- 1Biochemistry and Oral Biology Laboratory, Institute for Research in Dental Sciences, School of Dentistry, Universidad Chile, Santiago, Chile
- 2Department of Oral Pathology and Medicine, School of Dentistry, Universidad Chile, Santiago, Chile
- 3Department of Child and Dentomaxilar Orthopedics, School of Dentistry, Universidad Chile, Santiago, Chile
- 4School of Dentistry, Universidad Chile, Santiago, Chile
Objective: To identify which genes are associated with the clinical phenotype of amelogenesis Imperfecta (AI) and to elucidate which of these genes participate in the determination of isolated and syndromic forms.
Methods: In this review, all data on mutations described in AI-related genes were obtained from HGMD® Professional. The data in relation to the mutations, inheritance, phenotype, type of AI and country were supplemented with information from the literature. The identity codes and frequency values were obtained from the dbSNP, ClinVar and OMIM databases. The percentage of specificity (PE) was determined for each gene.
Results: HGMD® describes 27 genes involved in AI, which we propose to group into 5 categories: (1) genes whose mutations are associated only with isolated AI, (2) genes whose mutations cause only syndromic AI, (3) genes with both mutations that cause isolated AI and mutations responsible for other pathologies, (4) genes with mutations responsible for syndromic AI and mutations that cause other pathologies, and (5) genes with mutations that cause isolated AI and mutations that cause AI associated with syndromes and other pathologies. Using the PE calculation, the genes were ranked into 5 specificity groups. The genes of category 1 are specific for isolated AI, while the genes of categories 2 and 4 are non-specific. Interestingly, we observed that mutations in some genes were associated with different types of cancer.
Conclusion: The ACP4, AMTN, MMP20, ODAPH, RELT, SLC24A4 and SP6 genes participate in causing isolated AI, and the CNNM4, DLX3 and FAM20A genes participate in causing syndromic forms of AI.
Introduction
Amelogenesis imperfecta (AI) is a group of hereditary malformations of dental development that affect the structure and chemical composition of the enamel of most teeth (1). They occur during the process of odontogenesis, specifically when tooth enamel is formed. This condition is rare, and the different kinds of AI have a combined prevalence ranging from 1/4,000 in Sweden to 1/14,000 in the United States, depending on the demographics of the population studied (1, 2).
Defects in the secretory stage of amelogenesis lead to the formation of enamel with decreased or hypoplastic thickness. If failure occurs in the maturation stage, the enamel can retain proteins or present defects in mineralization, resulting in opaque, whitish tissue with lower hardness than normal tissue, that is, in hypomineralized enamel (2, 3).
According to the classification of Witkop C. (1989), four main types of AI are distinguished: hypoplastic, hypomature, hypocalcified, and a combined hypomature/hypoplastic AI phenotype with taurodontism. When considering specific enamel traits and inheritance patterns, there can be 15 different AI subtypes (3). Currently, hypomature and hypocalcified AI are included in a single type called hypomineralized, and genetic testing is necessary to distinguish them (4).
As AI is a genetically heterogeneous pathology, its origin involves several genes and possibly environmental factors, both external and internal, that can affect the process during development and after birth. Approximately 20 genes involved in non-syndromic AI have been described, with various inheritance patterns (4). AIs that are inherited in an autosomal dominant manner are caused by mutations in amelotin (AMTN), collagen type XVII, α1 (COL17A1), distal-less 3 (DLX3), enamelin (ENAM), family with sequence similarity 83 member H (FAM83H), laminin chain α3 (LAMA3), and laminin chain β3 (LAMB3) (4, 5). The causal genes of AI with autosomal recessive inheritance are acid phosphatase 4 (ACP4), ameloblastin (AMBN), family with sequence similarity 20 member A (FAM20A), G protein-coupled receptor 68 (GPR68), integrin subunit β6 (ITGB6), kallikrein-related peptidase 4 (KLK4), enamelysin (MMP20), odontogenesis-associated phosphoprotein (ODAPH), receptor expressed in lymphoid tissues (RELT), solute carrier family 24 member A4 (SLC24A4), stromal interaction molecule 1 (STIM1), and WD repeat–containing protein 72 (WDR72) (4, 5). Finally, the amelogenin gene (AMELX), located on the X chromosome, is responsible for AI with X-linked inheritance patterns (4, 5).
In families affected by AI, the trait may present as a single phenotype, or it may be associated with other oral or extraoral traits, forming part of a syndrome (6, 7). Given that complete deciduous dentition is present in the mouth at ~2 years of age, it is clinically possible to observe hereditary defects in enamel development very early in most cases, before the appearance of systemic manifestations. At the time of the initial diagnosis, affected enamel may be the only sign present (8). This has great relevance for affected families because even when it is not possible to discriminate between isolated or syndromic AI through clinical-radiographic examination, patients with AI associated with a syndrome benefit from an early diagnosis that specifies the genetic cause of their disease, which contributes to improving its treatment and prognosis (8).
In 2015, Wright et al. reported 91 conditions listed in the OMIM database, of which 11 corresponded to non-syndromic AI caused by the genes ENAM, DLX3, FAM83H, KLK4, MMP20, WDR72, FAM20A, C4ORF26 (now ODAPH), SLC24A4, and AMELX. The other 80 conditions were pathologies or syndromes associated with enamel defects such as hypoplasia and/or hypomineralization, with 23 of them being autosomal dominant, 45 autosomal recessive, and 12 X-linked inheritance patterns (6).
To date, the literature has not discussed which genes participate strictly in isolated AI and which genes are responsible for syndromic AI, whether a certain gene can be involved in cases of both isolated and syndromic AI, or which genes can cause other pathologies related or unrelated to AI, as reported in the clinical phenotype of dental agenesis (9).
In the context described above, we set the following specific objectives: (1) to identify the genes associated with the clinical phenotype of AI in the human gene mutation database (HGMD®) (10); (2) to construct a catalog to describe and characterize the pathogenic variants associated with each gene in relation to the type of inheritance, clinical phenotype, country/ethnicity of the carriers, identification number in the single-nucleotide polymorphism database (dbSNP), and population frequency; (3) to establish what other pathologies/conditions are associated with each of these genes; (4) to determine the percentage specificity of each gene in determining the clinical phenotypes of isolated and syndromic AI; and (5) to show that some genes involved in AI can present mutations associated with some types of cancer.
