- 1Department of Computer Science, Faculty of Science, University of Ruhuna, Matara, Sri Lanka
- 2Department of Information Technology, Sri Lanka Institute of Advanced Technological Education, Galle, Sri Lanka
Tropical diseases can often be caused by viruses, bacteria, parasites, and fungi. They can be spread over vectors. Analysis of multiple omics data types can be utilized in providing comprehensive insights into biological system functions and disease progression. To this end, bioinformatics tools and diverse AI techniques are pivotal in identifying and understanding tropical diseases through the analysis of omics data. In this article, we provide a thorough review of opportunities, challenges, and future directions of utilizing Bioinformatics tools and AI-assisted models on tropical disease identification using various omics data types. We conducted the review from 2015 to 2024 considering reliable databases of peer-reviewed journals and conference articles. Several keywords were taken for the article searching and around 40 articles were reviewed. According to the review, we observed that utilization of omics data with Bioinformatics tools like BLAST, and Clustal Omega can make significant outcomes in tropical disease identification. Further, the integration of multiple omics data improves biomarker identification, and disease predictions including disease outbreak predictions. Moreover, AI-assisted models can improve the precision, cost-effectiveness, and efficiency of CRISPR-based gene editing, optimizing gRNA design, and supporting advanced genetic correction. Several AI-assisted models including XAI can be used to identify diseases and repurpose therapeutic targets and biomarkers efficiently. Furthermore, recent advancements including Transformer-based models such as BERT and GPT-4, have been mainly applied for sequence analysis and functional genomics. Finally, the most recent GeneViT model, utilizing Vision Transformers, and other AI techniques like Generative Adversarial Networks, Federated Learning, Transfer Learning, Reinforcement Learning, Automated ML and Attention Mechanism have shown significant performance in disease classification using omics data.
1 Introduction
Tropical diseases may occur mainly in tropical and subtropical regions throughout the world. These regions include areas close to the equator, which are characterized by hot and humid climates. These diseases can often be caused by viruses, bacteria, parasites, and fungi (pathogens). Further, they are spread through vectors (i.e., mosquitoes, flies, and other insects). The main reason for the prevalence of these diseases is poor sanitation, inadequate and limitations in healthcare facilities, and poverty. For example Malaria, Dengue fever, chikungunya virus, Zika virus and yellow fever can be considered as tropical diseases.
Omics data include various studies of entire set of genes, RNAs, proteins and metabolites of various organisms are known as genomics, transcriptomics, proteomics and metabolomics respectively. Genomics data types include DNA sequences, single nucleotide polymorphisms (SNPs), copy number variations (CNVs), transcriptomics include mRNA, non-coding RNAs (e.g., miRNA, lncRNA), RNA-seq data, proteomics include protein sequences, post-translational modifications, protein-protein interactions, and metabolomics include metabolic profiles, metabolite concentrations. Out of various genomics applications, identifying genetic variations associated with diseases, evolutionary studies, and personalized medicine are a few applications. Understanding gene expression patterns, identifying gene regulatory networks, studying the effects of environmental factors on gene expression are some of the applications on transcriptomics. Some of the applications on proteomics are disease biomarker discovery, protein function and interaction identification, and drug target identification. Further, studying metabolic pathways, disease diagnostics, understanding the biochemical effects of drugs and toxins are a few applications in metabolomics. Integration of multiple omics data types can provide comprehensive understanding and insights into biological system functions, disease progression (i.e., cancers, infectious diseases, neurological disorders, etc.).
Early detection of tropical diseases is critical for effective treatments. Bioinformatics tools and diverse AI techniques are pivotal in identifying and understanding tropical diseases through the analysis of omics data. There exist several tools for bioinformatics analysis of genomics, transcriptomics, proteomics and metabolomics, as well as integrated multi-omics data. These tools can combine to enhance the ability to identify, understand, and predict tropical diseases by providing comprehensive analyses and insights of various omics data types. Wide range of AI techniques has significantly improved the field of tropical disease identification. Among them unsupervised and supervised Machine Learning (ML), Deep Learning (DL), Natural Language Processing (NLP) with GenAI and LLMs, Ensemble ML and Deep Ensemble Learning (DEL), and the most recent advances such as Explainable AI, Responsible AI etc. Various AI models can be trained on omics data to perform disease prediction, progression, and treatment response. These models can also be utilized to identify potential biomarkers for early diagnosis of tropical diseases and targeted therapy (1).
