- 1Center for Technology and Behavioral Health, Geisel School of Medicine, Dartmouth College, Lebanon, NH, United States
- 2The Dartmouth Institute for Health Policy and Clinical Practice, Geisel School of Medicine, Dartmouth College, Hanover, NH, United States
- 3Department of Psychology, Arizona State University, Tempe, AZ, United States
- 4Department of Health Behavior, University of North Carolina at Chapel Hill, Chapel Hill, NC, United States
- 5Department of Psychology, University of Florida, Gainesville, FL, United States
- 6Stanford Prevention Research Center, Stanford University, Stanford, CA, United States
- 7Department of Health Education and Behavior, University of Florida, Gainesville, FL, United States
- 8Department of Psychological and Brain Sciences, Drexel University, Philadelphia, PA, United States
- 9Department of Public Health and Primary Care, University of Cambridge, Cambridge, United Kingdom
- 10National Institute on Aging, National Institutes of Health, Bethesda, MD, United States
- 11Apple Inc., Cupertino, CA, United States
Introduction: Smoking, obesity, and insufficient physical activity are modifiable health risk behaviors. Self-regulation is one fundamental behavior change mechanism often incorporated within digital therapeutics as it varies momentarily across time and contexts and may play a causal role in improving these health behaviors. However, the role of momentary self-regulation in achieving behavior change has been infrequently examined. Using a novel momentary self-regulation scale, this study examined how targeting self-regulation through a digital therapeutic impacts adherence to the therapeutic and two different health risk behavioral outcomes.
Methods: This prospective interventional study included momentary data for 28 days from 50 participants with obesity and binge eating disorder and 50 participants who smoked regularly. An evidence-based digital therapeutic, called Laddr™, provided self-regulation behavior change tools. Participants reported on their momentary self-regulation via ecological momentary assessments and health risk behaviors were measured as steps taken from a physical activity tracker and breathalyzed carbon monoxide. Medical regimen adherence was assessed as daily Laddr usage. Bayesian dynamic mediation models were used to examine moment-to-moment mediation effects between momentary self-regulation subscales, medical regimen adherence, and behavioral outcomes.
Results: In the binge eating disorder sample, the perseverance [β1 = 0.17, 95% CI = (0.06, 0.45)] and emotion regulation [β1 = 0.12, 95% CI = (0.03, 0.27)] targets of momentary self-regulation positively predicted Laddr adherence on the following day, and higher Laddr adherence was subsequently a positive predictor of steps taken the same day for both perseverance [β2 = 0.335, 95% CI = (0.030, 0.717)] and emotion regulation [β2 = 0.389, 95% CI = (0.080, 0.738)]. In the smoking sample, the perseverance target of momentary self-regulation positively predicted Laddr adherence on the following day [β = 0.91, 95% CI = (0.60, 1.24)]. However, higher Laddr adherence was not a predictor of CO values on the same day [β2 = −0.09, 95% CI = (−0.24, 0.09)].
Conclusions: This study provides evidence that a digital therapeutic targeting self-regulation can modify the relationships between momentary self-regulation, medical regimen adherence, and behavioral health outcomes. Together, this work demonstrated the ability to digitally assess the transdiagnostic mediating effect of momentary self-regulation on medical regimen adherence and pro-health behavioral outcomes.
Clinical Trial Registration: ClinicalTrials.gov, identifier (NCT03774433).
Introduction
Health risk behaviors, such as smoking, obesity, and insufficient physical activity lead to the development of chronic diseases and early mortality (1, 2). As much as 40% of premature deaths in the United States and 60% of all deaths in Europe are attributed to health risk behaviors (3, 4). In low- and middle-income countries, deaths from noncommunicable, health behavior-related diseases are projected to rise substantially from 30 million to 41.8 million by the year 2030 (5). Predictive models suggest that by 2030, half of the adults in the United States will experience obesity with a prevalence above 50% in 29 states (6). Similar models predicted that 110,000 premature deaths could be prevented each year in the United States if adults engaged in ten more minutes of daily moderate-to-vigorous physical activity (7). While smoking prevalence has decreased as much as 38% from 1990 to 2020 worldwide, a total of 50.9 million adults in the United States still reported current use of tobacco in 2022 with 80.5% of those individuals reporting smoking tobacco products (8, 9).
While numerous pharmacotherapies and behavioral interventions seek to target health risk behaviors, non-adherence to prescribed medical regimens is ubiquitous and associated with worse health outcomes, higher disease prevalence, and increased healthcare costs across multiple chronic diseases (10, 11). This lack of adherence contributes to approximately 125,000 avoidable deaths and as much as 69% of hospitalizations every year in the United States (12). A meta-analysis of over 500 studies found an average rate of non-adherence to prescribed medical treatment of 24.8%, which equates to over 188 million medical visits where patients do not follow prescribed medical advice (13). Specifically, as much as 30% of prescription medications are never filled by patients, and over 50% of patients discontinue their prescription medications without consultation with their physician (14–16). Medication nonadherence can result from patient-related barriers such as lack of motivation, fear of side effects, cultural factors, or treatment-related barriers such as difficulty accessing pharmacies, high costs of brand name medications, poor patient-provider communication, or too little time to fill prescriptions (17). Targeting health risk behaviors can have an immense impact on improving chronic disease outcomes and reducing excessive healthcare utilization in people non-adherent to medical regimens (18–21).
One fundamental behavior change mechanism across different types of health behavior is self-regulation. Self-regulation is defined as a person's ability to control motivations, cognition, and emotions to avoid immediate gratification and achieve their long-term goals (22). Multiple studies have identified the role of self-regulation as a potential causal mechanism to promote better health behavior and have identified deficient regulation as a causal mechanism in health risk behaviors, including cigarette smoking, insufficient physical activity, and binge eating (23–31). Until recently, self-regulation has typically been studied as a static trait-level characteristic, but it is increasingly considered a dynamic process with intraindividual variability across time and contexts (32, 33). This malleable process suggests that both internal contexts, such as withdrawal or craving, and external contexts, such as environmental factors, may impact an individual's self-regulation in the moment.
Recent advancements in digital technology have created new opportunities to assess and modify self-regulation in large populations and in real-time. Specifically, the growing field of digital health uses tools such as smartphone applications and wearable devices to deliver targeted health interventions (34). Digital health interventions, typically referred to as digital therapeutics, can enable people to better monitor their health status, change behaviors, understand competing health priorities, make informed treatment decisions, manage medications, and improve patient-provider communication (35). These outcomes are achieved through complex interventional components, such as health monitoring tools, psychoeducation, decision aids, and evidence-based cognitive behavioral therapies and/or mindfulness interventions traditionally delivered only through in-person consultation (35).
Digital therapeutics offer the promise to be wide reaching, given that 85% of households in the United States have access to the internet, 84% own a smartphone, and 92% own a computer (36). Importantly, rural communities across the United States with less access to traditional healthcare still often have high rates of internet and computer access (37). Further, the majority of people around the world own a mobile phone with a prevalence as high as 83% in countries with emerging economies (38). Given this prevalence, digital health interventions have been tested in high-, low- and middle-income countries and have been found to improve adherence to medical regimens, appointment attendance, and gathering of health data to enhance clinical outcomes for a wide array of populations (39–43). Digital health interventions have been shown to lead to outcomes comparable to or better than in-person clinician-delivered interventions and “just in time” support during everyday activities or when clinicians are not available (44–49). The worldwide access to digital technologies provides the opportunity to broadly assess and promote self-regulation at a population level.
While numerous interventions have utilized digital health technology to increase medical regimen adherence and pro-health behavior, most of this work has been siloed, focusing on only one disease or disorder at a time (50). Additionally, the proposed mechanisms underlying these behavior change interventions are infrequently systematically examined. In response, the National Institutes of Health (NIH) Science of Behavior Change (SOBC) initiative was developed to overcome these silos and systematically examine the mechanisms of behavior change interventions using an “experimental medicine” approach (51). Particularly, the program sought to (1) use basic science to investigate mechanisms of behavior change across different health behaviors, (2) go beyond clinical endpoints to find common predictable targets of health behaviors, and (3) incorporate these mechanistically informed targets from basic science to modify clinical endpoints through replicable and scalable behavioral health interventions (51). Within the framework of the SOBC initiative, understanding the role of self-regulation as a key target across different health behaviors can help develop “precision medicine” approaches for more efficient, cost-effective, and patient-centered care across numerous populations. In response, our interdisciplinary team has been using the SOBC framework to study the role of self-regulation as a putative behavior change target across different health risk behaviors with the goal of developing efficacious and scalable digital health interventions.
To better understand these dynamic self-regulatory processes, we previously developed and validated a novel momentary self-regulation scale in a large sample of over 500 nationally recruited adults (52). Given the advantages of digital health platforms, this scale was administered via ecological momentary assessments (EMA) on mobile devices to assess momentary self-regulation in a nonlaboratory, naturalistic setting. As detailed in our previous publication, the scale comprises four factors of self-regulation, including momentary perseverance, sensation seeking, emotion regulation, and mindfulness (52). Initial testing of the selected items showed strong construct and predictive validity for health risk behaviors and suggests this novel momentary self-regulation scale can be successfully administered via mobile devices as ecological momentary assessments (EMAs) (52).
In another study, a novel digital health application called Laddr™ distributed this novel scale through EMA (33). Laddr is an evidence-based behavior change digital therapeutic delivered through an interactive, self-directed smartphone application and has been studied across numerous health risk behaviors, including smoking, binge eating, illicit drug use, alcohol use, and mental health concerns such as depression, anxiety, and panic disorder (39, 40, 53–55). Laddr includes self-regulation monitoring and behavior change tools to help people solve problems, conceptualize obstacles, and develop skills to overcome their health risk behaviors and maintain motivation to behavior change. The EMA data from this interventional platform were used to examine if putative targets of momentary self-regulation changed in different real-world environmental contexts and if the Laddr digital therapeutic modified the association between momentary self-regulation and these contexts (33). This prior work was conducted with 104 people who smoke regularly and 81 people with obesity/overweight and binge eating disorder (BED). Smoking and BED are two exemplar medical concerns in which the health behaviors may contribute to decreased medical regimen adherence (1). Results from this prior study suggest it is possible to trans-diagnostically measure momentary self-regulation and study the effect of a momentary mobile intervention on self-regulation in a non-laboratory setting (33). Specifically, this study suggests that momentary self-regulation may have intraindividual variability depending on real-world internal (e.g., negative affect) and external contexts (e.g., smelling smoke) applied to these different health risk behaviors (33). Smoking and overeating may decrease momentary self-regulation while the Laddr digital therapeutic may increase momentary self-regulation thus underscoring the malleability of self-regulation (33). This work was important in informing our next step, reported in the present manuscript, of studying the mediating effect of momentary self-regulation on health behavior, defined as adherence to medical regimens, and health outcomes related to smoking and obesity.
The primary objective of the current study was to examine how targeting self-regulation through the Laddr digital therapeutic engages putative behavior change targets in two defined subgroups of interest: (1) people who smoke regularly and (2) people with obesity/overweight status meeting criteria for BED. This mobile digital health intervention integrates both novel momentary self-regulation assessments and behavior change tools on a modifiable platform that allows the content to be quickly adapted as needed to better impact targets. Specifically, this study examined the degree to which Laddr engages putative self-regulatory targets of behavior change in people with obesity/overweight and BED and people who smoke regularly to impact adherence to the Laddr intervention as well as health behaviors of smoking and physical activity among the participant samples, respectively. We hypothesized that across both people with obesity/overweight and BED and people who smoke regularly (1) self-regulation will predict adherence to treatment using the Laddr intervention on the following day, (2) treatment adherence will then predict the objective behavioral health outcome through either the number of steps taken or the carbon monoxide sample value, and (3) treatment adherence and the behavioral outcome will both predict self-regulation at the next measurement. A key goal of this line of research is to demonstrate mechanisms of behavior change that are necessary to target to then help people achieve meaningful clinical endpoints.
