- 1Department of Developmental and Educational Psychology, Faculty of Psychology, University of Vienna, Vienna, Austria
- 2Austrian Research Institute for Artificial Intelligence (OFAI), Vienna, Austria
- 3TU Wien, Automation and Control Institute (ACIN), Vienna, Austria
Humans increasingly interact with social robots and artificial intelligence (AI) powered digital assistants in their daily lives. These machines are usually designed to evoke attributions of social agency and trustworthiness in the human user. Growing research on human-machine-interactions (HMI) shows that young children are highly susceptible to design features suggesting human-like social agency and experience. Older children and adults, in contrast, are less likely to over attribute agency and experience to machines. At the same time, they tend to over-trust machines as informants more than younger children. Based on these findings, we argue that research directly comparing the effects of HMI design features on different age groups, including infants and young children is urgently needed. We call for evidence-based evaluation of HMI design and for consideration of the specific needs and susceptibilities of children when interacting with social robots and AI-based technology.
1 Introduction
Humans today interact with machines in a variety of contexts and with rapidly increasing frequency. Here, we conceptualize human-machine-interactions (HMI) as behavioral and communicative exchanges of humans with artificial agents that possess human-like features or behavioral properties, including social robots and virtual assistant AI technologies. Social robots are employed in educational settings, such as kindergartens and museums, and as assistants and companions in people's homes. Virtual assistant AI technologies have quickly become near omnipresent in recent years through their deployment in smartphones (e.g., Siri), smart speakers (e.g., Amazon Alexa), and with the recent rapid advancement of generative AI, including large language and multimodal models such as OpenAI's GPT models (Open et al., 2023), DeepMind's Gemini (Gemini Team et al., 2023) and similar models.
Social robots and virtual assistants more and more show characteristics of human-like social responsiveness (Yu et al., 2010; Xu et al., 2016; Henschel et al., 2020), which, however, entails some risks. One potential problem a human-friendly digitalization has to address is the risk of over-trust in machines (Noles et al., 2015; Baker et al., 2018; Lewis et al., 2018; Yew, 2020), that is trust which exceeds system capabilities (Lee and See, 2004) and which may sometimes prevail even in the face of obvious technical failure (Robinette et al., 2016). For instance, in a notable study by Robinette and colleagues, all participating adults followed an emergency guide robot in a perceived emergency (fire alarm with smoke). This was despite the fact that half of the participants had just observed the same robot perform poorly in a navigation task few minutes before the apparent emergency (Robinette et al., 2016). As regards generative AI, recent research shows that adults tend to devalue its competence, but not the provided content and still follow its advice (Böhm et al., 2023). We need to understand better when and how social and epistemic over-trust are induced in HMI and how this can be mitigated (Baker et al., 2018; Lewis et al., 2018; Yew, 2020; Van Straten et al., 2023).
Increasing attention is now being paid to studying children's interactions with robots and AI (Stower et al., 2021; Van Brummelen et al., 2023). While robots and AI offer exciting opportunities for children to learn and acquire technological skills, young children are highly susceptible to social features. They readily attribute agency and intentionality to artificial agents displaying cues of animacy (Rakison and Poulin-Dubois, 2001). For instance, preschool-aged children readily imitate even obviously functionally irrelevant actions that were demonstrated to them by the humanoid robot Nao, very similar to children's imitation of human models (Schleihauf et al., 2020). Nao is designed with anthropomorphic features, including big “eyes,” which most probably appeal to children's propensity to identify social partners and learn from them. At the same time, children are known to carefully track the reliability of (human) informants over the course of a learning exchange (Koenig et al., 2004; Jaswal and Neely, 2006; Brooker and Poulin-Dubois, 2013). This combination of readily accepting human-like machines as informative agents on the one hand and closely tracking the reliability of informants on the other hand could make preschool-aged children ideal targets for educating them to enable a responsible and informed handling of machines.
Social robots are an increasingly widespread assistive technology, and we can expect their use to further expand in the near future given recent trends (Jung and Hinds, 2018). Digital assistants are already widely used, including latest developments of generative AIs such as ChatGPT, its successors and competing models which are already very good in mimicking human conversation (Kasneci et al., 2023). The presence of HMI in our everyday lives has increased dramatically since the introduction of digital assistants and even more so with recent developments in conversational AI (Fu et al., 2022; Bubeck et al., 2023). At the same time, the Covid-19 pandemic has shown that circumstance can require human-human interactions to be temporarily highly restricted, severely affecting children's access to education (Betthäuser et al., 2023), thus making a sensible use of HMI in childcare and education a highly timely endeavor.
In this perspective paper, we argue for research and development of HMI that balances human need for sociability with realistic understanding of artificial agents' functioning and their limitations. We therefore draw attention to the relevant – and partially divergent – psychological factors influencing social and epistemic trust toward a machine in children and in adults. While children are highly susceptible to social features and often over-attribute socialness to artificial agents, adults seem at a relatively higher risk for epistemic over-trust in machines. Yet, extant research leaves open whether these differences are due to developmental changes or variations in experiences with technology in different cohorts. We contend that longitudinal studies and research directly comparing children's and adults' behavior as well as their socio-cognitive and brain processes in interactions with technologies designed to encompass human-like features, is clearly needed.
2 Humans are social learners
Humans are fundamentally social learners (Over and Carpenter, 2012; Hoehl et al., 2019). The human propensity to transmit information and share knowledge among each other is considered key to our evolutionary success and cultural evolution (Henrich, 2017). As social learning has both instrumental and social affiliative functions (Over and Carpenter, 2012), it is impacted by both epistemic and social trust. Epistemic trust greatly depends on prior reliability of the informant: Children are more likely to use and endorse information that is provided by a previously reliable and competent informant (Tong et al., 2020). Social trust, on the other hand, i.e., trust in the benevolence of another agent (Mayer et al., 1995), depends on personal relationships and group affiliation. For instance, more faithful imitation of inefficient and ritual-like actions has been reported for models that belong to the same in-group as the imitator (Buttelmann et al., 2012; Krieger et al., 2020). When it comes to learning about social conventions and norms, children's behavior is not only influenced by epistemic trust, but also a motivation to create or maintain social affiliation (Nielsen and Blank, 2011; Over and Carpenter, 2012) with a benevolent other (Schleihauf and Hoehl, 2021).
