- Department of Psychology, University of Michigan, Ann Arbor, MI, United States
Introduction: Where do individual differences in theory of mind (ToM) development come from? This is an open-ended issue in developmental psychology. Correlational research suggests social interaction as a cause. However, causal experiments controlling children's extended, real-life social interactions are impractical and unethical, so the links between social interaction and ToM remain only indirectly demonstrated. To shed light on how social interaction, in different degrees and forms, may influence ToM development, we conducted computational experiments using agent-based modeling.
Methods: To begin with, we simulated agents (hypothetically, children) and assigned them to 10 groups, where each group was manipulated to allow a different amount of social interaction, from 1 to 10. Within each group, agents randomly roamed and encountered one another and then interacted. If these interactions continued long enough, agents' ToM probabilistically improved.
Results: As expected, results showed that the amount of social interaction strongly influenced how fast agents developed ToM, where more social interaction led to more rapid development. Given this confirmation of the assumed link, we more focally explored how other factors—such as having a prior, established social network or agents' network centrality—could influence the social interaction-ToM link. Then, we tested our model against real-world data from 84 deaf children and showed that our modeling results could explain the social interaction-ToM link observed in deaf children.
Discussion: These demonstrations illustrate how individual differences in ToM development may emerge and offer an explanation specifying a crucial mechanism for how all humans achieve ToM—faster or with delay—through processes of social interaction.
Introduction
Theory of mind (ToM), the ability to understand others' internal states, emerges early in life and develops in childhood (Wellman, 2014). Although all humans develop ToM (Yu and Wellman, 2022a), they do so at different paces. For example, children with autism spectrum disorders and deaf and hard-of-hearing (DHH) children usually have delayed ToM development (Peterson and Wellman, 2019; Yu et al., 2021b), whereas bilingual children often develop ToM more quickly than other children (Yu et al., 2021a). Even within typically-developing children, some develop ToM more quickly and some more slowly (Wellman et al., 2001; Yu and Wellman, 2022b).
Where do these differences come from? Researchers have explored many factors that potentially contribute to ToM individual differences, including neurobiological differences and maturation (e.g., brain development), domain-general cognitive skills (e.g., executive function; Devine and Hughes, 2014), domain-specific linguistic experiences (e.g., meta-linguistic and social-linguistic skills; Yu et al., 2021a), pretend play experience (e.g., Taylor and Carlson, 1997), early social conversations (e.g., mental state talks; Ensor et al., 2014), parental mind-mindedness (e.g., Hughes et al., 2018), and more. Among all these factors, social interaction with people, in particular, has been proposed to be a crucial cause (Astington, 2001; Carpendale and Lewis, 2004). For example, a meta-analysis summarizing 45 studies with 4996 children showed that the number of siblings is associated with children's ToM performance (Devine and Hughes, 2018)—children having one or more siblings pass ToM tasks before children with no siblings (see also Perner et al., 1994). Also, in a recent comprehensive review of how children's social tendencies can shape ToM, Lane and Bowman (2021) argued that children's tendencies to attend to social information and engage in social interaction play critical roles. These various studies argue that more social interaction means increased opportunities to cognize others and their internal states, thus enhancing ToM; in contrast, less social interaction restricts such opportunities, thus delaying ToM development.
Although all their findings are correlational in nature, based on these studies, including longitudinal-predictive studies where early social interactions predicted later ToM (e.g., Brink et al., 2015), it is safe to assume that social interactive experiences causally influence ToM development. However, past research does little to establish what sorts of interaction conditions shape and illuminate this link. We reasoned that manipulations within a casual experiment could do so.
Hypothetically, consider a thought experiment with a ten-group between-subject design. Young children with under-developed ToM are randomly assigned to ten groups (i.e., 200 children per group). We then manipulate the amount of social interaction experienced for each group. In Group 1, children are allowed to interact with another child one time a day. In Group 2, children are allowed to interact two times a day; in Group 3, they are allowed to interact three times a day, and so on. Then we track each child's ToM development every day to document how long (e.g., how many days/years) each child takes to fully develop his/her ToM. The expected result confirming a causal link from social interaction to ToM would be that children in groups with more social interaction (e.g., groups 7–10), on average, develop faster than children in groups with less social interaction (e.g., groups 1–4). Assuming this experiment provides firm findings that social interaction influences ToM development, it could then, more importantly, inform us about how it does so.
However, a thought experiment like this is impossible to conduct because manipulating and controlling children's extended, real-life social interaction is not feasible as well as unethical: How could researchers in good conscience recruit children for such a study; what parent would allow their child to participate; how could children's social interactions be precisely controlled; how could children's ToM be measured over weeks and months every single day given the limits of existing ToM assessments (e.g., false belief tasks) and the fact that ToM grows slowly over childhood (Wellman, 2014)? As a result, the links between social interaction and ToM remain indirectly and patchily demonstrated or investigated.
Here, we take a different approach: exploring social interaction-ToM links via a precise thought experiment on computers. Building upon the assumption that social interaction increases ToM, we use computational modeling, in the form of agent-based modeling (ABM), to demonstrate how social interaction, in different degrees and forms, may influence ToM development. ABMs are simulations where cognitive agents interact over time and space under specified conditions. ABM offers opportunities to do what is infeasible in the real world (Kennedy et al., 2009; Madsen et al., 2019), such as the unethical/impractical experiment mentioned earlier. As such, our ABM approach offers a valuable complement to existing correlational data by providing computational explorations that contribute to a more comprehensive understanding of the relationship between social interaction and ToM.
In our model, we set up a ten-group between-subject design, where we simulated agents (i.e., children) with almost no prior ToM competence and assigned them to ten groups. Each group was manipulated to allow a different amount of social interaction, from 1 to 10, within a time interval (i.e., a tick of a computer clock; for a child, e.g., an hour). Within each group, agents randomly roamed and occasionally ran into one another, and when they did, they interacted. If these interactions continued long enough, their ToM probabilistically improved following a loosely Bayesian learning formula, developing from near 0 (almost no ToM competence) to 1 (fully developed ToM). We tracked each agent's ToM development over time and how much time (i.e., how many ticks; for a child, e.g., how many hours and days) each agent needed to fully develop ToM.
More importantly, we tested several targeted manipulations to show how social interaction may influence the pace of ToM development. One manipulation was the sample size for a group of agents, where we manipulated four sample sizes: 50, 100, 150, and 200 agents. Another manipulation was whether agents began with a prior, established, existing social network or not (Barabási and Albert, 1999). Having no existing social network means that agents did not know each other before the experiment (e.g., for a child, the 1st day of a new school), so they would randomly move around in an environment and interact with the agents they encountered. In contrast, having an existing network means that agents had connections with some other agents before the experiment (e.g., for children, they already knew each other), so they would interact preferentially with their known as opposed to unknown neighbors. Further, we tested whether and how being initially central to a network (e.g., for children, perhaps, being popular or sought out) or not (e.g., perhaps, being shy) influenced ToM increases via social interaction. We computed each individual agent's network centrality (Zaki et al., 2014) at the beginning before they started their focal interactions. An agent who was more central in a social network had more social connections and thus more opportunities to engage in more social interaction than peripheral agents with a low network centrality. These additional manipulations also allowed us to test whether the links between social interaction and ToM are robust and how they function in various contrasting contexts.
Simultaneously, our study enabled us to track the developmental trajectory of each individual agent's ToM over time, thereby allowing us to assess the influence of their individual variation in social interaction instances on corresponding ToM competence within the group. This individual-focused analysis provides an examination of how social interaction instances impact ToM competence at a more nuanced level. Importantly, such individual-focused data align with the format commonly employed in correlational studies investigating social interaction-ToM links. Because, in this form, our model captures individual variation, we were able to compare our model results with empirical data from a cohort of 84 young DHH children (Yu et al., 2021b). This comparison aimed to ascertain the extent to which our model can effectively explain real-world data, further illuminating the capacity of our model to elucidate the social interaction-ToM links.
