- 1Bioinformatics, Istituto Nazionale Genetica Molecolare, Milan, Italy
- 2Translational Research, Istituto Nazionale Genetica Molecolare, Milan, Italy
Research and innovation are constant imperatives for the healthcare sector: medicine, biology and biotechnology support it, and more recently computational and data-driven disciplines gained relevance to handle the massive amount of data this sector is and will be generating. To be effective in translational and healthcare industrial research, big data in the life science domain need to be organized, well annotated, catalogued, correlated and integrated: the biggest the data silos at hand, the stronger the need for organization and tidiness. The degree of such organization marks the transition from data to knowledge for strategic decision making. Medicine is supported by observations and data and for certain aspects medicine is becoming a data science supported by clinicians. While medicine defines itself as personalized, quantified (precision med) or in high-definition, clinicians should be prepared to deal with a world in which Internet of People paraphrases the Internet of Things paradigm. Integrated use of electronic health records (EHRs) and quantitative data (both clinical and molecular) is a key process to develop precision medicine. Health records collection was originally designed for patient care and billing and/or insurance purposes. The digitization of health records facilitates and opens up new possibilities for science and research and they should be now collected and managed with this aim in mind. More data and the ability to efficiently handle them is a significant advantage not only for clinicians and life science researchers, but for drugs producers too. In an industrial sector spending increasing efforts on drug repurposing, attention to efficient methods to unwind the intricacies of the hugely complex reality of human physiology, such as network based methods and physical chemistry computational methods, became of paramount importance. Finally, the main pillars of industrial R&D processes for vaccines, include initial discovery, early—late pre clinics, pre-industrialization, clinical phases and finally registration—commercialization. The passage from one step to another is regulated by stringent pass/fail criteria. Bottlenecks of the R&D process are often represented by animal and human studies, which could be rationalized by surrogate in vitro assays as well as by predictive molecular and cellular signatures and models.
Introduction
Big Data in Health and Biotechnology
Big data analytics is potentially transformative. There are companies (such as Amazon, Facebook, or Uber) that thoroughly based their success on big data and their analysis; others, particularly in the telecommunication or financial sectors, that drastically changed their competitive strategies using big data. Industries and institutions of any sort can expect from the collection, creation and analysis of big data at least one of these outcomes: improving effectiveness and performances, thus increasing revenue; significantly reducing costs of processes; reducing costly risks such as lack of compliance, and production or service delivery risks. The pharmaceutical and biomedical communities are not immune to this process and are facing a data-driven transformation that need to be actively addressed. Data scientists usually describe “big data” as data having four main characteristics, famously known as the “four Vs”: volume, velocity, veracity, and variety.
Volume refers to data at scale, obtained from many sources with high number of data points. In the biomedical sciences next generation sequencing technologies are allowing a constant increase of data production: samples, tissues and patients are sequenced and re-sequenced both in bulk (extracting nucleic acids from whole tissues or cell cultures) and from single cells. Single cell sequencing also is expected to further skyrocket the amount of data produced, since, even though at a lower depth, thousands of cells are going to be analyzed for each tissue or patient (Poirion et al., 2016; Villani et al., 2017; Wang and Song, 2017; Wu et al., 2017).
Big data domains are those able to store data in the order of magnitude of Peta to Exabyte. One Exabyte equals 1 billion Gigabytes, being the Gigabyte the scale in which the current portable storage cards are measured (our smartphones work with memories of 16 Gigabytes on average). Storage volumes are actually much smaller than volumes produced by the acquisition processes, which globally sum up to the order of zettabytes (the actual footprint), due to the fact that intermediate data are often heavily pruned and selected by quality control and data reduction processes. According to the recorded historical growth rate, the growth of DNA sequencing (in number of genomes) is almost twice as fast as predicted by Moore's Law, i.e., it doubled every 7 months since the first Illumina genome sequences in 2008.
Due to these numbers genomics is comparable to other big data domains, such as astronomy, physics, and social media (particularly Twitter and YouTube). Research institutions and consortia are sequencing genomes at unprecedented rhythms, collecting genomic data for thousands of individuals, such as the Genomics England project (Genomics England, 2017) or Saudi Human Genome Program (Saudi Genome Project Team, 2015). UK's project alone will sequence 100,000 human genomes producing more than 20 petabytes of data, but many other sequencing initiatives on species other than humans will have a huge impact too. In vegetal kingdom research, pushed by agricultural applications, thousands to millions of vegetable varieties, as rice for instance, are also being sequenced (Zhu, 2012; Li et al., 2014). Moreover, given the central role that genome or exome sequencing has for personalized medicine it is reasonable to believe that a significant portion of the world's population will be sequenced, dwarfing current estimates of a five orders of magnitude factor by 2025, thus largely exceeding the growth of the other big data domain previously cited (Stephens et al., 2015).
Velocity, the second “V,” refers to the infrastructure speed in efficiently transferring big files. Extreme data volumes require extreme remedies: for data in the Exabyte scale the traditional four-wheeled method (truck delivery) is still the fastest and most secure way (https://aws.amazon.com/snowmobile/). In fact, uploading 100 petabytes over the internet would take about 30 years with nowadays hi-speed internet. Volume and velocity are not a big problem for biomedical data yet: computer power and storage dropped in costs and high volume data are easily produced and stores within the walls of the same institution. Data are not constantly transferred back and forth over the internet (transfers are usually mono directional, i.e., from service provider to researcher) and bigger volumes are usually shipped onto physical drives.
The other two “Vs” are more critical: veracity and variety. Veracity refers to data uncertainty. Biases are intrinsic to genomic sequencing data and are naturally occurring due to error rates, experimental batch effects, different statistical models applied. Variety is probably the most impacting characteristic of biomedical data. Data from this domain come in many different forms, biological data are highly heterogeneous, to this respect big data also means different signals and detection systems from the same source. Thus, heterogeneity of biological data is certainly a challenge but it is also what makes data integration needed and interesting, along with the technical possibility to use data to refine data and the consequent possibility to discover emergent properties and unpredictable results.
On the wave of the always bigger issue of reproducible research (Iqbal et al., 2016) awareness of the importance of collecting and organizing data facilitating quick storage and easy re-processing is spreading to the biological research domain. Data integration is becoming an independent and horizontal discipline and is generating a wealth of diverse projects and resources; here we are going to review some of them (Table 1).
How Data are Shaping Life Science and Health
Biomedical sciences are constantly evolving pushed by technological advances; life, health and disease are investigated in an increasingly quantitative way. Most laboratory equipment produces bigger volumes of data than it did in the past, and data points available in a common lab pile up to quantities not amenable to traditional processing such as electronic spreadsheets. Researchers have often to deal with many different data at different stages of research: experimental design, samples collection and data gathering and cleaning, analysis, quantification of experimental results and finally, interpretation. Biologists, technicians and clinical science professionals interact almost on a daily basis with analysts, statisticians, or bioinformaticians and they need to develop a correct and updated vocabulary and acquire skills up to the task of efficiently collaborate with them. Without necessarily transforming themselves into bioinformaticians, biomedical researchers have to make a cultural shift embracing a new domain of “infobiology” (Brazas et al., 2017). As a consequence, the many flavors of bioinformatics and computational biology skills are now a must-have in the technologically advanced research laboratories or R&D departments: companies and research institutions, as well as single laboratories, should also promote and organize computationally skilled personnel (Chang, 2015; Bartlett et al., 2017).
Basic and clinical research is now well aware of the value of collecting and organizing data in the form of tidy data i.e., a form that is both easy to read for humans and easy to process for computers (Wickham, 2014). Research as a whole is more and more computer assisted and fairly advanced statistical methods are regularly run through computers, such as machine learning (ML) methods to classify and discriminate samples through data. ML is the ensemble of methods giving computers the ability to learn without being explicitly programmed (Arthur, 1959) and it is considered a subfield of artificial intelligence. ML can be divided into two more subfields, very useful to biomedical research: supervised learning, which is used to make data classification and regressions, and unsupervised learning which fuels methods for clustering, reduction of dimensionality, recommendation. Such methods are nowadays regularly used in drug discovery and biomarker prioritization.
There is no research branch escaping this data-driven transformation. Data are equally percolating through—and are generated by—both traditionally “wet” experimental disciplines and domains that stemmed from mathematics and computer science. This is clearly observable in the industrial biotechnology sector, where traditionally experimental disciplines such as vaccine development, as well as more computer-driven disciplines such as network-mediated drug discovery are heavily influenced by the stream of different kind of data and the possibility to integrate them. Precision medicine, drug design/repurposing, and development of vaccines, are only three of the many domains of biomedical sciences that have quite a lot to gain from a structured, machine learning assisted and integrated use of data, either small or big.