From birth, humans preferentially orient their attention toward social information, such as faces and speech (Johnson et al., 1991; Vouloumanos et al., 2010). Toward the end of the first postnatal year, infants actively seek information from others, a behavior called social referencing (Campos et al., 2003). Their emerging ability to engage in shared attention with others allows them to learn through social communication (Siposova and Carpenter, 2019). By around 1 year of age, infants not only imitate others' action outcomes, but also precise action manners (Gergely et al., 2002).
Due to rapid technological developments, children's learning from conspecifics is increasingly complemented by their learning through and from technical devices (Meltzoff et al., 2009; Nielsen et al., 2021). For instance, children may learn from screen media or through social interactions mediated through video-chat (Sundqvist et al., 2021). While earlier studies often reported a “video-deficit,” concluding that real-life interactions offer children more effective social learning opportunities than screen-based media, more recent research suggests that children may sometimes attribute more normative value to social information presented through a screen than live (Nielsen et al., 2021; Sommer et al., 2023). Nielsen and colleagues suggest that this “digital screen effect” may be due to contemporary children's extensive experiences and often parasocial relationships with artificial agents and fictional characters they regularly encounter through a range of media and devices. Yet, we are far from understanding the potentially transformative effect this will have on children's learning and human cultural evolution in the long run (Hughes et al., 2023; Sommer et al., 2023). Whereas, children and adults are equipped with cognitive capacities to track both the reliability of informants (Koenig et al., 2004; Brooker and Poulin-Dubois, 2013) and the (social) relevance of the transmitted information (Csibra and Gergely, 2009) in human-human interactions, it is unclear whether these mechanisms are adaptive when dealing with the wealth of information and types of technological informants humans nowadays encounter in their everyday lives, and how the generative AI's potential to act as personalized tutor will influence epistemic trust (Jauhiainen and Guerra, 2023; Murgia et al., 2023).
3 What makes an agent social?
When judging whether an interaction partner is social and possesses a mind, people tend to rely on two key dimensions: agency, i.e. the assumed capacity to plan and act intentionally, and experience, i.e. the assumed capacity to sense (Waytz et al., 2010). The attribution of “socialness” based on perceived agency and experience (sometimes conceptualized as competence and warmth, respectively) is an active and dynamic process that unfolds over the course of an exchange (Hortensius and Cross, 2018). To what degree the interactive partner is attributed agency and/ or experience has substantive implications (Waytz et al., 2010; Marchesi et al., 2019; Sommer et al., 2019): We tend to empathize with agents, to whom we attribute the capacity to experience and we hold those responsible for their wrongdoing, to whom we attribute agency. For comparative research on the topic of agency in non-human animals and different definitions of the concept, we refer the interested reader to pertinent existing work (Bandura, 1989; McFarland and Hediger, 2009; Carter and Charles, 2013; Špinka, 2019; Felnhofer et al., 2023).
In the past few years, a growing number of studies have addressed children's interactions with artificial agents, including robots and digital assistants (e.g., Tanaka et al., 2007; Melson et al., 2009; Cameron et al., 2015, 2017; Noles et al., 2015; Sommer et al., 2019, 2020, 2021a,b; Wang et al., 2019; Aeschlimann et al., 2020; Di Dio et al., 2020a; Manzi et al., 2020). While a systematic review of this growing field is beyond the scope of this perspective paper, we briefly review some major findings in this section and include a table for better overview of the findings in relation to the age groups tested (Table 1). Across studies, a developmental trend has become apparent: Younger children and infants are highly susceptible to cues indicating that the machine is a social agent (Arita et al., 2005; Tanaka et al., 2007; Brink and Wellman, 2020; Okanda et al., 2021; Manzi et al., 2022). Notably, these cues of socialness impact whether infants and young children accept an agent as a potential source of information for social learning (Itakura et al., 2008; Csibra, 2010; Deligianni et al., 2011; Okumura et al., 2013). For instance, two-year-olds imitated the inferred “intended” (but unfinished) actions modeled from a robot only if the robot had established eye contact with them (Itakura et al., 2008).
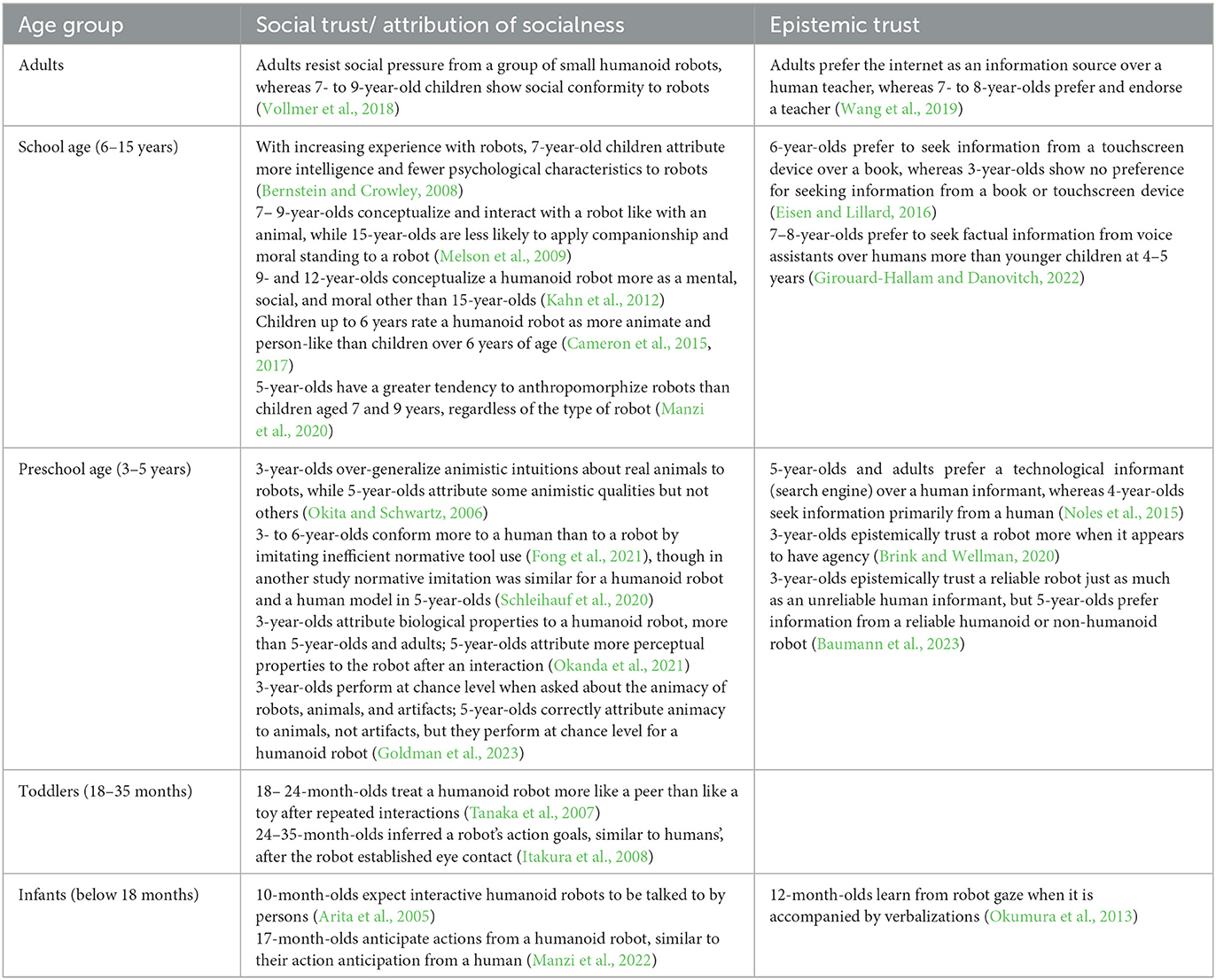
Table 1. Overview of the reviewed developmental studies on social and epistemic trust toward machines.
