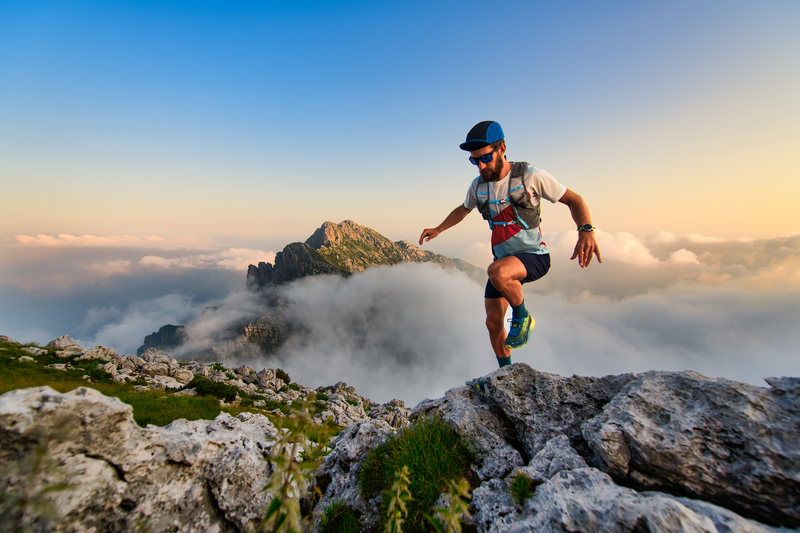
95% of researchers rate our articles as excellent or good
Learn more about the work of our research integrity team to safeguard the quality of each article we publish.
Find out more
ORIGINAL RESEARCH article
Front. Digit. Health , 12 February 2025
Sec. Digital Mental Health
Volume 7 - 2025 | https://doi.org/10.3389/fdgth.2025.1351955
The enigma of smartphone addiction (SA) has plagued academics for the last decade, now scholars believed this behaviour might affect physical and mental wellbeing. SA has become a complex problem, yet to date, there is limited research investigating the predictors of SA and its effect on “health-related quality of life (HRQoL)”. This study aimed to address this gap. The data was gathered from a convenience sample of 440 young adults completed between July 2021 and February 2022 through online survey in Bangladesh. On Logistic regression, after controlling for socio-demographic variables; friend support, process, social and compulsive usage were determined as significant predictors of SA. Those who were smartphone addicted were more presumably to have a lower quality of life. This study has significant implications for designing prevention pro-grams and policy development in relation to predictors of SA and its effect on HRQoL.
In 2007, Steve Jobs, co-founder of Apple, unveiled a revolutionary product that brought remarkable changes in the phone industry bringing the “smartphone” into existence (1). Since then, it become an indispensable component of modern lifestyle. It has changed the entire field of communication. The Smartphone affords easy internet access, is capable of impressive levels of data analysis and contains microphones, a camera, and lights (2, 3). In 2018, there were around three billion users of smartphone globally (4) and probably, it will reach over seven billion by 2026 (5). People use smartphones for social networking, gaming, shopping, taking photos, listening to music or watching movies (6). Despite its benefits, overuse of these devices can lead to SA (7) and SA is a growing public health concern all over the world (8). Different terminology was used to describe SA such as “problematic mobile phone use” “mobile phone addiction”, “smartphone overuse”, “(9), and “excessive use of smartphones” (10) and “addiction proneness” (11), and all representing a behavioral addiction. Till now, two types of behavioural addictions, gambling, and internet gaming disorders have been added to the “Diagnostic and Statistical Manual of Mental Disorders”, Fifth Edition (DSM-5) (12). Ching, Yee (13), defined SA as “mainly characterized by excessive or poorly controlled preoccupations, usage or behaviour regarding smartphone use; to the extent that individuals neglect other areas of life”. In many instances, smartphone users look at their devices while driving or walking (14) creating additional opportunities for harm. In addition SA use can make individuals “nomophobic”, which was narrated by Bhattacharya, Bashar (15) as “a psychological condition when people have a fear of being detached from mobile phone connectivity” [or fear of missing out (FOMO)].
