating inhibition as a specific facet of self-regulation. One explanation for this divergent pattern is that inhibition is more stable across the investigated age range (Laureys et al., 2021; Davidson et al., 2006). Stable constructs should be much less influenced by other factors. This notion is also supported by other studies which investigated social influences on inhibition during this age period and also did not report significant results (e.g., video games; Colzato et al., 2013). However, to really test this explanation, a larger age range in a similar setting would be necessary.
One research question asked whether the relationships between class-level self-regulation and the facets and total score of individual-level self-regulation are moderated by participants' age, gender, and self-regulation at T1. No moderation of initial self-regulation was found for impulsivity or working memory updating, or for the total score. The missing moderation effects would be in line with simple learning principle. Since the basic observational learning principles apply to everyone in a similar fashion, the finding that no moderation was found would support this theory (Skinner, 1974; Klein, 2012).
One significant interaction emerged: Figure 1 shows that participants with a lower initial level of flexibility gained more from being surrounded by highly flexible people, while students with higher initial levels of flexibility profited less. One possible explanation could be that a high level of flexibility is a protective factor against negative influences (e.g., Stepanyan et al., 2020; Harel et al., 2023). Since students with a high level of self-regulation should be above the mean level of self-regulation in that class, the effect of their follow students should be smaller or even non-existent. However, since this effect was small, a replication with a different data set seems necessary.
One limitation is that no data regarding the influence of teachers' behavior was available. Prior research has shown that the structure of lessons delivered by the teacher can influence the development of an individual's self-regulation (Schunk et al., 2022). However, longitudinal modeling controls for missing control variables which are stable over the investigated time period. Teachers normally stay with the same classes in Germany during that age period (Döbert, 2015), so teacher influence should not impact the results. Nevertheless, it is important to keep in mind that, although longitudinal modeling was used, no causal inference can be drawn. The only ethical experimental approach that would allow causal interference would be to train some of the students in a class to self-regulate and afterwards measure the spill-over effect on the untrained participants in the same class.
The time gap between the two measurement points was 8–9 months. The specific choice for the spacing between measurements influences the results (Driver et al., 2017). However, increasing the time interval can increase stability coefficients as well as reduce them, depending on the underlying processes. One possible solution to this problem would be to use Continuous Time Structural Equation Modeling (Voelkle et al., 2012).
One problem with this study, as well as most other studies (e.g., Wei et al., 2021), is that they are not designed with the intention of investigating group influences; rather, these analyses are often added post-hoc. This can lead to the problem that, often, instruments are employed that are not specifically designed for this use, or individual interactions between students are measured. While this applies also to this study, the use of well-validated psychological tests that measure self-regulation enables a comparison with the results on self-regulation in other contexts.
One point that should be addressed in future research is the investigation of the processes involved. Although the main hypothesis was based on observational learning theory and the results are in line with this theory, alternative explanations or other theoretical models should not be dismissed. Therefore, it seems advisable to include more fine-grained measures (e.g., including questionnaires assessing normative beliefs about self-regulation; Bandura, 2000) that allow researchers to investigate the different processes in more detail in future studies, as an understanding of the processes involved is essential for developing interventions (Glanz and Bishop, 2010).
The practical implications of these findings are multifaceted. First, they suggest that interventions aimed at improving self-regulation in children should mix children with different levels of self-regulation to leverage the benefits of observational learning. Since self-regulation is central to most activities (Robson et al., 2020), it can be assumed that children observe and learn from the self-regulation behaviors of their peers, even when self-regulation is not the primary focus of the group. This is particularly important for cognitive flexibility, as children with a high level of cognitive flexibility do not appear to experience detrimental effects from being surrounded by peers with lower levels of cognitive flexibility. This indicates that such mixed-level groupings can be beneficial without the risk of negatively impacting those who already exhibit strong self-regulation skills.
It is a widely established finding that a high level of self-regulation is important for individual development (Robson et al., 2020). Therefore, it seems sensible to test whether the changes in self-regulation also translate into other behaviors that are linked to self-regulation. This becomes even more important since self-regulation can be trained inside and outside of school (Karbach and Kray, 2016). Using approaches to train self-regulation for students who are in school would not only directly benefit the students who receive the training, but could also benefit other, untrained students in the same class.
The data analyzed in this study is subject to the following licenses/restrictions: the data will be made available to the scientific community after the completion of the PIER-Study. Requests to access these datasets should be directed to cGllckB1bmktcG90c2RhbS5kZQ==.