1.1 Road map
The rest of the article is organized as follows: Section 2 discusses the research methods and the process of the study. Section 3 then presents the literature review of both Bioinformatics and AI technology utilization in predicting tropical diseases using different omics datasets. It discusses the opportunities and challenges to predict tropical diseases with omics based AI and Bioinformatics technologies. Sections 4, 5 concludes the article, and suggests some future directions respectively.
2 Research methods and process
In this section, we provide details of our research methodology. Figure 1 indicates our research process. We have explored research papers using both extensive and explicit search approach. We selected research papers based on keywords, year of publication, their utilization of encompass Bioinformatics tools and AI techniques for tropical disease identification with omics data. We reviewed our selected papers to identify a few important questions and their answers. This article presents a comprehensive analysis, in which we describe Research Questions (RQs), and provide a detailed description of the answers to the questions. Our contribution is novel in identifying research questions, analyzing the opportunities and challenges, describing a detailed review of literature, and outlining future directions for research. Following are the major research questions we addressed throughout this paper.
(1) What are the key findings of state-of-the-art bioinformatics and AI-enabled techniques on tropical disease identification using omics data?
(2) What are the opportunities and challenges in current bioinformatics and AI-enabled systems specially using Advanced AI techniques?
(3) What are the future research directions of bioinformatics and AI-enabled systems to effectively use them in tropical disease identification?
Answers to the above mentioned questions are based on an extensive review of the existing literature, in which we collected and discussed information and knowledge related to Bioinformatics tools and techniques, AI-enabled techniques including the recent advances. In addition, we have also discussed limitations, challenges, and future directions of bioinformatics and AI-enabled techniques with recent advancements including transformer-based implementations (i.e., language models and vision transformers).
We initiate our research process by thoroughly formulating research questions and designing specified search strategies. Subsequently, we rigorously evaluate search results, adhering strictly to predetermined inclusion and exclusion criteria. Once we've selected relevant articles, we delve into their content, synthesizing our findings cohesively. Finally, we present our discoveries and present discussions. Here are the inclusion criteria guiding our research
1. Articles published within the last ten years (2015–2024) to capture recent advancements and developments in the field.
2. Research articles published in Journals and Conferences are considered
3. Research must focus on utilizing bioinformatics tools and AI technologies for tropical disease prediction and diagnosis with omics datasets
4. Only the articles published in English are considered.
Furthermore, we defined exclusion criteria for this systematic literature review to ensure that only associated and high-quality studies were included. Studies that meet the following criteria have been excluded from the review:
1. Articles published before 2015
2. Dissertations, Technical reports, and book chapters were excluded. In addition, articles that were not peer-reviewed, such as blog posts, opinion pieces, or other informal publications, were excluded to maintain the academic rigor of the review.
3. Studies that have not been published in English were excluded. While this can limit the inclusion of some relevant research, it ensures that all included studies are fully understood and accurately assessed by the reviewers.
4. Duplicate studies, or those with overlapping content, were identified and only the most comprehensive version was included. This was to avoid redundancy and ensure a wide range of perspectives and findings.
5. Research not focusing on utilizing bioinformatics tools and AI technologies for tropical disease prediction with omics datasets
6. Studies that lacked sufficient methodological detail citations, making it impossible to judge the reliability and validity of the findings, were excluded. This included studies with vague descriptions of their experimental or analytical methods.
By applying and choosing these exclusion criteria, we aimed to ensure that our systematic literature review includes only the most relevant and high-quality studies. This approach enhances the reliability and validity of the findings of this review, contributing a solid foundation for understanding how Bioinformatics tools and AI techniques provide insights into tropical diseases utilizing various omics data.
3 Literature review
Bioinformatics is a field which combines biology, computer science, and statistics to analyze various types of biological data. It plays a critical role in understanding complex biological processes, including disease mechanisms. Predictive modeling, often supported by Machine Learning (ML) techniques, is a key component of bioinformatics. Further, bioinformatics tools and techniques are highly utilized in tropical disease predication and identification with the input of omics datasets. In addition, wide flavors of AI play vital roles in different areas such as medicine, engineering, biology, linguistics, psychology, pharmacology, education, and neuroscience. Firstly, this review focuses on how Bioinformatics techniques bring transformative opportunities in the prediction and diagnosis of tropical diseases with the support of omics data. Then it investigates how AI can be utilized in advanced in the same purpose in more complex problem solving (2). Following section presents our main contributions of the systematic review.