Methods
Source of Information
This review was carried out using the professional database of human gene mutations (HGMD® Professional 2020.2 trial version) (10), which was consulted in October 2020, as the main source of information. The literature was obtained from this database, and the articles associated with causal mutations present in each gene involved in AI were selected and analyzed. Only the first report for each mutation was included. Subsequently, the information collected from the database and that obtained from the literature were analyzed in detail by the authors to build the catalog of AI mutations. This research was conducted in full accordance with the ethical principles of the Declaration of Helsinki and with local regulations.
Catalog of AI Genes and Causal Mutations
To fill out the catalog of AI genes and causal mutations, the HGMD® database was consulted for the “amelogenesis imperfecta” phenotype, which showed that 27 genes were associated with this clinical phenotype (10). For each of the 27 genes, data were collected on all associated diseases/phenotypes; the complete record and number of mutations classified by HGMD® as DM, FP, DP, and DFP [DM = mutation reported to be disease-causing, FP = functional polymorphism in vitro/laboratory or in vivo, DP = polymorphism associated with disease, and DFP = polymorphism associated with a disease with additional supporting functional evidence]; changes at the nucleotide level and at the protein level, according to the standard nomenclature of the Human Genome Variation Society1 (HGVS); and the bibliographic reference in which the mutation was cited for the first time. The inheritance pattern and the clinical diagnosis of the type and subtype of AI reported for each mutation, as well as the country/ethnicity of those affected, were manually corroborated by the authors through an analysis of the literature. When available, the reference SNP (rs) number from dbSNP or the identification number (VCV) in the Database of Clinical Variations, and the population frequency of the mutations were obtained from the databases: dbSNP,2 ClinVar,3 and OMIM.4
Specificity of AI Genes
To calculate the percentage specificity of a causal gene in determining the AI phenotype in isolation, the number of mutations underlying isolated AI reported for that gene (numerator) was divided by the total number of mutations of the same gene underlying other clinical conditions/diseases/phenotypes (denominator), and the result was expressed as a percentage.
Results
Genes Involved in AI According to HGMD®
The interrogation of the HGMD® database for clinical phenotype amelogenesis imperfecta revealed that the following 27 genes were associated with this clinical phenotype: ACP4, AMBN, AMELX, AMTN, CLDN16, CLDN19, CNNM4, COL17A1, DLX3, ENAM, FAM20A, FAM83H, GPR68, ITGB6, KLK4, LAMA3, LAMB3, LTBP3, MMP20, ODAPH, RELT, SLC10A7, SLC24A4, STIM1, TP63, WDR72, and SP6. The SP6 gene was included last because during the research for this study, two new articles were reported relating it to AI.
Catalog of Pathogenic Variants of AI
The catalog shown in Supplementary Table 1 was constructed with each of the above genes ordered alphabetically (except the SP6 gene) and each of the pathogenic variants reported by HGMD®. A total of 304 mutations constituted the pool of pathogenic variants associated with isolated AI (181 mutations; 22.4%) and syndromic AI (123 mutations; 15.3%) reported to date. The total number of mutations in the 27 genes, including those associated with pathologies/conditions other than AI (502 mutations, 62.3%), was 806.
In some of the 27 genes whose mutations were inherited in an autosomal recessive manner, cases with compound heterozygous mutations were found, such as in CLDN19 (1/3), ACP4 (3/10), LTBP3 (2/7), MMP20 (7/24), AMBN, CLDN1 6 and ITGB6 (1/5), FAM20A (9/46), ODAPH (1/6), CNNM4 (5/31), WDR72 (3/19), SLC10A7 (1/8), and ENAM (3/24). This type of case has not been reported for the AMELX, AMTN, DLX3, FAM83H, GPR68, KLK4, LAMB3, RELT, SLC24A4, STIM1, TP63, or SP6 genes (Supplementary Table 1). In one case, which showed a mutation in the COL17A1 gene, another mutation was found in the LAMA3 gene, leading the authors to suggest digenic inheritance. A similar situation was described in another case caused by mutations in the LAMA3 and ENAM genes simultaneously. Interestingly, to date, the ENAM gene is the only gene in which incomplete penetrance of some mutations has been identified. Another relevant gene to mention is FAM83H, in which 28 mutations (82%) were of the non-sense type, only two were missense (6%), and four were frameshift mutations (12%).
Figure 1 and Supplementary Table 2 describe the inheritance patterns of the causal mutations of isolated and syndromic AI in each gene analyzed. Mutations located in the genes ACP4, CLDN16, CLDN19, CNNM4, FAM20A, GPR68, ITGB6, KLK4, LTBP3, MMP20, ODAPH, RELT, SLC10A7, SLC24A4, STIM1, and WDR72 were inherited in an autosomal recessive manner. Those located in the genes AMTN, COL17A1, DLX3, FAM83H, LAMA3, LAMB3, TP63, and SP6 were transmitted in an autosomal dominant manner. Mutations in the ENAM and AMBN genes exhibited both types of inheritance; however, for the ENAM gene, most cases showed autosomal dominant inheritance, and for the AMBN gene, the mode of inheritance was mainly autosomal recessive. Mutations in the AMELX gene showed patterns of inheritance linked to the X chromosome, and as shown in Supplementary Table 1, most articles mentioned that this inheritance would be dominant.