With increasing age, children (similar to adults) seem to become less likely to conceptualize a machine as a social agent and are less affected by cues indicating agency and experience (Okita and Schwartz, 2006; Melson et al., 2009; Kahn et al., 2012; Cameron et al., 2015, 2017; Manzi et al., 2020; Goldman et al., 2023). This general trend is in line with the high sensitivity to identify social agents in young children, that is well-documented already in infancy (Rakison and Poulin-Dubois, 2001), and speaks to the notion that young children's concepts of “socialness” are rather broad and malleable.
At the same time, when learning from other humans, children carefully track the reliability of potential informants (Koenig et al., 2004; Jaswal and Neely, 2006; Poulin-Dubois and Chow, 2009; Brooker and Poulin-Dubois, 2013; Geiskkovitch et al., 2019). In classic studies on epistemic trust, children encounter informants that are either reliable (e.g., accurately labeling objects that are familiar to the child) or unreliable (e.g., mislabelling known objects or answering simple questions incorrectly). Children are then invited to solve a task or seek information. Researchers track whether children seek information selectively from previously reliable informants and sometimes also assess whether children actively endorse information they received from these informants. A recent meta-analysis found that 4–6-year-olds consistently prioritized epistemic cues over social characteristics when making decisions whom to trust and whose information to endorse, whereas younger children do not consistently prioritize epistemic over social cues (Tong et al., 2020).
Interestingly, when deciding between different informants, older children and adults tend to prefer and trust technological informants to a higher degree than younger children do (Noles et al., 2015; Eisen and Lillard, 2016; Wang et al., 2019; Girouard-Hallam and Danovitch, 2022; Baumann et al., 2023). Recent work on interaction between children (4–5-year-olds, 7–8-year-olds) and a digital voice assistant indicates that with increasing age, children increasingly seek factual information from a voice assistant whereas they preferably seek personal information from a human (Girouard-Hallam and Danovitch, 2022). In one study, 5-year-old children and adults preferred a technological informant (search engine) over a human informant, whereas younger children chose to seek information primarily from the human (Noles et al., 2015). Similarly, in another study, adults preferred the internet as an information source over a human teacher, whereas children preferred and endorsed the teacher (Wang et al., 2019). Experience seems to play a role in this process: With increasing experience with robots, 4–7-year-old children attributed more intelligence and less psychological characteristics to robots (Bernstein and Crowley, 2008).
Observations of excessive epistemic trust toward machines, predominantly in adults (Robinette et al., 2016), evoked calls for establishing calibrated trust in HMI, that is trust that matches system capabilities and which could be based, among other factors, on system transparency (Baker et al., 2018; Lewis et al., 2018; Yew, 2020; Van Straten et al., 2023). For instance, Van Straten et al. (2023) find that a social robot's own transparency (regarding its abilities, lack of human psychological capacities, machine status) leads to a decreased feeling of trust and closeness in 8–10-year-olds, whereby trust and closeness are mediated by children's tendency to anthropomorphise, and closeness is also mediated by the children's perception of the robot's similarity to themselves.
In a nutshell, children and adults seem to interact with and perceive artificial agents differently. Figure 1 displays a theory map (Gray, 2017) synthesizing the psychological factors determining and moderating social learning in HMI and their relations. A key factor for young children seems to be a high susceptibility to cues indicating socialness and consequently a potential over-attribution of agency and experiences to artificial agents which might affect how they engage with and learn from machines in the long run. In particular, over-attribution of socialness might lead to an abundance of unwarranted social trust (Di Dio et al., 2020b) and even normative social conformity toward robots which has been shown to be more pronounced in children than in adults (Vollmer et al., 2018). While children seem to prioritize normative instructions from a human over those from a robot (Fong et al., 2021), they are more likely than adults to socially conform in their judgements to a group of humanoid robots (Vollmer et al., 2018). Adults and older children, in contrast, are less affected than younger children by artificial social features. Yet, when engaging with technological informants, older children and adults sometimes display epistemic over-trust, as illustrated most strikingly in experiments where adults continue to trust machines as informants even after witnessing blatant failures (Robinette et al., 2016). It must be pointed out that age-related differences reported in the literature thus far may in part reflect cohort effects, driven by vastly different (early) experiences with machines across generations (Nielsen et al., 2021). Potential cohort effects could be due to technological advances (e.g., recently improved generative AIs) and the availability and pervasiveness of devices in daily lives (e.g., smart phones and speakers) that have the potential to change the quality and quantity of early human experiences with artificial agents. We are far from understanding the potentially long-lasting effects these experiences have and longitudinal research, ideally applying cohort sequential designs, is urgently needed.