Materials and methods
Study design
The present study was a 28-day prospective interventional study designed to target self-regulation to impact health behaviors and health outcomes. This study protocol was approved by the Dartmouth College Institutional Review Board, monitored by a Dartmouth College Data and Safety Monitoring Board, and the study protocol was preregistered on ClinicalTrials.gov (Identifier: NCT03774433).
Participants
Target population
This study recruited two distinct samples of participants: (1) people with obesity/overweight that met the screening criteria for BED and (2) people who smoke regularly. These are two exemplar groups of people where engaging in health risk behaviors can contribute to negative health outcomes (1, 2).
Target sample size
The total target sample size was 154 participants (77 people with obesity/overweight and BED and 77 people who smoke regularly) recruited nationally from the United States. The target of 77 participants from each group was determined to reach a final sample size of at least 50 participants in each group with adequate EMA participation of at least 10% of responses over the duration of the 28-day study (≥12 out of 112 total EMAs). These values were derived from power calculations of the percentage of participants who completed at least 10% of their EMAs in our earlier study examining how different contexts may impact momentary self-regulation (33). The maximum number of observations in the momentary dataset would be 8,624 with 77 participants in each group and 4 EMA responses per day for 28 days. The momentary dataset would be closer to 5,821 with a 10% study drop-out and 75% EMA completion rate. With this sample size, there would be adequate power to detect momentary relationships between contextual factors and momentary self-regulation.
Overall sample eligibility
All participants had to reside in the United States, be 18–50 years old, provide informed consent in English, have access to a computer in a comfortable setting conducive to providing sensitive information, and use a smartphone with an operating system compatible with Laddr. Exclusion criteria were: enrolled in our earlier study examining putative targets of momentary self-regulation and associations with Laddr, current diagnosis of a substance use disorder other than nicotine use disorder, use of prescription pain medications, use of medications for smoking (not including nicotine replacement therapy), use of medications for weight loss, pregnancy or plans to become pregnant in the next 3 months, lifetime history of a mental disorder specifically determined to be due to a medical condition or classified as a psychotic disorder (e.g., schizophrenia, schizoaffective disorder, or bipolar disorder, but not including depression), medical history of weight-loss surgery, and nighttime shift work or obstructive sleep apnea. These variables were all potential confounders that may be related to momentary self-regulation, Laddr adherence, or the outcomes of interest. The following study inclusion criteria intended to have participants in the two groups be non-overlapping samples so that we could examine the hypothesized relations across these two distinct health risk behaviors.
Binge eating sample eligibility
In addition to the overall study eligibility criteria, the BED sample had to have a BMI ≥ 27 kg/m2 and ≤45 kg/m2, screen positive for binge eating behavior based on the QEWP-5, currently non-smoking (defined as no cigarettes in past 12 months), confirmed interest in an eating intervention, and use a smartphone compatible with the Fitbit application (Fitbit Flex 2™). Additional exclusion criteria were: compensatory behavior (e.g., purging, excessive exercise, fasting) at a frequency of once or more per week (less than once per week, on average, was not considered exclusionary), weight loss >10 pounds in the past six months, currently in a weight-loss program (excluding online/mobile app weight-loss programs), current use of a special diet for a serious health condition, current therapy with a clinician for binge eating, and known nickel allergy because the Fitbit band contained nickel.
Smoking sample eligibility
In addition to the overall study eligibility criteria, the smoking sample had to smoke five or more cigarettes/day for the past year, have a BMI ≥ 17 kg/m2 and <27 kg/m2, confirmed interest in a smoking cessation intervention, and use a smartphone compatible with the iCO Smokerlyzer device (iCO™ Smokerlyzer®, Bedfont® Scientific Ltd.). Additional exclusion criteria were: screening positive for binge eating behavior according to the Questionnaire on Eating and Weight Patterns-5 (QEWP-5), and current therapy with a clinician for smoking and/or BED. Participants in the smoking sample were allowed to use nicotine replacement medications, but could not use any other prescription medications that may promote smoking cessation (e.g., bupropion, varenicline).
Recruitment
Participants were recruited online through social media platforms (Craigslist, Facebook, Instagram, and Google AdWords), which proved successful in the earlier study examining putative targets of momentary self-regulation and associations with Laddr (33). After engaging with an online study ad, participants were directed to an online eligibility screening questionnaire on the Dartmouth Research Electronic Data Capture (REDCap) platform (56, 57). Participants who met eligibility criteria on the online screening questionnaire were then verified via phone by study staff. Participants who provided informed consent were scheduled for the study and mailed study supplies with no in-person visit necessary. To obtain a diverse cohort representative of behavioral targets of interest, we developed and utilized demographically targeted ads and enrolled participants based on gender, race, and ethnicity. Recruitment and data collection occurred from February 27, 2019, through June 29, 2020.
Laddr intervention
The Laddr digital therapeutic is a transdisciplinary evidence-based behavior change intervention delivered via an interactive, self-directed mobile platform (58). Laddr is unique in that it uses science-based therapeutic tools for behavior change across a wide range of disorders and behaviors based on their specific goals and needs. The validated tools within Laddr have been informed by over 20 NIH-supported randomized trials and includes behavior change tools for multiple health risk behaviors, including smoking, binge eating, substance use, alcohol use, and mental health problems such as depression, anxiety, and panic disorder (39, 40, 53–55). This platform has various guides that provide specific self-regulation monitoring and behavior change tools to solve problems, overcome obstacles, and develop skills for maintenance of these learned behaviors. Information about binge eating and smoking are embedded within the guides to help users create informed personalized goals regarding their health risk behaviors. For example, the smoking guides included information on first recognizing and learning about smoking cues. Then, participants completed problem solving activities to help employ healthier solutions. Suggested healthier solutions included performing breathing exercises or going outside for a walk to remove themselves from the cues. Additionally, Laddr allows users to enter their health data into the platform, such as inputting daily Fitbit activity (BED sample) and daily carbon dioxide (CO) readings (smoking sample) to track progress over time.
Measures
Surveys
All participants were asked to complete a battery of baseline surveys at the beginning of their 28-day study period. These surveys were expected to take approximately 1.5–2 h at each time point. The baseline survey measured demographics and socioeconomic status (e.g., age, sex, ethnicity, race, education), physical health characteristics (e.g., height and weight for BMI calculation), and smoking and binge eating-related information (e.g., cigarettes smoked per day or ever in a weight loss program). Participants also completed a battery of 17 questionnaires on self-regulation, described in detail in our earlier study examining putative targets of momentary self-regulation (33). Additionally, participants in the BED sample completed questions adapted from the QEWP-5 regarding past month eating habits to screen for BED behaviors at baseline (59). In the smoking sample, participants completed the Fagerström Test for Nicotine Dependence (60). Participants were asked to abstain from smoking (smoking sample only) and eating (BED only) for three hours prior to the start of the baseline assessments. Regardless, participants were asked questions assessing their last consumption of nicotine (smoking sample only) or food (BED sample only) prior to starting the baseline survey.
Putative targets
During the study period, participants completed items related to self-regulation and context. The items included the momentary self-regulation questionnaire used in our earlier study examining putative targets of momentary self-regulation, which was delivered through mobile EMA (33, 52). This 12-item scale assesses four factors of self-regulation in the moment: (1) perseverance, (2) sensation seeking, (3) emotion regulation, and (4) mindfulness (52). Briefly, momentary perseverance includes items on goal setting, tracking, and continuing to focus on them until they are finished. Momentary sensation seeking includes items on taking and enjoying risks (reverse coded). Momentary emotion regulation includes items on effectively managing and responding to emotional experiences. Lastly, momentary mindfulness includes items of attention and mindfulness related to a specific task (52). These items had strong construct and predictive validity and intra- and interindividual variability for health risk behaviors in our previous studies and proved that this novel momentary self-regulation scale can be successfully administered through mobile devices (52).
The EMAs also assessed momentary context measures. These measures were described extensively in earlier work and included items such as mood (positive and negative affect, stress, and tiredness), companionship (whether they were alone or with others), location (e.g., home, friend or family member's house, work), and sample-specific temptations to smoke or binge eat (33). EMAs were delivered through Laddr four times daily at random times within time windows at least one hour apart (e.g., 8–11:30 AM, 11:30 AM–3 PM, 3–6:30 PM, 6:30–10 PM) based on participants' self-reported sleeping and waking hours in their local time zones. EMAs also included questions about the health risk behaviors of interest depending on the sample, including access to cigarettes or food, urge to smoke or binge eat, and if they smelled smoke or food. Based on the average EMA completion time in the previous study, we expected each EMA to take less than five minutes (33).
Medical regimen adherence
Participants were asked to use Laddr daily to update their progress towards self-regulatory goals and complete various activities on the smartphone application that were expected to improve their self-regulation. As part of this adherence to the intervention, participants were recommended priority therapy guides, located directly within the Laddr application, such as binge eating guides for the BED sample and smoking guides for the smoking sample, but could also choose to engage with other guides, such as those for depression, anxiety, and substance use. Participants were also instructed to enter their daily Fitbit activity (BED sample) and daily CO readings (smoking sample) into the Laddr platform to quantitatively track progress over time. The outcome for medical regimen adherence was the use of Laddr each day for at least 5 min over the 28 consecutive day study period. This was assessed through examining Laddr usage data for each day of the study and coded a binary adherence variable (yes or no). Participants who spent at least 5 min in the app on each day were adherent.
Health behavior outcomes
Participants were asked to use devices to measure health risk behaviors and collect putative mechanisms data. The BED sample was provided with a physical activity tracking device, the Fitbit Flex 2™, and downloaded the Fitbit app on their smartphone using study credentials to track steps, distance, calories burned, active minutes, hourly activity, and stationary time. Participants were asked to wear the activity tracker for at least 12 h per day and use a changing criterion design to set stepped goals. In this design, participants set a 7-day activity criterion goal and were encouraged to increase their criterion goals in each subsequent 7-day block if they achieve the goal on at least 4 out of the 7 days (61). Physical activity data were collected by the study team in near-real-time through Fitabase, which is a secure research data management platform designed specifically for Fitbit device use. Activity data were uploaded from the Fitbit device to Fitabase every 15 min if a wireless connection was maintained or all at once when a connection to the device was re-established.
The smoking sample was provided with a breath CO meter, the iCO™ Smokerlyzer® (Bedfont® Scientific Ltd.), and downloaded the Smokerlyzer app on their smartphones to provide one CO sample in the same time window each day. During this procedure, participants exhaled into the mouthpiece of the CO meter and recorded themselves via their device camera. Specifically, this device measured CO to CO2 conversion over a catalytically active electrode in parts per million (ppm) (62). We examined if participants in the smoking sample initially reduced their CO levels from baseline levels and then achieved smoking abstinence which equates to a CO measure less than 6 ppm (63). Breath CO has a half-life of about 4–6 h, which makes it an ideal, more objective measurement for this study (64, 65). This procedure to measure breathalyzed CO as an outcome of digital health interventions has been utilized by our team in numerous other studies (55, 66–68).
Retention
Compensation
Participants were compensated up to $250 in a single payment at the end of their study period via an Amazon gift card or a paper check after returning any borrowed research equipment. This included completion of surveys ($21 for all baseline and $21 for all follow-up surveys), EMAs ($0.50 per EMA with 4 EMAs per day over 28 days, up to $56 total; $10 bonus per week for completing minimum of 25 EMAs over 4 weeks, up to $40 total), Laddr activities ($2 per day for at least 5 min of daily engagement over 28 days, up to $56 total), and wearing the Fitbit wrist sensor daily (BED sample) or using the CO monitor daily (smoking sample) and inputting these values into Laddr ($2 per day over 28 days, up to $56 total). Participants were still compensated even if they did not return the borrowed research equipment, however, their total payment was reduced by up to $50.