In sum, our model was essentially an extended version of an optimal but unethical/impractical thought experiment described earlier. Furthermore, because we conducted the interactions computationally, we were able to repeat the various experimental conditions 50 times each to assess the robustness of our results. A screenshot of the crucial aspects of our model (written in Netlogo programming language; Wilensky and Rand, 2015) is presented in Figure 1. The model can be easily run using Netlogo software: https://ccl.northwestern.edu/netlogo/. Overall, our design, exploiting the assumption that social interaction increases ToM, allows us to address questions such as: (1) How does having vs. not having an existing network influence social interaction and thereby ToM competence? (2) How does an agent's network centrality influence the pace of ToM development? (3) Can our ABM realistically link to real-world data? The model, the source code, and the simulated data we show in this study are all available in Supporting Information. Readers can run our model and experiment with it themselves to experience how social interaction influences ToM development.
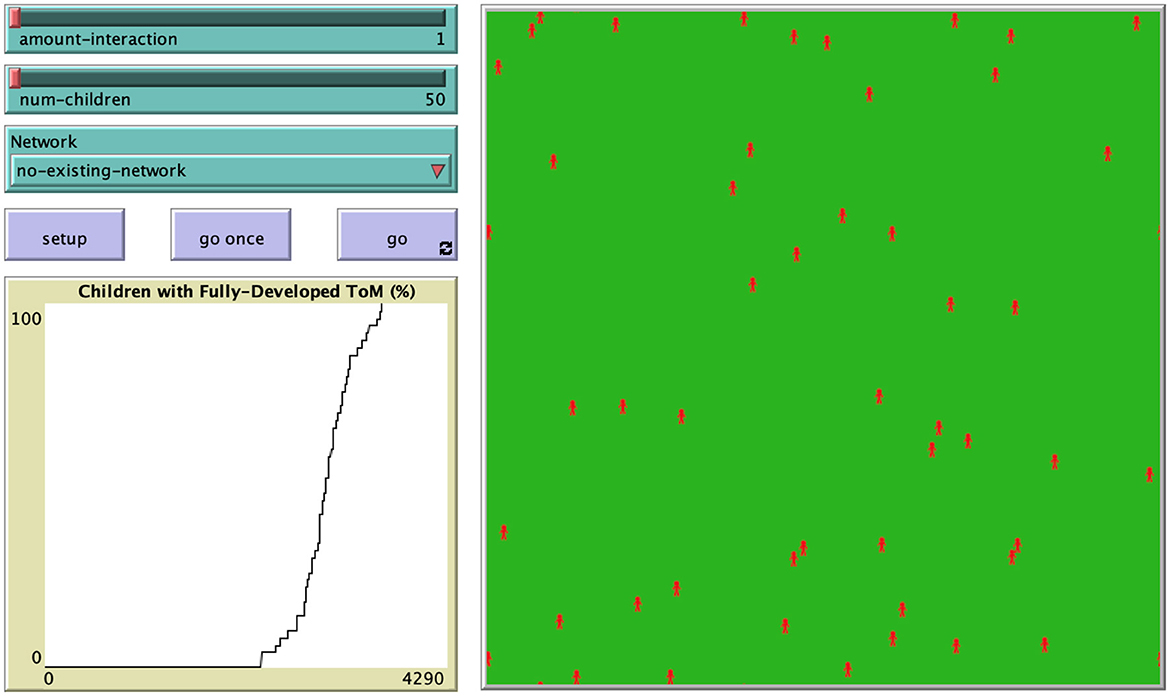
Figure 1. Our ABM built in Netlogo. The top left panel shows the focal experimental manipulations (e.g., ten different amounts of social interaction, four sample sizes, and two network scenarios). The bottom left panel shows the results of one example run as it proceeded over 4,290 ticks. The right panel graphically illustrates the child agents (i.e., the red human symbols).
Methods
Overall design
Our ABM was conducted in Netlogo (Wilensky and Rand, 2015), a language packaged with a programmable ABM environment. The overall design of our specific ABM was a ten-group between-subject experiment. We simulated identity-less agents (hypothetically, children) and randomly assigned them into ten experimental groups. Within each group, agents randomly roamed and occasionally ran into one another; when they did, they interacted. If these interactions accumulated sufficiently, their ToM probabilistically improved. Importantly, each group controlled each agent's maximal amount of social interaction within a tick of a computer clock (for a child, e.g., an hour). Groups 1–10 allowed agents to interact 1–10 times per tick, respectively. We recorded each agent's ToM development over time (ToM competence) and how much time (i.e., how many ticks of a computer clock; for a child, e.g., how many hours and days) each agent needed to fully develop their ToM. Using this model design, we explored how different amounts of social interaction influenced the pace of ToM development.
Sample size
To test whether the effects of social interaction manipulations were robust for different numbers of agent-interactors, we manipulated four different sample sizes in a group: 50, 100, 150, and 200 agents. Thus, the total sample sizes for experiments range from 500, 1,000, 1,500, to 2,000 (the sample size in a group * 10 groups).
Network scenario
To explore hypothetically relevant factors, we manipulated agents' network history—whether agents began with an existing social network or not. The existing network was the preferential attachment network in the Netlogo library (Barabási and Albert, 1999). Having no existing social network meant that agents had no links to one another before the experiment, so they would randomly move around within a set environment (a grid of 40 * 40 patches; the environment size remains the same for all agent sample sizes) and interact with whoever they encountered on that patch. To illustrate, picture a fixed-size neighborhood where individuals can roam freely and may run into others on the street (e.g., for a child, the 1st day of a new school). In contrast, having an existing network meant that agents had prior links to some other agents before the experiment (e.g., for children, they know each other) so that they would interact preferentially with their prior-linked “friends.”
These additional manipulations resulted in eight experimental setups, one for each combination of the four sample sizes and two network scenarios (4 * 2). Note that these critical experimental manipulations (i.e., ten amounts of social interaction, four sample sizes, and two network scenarios) are shown at the top left panel of our Netlogo model (Figure 1) and are set before running the model.
Agents and interaction
In our ABM, each agent represents a child. Within Netlogo, each agent can be defined to have “properties” that can take on different values. Our agents had a property of ToM competence. The initial ToM value was 0.0000001, meaning that the agent had almost no ToM (at the beginning). A higher ToM value represents better ToM competence, and the maximal ToM value is 1. An agent with ToM = 1 means that it has fully developed ToM.
The agents roamed in the environment, and when two agents moved into the exact same location (a patch representing one of the patches within a grid of 40 * 40 patches), this constituted an interaction-encounter—they interacted with each other. Note that in our ten-group between-subject experiment, each group controlled each agent's maximal amount of social interaction, 1–10, within a tick of a computer clock. The length of each interaction was simulated using a random number generator, which generates a random number from a uniform distribution (range from 0 to 1.5). If the interaction length was bigger than 1 (the interaction continued long enough), then the two agents' ToM probabilistically improved (see below). Having a threshold of 1 (with a full range of 1.5) means that only 33% of such interactions are long enough to be meaningful.
In addition, in the scenario where agents began with an existing social network, each agent also had a second property of network centrality computed based on eigenvector centrality (Zaki et al., 2014). A high value means that an agent is more central in a social network, so he/she would have more connections and opportunities to engage in more social interaction (e.g., have more neighbors to interact with). Conversely, a low value means an agent does not have many links with other agents and thus is less accessible and has fewer opportunities to interact with other agents.