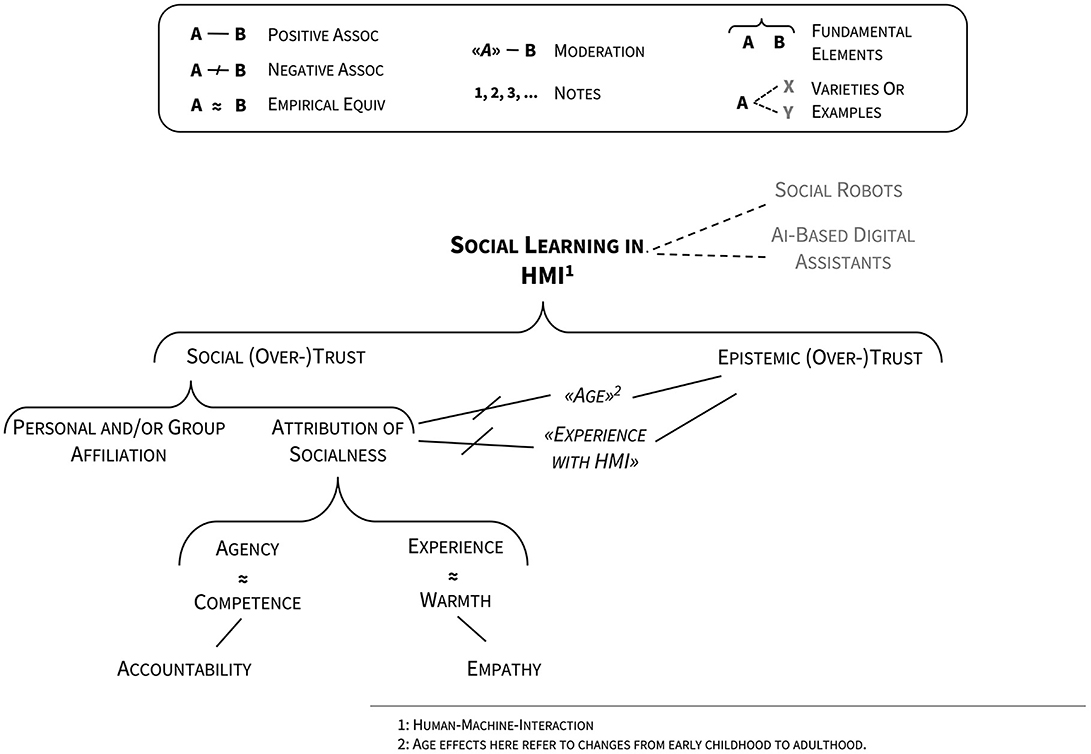
Figure 1. Theory map (Gray, 2017) providing an overview of the relevant factors influencing learning from HMI differently depending on age from early childhood to adulthood and with varying levels of experience with HMI.
4 Where do we go from here?
The key scientific challenge for the future is to delineate and better understand the processes leading to social and epistemic trust toward machines in children and adults. This will help answer one of the most central questions for the design of future technology: Should we design HMI for children and adults in fundamentally different ways to account for the different cognitive processes, potential risks and opportunities identified in both groups? The risk of epistemic over-trust of adults in machines is relatively well-researched and has resulted, e.g., in calls for system transparency (Baker et al., 2018; Lewis et al., 2018; Yew, 2020). At the same time, our understanding of HMI in children is much more limited. If children's higher susceptibility to social features promotes their normative conformity to robots, machines might in the future play an unprecedented role in cultural transmission and evolution, the implications of which are hard to foresee. Perhaps counter-intuitively, this might warrant equipping machines that are developed to interact with young children with fewer social characteristics. At the same time, children's cognitive plasticity puts them in an ideal position to be educated about system capabilities and limitations. Can we design HMI in such a way that children benefit sustainably from early experiences?
Addressing these fundamental questions and advancing HMI for long-term human benefit will necessitate collaboration across disciplines. Designing experiments with human participants requires insights into psychological and linguistic processes and research methodology. Implementation of HMI in these experiments requires state-of-the-art technological knowhow. Only by combining complementary skill sets and knowledge can we critically assess the effects of different strategies used in technological development on psychological processes in the human user.
Exciting opportunities arise with the combination of behavioral and neuroscientific methodology (Wykowska et al., 2016; Wiese et al., 2017; Cross et al., 2019; Henschel et al., 2020). Above and beyond behavioral paradigms from developmental psychology, measures of brain activity allow unraveling to what degree brain networks underlying human-human social interaction and social cognition are also involved in HMI. Of particular interest are the temporoparietal junction (TPJ) and the medial prefrontal cortex (mPFC). These regions are involved in mental perspective taking, i.e., reasoning about other persons' wishes, perspectives and beliefs, in both children and adults (Saxe et al., 2004) and are referred to as part of a “mentalizing network” in the human brain (Kanske et al., 2015). In adults TPJ and mPFC are activated specifically when participants believe that they play a game against a human, but not when believing they play against a humanoid robot and/or an AI (Krach et al., 2008; Chaminade et al., 2012).
It is of great scientific interest and importance to assess to what degree these brain regions are implicated in children's HMI because the mentalizing network may be less specialized for human-human interaction in early development. The high plasticity of functional brain networks in the first years of life opens up opportunities for intense learning and skill formation (Heckman, 2006), and ensures that our early experiences have a long-lasting impact on how we engage with the world and other people (Nelson et al., 2007; Feldman, 2017). Thus, early HMI may be foundational for how we engage with machines across the lifespan which could have profound long-term impact on the way humans communicate and transmit knowledge (Hughes et al., 2023; Sommer et al., 2023). This should have important implications for the ways we introduce HMI into the lives of children in order to enable competent handling of technology while keeping a firm grasp on machines' abilities and limitations.
Not least due to the potential long-lasting effects of early HMI, this field of research and technology development has profound ethical implications and researchers, developers, and practitioners should carefully consider the benefits and risks when introducing new technologies to children. Early HMI could not only impact the way children treat artificial agents later on but may also affect concurrent and later human-human interactions and relationships, including relations of care.
5 Conclusions
We have summarized evidence pointing toward age- and experience-related differences in how children and adults engage in HMI. Specifically, infants and young children tend to over-attribute socialness to machines, which may lead to inflated social trust and even normative conformity toward robots and AI. Older children and adults, in contrast, tend to show epistemic over-trust toward machines. While the ethical problems associated with “tricking” humans into attributing intentions and sociability to machines have been recognized and critically discussed in the field of HMI research (Prescott and Robillard, 2020; Sharkey and Sharkey, 2020), children's specific needs and susceptibilities are not always considered. Longitudinal research is urgently needed to delineate the potential long-term effects of early experiences in HMI. We call for more inter-disciplinary research on the cognitive basis of potential socio-technical problems associated with the design of HMI. Ideally this research will directly compare the effects of specific design features in diverse age groups across the lifespan. The ensuing insights can inform technology development on how to design artificial agents that truly and sustainably serve humans.
Data availability statement
The original contributions presented in the study are included in the article/supplementary material, further inquiries can be directed to the corresponding author.