3.1 Opportunities and challenges/limitations using bioinformatics and AI techniques for disease predictions
Though there are several improvements and advances in the field of disease prediction, diagnosis, predictive modeling etc. utilizing omics datasets including multi-omics data integration, there exist challenges and limitations of them allowing some room for the improvements.
Table 1 presents opportunities, challenges and future recommendations of past research on utilizing Bioinformatics tools for Omics Data Processing for disease predictions and Table 2. The opportunities, challenges and future recommendations utilizing suitable AI technologies with Omics data (at the end of the paper).
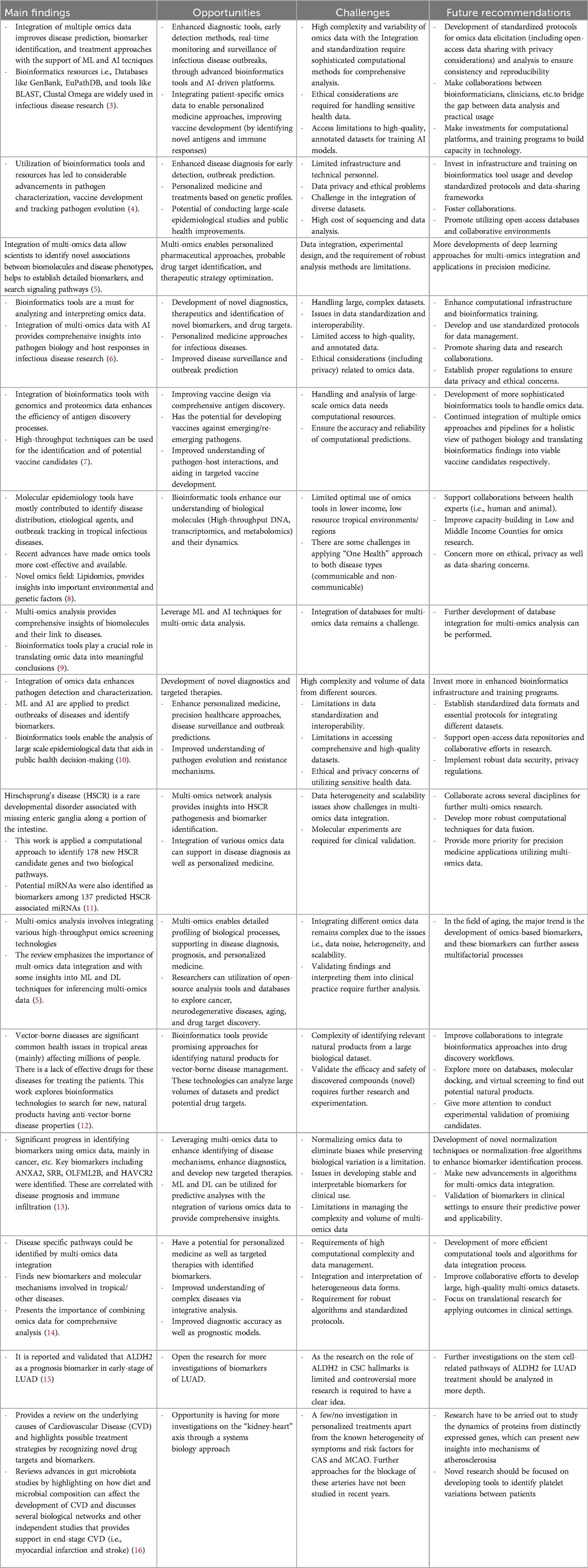
Table 1. Opportunities, challenges and future recommendations of past research on utilizing bioinformatics tools for omics data processing for disease predictions (including tropical diseases).