Development
After each interaction, and if the interaction continued long enough to be meaningful, agents' ToM probabilistically developed via the following formula: Posterior ToM = Prior ToM × Interaction length × eThe other perso n ′s ToM.
This formula augments some prior ToM value into an increased posterior value and is loosely based on a Bayesian learning framework: Posterior ToM ∝ Prior ToM × Data. Gopnik and Wellman (2012) elaborate on how Bayesian learning formulations can account for ToM learning and development. For our model, however, our formulation is simply a way to increment an agent's ToM maturity (from almost 0% up to 100%), given that they interact and interact with another agent with its own level of ToM maturity. Thus, in our formula, we parametrized the Data component in a loosely Bayesian learning framework as (1) interaction length and (2) the other person's ToM. Having interaction length in the formula means that if an agent interacts longer (under the case that such interactions are long enough to pass the cutoff of 1), he/she presumably accumulates more data and experience, leading to more ToM learning. On top of that, the formula also considers the other agent's ToM. Previous studies have suggested that interacting with a child with more advanced ToM competence (e.g., an older child) helps children learn more about mental states than interacting with a child with less developed ToM competence (e.g., a young child). For example, young children with frequent social interaction with an older sibling develop ToM faster (Dunn et al., 1991; Ruffman et al., 1998). Our formula incorporates these empirical findings by parametrizing the other person's ToM—interacting with those agents who have “higher” ToM facilitates ToM learning for the present agent himself/herself.
In two illustrative examples, if an agent has a prior ToM of 0.01 and encounters another agent with a ToM of 0.01 with an interaction time of 1.5, his/her posterior ToM after such an interaction would be 0.01 × 1.5 × e0.01 = 0.0151, indicating an improvement of ToM from 0.01 to 0.0151. Or, if an agent has a prior ToM of 0.01 and encounters another agent with a ToM of 0.5 with an interaction time of 1.25, his/her posterior ToM after such an interaction would be 0.01 × 1.25 × e0.5 = 0.0206, indicating an improvement of ToM from 0.01 to 0.0206.
Results
To reiterate, we began by exploiting and establishing the assumption that different amounts of social interaction influence the pace of ToM development within our model. Then we addressed three questions: (1) How does having vs. not having an existing network influence social interaction and thereby ToM competence? (2) How does an agent's network centrality influence the pace of ToM development? (3) Can our ABM realistically link to real-world data?
Different amounts of social interaction influence the pace of ToM development
In our model, we recorded how much time each individual agent (e.g., child) took to fully develop its ToM. Then, we aggregated the data from each group to indicate how much time, on average, an agent needed to fully develop its ToM (or how much time an average agent needed to fully develop its ToM). This demonstrated, as a baseline, how different amounts of social interaction between groups (i.e., each group allowed a different amount of social interaction) influence the pace of ToM development.
In a one-way between-group analysis of variance (ANOVA), the independent variable was the per-group different amounts of social interaction (1 to 10); the dependent variable was the average time an agent in each group needed to fully develop ToM. We repeated the model 50 times for each of the 10 amounts of social interaction, so that there were 500 observations for the one-way ANOVA. This format was used in eight planned one-way between-group ANOVAs, one for each combination of the four sample sizes and two network scenarios.
As evident in Figure 2, there were significant differences between the ten groups, differences that held across four sample sizes and two network scenarios—F(9, 490) values range from 7,484 to 48,591, all p-values <0.001. On average, agents in groups that allowed more social interaction developed significantly faster than agents in groups with less social interaction—as clear in Figure 2 on average, an agent in group 1 required the most time to fully develop his/her ToM, and an agent in group 10 required the least time.
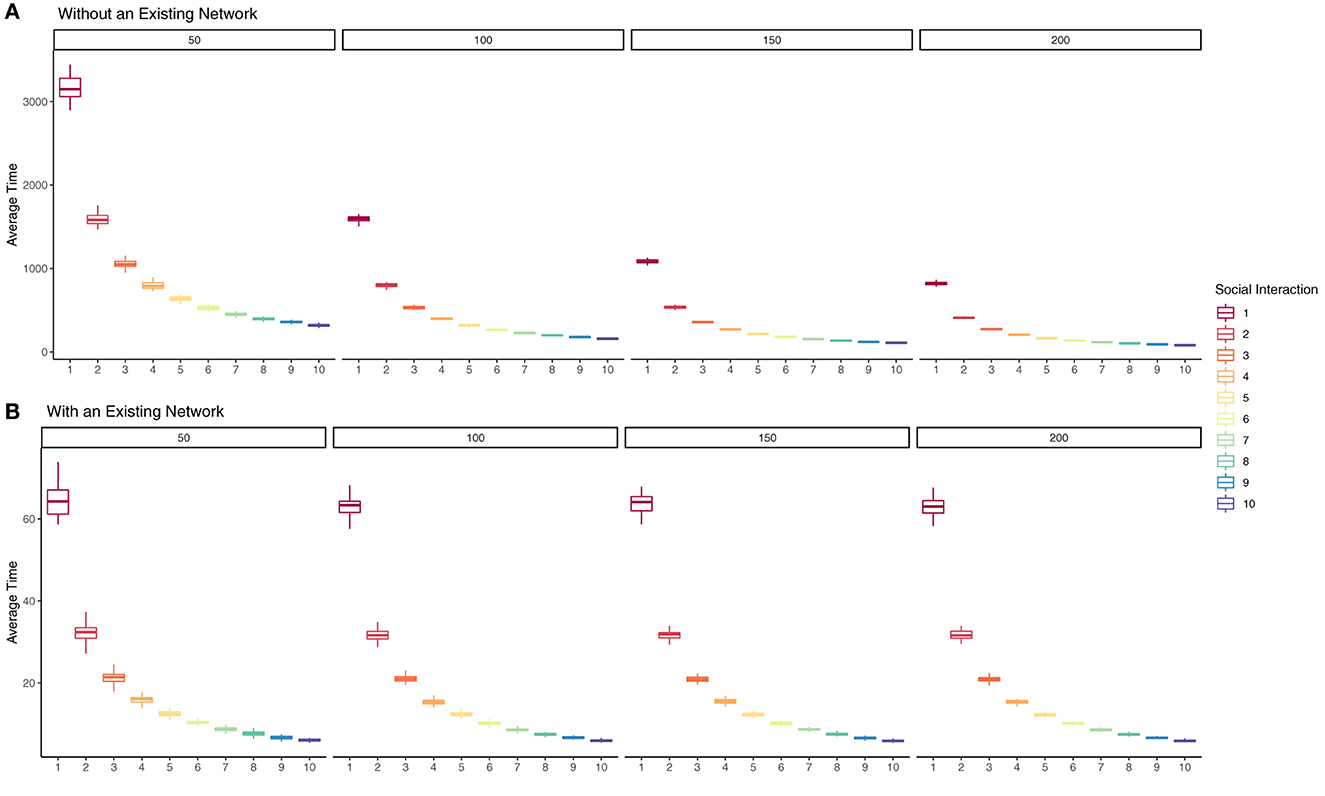
Figure 2. The average time needed for an agent to fully develop ToM across four sample sizes, two network scenarios, and ten different amounts of social interaction. Note that the simulations of the experiment for each situation were run for 50 repetitions. The boxplots depicted the distributions of the 50 repetitions. (A) Without an existing network. (B) With an existing network.
In addition, we tracked, within a group, the percentage (0 to 100%) of agents who had fully developed their ToM as time accumulated. For this, we used a similar analysis format in eight planned one-way between-group ANOVAs across four sample sizes and two network scenarios. The per-group different amounts of social interaction (1 to 10) were the independent variable. The total time a group of agents needed for all of them to fully develop ToM (i.e., to achieve 100%) was the dependent variable. Again, we repeated the model 50 times, so the total observations were 500 in each ANOVA.
