- 1Clinical, Neuro-, and Developmental Psychology, Vrije Universiteit Amsterdam, Amsterdam, Netherlands
- 2Amsterdam Public Health, Mental Health, Amsterdam, Netherlands
- 3Department of Medical Psychology, Amsterdam UMC Location University of Amsterdam, Amsterdam, Netherlands
- 4Unit of Cancer Survivorship, German Cancer Research Center (DKFZ), Heidelberg, Germany
- 5Department of Psychiatry, Amsterdam UMC, Amsterdam, Netherlands
- 6Center for Economic and Social Research, University of California, Los Angeles, CA, United States (emeritus)
- 7Department of Psychiatry, Interdisciplinary Center Psychopathology and Emotion Regulation, University Medical Center Groningen, University of Groningen, Groningen, Netherlands
- 8Department of Psychology, Ludwig Maximilian University of Munich, Munich, Germany
- 9German Center for Mental Health (DZPG), Partner Site Munich-Augsburg, Munich, Germany
Background: Just-In-Time Adaptive Interventions (JITAIs) are interventions designed to deliver timely tailored support by adjusting to changes in users' internal states and external contexts. To accomplish this, JITAIs often apply complex analytic techniques, such as machine learning or Bayesian algorithms to real- or near-time data acquired from smartphones and other sensors. Given the idiosyncratic, dynamic, and context dependent nature of mental health symptoms, JITAIs hold promise for mental health. However, the development of JITAIs is still in the early stages and is complex due to the multifactorial nature of JITAIs. Considering this complexity, Nahum-Shani et al. developed a conceptual framework for developing and testing JITAIs for health-related problems. This review evaluates the current state of JITAIs in the field of mental health including their alignment with Nahum-Shani et al.'s framework.
Methods: Nine databases were systematically searched in August 2023. Protocol or empirical studies self-identifying their intervention as a “JITAI” targeting mental health were included in the qualitative synthesis if they were published in peer-reviewed journals and written in English.
Results: Of the 1,419 records initially screened, 9 papers reporting on 5 JITAIs were included (sample size range: 5 to an expected 264). Two JITAIs were for bulimia nervosa, one for depression, one for insomnia, and one for maternal prenatal stress. Although most core components of Nahum-Shani's et al.'s framework were incorporated in the JITAIs, essential elements (e.g., adaptivity and receptivity) within the core components were missing and the core components were only partly substantiated by empirical evidence (e.g., interventions were supported, but the decision rules and points were not). Complex analytical techniques such as data from passive monitoring of individuals' states and contexts were hardly used. Regarding the current state of studies, initial findings on usability, feasibility, and effectiveness appear positive.
Conclusions: JITAIs for mental health are still in their early stages of development, with opportunities for improvement in both development and testing. For future development, it is recommended that developers utilize complex analytical techniques that can handle real-or near-time data such as machine learning, passive monitoring, and conduct further research into empirical-based decision rules and points for optimization in terms of enhanced effectiveness and user-engagement.
1 Introduction
Over the past two decades, a variety of digital interventions have been developed, evaluated, and implemented for the prevention and treatment of a range of mental health disorders, including depression, anxiety and substance use disorders (1–7). The diverse range of digital interventions include standalone guided and unguided, as well as blended (i.e., integration of digital and face-to-face components into one treatment protocol) or add-on formats, with treatments often based on cognitive behavioral therapy (CBT) (1–7). Digital interventions have demonstrated potential in improving clients' self-management skills, enhancing access to psychological interventions, and expanding mental health care at relatively lower costs (1, 2, 4) while achieving comparable clinical outcomes compared to traditional face-to-face interventions [e.g., (2, 7–10)]. While digital interventions were initially concentrated on internet-based platforms, in the past decade, the development, large ownership, and intensive use of smartphone functionalities have made it possible to deliver interventions via smartphones (11–14). Smartphone interventions enhance monitoring capabilities and enable real-time prevention and treatment options (4). Reviews indicate that smartphone interventions are potentially acceptable and effective across various mental health conditions, such as depression and anxiety (14–19).
A novel form of smartphone intervention is the Just-In-Time Adaptive Intervention (JITAI). JITAIs automatically offer support and dynamically adjust their type, content, timing, and intensity based on an individual's changing status and setting, and are ideally only initiated when needed (20, 21). Measuring individual's changing status and setting is often conducted using “ecological momentary assessment” (EMA) methods (22–27). EMA captures momentary symptoms, emotions, behaviors, and context, providing insights that are difficult to achieve through retrospective methods (22–27). They can be used for clinical and epidemiological research as well as in clinical practices, and EMA data can be collected through both active and passive methods (22, 24). In active EMA, users self-monitor their symptoms and related factors (e.g., social contacts and context) by completing short questionnaires, typically several times a day depending on the mood/behavior under study (22–24). Passive EMA refers to monitoring without active engagement of the user, such as counting the number of steps or geolocations, using deployable sensors or sensors in smartphones or smartwatches (22, 28). This data can inform decision-making about when, if, and which intervention should be offered (29). In making these decisions, various complex analytical techniques such as machine learning can be employed to classify the individual's state and context (20, 21, 30). These can range from simple if-then rules and decision trees over more complex supervised algorithms, such as support vector machines (SVM) to end-to-end learning models and large multimodal models (20, 21, 29–34). To date, investigations into JITAIs have primarily focused on addressing health challenges such as physical inactivity (35), obesity (36), smoking (37), and substance abuse (38). For example, HeartSteps, a JITAI designed to promote physical activity (39, 40). When passive EMA and a machine learning algorithm detect sedentary behavior, HeartSteps sends push notifications with interventions aimed at increasing physical activity. The machine learning algorithm determines the appropriate intervention based on various conditions. For instance, on weekends when individuals are at home and the weather is good, a suggested activity might be walking 500–1,000 steps. While during weekday work hours or bad weather conditions, a suggested activity might be standing up (39, 40). Meta-analytic findings demonstrate moderate to large effect sizes of JITAIs on distal outcomes such as weight loss and smoking cessation when compared to no-treatment control conditions and non-JITAI treatments (41).
JITAIs for mental health are still in their early stages. However, they are perceived as a promising future direction in the field of mental health (12, 13, 42). One reason is that a substantial body of research indicates that mental health symptoms are idiosyncratic (i.e., differ between individuals), dynamic (i.e., fluctuate over time), and multi-factorial (i.e., driven by multiple variables, such as setting, time of day, and personal characteristics) (26, 43–46). As a result, different individuals with mental health problems require personalized types of support in real-life situations, and the same individual may also need different forms of support at different moments in time (11, 47). JITAIs seem suitable for intervening in mental health symptoms, given that these interventions are based on individual characteristics and changing contexts and states within individuals.