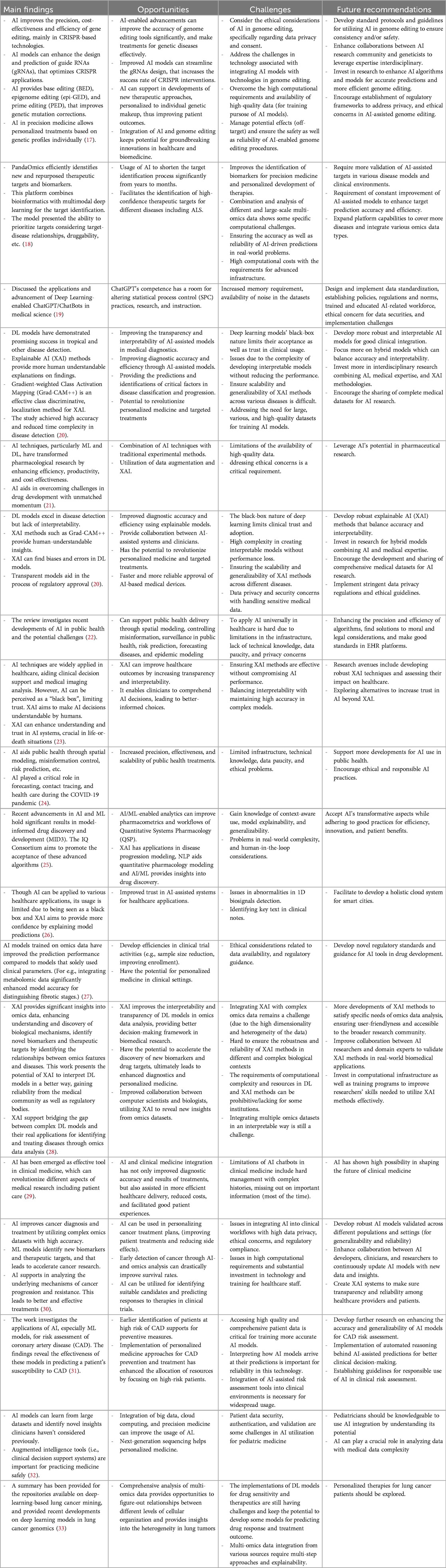
Table 2. Opportunities, challenges and future recommendations of past research on utilizing suitable AI techniques for omics data processing for disease predictions (including tropical diseases).
3.1.1 Opportunities and challenges in utilizing bioinformatics and AI techniques for tropical disease predictions with omics data
Though various Bioinformatics tools have been utilized for disease predictions with various types of datasets, there are a few research carried out for the predictions of tropical diseases using omics data types (8, 12). Apart from that, several AI based techniques have been utilized for tropical disease identification based on omics or other data types (35–37). A need analysis was conducted with 40 physicians in the work presented by (35), The predictors they have identified as the best parameters are Sodium, albumin, total bilirubin, platelets, and lymphocytes (laboratory parameters). Further, arthralgia, abdominal pain, myalgia and urine were identified as the best clinical predictors. And the common tropical infections identified in their settings were Dengue, Malaria, Leptospirosis and Scrub Typhus. Binary classification machine learning algorithms were observed providing maximum average of 79%–84% predictability in this analysis out of all ML methods used (i.e., multinomial logistic regression, multi classification ML models etc.). A review has been conducted in (36) based on 159 studies on the vector-borne diseases caused by aedes mosquito, the culex mosquito, the anopheles' mosquito, the triatome bug, the ticks, the lice, the fleas, and the blackflies etc. According to that they have recommended DL models in regular diagnostic predictions. ML and ensemble methods were reviewed in the article (37) disregarding the types of dataset utilized.
We have further reviewed the usage of advanced AI technique for the same purpose and they are presented below.
3.1.1.1 Opportunities and challenges in utilizing advanced AI techniques for tropical disease predictions
Here we present opportunities and challenges in utilizing Advanced AI techniques, except the DL (CNN, RNN and Transformers) and discuss about the models: Generative Adversarial Networks (GANs), Reinforcement Learning (RL), AutoML (Automated Machine Learning), Transfer Learning, Federated Learning, and Attention Mechanisms, etc.
Although some research have focused on infectious disease forecasting with statistical learning and interpretable modeling (38) and some reviews were conducted for analyzing clinical and genomics diagnostics with AI without focusing on diseases (39), here we thoroughly review state-of-the-arts advanced AI techniques available to predict diseases (specifically tropical) and their future perspectives. Here we have classified the advanced AI technique utilized by the research into several categories: Generative Adversarial Networks (GANs), Automated Machine Learning, Reinforcement Learning (RL), Deep Transfer Learning, Federated Learning/Federated Machine Learning and Attention Mechanisms for using omics datasets for tropical and other disease predictions and presented in Table 3.