Medicine, Drugs, and Vaccines
From Personalized to High Definition Medicine
Medicine has always been a clinical science supported by observations and data, but for certain aspects medicine is becoming a data science supported by clinicians. Clinicians should be prepared to handle data collected either horizontally from a large number of individuals, or vertically from granular, high resolution, multi-parameters analyses of a single individual (or few). In both cases all the caveats and challenges of big data hold (Brazas et al., 2017) and are similar to those encountered in the Internet of Things (IoT) domain. IoT is described as a network of electronic devices equipped with software, sensors, and connectivity used to collect data for many purposes. By 2020 40% of IoT devices will be related to medicine and health (Dimitrov, 2016 and references 1 and 2 therein) and a huge number of heterogeneous health data will be available beside the already complex datasets produced by omics technologies such as next generation sequencing. This scenario is typical of life science, where high data heterogeneity is both a challenge and an opportunity for data integration aimed at obtaining actionable results exploiting their medical, clinical, predictive values. The long term aim is to be able to measure everything, inside the human body and outside it: a recent example is the study carried out by Mike Snyder's team in Stanford, in which 250,000 measurements were taken daily from 43 individuals for a total of almost 2 billion health data points. Monitoring, elaboration and integration of those data were effectively picking up infections before they actually happened and helped distinguish participants with insulin resistance, a precursor for Type 2 diabetes (Li et al., 2017). Other projects by both academia and the private sector are designed based on the same approach but on a much larger scale, from thousands to millions of monitored volunteers (Ball et al., 2012; Maxmen, 2017).
The passage to the new century was marked by the delivery of the Human Genome (Lander et al., 2001; Venter et al., 2001): since then data took the lead in biology and medicine. Medicine gradually acquired new adjectives and characteristics, such as personalized medicine, precision medicine (Juengst et al., 2016) and now high definition medicine. The latter is the ability to assess human health in hi-definition. Since this high granularity is enabled by many new and diverse technologies (NGS applications, sensors monitoring personal physiology and parameters, quantified behavior and lifestyle, advanced imaging) is common to face today a highly heterogeneous flow of data requiring big data capabilities to be integrated (Torkamani et al., 2017).
Multiple and precise measures over time in healthy or diseased individual fits into the “quantified self” paradigm: the habit of self-monitoring during normal activities, trainings, or in the progress of a disease or therapy (Fawcett, 2015). It became more and more common to compare the self vs. the self (comparing “you to you”) in a practice of quantified self-knowledge that can transform disease prevention putting the patient at the center of the action (Mikk et al., 2017). With a similar transforming empowerment, a Harvard-MIT startup, NextGen-Jane, aims at a more efficient prevention of the vast number of women's health issues that go undetected. NextGen-Jane analyzes and digitizes menstrual blood as a rich biological matrix to draw a large number of informative data from, tackling hormone levels, fibroids conditions, the vaginal microbiome, fungi or bacterial infections potentially causing cancer (Erickson et al., 2014; Mutch, 2014; Tamaresis et al., 2014).
Electronic Health Records
Electronic health records (EHRs) are the digital format of patients' health information, either in the context of a hospitalization or inside a national or regional welfare system. Integrated use of EHRs and NGS data is a key process to develop precision medicine. In fact, direct use of NGS data in the clinical practice is unfeasible, but integration of EHRs into pipelines able to extract relevant information from analyzed NGS data would allow to hugely improve clinical outcomes. Examples of clinical data infrastructures exist and have been used in the past for different purposes such as investigation of inherited causes of common diseases (Gulcher et al., 1997) or use of existing genomic and clinical data to identify genes related to phenotype and environment (Butte and Kohane, 2006). More recently the US Precision Medicine Initiative (PMI) begun planning to integrate genomic and clinical data of a million of individuals to unveil environmental influences on disease treatments (Collins and Varmus, 2015), and other initiatives promoted by hospital networks begun the exploitation of shared assets such as electronic records and genomic data to implement a genomic based medicine (Rasmussen-Torvik et al., 2014).
EHRs are mainly designed for clinical (patient care) and billing/insurance purposes (Jensen et al., 2012), and are not usually designed with science and research in mind. For this reason, EHR-based research poses big challenges about bias and standardization. To this respect, EHRs are similar to any other large biological dataset suffering of integration weaknesses. As previously mentioned, well organized data or even data organized ab-initio for research are much easier to process with computer based method such as machine learning. With wide and standardized adoption of EHRs, millions of clinical data points from thousands of individuals become potentially available: these data are the subject of computational phenotyping (Conway et al., 2011; Hodapp, 2016; Kim et al., 2017) and the construction of organized phenome databases. The first issue to solve is therefore the adoption of an internal, logical and common infrastructure to implement standards, common annotations, interchangeable identification numbers. Precise and widely accepted standards on a smaller number of records are to be preferred over bigger datasets with incomplete or non-canonical annotations. The richness of clinical information stored in EHRs lays in its usability and interoperability, thus the quality and shape of data in the EHRs has a direct impact on research (Weiskopf and Weng, 2013).
The case of the Electronic Medical Records and Genomics (eMERGE) Network is a clear example of project spanning a long period, focusing the first efforts and deliverables on building the logic and standards to organize data and the institutions collecting and sharing them (Lemke et al., 2010; Deleger et al., 2014; Jiang et al., 2015). The primary goal of the eMERGE is to combine biorepositories with EHR systems aimed at genomic discovery and implementation of genomic in the medical practice. As a network of hospitals and research institutions, eMERGE had to ensure a correct and usable data infrastructure, then begun to integrate and analyse data (clinical, phenotypic, genomic) and subsequently delivered integrated results. Beside many disease-specific research papers published, two of the most noticeable outcomes impacting research as a whole are the Phenotype Knowledge Base (PheKB) that can be used to mine and discover phenotypes from electronic medical records (Kirby et al., 2016) and the catalog of phenome-wide association scans (PheWAS) which gathers disease/phenotype to gene associations obtained by the coupling of genetic data and EHR data (Denny et al., 2010).
Storage and manipulation of clinical data from millions of patients will become a challenge in the same way as it is happening with sequence data. Beside efficient mining and summarizing methods for better and quicker characterization and phenotyping, issues of privacy and security should also be addressed. Better reproducibility, secure data sharing between collaborating researchers or patient communities and enforced privacy of EHRs and trusted de-individualized access to EHRs, are highly desirable goals that can be met with different technologies. Considered the many issues that data sharing and privacy pose and technical approaches to address them (Raychaudhuri and Ray, 2010; Fernández-Alemán et al., 2013; Omotosho and Emuoyibofarhe, 2014), we like to point at the fact that, like any data-intensive discipline, biomedical research is now being considered a subject of choice for emerging informatics technologies such as block chain. The block chain, better known as the Bitcoin underlying technology, is based on distributed ledgers and it is a public, secure and decentralized database of ordered events or records, called blocks, that are time-stamped and linked to the previous block. The public and anonymized transactions are the foundation for both privacy and traceability, and this logic can be well adapted to the requirements for privacy, traceability and trusted sharing imposed by clinical trials (Benchoufi and Ravaud, 2017) and personal EHRs (Cunningham and Ainsworth, 2017).
Big Data for Systems Pharmacology and Drug Repurposing
If more data can serve the purpose of a better and a more effective prevention giving a whole view of the health status, more data and the ability to handle, prioritize and analyze them faster can also be a significant advantage for drugs producers, whose attention is particularly focused on drug repurposing.
How complex is to make a drug? The complexity and length of the process is directly reflected in the numbers: average time to develop a drug ranges from 10 to 15 years, and <12% of drugs entering clinical trials are approved as medicines. Costs of development, that include cost of failures, surged from 413 million dollars in the 1980s to 2.6 billion dollars in the 2000s, while industry investments in research passed from 2 billion dollars to 50 billion dollars in the same period (source FDA). These figures show how research became less effective, by a 4-fold factor, in channeling its results in drugs development pipelines, thus the imperative to look for alternative processes. Repurposing, or repositioning, drugs is the process of finding new therapeutic indications for existing drugs. A repurposed drug does not need brand new research processes and already obtained approval for preclinical phases and/or phase I clinical trials. Securing FDA approval of a repurposed drug costs only $40–$80 million in total, compared to the average of $1–$2 billion it takes to develop a new drug (Scannell et al., 2012).
Drug repurposing has been the subject of many computationally-driven efforts (March-Vila et al., 2017). Science and pharmaceutical research, are not new to computer aided research, and they are not new to hyped confidence in computational methods and failures. Computer assisted drug discovery, or CADD, was used and welcome by press with quite enthusiastic tones in the 1980s: the so called rational drug design seemed to anticipate an industrial revolution (Bartusiak, 1981) that actually did not happen. With these cultural and historical antibodies, the scientific community should be today more prepared to better handle and embed computer power in research and development processes. Today's computers are significantly improved in processing power, memory size and in the software and algorithms they run, and better analyses and methods, such as free energy perturbation analysis and quantum mechanics modeling, are available in the field of drug discovery.