Developing JITAIs, however, is a difficult task since JITAIs are considered complex interventions due to the multifactorial nature of (mental) health-related problems and intervention components (20, 21). Many decisions, ideally theory- and/or empirically-based, must be made during development about when to intervene, when to abstain from intervention, which interventions should be delivered to whom, and in which specific settings these modules can best be delivered. These choices include designing the right interventions, determining the factors on which to base these decisions, and deciding which methods will be used to measure and analyze the outcomes (20, 21). To support JITAI development, Nahum-Shani et al. (20, 21) introduced a conceptual framework designed to aid JITAI development for health-related problems by systematically considering core components and guide subsequent empirical work. This framework comprises six core components. (1) Distal outcome represents the long-term goal of the intervention, typically the primary clinical outcome. (2) Proximal outcome refers to short-term goals the intervention aims to achieve, often serving as mediators through which the interventions impact the distal outcome. (3) Intervention options refer to the array of possible interventions that can be employed to achieve the proximal outcome. (4) Tailoring variables pertain to information used to determine when and how to intervene for each individual. This includes “vulnerability/opportunity” and “receptivity”. Vulnerability refers to experiencing adverse health outcomes or engaging in maladaptive behaviors. For example, in a JITAI addressing depression, perhaps the best time to provide an intervention is when an increase in depressed mood is detected. However, on occasions where a vulnerable state may not be ideal for intervening (e.g., during stress), it may be better for a JITAI to adapt to states of “opportunity”. Receptivity pertains to the user's availability to receive and engage with the provided intervention effectively. For instance, for a JITAI for depression, this might be the time when the user is at home, as engaging with the intervention requires time and space. Often, information about vulnerability and receptivity is gathered through EMA methods and then analyzed using machine learning or other types of algorithms in JITAIs. Ideally, the algorithms used have the capacity to “learn” when a person is most receptive to a particular intervention. (5) Decision points are points in time at which an intervention may be delivered. (6) Decision rules, which are rules specifying which intervention to offer, to whom, and when. These rules systematically link decision points, tailoring variables, and intervention options. Nahum-Shani et al. emphasize that the six core components need to be substantiated based on behavioral change theory (20, 21).
The goal of this systematic review is to investigate the current state of JITAIs for mental health, and to assess the extent to which the identified JITAIs for mental health align with the Nahum-Shani et al. framework (20, 21). The secondary objective is to describe the studies on JITAIs for mental health in terms of their characteristics, aims, and outcomes. Furthermore, the review also aims to identify potential knowledge gaps. Through this, insights to guide the future development of JITAIs for mental health are drawn.
2 Methods
2.1 Overview, eligibility criteria, information sources and search
The protocol was registered with the Open Science Framework on 23 February 2023 (https://osf.io/4u27c/c). The review follows the updated PRISMA 2020 guidelines (48).
Studies were included if (1) the focus was on JITAIs interventions, only studies with interventions that were self-identified as “JITAIs” were included, (2) the intervention was targeted at (subclinical) mental disorders, (3) it concerned a protocol or empirical study, (4) it was published in a peer-reviewed journal, (5) written in English.
To identify relevant articles, a specialist librarian searched the following nine databases from inception until 01 Aug 2023: PubMed, Embase, APA PsycInfo, Cinahl, Web of Science, Cochrane CENTRAL, ACM Digital Library, DBLP, and Scopus. The search string is shown in Supplementary Table 1. The reference lists of relevant studies were checked for potentially eligible studies by two reviewers (AS, CvG). In PubMed, the following query was used: “JITAI” [tiab] OR “JITAIs” [tiab] OR “just-in-time adaptive intervention*” [tiab] OR [“just-in-time” (tiab) AND adaptive (tiab)] OR “momentary intervention*” [tiab]. To keep the terms as broad as possible and ensure no studies were missed due to overly strict criteria, no terms related to mental health were added. For the other databases, similar queries were used (see “Supplementary Table 1 Search Queries”).
2.2 Study selection
To identify papers that potentially met the eligibility criteria, titles and abstracts were screened independently by two reviewers (MT, CvG). The full text of relevant studies identified after the title and abstract screening were reviewed by two reviewers (AS or MT, CvG) to assess inclusion eligibility. Disagreements on study selection were resolved by mutual consensus or during discussion with the project principal investigator (HR), if needed.
2.3 Data extraction and synthesis
After inclusion of eligible studies, relevant data was extracted using data charting forms. First, general information was extracted, that is authors, publication year, country, and target population. Then, for the primary objective, which is the extent to which the identified JITAIs align with Nahum-Shani et al.'s framework (20, 21), the operationalization of core JITAI components based on Nahum-Shani et al.'s framework (20, 21) (i.e., distal outcome, proximal outcome, intervention options, tailoring variables, decision points, decision rules) was extracted. Subsequently, for the secondary aim, which is to describe the studies in terms of their characteristics and outcomes, information about the study aim, target population, study design, control group, sample size, recruitment and inclusion criteria, primary outcome, and main findings was extracted.
Two researchers (AS or MT, CvG) independently extracted and charted the data, discussed the results of the included studies, and updated the data charting forms in an iterative process. Disagreements between the two researchers were resolved by discussion with the project principal investigator (HR), if needed.
3 Results
3.1 Study selection and general study characteristics
The search resulted in 3,362 articles, 1,419 after duplicate removal. The flowchart of the inclusion process following PRISMA guidelines is presented in Figure 1. 1,402 articles were excluded based on title and abstract screening. Of the remaining 17 articles, 8 were excluded after full text screening, leaving 9 articles for inclusion. The studies were published between 2018 and 2023.
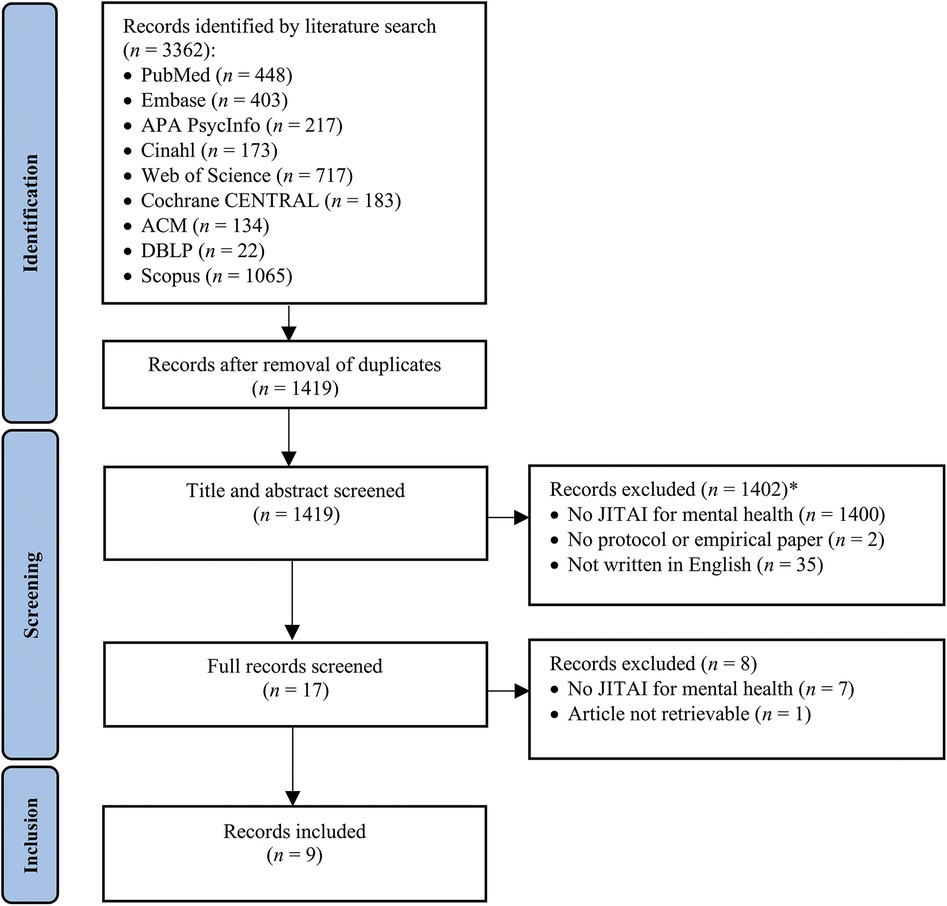
Figure 1. PRISMA flowchart of the study selection process. *35 papers neither had a focus on JITAI nor were written in English.