Several factors are discussed to be responsible for SA. One recent study found that habitual use could be transformed into SA (16). Another found, increased habits of checking phone's notifications can lead to increased problematic smartphone use (17). Smartphone has become a popular tool for process use (e.g., entertainment) and social use (e.g., communication) (18). Process use has been identified as gratifying effects of consuming media (19), whereas social use can give pleasurable experience to the participants through social interaction (18). These user patterns may trigger the “reward system of addictive behaviors” (20). A recent survey revealed that 58.7% of the world's population use social media (21). Previous studies reported process and social usage as a strong determinant of SA (18). Furthermore, compulsive behaviour has been identified as a diagnostic criteria of SA (22) and it is positively associated with SA. O'Guinn and Faber (23) defined compulsive usage as “an uncontrollable drive or desire to obtain, use, or experience a feeling, substance, or activity that leads the individual to repeatedly engage in behavior that will ultimately cause harm to the individual and/or others”. Previous study also revealed that the compulsive behaviors are more likely to result in negative psychological distress outcomes, including stress and depression, which is also relevant to smartphone addition (24). Previous studies found that lower levels of physical activity, musculoskeletal pain, or poor sleep were associated to SA (25–27). Studies also revealed that SA was directly correlated to anxiety, stress and negative emotions (28, 29). Moreover, SA can reduce face-to-face communication (30, 31) and lead to social isolation (32, 33). Previous studies emphasized that SA was related to poor HRQoL (34, 35), and HRQoL includes the “physical, mental, social, emotional, and behavioral” components of well-being (36).
In today's world, the smartphone has become an essential tool from highly developed to the less developed countries (37). Bangladesh represents one of the developing countries that has been digitalized by increasing internet use, at present, more than 176 million mobile phone users in Bangladesh (38). To our best knowledge, no previous study has explored the predictors of SA and its impact on HRQoL among young adults in Bangladesh. To reduce this gap, the current study aimed:
(1) To what extent different form of smartphone usage and social support can predict and determine SA among young adults in Bangladesh?
(2) To identify the effect of SA on HRQoL among young adults in Bangladesh.
This study was cross-sectional in design and conducted among the young adults in Bangladesh. In order to qualify for participation, applicants must be aged between 18 and 32 years old, fluent in spoken and written Bengali, and used a smartphone for at least one year. Participants who were under the age of 18 or over the age of 32, unable to read or write in Bengali, or who had a mobile phone that was less than one year old were excluded from the study.
The data was collected via an online survey which was administered through Qualtrics from July 2021 to February 2022. This study was advertised through flyers, social media platforms and emails. Participants were recruited through the advertisements, as the online survey link was attached to the study advertisement. “Participant Information Sheet (PIS)”and “Participant Consent Form (PCF)” were included with the online survey, where participants could read PIS and accept PCF before seeing and completing the survey. This current study received approval from the “University of Wollongong Human Research Ethics Committee (HREC)” in July 2021 (Reference number- 2021/059).
A power analytic methodology was utilized to calculate an appropriate sample size for the quantitative phase of the study. According to Cohen's formula, 304 respondents would give a 95% chance of identifying a correlation of less than 0.10 at the 0.05 level with a maximum of ten predicting variables in the model, allowing for a 15% possibility of incomplete surveys (39). The least effect that would be significant to detect for the purpose of this study was an effect size of 0.10. In total, 440 eligible young adults participated in the study.
Sociodemographic data included sex; age; gainfully employment status; family income; marital status; family size; semesters of study.
SA was assessed through the “Smartphone Addiction Scale (SAS-SV)” which is an internationally recognized scale (40). It is a 10-item questionnaire, scoring from 1 = “Strongly disagree” to 6 = “Strongly agree” on a Likert scale. The total score for SAS-SV ranges from 10 to 60, a higher score indicates more problematic smartphone use. For males, the cut-off value of SA is 31 but it is 33 for females.
The social and process usage of smartphone had been assessed by the social and process usage scales which was adapted from Chua, Goh (41). It consists of 12 items (five items for social usage and seven items for process usage) that include a Likert scale ranging from “strongly disagree = 1” to “strongly agree = 5”.
To determine the compulsive usage of smartphones, the research used the “compulsive usage scale”. it was developed by Lee, Chang (42) and has been modified by Dinesh and Arulchelvan (43). It consists of seven items (Cronbach's alpha = 0.84) with response options ranging from “strongly disagree = 1” to “strongly agree = 5”.