Just as for the first analysis, and as apparent in Figure 3, there were significant differences between the ten groups, and the effects held across four different sample sizes and two network scenarios—F(9, 490) values range from 4,872 to 17,481, all p-values <0.001. Groups allowing more social interaction achieved 100% (all the agents fully developed ToM) faster than groups allowing less social interaction; group 1 required the most time for all the agents to fully develop mature ToM, and group 10 required the least.
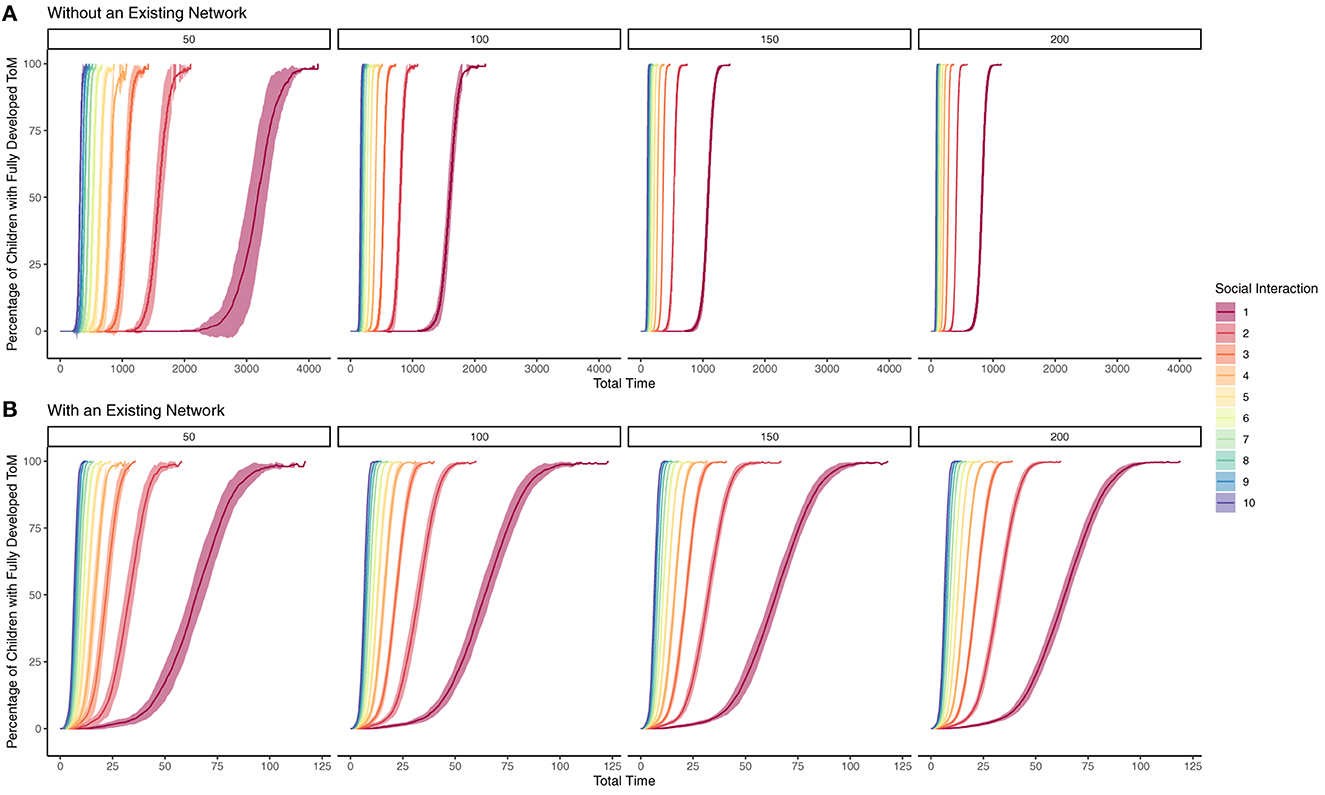
Figure 3. The percentage of agents with fully developed ToM over time, across four sample sizes, two network scenarios, and ten different amounts of social interaction. The X-axis shows the total time, and the y-axis shows the percentage of agents with fully developed ToM. Note that the simulations of the experiment for each situation were run for 50 repetitions. The shaded areas indicate the standard deviations computed based on those 50 repetitions. (A) Without an existing network. (B) With an existing network.
Moreover, in addition to manipulating social interaction at the group level across 10 amounts, we assessed variations among individual agents within their respective groups. Within each group, we observed individual differences, with some agents engaging in a higher number of social interaction instances within a given time window, while others participate in fewer instances. By simultaneously recording ToM levels, our ABM recorded every step of the process at all time points in terms of each individual agent's ToM competence and the number of social interaction instances they were involved in. This enabled us to explore whether and how individual agents who engaged in a greater number of social interaction instances demonstrated higher levels of ToM competence.
In this test of the per-group association between social interaction instances and ToM competence, as our focus was on the individual agent's social interaction instances and corresponding ToM competence, we set the between-group manipulation of social interaction = 1. Furthermore, we specifically targeted all time points where at least 10 percent, but not all agents, had achieved full ToM development. This ensured a sufficient range of variation to explore the associations, as having all agents with fully developed ToM would eliminate any variability in their ToM competence. Given the iterative nature of our model (50 repetitions) and the abundance of time points, we established empirical distributions of Pearson r correlation coefficients for each sample size and network scenario. Figure 4 illustrates these r-distributions for all four sample sizes and two network scenarios.
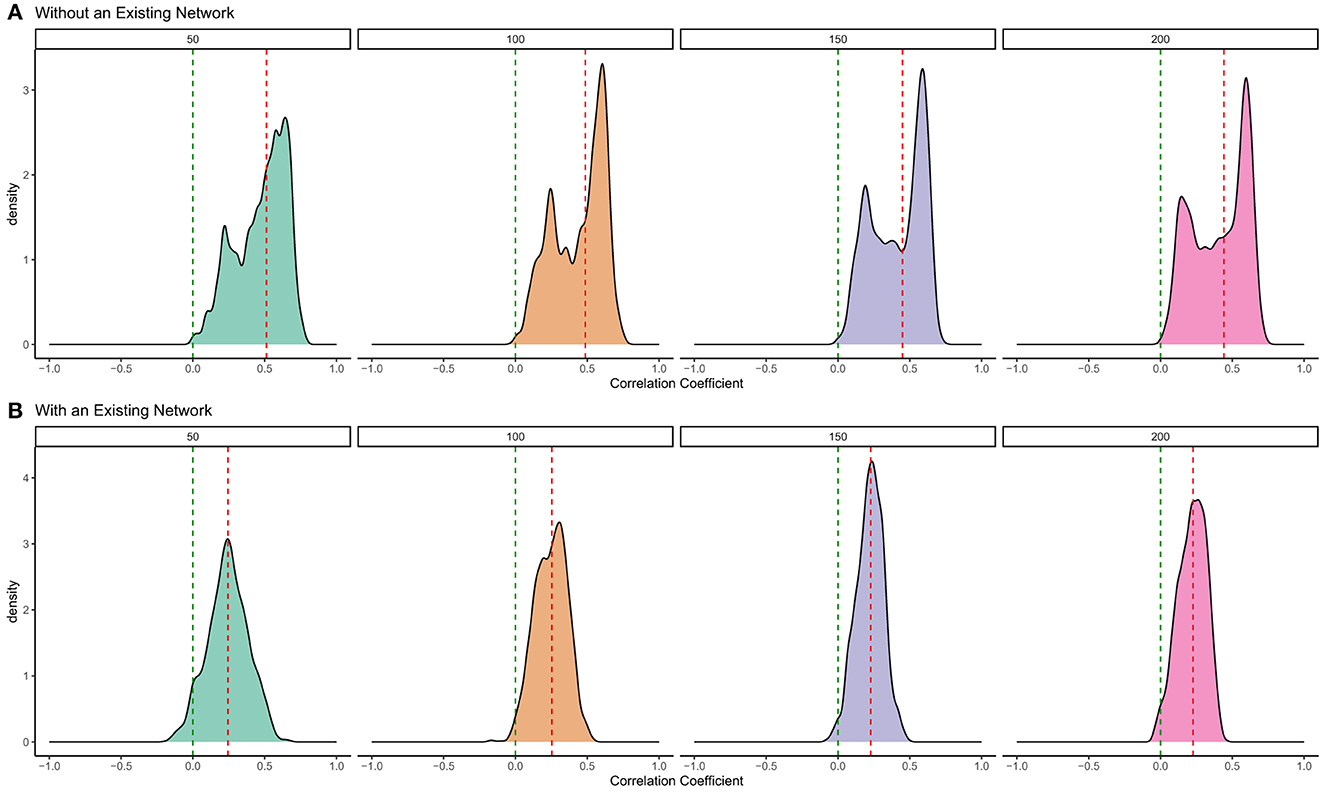
Figure 4. The relationship between individual agents' social interaction instances and ToM competence at time points with at least 10 percent, but not full, ToM development across four sample sizes (given the between-group manipulation of social interaction = 1). (A, B) Depict the r-distributions of correlation for scenarios without and with an existing network, respectively. The dashed red lines indicate the median values of the r-distributions (all > 0). The dashed green lines indicate r = 0. Most values in all distributions were located to the right of the dashed green lines, indicating a high probability of positive correlation for each distribution. (A) Without an existing network. (B) With an existing network.