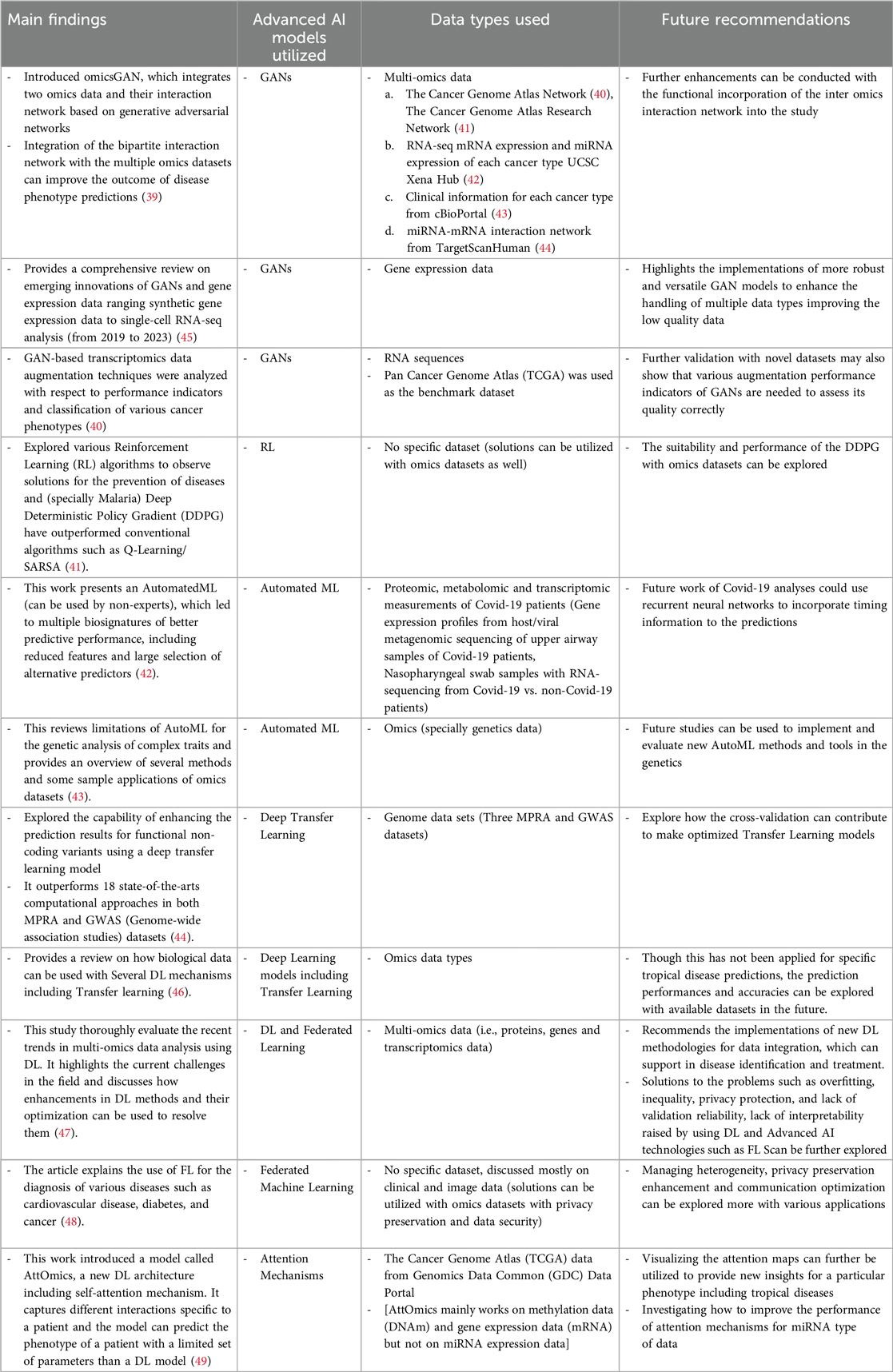
Table 3. Opportunities, challenges and future recommendations on utilizing other advanced AI techniques for omics data processing for tropical and other disease predictions.