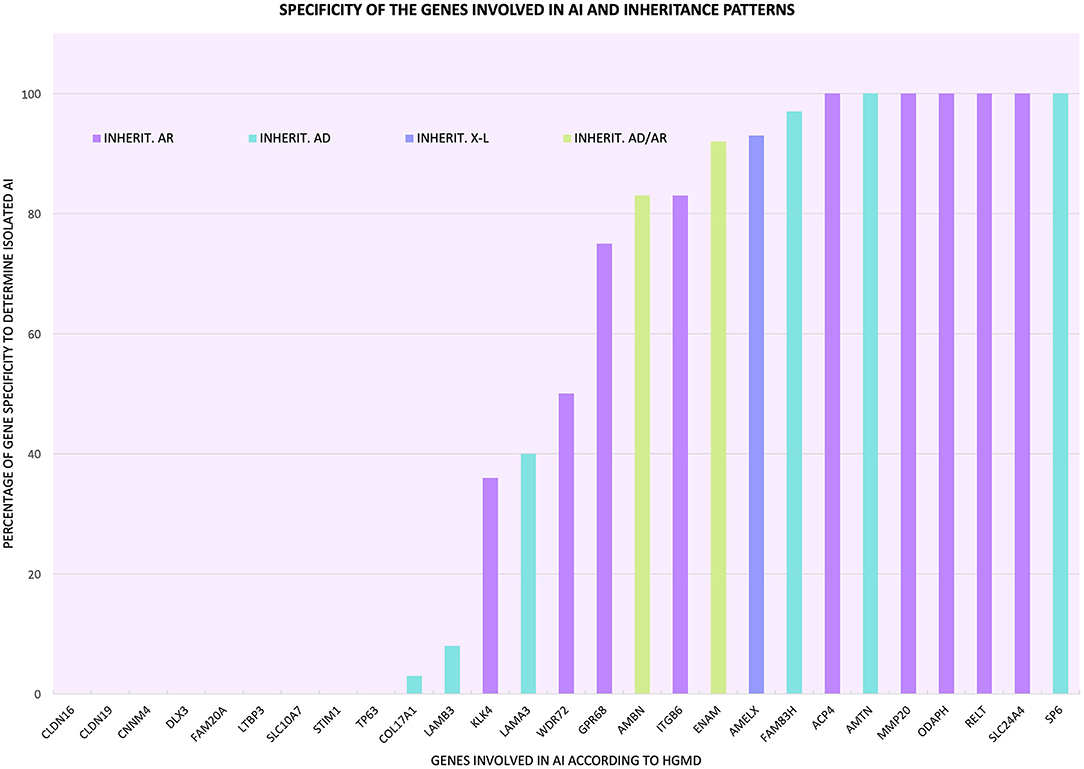
Figure 1. Percentage of specificity of the genes involved in AI to determine the phenotype in isolation form and inheritance patterns associated with the mutations of each gene ( autosomal recessive inheritance;
autosomal dominant inheritance;
X-linked inheritance;
autosomal dominant inheritance in some cases and autosomal recessive inheritance in other cases).
Supplementary Table 1 also allows us to determine which types and subtypes of AI were linked to certain syndromes and/or pathologies/conditions. For example, hypoplastic AI was part of renal enamel gingival syndrome (FAM20A), AI with brachyolmia (LTBP3) and hypomagnesemia with hypercalciuria and familial nephrocalcinosis (CLDN16). In trichodento-osseous syndrome (DLX3), we found hypoplastic AI and a combined phenotype of hypoplastic/hypomature AI. Jalili syndrome (CNNM4) presented hypoplastic AI, hypomature AI, and a combination of hypomature/hypoplastic AI (Supplementary Table 2).
The pool of isolated and syndromic AI mutations came from cases studied in approximately 47 countries. The countries where the most cases/mutations have been reported were Turkey (18.4%), the United States (9.9%), France (7.9%), Pakistan (6.3%), Korea (5.3%), China (4.9%), and the United Kingdom (4.6%) (Supplementary Tables 2, 3). The remaining countries reported between 0.3 and 3% of the cases/mutations. The distribution of mutations described in AI worldwide was quite broad, covering five continents (Supplementary Table 1, Supplementary Figure 1). Most cases were concentrated on the continents of Asia, Europe, and North America. In Central and South America, the cases contributed 2.6–3% of the mutations reported (Supplementary Figure 1). Oceania and Africa represented 0.7 and 3% of the mutations described, respectively (Supplementary Table 4).
In terms of frequency, several mutations of genes that cause isolated and syndromic AI were not yet reported in the databases, as was the case with the pathogenic variants of the AMTN, LAMA3, STIM1, TP63, and SP6 genes (Supplementary Table 1). Other genes had mutations with frequency values of 0.0000, attributable to the small size of the sampled population. In several genes, the minor allelic frequency was 0.000004; however, there were mutations in the AMBN, FAM83H, MMP20, and RELT genes that had frequency values equal to 4, 0.2, 0.16, and 0.12%, respectively, much higher than expected for a low-prevalence pathology such as AI (Supplementary Table 1).
Findings Based on the Mutational Spectrum of AI Genes
Categorization of AI Genes According to the Distribution of Causal Mutations
Table 1 and Figure 2 show the detailed analysis of the 27 genes and the distribution of their mutations causing isolated AI, AI associated with syndromes, and other pathologies/conditions (syndromic and non-syndromic). According to this distribution, the 27 genes could be categorized as follows:
1) Genes whose mutations were associated only with the isolated AI phenotype, such as ACP4, AMTN, MMP20, ODAPH, RELT, SLC24A4, and SP6 (light blue bars in Figure 2).
2) Genes whose mutations caused only syndromic AI, such as CNNM4, DLX3, and FAM20A (pink bars in Figure 2).
3) Genes that had mutations causing isolated AI and mutations responsible for pathologies/conditions other than AI, such as AMBN, AMELX, COL17A1, ENAM, FAM83H, GPR68, ITGB6, KLK4, LAMA3, and LAMB3 (light blue and purple bars in Figure 2).
4) Genes with mutations responsible for syndromic AI and mutations that caused other pathologies/conditions, such as CLDN16, CLDN19, LTBP3, SLC10A7, STIM1, and TP63 (pink and purple bars in Figure 2).
5) A fifth category formed by the WDR72 gene, which had mutations that caused isolated AI, AI associated with syndromes, and other pathologies/conditions (light-blue, pink, and purple bars in Figure 2).