The 26 items “World Health Organization Quality of Life Questionnaire (WHOQOL-BREF)” was used to measure “physical health (7 questions)”, “psychological health (6 questions)”, “environmental health” “(8 questions)” and “social relationships (3 questions)”. A “5-point scale” was used to evaluate each item and domain scores were transformed into a percentage “0–100; midpoint 50”.
There are many different approaches to survey translation, such as forward-only translation, forward-only translation with testing, back-translation, back-translation with a monolingual test, back-translation with a bilingual test, and back-translation with both a monolingual test and a bilingual test (44). The technique of back-translation, which was proposed by Brislin (45), was utilized to translate the study instruments into Bengali language, a predominant language spoken in Bangladesh. The instruments which were only available in the English language were translated into the Bengali language and then back into the English language. In order to check and ensure the consistency of the translations, it was carried out by two academics who were fluent in both Bengali and English. First the measures were translated from English into Bengali. Then it was translated back English by another academic. After that, the two academics compared the English versions of the measures to address any differences, and then they finalized the translated versions.
Data were subjected to analyse using “IBM SPSS Statistics Version 27.0.”. Univariate statistics were performed to determine the prevalence of SA and describe sociodemographic. Onley 15 cases with Z-scores higher than 3 or less than −3 in each of the study scales were identified as outliers and removed from the analysis (46).
The means of independent variables (smartphone usage and perceived social supports; quality of life scales) were compared by independent t-tests to determine whether there was any significant difference between the smartphone addicted and non-addicted groups.
“Forward stepwise logistic regression” analyses were done to find predictors of SA among the independent variables. The “forward stepwise regression model” adds the most significant variables into the model until none of the remaining variables can improve the model (47).
As shown in Table 1, smartphone addicted participants had higher scores for compulsive usage (M = 40.44, SD = 9.03), process usage (M = 25.16, SD = 3.37), and social usage (M = 19.18, SD = 3.00), but lower scores for family support (M = 19.27, SD = 7.03), friend support (M = 18.04, SD = 6.57), and significant other support (M = 19.27, SD = 6.49) than non-addicted participants.
Table 1. Comparison of process usage, social usage, compulsive usage, significant other support, family support, and friends support between smartphone addicted and non-addicted groups.
After performing univariate logistic regression analysis (Table 2), we found that social usage, process usage, compulsive usage, significant other support family support and friend support were the significant predictors of smartphone addiction among the participants. Process usage (OR = 1.14, 95% CI: 1.08–1.21), social usage (OR = 1.11 95% CI: 1.04–1.18), and compulsive usage (OR = 1.08, 95% CI: 1.05–1.10) increased the possibility of smartphone addiction by 14%, 11%, and 8% respectively. On the other hand, significant other support (OR = 0.96, 95% CI: 0.93–0.99), friend support (OR = 0.95, 95% CI: 0.92–0.98), and family support (OR = 0.96, 95% CI: 0.93–0.99) decreased the possibility of smartphone addiction by 4%, 4% and 5% respectively.
Table 2. Forward stepwise logistic regression analysis of associations of process usage, social usage, compulsive usage, significant other support, family support, and friends support, family support, and friends support with smartphone addiction.
A forward stepwise multivariate logistic regression analysis was performed to assess the significant predictors of smartphone addiction among the participants. The analysis suggested that process usage, social usage and compulsive usage were the predictors of smartphone addiction in the presence of each other (see Table 3).
Table 3. Forward stepwise logistic regression of analysis of associations of process usage, social usage, compulsive usage, significant other support, family support, and friends support with smartphone addiction.
As stated in Table 4, while controlling for the socio-demographic variables, social usage, process usage, friend support and compulsive usage were still significant predictors of smartphone addiction. Being male, having a younger age ≤ 25), and being unemployed were strong predictors of smartphone addiction. More precisely, male, younger and unemployed participants were 1.92–2.52 times more likely to be addicted.
Table 4. Forward stepwise logistic regression analysis of associations of process usage, social usage, compulsive usage, and friends support with smartphone addiction controlling for the potential confounding effects of socio-demographic variables.
As presented in Table 5, the individuals who were addicted to smartphone were more likely to have a reduced quality of life assessed by WHOQOL-BREF in terms of Physical health, psychological health, social relationships and environmental health compared to their none addicted counterparts.