Compliance and reminders
The study team monitored data quality on a daily basis. EMA completion rates, Laddr app usage, and input CO values were downloaded and examined daily, while steps were tracked daily in Fitabase. Poor compliance regarding any data source was addressed through reminders sent to the participant's smartphone through both text message or Laddr app notifications and through their email addresses.
Psychometric analyses
In the Bayesian structural equation models used in this mediation analysis, described later in the methods, it is important to first establish invariance of measures across both time and people to (a) ensure that the meaning of the items does not drift over time and (b) that the items are interpreted similarly for different people in the sample (69–72). Otherwise, changes in the measurement process might be conflated with changes in the underlying constructs. Cross-classified factor analysis was used to assess between-person and between-time invariance in EMA data (73, 74). Approximate invariance was achieved if the variance in the measurement model parameters was small (75).
In the BED sample, the perseverance items were modeled as continuous and items from all other scales were modeled as categorical with a probit link, so loading means are interpreted as regression coefficients for perseverance but item discriminations for all other scales (76). This was done because only perseverance had approximately symmetric distributions. None of the scales had large between-time variability, meaning that the scales were stable across time points. However, all scales had some between-person variability, meaning that different people did not always interpret the scales in the same way. For perseverance, one item loaded weaker than the other two items, so the scale was modeled as a latent variable because a sum score would equally weigh all items (74). The full dynamic mediation model for perseverance included between-person random effects on the item parameters to account for possible differences in scale interpretation. For both the emotion regulation and mindfulness scales, one item was highly discriminating on each scale, but all items contributed to each latent variable. Therefore, the mediation models of emotion regulation and mindfulness included between-person random effects on all item parameters. Similar to the CO readings, steps taken were rescaled by dividing the raw value by 1,000 (e.g., a one-unit change corresponds to 1,000 steps) to improve the estimation by placing all variables on the same order of magnitude.
In the smoking sample, none of the scales had large between-time variability in item parameters but all the scales had between-person differences. Perseverance was modeled as a latent variable with all three indicators and included between-person random effects. The emotion regulation item only had two of the three categories endorsed with regularity, so the item was treated as dichotomous. Similarly, the mindfulness item rarely had the most extreme response selected so the two most extreme categories were collapsed into one and the item was treated as four categories instead of five. Additionally, the quantitative CO readings were rescaled on a 0–10 scale rather than 0–100 to make the scales in the analyses more congruent as well (e.g., 12 ppm on 0–100 transformed to 1.2 on 1–10).
Supplementary Table S1 shows the results from the cross-classified factor analysis for each of the three self-regulation scales and the average loading of each item across all people and measurement occasions, the variance of the loadings across people, the variance of the loadings across time, the variance of the intercepts/thresholds across people, and the variance of the intercepts/thresholds across time (74).
Statistical analyses
For the statistical models, we hypothesized that (1) participants' self-regulation will predict whether they will adhere to treatment using the Laddr intervention on the following day across both people with obesity/overweight and BED and people who smoke regularly. We hypothesized that (2) treatment adherence will then predict the objective behavioral health outcome through either the number of steps taken or the CO sample value. Lastly, we hypothesized that (3) treatment adherence and the behavioral outcome then predict self-regulation at the next measurement occasion. The models examined whether self-regulation affected health behavior through treatment adherence.
Data analysis was performed using Mplus version 8.3 with Bayesian Markov chain Monte Carlo estimation with a Gibbs sampler with two chains (77). Bayesian dynamic mediation models were used to examine moment-to-moment mediation effects between the momentary self-regulation subscales, medical regimen adherence (use of Laddr at least daily), and number of steps taken for BED sample or CO samples for the smoking sample (78, 79). This model allowed for maximized use of the repeated measures collected through EMA to examine changes in means over time and to inspect fluctuation within a person over time (Supplementary Figure S1). To overcome unequal intervals between measurements inherent in EMA modeling, a Kalman filter was applied to naturally accommodate data with unequal time intervals between measurements with a maximum interval of four hours (78–80).
Dynamic mediation models were fit for both the BED and smoking samples. These included one model for each permutation of three self-regulation scales (perseverance, emotion regulation, mindfulness) and the two behavioral outcomes (CO samples or steps taken). The sensation seeking scale was not included in the analysis. Psychometric analyses indicated that the scale items did not represent a single construct in the sample, thus the decision was made not to model the sensation seeking items as one construct like the other self-regulation scales, which would be inappropriate.
Because Bayesian estimation was used, there are no p-values or significance tests, and inference is based upon whether the null value of zero appears in the credible interval, similar to using confidence intervals for inference in models estimated with frequentist methods. Instead, the results are interpreted as either “plausibly non-null” (significant) or “found to be null” (non-significant) (81). To fit the models, preliminary psychometric analysis was performed on the three self-regulation scales for both the smoking and BED samples to examine between-time and between-person differences (Supplementary Figure S2). If meaningful, these differences were included in our dynamic mediation models to best tailor the measurement of the self-regulation constructs to each individual person. Models were used to examine the indirect effect of momentary context on health behavior as well as the direct effect of context on health behavior, which was independent of momentary self-regulation.
Results
Study participants
Binge eating sample
In the BED sample, a total of 3,381 online eligibility screenings were started (Figure 1). Of these individuals, 122 completed the screener and were eligible for the study and 53 of those individuals provided consent. While 52 participants completed the baseline survey and were provided with Laddr subscriptions, 51 participants actively started the Laddr intervention. A total of 50 participants completed at least one EMA and all 50 (100%) had at least 10% of responses over the duration of the 28-day study (≥12 out of 112 total EMAs). Of these 50 participants, 45 participants completed the follow-up surveys (90%). Participants in the BED sample who completed at least 10% of EMAs contributed a total of 4,028 momentary observations with an overall EMA response rate of 72%.
Smoking sample
In the smoking sample, a total of 15,796 online eligibility screenings were started (Figure 1). Of these individuals, 511 completed the screener and were eligible for the study and 61 of those individuals provided consent. A total of 55 participants completed the baseline survey and were provided with Laddr subscriptions, and 53 participants actively started the Laddr intervention. These 53 participants also completed at least one EMA and 50 participants (94%) completed at least 10% of responses over the duration of the four-week study (≥12 out of 112 total EMAs). Of the 50 participants who completed at least 10% of EMAs, 43 participants completed the follow-up surveys (86%). Participants in the smoking sample who completed at least 10% of EMAs contributed a total of 3,525 momentary observations with an overall EMA response rate of 63%.
Baseline characteristics
Binge eating sample
The mean age was 34.3 years (SD = 7.8, range = 18–50 years) and mean BMI was 34.0 kg/m2 (SD = 5.1, range = 27.4–44.8; Table 1). Most participants were female (58.0%), white (76.0%), non-Hispanic (90.0%), had a bachelor's degree (46.0%) and were married (54.0%). Four (8.0%) participants had been diagnosed with a mental disorder that was not determined to be due to a medical condition or classified as a psychotic disorder. Most participants never smoked (60.0%), while those who had smoked in the past did so for less than one year (55.0%), and all smoked less than 20 cigarettes per day (100.0%; Table 2). Most participants (80.0%) endorsed drinking alcohol in the past year. Among participants who drank alcohol, the highest frequency of drinking was two to four times a week (35.4%), while most participants never engaged in binge drinking (60.0%). Most participants had not smoked cannabis in the past 6 months (77.1%), while the highest frequency among those who did smoke cannabis smoked it weekly (36.4%). Few (20.0%) participants were ever on a diet to lose weight during their life and even fewer (2.0%) had ever been in therapy with a clinician for BED. Of the 50 participants who screened positive for BED on the QEWP-5, the majority reported eating an unusually large quantity of food during a short time period (94.0%), while few participants also reported the use of compensatory behaviors following episodes of binge eating at a frequency only less than once per week required: (1) vomiting to avoid weight gain (4.0%), (2) fasting to avoid weight gain (12.0%), (3) excessive exercise to avoid weight gain (6.0%), and (4) misusing laxatives (2.0%), diuretics (0.0%), or diet pills (0.0%) to avoid weight gain.
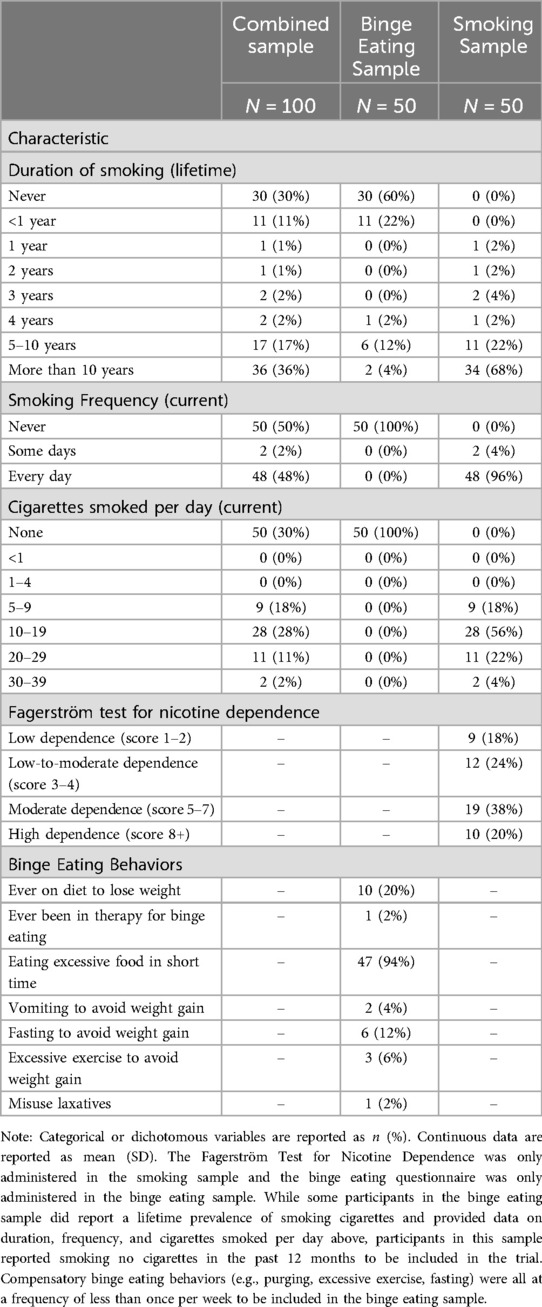
Table 2. Participant smoking and binge eating behaviors in the combined, smoking, and binge eating samples.
Smoking sample
The mean age was 33.6 years (SD = 7.1, range = 18–49 years) and mean BMI was 23.6 kg/m2 (SD = 2.3, range = 18.4–26.8; Table 1). Most participants were female (58.0%), white (86.0%), non-Hispanic (92.0%), and had some college education (54.0%), and 38.0% were married. Two (4.0%) participants had been diagnosed with a mental disorder that was not determined to be due to a medical condition or classified as a psychotic disorder. Most participants smoked more than 10 years (68.0%), smoked every day (96.0%), and smoked between 10 and 19 cigarettes per day (56.0%; Table 2). The mean score on the Fagerström Test for Nicotine Dependence was 5.1 of 10 total (SD = 2.3, range = 1–9). When categorized based on accepted cut-points, 9 (18.0%) participants had low dependence (score 1–2), 12 (24.0%) had low-to-moderate dependence (score 3–4), 19 (38.0%) had moderate dependence (score 5–7), and 10 (20.0%) had high dependence (score 8+). Most participants (70.0%) endorsed drinking alcohol in the past year. Among participants who drank alcohol, the highest frequency of drinking was once monthly or less (32.7%), while about half of participants did endorse engaging in binge drinking (51.4%). Most participants had not smoked cannabis in the past 6 months (73.5%), while most who did smoke cannabis smoked it daily or almost daily (53.9%).