Author contributions
SH: Conceptualization, Writing – original draft. BK: Conceptualization, Writing – review & editing. MV: Conceptualization, Writing – review & editing.
Funding
The author(s) declare that no financial support was received for the research, authorship, and/or publication of this article.
Conflict of interest
The authors declare that the research was conducted in the absence of any commercial or financial relationships that could be construed as a potential conflict of interest.
Publisher's note
All claims expressed in this article are solely those of the authors and do not necessarily represent those of their affiliated organizations, or those of the publisher, the editors and the reviewers. Any product that may be evaluated in this article, or claim that may be made by its manufacturer, is not guaranteed or endorsed by the publisher.
References
Aeschlimann, S., Bleiker, M., Wechner, M., and Gampe, A. (2020). Communicative and social consequences of interactions with voice assistants. Comput. Hum. Behav. 112, 106466. doi: 10.1016/j.chb.2020.106466
Arita, A., Hiraki, K., Kanda, T., and Ishiguro, H. (2005). Can we talk to robots? Ten-month-old infants expected interactive humanoid robots to be talked to by persons. Cognition 95, B49–B57. doi: 10.1016/j.cognition.2004.08.001
Baker, A. L., Phillips, E. K., Ullman, D., and Keebler, J. R. (2018). Toward an understanding of trust repair in human-robot interaction: current research and future directions. ACM Trans. Int. Int. Syst. 8, 1–30. doi: 10.1145/3181671
Bandura, A. (1989). Human agency in social cognitive theory. Am. Psychol. 44, 1175–1184. doi: 10.1037/0003-066X.44.9.1175
Baumann, A. E., Goldman, E. J., Meltzer, A., and Poulin-Dubois, D. (2023). People do not always know best: preschoolers' trust in social robots. J. Cognit. Dev. 12, 1–28. doi: 10.1080/15248372.2023.2178435
Bernstein, D., and Crowley, K. (2008). Searching for signs of intelligent life: an investigation of young children's beliefs about robot intelligence. J. Learning Sci. 17, 225–247. doi: 10.1080/10508400801986116
Betthäuser, B. A., Bach-Mortensen, A. M., and Engzell, P. (2023). A systematic review and meta-analysis of the evidence on learning during the COVID-19 pandemic. Nat. Hum. Behav. 7, 375–385. doi: 10.1038/s41562-022-01506-4
Böhm, R., Jörling, M., Reiter, L., and Fuchs, C. (2023). People devalue generative AI's competence but not its advice in addressing societal and personal challenges. Commun. Psychol. 1, 32. doi: 10.1038/s44271-023-00032-x
Brink, K. A., and Wellman, H. M. (2020). Robot teachers for children? Young children trust robots depending on their perceived accuracy and agency. Dev. Psychol. 56, 1268–1277. doi: 10.1037/dev0000884
Brooker, I., and Poulin-Dubois, D. (2013). Is a bird an apple? The effect of speaker labeling accuracy on infants' word learning, imitation, and helping behaviors. Infancy 18, E46–E68. doi: 10.1111/infa.12027
Bubeck, S., Chandrasekaran, V., Eldan, R., Gehrke, J., Horvitz, E., Kamar, E., et al. (2023). Sparks of Artificial General Intelligence: Early experiments with GPT-4. doi: 10.48550/ARXIV.2303.12712
Buttelmann, D., Zmyj, N., Daum, M., and Carpenter, M. (2012). Selective imitation of in-group over out-group members in 14-month-old infants. Child Dev. 84, 422–428. doi: 10.1111/j.1467-8624.2012.01860.x
Cameron, D., Fernando, S., Collins, E. C., Millings, A., Szollosy, M., Moore, R., et al. (2017). “You made him be alive: children's perceptions of animacy in a humanoid robot,” in Biomimetic and Biohybrid Systems, eds M. Mangan, M. Cutkosky, A. Mura, P. F. M. J. Verschure, T. Prescott (Cham: Springer International Publishing), 73–85.
Cameron, D., Fernando, S., Millings, A., Moore, R., Sharkey, A., Prescott, T., et al. (2015). “Children's Age Influences Their Perceptions of a Humanoid Robot as Being Like a Person or Machine,” in Biomimetic and Biohybrid Systems, eds S. P. Wilson, P. F. M. J. Verschure, A. Mura, and T. J. Prescott (Cham: Springer International Publishing), 348–353.
Campos, J. J., Thein, S., and Owen, D. (2003). A Darwinian legacy to understanding human infancy—Emotional expressions as behavior regulators. Annal. N. Y. Acad. Sci. 1000, 110–134. doi: 10.1196/annals.1280.040
Carter, B., and Charles, N. (2013). Animals, agency and resistance. J. Theor. Soc. Behav. 43, 322–340. doi: 10.1111/jtsb.12019
Chaminade, T., Rosset, D., Fonseca, D., Nazarian, D., Lutcher, B., Cheng, E., et al. (2012). How do we think machines think? An fMRI study of alleged competition with an artificial intelligence. Front. Hum. Neurosci. 6, 103. doi: 10.3389/fnhum.2012.00103
Cross, E. S., Hortensius, R., and Wykowska, A. (2019). From social brains to social robots: applying neurocognitive insights to human–robot interaction. Philos. Trans. Royal Soc. Biol. Sci. 374, 20180024. doi: 10.1098/rstb.2018.0024
Csibra, G. (2010). Recognizing communicative intentions in infancy. Mind Lang. 25, 141–168. doi: 10.1111/j.1468-0017.2009.01384.x
Csibra, G., and Gergely, G. (2009). Natural pedagogy. Trends Cognit. Sci. 13, 148–153. doi: 10.1016/j.tics.2009.01.005
Deligianni, F., Senju, A., Gergely, G., and Csibra, G. (2011). Automated gaze-contingent objects elicit orientation following in 8-month-old infants. Dev. Psychol. 47, 1499–1503. doi: 10.1037/a0025659
Di Dio, D., Manzi, C., Itakura, F., Kanda, S., Ishiguro, T., Massaro, H., et al. (2020a). It does not matter who you are: fairness in pre-schoolers interacting with human and robotic partners. Int. J. Soc. Robotics 12, 1045–1059. doi: 10.1007/s12369-019-00528-9
Di Dio, D., Manzi, C., Peretti, F., Cangelosi, G., Harris, A., Massaro, P. L., et al. (2020b). Shall I trust you? From child–robot interaction to trusting relationships. Front. Psychol. 11, 469. doi: 10.3389/fpsyg.2020.00469
Eisen, S., and Lillard, A. S. (2016). Just google it: young children's preferences for touchscreens versus books in hypothetical learning tasks. Front. Psychol. 7, 1431. doi: 10.3389/fpsyg.2016.01431
Feldman, R. (2017). The neurobiology of human attachments. Trends Cognit. Sci. 21, 80–99. doi: 10.1016/j.tics.2016.11.007
Felnhofer, A., Knaust, T., Weiss, L., Goinska, K., Mayer, A., and Kothgassner, O. D. (2023). A virtual character's Agency affects social responses in immersive virtual reality: a systematic review and meta-analysis. Int. J. Hum. Computer Interact. 1–16.