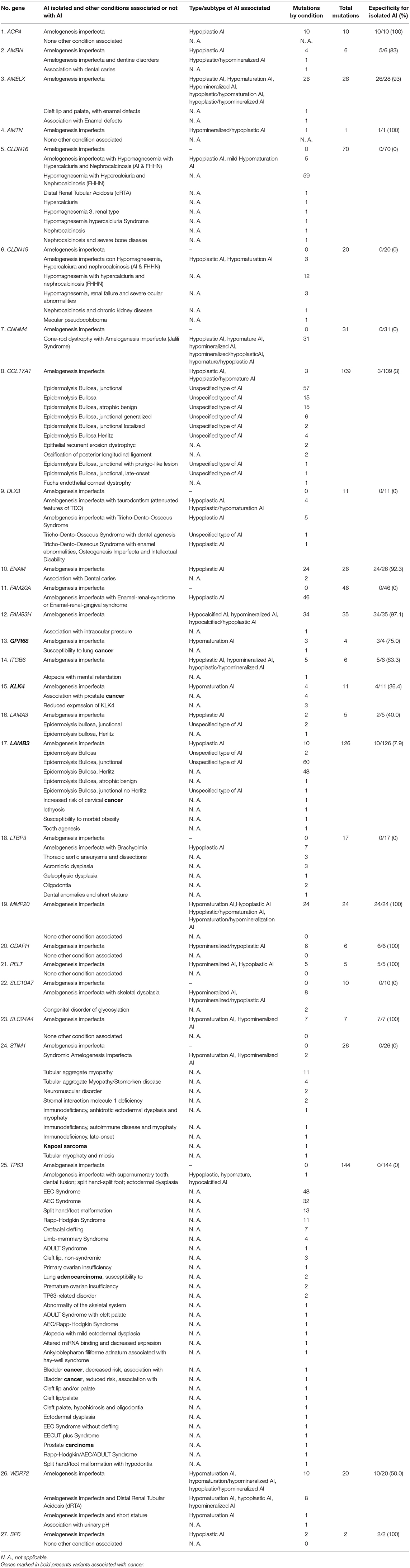
Table 1. Specificity of the causative genes of Amelogenesis imperfecta to determine AI in isolation form.
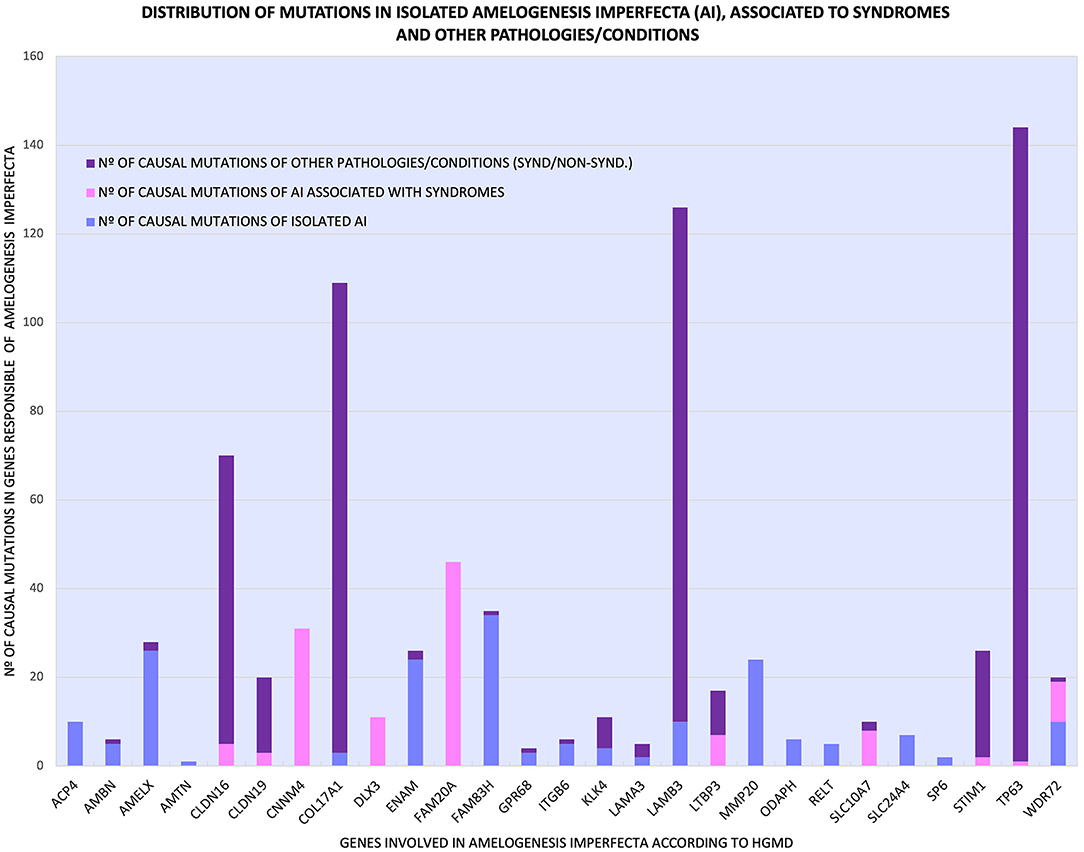
Figure 2. Distribution of the various mutations of 27 genes that cause isolated AI (), AI related to syndromes (
) and other pathologies/conditions (
), syndromic and non-syndromic.
Additionally, as shown in Figure 2 and Table 1, some genes had many mutations that caused pathologies/conditions other than AI, either syndromic or non-syndromic, such as CLDN16 (65), COL17A1 (106), LAMB3 (116), and TP63 (143), which corresponded to 13, 21, 23, and 28% of such mutations (502), respectively. Among the genes involved in isolated AI and other pathologies/conditions, FAM83H, AMELX, and ENAM were the most frequently mutated genes. On the other hand, in syndromic AI, only the FAM20A and CNNM4 genes showed a greater number of mutations (Supplementary Table 1; Figure 2).