Nevertheless, the primary failure of new drug candidates is due to a very simple fact: that human biology is hugely complicated. Off-targets or mechanism-based toxicities are the very common result of unpredictable interactions or processing and delivery.
Network Based Methods
Network based approaches are used to allow more comprehensive views of complex systems. Networks are a convenient way to describe molecular and biochemical interactions, either experimentally validated or predicted; drugs and diseases relationships have been also investigated with network metrics (Yildirim et al., 2007) or to predict novel targets for existing drugs (Berger and Iyengar, 2009; Wu et al., 2013).
Networks, which are the description of apparently unstructured entities that interact each other, can be considered big data, depending on the number of entities connected and on their annotated properties. The visually compelling blobs of a network offered by many graphic displays deliver high visual impact and serve the purpose to show the complexity and number of interactions, but precise metrics are needed to unwind the intricacies of a highly connected network, such as hierarchies and upstream/downstream effects (activation, inhibition). Much of these metrics have been developed in the context of graph theory, and they are generally used as a way of summarizing the complexity in a convenient way. Some metrics provide information about individual nodes (the entities in the network), others about the edges (the connections between entities). Centrality metrics for instance identify the most important nodes in a network, those that are most influential, while the node influence metrics serve to measure the influence of every node in the network (Dorogovtsev and Mendes, 2013).
In the systems pharmacology domain global drug networks obtained integrating protein-protein interactions or gene regulation data with information of many kinds of drugs have been used to define druggable targets. This is probably the most general method, able to describe the borders within which to look for candidate targets. A typical way is to look for hubs, i.e., highly connected nodes: such nodes are likely to have key roles in the regulation of multiple biological processes (Jeong et al., 2000, 2001). Ligands' chemical similarity is another property that can be used to define edges between drug targets (nodes): biochemically important properties enter into play in the network-mediated discovery process (Hert et al., 2008).
For a narrower focus, disease-gene networks are obtained adding disease information beside biological datasets and drug information, to find possible targets for specific therapies. Proteins which interact with each other are frequently involved in a common biological process (Luo et al., 2007) or are involved in a disease process (Goh et al., 2007; Ozgür et al., 2008; Goh and Choi, 2012): to this end, networks focusing on specific disease processes have been built and mined for new candidate drug target (Köhler et al., 2008; Chen et al., 2009).
Instead of focusing on specific pathological domains, another method that proved useful for drug repositioning was the introduction of new or refined network metrics, able to capture the essence of a potential drug target in a more unbiased way. Traditional metrics relies on the actual topology of described connectomes, like the shortest path between targets (Lee et al., 2012; Zhao and Li, 2012) or common targets between drugs (Daminelli et al., 2012). Such methods are potentially biased by the known interactomes already described in details in the literature, thus biased in favor of the most studied genes or proteins. For this reason, new unsupervised network metrics have been recently proposed by the Barabasi group, based on the observation that disease genes preferentially cluster in the same network neighborhood. Thus, they reasoned, the immediate vicinity of target proteins to disease modules should have been a proxy for effectiveness of the drug through the action of those targets. They introduced a proximity index that quantifies the topological relationship between drugs and disease proteins and used it to investigate relationship between drug targets and disease proteins (Sharma et al., 2015; Guney et al., 2016). Thousands of drug-disease associations either reported in the literature or unknown were grouped, for a total of more than 36 thousand associations. Both known and unknown drug-disease associations were tested with the proximity index method and it was found that drugs do not target the whole disease modules, but only a smaller subset of molecules in it. Proximity measure was also used to find similarity between drugs covering a larger number of associations than other methods, and to mine for potential repurposing candidates for rare diseases (Guney et al., 2016).
Physical Chemistry in Pharmacology
The making of a drug is complex by design. Computational methods for drug design (Computer aided drug design, or CADD) belong to two major categories based on molecular mechanics (MM) or quantum mechanics (QM). The first methods are essentially used to determine molecular structures and the potential energies of their conformation and atomic arrangement. The elementary particle under investigation in these methods is the atom, taken as a whole, without taking into account electrons, contribution. On the contrary, methods of the second class consider the electrons and the systems behavior is investigated as an ensemble of nuclei and electrons.
As a result, MM describes molecules as atoms which are bonded each other: not considering electrons motion MM requires precise and explicit information about bonds and structure. QM is able to compute systems energy as a function of electrons and atomic nuclei (not just as a function of atomic position) and incorporates physical principles such as quantum entanglement which refers to the correlated interaction of particles or group of particles providing a quantum view of the concept of chemical bond (Tapia, 2014). This quality is particularly useful in the study of interactions of drugs and active sites of enzymes (Lipkowitz, 1995; Chakraborty and Saha, 2016).
With QM methods it's possible to calculate crucial system properties which cannot be achieved by experimental procedures: vibrational frequencies, equilibrium molecular structure, dipole moments (Atkins and De Paula, 2006). These properties are useful in computer models predicting how a particular chemical compound might interact with a target of interest, for instance a drug and a pocket of an enzyme involved in a disease. This is traditionally done by modeling and molecular docking, but these methods better fit the aim of data reduction when screening millions of compounds and high reliability of the model is not strictly requested. Starting from a much lower number of candidates, i.e., a few hundreds, and needing to shortlist them to 10, extreme accuracy of the model is a must, and current techniques are not sufficient. To achieve higher accuracy both machine learning and QM methods come into help.
In a recent proof of principle study Ash and Fourches studied ERK2 kinase, which is a key player in various cancers, and 87 ERK2 ligands in search of new kinase inhibitors. They incorporated the molecular dynamics results into prediction models generated by machine learning and obtained an hyper predictive computer model using molecular dynamic descriptors able to discriminate the most potent ERK2 binders (Ash and Fourches, 2017). The availability of modern computer with high processing power, especially GPU accelerated computers, allow even longer simulations for a larger number of proteins.
The concept of “magic bullet” (Strebhardt and Ullrich, 2008), as drugs binding a single molecular disease target, is probably a minority case. Since small molecule drugs still account for the majority of the therapeutics in today's pharmaceutical market, finding new targets for them is a highly sought after opportunity, even if it's nearly impossible to find small molecules with no off-target effects. This is mainly due to the fact that the research attention is traditionally focused on the details and that investigations are usually not at the genomic scale.
Due to the complexity of protein-ligands interactions a view at the genomic scale can make the difference: an off target can turn to be a repurposable drug thanks to the complete change of genomic context. Thus, being able to maintain attention to molecular details and to the single biochemical properties while doing it at a genomic scale is a major challenge of today pharmacology.
Such a wide view is typical of systems biology approaches, and it is more commonly exploited with network and interactions databases. Paolini et al. (2006) analyzed all known drug-target interactions creating a human pharmacology interaction network connecting proteins that share one or more chemical binders. Mestres et al. (2009) integrated seven drug-target interaction databases and found that drugs interact on average with six different targets. Quantum mechanics applied to drug discovery operated in a machine learning context allows to significantly scale up the process. Even if application of this approach requires a massive computational effort and ability to handle and analyse big data, thousands of generics are now scanned for interactions with computational methods and off targets are new opportunities as potential new repurposing drugs.
The Making of a Vaccine
Vaccines represent one of the most successful public health intervention to improve the quality of life and prevent life-threatening diseases. The eradication of smallpox and the substantial reduction in the incidence of poliomyelitis, hepatitis, measles, mumps, diphtheria, tetanus and meningitis have largely demonstrated that vaccination is a very cost-effective method for preventing, managing and even eradicating a disease. It has been estimated that between 2011 and 2020 approximately 20 million deaths were avoided (Kellokumpu-Lehtinen and Halme, 1990). In the past, the traditional way of vaccine development was essentially an empirical method in which microorganisms were cultured, inactivated and injected in animals and the elicited immune response were then scrutinized with a number of immunoassays useful to identify promising vaccine target candidates. The selection of immunogenic antigens to be included in vaccines resulted after a long screening process, which was time-consuming, inherently expensive, and often paralleled by a high burden of failure. However, it is now well accepted that vaccine development process is a rather complex workflow that requires integration of information from multiple and heterogeneous areas and technologies. They include knowledge on the biology, genomics and epidemiology of the etiologic agent to be targeted by the vaccine and its infection mechanism, understanding of the cell mediated and humoral immune responses elicited during natural infection and correlated with protective immunity. Once identified, the biological role of vaccine's target molecule should be unraveled. Moreover, other important aspects that influence vaccine efficacy are related to the mode of action of typical components of vaccine formulations, like adjuvants, co-stimulatory molecules or delivery systems. Finally, considerations on reactogenicity/safety of the vaccines strongly influence the development process. In the last two decades, such high level complexity has been addressed by a growing interest in OMICs and high throughput technologies generating huge amount of data. Indeed, the recent advancements of high throughput proteomics, high resolution genomics and transcriptomics, structural biology, sophisticated bioinformatics tools combined to multi-parametric cellular immunology provide important opportunities to improve our understanding of the molecular mechanisms that underpin vaccine-mediated protection. Even more powerful are approaches integrating multiple OMICs, a process also known as systems biology, which have opened new opportunities for rationalizing vaccine target identification and for speeding up preclinical vaccines studies.