This study revealed that “process usage and social usage” were significant predictors of SA, which is similar to previous studies (18, 48). This might be explained by the fact that process usage and social usage opened a newer horizon in the entertainment and communication industries that leads pleasurable experience. For instance, revealing new features, or checking new notifications in social media can stimulate the “reward center” of the brain (49), leading to increased recurrence of behaviour (50). This pattern of use may result in habitual use, which is ultimately difficult to control. It is worth noting that at present different musical applications, social media platforms have become the prime source of entertainment especially for young people (51, 52), and regular notifications from these sources can provoke the “Fear of missing out (FoMO)” which can lead to SA (53). In addition, compulsive behaviour has been recognized as one of the core components of addiction (54, 55), and interestingly, those who are addicted to their devices are more likely to lose self-control or self-regulation due to compulsive usage (56).
Our results also reported that SA can reduce quality of life in all four domains among smartphone addicted group compared to the non-addicted group. These results in line with the findings of previous studies (57–59) which also reported a negative correlation between SA and the quality of life. Those who are addicted to SA, may have a physical connection to their device and carry their device with them all of the time (60). This phenomenon was explained by Natasha et al. through attachment theory (61), a constant connectivity with their devices may be used to maintain a sense of security and inhibit the feelings of insecurity, ultimately, this pattern of behaviour maintains persistent brain use. There is growing evidence that SA can deteriorate physical and mental health (62–64), in addition, insomnia and poor sleep quality has been identified as a negative consequences of SA (65, 66). Previous studies also suggested that SA is associated with Attention-deficit hyperactivity disorder (ADHD) (67, 68). Moreover, previous research reported that SA is associated with social isolation and loneliness (32, 69). Ultimately, all these conditions diminish the quality of life those who are addicted to their devices.
The results of this study provide invaluable information to understand the effects of SA, which may have significant implications for developing interventions to reduce smartphone addiction. Uninstalling applications from phones, adding time limits or limited internet access during work or sleep may be helpful in reducing smartphone use among the youth. This current study has several limitations that deserve to be mentioned. It is a cross-sectional study by design, so it is difficult to determine a causal relationship. Moreover, data were self-reported which can create recall bias. In addition, the data was collected using a convenience sample, so these findings may not be generalized for the wider Bangladeshi population of youth.
The raw data supporting the conclusions of this article will be made available by the authors, without undue reservation.
The studies involving humans were approved by University of Wollongong Human Research Ethics Committee (HREC)” in July 2021 “(Reference number- 2021/059). The studies were conducted in accordance with the local legislation and institutional requirements. The participants provided their written informed consent to participate in this study.
ZR: Conceptualization, Formal Analysis, Investigation, Methodology, Writing – original draft. AP: Supervision, Writing – review & editing. MA: Writing – review & editing. HH: Supervision, Writing – review & editing.
The author(s) declare that no financial support was received for the research, authorship, and/or publication of this article.
The authors declare that the research was conducted in the absence of any commercial or financial relationships that could be construed as a potential conflict of interest.
The author(s) declared that they were an editorial board member of Frontiers, at the time of submission. This had no impact on the peer review process and the final decision.
All claims expressed in this article are solely those of the authors and do not necessarily represent those of their affiliated organizations, or those of the publisher, the editors and the reviewers. Any product that may be evaluated in this article, or claim that may be made by its manufacturer, is not guaranteed or endorsed by the publisher.