The studies involving humans were approved by Ethikkommission der Universität Potsdam and the Ministry of Education, Youth and Sport of the Federal State of Brandenburg, Germany. The studies were conducted in accordance with the local legislation and institutional requirements. Written informed consent for participation in this study was provided by the participants' legal guardians/next of kin.
RB: Conceptualization, Data curation, Funding acquisition, Investigation, Methodology, Resources, Software, Supervision, Validation, Visualization, Writing – original draft, Writing – review & editing.
The author(s) declare financial support was received for the research, authorship, and/or publication of this article. The article was funded by the DFG (project number 426314138).
The author wish to thank Barbara Krahé, Petra Warschburger, and Nele Westermann for helpful comments on an earlier version of this article.
The author declares that the research was conducted in the absence of any commercial or financial relationships that could be construed as a potential conflict of interest.
All claims expressed in this article are solely those of the authors and do not necessarily represent those of their affiliated organizations, or those of the publisher, the editors and the reviewers. Any product that may be evaluated in this article, or claim that may be made by its manufacturer, is not guaranteed or endorsed by the publisher.
The Supplementary Material for this article can be found online at: https://www.frontiersin.org/articles/10.3389/fdpys.2024.1371500/full#supplementary-material
1. ^For privacy reasons, it was not possible to collect any data about individuals who chose not to participate in this study.
Adam, K. C. S., and Vogel, E. K. (2016). Reducing failures of working memory with performance feedback. Psychon. Bull. Rev. 23, 1520–1527. doi: 10.3758/s13423-016-1019-4
Archibald, S. J., and Kerns, K. A. (1999). Identification and description of new tests of executive functioning in children. Child Neuropsychol. 5, 115–129. doi: 10.1076/chin.5.2.115.3167
Bandura, A. (2000). “Social-cognitive theory,” in Encyclopedia of Psychology, ed. A. E. Kazdin (Washington, DC: American Psychological Association; Oxford University Press), 329–332.
Barnes, S. P., Jones, S. M., and Bailey, R. (2022). An ecological view of executive function in young children: variation in and predictors of executive function skills over one school year. Dev. Sci. 26:e13355. doi: 10.1111/desc.13355
Bates, D., Maechler, M., and Bolker, B. (2012). lme4: Linear Mixed-Effects Models Using S4 Classes. Wien: Comprehensive R Archive Network.
Bronfenbrenner, U. (1979). The Ecology of Human Development: Experiments by Nature and Design. Cambridge, MA: Harvard University Press.
Burman, J. T., Green, C. D., and Shanker, S. (2015). On the meanings of self-regulation: digital humanities in service of conceptual clarity. Child Dev. 86, 1507–1521. doi: 10.1111/cdev.12395
Busching, R., and Krahé, B. (2015). The girls set the tone: gendered classroom norms and the development of aggression in adolescence. Pers. Soc. Psychol. Bull. 41, 659–676. doi: 10.1177/0146167215573212
Busching, R., and Krahé, B. (2018). The contagious effect of deviant behavior in adolescence. Soc. Psychol. Personal. Sci. 9, 815–824. doi: 10.1177/1948550617725151
Carver, C. S., and Scheier, M. (2016). “Self-regulation of action and affect,” in Handbook of Self-Regulation: Research, Theory, and applications, 3rd Edn, paperback edition, eds. K. D. Vohs, and R. F. Baumeister (New York, NY; London: The Guilford Press), 3–23.
Colzato, L. S., van den Wildenberg, W. P. M., Zmigrod, S., and Hommel, B. (2013). Action video gaming and cognitive control: playing first person shooter games is associated with improvement in working memory but not action inhibition. Psychol. Res. 77, 234–239. doi: 10.1007/s00426-012-0415-2
Davidson, M. C., Amso, D., Anderson, L. C., and Diamond, A. (2006). Development of cognitive control and executive functions from 4 to 13 years: evidence from manipulations of memory, inhibition, and task switching. Neuropsychologia 44, 2037–2078. doi: 10.1016/j.neuropsychologia.2006.02.006
Diamond, A. (2013). Executive functions. Annu. Rev. Psychol. 64, 135–168. doi: 10.1146/annurev-psych-113011-143750
Döbert, H. (2015). “Germany,” in The Education Systems of Europe, 2nd Edn (Cham: Springer International Publishing), 305–333.