Models for binge eating sample
Perseverance
Perseverance positively predicted Laddr adherence on the following day [β1 = 0.17, 95% CI = (0.06, 0.45); Table 3]. This coefficient was on the probit scale because adherence was binary, so a one unit increase in perseverance predicted a 0.17 unit increase in the normal latent variable presumed to underlie adherence. Higher Laddr adherence was subsequently a positive predictor of the steps taken on the same day such that those who adhere took about 335 more steps compared to those who did not adhere [β2 = 0.34, 95% CI = (0.03, 0.72)]. The indirect effect of perseverance on steps taken through Laddr adherence was also positive and plausibly non-null [β1 × β2 = 0.05, 95% CI = (0.004, 0.24)]. The conditional direct effect of perseverance on steps taken was found to be null [β3 = −0.05, 95% CI = (−0.60, 0.45)], suggesting complete mediation. There was a positive feedback loop such that Laddr adherence [β5 = 0.12, 95% CI = (0.05, 0.26)] and steps taken [β4 = 0.03, 95% CI = (0.002, 0.07)] both predicted increases in perseverance on the following day.
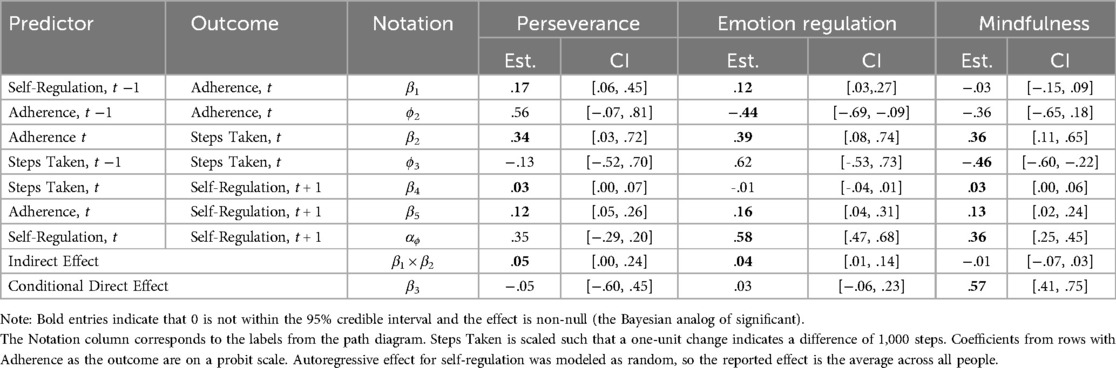
Table 3. Estimates of focal parameters for dynamic mediation models using steps taken as the behavior outcome.
Emotion regulation
Emotion regulation predicted Laddr adherence on the following day [β1 = 0.12, 95% CI = (0.03, 0.27)], where a one unit increase in emotion regulation predicted a 0.12 unit increase in the normal latent variable presumed to underlie adherence. Higher Laddr adherence was subsequently a positive predictor of steps taken the same day such that adherence predicted an increase of about 389 steps [β2 = 0.389, 95% CI = (0.080, 0.738)]. The indirect effect of emotion regulation on steps taken through Laddr adherence was also positive and plausibly non-null [β1 × β2 = 0.04, 95% CI = (0.01, 0.14)]. The conditional direct effect of emotion regulation on steps taken was found to be null [β3 = 0.03, 95% CI = (−0.06, 0.23)], suggesting complete mediation through Laddr adherence. There was a positive feedback loop such that Laddr adherence [β5 = 0.16, 95% CI = (0.04, 0.31)] predicted increases in emotion regulation on the following day, however, steps taken did not predict emotion regulation on the following day [β4 = −0.01, 95% CI = (−0.04, 0.01)].
Mindfulness
Mindfulness did not predict Laddr adherence on the following day [β1 = −0.03, 95% CI = (−0.15, 0.09)], but Laddr adherence did positively predict the number of steps taken on the same day [β2 = 0.355, 95% CI = (0.108, 0.648)]. The indirect effect of mindfulness on steps taken through Laddr adherence was found to be null [β1 × β2 = −0.01, 95% CI = (−0.07, 0.03)], but the conditional direct effect of mindfulness on steps taken on the following day was positive and plausibly non-null [β3 = 0.57, 95% CI = (0.41, 0.75)] such that a one standard deviation increase above the person-specific baseline of mindfulness predicted an increase in 570 steps on the following day. There was a positive feedback loop such that Laddr adherence [β5 = 0.13, 95% CI = (0.02, 0.24)] and steps taken [β4 = 0.03, 95% CI = (0.00, 0.06)] both predicted increases in mindfulness on the following day.
Models for smoking sample
Perseverance
Perseverance was found to positively predict Laddr adherence on the following day [β1 = 0.91, 95% CI = (0.60, 1.24)] where a one unit increase in perseverance predicted a 0.91 unit increase in the normal latent variable presumed to underlie adherence (Table 4). However, higher Laddr adherence was not subsequently a predictor of CO values on the same day [β2 = −0.09, 95% CI = (−0.24, 0.09)]. Consequently, the indirect effect of perseverance on CO values through Laddr adherence was found to be null [β1 × β2 = −0.07, 95% CI = (−0.24, 0.08)]. The conditional direct effect of perseverance on CO values on the following day was also found to be null [β3 = −0.20, 95% CI = (−0.59, 0.10)]. There was a positive feedback loop such that Laddr adherence [β5 = 0.25, 95% CI = (0.18, 0.35)] and CO values [β4 = 0.20, 95% CI = (0.09, 0.27)] both predicted increases in perseverance on the following day.
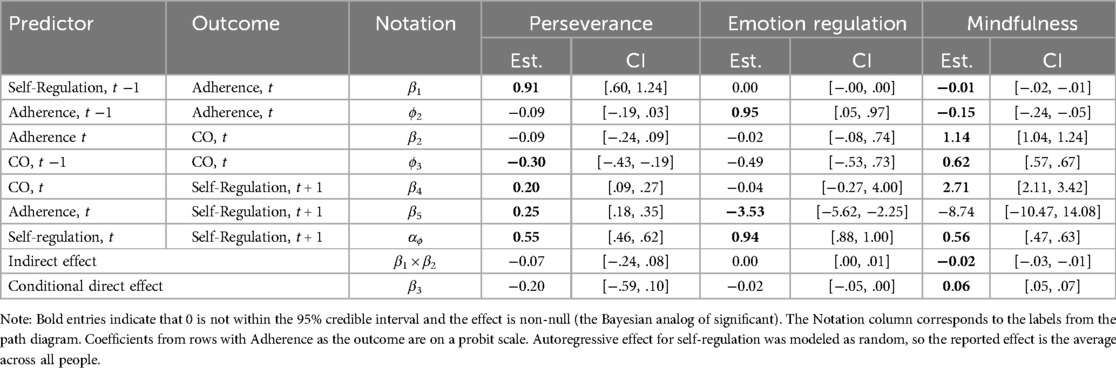
Table 4. Estimates of focal parameters for dynamic mediation models using carbon monoxide (CO) samples as the behavior outcome.
Emotion regulation
Emotion regulation did not predict Laddr adherence on the following day [β1 = 0.00, 95% CI = (−0.00, 0.01)]. Laddr adherence also did not predict CO values on the same day [β2 = −0.02, 95% CI = (−0.11, 1.14)]. The indirect effect of emotion regulation on CO values was also found to be null [β1 × β2 = 0.00, 95% CI = (0.00, 0.01)]. The conditional direct effect of perseverance on CO values on the following day was also found to be null [β3 = −0.02, 95% CI = (−0.05, 0.00)]. There was a negative feedback loop such that Laddr adherence [β5 = −3.53, 95% CI = (−5.62, −2.25)] predicted decreases in emotion regulation on the following day, however, CO values did not predict emotion regulation on the following day [β4 = −0.04, 95% CI = (−0.27, 4.00)].
Mindfulness
Mindfulness was found to negatively predict Laddr adherence on the following day [β1 = −0.01, 95% CI = (−0.02, −0.005)] where a one unit increase in mindfulness predicted a 0.01 unit decrease in the normal latent variable presumed to underlie adherence. Higher Laddr adherence was related to higher CO values on the same day [β2 = 1.14, 95% CI = (1.04, 1.24)]. The indirect effect of mindfulness on CO values through Laddr adherence was negative and plausibly non-null [β1 × β2 = −0.015, 95% CI = (−0.03, −0.01)], indicating the possibility of a suppression effect. The conditional direct effect of mindfulness on CO values on the following day was positive and plausibly non-null [β3 = 0.06, 95% CI = (0.05, 0.07)]. There was a positive feedback loop such that CO values predicted increases in mindfulness on the following day [β4 = 2.71, 95% CI = (2.11, 3.42)], but Laddr adherence did not predict mindfulness on the following day [β5 = −8.74, 95% CI = (−10.47, 14.08)].
Discussion
To our knowledge, this is the first study to examine momentary self-regulation as a behavior change mechanism across different health risk behaviors and to explore how adherence to a digital therapeutic can mediate the relationship between self-regulation and these risk behaviors. While other studies have found that lapses in self-regulation are related to increased smoking, physical inactivity, and binge eating, among other risky behaviors, there has been little evaluation of the mechanisms behind these relationships, and especially the dynamic nature of these mechanistic processes in daily life (23, 29, 31, 82). The present study explored these dynamic mechanisms in real-life situations.
First, we hypothesized that several components of momentary self-regulation would predict adherence to the Laddr intervention on the following day in both samples and found that higher perseverance increased adherence to the intervention across both people with obesity/overweight and BED and people who smoke regularly. Next, we hypothesized that treatment adherence would predict the objective behavioral health outcomes and found that higher adherence increased physical activity levels among people with obesity/overweight and BED. Lastly, we hypothesized that treatment adherence and the behavioral outcomes would act as a positive feedback loop to predict self-regulation at the next measurement. We found that both adherence to the intervention and the behavioral outcomes (steps taken and CO values) predicted several components of increased momentary self-regulation across both samples, although results were more mixed in the smoking sample.
Momentary self-regulation impacted adherence to a digital therapeutic
While self-regulation is usually examined as a static trait-level characteristic, this study utilized a novel momentary self-regulation scale developed by our interdisciplinary team to measure self-regulation across time and contexts (33, 52). Recent studies suggest that self-regulation is a dynamic process that changes based on individual contexts, but, to our knowledge, this metric is the first assessment tool to examine the dynamic nature of self-regulation (33). This scale was embedded within a novel digital therapeutic called Laddr and successfully administered through mobile devices to assess momentary self-regulation in a nonlaboratory, naturalistic setting (52). As also suggested by prior studies, our results demonstrate that self-regulation has variability depending on real-world internal and external contexts in two exemplar groups of people with health risk behaviors (33, 52).
This current study utilized the novel momentary self-regulation scale to examine if momentary self-regulation impacted adherence to a digital health intervention. It is critically important to understand the mechanisms underlying effective health behavior change and determine how these mechanisms may differ across different health conditions (83). Here, “mechanism” refers to intervention-induced changes in psychological, behavioral, or biological factors, which then cause changes in health risk behaviors (84).
Results suggest that putative targets of momentary self-regulation did impact adherence to the digital therapeutic across groups of people with different health risk behaviors. The perseverance target of momentary self-regulation was the only singular target found to positively predict Laddr adherence on the following day in both people with obesity/overweight and BED and people who smoke regularly. This suggests that higher goal setting, tracking, and continual focus on achieving goals aimed at increased levels of physical activity or decreased levels of smoking, respectively—all key components of perseverance—may be related to future adherence to a digital health intervention on the following day for both of these health risk behaviors (52). Additionally, the emotion regulation target of momentary self-regulation was found to positively predict Laddr adherence on the following day in people with obesity/overweight and BED. This suggests that effectively managing and responding to emotional experiences may also be related to future adherence to a digital health intervention on the following day, particularly in people with obesity/overweight and BED (52).