Percentage Specificity of AI Genes
Categories 1 and 2 are all or nothing: they separate the genes that determine the clinical entity of isolated AI from those genes that participate in syndromic AI, a more complex phenotype where other tissues or organs may be affected in addition to craniofacial structures. Categories 3–5 all include genes with mutations that cause isolated and/or syndromic AI in addition to mutations that cause other pathologies/conditions, making these genes less specific in the determination of the pathology, since their mutational spectra include conditions other than AI. As shown in Table 1, Figure 1, and Supplementary Table 5, we determined the percentage specificity for each of the 27 genes to discern how many of them participate in the determination of AI pathology in isolation or non-syndromically. The ACP4, AMTN, MMP20, ODAPH, RELT, SLC24A4, and SP6 genes were in category 1, with 100% specificity. These were the only “totally specific” genes whose mutations caused isolated AI (Figure 1, Table 1; Supplementary Table 5). The genes ENAM, AMELX, and FAM83H, which belonged to category 3 and presented values of 92–97% specificity, made up the “very specific” group. The genes GPR68, AMBN, ITGB6 (category 3) and WDR72 (category 5) had specificity values of 50 to 83%, which would allow them to be considered “moderately specific” (Figure 1, Table 1; Supplementary Table 5). Likewise, the COL17A1, LAMB3, KLK4, and LAMA3 genes, included in category 3, could be considered “not very specific”, with specificity percentages of 3-40%. In contrast, the genes CNNM4, DLX3, FAM20A (category 2), CLDN16, CLDN19, LTBP3, SLC10A7, STIM1, and TP63 (category 4) did not show specificity to determine the AI phenotype in isolation (Figure 1, Table 1; Supplementary Table 5).
An alarming observation from Table 1 on some mutations in genes that are related to AI according to HGMD® is that some genes in category 3, such as GPR68, KLK4, and LAMB3, and others in category 4, such as STIM1 and TP63, had mutations that were associated with susceptibility to lung cancer, prostate cancer, cervical cancer, Kaposi's sarcoma, and lung adenocarcinoma, respectively.
Discussion
In this study, we report the results of a detailed analysis of the genes related to isolated and syndromic AI performed with HGMD®, considering all the pathogenic variants of these genes described to date. The main purpose was to elucidate which genes are specifically associated with the clinical phenotypes of isolated and syndromic AI.
Genes Involved in AI According to HGMD®
For each gene, HGMD® provides the mutations expressed in the standard nomenclature, the total number of reported mutations, and the type of mutation. Information on the number of mutations described for each disease or phenotype associated with the gene of interest and the pathogenicity and population frequency of each mutation can also be obtained from several databases, if available. Using these data for the 27 genes that, according to the data extracted from HGMD®, participate in AI, we constructed a catalog of mutations that informs about the genetic architecture of this enamel pathology.
Findings Gleaned From the Catalog of AI Mutations
When genes involved in AI are mentioned, generally no distinction is made between those that cause isolated AI and syndromic AI. It is assumed that the genes ENAM, DLX3, FAM83H, KLK4, MMP20, WDR72, FAM20A, ODAPH (C4orf26), SLC24A4, and AMELX are responsible for non-syndromic conditions that represent hereditary enamel development defects (5, 11–15). However, the findings of our study and those of new reports show that mutations in these genes also cause clinical phenotypes of AI associated with syndromes and other pathologies/conditions (8, 16, 17). In this regard, it is important to explain that, based on the percentage specificity determined, the AMELX, ENAM, and FAM83H genes did not belong to the “total specificity” group as expected. This is because AMELX has mutations associated with cleft palate/lip and with unspecified enamel defects, probably of environmental origin, that do not correspond to AI (Table 1). Some variants of the ENAM gene (and other genes that encode enamel proteins) have been associated with susceptibility to dental caries, a multifactorial oral pathology with a completely different etiopathogenesis from AI, and on the other hand, variants of the FAM83H gene may be associated with increased intraocular pressure (Table 1).
In our opinion, the problem of association with syndromes lies in the fact that many articles that reported cases of AI, especially the oldest ones, did not contain an exhaustive clinical analysis of biochemical parameters and other tests that would have allowed them to diagnose a syndrome. Some cases reported by dentists contain very good descriptions of oral phenotypes but lack medical examinations, while some cases reported by physicians include many specialized medical examinations but lack a complete oral clinical-radiographic examination. This increasingly reveals the urgent need for multidisciplinary teams to collaborate, sharing information for the benefit of the patient.
Specifically, in the 80 pathologies and syndromes described by Wright et al. (6), that present enamel defects, only in Jalili syndrome (OMIM 217080) and rhizomelic dysplasia (OMIM 610319) is the diagnosis of AI mentioned: the hypomature type, in the last example. The most mentioned conditions are hypoplasia, defects, hypocalcification, hypomaturation, hypomineralization, abnormalities, dysplasia, discoloration, and pitting of the enamel. It is worth asking how many of these conditions truly represent AI. Could AI form a clinical spectrum going from defects in the development of the enamel (DDE, hypoplasia, diffuse and demarcated opacities) to localized circumscribed hypoplastic enamel defects (LHED) such as those caused by ENAM mutations: c.1258_1259insAG and c.1020_1021ins21bp in the heterozygous state (18, 19), then molar–incisor hypomineralization (MIH) and finally to AI?
The catalog shown in Supplementary Table 1, which provides rapid access to all the genes of interest, is important for those who perform genetic counseling, as well as for clinicians in general, whether they are doctors or dentists, because the presence of AI can indicate association with syndromes when the genes in category 2 (CNNM4, DLX3, FAM20A) or category 4 (CLDN16, CLDN19, LTBP3, SLC10A7, STIM1, and TP63) are mutated. Mutations in the category 3 genes AMBN, AMELX, COL17A1, ENAM, FAM83H, GPR68, ITGB6, KLK4, LAMA3 and LAMB3, and the category 5 gene WDR72 can be suspected to be associated with other pathologies/conditions of varying severity.