Five papers targeted Bulimia Nervosa Spectrum Disorders (BNSD) (49–53). Four of these discussed the “CBT+” JITAI intervention (49–52), while the fifth described the “SenseSupport” JITAI (53). Both JITAIs were delivered alongside standard weekly CBT face-to-face sessions. One paper focused on depression and introduced a 3-week stand-alone mobile rumination-focused CBT known as “JITAI-MRFCBT” to address depressive rumination (54). Two papers focused on insomnia and described the guided “iREST” JITAI, aimed at addressing clinical sleep disturbances in military personnel (55, 56). Finally, one paper focused on maternal prenatal stress and introduced “Wellness-4-2”, a JITAI delivered alongside a validated 12-session face-to-face prenatal maternal stress reduction course (57).
3.2 JITAI development: operationalization of JITAIs
The operationalization of the JITAIs according to the core components of Nahum-Shani et al.'s framework (20, 21) is described in Table 1. For a detailed description per JITAI, please refer to “Supplementary Material 1 JITAI development: Operationalization of JITAIs”. Below is a summary at an aggregated level. In total, five distinct JITAIs were described in nine papers.
3.2.1 Distal outcomes
Concerning distal outcomes, Nahum-Shani et al.'s framework (20, 21) was adhered to across all JITAIs (49–57). The outcomes represented clinical outcomes, mostly derived from the treatment outcome of face-to-face CBT, specific for the mental health disorder targeted. For instance, within the target population of military personnel experiencing clinically significant sleep disturbances, insomnia served as the distal outcome (55, 56).
3.2.2 Proximal outcomes
For the four JITAIs that specified the proximal outcomes (49–54, 57), the selection of these outcomes largely adhered to Nahum-Shani et al.'s (20, 21). The proximal outcomes were theoretically justified based on non-JITAI (mainly face-to-face CBT) interventions, with the treatment mechanisms of these non-JITAI interventions considered as the JITAI's proximal outcomes. For instance, in face-to-face CBT for depressive rumination, treatment is focused on disrupting ruminative thoughts in the short term since this leads to a reduction in these thoughts over the long term (58). In the JITAI-MRFCBT, disrupting ruminative thoughts in the short term was the proximal outcome (54). Only CBT+'s proximal outcome was adaptive between and within users, with the user's proximal outcome changing weekly (49–52). Adaptivity was not reported in the other JITAIs (53, 54, 57).
3.2.3 Intervention options
Most interventions adhered to this aspect of Nahum-Shani et al.'s framework (20, 21). Each intervention was primarily based on face-to-face CBT with an emphasis on behavioral change. Different interventions were sent to and among participants based on tailored variables (49–57). Four out of the five JITAIs linked the positive impact of interventions to a positive impact on the proximal outcome (49, 51–54, 57). The exception was iREST, which linked their interventions to a positive impact on the distal outcome (55, 56). The interventions in all the five JITAIs were adaptive as they were adjusted both between and within users over time (49–57).
3.2.4 Tailoring variables
For the operationalization of the tailoring variables, some aspects of Nahum-Shani et al.'s framework (20, 21) were adhered to, but essential elements within the component were missing. Diverse methods were utilized for (self)monitoring, with active EMA in four JITAIs (49–52, 54–57), passive EMA in two (53, 57) sometimes supplemented with other sources such as weekly retrospective monitoring or clinician involvement (49, 51, 55, 56). Most choices for the tailoring variables were based on face-to-face CBT. However, the tailoring variables were not adaptive between and within users over time. Moreover, only JITAI-MFRCBT assessed both states of vulnerability and receptivity (54). In the other four JITAIs, only vulnerability was considered (49–53, 55).
3.2.5 Decision points
The decision points were specified in four out of five JITAIs and Nahum-Shani et al.'s framework (20, 21) was partly followed (49–56). SenseSupport's decision point occurred every five minutes, so the likelihood of missing a user's moment of need for an intervention was low (53). However, in the other JITAIs, there was a longer interval between decision points (49–52, 54–56). In two JITAIs, the decision points occurred after the active EMAs were completed (49–52, 54), and with iREST, adjustments were made only after the clinician had approved the intervention (55, 56). The decision points were not adaptive between and within users over time. Since this frequency was not based on theory, it cannot be determined whether the timing of interventions (and thus the “in-time” concept) is accurate or if crucial moments were missed (20, 21).
3.2.6 Decision rules
Regarding the decision rules, Nahum-Shani et al.'s framework (20, 21) was partially followed. All JITAIs employed machine learning algorithms for decision rules (49–57). Four used decision trees of varying complexity (49–52, 54–57), while SenseSupport utilized a more complex algorithm (i.e., parameter invariant-algorithm) (53). CBT+ and iREST included online clinician involvement (49–52, 55, 56), which is not described in Nahum-Shani et al.'s framework (20, 21). As with most other components, decision rules were not adaptive between and within users over time (49–57).
3.3 Studies on JITAIs in mental health research
The general characteristics, aim of the study, target population, study design, control group, sample size, recruitment and inclusion criteria, primary outcome, and main findings of the nine conducted studies are described in Table 2. For detailed narrative descriptions per study, please refer to “Supplementary Material 2 Narrative descriptions of the included studies”. Below is a summary at an aggregated level.