Targeting self-regulation through a digital therapeutic
Next, we examined if increasing self-regulation through a digital therapeutic then increased adherence to the intervention and increased pro-health behaviors. This was another highly innovative aspect of the present study. Indeed, there are over 300,000 health-related smartphone applications on both the Apple and Google Play stores, with over 10,000 claiming to influence behavioral health (85). Most of these interventions are uploaded to the store without evaluation with scientific methods, and even fewer have examined underlying mechanisms of their claimed behavior change (85).
This is in stark contrast to the drug discovery process, which includes strict guidelines, including early drug discovery, a pre-clinical phase of research, clinical phases of research, and regulatory approval, often over numerous years of testing (86). These studies examine the effect of the drug on a biological target (e.g., a receptor, enzyme, protein, gene) and must be performed across numerous assays, doses, and populations as applicable (87). The goal of this process is to identify a biological target, and not solely to develop a treatment with clinical benefit. In contrast, most studies of behavior change interventions only examine specific clinical endpoints like smoking or obesity and do not routinely examine these putative targets or the mechanisms of behavior change (51).
This study utilized the experimental medicine approach, proposed by the NIH's Science of Behavior Change (SOBC) initiative and demonstrated that targeting self-regulatory targets through a digital health intervention impacted adherence to the intervention and increased pro-health behaviors. Higher Laddr adherence was found to mediate the relationship between the perseverance and emotion regulation targets of momentary self-regulation and the number of steps taken in people with obesity/overweight and BED. This suggests that adherence to Laddr increased the behavioral health outcome of physical activity in this sample. Thus, this study demonstrated that engaging the self-regulatory targets of perseverance and emotion regulation produced a desired change in medical regimen adherence and in health behavioral outcomes in people with obesity/overweight and BED.
Health behavior outcomes between the binge eating and smoking samples
Although numerous interventions have been effective in initiating and maintaining health behavior change and medical regimen adherence, including those targeting smoking and binge eating, most of this work has been siloed (50, 88–90). That is, most interventions target only one disease or disorder at a time, while the need to reduce health risk behaviors is ubiquitous across numerous clinical populations. This prompted us to examine these mechanisms in two exemplar samples within this work, people with obesity/overweight and BED and people who smoke regularly, to transdiagnostically study momentary self-regulation across different health-risk behaviors.
That said, the relationships between self-regulation, adherence to Laddr and pro-health behaviors were more mixed in the smoking sample compared to the BED sample. One possible reason for this may be the specific medical regimen—an exclusively digital behavioral intervention—offered to the participants in this study. That is, numerous other studies have suggested that BED and obesity can be effectively managed with behavioral health treatments. Specifically, cognitive behavior therapy (CBT) has repeatedly been shown to be effective in managing BED (91). The Laddr intervention incorporated CBT-based self-monitoring, control strategies, activity goals, and problem solving to help participants develop regular, moderate eating patterns, as web-based, self-help strategies have been shown in previous studies to be efficacious with decreased average number of binges (91).
However, behavioral interventions alone have not always been as efficacious for quitting smoking compared to combined behavioral and pharmacological interventions across other health conditions (92–94). Pharmacotherapies are often a critical part of effective smoking cessation interventions to treat nicotine dependence. Multiple systematic reviews have found that combining both behavioral therapy and pharmacotherapy is more effective in promoting smoking abstinence compared to either strategy alone (95–97). Most clinical trials examining behavioral support for smoking cessation also include a pharmacotherapy arm, and a recent meta-analysis of over 23,000 participants in 65 studies found that combined treatment was associated with 15% higher rates of smoking abstinence (95).
Although the present study did not exclude individuals taking nicotine replacement therapy, use of other medications for smoking cessation (e.g., bupropion, varenicline) was exclusionary. And prior work has demonstrated the value of these medications as part of smoking cessation treatment. For example, a randomized controlled trial of varenicline and CBT vs. placebo medication and CBT for smoking cessation found that 60% of participants in the varenicline group and only 19% in the placebo group had smoking abstinence at 1 year follow-up (93, 94). Together, these studies and our exclusion of participants with concurrent pharmacotherapy for smoking cessation might suggest why adherence to the Laddr behavioral health intervention demonstrated increased pro-health behaviors in the BED sample compared to the smoking sample. Future studies examining digital therapeutics in people who smoke regularly should also add a digital intervention-plus-pharmacotherapy arm to further examine the impact of combining these two interventions on promoting smoking cessation.
Understanding the role of mindfulness in promoting adherence and health behavior change
The mindfulness factor of momentary self-regulation also had mixed results in the BED and smoking samples. Mindfulness alone did not predict greater Laddr adherence on the following day in the BED sample and predicted less adherence on the following day within the smoking sample. One possible explanation of this finding may be because mindfulness is “the act of paying attention in the present moment,” as opposed to future orientation (98). People practicing mindfulness try to self-regulate their attention to the current moment and this may direct their attention away from behaviors that promote future medical regimen adherence (99). Similar to our findings, a recent systematic review examining mindfulness interventions to improve medication adherence across different medical conditions found mixed results with only 44% of the included studies reporting increased medication adherence with mindfulness training (88). The review concluded that the use of mindfulness interventions to promote medication adherence remains understudied across different diseases and our current study also supports the need for future research on the impact of momentary mindfulness on medical regimen adherence (88).
When examining the health behavior outcome in the smoking sample, mindfulness directly predicted higher CO values on the following day, but also indirectly predicted lower CO values on the following day through Laddr adherence. This finding may suggest that higher mindfulness, possibly focused on smoking abstinence, on the preceding day may be related to increased smoking as a compensatory mechanism on the following day. Nicotine compensation is a behavior related to biological dependence of nicotine where people who smoke regularly increase their number of cigarettes, puffs, or depth of the inhalation in response to a period of abstinence from nicotine (100). Laboratory studies have shown that short-term nicotine restriction may lead to compensatory smoking and increased levels of CO, which might help explain why mindfulness predicted higher CO values on the following day in our study (101). But continued adherence to Laddr may disrupt that relationship by providing more tools to individuals to help them effectively reduce smoking behavior.
Lastly, it is important to recognize the possible implications of these results to the mindfulness subscale itself, which may be measuring a distinct construct from other measures of momentary self-regulation. Several studies have described mindfulness as a unique self-regulation strategy that differs from other emotion regulation strategies (102–105). Additionally, the actual neural mechanisms behind mindfulness-based regulation may differ between participants, where some participants may use cognitive reappraisal strategies based on top-down neural systems, and other participants engage in more emotion regulation based on bottom-up neural systems (106, 107).
Transdiagnostic mediating effects of momentary self-regulation
Overall, our series of studies was able to accomplish the three initiatives of the NIH SOBC initiative, including (1) investigating mechanisms of behavior change across different health behaviors, (2) finding common predictable targets of health behaviors, and (3) modifying clinical endpoints through replicable and scalable behavioral health interventions (51). First, our interdisciplinary team studied the ontology of self-regulatory processes in people with obesity/overweight and BED and people who smoke regularly. These are two exemplar samples where “lapses” in self-regulation can contribute to reduced medical regimen adherence and negative health outcomes (1, 2). Second, three momentary self-regulation targets of perseverance, emotion regulation, and mindfulness were examined as common predictable targets across health risk behaviors of smoking and physical inactivity (33). Third, adherence to the Laddr digital therapeutic was examined as a predictor of the clinical endpoints: number of steps taken for physical inactivity and CO values for smoking.
Our studies applied novel technologies and methods to inform the ontology of momentary self-regulation in people with obesity/overweight and BED and people who smoke regularly. Our work complements and extends the important work of four other NIH SOBC-funded studies which examined self-regulation in the context of prediabetes, mental and physical health, mood and weight, and health behaviors in children (108). Three additional SOBC-funded studies examined other key factors known to impact health behavior change, including (1) stress reactivity and stress resilience and (2) interpersonal and social processes (51). All these projects utilized the experimental medicine approach to perform assay development and validation of targets on one of these three domains: self-regulation, stress, and social processes (108). While these studies allowed for the comparison of different behavior change targets, future studies could examine the combined influence of these factors on how self-regulation, stress, and social processes work together to impact health behavior change. While studying these domains is important in understanding the putative targets of health behavior change, it is also important to recognize that there are many factors that can impact behavior change beyond those included in the SOBC initiative (109). More recently, the SOBC program now hosts a resource and coordinating center, including the Checklist for Investigating Mechanisms in Behavior-change Research (CLIMBR) guidelines, designed to support the use of the experimental medicine approach in the experimental study of other malleable behavioral processes (109).
Future studies could also incorporate additional passive sensing data, such as smartphone geospatial data, which can allow for the examination of external contexts on an individual's self-regulation and adherence to a digital health intervention. Passive sensing can incorporate different streams of smartphone data including physiology, movement, location, audio, and smartphone app usage to predict current and future changes in health behavior (110–112). Such passive sensing data have predicted future behavioral outcomes, including, for example, momentary changes in depressed mood in people with depression, and have been studied across other mental health problems, sleep disorders, substance use, stress, and cardiovascular disease (112, 113). While our study included measures of momentary self-regulation collected through EMAs, future studies can also include smartphone passive sensing to determine how external contexts affect an individual's self-regulation and adherence to digital health interventions.
Limitations
This study had several limitations. First, while we examined two exemplar samples of people with BED and people who smoke, the inclusion criteria prevented overlapping samples. Therefore, this study may not be generalizable to people who smoke and have co-occurring BED and therefore not generalizable to people with multiple health risk behaviors. Second, while this study included a racially diverse sample comparable to the 2022 United States census, individuals identifying as Hispanic or Latino were underrepresented in this sample. This suggests that targeted sampling strategies need to do better at recruiting based on ethnicity within these groups of people with health risk behaviors. Third, our online screening procedure did not initially prohibit prospective participants from completing the screening questionnaire more than once, so it is possible that some participants may have been included in the study who should have been deemed ineligible. We implemented guardrails against repeated screening attempts in the later stages of recruitment by manually reviewing the screening questionnaires for identifiers (e.g., name, phone number, ZIP Code) prior to enrolling participants. Participants who enrolled before we implemented these additional checks would have remained in the study. Fourth, the dynamic mediation models only included three self-regulation scales (perseverance, emotion regulation, mindfulness) and excluded the sensation seeking scale. Psychometric analysis indicated that the items of sensation seeking did not represent a single construct in the sample, so it may be considered inappropriate to model these items as one construct like the other self-regulation scales. Because an objective of this study was to examine mechanisms across different targets of momentary self-regulation, we determined that modeling sensation seeking in a different way than the other three scales would make the results less generalizable and harder to compare between targets. Our prior study examining putative targets of momentary self-regulation did not find any significant associations between urge to smoke or urge to binge eat and momentary sensation seeking (33). This suggests that sensation seeking might play a different role than the other targets of momentary self-regulation in both the smoking and BED samples. Although our momentary self-regulation scale had strong construct and predictive validity and intra- and interindividual variability, future research should further explore the sensation seeking items. Lastly, the current study was an observational study of self-regulation targets, medical regimen adherence, and health behavior outcomes, so other unmeasured confounding variables may have contributed to these observed relations. However, the longitudinal nature of the study reduces the influence of potential confounders. Future directions of this research include evaluating effects of randomization to the Laddr intervention or comparison conditions and applications to other momentary behaviors.