1. Gobble MM. Apple scores a win in the smartphone wars. Res Technol Manage. (2012) 55(6):4. doi: 10.5437/08956308X5506005
2. Suarez-Tangil G, Tapiador JE, Peris-Lopez P, Ribagorda A. Evolution, detection and analysis of malware for smart devices. IEEE Commun Surv Tutor. (2013) 16(2):961–87. doi: 10.1109/SURV.2013.101613.00077
3. Panova T, Carbonell X. Is smartphone addiction really an addiction? J Behav Addict. (2018) 7(2):252–9. doi: 10.1556/2006.7.2018.49
4. Li T, Zhang M, Li Y, Lagerspetz E, Tarkoma S, Hui P. The impact of COVID-19 on smartphone usage. IEEE Int Things J. (2021) 8(23):16723–33. doi: 10.1109/JIOT.2021.3073864
5. Croce AI, Musolino G, Rindone C, Vitetta A. Estimation of travel demand models with limited information: floating car data for parameters’ calibration. Sustainability. (2021) 13(16):8838. doi: 10.3390/su13168838
6. Alhazmi AA, Alzahrani SH, Baig M, Salawati EM, Alkatheri A. Prevalence and factors associated with smartphone addiction among medical students at king abdulaziz university, jeddah. Pak J Med Sci. (2018) 34(4):984. doi: 10.12669/pjms.344.15294
7. Elhai JD, Dvorak RD, Levine JC, Hall BJ. Problematic smartphone use: a conceptual overview and systematic review of relations with anxiety and depression psychopathology. J Affect Disord. (2017) 207:251–9. doi: 10.1016/j.jad.2016.08.030
8. Lee HK, Kim J-H, Fava M, Mischoulon D, Park J-H, Shim E-J, et al. Development and validation study of the smartphone overuse screening questionnaire. Psychiatry Res. (2017) 257:352–7. doi: 10.1016/j.psychres.2017.07.074
9. Ding D, Li J. Smartphone overuse—a growing public health issue. J Psychol Psychother. (2017) 07(01):2–4. doi: 10.4172/2161-0487.1000289
10. Kahyaoglu H, et al. Effects of smartphone addiction level on social and educational life in health sciences students. Nurasian J Fam Med. (2016) 5(1):13–9.
11. Kim D, Lee Y, Lee J, Nam JK, Chung Y. Development of Korean smartphone addiction proneness scale for youth. PLoS One. (2014) 9(5):e97920. doi: 10.1371/journal.pone.0097920
12. Rosenberg KP, Feder LC. An introduction to behavioral addictions. In: Rosenberg KP, Feder LC, editors. Behavioral Addictions: Criteria, Evidence, and Treatment. Amsterdam: Academic Press (2014). p. 1–17.
13. Ching SM, Yee A, Ramachandran V, Sazlly Lim SM, Wan Sulaiman WA, Foo YL, et al. Validation of a malay version of the smartphone addiction scale among medical students in Malaysia. PLoS One. (2015) 10(10):e0139337. doi: 10.1371/journal.pone.0139337
14. Lee M, Han M, Pak J. Analysis of behavioral characteristics of smartphone addiction using data mining. Appl Sci. (2018) 8(7):1191. doi: 10.3390/app8071191
15. Bhattacharya S, Bashar M, Srivastava A, Singh A. NOMOPHOBIA: nO MObile PHone PhoBIA. J Family Med Prim Care. (2019) 8(4):1297–300. doi: 10.4103/jfmpc.jfmpc_71_19
16. Elhai JD, Levine JC, Dvorak RD, Hall BJ. Fear of missing out, need for touch, anxiety and depression are related to problematic smartphone use. Comput Human Behav. (2016) 63:509–16. doi: 10.1016/j.chb.2016.05.079
17. Oulasvirta A, Rattenbury T, Ma L, Raita E. Habits make smartphone use more pervasive. Pers Ubiquitous Comput. (2012) 16(1):105–14. doi: 10.1007/s00779-011-0412-2
18. Van Deursen AJ, Bolle CL, Hegner SM, Kommers PAM. Modeling habitual and addictive smartphone behavior: the role of smartphone usage types, emotional intelligence, social stress, self-regulation, age, and gender. Comput Human Behav. (2015) 45:411–20. doi: 10.1016/j.chb.2014.12.039
19. Song I, Larose R, Eastin MS, Lin CA. Internet gratifications and internet addiction: on the uses and abuses of new media. Cyberpsychol Behav. (2004) 7(4):384–94. doi: 10.1089/cpb.2004.7.384