Concerning the selection of vaccine targets, accumulating evidence clearly indicate that potential candidates should fall in more than one of the following categories: (i) Secreted or membrane associated antigens. This is particularly important when antibodies are the primary mediators of vaccine-induced immunity vaccination; (ii) Abundantly expressed; for infectious diseases vaccine, such expression should be rather constant throughout the natural lifespan of the pathogen and particularly during host invasion; (iii) Conserved among epidemiologically relevant serogroups; (iv) Involved in relevant biological processes; for pathogens, toxin or virulence factors are preferred antigens. Approximately 20 years ago, genome sequencing really transformed vaccinology by allowing vaccine target selection starting directly from bacterial genome information. This strategy, termed Reverse Vaccinology (Rappuoli, 2001; Grandi and Zagursky, 2004), was pioneered by Rappuoli R. and collaborators who established a real vaccine discovery platform: the combination of genomics and bioinformatics is applied to identify the bacterial surface exposed/secreted proteins to be cloned, purified and tested in surrogate in vitro studies useful to predict a protective immune response. The approach was applied for the first time to the development of a vaccine against Neisseria meningitidis serogroup B (MenB), one of the major cause of bacterial sepsis and meningitis in children and young adults. Bexxero, a multicomponent broad coverage vaccine originated from this study, approved and commercialized in different countries. Later on, the complementation of Reverse Vaccinology with proteomics technologies approaches in different vaccine research programs, such as on Group B Streptococcus (Maione et al., 2005), Group A Streptococcus (Bensi et al., 2012), and Chlamydia C. trachomatis (Finco et al., 2011), was instrumental to further refine the selection of potential vaccine candidates and rationalize downstream expensive in vivo efficacy assays. One interesting study showed that this approach can also be used for the identification of antigens that stimulate T cell responses (Finco et al., 2011). In this studies, an initial bioinformatic selection of the membrane-associated proteins was combined with protein array screening of human sera from individuals infected by different virulent serovars to identify the immunogenic antigens. Moreover, mass spectrometry analysis was also used for the identification of antigens expressed on the bacterial surface in different pathogenic serovars, an information which would improve the likelihood of eliciting broad coverage immune responses.
More recently, systems biology approaches integrating data from multiple OMICs and advanced bioinformatics methods have been successfully employed not only for vaccine antigen identification but also to predict the specific immune responses that correlate with protective immunity. This way, the burden of data to be managed grew up enormously. One essential aspect that needs to be addressed before starting a vaccine development program consists in the understanding of the natural infection mechanisms and the evoked immune responses able to effectively eliminate the pathogen and induce long lasting protection against re-infection. Since some pathogens are capable of manipulating the immune systems, it is important to select vaccine able to overwhelm these diverting mechanisms. Moreover, of particular significance are early gene signatures elicited days or even hours after vaccination that could exploited as novel correlates of protection or reveal mechanisms that are critical in the elicitation of the appropriate immune response, and possibly even optimize the vaccination regimen. A number of studies addressed these aspects. For instance, a systematic analysis of published transcriptional profile datasets involving 77 different host-pathogen interactions allowed to identify, shared host signatures induced in different cell types in response to different pathogens, as well as specific responses (Jenner and Young, 2005). The study also described early and late transcriptional signatures associated to antigen presenting cells during viral or bacterial infection (Jenner and Young, 2005). Other emblematic studies are those on influenza vaccines. Immunobiology events and molecular profiles underlying symptomatic influenza virus infections and signatures predictive of vaccine immunogenicity have been recently reviewed (Gomez Lorenzo and Fenton, 2013). Transcriptomics data have been used to describe specific immune signatures for live attenuated vaccines and trivalent inactivated flu vaccines (Zhu et al., 2010; Bucasas et al., 2011; Nakaya et al., 2011). In addition, early gene signatures elicited upon vaccination with different flu vaccine formulations were identified (Nakaya et al., 2011). Similar approaches were also applied on Yellow fever as well as for pathogens causing major infectious diseases such as Plasmodium falciparum, human immunodeficiency virus (HIV), Mycobacterium tuberculosis (for review, Maertzdorf et al., 2015).
Approaches exploiting data from Next Generation Sequencing (NGS), quantitative mass spectrometry, novel single cell sorting technologies offer a unique opportunity to understand the complex cellular and molecular interplay underlying the elicitation of B and T cell responses. In addition, mass cytometry (CyTOF) technologies allow to integrate multi-OMICs data with phenotypic information from different immune cell subpopulations. These holistic approaches have been used to describe the complete B cell repertoire and the T cell profiles induced in response to infection or vaccination. For vaccines conferring protective immunity by elicitation pathogen-specific antibodies, which represent the large majority on all licensed vaccines, the isolation and characterization of the antibody repertoire produced by antigen-specific B cells has acquired a central importance. This process also provides an accurate overview of the antibody maturation process and can drive effective strategies aimed at priming B cell precursors expressing germline encoded antibodies before initiation of somatic mutations. Moreover, it is instrumental to generate of functional monoclonal antibodies with therapeutic properties (Galson et al., 2014) and, in general, to design new vaccines. For instance, the B cell specific repertoire pattern that is associated with serum antibody responses to vaccination has been shown for the tetanus toxoid vaccine (Lavinder et al., 2014). The unraveling of the B cell repertoire elicited by protective immunization, combined to single cell sorting of antigen-specific B cells and to recombinant antibody technologies provide a powerful platform to generate functionally active recombinant human antibodies (Rappuoli et al., 2016). Moreover, powerful approaches also include structural proteomics technologies, such as x-ray crystallography and cryoelectron microscopy. The integration of high resolution data generated by these technologies allow to identify the protective antigen/epitope conformation eliciting functional antibodies and consequently re-instruct or optimize the vaccine design process. Relevant findings from this type of approach have been used for the optimal design of HIV and Respiratory Syncytial Virus (RSV) vaccine antigens (for review, Rappuoli et al., 2016). Finally, another interesting example to scrutinize the characteristic of antibody responses elicited by vaccination consists in the combination of serum proteomics and multiple functional / biochemical assays, by which it is possible to dissect the polyclonal humoral response elicited by vaccination, as done by Chung et al. for the HIV vaccine (Chung et al., 2015).
A rational development of new vaccines also requires a thorough understanding of their mode of action. Indeed, unraveling the interaction network between innate and adaptive immunity could allow to develop vaccine able to selectively target desired immune responses with expected less reactogenicity. This is particularly relevant for most modern vaccines employing highly purified subunits of pathogens or recombinant antigens that necessitate the use of adjuvants or appropriate delivery systems to enhance and prolong the desired immune responses. Various systems biology approaches were used to understand the mechanism underlying the specific immune activation induced by vaccines approved for use in humans (Pulendran, 2014). More recently, such approaches have been used to study and compare the mode of action of different adjuvants, leading to the identification of early molecular signatures rapidly induced in the blood after vaccination that correlate and predict a protective immune response, or where associated to a better vaccine safety (for review, Olafsdottir et al., 2015). A direct comparison of different adjuvants, either approved or in clinical and preclinical development stage, led to the identification of molecular signatures, pathways and networks shared by them or otherwise exclusive (Maertzdorf et al., 2015; Olafsdottir et al., 2016). In addition, studies were also dedicated to understand cytopathic effects associated to delivery systems based on viral vectors used for decades for the administration of heterogeneous antigens, by virtue of their ability to induce effectors CD8 T cells, like attenuated vaccinia virus or poxvirus, and adenovirus (for review, Maertzdorf et al., 2015).
Beside contributing to the vaccine development process, computational approaches open the way to investigate the influence of additional parameters in the responsiveness to vaccines, such as environmental and lifestyle factors, pre-existing immune status, chronic infections, metabolism and geographic localization or other non-canonical factors (Mannick et al., 2014; Pulendran, 2014; Reese et al., 2016). For instance, pre-existing immunity, sex, or age related factors were shown to affect the response to hepatitis B and the influenza vaccine (Furman et al., 2014; Fourati et al., 2016). Microbiota is also an important factor influencing immune responses elicited by vaccination (Walker, 2016). Analysis of these parameters further amplifies the variety and size of data that should be managed, integrated and, rationally interpreted to provide new knowledge in the development process, a move steps toward to personalized vaccine strategies.