Conclusions
This study provides evidence that a digital therapeutic targeting self-regulation can modify several relationships between momentary self-regulation, medical regimen adherence and behavioral health outcomes in people with health risk behaviors. Results demonstrate that the perseverance target of momentary self-regulation increased Laddr medical regimen adherence on the following day in both people with obesity/overweight and BED and people who smoke regularly. Then, higher adherence to the Laddr intervention predicted the behavioral health outcome of increased physical activity. Additionally, higher Laddr adherence then predicted increased perseverance and emotion regulation targets on the following day people with obesity/overweight and BED. Together this suggests that the influence of momentary self-regulation on health risk behaviors may occur through increased medical regimen adherence to the Laddr digital health intervention, particularly in people with obesity/overweight and BED. Future studies should validate these findings across various other digital health interventions and other health risk behaviors. Within the larger NIH SOBC initiative, this work demonstrated the ability to assess several transdiagnostic mediating effects of momentary self-regulation on medical regimen adherence and pro-health behavioral outcomes.
Data availability statement
The raw data supporting the conclusions of this article will be made available by the authors, without undue reservation.
Ethics statement
The studies involving humans were approved by Dartmouth College Institutional Review Board. The studies were conducted in accordance with the local legislation and institutional requirements. The participants provided their written informed consent to participate in this study.
Author contributions
EP: Data curation, Formal Analysis, Visualization, Writing – original draft, Writing – review & editing. DM: Data curation, Formal Analysis, Methodology, Visualization, Writing – original draft, Writing – review & editing. SB: Data curation, Writing – review & editing. KB: Data curation, Writing – review & editing. JD: Methodology, Writing – review & editing. MG: Data curation, Software, Writing – review & editing. MK: Methodology, Writing – review & editing. HL: Data curation, Writing – review & editing. SL: Data curation, Writing – review & editing. ML: Methodology, Writing – review & editing. DPM: Conceptualization, Funding acquisition, Methodology, Writing – review & editing. SM: Data curation, Methodology, Writing – review & editing. LO: Methodology, Writing – review & editing. JP: Methodology, Writing – review & editing. CS: Data curation, Writing – review & editing. ES: Data curation, Methodology, Writing – review & editing. LS: Methodology, Writing – review & editing. HX: Methodology, Writing – review & editing. LM: Conceptualization, Funding acquisition, Methodology, Writing – original draft, Writing – review & editing.
Funding
The author(s) declare financial support was received for the research, authorship, and/or publication of this article. This research was supported by the National Institutes of Health (NIH) Science of Behavior Change (SOBC) Common Fund through an award administered by the National Institute on Drug Abuse (Grant 5UH3DA041713-05), NIDA P30 center grant (P30DA029926) awarded to the Dartmouth Center for Technology and Behavioral Health, and a NIDA T32 Training Grant (5T32DA037202-10). Co-authors were also supported by a T32 Training Grant (ST32DA037202-10) and NIDA grant (R37DA09757).
Acknowledgments
The authors want to thank all of the participants who contributed to this study. The authors are grateful to John Higgins, MS, at Dartmouth College, who provided online survey support via the Research Electronic Data Capture (REDCap) platform through a Dartmouth SYNERGY Biomedical Informatics Core grant. We want to thank Patrick Bissett, PhD and Russell Poldrack, PhD from the Department of Psychology at Stanford University for their contributions to the study design and Cara Bohon, PhD from the Department of Psychiatry and Behavioral Sciences at Stanford University for her contributions to the recruitment of people with obesity/overweight and binge eating disorder.
Conflict of interest
In addition to her academic affiliation with Dartmouth College, LM is affiliated with Square2 Systems, Inc., the small business that developed the mobile Laddr intervention and has worked extensively with relevant institutions (including Dartmouth College and the NIH) to manage any potential conflict of interest. All research data collection, data management, and statistical analyses are conducted by individuals with no affiliation with Square2 Systems. In addition to her academic affiliation with Stanford University, JP has served as an expert witness against the tobacco companies in lawsuits for which she has received fees for the work and has provided consultation to pharmaceutical and technology companies that make medications and other treatments for quitting smoking.
CS was employed by Apple Inc.
The remaining authors declare that the research was conducted in the absence of any commercial or financial relationships that could be construed as a potential conflict of interest.
The author(s) declared that they were an editorial board member of Frontiers, at the time of submission. This had no impact on the peer review process and the final decision.
Publisher's note
All claims expressed in this article are solely those of the authors and do not necessarily represent those of their affiliated organizations, or those of the publisher, the editors and the reviewers. Any product that may be evaluated in this article, or claim that may be made by its manufacturer, is not guaranteed or endorsed by the publisher.
Author disclaimer
The views expressed in this article do not necessarily represent the views of the National Institutes of Health, the Department of Health and Human Services, or the United States Government.
Supplementary material
The Supplementary Material for this article can be found online at: https://www.frontiersin.org/articles/10.3389/fdgth.2025.1467772/full#supplementary-material
Supplementary Figure S1 | The within-person structural model portion of the dynamic mediation model. Self-regulation is a latent variable that predicts (latent-centered) behavior and treatment adherence at the next time-point. Treatment adherence then predicts behavior at the same time-point. Both treatment adherence and behavior then predict self-regulation as the next time-point. Lagged effects of self-regulation and the amplitude of the self-regulation time-series are permitted to vary across people.
Supplementary Figure S2 | The between-person portion of the dynamic mediation model. Each random parameter from the within-person model is featured as a latent variable with a fixed effect, between-person variance, and possible covariances with other latent variables. The residual variance capturing the amplitude of the time-series is modeled on the natural log scale to ensure that values are strictly positive.
References
1. GBD 2013 Risk Factors Collaborators, Forouzanfar MH, Alexander L, Anderson HR, Bachman VF, Biryukov S, et al. Global, regional, and national comparative risk assessment of 79 behavioural, environmental and occupational, and metabolic risks or clusters of risks in 188 countries, 1990–2013: a systematic analysis for the global burden of disease study 2013. Lancet. (2015) 386(10010):2287–323. doi: 10.1016/S0140-6736(15)00128-2
2. Peltzer K, Pengpid S, Yung TKC, Aounallah-Skhiri H, Rehman R. Comparison of health risk behavior, awareness, and health benefit beliefs of health science and non-health science students: an international study. Nurs Health Sci. (2016) 18(2):180–7. doi: 10.1111/nhs.12242
3. McGinnis JM, Foege WH. Actual causes of death in the United States. JAMA. (1993) 270(18):2207–12. doi: 10.1001/jama.1993.03510180077038
4. Knoops KT, de Groot LCPGM, Kromhout D, Perrin A-E, Moreiras-Varela O, Menotti A, et al. Mediterranean Diet, lifestyle factors, and 10-year mortality in elderly European men and women: the HALE project. JAMA. (2004) 292(12):1433–9. doi: 10.1001/jama.292.12.143315383513
5. Piot P, Caldwell A, Lamptey P, Nyrirenda M, Mehra S, Cahill K, et al. Addressing the growing burden of non-communicable disease by leveraging lessons from infectious disease management. J Glob Health. (2016) 6(1):010304. doi: 10.7189/jogh.06.010304
6. Ward ZJ, Bleich SN, Cradock AL, Barrett JL, Giles CM, Flax C, et al. Projected U.S. state-level prevalence of adult obesity and severe obesity. N Engl J Med. (2019) 381(25):2440–50. doi: 10.1056/NEJMsa1909301
7. Saint-Maurice PF, Graubard BI, Troiano RP, Berrigan D, Galuska DA, Fulton JE, et al. Estimated number of deaths prevented through increased physical activity among US adults. JAMA Intern Med. (2022) 182(3):349–52. doi: 10.1001/jamainternmed.2021.7755
8. Dai X, Gakidou E, Lopez AD. Evolution of the global smoking epidemic over the past half century: strengthening the evidence base for policy action. Tob Control. (2022) 31(2):129–37. doi: 10.1136/tobaccocontrol-2021-056535
9. 2022 NSDUH annual national report. Available online at: https://www.samhsa.gov/data/report/2022-nsduh-annual-national-report (cited 2024 March 28).
10. Cutler RL, Fernandez-Llimos F, Frommer M, Benrimoj C, Garcia-Cardenas V. Economic impact of medication non-adherence by disease groups: a systematic review. BMJ Open. (2018) 8(1):e016982. doi: 10.1136/bmjopen-2017-016982
11. DiMatteo MR, Giordani PJ, Lepper HS, Croghan TW. Patient adherence and medical treatment outcomes: a meta-analysis. Med Care. (2002) 40(9):794–811. doi: 10.1097/00005650-200209000-00009
12. Osterberg L, Blaschke T. Adherence to medication. N Engl J Med. (2005) 353(5):487–97. doi: 10.1056/NEJMra050100
13. DiMatteo MR. Variations in patients’ adherence to medical recommendations: a quantitative review of 50 years of research. Med Care. (2004) 42(3):200–9. doi: 10.1097/01.mlr.0000114908.90348.f9
14. Bosworth HB, Oddone EZ, Weinberger M. Patient Treatment Adherence: Concepts, Interventions, and Measurement. New York, NY: Psychology Press (2006). p. 278. Available online at: https://play.google.com/store/books/details?id=goF5AgAAQBAJ
15. Shah ND, Dunlay SM, Ting HH, Montori VM, Thomas RJ, Wagie AE, et al. Long-term medication adherence after myocardial infarction: experience of a community. Am J Med. (2009) 122(10):961.e7–13. doi: 10.1016/j.amjmed.2008.12.021
16. Vanelli M, Pedan A, Liu N, Hoar J, Messier D, Kiarsis K. The role of patient inexperience in medication discontinuation: a retrospective analysis of medication nonpersistence in seven chronic illnesses. Clin Ther. (2009) 31(11):2628–52. doi: 10.1016/j.clinthera.2009.11.028
17. Kleinsinger F. The unmet challenge of medication nonadherence. Perm J. (2018) 22:18–033. doi: 10.7812/TPP/18-033
18. Hagger MS, Cameron LD, Hamilton K, Hankonen N, Lintunen T. The Handbook of Behavior Change. Cambridge: Cambridge University Press (2020). p. 730. Available online at: https://play.google.com/store/books/details?id=IfEFEAAAQBAJ
19. Allegrante JP, Wells MT, Peterson JC. Interventions to support behavioral self-management of chronic diseases. Annu Rev Public Health. (2019) 40:127–46. doi: 10.1146/annurev-publhealth-040218-044008
20. Kvarnström K, Westerholm A, Airaksinen M, Liira H. Factors contributing to medication adherence in patients with a chronic condition: a scoping review of qualitative research. Pharmaceutics. (2021) 13(7):1100. doi: 10.3390/pharmaceutics13071100
21. Conn VS, Ruppar TM, Enriquez M, Cooper P. Medication adherence interventions that target subjects with adherence problems: systematic review and meta-analysis. Res Social Adm Pharm. (2016) 12(2):218–46. doi: 10.1016/j.sapharm.2015.06.001
22. Eisenberg IW, Bissett PG, Canning JR, Dallery J, Enkavi AZ, Whitfield-Gabrieli S, et al. Applying novel technologies and methods to inform the ontology of self-regulation. Behav Res Ther. (2018) 101:46–57. doi: 10.1016/j.brat.2017.09.014
23. Bickel WK, Odum AL, Madden GJ. Impulsivity and cigarette smoking: delay discounting in current, never, and ex-smokers. Psychopharmacology. (1999) 146(4):447–54. doi: 10.1007/PL00005490
24. Henningfield JE, Santora PB, Bickel WK. Addiction Treatment: Science and Policy for the Twenty-first Century. Baltimore, MD: JHU Press (2007). p. 221. Available online at: https://play.google.com/store/books/details?id=-mw0UFhQPfYC
25. Alessi SM, Petry NM. Pathological gambling severity is associated with impulsivity in a delay discounting procedure. Behav Processes. (2003) 64(3):345–54. doi: 10.1016/S0376-6357(03)00150-5
26. Mackillop J, Anderson EJ, Castelda BA, Mattson RE, Donovick PJ. Convergent validity of measures of cognitive distortions, impulsivity, and time perspective with pathological gambling. Psychol Addict Behav. (2006) 20(1):75–9. doi: 10.1037/0893-164X.20.1.75
27. MacKillop J, Anderson EJ, Castelda BA, Mattson RE, Donovick PJ. Divergent validity of measures of cognitive distortions, impulsivity, and time perspective in pathological gambling. J Gambl Stud. (2006) 22(3):339–54. doi: 10.1007/s10899-006-9021-9
28. Petry NM. Pathological gamblers, with and without substance use disorders, discount delayed rewards at high rates. J Abnorm Psychol. (2001) 110(3):482–7. doi: 10.1037/0021-843X.110.3.482
29. Epstein LH, Salvy SJ, Carr KA, Dearing KK, Bickel WK. Food reinforcement, delay discounting and obesity. Physiol Behav. (2010) 100(5):438–45. doi: 10.1016/j.physbeh.2010.04.029
30. Schwarzer R, Lippke S, Luszczynska A. Mechanisms of health behavior change in persons with chronic illness or disability: the health action process approach (HAPA). Rehabil Psychol. (2011) 56(3):161–70. doi: 10.1037/a0024509
31. de Ridder D, de Wit J. Self-Regulation in Health Behavior. Hoboken, NJ: John Wiley & Sons (2006). p. 264. Available online at: https://play.google.com/store/books/details?id=CoGNDeVdjoQC
32. Roos CR, Witkiewitz K. A contextual model of self-regulation change mechanisms among individuals with addictive disorders. Clin Psychol Rev. (2017) 57:117–28. doi: 10.1016/j.cpr.2017.08.008
33. Scherer EA, Metcalf SA, Whicker CL, Bartels SM, Grabinski M, Kim SJ, et al. Momentary influences on self-regulation in two populations with health risk behaviors: adults who smoke and adults who are overweight and have binge-eating disorder. Front Digit Health. (2022) 4:798895. doi: 10.3389/fdgth.2022.798895
34. Lee M, Lee H, Kim Y, Kim J, Cho M, Jang J, et al. Mobile app-based health promotion programs: a systematic review of the literature. Int J Environ Res Public Health. (2018) 15(12):2838. doi: 10.3390/ijerph15122838
35. Murray E, Hekler EB, Andersson G, Collins LM, Doherty A, Hollis C, et al. Evaluating digital health interventions: key questions and approaches. Am J Prev Med. (2016) 51(5):843–51. doi: 10.1016/j.amepre.2016.06.008
36. Martin M. Computer and internet use in the United States: 2018 (2021). Available from: Available online at: https://www.census.gov/history/pdf/computer-internetuse2018.pdf (cited 2023 September 6).