Approximately half of the AI genes have compound heterozygous mutations, with CLDN19, ACP4, LTBP3, and MMP20 showing the most. These types of mutations occur when different variants at the same locus are inherited from each parent by a descendant. For this situation ocurr, the allelic frequencies of both variants (or at least one) must be “relatively high” in the population; for example, in the MMP20 gene, the mutation c.126+6T>G has a frequency of 0.002176 and is found in compound heterozygosity with the variant c.954–2A>G of unknown frequency (Supplementary Table 1). It is possible that composite heterozygosity in AI is underestimated since many of the published cases have been resolved using only Sanger sequencing. In this context, it would be interesting to re-evaluate the genotype of these cases using next-generation sequencing (NGS).
Another gene that illustrates the complexity of AI is ENAM, which can present autosomal dominant and autosomal recessive inheritance patterns (20–22) that show dose effects (19), include a mutational hot spot in its sequence (20, 23), and exhibit incomplete penetrance (24) or variable expressivity (25). Additionally, it has been found that the ENAM: c.588+1delG mutation can interact with a defective allele of another gene (LAMA3: c.1559G>A), causing digenic inheritance with a generalized hypoplastic AI phenotype and enamel pitting (7). This type of inheritance is considered a link of greater complexity in a pathology since the additive effect of the genotypes in two or more loci is required. Another case of digenic inheritance is exemplified by the finding of the genotype [COL17A1: c.1141+1G>A; LAMA3: c.6477_6486del10], which notably results in hypoplastic AI as well (26).
Another interesting finding of this work is the possible connection of some of the AI genes with cancer (STIM1, TP63, GPR68, KLK4, LAMB3). Although HGMD® had no information that would relate them to cancer through the clinical phenotype of AI, there is evidence in the current literature that the AMBN (prostate cancer) (27), CNNM4 as a PRL3 binding target (liver cancer) (28), DLX3 (cutaneous squamous-cell carcinoma) (29), FAM83H (liver cancer, osteosarcoma) (30, 31) and MMP20 (lung adenocarcinoma, oral squamous-cell carcinoma) (32, 33) could also play roles in the development and progression of cancer. In vitro or in vivo functional assays will be required to determine which mutations have the potential to predispose patients to the cellular changes necessary for the initiation and progression of cancer.
Most cases of AI shown in the catalog originate from Asian, European, and North American countries, but according to the literature consulted, molecular diagnosis has been performed mainly in the United States, United Kingdom, France, Korea, and China, revealing the large gap and inequality of resources in the countries of Central and South America, possibly as a result of being developing countries that have small budgets for research. Unfortunately, the latter will be accentuated in the future due to the restrictions applied to these budgets, which will be further reduced by the priorities imposed by the COVID-19 pandemic.
From the molecular perspective, the classification of the genes involved in AI into five categories, from totally specific to non-specific, could represent a gradient from lower to higher expression in tissues; the number, type, and location of mutations; the number of functions a gene has; the structural diversity and the ability to interact with other genes. The best example of this is WDR72, a gene with medium AI specificity whose mutations are involved in the determination of isolated AI, syndromic AI, and other pathologies/conditions at the same time (8, 34).
The strengths of this work are the construction of a catalog of mutations that informs about the genetic architecture of this enamel pathology and that allows the researcher to determine which types and subtypes of AI are associated with certain syndromes and pathologies/conditions. This study shows a panoramic view of the geographical distribution of AI in the world. In addition, the catalog provides rapid access to all the genes of interest, which is important for those who perform genetic counseling, particularly considering the possible relationship of AI genes with cancer. Finally, this investigation contributes to the determination of phenotype–genotype correlations in AI.
Among the limitations of this work are its reliance on a frequently updated database, which can make the information presented here out of date. In addition, this study would be enriched by a complementary analysis of other pathologies/conditions related to AI genes with information from other databases. In addition, considering only publications included in the HGMD® database could exclude publications in which a disease is phenotypically analyzed without determining the underlying mutation.
We think that the information obtained in this study is relevant for the determination of phenotype–genotype correlations in AI, for early consideration of the presence of mutations in certain genes as markers of association with syndromes, and for understanding how some of these genes could be related to each other and how this relationship could increase the risk of cancer development.
Conclusions
The main conclusions of this work based on our specific objectives are as follows: (1) 27 AI-related genes were identified in the human gene mutation database (HGMD®). (2) The constructed catalog showed that 7 genes (ACP4, AMTN, MMP20, ODAPH, RELT, SLC24A4, SP6) were associated with isolated AI and 3 genes (CNNM4, DLX3, FAM20A) with syndromic AI. (3) Furthermore, it was observed that AI can be associated with multiple pathologies/conditions other than AI, either syndromic or non-syndromic. (4) The PE calculation allowed us to corroborate that the 7 genes in category 1, 100% specificity, determine isolated AI, and the 3 genes in category 2, 0% specificity, participate in syndromic AI. (5) From the analysis of phenotypes caused by the different AI mutations, it was observed that the GPR68, KLK4, LAMB3, STIM1 and TP63 genes are associated with both AI and cancer.
Data Availability Statement
The original contributions presented in the study are included in the article/Supplementary Material, further inquiries can be directed to the corresponding author/s.
Author Contributions
BU and AO-P contributed to conception, design, data acquisition, analysis, interpretation, drafted, and critically revised the manuscript. DA-F, IM-B, and SR-F contributed to conception, design, data acquisition, and critically revised the manuscript. DB-M and CL-T contributed to data acquisition, analysis, and interpretation. All authors contributed to the article and approved the submitted version.
Funding
This work was funded by Comisión Nacional de Ciencia y Tecnología CONICYT, Chile (Fondecyt Project No. 1140905) and School of Dentistry, Universidad de Chile (FIOUCh Project No. 19-02).
Conflict of Interest
The authors declare that the research was conducted in the absence of any commercial or financial relationships that could be construed as a potential conflict of interest.
Publisher's Note
All claims expressed in this article are solely those of the authors and do not necessarily represent those of their affiliated organizations, or those of the publisher, the editors and the reviewers. Any product that may be evaluated in this article, or claim that may be made by its manufacturer, is not guaranteed or endorsed by the publisher.