Overall, systems biology approaches integrating big data have revolutionized vaccinology research and have delivered new tools to inform and accelerate the research and development process. Nevertheless, there are still areas that need additional efforts. This is the case of vaccines for which protective immunity is not correlated to the elicitation of functional antibodies, but it is based on antigen specific CD8 and CD8 T cells, and different T lymphocyte subsets, such as malaria, tuberculosis and Chlamydia infections. One possible reason for this knowledge gap may be ascribed that, for ethical and pure feasibility reasons, most studies routinely analyze the immune responses in the blood, whereas functional T cells mainly reside in the tissues were they exert their protective functions. For this cases, a rational use of animal models of infection, when available, could help identify protective molecular and cellular signatures. In addition, a thorough exploitation of data from clinical trials represent another area of future improvement. High throughput technologies should be complemented to allow modeling of molecular signatures that could be associated with protective vaccination, thus enabling to establish robust correlate of protection, to predict vaccine outcome and to monitor safety. They could take into account multiple biomarkers, immunological read-outs, as well as lifestyle and environmental variables parameters possible influencing vaccination in specific population subsets. A correct integration and interpretation of data from genetics, transcriptomics, proteomics, single cell analysis, immunogenicity, toxicology and efficacy studies could tangibly accelerate vaccine development at reduced costs, and could re-inform the initial vaccine design process. To address this objective, an important challenge consists in harmonizing, processing and analyzing big data derived from heterogeneous technologies and data sources, so as to give useful interpretation. Indeed, right from the start, OMICs approaches were paralleled by the evolution of bioinformatics tools and databases to support vaccine selection. They include tools for sequence analysis, antigen topology and epitope prediction (He et al., 2010a). For instance, Vaxign was a first web-based vaccine design program based on the Reverse Vaccinology strategy (He et al., 2010b). Different methods and databases for storage, mining and interrogation of big data accumulated from OMICs and from literature annotation, are in continuous development to support vaccine research. Collected data include genomics, transcriptomics, proteomics, metabolomics, functional immunology, as well as information on protective antigens, DNA vaccines, and many others. Beside these research-oriented data sources, other relevant vaccine-related databases collect data from vaccine safety and reports vaccine adverse events (VAE) from many post-licensure vaccines (such as the Vaccine Adverse Event Reporting System available at www.vaers.hhs.gov), and could facilitate the association between particular adverse events and specific vaccinations. Other research databases could help overcome bottlenecks in vaccinology (for review, He, 2014).
Concluding Remarks
Biomedical data are by definition characterized by high variety and heterogeneity; the diversity of possible measurements directly depends on the many levels through which biology can be investigated. These data-producing biological levels are genomics, transcriptomics, proteomics (from the side of more traditional omics), tissues or single cells specificity, imaging and clinical quantification of a big number of parameters, often repeated over time. Connected to the biology there is the phenotypic layer which is the ensemble of physiologic readouts impacting health status, disease and individual characteristics. The personal clinical parameters are also collected and usually stored in EHRs, along with any other medical treatment information. Traditionally experimental disciplines, i.e., life science sectors that just few years ago were relying solely on wet bench work, have been flooded by data, and almost any laboratory technique has been digitized and can be quantified numerically. We are constantly producing and collecting higher volumes of diverse data, thus besides the 4Vs it is an imperative to add an additional “V”: value.
Is there actual value in embracing the big data paradigm? Data are not good data just because of their size, so big data per se are not a value. The added value is actually present and perceivable only if simple, comprehensible and possibly new and actionable information can be extracted from the big mass of data with a reasonable effort.
Information is actionable in research and in clinics if it allows to form further hypotheses or take medical decisions, respectively. In fact, ever increasing data volume and variety challenge human cognitive capacity and too much data is not usable for informed decisions. There is a significant gap between the human cognitive capacity and data availability. The decision, or educated guess, by clinical phenotype is a hallmark of traditional healthcare, but nowadays and future biomedical sciences need to rely on multiple data, analyzed and integrated by computational methods, and finally summarized into smaller annotated pieces of rich information to produce new actionable knowledge allowing augmented decision-making capacity (Figure 1).
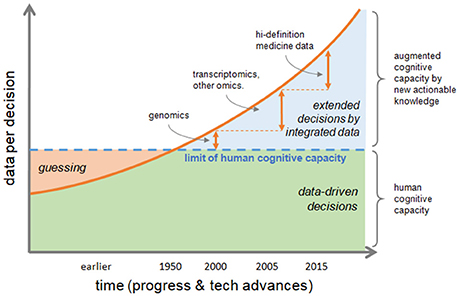
Figure 1. Transformation of decision making process. The increasing speed of data production and their volume and variety, are challenging human cognitive capacity. Educated guessing as the process of inference when information is not at hand was quite the norm in the past; today the bottleneck of human ability to process information can be bypassed if data are correctly integrated to produce new actionable knowledge, thus augmenting human cognitive capacity. Decisions by phenotype, which is typical of traditional healthcare, tends to be replaced by data-driven decisions extending the reach of medical actions either by efficacy or by speed.
In this context, data integration assumes a primary importance for biomedical sciences. We can describe at least two different scenarios for integration: horizontal and vertical integration, which are not necessarily happening in a strictly separated manner.
Horizontal integration (across many data from independent sources) applies more commonly to basic research and academia. Public databases and repositories of published datasets are a gold mine for research and can be used to look for correlations, confirm hypotheses, validate own results. Integration processes require computational skills often prerogative of computational biologists, bioinformaticians or computer scientists, and usually take a significant part of research time.
Vertical integration (across data produced by a single organization) is particularly important for pharma industries, which often produce and handle different kind of data, but have the difficulty or even impossibility to work with data outside personal or departmental silos. Difficulties are not of technical nature only, but can originate from organizational and decisional issues.
Finally, data collection needs to be curated and quality controlled and then published and shared in a way that they can be easily reused and reproduced: the publication of the FAIR principles (Bourne et al., 2015; Wilkinson et al., 2016) emphasizes four key aspects that should be a priority for data practices across the scientific community: published data should be Findable, Accessible, Interoperable and Reusable.
In the autumn of 2017 a course at the University of Washington in Seattle taught by biologist Carl Bergstrom and information scientist Jevin West quickly filled up to capacity in a few minutes as soon as the syllabus went public: the running title of the course was quite irreverently addressing the wrong way to approach information in the era of big data (Bergstrom and West, 2017). While the course was designed to teach the ability to recognize falsehood and weakness in many different domains, there were specific lectures on big data and scientific publication bias. One of the message there is that good science should beware of the so called “big data hubris,” the often implicit assumption that big data are a substitute for traditional data collection and analysis: the textbook example is the Google Flu Trends project (Lazer et al., 2014), which claimed to be able to anticipate seasonal flu outbreaks just by tracking internet user searches for flu-related terms: this actually turned out to be a predictor of outbreaks much less reliable than a simple model of local temperatures. The scientific community sometimes slips into the problem of data overfitting which is a common concern in data analysis, i.e., when too many parameters are used to match a particular set of data and following too close the training data (the data set used to infer the model), thus running the risk to infer models that are ambiguously artificial. A common clue and warning of possible overfitting, yet too often disregarded, is the occurrence of odd correlations, even if it is widely known and accepted that correlation does not imply causation (Aldrich, 1995).
In the quantifying era we live in, the dream of many analysts is to reduce every signal to a common metric which would make them much easier to integrate and compare. The reduction of physiology to quantitative signals and the ability to measure biological quantities, somehow allowed a first digitization of the human being: in this way we can ask and hope to be able to infer much more about health and disease. But as J. Derridà stated in his 1967 De la grammatologie: “il n'y a pas de hors-texte” (there is no outer text). In other words, everything we receive is interpreted and no matter the effort in peeling off all the interpretation levels, we cannot be connected to an un-interpreted reality. We need models to interpret the reality and models to fit the reality to something that allows forecasting. Weather, health, biology, behavior and financial markets are predicted with models relying on data points. The more the points the better the prediction.
In conclusion, if we want to make biomedical sciences a productive big data science and precision medicine a reality we certainly need to address challenges given by technicalities of computational methods and infrastructure scalability, but we will need to allow a real and productive data integration focusing on issues of data governance, policies of data sharing, curation and standardization.
Author Contributions
RG proposed the structure and topics to be discussed in the review. RR and RG wrote and refined the introductory and conclusion sections of the review. RR wrote and refined the sections related to personalized medicine, health records and drug repurposing. RG wrote and refined the section relating to vaccine development.
Conflict of Interest Statement
The authors declare that the research was conducted in the absence of any commercial or financial relationships that could be construed as a potential conflict of interest.