A consistently positive correlation was observed between social interaction instances and ToM competence, where the median values of the r-distributions were positive, ranging from 0.23 to 0.51, for all four sample sizes and two network scenarios (Figure 4; the dashed red lines indicate the median values of the r-distributions). Furthermore, we calculated the probabilities of the correlations being positive—they ranged from 95 to 99% across all four sample sizes and two network scenarios (most values in all distributions were positioned well to the right/positive side of the dashed green lines in Figure 4 which indicates r = 0).
Supplementary Figure S1 in our Supporting Information further demonstrates that positive correlations are not limited to the between-group manipulation of social interaction = 1 but are observed across all ten amounts. The median values of the r-distributions were all positive, ranging from 0.21 to 0.54, across all four sample sizes, two network scenarios, and ten amounts of between-group social interaction manipulations. Similarly, the probabilities of positive correlations between social interaction instances and ToM competence ranged from 93 to 99%. Overall, these findings suggest that engaging in more social interaction instances is associated with greater ToM competence. Again, this effect holds true across various sample sizes and network scenarios, providing robust evidence for the relationship.
How does having vs. not having an existing network influence social interaction and thereby ToM competence?
Based on the baseline results in the previous section, we more focally examined the influence of other social-interaction factors on ToM development. First, we examined the difference between having an existing network vs. not having an existing network. As detailed in our Methods, having no existing network meant that agents had no links to one another before the simulation, so they would randomly move around within a set environment and interact with whoever they encountered. Having an existing network meant that agents had prior links to some other agents before the simulation so that they would have their linked “friends” to interact with. This setup leads to substantial differences in terms of the likelihood of agents having an interaction—having neighbors nearby would be much more likely to trigger interactions than roaming freely on the street.
As clear in Figures 2, 3, our results demonstrated that beginning with an existing network substantially facilitated the pace of ToM development. We further used a one-way ANOVA to evidence the results more clearly (note that a simple t-test could be used, but for consistency purposes, we chose the same ANOVA setup as before). In such ANOVAs, the independent variable was the two network scenarios (beginning with an existing network vs. not beginning with an existing network), and the dependent variable was the average time an agent in each group needed to fully develop ToM. We repeated the model 50 times for each network scenario so that there were 100 observations for the one-way ANOVA. This format resulted in forty planned one-way between-group ANOVAs, one for each combination of the four sample sizes and ten different amounts of social interaction. As evident in, for example, Figure 2, there were significant differences between the two network scenarios, differences that held across four sample sizes and ten different amounts of social interaction—F(1, 98) values range from 13,689 to 39,469, all p-values <0.001. On average, agents who began with an existing network developed much faster than agents who began without an existing network.
How does an agent's network centrality influence the pace of ToM development?
In the scenario where agents (e.g., children) began with an existing social network, we computed network centrality of each agent at the beginning before they started interacting (Zaki et al., 2014). This allowed us to test, within each group, whether different levels of network centrality influence how much time agents need to fully develop ToM. Because here we focused on each individual agent's network centrality and its developmental pace to demonstrate the relevant effects of network centrality, we set the between-group manipulation of social interaction = 1.
We explored the per-group association between levels of network centrality and the time an individual agent needed to fully develop ToM. We did this across four sample sizes. Figure 5A shows an example scatter plot for the correlations. As clear, there was a negative correlation between network centrality and the time needed—higher centrality was associated with less time to fully develop ToM—and this effect held across four sample sizes.
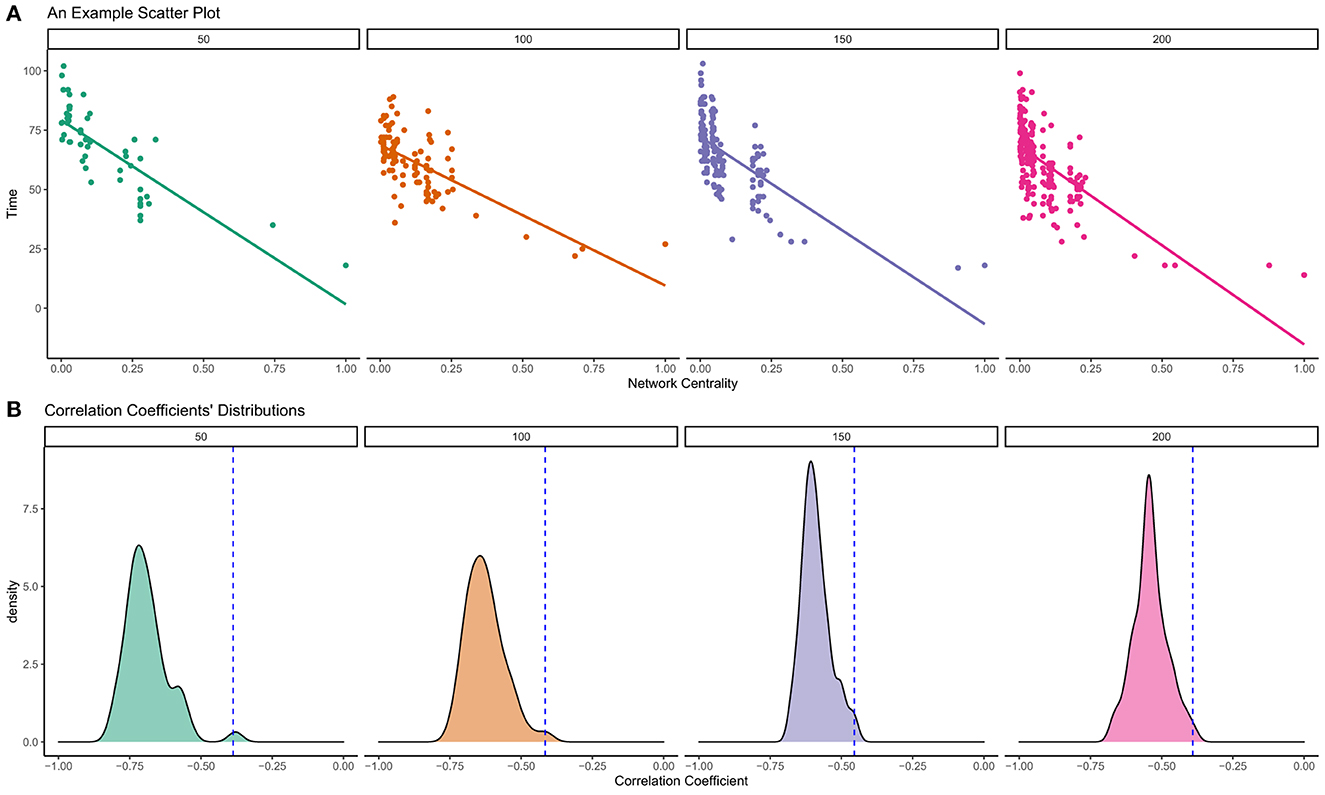
Figure 5. The relationship between network centrality and the time an individual agent needed to fully develop ToM across four different sample sizes (given the between-group manipulation of social interaction = 1). (A) Shows the scatter plots with correlation analyses of an example run. (B) Shows the r-distributions. The dashed lines indicate the 99.9% quantile of these r-distributions (r values at the 99.9% quantile = −0.39, −0.42, −0.45, and −0.39 for sample sizes = 50, 100, 150, and 200 respectively). (A) An example scatter plot. (B) Correlation coefficient's distributions.