Fong, F. T. K., Sommer, K., Redshaw, J., Kang, J., and Nielsen, M. (2021). The man and the machine: Do children learn from and transmit tool-use knowledge acquired from a robot in ways that are comparable to a human model? J. Exp. Child Psychol. 208, 105148. doi: 10.1016/j.jecp.2021.105148
Fu, T., Gao, S., Zhao, X., Wen, J., and Yan, R. (2022). Learning towards conversational AI: a survey. AI Open 3, 14–28. doi: 10.1016/j.aiopen.2022.02.001
Geiskkovitch, D. Y., Thiessen, R., Young, J. E., and Glenwright, M. R. (2019). “What? That's Not a Chair!: How robot informational errors affect children's trust towards robots,” in 2019 14th ACM/IEEE International Conference on Human-Robot Interaction (HRI) (New York, NY: IEEE), 48–56.
Gemini Team, Anil, R., Borgeaud, S., Wu, Y., Alayrac, J.-B., Yu, J., et al. (2023). Gemini: A Family of Highly Capable Multimodal Models. doi: 10.48550/ARXIV.2312.11805
Gergely, G., Bekkering, H., and Kiraly, I. (2002). Rational imitation in preverbal infants. Nature 415, 755. doi: 10.1038/415755a
Girouard-Hallam, L. N., and Danovitch, J. H. (2022). Children's trust in and learning from voice assistants. Dev. Psychol. 58, 646–661. doi: 10.1037/dev0001318
Goldman, E. J., Baumann, A. E., and Poulin-Dubois, D. (2023). Preschoolers' anthropomorphizing of robots: Do human-like properties matter? Front. Psychol. 13, 1102370. doi: 10.3389/fpsyg.2022.1102370
Gray, K. (2017). How to map theory: reliable methods are fruitless without rigorous theory. Persp. Psychol. Sci. 12, 731–741. doi: 10.1177/1745691617691949
Heckman, J. J. (2006). Skill formation and the economics of investing in disadvantaged children. Science 312, 1900–1902. doi: 10.1126/science.1128898
Henrich, J. (2017). The Secret of Our Success: How Culture Is Driving Human Evolution, Domesticating Our Species, and Making Us Smarter. Princeton, NJ: Princeton University Press.
Henschel, A., Hortensius, R., and Cross, E. S. (2020). Social cognition in the age of human–robot interaction. Trends Neurosci. 43, 373–384. doi: 10.1016/j.tins.2020.03.013
Hoehl, S., Keupp, S., Schleihauf, H., McGuigan, N., Buttelmann, D., Whiten, A., et al. (2019). ‘Over-imitation': a review and appraisal of a decade of research. Dev. Rev. 51, 90–108. doi: 10.1016/j.dr.2018.12.002
Hortensius, R., and Cross, E. S. (2018). From automata to animate beings: The scope and limits of attributing socialness to artificial agents: Socialness attribution and artificial agents. Annal. New York Acad. Sci. 1426, 93–110. doi: 10.1111/nyas.13727
Hughes, R. C., Bhopal, S. S., Manu, A. A., and Van Heerden, A. C. (2023). Hacking childhood: Will future technologies undermine, or enable, optimal early childhood development? Arch. Dis. Childhood 108, 82–83. doi: 10.1136/archdischild-2021-323158
Itakura, S., Ishida, H., Kanda, T., Shimada, Y., Ishiguro, H., Lee, K., et al. (2008). How to build an intentional android: infants' imitation of a robot's goal-directed actions. Infancy 13, 519–532. doi: 10.1080/15250000802329503
Jaswal, V. K., and Neely, L. A. (2006). Adults don't always know best: preschoolers use past reliability over age when learning new words. Psychol. Sci. 17, 757–758. doi: 10.1111/j.1467-9280.2006.01778.x
Jauhiainen, J. S., and Guerra, A. G. (2023). Generative AI and ChatGPT in School Children's education: evidence from a school lesson. Sustainability 15:14025. doi: 10.3390/su151814025
Johnson, M. H., Dziurawiec, S., Ellis, H., and Morton, J. (1991). Newborns' preferential tracking of face-like stimuli and its subsequent decline. Cognition 40, 1–19. doi: 10.1016/0010-0277(91)90045-6
Jung, M., and Hinds, P. (2018). Robots in the wild: a time for more robust theories of human-robot interaction. ACM Trans. Hum. Robot Int. 7, 1–5. doi: 10.1145/3208975
Kahn, P. H., Kanda, T., Ishiguro, H., Freier, N. G., Severson, R. L., Gill, B. T., et al. (2012). “Robovie, you'll have to go into the closet now”: children's social and moral relationships with a humanoid robot. Dev. Psychol. 48, 303–314. doi: 10.1037/a0027033
Kanske, P., Böckler, A., Trautwein, F. M., and Singer, T. (2015). Dissecting the social brain: Introducing the EmpaToM to reveal distinct neural networks and brain–behavior relations for empathy and theory of mind. NeuroImage 122, 6–19. doi: 10.1016/j.neuroimage.2015.07.082
Kasneci, E., Sessler, K., Küchemann, S., Bannert, M., Dementieva, D., Fischer, F., et al. (2023). ChatGPT for good? On opportunities and challenges of large language models for education. Learning Ind. Diff. 103, 102274. doi: 10.1016/j.lindif.2023.102274
Koenig, M. A., Clement, F., and Harris, P. L. (2004). Trust in testimony: children's use of true and false statements. Psychol. Sci. 15, 694–698. doi: 10.1111/j.0956-7976.2004.00742.x
Krach, S., Hegel, F., Wrede, B., Sagerer, G., Binkofski, F., Kircher, T., et al. (2008). Can machines think? Interaction and perspective taking with robots investigated via fMRI. PLoS ONE 3, e2597. doi: 10.1371/journal.pone.0002597
Krieger, A. A. R., Aschersleben, G., Sommerfeld, L., and Buttelmann, D. (2020). A model's natural group membership affects over-imitation in 6-year-olds. J. Exp. Child Psychol. 192, 104783. doi: 10.1016/j.jecp.2019.104783
Lee, J. D., and See, K. A. (2004). Trust in automation: designing for appropriate reliance. Hum. Factors J. Erg. Soc. 46, 50–80. doi: 10.1518/hfes.46.1.50.30392
Lewis, M., Sycara, K., and Walker, P. (2018). “The Role of Trust in Human-Robot Interaction,” in Foundations of Trusted Autonomy, eds H. A. Abbass, J. Scholz, and D. J. Reid (Cham: Springer International Publishing), 135–159.