Acknowledgments
The authors want to thank E. Capobianco for critical discussion and sharing views.
References
Arthur, S. (1959). Some studies in machine learning using the game of checkers. IBM J. Res. Dev. 3, 535–554.
Ash, J., and Fourches, D. (2017). Characterizing the chemical space of ERK2 kinase inhibitors using descriptors computed from molecular dynamics trajectories. J. Chem. Inf. Model. 57, 1286–1299. doi: 10.1021/acs.jcim.7b00048
Atkins, P. W., and De Paula, J. (2006). Atkins' Physical Chemistry. Oxford: Oxford University Press.
Ball, M. P., Thakuria, J. V., Zaranek, A. W., Clegg, T., Rosenbaum, A. M., Wu, X., et al. (2012). A public resource facilitating clinical use of genomes. Proc. Natl. Acad. Sci. U.S.A. 109, 11920–11927. doi: 10.1073/pnas.1201904109
Bartlett, A., Penders, B., and Lewis, J. (2017). Bioinformatics: indispensable, yet hidden in plain sight? BMC Bioinformatics 18:311. doi: 10.1186/s12859-017-1730-9
Benchoufi, M., and Ravaud, P. (2017). Blockchain technology for improving clinical research quality. Trials 18:335. doi: 10.1186/s13063-017-2035-z
Bensi, G., Mora, M., Tuscano, G., Biagini, M., Chiarot, E., Bombaci, M., et al. (2012). Multi high-throughput approach for highly selective identification of vaccine candidates: the Group A Streptococcus case. Mol. Cell. Proteomics 11:M111.015693. doi: 10.1074/mcp.M111.015693
Berger, S. I., and Iyengar, R. (2009). Network analyses in systems pharmacology. Bioinformatics 25, 2466–2472. doi: 10.1093/bioinformatics/btp465
Bergstrom, C. T., and West, J. (2017). Calling Bullshits in the Age of Big Data. Calling Bullshits. Data Reasoning in a Digital World. Available online at: http://callingbullshit.org
Bourne, P. E., Bonazzi, V., Dunn, M., Green, E. D., Guyer, M., Komatsoulis, G., et al. (2015). The NIH big data to knowledge (BD2K) initiative. J. Am. Med. Inform. Assoc. 22:1114. doi: 10.1093/jamia/ocv136
Brazas, M. D., Blackford, S., and Attwood, T. K. (2017). Training: plug gap in essential bioinformatics skills. Nature 544:161. doi: 10.1038/544161c
Bucasas, K. L., Franco, L. M., Shaw, C. A., Bray, M. S., Wells, J. M., Niño, D., et al. (2011). Early patterns of gene expression correlate with the humoral immune response to influenza vaccination in humans. J. Infect. Dis. 203, 921–929. doi: 10.1093/infdis/jiq156
Butte, A. J., and Kohane, I. S. (2006). Creation and implications of a phenome-genome network. Nat. Biotechnol. 24, 55–62. doi: 10.1038/nbt1150
Chakraborty, S., and Saha, C. (2016). The Curtin-Hammett principle. Reson 21, 151–171. doi: 10.1007/s12045-016-0307-7
Chang, J. (2015). Core services: reward bioinformaticians. Nature 520, 151–152. doi: 10.1038/520151a
Chen, J., Aronow, B. J., and Jegga, A. G. (2009). Disease candidate gene identification and prioritization using protein interaction networks. BMC Bioinformatics 10:73. doi: 10.1186/1471-2105-10-73
Chen, R., Mias, G. I., Li-Pook-Than, J., Jiang, L., Lam, H. Y. K., Chen, R., et al. (2012). Personal omics profiling reveals dynamic molecular and medical phenotypes. Cell 148, 1293–1307. doi: 10.1016/j.cell.2012.02.009
Chung, A. W., Kumar, M. P., Arnold, K. B., Yu, W. H., Schoen, M. K., Dunphy, L. J., et al. (2015). Dissecting polyclonal vaccine-induced humoral immunity against HIV using systems serology. Cell 163, 988–998. doi: 10.1016/j.cell.2015.10.027
Collins, F. S., and Varmus, H. (2015). A new initiative on precision medicine. N. Engl. J. Med. 372, 793–795. doi: 10.1056/NEJMp1500523
Conway, M., Berg, R. L., Carrell, D., Denny, J. C., Kho, A. N., Kullo, I. J., et al. (2011). Analyzing the heterogeneity and complexity of electronic health record oriented phenotyping algorithms. AMIA Annu. Symp. Proc. 2011, 274–283.
Cunningham, J., and Ainsworth, J. (2017). Enabling patient control of personal electronic health records through distributed ledger technology. Stud. Health Technol. Inform. 245, 45–48. doi: 10.3233/978-1-61499-830-3-45
Daminelli, S., Haupt, V. J., Reimann, M., and Schroeder, M. (2012). Drug repositioning through incomplete bi-cliques in an integrated drug-target-disease network. Integr. Biol. 4, 778–788. doi: 10.1039/c2ib00154c
Deleger, L., Lingren, T., Ni, Y., Kaiser, M., Stoutenborough, L., Marsolo, K., et al. (2014). Preparing an annotated gold standard corpus to share with extramural investigators for de-identification research. J. Biomed. Inform. 50, 173–183. doi: 10.1016/j.jbi.2014.01.014
Denny, J. C., Ritchie, M. D., Basford, M. A., Pulley, J. M., Bastarache, L., Brown-Gentry, K., et al. (2010). PheWAS: demonstrating the feasibility of a phenome-wide scan to discover gene-disease associations. Bioinformatics 26, 1205–1210. doi: 10.1093/bioinformatics/btq126
Dimitrov, D. V. (2016). Medical internet of things and big data in healthcare. Healthc. Inform. Res. 22, 156–163. doi: 10.4258/hir.2016.22.3.156
Dorogovtsev, S. N., and Mendes, J. F. F. (2013). Evolution of Networks: From Biological Nets to the Internet and WWW. OUP Oxford. Available online at: https://market.android.com/details?id=book-FFL1AgAAQBAJ
Erickson, B. K., Kinde, I., Dobbin, Z. C., Wang, Y., Martin, J. Y., Alvarez, R. D., et al. (2014). Detection of somatic TP53 mutations in tampons of patients with high-grade serous ovarian cancer. Obstet. Gynecol. 124, 881–885. doi: 10.1097/AOG.0000000000000484
Fawcett, T. (2015). Mining the quantified self: personal knowledge discovery as a challenge for data science. Big Data 3, 249–266. doi: 10.1089/big.2015.0049
Fernández-Alemán, J. L., Señor, I. C., Lozoya, P. Á. O., and Toval, A. (2013). Security and privacy in electronic health records: a systematic literature review. J. Biomed. Inform. 46, 541–562. doi: 10.1016/j.jbi.2012.12.003
Finco, O., Frigimelica, E., Buricchi, F., Petracca, R., Galli, G., Faenzi, E., et al. (2011). Approach to discover T- and B-cell antigens of intracellular pathogens applied to the design of Chlamydia trachomatis vaccines. Proc. Natl. Acad. Sci. U.S.A. 108, 9969–9974. doi: 10.1073/pnas.1101756108
Fourati, S., Cristescu, R., Loboda, A., Talla, A., Filali, A., Railkar, R., et al. (2016). Pre-vaccination inflammation and B-cell signalling predict age-related hyporesponse to hepatitis B vaccination. Nat. Commun. 7:10369. doi: 10.1038/ncomms10369
Furman, D., Hejblum, B. P., Simon, N., Jojic, V., Dekker, C. L., Thiébaut, R., et al. (2014). Systems analysis of sex differences reveals an immunosuppressive role for testosterone in the response to influenza vaccination. Proc. Natl. Acad. Sci. U.S.A. 111, 869–874. doi: 10.1073/pnas.1321060111
Galson, J. D., Pollard, A. J., Trück, J., and Kelly, D. F. (2014). Studying the antibody repertoire after vaccination: practical applications. Trends Immunol. 35, 319–331. doi: 10.1016/j.it.2014.04.005
Genomics England (2017). The 100,000 Genomes Project Protocol v4. Genomics England. doi: 10.6084/m9.figshare.4530893.v4
Goh, K.-I., and Choi, I.-G. (2012). Exploring the human diseasome: the human disease network. Brief. Funct. Genomics 11, 533–542. doi: 10.1093/bfgp/els032
Goh, K.-I., Cusick, M. E., Valle, D., Childs, B., Vidal, M., and Barabási, A.-L. (2007). The human disease network. Proc. Natl. Acad. Sci. U.S.A. 104, 8685–8690. doi: 10.1073/pnas.0701361104
Gomez Lorenzo, M. M., and Fenton, M. J. (2013). Immunobiology of influenza vaccines. Chest 143, 502–510. doi: 10.1378/chest.12-1711