20. Yang SC, Tung C-J. Comparison of internet addicts and non-addicts in Taiwanese high school. Comput Human Behav. (2007) 23(1):79–96. doi: 10.1016/j.chb.2004.03.037
22. Lin Y-H, Chang L-R, Lee Y-H, Tseng H-W, Kuo TBJ, Chen S-H. Development and validation of the smartphone addiction inventory (SPAI). PLoS One. (2014) 9(6):e98312. doi: 10.1371/journal.pone.0098312
23. O'Guinn TC, Faber RJ. Compulsive buying: a phenomenological exploration. J Consum Res. (1989) 16(2):147–57. doi: 10.1086/209204
24. Matusik SF, Mickel AE. Embracing or embattled by converged mobile devices? Users’ experiences with a contemporary connectivity technology. Hum Relat. (2011) 64(8):1001–30. doi: 10.1177/0018726711405552
25. Alosaimi FD, Alyahya H, Alshahwan H, Mahyijari NA, Shaik SA. Smartphone addiction among university students in Riyadh, Saudi Arabia. Saudi Med J. (2016) 37(6):675–83. doi: 10.15537/smj.2016.6.14430
26. Matar Boumosleh J, Jaalouk D. Depression, anxiety, and smartphone addiction in university students- a cross sectional study. PLoS One. (2017) 12(8):e0182239. doi: 10.1371/journal.pone.0182239
27. Demirci K, Akgonul M, Akpinar A. Relationship of smartphone use severity with sleep quality, depression, and anxiety in university students. J Behav Addict. (2015) 4(2):85–92. doi: 10.1556/2006.4.2015.010
28. Samaha M, Hawi NS. Relationships among smartphone addiction, stress, academic performance, and satisfaction with life. Comput Human Behav. (2016) 57:321–5. doi: 10.1016/j.chb.2015.12.045
29. Wan Ismail WS, Sim ST, Tan K-A, Bahar N, Ibrahim N, Mahadevan R, et al. The relations of internet and smartphone addictions to depression, anxiety, stress, and suicidality among public university students in Klang Valley, Malaysia. Perspect Psychiatr Care. (2020) 56(4):949–55. doi: 10.1111/ppc.12517
30. Dwyer RJ, Kushlev K, Dunn EW. Smartphone use undermines enjoyment of face-to-face social interactions. J Exp Soc Psychol. (2018) 78:233–9. doi: 10.1016/j.jesp.2017.10.007
31. Abeele MMV, Antheunis ML, Schouten AP. The effect of mobile messaging during a conversation on impression formation and interaction quality. Comput Human Behav. (2016) 62:562–9. doi: 10.1016/j.chb.2016.04.005
32. Al-Kandari YY, Al-Sejari MM. Social isolation, social support and their relationship with smartphone addiction. Inf Commun Soc. (2021) 24(13):1925–43. doi: 10.1080/1369118X.2020.1749698
33. Enez Darcin A, Kose S, Noyan CO, Nurmedov S, Yılmaz O, Dilbaz N. Smartphone addiction and its relationship with social anxiety and loneliness. Behav Inf Technol. (2016) 35(7):520–5. doi: 10.1080/0144929X.2016.1158319
34. Buctot DB, Kim N, Kim JJ. Factors associated with smartphone addiction prevalence and its predictive capacity for health-related quality of life among Filipino adolescents. Child Youth Serv Rev. (2020) 110:104758. doi: 10.1016/j.childyouth.2020.104758
35. Mascia ML, Agus M, Penna MP. Emotional intelligence, self-regulation, smartphone addiction: which relationship with student well-being and quality of life? Front Psychol. (2020) 11:375. doi: 10.3389/fpsyg.2020.00375
36. Ravens-Sieberer U, Gosch A, Erhart M, von Rueden U, Nickel J. The KIDSCREEN Questionnaires: Quality of Life Questionnaires for Children and Adolescents. Lengerich: Pabst Science Publisher (2006).
37. Van der Boor P, Oliveira P, Veloso F. Users as innovators in developing countries: the global sources of innovation and diffusion in mobile banking services. Res Policy. (2014) 43(9):1594–607. doi: 10.1016/j.respol.2014.05.003
39. Cohen J, Cohen P, West SG, Aiken LS. Applied Multiple Regression/correlation Analysis for the Behavioral Sciences. Mahwah, NJ: Lawrence Erlbaum Associates Publishers (2003). p. 703–xxviii.