Again, we repeated the model 50 times, so there was an empirical distribution of Pearson r correlation coefficients for each sample size. These empirical distributions helped better estimate if such a negative correlation was significant. Figure 5B shows the r-distributions for all four sample sizes. As clear in Figure 5B, all negative correlations were significant at a p < 0.001 level: the 99.9% quantile of these r-distributions were lower than 0 (r values at the 99.9% quantile = −0.39, −0.42, −0.45, and −0.39 for sample sizes = 50, 100, 150, and 200 respectively).
In Supplementary Figure S2 in our Supporting Information, we further show that the negative correlations were not only significant under the between-group manipulation of social interaction = 1, but also across all ten amounts of social interaction (the 99.9% quantiles of all the r-distributions were lower than 0; the r values at the 99.9% quantile ranges from −0.27 to −0.52). These negative correlations demonstrate that agents who were more central in a social network developed ToM faster than those who were not central.
Can our ABM realistically link to real-world data?
By looking at the individual-level associations between social interaction instances as a predictor and ToM competence as an outcome (e.g., Figure 4), it is possible to establish a connection between our ABM and real-world data. This is because, in this form, our ABM data align with the reporting format commonly found in existing studies, where a variable of interest is employed as a predictor (in this case, social interaction indexed via some measure) and ToM competence is assessed as an outcome (measured via, e.g., false-belief task performance or ToM Scale score).
While many studies capture social interaction via a brief snapshot (e.g., a child's social interaction instances during a half-hour time sample at preschool), we reasoned that a measure that indexed cumulative interactions over months or years would be more informative and better aligned with our ABM. To this end, we turned to data from DHH children born to hearing parents, specifically focusing on those who received early hearing provisions such as cochlear implants or advanced hearing aids. Prior DHH research has shown that ToM development as measured in kindergarten varies according to the timing of provisions in earlier childhood of children's hearing devices, with earlier provision meaning more months of hearing by kindergarten age and thus indexing greater accumulation of interactive hearing experiences and language ability (e.g., Yu et al., 2021b). This influence is found to surpass the effects of chronological age and other factors within these DHH samples. Thus, the data from DHH children are especially informative because DHH children demonstrate huge variabilities in both their ToM and their language abilities, unlike typically developing children whose chronological age serves as the greatest determinant (a proxy for all unobserved and unmeasured processes) for ToM development. These variabilities in DHH children allow a clearer differentiation and exploration of potential causal relations in that their social-conversational interaction experiences are manifested in their language ability (Yu et al., 2021b).
Building on this background, we utilized data from a sample of 84 young DHH children (average chronological age = 4.64 years) with hearing parents, sourced from Yu et al. (2021b). In our analysis, we focused on two variables to parallel the two variables—social interaction and ToM competence—in our ABM. For social interaction, we used language abilities (measured by raw scores obtained from the Peabody Picture Vocabulary Test; Dunn and Dunn, 1997). In Yu et al. (2021b), accumulated months since providing hearing devices demonstrably influenced language ability, which then, in turn, influenced ToM. Thus, language competence serves as an empirically validated proxy for earlier conversational-communicative interaction experiences. Note that there are other types of social interactions across development that do not involve hearing and language (e.g., playing non-verbally with parents; gesturing), but for young DHH children, a focus on language and language-based interactions is sensible. This is because, in the case of young DHH children with hearing parents, their hearing parents (their main source of social interaction experiences) do not sign and instead prefer to and are encouraged to speak to their children. Clinicians encourage this to ensure that the children use their hearing provisions (e.g., their cochlear implants, which at first can be annoyingly noisy and require effortful continued use to provide auditory benefits). Because language-based interactions are emphasized, language competence serves as a reasonable proxy for their accumulated social interactions. For ToM, we used the level of ToM competence assessed using the Wellman and Liu's (2004) ToM Scale. In this way, these real-world data provide a meaningful basis for investigating the relationship between social interaction and ToM competence.
To examine how well our ABM aligns with these DHH real-world data, we focused on the ABM results involving 100 agents, as it closely matched the sample size of 84 DHH children in the real-world dataset. For the sake of simplicity, we specifically considered ABM results where the between-group manipulation of social interaction = 1. To effectively capture the relationship between social interaction instances and ToM competence in our ABM, we randomly selected a representative ABM result that had a median r value from our aforementioned analysis in Figure 4 (i.e., one data point from the dashed red line in the N = 100 panel of Figure 4B). Then we trained a power 2 polynomial regression model to learn the underlying relationship between social interaction instances and ToM competence within this selected, representative ABM data point.
Then we fit the ABM-trained polynomial regression model to our DHH data, generating model-predicted ToM competence values for the DHH children, which could be compared to the actual ToM competence values for the DHH children, as reported in Yu et al. (2021b). The results revealed a significant positive correlation between the model-predicted and actual ToM competence (r = 0.26, p < 0.05), indicating a reasonable alignment between our ABM and the real-world DHH data.
In fact, the effect was found not only in the between-group manipulation of social interaction = 1. Consistent patterns were observed across all ten amounts of the between-group manipulation of social interaction, with correlations ranging from 0.21 to 0.27 (all ps <0.05). These findings confirm the correspondence between our ABM and the real-world DHH data.
Discussion
A real-life experimental test of hypothesized causal links between social interaction and theory of mind (ToM) is neither ethically nor practically possible. To shed light on these links, we explored them and the potential mechanisms underlying these links with simulated agents (e.g., children) and interaction-situations via agent-based modeling (ABM).
We began with a baseline assumption resulting from prior correlational and longitudinal-predictive research that social interaction causally influences ToM development, and then first explored within our model how different amounts of social interaction influence the pace of ToM development. Our results showed that more social interactions indeed facilitated the pace of ToM development. This effect was evident, across two network scenarios and four sample sizes, when considering both the average developmental pace across individual agents (Figure 2) and the collective developmental pace of entire agent groups (Figure 3). Also, the findings equally highlight the importance of cumulative social interaction instances, where increased engagement in social interactions was associated with higher levels of ToM competence at any given time point of the ABM simulations (Figure 4).