Four papers reported on the usability and/or feasibility, with most findings revealing that users perceived the JITAIs as feasible and usable (49, 51, 53, 55). Feasibility was evaluated either by study retention rates or app usage data. Three of the four papers assessed the usability of the JITAI with rating scales that were based on the Technology Acceptance Model, and one study used the System Usability Scale and a modified Telerehabilitation Usability Questionnaire. In addition, qualitative interviews or feedback were used in all studies to assess usability. Five papers reported a change in distal outcomes from pre-to-post-test in small pilot (RCT) studies, showing within-person decreased symptomatology from pre-to-post intervention (49, 51, 54–56). In three papers, comparisons were made between the JITAI groups and control groups (51, 54, 56), of which two were RCTs and in one paper the control group consisted of data extracted from previously published trials on face-to-face CBT for insomnia. These studies found that JITAIs led to a greater reduction in symptoms when compared to a non-active control group (54), but no difference in reduction in symptoms was found when compared to an active control group (51, 56). In two studies (38, 40), attention was also given to subgroups, examining whether JITAIs (e.g., offering a JITAI vs. not offering a JITAI) moderated treatment outcomes for specific subgroups based on BNSD symptom profiles (50, 52). Last, two papers were protocols of RCTs, that started recruiting in 2023.
4 Discussion
This review identified nine papers describing a total of five distinct JITAIs for mental health, targeting BSND, insomnia, depressive rumination, and maternal prenatal stress. Regarding the first aim, three out of the five JITAIs described all six core components of Nahum-Shani et al.'s framework (20, 21), while two JITAIs lacked one core component (i.e., a proximal outcome or decision point). However, not all essential elements within the core components were incorporated (e.g., receptivity and adaptivity as part of the tailoring variables) and there is uncertainty regarding whether the interventions were delivered “in-time” or whether crucial moments were missed since the decision points were not theory-based but based on pragmatic choices (e.g., being able to ask users to complete a few EMAs a day). No components outside Nahum-Shani et al.'s framework (20, 21) were identified. Regarding the second aim, two papers were protocols of RCTs' that started recruiting in 2023. The other studies demonstrated that the JITAIs were generally usable, feasible, and led to a reduction in mental health symptoms compared to pre-intervention. In studies with a control group, the reduction was greater than in no-treatment control conditions but did not significantly differ from non-JITAI interventions.
4.1 Gaps of knowledge and recommendations for developing and testing JITAIs for mental health
Based on the comparison of the alignment of JITAIs in mental health with Nahum-Shani et al.'s framework (20, 21) and the status of the conducted studies, we identified gaps of knowledge and make several recommendations for future development and testing of JITAIs in mental health. This will aid in optimizing personalization, effectiveness, and user engagement in JITAIs (20, 21).
4.1.1 The use of passive EMA and (complex) machine learning techniques
Receptivity was only included in JITAI-MRFCBT, assessed through a daily questionnaire where users self-reported their expected windows of receptivity (43). JITAIs in other domains sometimes measure receptivity using passive EMA. For example, in the HeartSteps JITAI for physical activity and sedentary behavior, phone-based accelerometer data is analyzed by machine learning algorithms at each decision point to determine the user's receptivity by determining the user's current activity (39, 40). A benefit of using passive EMA to measure receptivity compared to active EMA is that it does not rely on users completing questionnaires, reducing user burden and minimizing the risk of missing data (20, 21, 59). Moreover, passive EMA has been shown to incrementally explain variance beyond active EMA in various outcomes (e.g., depression, anxiety, stress) (60).
Although it is possible to passively assess mood states or stress using a smartphone or a wearable device (61, 62), the measurement applications may not be validated compared to devices that track activity or sleep (e.g., accelerometry). Therefore, researchers who plan to include passive assessment of mood states need to be aware of this potential limitation. Currently, there are commercial (e.g., M-path or Movisens) and research platforms, some of which are open-source, available for passive sensing. A recently published guideline for e-health included recommendations for sensing research (63).
States of vulnerability were incorporated in the five JITAIs, but only one of five used passive EMA and complex machine learning techniques to measure and analyze states of vulnerability. Three systematic reviews demonstrated that when machine learning algorithms analyze passive data (e.g., call logs, social media use, or vocabulary of the text individuals write), future mental health states in both general and clinical populations could be predicted (61, 64, 65). The predictive accuracy of these models ranges from acceptable to very good (61, 64, 65). However, both reviews concluded that the field, despite advancing rapidly, is still in its early stages, with limited evidence supporting high-quality features for predicting mental states (61, 64, 65). In addition, the provision of adequate computational power and infrastructure required to accomplish the execution of machine-learning approaches in real-time remains a challenge. Furthermore, heterogeneity between studies is high, and assessment, modeling, and reporting procedures need to be critically reviewed (61, 66). This may explain why passive EMA and complex learning algorithms have been infrequently integrated into JITAIs for mental health until now. Finally, other complex analytic techniques including control systems engineering and Bayesian approaches, need to be developed to handle the enormous amount of dynamic data provided in JITAIs using sensors and smartphones (38, 61).
Another point of interest is that in three of the four JITAIs where decision points were specified, these decision points occurred after the user had completed an active EMA about the tailoring variable (49–52, 54–56). While active EMA can indicate vulnerability and receptivity to interventions at specific moments, its infrequent measurements throughout the day (typically only a few times) may miss critical moments (20, 21). This can compromise the “just-in-time” component of JITAI. Passive EMA analyzed on the fly using complex analytic techniques could reduce interval times between decision points, as seen in SenseSupport measuring glucose levels every five minutes (53), increasing the chance that interventions are delivered in-time. Nevertheless, there are potential therapeutic benefits of active EMA such as the empowerment of participants as they acquire knowledge about their personalized moods and activity dynamics. In turn, this knowledge may enable them to make informed behavioral changes, as well as improve communication with their therapist/health care provider. Based on EMA data, personalized feedback can be provided either by the therapist or via advanced algorithms.
Moreover, in the identified JITAIs, the proximal outcomes, tailoring variables, decision points, and decision rules were not adaptive over time and were uniform for all users (49–57). However, according to the conceptual framework, adaptivity across these core components is essential for JITAIs: this adaptivity ensures that the delivered interventions are tailored to meet the unique and changing needs of individuals, aiming to achieve the best possible outcome for each person (20, 21). Using complex machine learning algorithms, such as (deep) reinforcement learning enhanced adaptivity (19, 22, 23). This type of algorithm analyzes if an intervention prompts engagement and reduces the targeted proximal outcome (e.g., reduction in ruminative thoughts) (22, 23). By learning and adapting over time, these algorithms continuously improve the predictive power of the model (22, 23).
In conclusion, we recommend that JITAI developers in mental health adopt passive EMA more comprehensively. To effectively measure states of vulnerability and receptivity, developers should explore which features of passive data can serve as indicators for them. Utilizing passive EMA for measuring these states can also enhance the frequency of decision points, thereby facilitating timely intervention delivery. Passive and active EMA complement each other as well as more regular research instruments such as standardized retrospective questionnaires (60, 63). EMA enables more insightful gains in the personal dynamics of depressive symptoms as well as treatment outcome prediction. For EMA to work well, a substantial number of data points are required as well and sophisticated analytic methods are needed such as Bayesian models. In addition, complex analytic techniques such as machine learning or system identification could also be incorporated. Employing complex analytic techniques can further enable adaptivity of core components like decision points and rules across and within users. The validation of EMA and its analysis with machine learning models are still in development but making rapid improvements. Furthermore, there is still debate as to whether EMA measures different constructs than traditional measurements do. Recent publications provide recommendations for the incorporation of passive sensing and machine-learning based methods in health interventions (29, 63). Potential challenges with ethical, privacy, and security issues associated with mobile sensing should also be considered (29).