40. Kwon M, Kim DJ, Cho H, Yang S. The smartphone addiction scale: development and validation of a short version for adolescents. PLoS One. (2014) 8(12):e83558. doi: 10.1371/journal.pone.0083558
41. Chua AYK, Goh DHL, Lee CS. Mobile content contribution and retrieval: an exploratory study using the uses and gratifications paradigm. Inf Process Manag. (2012) 48(1):13–22. doi: 10.1016/j.ipm.2011.04.002
42. Lee Y-K, Chang C-T, Lin Y, Cheng Z-H. The dark side of smartphone usage: psychological traits, compulsive behavior and technostress. Comput Human Behav. (2014) 31:373–83. doi: 10.1016/j.chb.2013.10.047
43. Dinesh K, Arulchelvan S. The attitude towards smartphones and its influence on process, social and compulsive usage. Athens J Mass Media Commun. (2018) 4:301–18. doi: 10.30958/ajmmc.4-4-4
44. Maneesriwongul W, Dixon JK. Instrument translation process: a methods review. J Adv Nurs. (2004) 48(2):175–86. doi: 10.1111/j.1365-2648.2004.03185.x
45. Brislin RW. Back-translation for cross-cultural research. J Cross Cult Psychol. (1970) 1(3):185–216. doi: 10.1177/135910457000100301
46. Tabachnick BG, Fidell LS, Ullman JB. Using Multivariate Statistics. Boston, MA: Pearson (2007) 5.
47. Ranganathan P, Pramesh C, Aggarwal R. Common pitfalls in statistical analysis: logistic regression. Perspect Clin Res. (2017) 8(3):148. doi: 10.4103/picr.PICR_87_17
48. Wacks Y, Weinstein AM. Excessive smartphone use is associated with health problems in adolescents and young adults. Front Psychiatry. (2021) 12:669042. doi: 10.3389/fpsyt.2021.669042
49. Alter A. Irresistible: Why we Can’t Stop Checking, Scrolling, Clicking and Watching. London: Random House (2017).
50. Kawamichi H, Sugawara SK, Hamano YH, Makita K, Kochiyama T, Sadato N. Increased frequency of social interaction is associated with enjoyment enhancement and reward system activation. Sci Rep. (2016) 6(1):24561. doi: 10.1038/srep24561
51. Bhave K, Jain V, Roy S. Understanding the orientation of gen Y toward mobile applications and in-app advertising in India. Int J Mobile Marketing. (2013) 8(1):62–74.
52. Allcott H, Gentzkow M, Song L. Digital addiction. Am Econ Rev. (2022) 112(7):2424–63. doi: 10.1257/aer.20210867
53. Brown L, Kuss DJ. Fear of missing out, mental wellbeing, and social connectedness: a seven-day social media abstinence trial. Int J Environ Res Public Health. (2020) 17(12):4566. doi: 10.3390/ijerph17124566
54. Gau SS, Liu C-Y, Lee C-S, Chang J-C, Chang C-J, Li C-F, et al. Development of a Chinese version of the Yale-brown obsessive compulsive scale for heavy drinking. Alcohol Clin Exp Res. (2005) 29(7):1172–9. doi: 10.1097/01.ALC.0000172167.20119.9F
55. Lin Y-H, Lin S-H, Yang CC, Kuo TB. Psychopathology of everyday life in the 21st century: smartphone addiction. In: Montag C, Reuter M, editors. Internet Addiction: Neuroscientific Approaches and Therapeutical Implications Including Smartphone Addiction. Cham: Springer International Publishing (2017). p. 339–58.
56. Ting CH, Chen YY. Chapter 8—smartphone addiction. In: Essau CA, Delfabbro PH, editors. Adolescent Addiction. 2nd ed. San Diego: Academic Press (2020). p. 215–40.