Driver, C. C., Oud, J. H. L., and Voelkle, M. C. (2017). Continuous time structural equation modeling with R package ctsem. J. Stat. Soft. 77, 1–35. doi: 10.18637/jss.v077.i05
Eisenberg, I. W., Bissett, P. G., Zeynep Enkavi, A., Li, J., Mackinnon, D. P., Marsch, L. A., et al. (2019). Uncovering the structure of self-regulation through data-driven ontology discovery. Nat. Commun. 10:2319. doi: 10.1038/s41467-019-10301-1
Finch, J. E., Garcia, E. B., Sulik, M. J., and Obradovi,ć, J. (2019). Peers matter: links between classmates' and individual students' executive functions in elementary school. AERA Open 5, 1–14. doi: 10.1177/2332858419829438
Friedman, N. P., and Miyake, A. (2017). Unity and diversity of executive functions: individual differences as a window on cognitive structure. Cortex 86, 186–204. doi: 10.1016/j.cortex.2016.04.023
Giletta, M., Choukas-Bradley, S., Maes, M., Linthicum, K. P., Card, N. A., and Prinstein, M. J. (2021). A meta-analysis of longitudinal peer influence effects in childhood and adolescence. Psychol. Bull. 147, 719–747. doi: 10.1037/bul0000329
Glanz, K., and Bishop, D. B. (2010). The role of behavioral science theory in development and implementation of public health interventions. Annu. Rev. Public Health 31, 399–418. doi: 10.1146/annurev.publhealth.012809.103604
Harel, O., Hemi, A., and Levy-Gigi, E. (2023). The role of cognitive flexibility in moderating the effect of school-related stress exposure. Sci. Rep. 13:5241. doi: 10.1038/s41598-023-31743-0
Hedges, L. V., and Hedberg, E. C. (2007). Intraclass correlation values for planning group-randomized trials in education. Educ. Eval. Policy Anal. 29, 60–87. doi: 10.3102/0162373707299706
Henry, D., Guerra, N., Huesmann, L. R., Tolan, P., VanAcker, R., and Eron, L. (2000). Normative influences on aggression in urban elementary school classrooms. Am. J. Commun. Psychol. 28, 59–81. doi: 10.1023/A:1005142429725
Hox, J. J., Moerbeek, M., and Van De Schoot, R. (2017). Multilevel Analysis, 3rd Edn. New York, NY: Routledge.
Karbach, J., and Kray, J. (2016). “Executive functions,” in Cognitive Training, eds. T. Strobach, and J. Karbach (Cham: Springer International Publishing), 93–103.
Keller, B. T. (2023). mlmpower: Power Analysis and Data Simulation for Multilevel Models. Available at: https://CRAN.R-project.org/package=mlmpower (accessed December 20, 2023).
Korucu, I., Paes, T. M., Costello, L. A., Duncan, R. J., Purpura, D. J., and Schmitt, S. A. (2023). The role of peers' executive function and classroom quality in preschoolers' school readiness. J. Appl. Dev. Psychol. 86:101532. doi: 10.1016/j.appdev.2023.101532
Laureys, F., Middelbos, L., Rommers, N., Waelle, S., de Coppens, E., Mostaert, M., et al. (2021). The effects of age, biological maturation and sex on the development of executive functions in adolescents. Front. Physiol. 12:703312. doi: 10.3389/fphys.2021.703312
Little, R. J. (2024). Missing data analysis. Annu. Rev. Clin. Psychol. 20, 149–173. doi: 10.1146/annurev-clinpsy-080822-051727
Loy, A., Steele, S., and Korobova, J. (2022). lmeresampler: Bootstrap Methods for Nested Linear Mixed-Effects Models. Available at https://cran.r-project.org/package=lmeresampler (accessed September 2, 2024).
Lüdtke, O., Marsh, H. W., Robitzsch, A., Trautwein, U., Asparouhov, T., and Muthén, B. O. (2008). The multilevel latent covariate model. A new, more reliable approach to group-level effects in contextual studies. Psychol. Methods 13, 203–229. doi: 10.1037/a0012869
McCabe, C. J., Kim, D. S., and King, K. M. (2018). Improving present practices in the visual display of interactions. Adv. Methods Pract. Psychol. Sci. 1, 147–165. doi: 10.1177/2515245917746792
Miller-Slough, R. L., and Dunsmore, J. C. (2016). Parent and friend emotion socialization in adolescence: associations with psychological adjustment. Adolesc. Res. Rev. 1, 287–305. doi: 10.1007/s40894-016-0026-z
Montroy, J. J., Bowles, R. P., and Skibbe, L. E. (2016). The effect of peers' self-regulation on preschooler's self-regulation and literacy growth. J. Appl. Dev. Psychol. 46, 73–83. doi: 10.1016/j.appdev.2016.09.001
Müller, U., Kerns, K. A., and Konkin, K. (2012). Test-retest reliability and practice effects of executive function tasks in preschool children. Clin. Neuropsychol. 26, 271–287. doi: 10.1080/13854046.2011.645558
Munakata, Y., and Michaelson, L. E. (2021). Executive functions in social context: implications for conceptualizing, measuring, and supporting developmental trajectories. Annu. Rev. Dev. Psychol. 3:139. doi: 10.1146/annurev-devpsych-121318-085005
Murray, D. W., Rosanbalm, K., and Christopoulos, K. (2016). Self-Regulation and Toxis Stress Report 3: A Comprehensive Review of Self-Regulation Interventions From Birth Through Young Adulhood. Washington, DC: Office of Planning, Research and Evaluation, Administration for Children and Families.