4.1.2 The necessity of human involvement and patient subgroups
The developers of CBT+ argued that for BNSD patients, treatment-as-usual should accompany a JITAI due to the severity and complexity of BNSD symptoms (42). Similarly, a position paper on JITAIs for suicide prevention recommended professional guidance for severe symptoms, suggesting that smartphone interventions may be suitable for low-risk individuals but not for those at high risk of suicidal behavior (12). The meta-analysis encompassing 33 studies on the efficacy of JITAIs across various health-related areas found that combining complex analytic techniques including machine learning with human involvement in the JITAIs produced more significant effects than relying solely on machine learning algorithms (41). It should therefore be investigated whether, and if so for whom, human guidance in for example the decision rules and interventions should be incorporated. Due to the varied nature of many mental health disorders, JITAIs might work differently for different subgroups of patients. It would therefore be interesting to investigate differences in the effectiveness of JITAIs within subgroups based on patient profiles, aligning with the developers of CBT+ interventions (50, 52).
4.1.3 The added value of JITAIs over existing interventions
Current studies show promising initial findings regarding usability, feasibility, and effectiveness (49, 51, 53–56). However, the number of studies conducted so far is limited and the conducted feasibility and (small-pilot) RCT studies do not allow robust meta-analytic conclusions (49, 51, 53–56). Additionally, longer follow-up periods and considerations for cost-effectiveness were lacking. Consequently, it is premature to draw definitive conclusions about the effectiveness of JITAIs and the added value of JITAIs over existing interventions in addressing mental health issues, a conclusion consistent with findings in other domains. For instance, a meta-analysis encompassing 33 studies across various health-related areas found moderate to large effect sizes for JITAIs compared to waitlist controls and non-JITAI interventions yet highlighted the general lack of statistical power in these studies (41). Similarly, reviews examining JITAIs for promoting physical activity (35) and reducing harmful substance use (38), consisting of 19 and 17 papers respectively, indicated that JITAIs were generally acceptable and showed some symptom reduction from pre- to post-intervention, but most studies lacked the statistical power to detect significant clinical effects.
None of the five included JITAIs used micro-randomized trials (MRT) in their development. It is advised to use innovative research methods such as the micro-randomized trial (MRT). An MRT is designed to investigate the effects of adaptive interventions in real-time and can complement the JITAI development (67, 68). This approach is not only recommended by Nahum-Shani et al. (20, 21), but is also recommended by the innovative Multiphase Optimization Strategy (MOST) framework (69). MOST is a novel framework developed to optimize interventions, including adaptive interventions. Another option is the use control optimization trials to optimize the individual and adaptive elements of interventions (70, 71). The advice is therefore that future studies, investigating the added value of JITAIs over existing interventions, be adequately powered and make use of innovative research methods.
4.1.4 Different labels for similar interventions
In the field of smartphone intervention developments, there are also JITAI-like developments where developers label their interventions differently. This means that it is possible that interventions, when evaluated against the framework of Nahum-Shani et al. (20, 21), could be considered JITAI but are labeled differently, such as “Ecological Momentary Interventions” (EMI) (15, 22) or micro-interventions (72). The use of different labels leads to confusion and hinders collaboration between developers and the synthesis of evidence. Therefore, we recommend the consistent use of shared terms and conceptual frameworks, which is a specific challenge due to the multidisciplinary nature of JITAI developments.
4.2 Strengths and limitations
This review has several limitations. Firstly, we only included studies that self-identified as “JITAIs”. This may have resulted in missing (smartphone) interventions that could be considered JITAIs according to the Nahum-Shani et al.'s framework (20, 21). Secondly, all studies we found were conducted in the United States of America. This raises questions about the extent to which the results can be generalized to other countries. In addition, no quality assessments of the included studies were conducted due to the heterogeneity of the study designs. Nevertheless, the review also offers several strengths, including providing an overview of a new and relevant field for the prevention and treatment of mental health symptoms, a pre-registered study protocol, adherence to international reporting guidelines, and an extensive search in nine databases.
4.3 Conclusion
It is concluded that JITAIs for mental health domain are still in their early stages, with opportunities for improvement in both development and testing. For the future, we recommend that developers explore the use of passive EMA and complex analytical techniques including but not limited to machine learning to measure and analyze states of receptivity and vulnerability and to enhance in-time and adaptive delivery of the JITAIs. Additionally, evaluating the potential added value of human involvement and investigating patient subgroups, conducting fully powered studies using innovative research methods such as MRTs and the MOST framework, and adopting a consistent use of the term “JITAI” are also recommended. This will aid in optimizing personalization, effectiveness, and user engagement in JITAIs.
Data availability statement
The original contributions presented in the study are included in the article/Supplementary Material, further inquiries can be directed to the corresponding author.
Author contributions
CG: Conceptualization, Formal Analysis, Methodology, Writing – original draft. MT: Conceptualization, Methodology, Writing – review & editing. WB: Writing – review & editing. AK: Writing – review & editing. DS-M: Writing – review & editing. AS: Conceptualization, Formal Analysis, Methodology, Writing – review & editing. MS: Conceptualization, Formal Analysis, Methodology, Writing – review & editing. YT: Writing – review & editing. HR: Conceptualization, Formal Analysis, Funding acquisition, Methodology, Supervision, Writing – review & editing.
Funding
The author(s) declare financial support was received for the research, authorship, and/or publication of this article. This study was funded by Amsterdam Public Health (APH) (grant no. 2021084/MdB/EdB).
Conflict of interest
The authors declare that the research was conducted in the absence of any commercial or financial relationships that could be construed as a potential conflict of interest.
The author(s) declared that they were an editorial board member of Frontiers, at the time of submission. This had no impact on the peer review process and the final decision.
Publisher's note
All claims expressed in this article are solely those of the authors and do not necessarily represent those of their affiliated organizations, or those of the publisher, the editors and the reviewers. Any product that may be evaluated in this article, or claim that may be made by its manufacturer, is not guaranteed or endorsed by the publisher.