Supplementary Material
The Supplementary Material for this article can be found online at: https://www.frontiersin.org/articles/10.3389/fdmed.2021.785382/full#supplementary-material
Supplementary Figure 1. Global distribution of AI cases. In the red circle, the countries with the highest frequency of described cases are highlighted; in the following order: Turkey (56), United States (30), France (24), Pakistan (19), Korea (16), China (15) and United Kingdom (14). The blue circles represent the rest of the countries in the world where AI cases have been found with a range of 1 to 9. (https://www.mapamundiparaimprimir.com/wp-content/uploads/2018/07/mapa-mundi-politico-con-nombres.jpg).
Supplementary Table 1. Mutational catalog of genes involved in amelogenesis imperfecta according to the human gene mutations database (HGMD®).
Supplementary Table 2. Genes that participate in isolated and syndromic AI, inheritance patterns and associated type and subtype of AI.
Supplementary Table 3. Countries with the highest number and percentage of mutations in the five continents.
Supplementary Table 4. Number and percentage of mutations by continent.
Supplementary Table 5. Summary that relates the categorization of the AI genes with their specificity. Genes marked in bold and blue color show association with cancer.
Footnotes
1. ^HGVS: https://varnomen.hgvs.org/
2. ^dbSNP: https://www.ncbi.nlm.nih.gov/snp/
3. ^ClinVar: https://www.ncbi.nlm.nih.gov/clinvar/
References
1. Aldred MJ, Savarirayan R, Crawford PJ. Amelogenesis imperfecta: a classification and catalogue for the 21st century. Oral Dis. (2003) 9:19–23. doi: 10.1034/j.1601-0825.2003.00843.x
2. Crawford PJ, Aldred M, Bloch-Zupan A. Amelogenesis imperfecta. Orphanet J Rare Dis. (2007) 2:17. doi: 10.1186/1750-1172-2-17
3. Witkop CJ Jr. Amelogenesis imperfecta, dentinogenesis imperfecta and dentin dysplasia revisited: problems in classification. J Oral Pathol. (1988) 17:547–53. doi: 10.1111/j.1600-0714.1988.tb01332.x
4. Smith CEL, Poulter JA, Antanaviciute A, Kirkham J, Brookes SJ, Inglehearn CF, et al. Amelogenesis imperfecta; genes, proteins, and pathways. Front Physiol. (2017) 8:435. doi: 10.3389/fphys.2017.00435
5. Kim YJ, Lee Y, Kasimoglu Y, Seymen F, Simmer JP, Hu JC, et al. Recessive mutations in ACP4 cause amelogenesis imperfecta. J Dent Res. (2021) 26:220345211015119. doi: 10.1177/00220345211015119
6. Wright JT, Carrion IA, Morris C. The molecular basis of hereditary enamel defects in humans. J Dent Res. (2015) 94:52–61. doi: 10.1177/0022034514556708
7. Zhang H, Hu Y, Seymen F, Koruyucu M, Kasimoglu Y, Wang SK, et al. ENAM mutations and digenic inheritance. Mol Genet Genomic Med. (2019) 7:e00928. doi: 10.1002/mgg3.928
8. Zhang H, Koruyucu M, Seymen F, Kasimoglu Y, Kim JW, Tinawi S, et al. WDR72 mutations associated with amelogenesis imperfecta and acidosis. J Dent Res. (2019) 98:541–8. doi: 10.1177/0022034518824571
9. Yu M, Wong SW, Han D, Cai T. Genetic analysis: Wnt and other pathways in nonsyndromic tooth agenesis. Oral Dis. (2019) 25:646–51. doi: 10.1111/odi.12931
10. Stenson PD, Mort M, Ball EV, Evans K, Hayden M, Heywood S, et al. The Human Gene Mutation Database: towards a comprehensive repository of inherited mutation data for medical research, genetic diagnosis and next-generation sequencing studies. Hum Genet. (2017) 136:665–77. doi: 10.1007/s00439-017-1779-6
11. Zheng Y, Lu T, Chen J, Li M, Xiong J, He F, et al. The gain-of-function FAM83H mutation caused hypocalcification amelogenesis imperfecta in a Chinese family. Clin Oral Investig. (2021) 25:2915–23. doi: 10.1007/s00784-020-03609-6
12. Liang T, Hu Y, Smith CE, Richardson AS, Zhang H, Yang J, et al. AMBN mutations causing hypoplastic amelogenesis imperfecta and Ambn knockout-NLS-lacZ knockin mice exhibiting failed amelogenesis and Ambn tissue-specificity. Mol Genet Genomic Med. (2019) 7:e929. doi: 10.1002/mgg3.929
13. Gasse B, Prasad M, Delgado S, Huckert M, Kawczynski M, Garret-Bernardin A, et al. Evolutionary analysis predicts sensitive positions of MMP20 and validates newly- and previously-identified mmp20 mutations causing amelogenesis imperfecta. Front Physiol. (2017) 8:398. doi: 10.3389/fphys.2017.00398
14. Kim YJ, Kang J, Seymen F, Koruyucu M, Gencay K, Shin TJ, et al. Analyses of MMP20 missense mutations in two families with hypomaturation amelogenesis imperfecta. Front Physiol. (2017) 8:229. doi: 10.3389/fphys.2017.00229
15. Smith CEL, Whitehouse LLE, Poulter JA, Wilkinson Hewitt L, Nadat F, Jackson BR, et al. A missense variant in specificity protein 6 (SP6) is associated with amelogenesis imperfecta. Hum Mol Genet. (2020) 29:1417–25. doi: 10.1093/hmg/ddaa041
16. Rungroj N, Nettuwakul C, Sawasdee N, Sangnual S, Deejai N, Misgar RA, et al. Distal renal tubular acidosis caused by tryptophan-aspartate repeat domain 72 (WDR72) mutations. Clin Genet. (2018) 94:409–18. doi: 10.1111/cge.13418
17. Wang Z, Fan X, Shen Y, Pagadala MS, Signer R, Cygan KJ, et al. Non-cancer-related pathogenic germline variants and expression consequences in ten-thousand cancer genomes. Genome Med. (2021) 13:147. doi: 10.1186/s13073-021-00964-1