57. Demir YP, Sumer MM. Effects of smartphone overuse on headache, sleep and quality of life in migraine patients. Neurosciences. (2019) 24(2):115–21. doi: 10.17712/nsj.2019.2.20180037
58. Zhang MX, Wu AMS. Effects of smartphone addiction on sleep quality among Chinese university students: the mediating role of self-regulation and bedtime procrastination. Addict Behav. (2020) 111:106552. doi: 10.1016/j.addbeh.2020.106552
59. Gao T, Xiang Y-T, Zhang H, Zhang Z, Mei S. Neuroticism and quality of life: multiple mediating effects of smartphone addiction and depression. Psychiatry Res. (2017) 258:457–61. doi: 10.1016/j.psychres.2017.08.074
60. Kumcagiz H. Quality of life as a predictor of smartphone addiction risk among adolescents. Technol Knowl Learning. (2019) 24(1):117–27. doi: 10.1007/s10758-017-9348-6
61. Parent N, Shapka J. Moving beyond addiction: an attachment theory framework for understanding young adults’ relationships with their smartphones. Hum Behav Emerging Technol. (2020) 2(2):179–85. doi: 10.1002/hbe2.180
62. Ratan ZA, Parrish A-M, Zaman S, Alotaibi M, Hosseinzadeh H. Smartphone addiction and associated health outcomes in adult populations: a systematic review. Int J Environ Res Public Health. (2021) 18(22):12257. doi: 10.3390/ijerph182212257
63. Davey S, Davey A. Assessment of smartphone addiction in Indian adolescents: a mixed method study by systematic-review and meta-analysis approach. Int J Prev Med. (2014) 5(12):1500.25709785
64. Rho MJ, Park J, Na E, Jeong J-E, Kim JK, Kim D-J, et al. Types of problematic smartphone use based on psychiatric symptoms. Psychiatry Res. (2019) 275:46–52. doi: 10.1016/j.psychres.2019.02.071
65. Höhn C, Schmid SR, Plamberger CP, Bothe K, Angerer M, Gruber G, et al. Preliminary results: the impact of smartphone use and short-wavelength light during the evening on circadian rhythm, sleep and alertness. Clocks Sleep. (2021) 3(1):66–86. doi: 10.3390/clockssleep3010005
66. Zhang MX, Zhou H, Yang HM, Wu AM. The prospective effect of problematic smartphone use and fear of missing out on sleep among Chinese adolescents. Curr Psychol. (2023) 42(7):5297–305. doi: 10.1007/s12144-021-01863-9
67. Kwon SJ, Kim Y, Kwak Y. Influence of smartphone addiction and poor sleep quality on attention-deficit hyperactivity disorder symptoms in university students: a cross-sectional study. J Am Coll Health. (2022) 70(1):209–15. doi: 10.1080/07448481.2020.1740228
68. Park Y, Lee S. Gender differences in smartphone addiction and depression among Korean adolescents: focusing on the internal mechanisms of attention deficit and self-control. Comput Human Behav. (2022) 136:107400. doi: 10.1016/j.chb.2022.107400
69. Taghizadeh F, Reyhani P, Molavi N, Babakhanian M, Ghazanfarpour M, Mirzaee F, et al. Investigating the relationship between smartphone addiction and loneliness and its impact on motivation to progress in high school students. Int J Pediatr. (2019) 7(10):10187–93. doi: 10.22038/ijp.2019.41716.3514
Keywords: smartphone addiction, predictors, quality of life, Bangladesh, compulsive usage
Citation: Ratan ZA, Parrish A-M, Alotaibi MS and Hosseinzadeh H (2025) Predictors of smartphone addiction and its effect on quality of life: a cross-sectional study among the young adults in Bangladesh. Front. Digit. Health 7:1351955. doi: 10.3389/fdgth.2025.1351955
Received: 19 December 2023; Accepted: 22 January 2025;
Published: 12 February 2025.
Edited by:
Hui Zheng, National Institute on Drug Abuse (NIH), United StatesReviewed by:
Nipin Kalal, All India Institute of Medical Sciences Jodhpur, IndiaCopyright: © 2025 Ratan, Parrish, Alotaibi and Hosseinzadeh. This is an open-access article distributed under the terms of the Creative Commons Attribution License (CC BY). The use, distribution or reproduction in other forums is permitted, provided the original author(s) and the copyright owner(s) are credited and that the original publication in this journal is cited, in accordance with accepted academic practice. No use, distribution or reproduction is permitted which does not comply with these terms.
*Correspondence: Hassan Hosseinzadeh, aGFzc2FuaEB1b3cuZWR1LmF1
Disclaimer: All claims expressed in this article are solely those of the authors and do not necessarily represent those of their affiliated organizations, or those of the publisher, the editors and the reviewers. Any product that may be evaluated in this article or claim that may be made by its manufacturer is not guaranteed or endorsed by the publisher.
Research integrity at Frontiers
Learn more about the work of our research integrity team to safeguard the quality of each article we publish.