Supplementary material
The Supplementary Material for this article can be found online at: https://www.frontiersin.org/articles/10.3389/fdgth.2025.1460167/full#supplementary-material
References
1. Riper H, Cuijpers PJ. Telepsychology and eHealth. In: Norcross JC, Freedheim DK, VandenBos GR, editors. APA handbook of Clinical Psychology: Applications and Methods (Vol. 3). Washington: American Psychological Association (2016). p. 451–63. doi: 10.1037/14861-024
2. Etzelmueller A, Vis C, Karyotaki E, Baumeister H, Titov N, Berking M, et al. Effects of internet-based cognitive behavioral therapy in routine care for adults in treatment for depression and anxiety: systematic review and meta-analysis. J Med Internet Res. (2020) 22:e18100. doi: 10.2196/18100
3. Erbe D, Psych D, Eichert HC, Riper H, Ebert DD. Blending face-to-face and internet-based interventions for the treatment of mental disorders in adults: systematic review. J Med Internet Res. (2017) 19:e306. doi: 10.2196/jmir.6588
4. Cuijpers P, Kleiboer A, Karyotaki E, Riper H. Internet and mobile interventions for depression: opportunities and challenges. Depress Anxiety. (2017) 34:596–602. doi: 10.1002/da.22641
5. Riper H, Hoogendoorn A, Cuijpers P, Karyotaki E, Boumparis N, Mira A, et al. Effectiveness and treatment moderators of internet interventions for adult problem drinking: an individual patient data meta-analysis of 19 randomised controlled trials. PLoS Med. (2018) 15:e1002714. doi: 10.1371/journal.pmed.1002714
6. Schulte MHJ, Boumparis N, Huizink AC, Riper H. Technological interventions for the treatment of substance use disorders. Compr Clin Psychol. (2022):264–82. doi: 10.1016/B978-0-12-818697-8.00010-8
7. Moshe I, Terhorst Y, Philippi P, Domhardt M, Cuijpers P, Cristea I, et al. Digital interventions for the treatment of depression: a meta-analytic review. Psychol Bull. (2021) 147:749–86. doi: 10.1037/bul0000334
8. Karyotaki E, Riper H, Twisk J, Hoogendoorn A, Kleiboer A, Mira A, et al. Efficacy of self-guided internet-based cognitive behavioral therapy in the treatment of depressive symptoms a meta-analysis of individual participant data. JAMA Psychiatry. (2017) 74:351–9. doi: 10.1001/jamapsychiatry.2017.0044
9. Karyotaki E, Ebert DD, Donkin L, Riper H, Twisk J, Burger S, et al. Do guided internet-based interventions result in clinically relevant changes for patients with depression? An individual participant data meta-analysis. Clin Psychol Rev. (2018) 63:80–92. doi: 10.1016/j.cpr.2018.06.007
10. Karyotaki E, Efthimiou O, Miguel C, Bermpohl FMG, Furukawa TA, Cuijpers P, et al. Internet-Based cognitive behavioral therapy for depression: a systematic review and individual patient data network meta-analysis. JAMA Psychiatry. (2021) 78:361–71. doi: 10.1001/jamapsychiatry.2020.4364
11. Cohen ZD, Delgadillo J, DeRubeis RJ. Personalized treatment approaches. In: Barkham M, Lutz W, Castonguay LG, editors. Bergin and Garfield’s Handbook of Psychotherapy and Behavior Change: 50th Anniversary Edition. New York, NY: John Wiley & Sons, Inc. (2021). p. 673–703.
12. Coppersmith DDL, Dempsey W, Kleiman EM, Bentley KH, Murphy SA, Nock MK. Just-in-Time adaptive interventions for suicide prevention: promise, challenges, and future directions. Psychiatry. (2022) 85:317–33. doi: 10.1080/00332747.2022.2092828
13. Teepe GW, Da Fonseca A, Kleim B, Jacobson NC, Salamanca Sanabria A, Tudor Car L, et al. Just-in-time adaptive mechanisms of popular Mobile apps for individuals with depression: systematic app search and literature review. J Med Internet Res. (2021) 23:e29412. doi: 10.2196/29412
14. Linardon J, Torous J, Firth J, Cuijpers P, Messer M, Fuller-Tyszkiewicz M. Current evidence on the efficacy of mental health smartphone apps for symptoms of depression and anxiety. A meta-analysis of 176 randomized controlled trials. World Psychiatry. (2024) 23:139–49. doi: 10.1002/wps.21183
15. Balaskas A, Schueller SM, Cox AL, Doherty G. Ecological momentary interventions for mental health: a scoping review. PLoS One. (2021) 16:e0248152. doi: 10.1371/journal.pone.0248152
16. MyinGermeys I, Klippel A, Steinhart H, Reininghaus U. Ecological momentary interventions in psychiatry. Curr Opin Psychiatry. (2016) 29:258–63. doi: 10.1097/YCO.0000000000000255
17. Schueller SM, Aguilera A, Mohr DC. Ecological momentary interventions for depression and anxiety. Depress Anxiety. (2017) 34:540–5. doi: 10.1002/da.22649
18. Versluis A, Verkuil B, Spinhoven P, van der Ploeg MM, Brosschot JF. Changing mental health and positive psychological well-being using ecological momentary interventions: a systematic review and meta-analysis. J Med Internet Res. (2016) 18:e152. doi: 10.2196/jmir.5642
19. Firth J, Torous J, Nicholas J, Carney R, Rosenbaum S, Sarris J. Can smartphone mental health interventions reduce symptoms of anxiety? A meta-analysis of randomized controlled trials. J Affect Disord. (2017) 218:15–22. doi: 10.1016/j.jad.2017.04.046
20. Nahum-Shani I, Hekler EB, Spruijt-Metz D. Building health behavior models to guide the development of just-in-time adaptive interventions: a pragmatic framework. Health Psychol. (2015) 34:1209–19. doi: 10.1037/hea0000306
21. Nahum-Shani I, Smith SN, Spring BJ, Collins LM, Witkiewitz K, Tewari A, et al. Just-in-time adaptive interventions (JITAIs) in mobile health: key components and design principles for ongoing health behavior support. Ann Behav Med. (2018) 52:446–62. doi: 10.1007/s12160-016-9830-8
22. Myin-Germeys I, Kuppens P. The Open Handbook of Experience Sampling Methodology: A Step-by-step guide to Designing, Conducting, and Analyzing ESM Studies. Leuven: Center for Research on Experience Sampling and Ambulatory Methods Leuven (2021).