Grandi, G., and Zagursky, R. (2004). The impact of genomics in vaccine discovery: achievements and lessons. Expert Rev. Vaccines 3, 621–623. doi: 10.1586/14760584.3.6.621
Gulcher, J. R., Jónsson, P., Kong, A., Kristjánsson, K., Frigge, M. L., Kárason, A., et al. (1997). Mapping of a familial essential tremor gene, FET1, to chromosome 3q13. Nat. Genet. 17, 84–87. doi: 10.1038/ng0997-84
Guney, E., Menche, J., Vidal, M., and Barábasi, A.-L. (2016). Network-based in silico drug efficacy screening. Nat. Commun. 7:10331. doi: 10.1038/ncomms10331
He, Y. (2014). Ontology-supported research on vaccine efficacy, safety and integrative biological networks. Expert Rev. Vaccines 13, 825–841. doi: 10.1586/14760584.2014.923762
He, Y., Rappuoli, R., De Groot, A. S., and Chen, R. T. (2010a). Emerging vaccine informatics. J. Biomed. Biotechnol. 2010:218590. doi: 10.1155/2010/218590
He, Y., Xiang, Z., and Mobley, H. L. T. (2010b). Vaxign: the first web-based vaccine design program for reverse vaccinology and applications for vaccine development. J. Biomed. Biotechnol. 2010:297505. doi: 10.1155/2010/297505
Hert, J., Keiser, M. J., Irwin, J. J., Oprea, T. I., and Shoichet, B. K. (2008). Quantifying the relationships among drug classes. J. Chem. Inf. Model. 48, 755–765. doi: 10.1021/ci8000259
Iqbal, S. A., Wallach, J. D., Khoury, M. J., Schully, S. D., and Ioannidis, J. P. A. (2016). Reproducible research practices and transparency across the biomedical literature. PLoS Biol. 14:e1002333. doi: 10.1371/journal.pbio.1002333
Jenner, R. G., and Young, R. A. (2005). Insights into host responses against pathogens from transcriptional profiling. Nat. Rev. Microbiol. 3, 281–294. doi: 10.1038/nrmicro1126
Jensen, P. B., Jensen, L. J., and Brunak, S. (2012). Mining electronic health records: towards better research applications and clinical care. Nat. Rev. Genet. 13, 395–405. doi: 10.1038/nrg3208
Jeong, H., Mason, S. P., Barabási, A. L., and Oltvai, Z. N. (2001). Lethality and centrality in protein networks. Nature 411, 41–42. doi: 10.1038/35075138
Jeong, H., Tombor, B., Albert, R., Oltvai, Z. N., and Barabási, A. L. (2000). The large-scale organization of metabolic networks. Nature 407, 651–654. doi: 10.1038/35036627
Jiang, G., Solbrig, H. R., Kiefer, R., Rasmussen, L. V., Mo, H., Speltz, P., et al. (2015). A standards-based semantic metadata repository to support EHR-driven phenotype authoring and execution. Stud. Health Technol. Inform. 216:1098. doi: 10.3233/978-1-61499-564-7-1098
Juengst, E., McGowan, M. L., Fishman, J. R., and Settersten, R. A. Jr. (2016). From “Personalized” to “Precision” medicine: the ethical and social implications of rhetorical reform in genomic medicine. Hastings Cent. Rep. 46, 21–33. doi: 10.1002/hast.614
Kellokumpu-Lehtinen, P., and Halme, A. (1990). Results of treatment in irradiated testicular seminoma patients. Radiother. Oncol. 18, 1–7.
Kim, Y., Sun, J., Yu, H., and Jiang, X. (2017). Federated tensor factorization for computational phenotyping. KDD 2017, 887–895. doi: 10.1145/3097983.3098118
Kirby, J. C., Speltz, P., Rasmussen, L. V., Basford, M., Gottesman, O., Peissig, P. L., et al. (2016). PheKB: a catalog and workflow for creating electronic phenotype algorithms for transportability. J. Am. Med. Inform. Assoc. 23, 1046–1052. doi: 10.1093/jamia/ocv202
Köhler, S., Bauer, S., Horn, D., and Robinson, P. N. (2008). Walking the interactome for prioritization of candidate disease genes. Am. J. Hum. Genet. 82, 949–958. doi: 10.1016/j.ajhg.2008.02.013
Lander, E. S., Linton, L. M., Birren, B., Nusbaum, C., Zody, M. C., Baldwin, J., et al. (2001). Initial sequencing and analysis of the human genome. Nature 409, 860–921. doi: 10.1038/35057062
Lavinder, J. J., Wine, Y., Giesecke, C., Ippolito, G. C., Horton, A. P., Lungu, O. I., et al. (2014). Identification and characterization of the constituent human serum antibodies elicited by vaccination. Proc. Natl. Acad. Sci. U.S.A. 111, 2259–2264. doi: 10.1073/pnas.1317793111
Lazer, D., Kennedy, R., King, G., and Vespignani, A. (2014). Big data. The parable of Google Flu: traps in big data analysis. Science 343, 1203–1205. doi: 10.1126/science.1248506
Lee, H. S., Bae, T., Lee, J.-H., Kim, D. G., Oh, Y. S., Jang, Y., et al. (2012). Rational drug repositioning guided by an integrated pharmacological network of protein, disease and drug. BMC Syst. Biol. 6:80. doi: 10.1186/1752-0509-6-80
Lemke, A. A., Wu, J. T., Waudby, C., Pulley, J., Somkin, C. P., and Trinidad, S. B. (2010). Community engagement in biobanking: experiences from the eMERGE network. Genomics Soc. Policy 6, 35–52.
Li, J.-Y., Wang, J., and Zeigler, R. S. (2014). The 3,000 rice genomes project: new opportunities and challenges for future rice research. Gigascience 3:8. doi: 10.1186/2047-217X-3-8
Li, X., Dunn, J., Salins, D., Zhou, G., Zhou, W., Schüssler-Fiorenza Rose, S. M., et al. (2017). Digital health: tracking physiomes and activity using wearable biosensors reveals useful health-related information. PLoS Biol. 15:e2001402. doi: 10.1371/journal.pbio.2001402
Lipkowitz, K. (1995). Abuses of molecular mechanics: pitfalls to avoid. J. Chem. Educ. 72:1070. doi: 10.1021/ed072p1070
Luo, F., Yang, Y., Chen, C.-F., Chang, R., Zhou, J., and Scheuermann, R. H. (2007). Modular organization of protein interaction networks. Bioinformatics 23, 207–214. doi: 10.1093/bioinformatics/btl562
Maertzdorf, J., Kaufmann, S. H. E., and Weiner, J. III. (2015). Molecular signatures for vaccine development. Vaccine 33, 5256–5261. doi: 10.1016/j.vaccine.2015.03.075
Maione, D., Margarit, I., Rinaudo, C. D., Masignani, V., Mora, M., Scarselli, M., et al. (2005). Identification of a universal Group B streptococcus vaccine by multiple genome screen. Science 309, 148–150. doi: 10.1126/science.1109869
Mannick, J. B., Del Giudice, G., Lattanzi, M., Valiante, N. M., Praestgaard, J., Huang, B., et al. (2014). mTOR inhibition improves immune function in the elderly. Sci. Transl. Med. 6:268ra179. doi: 10.1126/scitranslmed.3009892
March-Vila, E., Pinzi, L., Sturm, N., Tinivella, A., Engkvist, O., Chen, H., et al. (2017). On the integration of in silico drug design methods for drug repurposing. Front. Pharmacol. 8:298. doi: 10.3389/fphar.2017.00298
Maxmen, A. (2017). Google spin-off deploys wearable electronics for huge health study. Nature 547, 13–14. doi: 10.1038/547013a
Mestres, J., Gregori-Puigjané, E., Valverde, S., and Solé, R. V. (2009). The topology of drug-target interaction networks: implicit dependence on drug properties and target families. Mol. Biosyst. 5, 1051–1057. doi: 10.1039/b905821b
Mikk, K. A., Sleeper, H. A., and Topol, E. J. (2017). The pathway to patient data ownership and better health. JAMA 318, 1433–1434. doi: 10.1001/jama.2017.12145
Mutch, D. G. (2014). Can molecular diagnostics usher in a new era for screening, diagnosis, and treatment of ovarian cancer? Obstet. Gynecol. 124, 870–872. doi: 10.1097/AOG.0000000000000534
Nakaya, H. I., Wrammert, J., Lee, E. K., Racioppi, L., Marie-Kunze, S., Haining, W. N., et al. (2011). Systems biology of vaccination for seasonal influenza in humans. Nat. Immunol. 12, 786–795. doi: 10.1038/ni.2067
Olafsdottir, T. A., Lindqvist, M., Nookaew, I., Andersen, P., Maertzdorf, J., Persson, J., et al. (2016). Comparative systems analyses reveal molecular signatures of clinically tested vaccine adjuvants. Sci. Rep. 6:39097. doi: 10.1038/srep39097