Newcomb, T. M., and Charters, W. W. (1950). “Change and persistence of attitudes,” in Social Psychology, eds. T. M. Newcomb, and W. W. Charters (Ft Worth, TX: Dryden Press), 194–232.
Nigg, J. T. (2017). Annual research review: On the relations among self-regulation, self-control, executive functioning, effortful control, cognitive control, impulsivity, risk-taking, and inhibition for developmental psychopathology. J. Child Psychol. Psychiatry 58, 361–383. doi: 10.1111/jcpp.12675
Orange, C. (1999). Using peer modeling to teach self-regulation. J. Exp. Educ. 68, 21–39. doi: 10.1080/00220979909598492
Petermann, F., and Petermann, U. (2007). Hamburg-Wechsler-Intelligenztest für Kinder IV (HAWIK-IV). Bern: Huber.
Reid, J. L., and Ready, D. D. (2022). Heterogeneity in the development of executive function: evidence from nationally representative data. Early Educ. Dev. 33, 243–267. doi: 10.1080/10409289.2020.1866949
Robson, D. A., Allen, M. S., and Howard, S. J. (2020). Self-regulation in childhood as a predictor of future outcomes: a meta-analytic review. Psychol. Bull. 146, 324–354. doi: 10.1037/bul0000227
Roebers, C. M., Röthlisberger, M., Cimeli, P., Michel, E., and Neuenschwander, R. (2011). School enrolment and executive functioning: a longitudinal perspective on developmental changes, the influence of learning context, and the prediction of pre-academic skills. Eur. J. Dev. Psychol. 8, 526–540. doi: 10.1080/17405629.2011.571841
Rohlf, H., Krahé, B., and Busching, R. (2016). The socializing effect of classroom aggression on the development of aggression and social rejection: a two-wave multilevel analysis. J. Sch. Psychol. 58, 57–72. doi: 10.1016/j.jsp.2016.05.002
Rose, A. J., Borowski, S. K., Spiekerman, A., and Smith, R. L. (2021). “Children's Friendships,” in The Wiley-Blackwell Handbook of Childhood Social Development, 3rd Edn, eds. P. K. Smith, C. H. Hart, P. K. Smith, and C. H. Hart. (Hoboken NJ: Wiley-Blackwell), 487–502.
Röthlisberger, M., Neuenschwander, R., Michel, E., and Roebers, C. M. (2010). Exekutive Funktionen: Zugrundeliegende kognitive Prozesse und deren Korrelate bei Kindern im späten Vorschulalter. Zeitschrift Entwicklungspsychol. Pädagogische Psychol. 42, 99–110. doi: 10.1026/0049-8637/a000010
Rubin, K. H., Bukowski, W. M., and Bowker, J. C. (2015). “Children in peer groups,” in Handbook of Child Psychology and Developmental Science: Ecological Settings and Processes, Vol. 4, 7th Edn, eds. R. M. Lerner, M. H. Bornstein, and T. Leventhal (Hoboken, NJ: John Wiley & Sons, Inc), 175–222.
Salvy, S.-J., La Haye, K., de Bowker, J. C., and Hermans, R. C. J. (2012). Influence of peers and friends on children's and adolescents' eating and activity behaviors. Physiol. Behav. 106, 369–378. doi: 10.1016/j.physbeh.2012.03.022
Schunk, D., Berger, E. M., Hermes, H., Winkel, K., and Fehr, E. (2022). Teaching self-regulation. Nat. Hum. Behav. 6, 1680–1690. doi: 10.1038/s41562-022-01449-w
Singer, J. D., and Willett, J. B. (2003). Applied Longitudinal Data Analysis: Modeling Change and Event Occurrence. Oxford; New York, NY: Oxford University Press.