Having confirmed the expected link between social interaction and ToM in our model, we examined more focal questions and factors via three questions. First, how does having vs. not having an existing network influence social interaction and thereby ToM competence? Our findings illustrated that beginning within a prior, established network substantially impacted how quickly agents achieved ToM competence (Figures 2, 3). This result sensibly reflects real-world interactions where it is arguably more challenging to randomly roam among and interact with other unknown children on the street than hanging out with friends and acquaintances. This effect—an advantage for presence as opposed to absence of an established network—was robustly observed across four sample sizes and ten distinct amounts of social interaction.
Similarly, for a second question—How does an agent's network centrality influence the pace of ToM development?—We showed that when agents commenced with an existing social network, the extent of network centrality influenced the pace of ToM development (Figure 5). By implication, in real-life central/popular agents (i.e., children) with more connections grow their ToM faster than peripheral agents with fewer connections. This result offers a computational explanation for correlational findings in the literature on peer popularity and ToM (e.g., Slaughter et al., 2015). This effect too—the benefit of network centrality—was robustly observed across four sample sizes and ten distinct amounts of social interaction (Supplementary Figure S2).
These results led to our third research question: Can our ABM realistically link to real-world data? Our model's outcomes indeed aligned with empirical real-world findings from deaf and hard-of-hearing (DHH) children, where increased language-based social interactions, enabled by earlier rather than later provision cochlear implants and advanced hearing aids influenced language competence which in turn led to enhanced ToM development. Via these exemplary findings, our model, despite being an indirect computational simulation, helpfully illuminates the real-world influences of social interactions on ToM development more generally.
As noted in our introduction, considerable research documents associations between indexes of children's social interaction on their ToM development. For example, studies have reported that young children's conversational environments and exchanges relate to their ToM competence; more conversational exchanges presumably increase children's opportunities to learn about their conversational partners' mental states, leading to positive associations with ToM development (De Rosnay and Hughes, 2006). Studies have also consistently shown that larger social contexts of family and peers (e.g., the number of siblings or schoolmates) as well as broader social-interactive features (e.g., parental talk and especially mental states talk) positively relate to ToM development (Devine and Hughes, 2018). Longitudinally, early infant/toddler social interactive experiences predict enhanced ToM in preschool (e.g., Brink et al., 2015). Other studies have focused on children's personality and temperament; for instance, a higher tendency to approach social situations and engage in social interactions positively enhances ToM development (Wellman et al., 2011; Lane and Bowman, 2021).
By hypothesis, all these findings show that social interaction provides opportunities to engage in learning about other people's mental states, thus facilitating ToM development. Results from our ABM provide complementary support to these correlational findings and illuminate how the links between social interaction and ToM might work. Just as correlational studies offer only indirect and partial support, our computational modeling approach, because of its computational rather than real-life nature, also only provides indirect and partial support and instead focuses more on how the links may entail. Fortunately, considering the collective evidence from both correlational studies and computational modeling results provides a more complete picture of the complex relationship between social interaction and ToM development.
Intriguingly, our data suggest that the link between social interaction and ToM development may well not be linear. That is, increasing the amount of social interaction from 1 to 2 and increasing the amount from 9 to 10 had different effects. As shown by the flattening slopes in Figure 2, for example, increasing the amount of social interaction from 1 to 2 had a large effect on the pace of ToM development (the needed time to achieve ToM maturity was almost halved), whereas increasing the amount of social interaction from 9 to 10 had an almost negligible effect.
At first glance, this finding might seem to contradict existing studies because the literature on the social correlates of ToM apparently demonstrates linear relationships between social interaction and ToM development (Devine and Hughes, 2018). But, these studies are based almost exclusively on linear regression analyses, so the apparent linearity may be due to the constraints and assumptions of linear regression. Indeed, recent developmental research has challenged this linear approach to understanding social causation in child development because social factors often have complex and unpredictable interactions with developmental outcomes (Hertzman and Boyce, 2010). Our findings support this view by providing a clear pattern of non-linear effects. In our results, although having more social interaction is generally beneficial, it is even more beneficial early on for agents who begin with relatively limited social interaction.
One might argue our model is remarkably unlike real life. For example, no child would only have one interaction per day and be isolated for the rest of the day; children interact not only with similar children but with adult parents and caregivers; interaction partners' ages, same or different, would matter; and there are undoubtedly many other hidden factors which require future research to offer alternative or complementary explanations. We use our model only to show, in principle, how social interaction influences ToM development. Here, it is helpful that this modeling approach provides data that help explain the findings observed in DHH children. Nonetheless, our work is a simplified first step in modeling how social interaction may impact ToM development and the ways it may do so.
Our model is simplistic in one other important fashion. We made little attempt to computationally model how ToM develops step-by-step, with early steps providing the building blocks for generating later insights. We computed ToM progression only as going from almost 0 to 1 (1 as 100% maturity) and incremented ToM only via a simplified formula in a loosely Bayesian learning framework; that is, a prior level of ToM was increased given the length of an interaction with another agent and that other agent's own ToM maturity. Uncovering the computational processes underlying the building of ToM understandings is being tackled in other computational research, such as that using Bayesian learning models (Gopnik and Wellman, 2012; Baker et al., 2017), neural networks (Berthiaume et al., 2013), and reinforcement learning models (Jara-Ettinger, 2019). Combining ABMs and other computational learning models seems an exciting future direction. The current initial ABM of ToM development helps show how future research of this more complex sort would be feasible.
In conclusion, the present study contributes to our understanding of ToM development through the perspective of computational agent-based modeling. Our demonstrations empirically show how social interaction can account for differences in the pace of ToM development and illuminate various social interaction-ToM links. Our study not only helps show how differences in ToM development may emerge but also offers a parsimonious explanation to specify a crucial mechanism for how all humans may develop and achieve ToM—faster or with delay—through processes of social interaction.
Data availability statement
The original contributions presented in the study are included in the article/Supplementary material, further inquiries can be directed to the corresponding author.
Ethics statement
Ethical approval was not required for the study involving humans in accordance with the local legislation and institutional requirements. Written informed consent to participate in this study was not required from the participants or the participants' legal guardians/next of kin in accordance with the national legislation and the institutional requirements.
Author contributions
C-LY and HW designed the research and wrote the manuscript. C-LY performed the research and analyzed the data. HW supervised the research. Both authors contributed to the article and approved the submitted version.
Funding
The author(s) declare that no financial support was received for the research, authorship, and/or publication of this article.
Conflict of interest
The authors declare that the research was conducted in the absence of any commercial or financial relationships that could be construed as a potential conflict of interest.
Publisher's note
All claims expressed in this article are solely those of the authors and do not necessarily represent those of their affiliated organizations, or those of the publisher, the editors and the reviewers. Any product that may be evaluated in this article, or claim that may be made by its manufacturer, is not guaranteed or endorsed by the publisher.