37. Douthit N, Kiv S, Dwolatzky T, Biswas S. Exposing some important barriers to health care access in the rural USA. Public Health. (2015) 129(6):611–20. doi: 10.1016/j.puhe.2015.04.001
38. Silver L. Smartphone ownership is growing rapidly around the world, but not always equally. Pew Research Center (2019). Available online at: https://www.pewresearch.org/global/2019/02/05/smartphone-ownership-is-growing-rapidly-around-the-world-but-not-always-equally/ (cited 2024 May 10).
39. Torrey WC, Cepeda M, Castro S, Bartels SM, Cubillos L, Obando FS, et al. Implementing technology-supported care for depression and alcohol use disorder in primary care in Colombia: preliminary findings. Psychiatr Serv. (2020) 71(7):678–83. doi: 10.1176/appi.ps.201900457
40. Marsch LA, Gómez-Restrepo C, Bartels SM, Bell K, Camblor PM, Castro S, et al. Scaling up science-based care for depression and unhealthy alcohol use in Colombia: an implementation science project. Psychiatr Serv. (2022) 73(2):196–205. doi: 10.1176/appi.ps.202000041
41. Jacobson NC, Kowatsch T, Marsch LA, editors. Digital Therapeutics for Mental Health and Addiction: The State of the Science and Vision for the Future. San Diego, CA: Academic Press (2022). p. 268. Available online at: https://shop.elsevier.com/books/digital-therapeutics-for-mental-health-and-addiction/jacobson/978-0-323-90045-4 (cited 2024 May 10).
42. Skinner R, Gonet V, Currie S, Hoddinott P, Dombrowski SU. A systematic review with meta-analyses of text message-delivered behaviour change interventions for weight loss and weight loss maintenance. Obes Rev. (2020) 21(6):e12999. doi: 10.1111/obr.12999
43. Hall CS, Fottrell E, Wilkinson S, Byass P. Assessing the impact of mHealth interventions in low- and middle-income countries–what has been shown to work? Glob Health Action. (2014) 7:25606. doi: 10.3402/gha.v7.25606
44. Marsch LA, Guarino H, Acosta M, Aponte-Melendez Y, Cleland C, Grabinski M, et al. Web-based behavioral treatment for substance use disorders as a partial replacement of standard methadone maintenance treatment. J Subst Abuse Treat. (2014) 46(1):43–51. doi: 10.1016/j.jsat.2013.08.012
45. Sasseville M, LeBlanc A, Tchuente J, Boucher M, Dugas M, Gisèle M, et al. The impact of technology systems and level of support in digital mental health interventions: a secondary meta-analysis. Syst Rev. (2023) 12(1):78. doi: 10.1186/s13643-023-02241-1
46. Marsch LA, Lord SE, Dallery J. Behavioral Healthcare and Technology: Using Science-based Innovations to Transform Practice. Oxford: Oxford University Press (2015). p. 348. Available online at: https://play.google.com/store/books/details?id=_mfDBAAAQBAJ
47. Marsch LA. Leveraging technology to enhance addiction treatment and recovery. J Addict Dis. (2012) 31(3):313–8. doi: 10.1080/10550887.2012.694606
48. Marsch LA, Dallery J. Advances in the psychosocial treatment of addiction: the role of technology in the delivery of evidence-based psychosocial treatment. Psychiatr Clin North Am. (2012) 35(2):481–93. doi: 10.1016/j.psc.2012.03.009
49. Marsch LA, Gustafson DH. The role of technology in health care innovation: a commentary. J Dual Diagn. (2013) 9(1):101–3. doi: 10.1080/15504263.2012.750105
50. Krebs P, Prochaska JO, Rossi JS. A meta-analysis of computer-tailored interventions for health behavior change. Prev Med. (2010) 51(3–4):214–21. doi: 10.1016/j.ypmed.2010.06.004
51. Nielsen L, Riddle M, King JW, NIH Science of Behavior Change Implementation Team, Aklin WM, Chen W, et al. The NIH science of behavior change program: transforming the science through a focus on mechanisms of change. Behav Res Ther. (2018) 101:3–11. doi: 10.1016/j.brat.2017.07.002
52. Scherer EA, Kim SJ, Metcalf SA, Sweeney MA, Wu J, Xie H, et al. Momentary self-regulation: scale development and preliminary validation. JMIR Ment Health. (2022) 9(5):e35273. doi: 10.2196/35273
53. Sverdlov O, van Dam J. Digital Therapeutics: Strategic, Scientific, Developmental, and Regulatory Aspects. New York, NY: CRC Press (2022). p. 462. Available online at: https://play.google.com/store/books/details?id=uPeJEAAAQBAJ
54. Acosta MC, Possemato K, Maisto SA, Marsch LA, Barrie K, Lantinga L, et al. Web-delivered CBT reduces heavy drinking in OEF-OIF veterans in primary care with symptomatic substance use and PTSD. Behav Ther. (2017) 48(2):262–76. doi: 10.1016/j.beth.2016.09.001
55. Dallery J, Raiff BR, Kim SJ, Marsch LA, Stitzer M, Grabinski MJ. Nationwide access to an internet-based contingency management intervention to promote smoking cessation: a randomized controlled trial. Addiction. (2017) 112(5):875–83. doi: 10.1111/add.13715
56. Harris PA, Taylor R, Thielke R, Payne J, Gonzalez N, Conde JG. Research electronic data capture (REDCap)–a metadata-driven methodology and workflow process for providing translational research informatics support. J Biomed Inform. (2009) 42(2):377–81. doi: 10.1016/j.jbi.2008.08.010
57. Harris PA, Taylor R, Minor BL, Elliott V, Fernandez M, O’Neal L, et al. The REDCap consortium: building an international community of software platform partners. J Biomed Inform. (2019) 95(103208). doi: 10.1016/j.jbi.2019.103208