23. Stone AA, Shiffman S. Ecological momentary assessment (ema) in behavioral medicine. Ann Behav Med. (1994) 16:199–202. doi: 10.1093/abm/16.3.199
24. Shiffman S, Stone AA, Hufford MR. Ecological momentary assessment. Annu Rev Clin Psychol. (2008) 4:1–32. doi: 10.1146/annurev.clinpsy.3.022806.091415
25. Trull TJ, Ebner-Priemer UW. Ambulatory assessment in psychopathology research: a review of recommended reporting guidelines and current practices. J Abnorm Psychol. (2020) 129:56–63. doi: 10.1037/abn0000473
26. Colombo D, Fernández-Álvarez J, Patané A, Semonella M, Kwiatkowska M, García-Palacios A, et al. Current state and future directions of technology-based ecological momentary assessment and intervention for major depressive disorder: a systematic review. J Clin Med. (2019) 8:465. doi: 10.3390/jcm8040465
27. Hall M, Scherner PV, Kreidel Y, Rubel JA. A systematic review of momentary assessment designs for mood and anxiety symptoms. Front Psychol. (2021) 12:642044. doi: 10.3389/fpsyg.2021.642044
28. Garatva P, Terhorst Y, Messner E-M, Karlen W, Pryss R, Baumeister H. Smart sensors for health research and improvement. In: Montag C, Baumeister H, editors. Digital Phenotyping and Mobile Sensing. Cham: Springer (2023). p. 395–411. doi: 10.1007/978-3-030-98546-2_23
29. Terhorst Y, Knauer J, Baumeister H. Smart sensing enhanced diagnostic expert systems. In: Montag C, Baumeister H, editors. Digital Phenotyping and Mobile Sensing. Cham: Springer (2023). p. 413–25. doi: 10.1007/978-3-030-98546-2_24
30. Kumar S, Al’Absi M, Beck JG, Ertin E, Scott MS. Behavioral monitoring and assessment via Mobile sensing technologies. In: Marsch LA, Lord SE, Dallery J, editors. Behavioral Healthcare and Technology: Using Science-Based Innovation to Transform Practice. New York: Oxford University Press (2015). p. 27–39.
31. el Hassouni A, Hoogendoorn M, van Otterlo M, Eiben AE, Muhonen V, Barbado E. A clustering-based reinforcement learning approach for tailored personalization of e-Health interventions. Arxiv Preprint (2018).
32. Liu M, Shen X, Pan W. Deep reinforcement learning for personalized treatment recommendation. Stat Med. (2022) 41:4034–56. doi: 10.1002/sim.9491
33. Pargent F, Schoedel R, Stachl C. Best practices in supervised machine learning: a tutorial for psychologists. Adv Methods Pract Psychol Sci. (2023) 6:25152459231162559. doi: 10.1177/25152459231162559
34. Stade EC, Stirman SW, Ungar LH, Boland CL, Schwartz HA, Yaden DB, et al. Large language models could change the future of behavioral healthcare: a proposal for responsible development and evaluation. NPJ Ment Health Res. (2024) 3:12. doi: 10.1038/s44184-024-00056-z
35. Hardeman W, Houghton J, Lane K, Jones A, Naughton F. A systematic review of just-in-time adaptive interventions (JITAIs) to promote physical activity. Int J Behav Nutr Phys Act. (2019) 16:31. doi: 10.1186/s12966-019-0792-7
36. Goldstein SP, Zhang F, Klasnja P, Hoover A, Wing RR, Thomas JG. Optimizing a just-in-time adaptive intervention to improve dietary adherence in behavioral obesity treatment: protocol for a microrandomized trial. JMIR Res Protoc. (2021) 10:e33568. doi: 10.2196/33568
37. Battalio SL, Conroy DE, Dempsey W, Liao P, Menictas M, Murphy S, et al. Sense2Stop: a micro-randomized trial using wearable sensors to optimize a just-in-time-adaptive stress management intervention for smoking relapse prevention. Contemp Clin Trials. (2021) 109:106534. doi: 10.1016/j.cct.2021.106534
38. Perski O, Hébert ET, Naughton F, Hekler EB, Brown J, Businelle MS. Technology-mediated just-in-time adaptive interventions (JITAIs) to reduce harmful substance use: a systematic review. Addiction. (2022) 117:1220–41. doi: 10.1111/add.15687
39. Spruijt-Metz D, Marlin BM, Pavel M, Rivera DE, Hekler E, De La Torre S, et al. Advancing behavioral intervention and theory development for Mobile health: the HeartSteps II protocol. Int J Environ Res Public Health. (2022) 19:2267. doi: 10.3390/ijerph19042267
40. Klasnja P, Smith S, Seewald NJ, Lee A, Hall K, Luers B, et al. Efficacy of contextually tailored suggestions for physical activity: a micro-randomized optimization trial of HeartSteps. Ann Behav Med. (2019) 53:573–82. doi: 10.1093/abm/kay067
41. Wang L, Miller LC. Just-in-the-moment adaptive interventions (JITAI): a meta-analytical review. Health Commun. (2020) 35:1531–44. doi: 10.1080/10410236.2019.1652388
42. Juarascio AS, Parker MN, Lagacey MA, Godfrey KM. Just-in-time adaptive interventions: a novel approach for enhancing skill utilization and acquisition in cognitive behavioral therapy for eating disorders. Int J Eating Disord. (2018) 51:826–30. doi: 10.1002/eat.22924
43. Houben M, Van Den Noortgate W, Kuppens P. The relation between short-term emotion dynamics and psychological well-being: a meta-analysis. Psychol Bull. (2015) 141:901–30. doi: 10.1037/a0038822
44. Allsopp K, Read J, Corcoran R, Kinderman P. Heterogeneity in psychiatric diagnostic classification. Psychiatry Res. (2019) 279:15–22. doi: 10.1016/j.psychres.2019.07.005
45. Cuthbert BN. The RDoC framework: facilitating transition from ICD/DSM to dimensional approaches that integrate neuroscience and psychopathology. World Psychiatry. (2014) 13:28–35. doi: 10.1002/wps.20087
46. van Genugten CR, Schuurmans J, Hoogendoorn A, Smit JH, Riper H. Data-driven clustering to discover profiles in the dynamics of major depressive disorder based on smartphone-based ecological momentary assessment of mood. Front Psychiatry. (2022) 13:755809. doi: 10.3389/fpsyt.2022.755809
47. Huibers MJH, Lorenzo-Luaces L, Cuijpers P, Kazantzis N. On the road to personalized psychotherapy: a research agenda based on cognitive behavior therapy for depression enhanced reader. Front Psychiatry. (2021) 11:1551. doi: 10.3389/fpsyt.2020.607508
48. Page MJ, Moher D, Bossuyt PM, Boutron I, Hoffmann TC, Mulrow CD, et al. PRISMA 2020 explanation and elaboration: updated guidance and exemplars for reporting systematic reviews. Br Med J. (2021) 372:n160. doi: 10.1136/bmj.n160
49. Juarascio A, Srivastava P, Clark K, Presseller E, Manasse S, Forman E. A clinician-controlled just-in-time adaptive intervention system (CBT+) designed to promote acquisition and utilization of cognitive behavioral therapy skills in bulimia nervosa: development and preliminary evaluation study. JMIR Form Res. (2021) 5:e18261. doi: 10.2196/18261
50. Presseller EK, Wilkinson ML, Trainor C, Lampe EW, Juarascio AS. Self-regulation deficits moderate treatment outcomes in a clinical trial evaluating just-in-time adaptive interventions as an augmentation to cognitive-behavioral therapy for bulimia-spectrum eating disorders. Int J Eating Disord. (2022) 55:709–16. doi: 10.1002/eat.23695