Manzi, F., Ishikawa, M., Di Dio, C., Itakura, S., Kanda, T., Ishiguro, H., et al. (2022). Infants' prediction of humanoid robot's goal-directed action. Int. J. Soc. Robotics 32, 1–11. doi: 10.1007/s12369-022-00941-7
Manzi, F., Peretti, G., Di Dio, D., Cangelosi, C., Itakura, A., Kanda, S., et al. (2020). A robot is not worth another: exploring children's mental state attribution to different humanoid robots. Front. Psychol. 11, 2011. doi: 10.3389/fpsyg.2020.02011
Marchesi, S., Ghiglino, D., Ciardo, F., Perez-Osorio, J., Baykara, E., Wykowska, A., et al. (2019). Do we adopt the intentional stance toward humanoid robots? Front. Psychol. 10, 450. doi: 10.3389/fpsyg.2019.00450
Mayer, R. C., Davis, J. H., and Schoorman, F. D. (1995). An integrative model of organizational trust. The Acad. Manage. Rev. 20, 709. doi: 10.2307/258792
McFarland, S. E., and Hediger, R. (2009). Animals and Agency: An Interdisciplinary Exploration. London: Brill.
Melson, G. F., Kahn, P. H., Beck, A., Friedman, B., Roberts, T., Garrett, E., et al. (2009). Children's behavior toward and understanding of robotic and living dogs. J. Appl. Dev. Psychol. 30, 92–102. doi: 10.1016/j.appdev.2008.10.011
Meltzoff, A. N., Kuhl, P. K., Movellan, J., and Sejnowski, T. J. (2009). Foundations for a new science of learning. Science 325, 284–288. doi: 10.1126/science.1175626
Murgia, E., Pera, M. S., Landoni, M., and Huibers, T. (2023). “Children on ChatGPT readability in an educational context: myth or opportunity?” in UMAP 2023 - Adjunct Proceedings of the 31st ACM Conference on User Modeling, Adaptation and Personalization (Association for Computing Machinery), 311–316. doi: 10.1145/3563359.3596996
Nelson, C. A., Zeanah, C. H., Fox, N. A., Marshall, P. J., Smyke, A. T., Guthrie, D., et al. (2007). Cognitive recovery in socially deprived young children: the bucharest early intervention project. Science 318, 1937–1940. doi: 10.1126/science.1143921
Nielsen, M., and Blank, C. (2011). Imitation in young children: When who gets copied is more important than what gets copied. Dev. Psychol. 47, 1050–1053. doi: 10.1037/a0023866
Nielsen, M., Fong, F. T. K., and Whiten, A. (2021). Social learning from media: the need for a culturally diachronic developmental psychology. Adv. Child Dev. Behav. 32, 317–334. doi: 10.1016/bs.acdb.2021.04.001
Noles, S., Danovitch, J. H., and Shafto, P. (2015). “Children's trust in technological and human informants,” in Proceedings of the 37th Annual Conference of the Cognitive Science Society (Seattle, WA: The Cognitive Science Society).
Okanda, M., Taniguchi, K., Wang, Y., and Itakura, S. (2021). Preschoolers' and adults' animism tendencies toward a humanoid robot. Comput. Hum. Behav. 118, 106688. doi: 10.1016/j.chb.2021.106688
Okita, S. Y., and Schwartz, D. L. (2006). Young children's understanding of animacy and entertainment robots. Int. J. Human. Robot. 03, 393–412. doi: 10.1142/S0219843606000795
Okumura, Y., Kanakogi, Y., Kanda, T., Ishiguro, H., and Itakura, S. (2013). Can infants use robot gaze for object learning?: The effect of verbalization. Int. Stu. Soc. Behav. Commun. Biol. Artif. Syst. 14, 351–365. doi: 10.1075/is.14.3.03oku
Open, A. I., Achiam, J., Adler, S., Agarwal, S., Ahmad, L., Akkaya, I., et al. (2023). GPT-4 Technical Report. doi: 10.48550/ARXIV.2303.08774
Over, H., and Carpenter, M. (2012). Putting the social into social learning: explaining both selectivity and fidelity in children's copying behavior. J. Comp. Psychol. 126, 182–192. doi: 10.1037/a0024555
Poulin-Dubois, D., and Chow, V. (2009). The effect of a looker's past reliability on infants' reasoning about beliefs. Dev. Psychol. 45, 1576–1582. doi: 10.1037/a0016715
Prescott, T. J., and Robillard, J. M. (2020). Are friends electric? The benefits and risks of human-robot relationships. iScience 101993. doi: 10.1016/j.isci.2020.101993
Rakison, D. H., and Poulin-Dubois, D. (2001). Developmental origin of the animate-inanimate distinction. Psychol. Bullet. 127, 209–228. doi: 10.1037/0033-2909.127.2.209
Robinette, P., Li, W., Allen, R., Howard, A. M., and Wagner, A. R. (2016). “Overtrust of robots in emergency evacuation scenarios,” in 2016 11th ACM/IEEE International Conference on Human-Robot Interaction (HRI) (New York, NY: IEEE), 101–108.