58. Square2 - Who we Are. Available online at: https://www.square2.co/about.html (cited 2024 Jun 12).
59. Yanovski SZ, Marcus MD, Wadden TA, Walsh BT. The questionnaire on eating and weight patterns-5: an updated screening instrument for binge eating disorder. Int J Eat Disord. (2015) 48(3):259–61. doi: 10.1002/eat.22372
60. Heatherton TF, Kozlowski LT, Frecker RC, Fagerström KO. The Fagerström test for nicotine dependence: a revision of the Fagerström tolerance questionnaire. Br J Addict. (1991) 86(9):1119–27. doi: 10.1111/j.1360-0443.1991.tb01879.x
61. Lyons EJ, Lewis ZH, Mayrsohn BG, Rowland JL. Behavior change techniques implemented in electronic lifestyle activity monitors: a systematic content analysis. J Med Internet Res. (2014) 16(8):e192. doi: 10.2196/jmir.3469
62. Wong HY, Subramaniyan M, Bullen C, Amer Siddiq AN, Danaee M, Yee A. The mobile-phone-based iCOTM Smokerlyzer®: comparison with the piCO+ Smokerlyzer® among smokers undergoing methadone-maintained therapy. Tob Induc Dis. (2019) 17:65. doi: 10.18332/tid/111355
63. Benowitz NL, Bernert JT, Foulds J, Hecht SS, Jacob P, Jarvis MJ, et al. Biochemical verification of tobacco use and abstinence: 2019 update. Nicotine Tob Res. (2020) 22(7):1086–97. doi: 10.1093/ntr/ntz132
64. Crowley TJ, MacDonald MJ, Zerbe GO, Petty TL. Reinforcing breath carbon monoxide reductions in chronic obstructive pulmonary disease. Drug Alcohol Depend. (1991) 29(1):47–62. doi: 10.1016/0376-8716(91)90021-P
65. Middleton ET, Morice AH. Breath carbon monoxide as an indication of smoking habit. Chest. (2000) 117(3):758–63. doi: 10.1378/chest.117.3.758
66. Meredith SE, Grabinski MJ, Dallery J. Internet-based group contingency management to promote abstinence from cigarette smoking: a feasibility study. Drug Alcohol Depend. (2011) 118(1):23–30. doi: 10.1016/j.drugalcdep.2011.02.012
67. Dallery J, Raiff BR, Grabinski MJ. Internet-based contingency management to promote smoking cessation: a randomized controlled study. J Appl Behav Anal. (2013) 46(4):750–64. doi: 10.1002/jaba.89
68. Dallery J, Stinson L, Bolívar H, Modave F, Salloum RG, Viramontes TM, et al. Mmotiv8: a smartphone-based contingency management intervention to promote smoking cessation. J Appl Behav Anal. (2021) 54(1):38–53. doi: 10.1002/jaba.800
69. Adolf J, Schuurman NK, Borkenau P, Borsboom D, Dolan CV. Measurement invariance within and between individuals: a distinct problem in testing the equivalence of intra- and inter-individual model structures. Front Psychol. (2014) 5:883. doi: 10.3389/fpsyg.2014.00883
70. Widaman KF, Ferrer E, Conger RD. Factorial invariance within longitudinal structural equation models: measuring the same construct across time. Child Dev Perspect. (2010) 4(1):10–8. doi: 10.1111/j.1750-8606.2009.00110.x
71. Borsboom D, Mellenbergh GJ. Test validity in cognitive assessment. In: Leighton JP, editor. Cognitive Diagnostic Assessment for Education: Theory and Applications. New York, NY, US: Cambridge University Press (2007). p. 85–115, viii. Available online at: https://psycnet.apa.org/fulltext/2007-14745-004.pdf
72. Millsap RE. Statistical Approaches to Measurement Invariance. London, England: Routledge (2012). p. 368. Available online at: https://www.taylorfrancis.com/books/mono/10.4324/9780203821961/statistical-approaches-measurement-invariance-roger-millsap
73. Asparouhov T, Muthén B. Residual associations in latent class and latent transition analysis. Struct Equ Modeling. (2015) 22(2):169–77. doi: 10.1080/10705511.2014.935844
74. McNeish D, Mackinnon DP, Marsch LA, Poldrack RA. Measurement in intensive longitudinal data. Struct Equ Modeling. (2021) 28(5):807–22. doi: 10.1080/10705511.2021.1915788
75. De Jong MG, Steenkamp JBEM, Fox JP. Relaxing measurement invariance in cross-national consumer research using a hierarchical IRT model. J Consum Res. (2007) 34(2):260–78. doi: 10.1086/518532
76. Wirth RJ, Edwards MC. Item factor analysis: current approaches and future directions. Psychol Methods. (2007) 12(1):58–79. doi: 10.1037/1082-989X.12.1.58
77. Muthen LK, Muthen B. Mplus Version 8 User’s Guide. Los Angeles, CA: Muthen & Muthen (2017). p. 944. Available online at: https://play.google.com/store/books/details?id=dgDlAQAACAAJ
78. McNeish D, MacKinnon DP. Intensive longitudinal mediation in mplus. Psychol Methods. (2022). doi: 10.1037/met0000536
79. McNeish D, Hamaker EL. A primer on two-level dynamic structural equation models for intensive longitudinal data in Mplus. Psychol Methods. (2020) 25(5):610–35. doi: 10.1037/met0000250
80. Hamaker EL, Grasman RPPP. Regime switching state-space models applied to psychological processes: handling missing data and making inferences. Psychometrika. (2012) 77(2):400–22. doi: 10.1007/s11336-012-9254-8
81. McNeish D, Somers JA, Savord A. Dynamic structural equation models with binary and ordinal outcomes in mplus. Behav Res Methods. (2024) 56(3):1506–32. doi: 10.3758/s13428-023-02107-3
82. Dingemans A, Danner U, Parks M. Emotion regulation in binge eating disorder: a review. Nutrients. (2017) 9(11):1274. doi: 10.3390/nu9111274
83. Morgenstern J, Naqvi NH, Debellis R, Breiter HC. The contributions of cognitive neuroscience and neuroimaging to understanding mechanisms of behavior change in addiction. Psychol Addict Behav. (2013) 27(2):336–50. doi: 10.1037/a0032435
84. Baron RM, Kenny DA. The moderator-mediator variable distinction in social psychological research: conceptual, strategic, and statistical considerations. J Pers Soc Psychol. (1986) 51(6): 1173–82. doi: 10.1037//0022-3514.51.6.1173
85. Carlo AD, Hosseini Ghomi R, Renn BN, Areán PA. By the numbers: ratings and utilization of behavioral health mobile applications. NPJ Digit Med. (2019) 2:54. doi: 10.1038/s41746-019-0129-6
86. DiMasi JA, Feldman L, Seckler A, Wilson A. Trends in risks associated with new drug development: success rates for investigational drugs. Clin Pharmacol Ther. (2010) 87(3):272–7. doi: 10.1038/clpt.2009.295
87. Mohs RC, Greig NH. Drug discovery and development: role of basic biological research. Alzheimers Dement. (2017) 3(4):651–7. doi: 10.1016/j.trci.2017.10.005
88. Nardi WR, Loucks EB, Springs S, Operario D, Kronish IM, Gaudiano BA, et al. Mindfulness-based interventions for medication adherence: a systematic review and narrative synthesis. J Psychosom Res. (2021) 149:110585. doi: 10.1016/j.jpsychores.2021.110585
89. Sha L, Yang X, Deng R, Wang W, Tao Y, Cao H, et al. Automated digital interventions and smoking cessation: systematic review and meta-analysis relating efficiency to a psychological theory of intervention perspective. J Med Internet Res. (2022) 24(11):e38206. doi: 10.2196/38206
90. Ahmadiankalati M, Steins-Loeber S, Paslakis G. Review of randomized controlled trials using e-health interventions for patients with eating disorders. Front Psychiatry. (2020) 11:568. doi: 10.3389/fpsyt.2020.00568
91. Wilson GT, Wilfley DE, Agras WS, Bryson SW. Psychological treatments of binge eating disorder. Arch Gen Psychiatry. (2010) 67(1):94–101. doi: 10.1001/archgenpsychiatry.2009.170
92. Aubin HJ, Luquiens A, Berlin I. Pharmacotherapy for smoking cessation: pharmacological principles and clinical practice. Br J Clin Pharmacol. (2014) 77(2):324–36. doi: 10.1111/bcp.12116
93. Evins AE, Cather C, Pratt SA, Pachas GN, Hoeppner SS, Goff DC, et al. Maintenance treatment with varenicline for smoking cessation in patients with schizophrenia and bipolar disorder: a randomized clinical trial. JAMA. (2014) 311(2):145–54. doi: 10.1001/jama.2013.285113
94. Cather C, Pachas GN, Cieslak KM, Evins AE. Achieving smoking cessation in individuals with schizophrenia: special considerations. CNS Drugs. (2017) 31(6):471–81. doi: 10.1007/s40263-017-0438-8
95. Hartmann-Boyce J, Hong B, Livingstone-Banks J, Wheat H, Fanshawe TR. Additional behavioural support as an adjunct to pharmacotherapy for smoking cessation. Cochrane Database Syst Rev. (2019) 6(6):CD009670. doi: 10.1002/14651858.CD009670.pub4
96. Patnode CD, Henderson JT, Coppola EL, Melnikow J, Durbin S, Thomas RG. Interventions for tobacco cessation in adults, including pregnant persons: updated evidence report and systematic review for the US preventive services task force. JAMA. (2021) 325(3):280–98. doi: 10.1001/jama.2020.23541
97. Stead LF, Koilpillai P, Fanshawe TR, Lancaster T. Combined pharmacotherapy and behavioural interventions for smoking cessation. Cochrane Database Syst Rev. (2016) 3(3):CD008286. doi: 10.1002/14651858.CD008286.pub3
98. Kabat-Zinn J, Hanh TN. Full Catastrophe Living: Using the Wisdom of Your Body and Mind to Face Stress, Pain, and Illness. New York, NY: Random House Publishing Group (2009). p. 512. Available online at: https://play.google.com/store/books/details?id=TVsrK0sjGiUC
99. Bishop SR, Lau M, Shapiro S, Carlson L, Anderson ND, Carmody J, et al. Mindfulness: a proposed operational definition. Clin Psychol Sci Pract. (2004) 11(3):230–41. doi: 10.1093/clipsy.bph077
100. Scherer G. Smoking behaviour and compensation: a review of the literature. Psychopharmacology. (1999) 145(1):1–20. doi: 10.1007/s002130051027
101. Strasser AA, Lerman C, Sanborn PM, Pickworth WB, Feldman EA. New lower nicotine cigarettes can produce compensatory smoking and increased carbon monoxide exposure. Drug Alcohol Depend. (2007) 86(2–3):294–300. doi: 10.1016/j.drugalcdep.2006.06.017
102. Chambers R, Gullone E, Allen NB. Mindful emotion regulation: an integrative review. Clin Psychol Rev. (2009) 29(6):560–72. doi: 10.1016/j.cpr.2009.06.005
103. Farb NAS, Anderson AK, Segal ZV. The mindful brain and emotion regulation in mood disorders. Can J Psychiatry. (2012) 57(2):70–7. doi: 10.1177/070674371205700203
104. Chiesa A, Serretti A, Jakobsen JC. Mindfulness: top-down or bottom-up emotion regulation strategy? Clin Psychol Rev. (2013) 33(1):82–96. doi: 10.1016/j.cpr.2012.10.006
105. Grecucci A, Pappaianni E, Siugzdaite R, Theuninck A, Job R. Mindful emotion regulation: exploring the neurocognitive mechanisms behind mindfulness. Biomed Res Int. (2015) 2015:670724. doi: 10.1155/2015/670724
106. Lutz J, Herwig U, Opialla S, Hittmeyer A, Jäncke L, Rufer M, et al. Mindfulness and emotion regulation—an fMRI study. Soc Cogn Affect Neurosci. (2013) 9(6):776–85. doi: 10.1093/scan/nst043
107. Taylor VA, Grant J, Daneault V, Scavone G, Breton E, Roffe-Vidal S, et al. Impact of mindfulness on the neural responses to emotional pictures in experienced and beginner meditators. Neuroimage. (2011) 57(4):1524–33. doi: 10.1016/j.neuroimage.2011.06.001
108. Projects. Available online at: https://scienceofbehaviorchange.org/projects/?test=true&category=uh3 (cited 2024 May 10).
109. Stoeckel LE, Hunter C, Onken L, Green P, Nielsen L, Aklin WM, et al. The NIH science of behavior change program: looking toward the future. Behav Ther. (2023) 54(4):714–8. doi: 10.1016/j.beth.2023.03.006
110. Trifan A, Oliveira M, Oliveira JL. Passive sensing of health outcomes through smartphones: systematic review of current solutions and possible limitations. JMIR Mhealth Uhealth. (2019) 7(8):e12649. doi: 10.2196/12649
111. Sheikh M, Qassem M, Kyriacou PA. Wearable, environmental, and smartphone-based passive sensing for mental health monitoring. Front Digit Health. (2021) 3:662811. doi: 10.3389/fdgth.2021.662811
112. Cornet VP, Holden RJ. Systematic review of smartphone-based passive sensing for health and wellbeing. J Biomed Inform. (2018) 77:120–32. doi: 10.1016/j.jbi.2017.12.008
Keywords: digital therapeutic, momentary self-regulation, health risk behavior, smoking, binge eating disorder, obesity, ecological momentary assessment (EMA)
Citation: Plaitano EG, McNeish D, Bartels SM, Bell K, Dallery J, Grabinski M, Kiernan M, Lavoie HA, Lemley SM, Lowe MR, MacKinnon DP, Metcalf SA, Onken L, Prochaska JJ, Sand CL, Scherer EA, Stoeckel LE, Xie H and Marsch LA (2025) Adherence to a digital therapeutic mediates the relationship between momentary self-regulation and health risk behaviors. Front. Digit. Health 7:1467772. doi: 10.3389/fdgth.2025.1467772
Received: 20 July 2024; Accepted: 16 January 2025;
Published: 4 February 2025.
Edited by:
Carla Tatiana Toro, University of Warwick, United KingdomReviewed by:
Larry R Price, Texas State University, United StatesLindsey Potter, The University of Utah, United States
Copyright: © 2025 Plaitano, McNeish, Bartels, Bell, Dallery, Grabinski, Kiernan, Lavoie, Lemley, Lowe, MacKinnon, Metcalf, Onken, Prochaska, Sand, Scherer, Stoeckel, Xie and Marsch. This is an open-access article distributed under the terms of the Creative Commons Attribution License (CC BY). The use, distribution or reproduction in other forums is permitted, provided the original author(s) and the copyright owner(s) are credited and that the original publication in this journal is cited, in accordance with accepted academic practice. No use, distribution or reproduction is permitted which does not comply with these terms.
*Correspondence: Enzo G. Plaitano, ZW56by5nLnBsYWl0YW5vLkdSQGRhcnRtb3V0aC5lZHU=
†ORCID:
Enzo G. Plaitano
orcid.org/0000-0001-9701-8925