Stepanyan, S. T., Natsuaki, M. N., Cheong, Y., Hastings, P. D., Zahn-Waxler, C., and Klimes-Dougan, B. (2020). Early pubertal maturation and externalizing behaviors: Examination of peer delinquency as mediator and cognitive flexibility as a moderator. J. Adolesc. 84, 45–55. doi: 10.1016/j.adolescence.2020.07.008
Stroop, J. R. (1935). Studies of interference in serial verbal reactions. J. Exp. Psychol. 18, 643–662. doi: 10.1037/h0054651
Sullivan, N. J., Li, R., and Huettel, S. A. (2022). Peer presence increases the prosocial behavior of adolescents by speeding the evaluation of outcomes for others. Sci. Rep. 12:6477. doi: 10.1038/s41598-022-10115-0
Toplak, M. E., West, R. F., and Stanovich, K. E. (2013). Practitioner review: do performance-based measures and ratings of executive function assess the same construct? J. Child Psychol. Psychiatry 54, 131–143. doi: 10.1111/jcpp.12001
Valcan, D. S., Davis, H., and Pino-Pasternak, D. (2018). Parental behaviours predicting early childhood executive functions: a meta-analysis. Educ. Psychol. Rev. 30, 607–649. doi: 10.1007/s10648-017-9411-9
Voelkle, M. C., Oud, J. H. L., Davidov, E., and Schmidt, P. (2012). An SEM approach to continuous time modeling of panel data: relating authoritarianism and anomia. Psychol. Methods 17, 176–192. doi: 10.1037/a0027543
Walper, S., Lux, U., and Witte, S. (2018). “Sozialbeziehungen zur Herkunftsfamilie,” in Entwicklungspsychologie des Jugendalters, ed. A. Lohaus (Berlin, Heidelberg: Springer), 114–137.
Warschburger, P., Gmeiner, M. S., Bondü, R., Klein, A. M., Busching, R., and Elsner, B. (2023). Self-regulation as a resource for coping with developmental challenges during middle childhood and adolescence: the prospective longitudinal PIERYOUTH-study. BMC Psychol. 11:97. doi: 10.1186/s40359-023-01140-3
Wei, W. S., McCoy, D. C., and Hanno, E. C. (2021). Classroom-level peer self-regulation as a predictor of individual self-regulatory and social-emotional development in Brazil. J. Appl. Dev. Psychol. 77:101347. doi: 10.1016/j.appdev.2021.101347
Willems, Y. E., Boesen, N., Li, J., Finkenauer, C., and Bartels, M. (2019). The heritability of self-control: a meta-analysis. Neurosci. Biobehav. Rev. 100, 324–334. doi: 10.1016/j.neubiorev.2019.02.012
Zakszeski, B., Hojnoski, R. L., Dever, B. V., DuPaul, G. J., and McClelland, M. M. (2020). Early elementary trajectories of classroom behavior self-regulation: prediction by student characteristics and malleable contextual factors. School Psych. Rev. 49, 161–177. doi: 10.1080/2372966X.2020.1717373
Keywords: multilevel, self-regulation, inhibition, working memory updating, flexibility, school class, executive functions
Citation: Busching R (2024) Do peers matter? The influence of peers' self-regulation on individual self-regulation: a longitudinal multilevel analysis. Front. Dev. Psychol. 2:1371500. doi: 10.3389/fdpys.2024.1371500
Received: 16 January 2024; Accepted: 28 August 2024;
Published: 18 September 2024.
Edited by:
Stephanie M. Carlson, University of Minnesota Twin Cities, United StatesReviewed by:
Lisa Rosen, Texas Woman's University, United StatesCopyright © 2024 Busching. This is an open-access article distributed under the terms of the Creative Commons Attribution License (CC BY). The use, distribution or reproduction in other forums is permitted, provided the original author(s) and the copyright owner(s) are credited and that the original publication in this journal is cited, in accordance with accepted academic practice. No use, distribution or reproduction is permitted which does not comply with these terms.
*Correspondence: Robert Busching, YnVzY2hpbmdAdW5pLXBvdHNkYW0uZGU=
Disclaimer: All claims expressed in this article are solely those of the authors and do not necessarily represent those of their affiliated organizations, or those of the publisher, the editors and the reviewers. Any product that may be evaluated in this article or claim that may be made by its manufacturer is not guaranteed or endorsed by the publisher.
Research integrity at Frontiers
Learn more about the work of our research integrity team to safeguard the quality of each article we publish.