Supplementary material
The Supplementary Material for this article can be found online at: https://www.frontiersin.org/articles/10.3389/fdpys.2023.1237033/full#supplementary-material
References
Astington, J. W. (2001). The future of theory-of-mind research: understanding motivational states, the role of language, and real-world consequences. Child Dev. 72, 685–687. doi: 10.1111/1467-8624.00305
Baker, C. L., Jara-Ettinger, J., Saxe, R., and Tenenbaum, J. B. (2017). Rational quantitative attribution of beliefs, desires and percepts in human mentalizing. Nat. Hum. Behav. 1, 64. doi: 10.1038/s41562-017-0064
Barabási, A.-L., and Albert, R. (1999). Emergence of scaling in random networks. Science 286, 509–512. doi: 10.1126/science.286.5439.509
Berthiaume, V. G., Shultz, T. R., and Onishi, K. H. (2013). A constructivist connectionist model of transitions on false-belief tasks. Cognition 126, 441–458. doi: 10.1016/j.cognition.2012.11.005
Brink, K. A., Lane, J. D., and Wellman, H. M. (2015). Developmental pathways for social understanding: linking social cognition to social contexts. Front. Psychol. 6, 719. doi: 10.3389/fpsyg.2015.00719
Carpendale, J. I. M., and Lewis, C. (2004). Constructing an understanding of mind: the development of children's social understanding within social interaction. Behav. Brain Sci. 27, 79–96. doi: 10.1017/S0140525X04000032
De Rosnay, M., and Hughes, C. (2006). Conversation and theory of mind: do children talk their way to socio-cognitive understanding? Br. J. Dev. Psychol. 24, 7–37. doi: 10.1348/026151005X82901
Devine, R. T., and Hughes, C. (2014). Relations between false belief understanding and executive function in early childhood: a meta-analysis. Child Dev. 85, 1777–1794. doi: 10.1111/cdev.12237
Devine, R. T., and Hughes, C. (2018). Family correlates of false belief understanding in early childhood: a meta-analysis. Child Dev. 89, 971–987. doi: 10.1111/cdev.12682
Dunn, J., Brown, J., Slomkowski, C., Tesla, C., and Youngblade, L. (1991). Young children's understanding of other people's feelings and beliefs: individual differences and their antecedents. Child Dev. 62, 1352–1366. doi: 10.2307/1130811
Dunn, L. M., and Dunn, L. M. (1997). Peabody Picture Vocabulary Test–Third Edition. Circle Pines, MN: American Guidance Service. doi: 10.1037/t15145-000
Ensor, R., Devine, R. T., Marks, A., and Hughes, C. (2014). Mothers' cognitive references to 2-year-olds predict theory of mind at ages 6 and 10. Child Dev. 85, 1222–1235. doi: 10.1111/cdev.12186
Gopnik, A., and Wellman, H. M. (2012). Reconstructing constructivism: causal models, Bayesian learning mechanisms, and the theory theory. Psychol. Bull. 138, 1085–1108. doi: 10.1037/a0028044
Hertzman, C., and Boyce, T. (2010). How experience gets under the skin to create gradients in developmental health. Ann. Rev. Public Health 31, 329–347. doi: 10.1146/annurev.publhealth.012809.103538
Hughes, C., Devine, R. T., and Wang, Z. (2018). Does parental mind-mindedness account for cross-cultural differences in preschoolers' theory of mind? Child Dev. 89, 1296–1310. doi: 10.1111/cdev.12746
Jara-Ettinger, J. (2019). Theory of mind as inverse reinforcement learning. Curr. Opin. Behav. Sci. 29, 105–110. doi: 10.1016/j.cobeha.2019.04.010
Kennedy, W. G., Bugajska, M. D., Harrison, A. M., and Trafton, J. G. (2009). “Like-me” simulation as an effective and cognitively plausible basis for social robotics. Int. J. Soc. Robot. 1, 181–194. doi: 10.1007/s12369-009-0014-6
Lane, J. D., and Bowman, L. C. (2021). How children's social tendencies can shape their theory of mind development: access and attention to social information. Dev. Rev. 61, 100977. doi: 10.1016/j.dr.2021.100977
Madsen, J. K., Bailey, R., Carrella, E., and Koralus, P. (2019). Analytic versus computational cognitive models: agent-based modeling as a tool in cognitive sciences. Curr. Direct. Psychol. Sci. 28, 299–305. doi: 10.1177/0963721419834547
Perner, J., Ruffman, T., and Leekam, S. R. (1994). Theory of mind is contagious: you catch it from your sibs. Child Dev. 65, 1228–1238. doi: 10.2307/1131316
Peterson, C. C., and Wellman, H. M. (2019). Longitudinal theory of mind (ToM) development from preschool to adolescence with and without ToM delay. Child Dev. 90, 1917–1934. doi: 10.1111/cdev.13064
Ruffman, T., Perner, J., Naito, M., Parkin, L., and Clements, W. A. (1998). Older (but not younger) siblings facilitate false belief understanding. Dev. Psychol. 34, 161–174. doi: 10.1037/0012-1649.34.1.161
Slaughter, V., Imuta, K., Peterson, C. C., and Henry, J. D. (2015). Meta-analysis of theory of mind and peer popularity in the preschool and early school years. Child Dev. 86, 1159–1174. doi: 10.1111/cdev.12372
Taylor, M., and Carlson, S. M. (1997). The relation between individual differences in fantasy and theory of mind. Child Dev. 68, 436–455. doi: 10.2307/1131670
Wellman, H. M. (2014). Making Minds: How Theory of Mind Develops. Oxford: Oxford University Press. doi: 10.1093/acprof:oso/9780199334919.001.0001
Wellman, H. M., Cross, D., and Watson, J. (2001). Meta-analysis of theory-of-mind development: the truth about false belief. Child Dev. 72, 655–684. doi: 10.1111/1467-8624.00304
Wellman, H. M., Lane, J. D., LaBounty, J., and Olson, S. L. (2011). Observant, nonaggressive temperament predicts theory-of-mind development. Dev. Sci. 14, 319–326. doi: 10.1111/j.1467-7687.2010.00977.x
Wellman, H. M., and Liu, D. (2004). Scaling of theory-of-mind tasks. Child Development, 75, 523–541.
Wilensky, U., and Rand, W. (2015). An Introduction to Agent-Based Modeling: Modeling Natural, Social, and Engineered Complex Systems with NetLogo. London: MIT Press.
Yu, C.-L., Kovelman, I., and Wellman, H. M. (2021a). How bilingualism informs theory of mind development. Child Dev. Perspect. 15, 154–159. doi: 10.1111/cdep.12412
Yu, C.-L., Stanzione, C. M., Wellman, H. M., and Lederberg, A. R. (2021b). Theory-of-mind development in young deaf children with early hearing provisions. Psychol. Sci. 32, 109–119. doi: 10.1177/0956797620960389
Yu, C.-L., and Wellman, H. M. (2022a). All humans have a ‘theory of mind.' J. Autism Dev. Disor. 53, 2531–2534. doi: 10.1007/s10803-022-05584-1
Yu, C.-L., and Wellman, H. M. (2022b). Young children treat puppets and dolls like real persons in theory of mind research: a meta-analysis of false-belief understanding across ages and countries. Cogn. Dev. 63, 101197. doi: 10.1016/j.cogdev.2022.101197
Keywords: theory of mind, social interaction, cognitive development, social development, agent-based modeling
Citation: Yu C-L and Wellman HM (2023) Where do differences in theory of mind development come from? An agent-based model of social interaction and theory of mind. Front. Dev. Psychol. 1:1237033. doi: 10.3389/fdpys.2023.1237033
Received: 08 June 2023; Accepted: 19 October 2023;
Published: 07 November 2023.
Edited by:
Rachel L. Severson, University of Montana, United StatesReviewed by:
Antonya Gonzalez, Western Washington University, United StatesJosé F. Fontanari, University of São Paulo, Brazil
Copyright © 2023 Yu and Wellman. This is an open-access article distributed under the terms of the Creative Commons Attribution License (CC BY). The use, distribution or reproduction in other forums is permitted, provided the original author(s) and the copyright owner(s) are credited and that the original publication in this journal is cited, in accordance with accepted academic practice. No use, distribution or reproduction is permitted which does not comply with these terms.
*Correspondence: Henry M. Wellman, aG13QHVtaWNoLmVkdQ==