18. Hart TC, Hart PS, Gorry MC, Michalec MD Ryu OH, Uygur C, et al. Novel ENAM mutation responsible for autosomal recessive amelogenesis imperfecta and localised enamel defects. J Med Genet. (2003) 40:900–6. doi: 10.1136/jmg.40.12.900
19. Ozdemir D, Hart PS, Firatli E, Aren G, Ryu OH, Hart TC. Phenotype of ENAM mutations is dosage dependent. J Dent Res. (2005) 84:1036–41. doi: 10.1177/154405910508401113
20. Kida M, Ariga T, Shirakawa T, Oguchi H, Sakiyama Y. Autosomal-dominant hypoplastic form of amelogenesis imperfecta caused by an enamelin gene mutation at the exon-intron boundary. J Dent Res. (2002) 81:738–42. doi: 10.1177/0810738
21. Gutierrez SJ, Chaves M, Torres DM, Briceño I. Identification of a novel mutation in the enamalin gene in a family with autosomal-dominant amelogenesis imperfecta. Arch Oral Biol. (2007) 52:503–6. doi: 10.1016/j.archoralbio.2006.09.014
22. Yu S, Zhang C, Zhu C, Quan J, Liu D, Wang X, et al. A novel ENAM mutation causes hypoplastic amelogenesis imperfecta. Oral Dis. (2021) (in press). doi: 10.1111/odi.13877
23. Kim JW, Seymen F, Lin BP, Kiziltan B, Gencay K, Simmer JP, et al. ENAM mutations in autosomal-dominant amelogenesis imperfecta. J Dent Res. (2005) 84:278–82. doi: 10.1177/154405910508400314
24. Seymen F, Lee KE, Koruyucu M, Gencay K, Bayram M, Tuna EB, et al. ENAM mutations with incomplete penetrance. J Dent Res. (2014) 93:988–92. doi: 10.1177/0022034514548222
25. Koruyucu M, Kang J, Kim YJ, Seymen F, Kasimoglu Y, Lee ZH, et al. Hypoplastic AI with highly variable expressivity caused by ENAM mutations. J Dent Res. (2018) 97:1064–9. doi: 10.1177/0022034518763152
26. Prasad MK, Geoffroy V, Vicaire S, Jost B, Dumas M, Le Gras S, et al. A targeted next-generation sequencing assay for the molecular diagnosis of genetic disorders with orodental involvement. J Med Genet. (2016) 53:98–110. doi: 10.1136/jmedgenet-2015-103302
27. Xu N, Wu YP, Yin HB, Xue XY, Gou X. Molecular network-based identification of competing endogenous RNAs and mRNA signatures that predict survival in prostate cancer. J Transl Med. (2018) 16:274. doi: 10.1186/s12967-018-1637-x
28. Kozlov G, Funato Y, Chen YS, Zhang Z, Illes K, Miki H, et al. PRL3 pseudophosphatase activity is necessary and sufficient to promote metastatic growth. J Biol Chem. (2020) 295:11682–92. doi: 10.1074/jbc.RA120.014464
29. Bajpai D, Mehdizadeh S, Uchiyama A, Inoue Y, Sawaya A, Overmiller A, et al. Loss of DLX3 tumor suppressive function promotes progression of SCC through EGFR-ERBB2 pathway. Oncogene. (2021) 40:3680–94. doi: 10.1038/s41388-021-01802-9
30. Kim KM, Park SH, Bae JS, Noh SJ, Tao GZ, Kim JR, et al. FAM83H is involved in the progression of hepatocellular carcinoma and is regulated by MYC. Sci Rep. (2017) 7:3274. doi: 10.1038/s41598-017-03639-3
31. Kim KM, Hussein UK, Park SH, Kang MA, Moon YJ, Zhang Z, et al. FAM83H is involved in stabilization of beta-catenin and progression of osteosarcomas. J Exp Clin Cancer Res. (2019) 38:267. doi: 10.1186/s13046-019-1274-0
32. Mok PL, Anandasayanam ANK, Oscar David HM, Tong J, Farhana A, Khan MSA, et al. Lung development, repair and cancer: A study on the role of MMP20 gene in adenocarcinoma. PLoS ONE. (2021) 16:e0250552. doi: 10.1371/journal.pone.0250552
33. Aseervatham J, Ogbureke KUE. Effects of DSPP and MMP20 silencing on adhesion, metastasis, angiogenesis, and epithelial-mesenchymal transition proteins in oral squamous cell carcinoma cells. Int J Mol Sci. (2020) 21:4734. doi: 10.3390/ijms21134734
Keywords: pathogenic variants, amelogenesis imperfecta, hereditary disease, dental enamel, syndromic, non-syndromic, genetic architecture
Citation: Urzúa B, Ortega-Pinto A, Adorno-Farias D, Morales-Bozo I, Rojas-Flores S, Briones-Marín D and Lepiman-Torres C (2021) Exploring the Pool of Pathogenic Variants of Amelogenesis Imperfecta: An Approach to the Understanding of Its Genetic Architecture. Front. Dent. Med. 2:785382. doi: 10.3389/fdmed.2021.785382
Received: 29 September 2021; Accepted: 03 November 2021;
Published: 26 November 2021.
Edited by:
Elif Bahar Tuna, Istanbul University, TurkeyReviewed by:
Sivakumar Nuvvula, Narayana Dental College and Hospital, IndiaAhmad Faisal Ismail, International Islamic University Malaysia, Malaysia
Copyright © 2021 Urzúa, Ortega-Pinto, Adorno-Farias, Morales-Bozo, Rojas-Flores, Briones-Marín and Lepiman-Torres. This is an open-access article distributed under the terms of the Creative Commons Attribution License (CC BY). The use, distribution or reproduction in other forums is permitted, provided the original author(s) and the copyright owner(s) are credited and that the original publication in this journal is cited, in accordance with accepted academic practice. No use, distribution or reproduction is permitted which does not comply with these terms.
*Correspondence: Blanca Urzúa, YnJ1cnp1YUBvZG9udG9sb2dpYS51Y2hpbGUuY2w=
†These authors share first authorship