Olafsdottir, T., Lindqvist, M., and Harandi, A. M. (2015). Molecular signatures of vaccine adjuvants. Vaccine 33, 5302–5307. doi: 10.1016/j.vaccine.2015.04.099
Omotosho, A., and Emuoyibofarhe, J. (2014). A criticism of the current security, privacy and accountability issues in electronic health records. IJAIS 7, 11–18. doi: 10.5120/ijais14-451225
Ozgür, A., Vu, T., Erkan, G., and Radev, D. R. (2008). Identifying gene-disease associations using centrality on a literature mined gene-interaction network. Bioinformatics 24, i277–i285. doi: 10.1093/bioinformatics/btn182
Paolini, G. V., Shapland, R. H. B., van Hoorn, W. P., Mason, J. S., and Hopkins, A. L. (2006). Global mapping of pharmacological space. Nat. Biotechnol. 24, 805–815. doi: 10.1038/nbt1228
Poirion, O. B., Zhu, X., Ching, T., and Garmire, L. (2016). Single-cell transcriptomics bioinformatics and computational challenges. Front. Genet. 7:163. doi: 10.3389/fgene.2016.00163
Pulendran, B. (2014). Systems vaccinology: probing humanity's diverse immune systems with vaccines. Proc. Natl. Acad. Sci. U.S.A. 111, 12300–12306. doi: 10.1073/pnas.1400476111
Rappuoli, R. (2001). Reverse vaccinology, a genome-based approach to vaccine development. Vaccine 19, 2688–2691. doi: 10.1016/S0264-410X(00)00554-5
Rappuoli, R., Bottomley, M. J., D'Oro, U., Finco, O., and De Gregorio, E. (2016). Reverse vaccinology 2.0: human immunology instructs vaccine antigen design. J. Exp. Med. 213, 469–481. doi: 10.1084/jem.20151960
Rasmussen-Torvik, L. J., Stallings, S. C., Gordon, A. S., Almoguera, B., Basford, M. A., Bielinski, S. J., et al. (2014). Design and anticipated outcomes of the eMERGE-PGx project: a multicenter pilot for preemptive pharmacogenomics in electronic health record systems. Clin. Pharmacol. Ther. 96, 482–489. doi: 10.1038/clpt.2014.137
Raychaudhuri, K., and Ray, P. (2010). “Privacy challenges in the use of ehealth systems for public health management,” in Emerging Communication Technologies for E-Health and Medicine, ed J. Rodrigues (Hershey, PA: IGI Global), 155–166.
Reese, T. A., Bi, K., Kambal, A., Filali-Mouhim, A., Beura, L. K., Bürger, M. C., et al. (2016). Sequential infection with common pathogens promotes human-like immune gene expression and altered vaccine response. Cell Host Microbe 19, 713–719. doi: 10.1016/j.chom.2016.04.003
Saudi Genome Project Team (2015). The Saudi Human Genome Project: an oasis in the desert of Arab medicine is providing clues to genetic disease. IEEE Pulse 6, 22–26. doi: 10.1109/MPUL.2015.2476541
Scannell, J. W., Blanckley, A., Boldon, H., and Warrington, B. (2012). Diagnosing the decline in pharmaceutical R&D efficiency. Nat. Rev. Drug Discov. 11, 191–200. doi: 10.1038/nrd3681
Sharma, A., Menche, J., Huang, C. C., Ort, T., Zhou, X., Kitsak, M., et al. (2015). A disease module in the interactome explains disease heterogeneity, drug response and captures novel pathways and genes in asthma. Hum. Mol. Genet. 24, 3005–3020. doi: 10.1093/hmg/ddv001
Stephens, Z. D., Lee, S. Y., Faghri, F., Campbell, R. H., Zhai, C., Efron, M. J., et al. (2015). Big data: astronomical or genomical? PLoS Biol. 13:e1002195. doi: 10.1371/journal.pbio.1002195
Strebhardt, K., and Ullrich, A. (2008). Paul Ehrlich's magic bullet concept: 100 years of progress. Nat. Rev. Cancer 8, 473–480. doi: 10.1038/nrc2394
Tamaresis, J. S., Irwin, J. C., Goldfien, G. A., Rabban, J. T., Burney, R. O., Nezhat, C., et al. (2014). Molecular classification of endometriosis and disease stage using high-dimensional genomic data. Endocrinology 155, 4986–4999. doi: 10.1210/en.2014-1490
Tapia, O. (2014). Quantum photonic base states: concept and molecular modeling. Managing chemical process descriptions beyond semi-classic schemes. J. Mol. Model. 20:2110. doi: 10.1007/s00894-014-2110-2
Torkamani, A., Andersen, K. G., Steinhubl, S. R., and Topol, E. J. (2017). High-definition medicine. Cell 170, 828–843. doi: 10.1016/j.cell.2017.08.007
Venter, J. C., Adams, M. D., Myers, E. W., Li, P. W., Mural, R. J., Sutton, G. G., et al. (2001). The sequence of the human genome. Science 291, 1304–1351. doi: 10.1126/science.1058040
Villani, A.-C., Satija, R., Reynolds, G., Sarkizova, S., Shekhar, K., Fletcher, J., et al. (2017). Single-cell RNA-seq reveals new types of human blood dendritic cells, monocytes, and progenitors. Science 356:eaah4573. doi: 10.1126/science.aah4573
Walker, A. W. (2016). Studying the human microbiota. Adv. Exp. Med. Biol. 902, 5–32. doi: 10.1007/978-3-319-31248-4_2
Wang, J., and Song, Y. (2017). Single cell sequencing: a distinct new field. Clin. Transl. Med. 6:10. doi: 10.1186/s40169-017-0139-4
Weiskopf, N. G., and Weng, C. (2013). Methods and dimensions of electronic health record data quality assessment: enabling reuse for clinical research. J. Am. Med. Inform. Assoc. 20, 144–151. doi: 10.1136/amiajnl-2011-000681
Wilkinson, M. D., Dumontier, M., Aalbersberg, I. J. J., Appleton, G., Axton, M., Baak, A., et al. (2016). The FAIR Guiding Principles for scientific data management and stewardship. Sci Data 3:160018. doi: 10.1038/sdata.2016.18
Wu, H., Wang, C., and Wu, S. (2017). Single-cell sequencing for drug discovery and drug development. Curr. Top. Med. Chem. 17, 1769–1777. doi: 10.2174/1568026617666161116145358
Wu, Z., Wang, Y., and Chen, L. (2013). Network-based drug repositioning. Mol. Biosyst. 9, 1268–1281. doi: 10.1039/c3mb25382a
Yildirim, M. A., Goh, K.-I., Cusick, M. E., Barabási, A.-L., and Vidal, M. (2007). Drug-target network. Nat. Biotechnol. 25, 1119–1126. doi: 10.1038/nbt1338
Zhao, S., and Li, S. (2012). A co-module approach for elucidating drug-disease associations and revealing their molecular basis. Bioinformatics 28, 955–961. doi: 10.1093/bioinformatics/bts057
Zhu, W., Higgs, B. W., Morehouse, C., Streicher, K., Ambrose, C. S., Woo, J., et al. (2010). A whole genome transcriptional analysis of the early immune response induced by live attenuated and inactivated influenza vaccines in young children. Vaccine 28, 2865–2876. doi: 10.1016/j.vaccine.2010.01.060
Keywords: big data, healthcare, data integration, electronic health records, drug repurposing, vaccines, omics, systems biology
Citation: Rossi RL and Grifantini RM (2018) Big Data: Challenge and Opportunity for Translational and Industrial Research in Healthcare. Front. Digit. Humanit. 5:13. doi: 10.3389/fdigh.2018.00013
Received: 31 January 2018; Accepted: 15 May 2018;
Published: 31 May 2018.
Edited by:
Liliana Minelli, University of Perugia, ItalyReviewed by:
Carson Leung, University of Manitoba, CanadaPierpaolo Cavallo, Università degli Studi di Salerno, Italy
Copyright © 2018 Rossi and Grifantini. This is an open-access article distributed under the terms of the Creative Commons Attribution License (CC BY). The use, distribution or reproduction in other forums is permitted, provided the original author(s) and the copyright owner are credited and that the original publication in this journal is cited, in accordance with accepted academic practice. No use, distribution or reproduction is permitted which does not comply with these terms.
*Correspondence: Riccardo L. Rossi, cm9zc2lAaW5nbS5vcmc=
Renata M. Grifantini, Z3JpZmFudGluaUBpbmdtLm9yZw==