51. Juarascio AS, Presseller EK, Srivastava P, Manasse SM, Forman EM. A randomized controlled trial of CBT+: a clinician-controlled, just-in-time, adjunctive intervention for bulimia-Spectrum disorders. Behav Modif. (2023) 47:551–72. doi: 10.1177/01454455221109434
52. Juarascio AS, Presseller EK, Trainor C, Boda S, Manasse SM, Srivastava P, et al. Optimizing digital health technologies to improve therapeutic skill use and acquisition alongside enhanced cognitive-behavior therapy for binge-spectrum eating disorders: protocol for a randomized controlled trial. Int J Eating Disord. (2023) 56:470–7. doi: 10.1002/eat.23864
53. Juarascio AS, Srivastava P, Presseller EK, Lin M, Patarinski AGG, Manasse SM, et al. Using continuous glucose monitoring to detect and intervene on dietary restriction in individuals with binge eating: the SenseSupport withdrawal design study. JMIR Form Res. (2022) 6:e38479. doi: 10.2196/38479
54. Wang L, Miller L. Assessment and disruption of ruminative episodes to enhance mobile cognitive behavioral therapy just-in-time adaptive interventions in clinical depression: pilot randomized controlled trial. JMIR Form Res. (2023) 7:e37270. doi: 10.2196/37270
55. Pulantara IW, Parmanto B, Germain A. Development of a just-in-time adaptive mHealth intervention for insomnia: usability study. JMIR Hum Factors. (2018) 5:e21. doi: 10.2196/humanfactors.8905
56. Pulantara IW, Parmanto B, Germain A. Clinical feasibility of a just-in-time adaptive intervention app (iREST) as a behavioral sleep treatment in a military population: feasibility comparative effectiveness study. J Med Internet Res. (2018) 20:e10124. doi: 10.2196/10124
57. Wakschlag LS, Tandon D, Krogh-Jespersen S, Petitclerc A, Nielsen A, Ghaffari R, et al. Moving the dial on prenatal stress mechanisms of neurodevelopmental vulnerability to mental health problems: a personalized prevention proof of concept. Dev Psychobiol. (2021) 63(4):622–40. doi: 10.1002/dev.22057
58. Watkins ER. Rumination-Focused Cognitive-Behavioral Therapy for Depression. New York: The Guilford Press (2016).
59. Reiter T, Schoedel R. Never miss a beep: using mobile sensing to investigate (non-)compliance in experience sampling studies. Behav Res Methods. (2024) 56:4038–60. doi: 10.3758/s13428-023-02252-9
60. Moshe I, Terhorst Y, Opoku Asare K, Sander LB, Ferreira D, Baumeister H, et al. Predicting symptoms of depression and anxiety using smartphone and wearable data. Front Psychiatry. (2021) 12:625247. doi: 10.3389/fpsyt.2021.625247
61. Abd-Alrazaq A, AlSaad R, Shuweihdi F, Ahmed A, Aziz S, Sheikh J. Systematic review and meta-analysis of performance of wearable artificial intelligence in detecting and predicting depression. NPJ Digit Med. (2023) 6:84. doi: 10.1038/s41746-023-00828-5
62. Terhorst Y, Knauer J, Philippi P, Baumeister H. The relation between passively collected GPS mobility metrics and depressive symptoms: systematic review and meta-analysis. J Med Internet Res. (2024) 26:e51875. doi: 10.2196/51875
63. Seiferth C, Vogel L, Aas B, Brandhorst I, Carlbring P, Conzelmann A, et al. How to e-mental health: a guideline for researchers and practitioners using digital technology in the context of mental health. Nat Ment Health. (2023) 1:542–54. doi: 10.1038/s44220-023-00085-1
64. Ciharova M, Amarti K, van Breda W, Peng X, Lorente-Català R, Funk B, et al. Use of machine-learning algorithms based on text, audio and video data in the prediction of anxiety and post-traumatic stress in general and clinical populations: a systematic review. Biol Psychiatry. (2024) 96(7):519–31. doi: 10.1016/j.biopsych.2024.06.002
65. Mendes JPM, Moura IR, Van de Ven P, Viana D, Silva FJS, Coutinho LR, et al. Sensing apps and public data sets for digital phenotyping of mental health: systematic review. J Med Internet Res. (2022) 24:e28735. doi: 10.2196/28735
66. De Angel V, Lewis S, White K, Oetzmann C, Leightley D, Oprea E, et al. Digital health tools for the passive monitoring of depression: a systematic review of methods. NPJ Digit Med. (2022) 5:3. doi: 10.1038/s41746-021-00548-8
67. Liao P, Klasnja P, Tewari A, Murphy SA. Sample size calculations for micro-randomized trials in mHealth. Stat Med. (2016) 35:1944–71. doi: 10.1002/sim.6847
68. Klasnja P, Hekler EB, Shiffman S, Boruvka A, Almirall D, Tewari A, et al. Microrandomized trials: an experimental design for developing just-in-time adaptive interventions. Health Psychol. (2015) 34:1220–8. doi: 10.1037/hea0000305
69. Collins LM. Optimization of Behavioral, Biobehavioral, and Biomedical Interventions: The Multiphase Optimization Strategy (MOST). New York: Springer (2018).
70. Mistiri ME, Khan O, Rivera DE, Hekler E. System identification and hybrid model predictive control in personalized mHealth interventions for physical activity. 2023 American Control Conference (ACC). IEEE (2023). p. 2240–5. doi: 10.23919/ACC55779.2023.10156652
71. Hekler EB, Rivera DE, Martin CA, Phatak SS, Freigoun MT, Korinek E, et al. Tutorial for using control systems engineering to optimize adaptive Mobile health interventions. J Med Internet Res. (2018) 20:e214. doi: 10.2196/jmir.8622
Keywords: just-in-time adaptive intervention, digital mental health, intervention development, smartphone intervention, JITAI
Citation: van Genugten CR, Thong MSY, van Ballegooijen W, Kleiboer AM, Spruijt-Metz D, Smit AC, Sprangers MAG, Terhorst Y and Riper H (2025) Beyond the current state of just-in-time adaptive interventions in mental health: a qualitative systematic review. Front. Digit. Health 7:1460167. doi: 10.3389/fdgth.2025.1460167
Received: 5 July 2024; Accepted: 7 January 2025;
Published: 28 January 2025.
Edited by:
Yue Liao, University of Texas at Arlington, United StatesReviewed by:
Maria C. Swartz, University of Texas MD Anderson Cancer Center, United StatesStephanie Paige Goldstein, Brown University, United States
Copyright: © 2025 van Genugten, Thong, van Ballegooijen, Kleiboer, Spruijt-Metz, Smit, Sprangers, Terhorst and Riper. This is an open-access article distributed under the terms of the Creative Commons Attribution License (CC BY). The use, distribution or reproduction in other forums is permitted, provided the original author(s) and the copyright owner(s) are credited and that the original publication in this journal is cited, in accordance with accepted academic practice. No use, distribution or reproduction is permitted which does not comply with these terms.
*Correspondence: Heleen Riper, aC5yaXBlckB2dS5ubA==