Saxe, R., Carey, S., and Kanwisher, N. (2004). Understanding other minds: linking developmental psychology and functional neuroimaging. Ann. Rev. Psychol. 55, 87–124. doi: 10.1146/annurev.psych.55.090902.142044
Schleihauf, H., and Hoehl, S. (2021). Evidence for a dual-process account of over-imitation: Children imitate anti- and prosocial models equally, but prefer prosocial models once they become aware of multiple solutions to a task. PLoS ONE 16, e0256614. doi: 10.1371/journal.pone.0256614
Schleihauf, H., Hoehl, S., Tsvetkova, N., König, A., Mombaur, K., Pauen, S., et al. (2020). Preschoolers' Motivation to Over-Imitate Humans and Robots. Child Dev. 14, 13403. doi: 10.1111/cdev.13403
Sharkey, A., and Sharkey, N. (2020). We need to talk about deception in social robotics! Ethics Inf. Technol. 23, 306–313. doi: 10.1007/s10676-020-09573-9
Siposova, B., and Carpenter, M. (2019). A new look at joint attention and common knowledge. Cognition 189, 260–274. doi: 10.1016/j.cognition.2019.03.019
Sommer, K., Davidson, R., Armitage, K. L., Slaughter, V., Wiles, J., Nielsen, M., et al. (2020). Preschool children overimitate robots, but do so less than they overimitate humans. J. Exp. Child Psychol. 191, 104702. doi: 10.1016/j.jecp.2019.104702
Sommer, K., Nielsen, M., Draheim, M., Redshaw, J., Vanman, E. J., Wilks, M., et al. (2019). Children's perceptions of the moral worth of live agents, robots, and inanimate objects. J. Exp. Child Psychol. 187, 104656. doi: 10.1016/j.jecp.2019.06.009
Sommer, K., Redshaw, J., Slaughter, V., Wiles, J., and Nielsen, M. (2021a). The early ontogeny of infants' imitation of on screen humans and robots. Infant Behav. Dev. 64, 101614. doi: 10.1016/j.infbeh.2021.101614
Sommer, K., Slaughter, V., Wiles, J., and Nielsen, M. (2023). Revisiting the video deficit in technology-saturated environments: Successful imitation from people, screens, and social robots. J. Exp. Child Psychol. 232, 105673. doi: 10.1016/j.jecp.2023.105673
Sommer, K., Slaughter, V., Wiles, J., Owen, K., Chiba, A. A., Forster, D., et al. (2021b). Can a robot teach me that? Children's ability to imitate robots. J. Exp. Child Psychol. 203, 105040. doi: 10.1016/j.jecp.2020.105040
Špinka, M. (2019). Animal agency, animal awareness and animal welfare. Animal Welfare 28, 11–20. doi: 10.7120/09627286.28.1.011
Stower, R., Calvo-Barajas, N., Castellano, G., and Kappas, A. (2021). A meta-analysis on children's trust in social robots. Int. J. Soc. Robotics 13, 1979–2001. doi: 10.1007/s12369-020-00736-8
Sundqvist, A., Koch, F. S., Birberg Thornberg, U., Barr, R., and Heimann, M. (2021). Growing up in a digital world – digital media and the association with the child's language development at two years of age. Front. Psychol. 12, 569920. doi: 10.3389/fpsyg.2021.569920
Tanaka, F., Cicourel, A., and Movellan, J. R. (2007). Socialization between toddlers and robots at an early childhood education center. Proc. Nat. Acad. Sci. 104, 17954–17958. doi: 10.1073/pnas.0707769104
Tong, Y., Wang, F., and Danovitch, J. (2020). The role of epistemic and social characteristics in children's selective trust: Three meta-analyses. Dev. Sci. 23, 12895. doi: 10.1111/desc.12895
Van Brummelen, J., Tian, M. C., Kelleher, M., and Nguyen, N. H. (2023). Learning affects trust: design recommendations and concepts for teaching children—and nearly anyone—about conversational agents. Proc. AAAI Conf. Artif. Int. 37, 15860–15868. doi: 10.1609/aaai.v37i13.26883
Van Straten, C. L., Peter, J., and Kühne, R. (2023). Transparent robots: How children perceive and relate to a social robot that acknowledges its lack of human psychological capacities and machine status. Int. J. Hum. Comput. Stu. 177, 103063. doi: 10.1016/j.ijhcs.2023.103063
Vollmer, A. L., Read, R., Trippas, D., and Belpaeme, T. (2018). Children conform, adults resist: a robot group induced peer pressure on normative social conformity. Sci. Robot. 3, eaat7111. doi: 10.1126/scirobotics.aat7111
Vouloumanos, A., Hauser, M. D., Werker, J. F., and Martin, A. (2010). The tuning of human neonates' preference for speech. Child Dev. 81, 517–527. doi: 10.1111/j.1467-8624.2009.01412.x
Wang, F., Tong, Y., and Danovitch, J. (2019). Who do I believe? Children's epistemic trust in internet, teacher, and peer informants. Cognit. Dev. 50, 248–260. doi: 10.1016/j.cogdev.2019.05.006
Waytz, A., Gray, K., Epley, N., and Wegner, D. M. (2010). Causes and consequences of mind perception. Trends Cognit. Sci. 14, 383–388. doi: 10.1016/j.tics.2010.05.006
Wiese, E., Metta, G., and Wykowska, A. (2017). Robots as intentional agents: using neuroscientific methods to make robots appear more social. Front. Psychol. 8, 1663. doi: 10.3389/fpsyg.2017.01663
Wykowska, A., Chaminade, T., and Cheng, G. (2016). Embodied artificial agents for understanding human social cognition. Philos. Trans. Royal Soc. Biol. Sci. 371, 20150375. doi: 10.1098/rstb.2015.0375
Xu, T., Zhang, H., and Yu, C. (2016). See you see me: the role of eye contact in multimodal human-robot interaction. ACM Trans. Int. Int. Syst. 6, 1–22.
Yew, G. C. K. (2020). Trust in and ethical design of carebots: the case for ethics of care. Int. J. Soc. Robotics. 13, 629–645. doi: 10.1007/s12369-020-00653-w
Keywords: human-machine-interaction, social learning, epistemic trust, children, robots, AI
Citation: Hoehl S, Krenn B and Vincze M (2024) Honest machines? A cross-disciplinary perspective on trustworthy technology for children. Front. Dev. Psychol. 2:1308881. doi: 10.3389/fdpys.2024.1308881
Received: 07 October 2023; Accepted: 06 February 2024;
Published: 28 February 2024.
Edited by:
Stephanie M. Carlson, University of Minnesota Twin Cities, United StatesReviewed by:
Catherine Sandhofer, University of California, Los Angeles, United StatesCopyright © 2024 Hoehl, Krenn and Vincze. This is an open-access article distributed under the terms of the Creative Commons Attribution License (CC BY). The use, distribution or reproduction in other forums is permitted, provided the original author(s) and the copyright owner(s) are credited and that the original publication in this journal is cited, in accordance with accepted academic practice. No use, distribution or reproduction is permitted which does not comply with these terms.
*Correspondence: Stefanie Hoehl, stefanie.hoehl@univie.ac.at