According to the Table 1. Following is the summary of the opportunities of utilizing Bioinformatics tools for omics data processing;
The integration of enhanced bioinformatics tools and AI-enabled platforms is improving diagnostic tools, early detection techniques, real-time monitoring, and surveillance of infectious disease pandemics. By utiliazing patient-specific omics datasets, personalized medicine techniques are being developed, which improves vaccine development by the identification of novel antigens and immune responses.
From all the existing opportunities, key points include:
• AI for multi-omics: Multi-omics data, combined with ML and AI techniques, can provide detailed profiling of various biological processes, facilitating disease diagnosis, prognosis, and personalized medicine.
• Enhanced Diagnostics and Disease Surveillance: Early detection and prediction (i.e., outbreaks) can be improved through advanced diagnostic tools and surveillance methods.
• Personalized Medicine: Genetic profiles can be utilized in tailoring personalized treatments, with multiple omics data by enabling personalized pharmaceutical approaches and optimizing therapeutic strategies.
• Vaccine Development: Comprehensive antigen discovery has advanced vaccine design, including both emerging and re-emerging pathogens, improving our knowledge of pathogen-host interactions.
• Novel Diagnostics and Therapeutics: The identification of new biomarkers and drug targets supports the development of novel diagnostics and targeted therapies.
• Understanding Pathogen Evolution: Insights on pathogen evolution and resistance mechanisms can be enhanced.
• Open-Source Tools and Databases: Researchers can use the available resources to explore diseases such as cancer, neurodegenerative diseases, and aging, and to discover drug targets.
• Natural Product Identification: Bioinformatics tools are good for the identification of natural products for handling vector-borne diseases.
• Improved Diagnostic Accuracy: Integrative analysis of different omics datasets leads to more diagnostic accuracy and prognostic models.
To summarize, the integration of multiple omics data and more advanced bioinformatics is providing significant advancements in disease diagnosis, personalized medicine, vaccine development, and identifying and gain knowledege of complex diseases.
According to the Table 1. following is the summary of the limitations and challenges of utilizing Bioinformatics tools for analyzing and integration of omics datasets
• Data Complexity and Variability: High complexity and variability of omics dataset require advanced computational methods for comprehensive analysis. The integration of different datasets has limitations due to issues such as data noise, scalability, and heterogeneity.
• Ethical and Privacy Concerns: Handling sensitive health datasets involves important ethical considerations, including privacy issues.
• Access and Infrastructure: There exist limitations in accessing annotated, high-quality, data for training AI-assisted models. Several regions, including lower-income and low-resource tropical areas, facing constraints in infrastructure and technical support.
• Cost: The high expenses of sequencing and data analysis leads limiting widespread application.
• Data Standardization and Interoperability: Issues in data standardization and interoperability slow down the integration process of diverse datasets.
• Computational Resources: Limitations in processing and analyzing huge omics datasets i.e., requirements of enough computational resources and robust algorithms.
• Experimental Validation: Some molecular experiments are required for clinical validation of findings, and converting these findings into clinical practice needs more analysis.
• Clinical Application: Issues in developing stable and interpretable biomarkers for clinical purpose is complex, and validating the efficiency and safety of newly discovered biomarkers requires more research and experiments.
• “One Health” Approach: Utilizing the “One Health” approach to communicable and non-communicable diseases shows some challenges.
• Natural Product Identification: Identifying relevant natural products from huge biological datasets and validating their efficacy, reliability and safety needs further research.
• Proper Data Management: Managing the complexity of high volume multi-omics data, ensuring their accuracy and reliability of computational predictions, and normalizing omics data to remove biases while protecting biological variation are some limitations.
To summarize, the successful integration and application of omics data require some improved computational methods, good analysis, ethical considerations of data, sufficient infrastructure, and enough research efforts for validation and clinical developments. Further, if the real-world applicability of these opportunities considered, mainly the heterogeneity and the complexity of the omics data play a major role. While doing the integration, this complexity can lead in several difficulties to integrate datasets in a meaningful way. For example if we try to integrate proteomics and transcriptomics data for an analysis of a specific disease, due to the complexity of the data it prevents from meaningful analysis. It may need some advanced computational techniques and careful normalization techniques to overcome this issue. Without proper handling of this, it can result in incomplete and biased analyses. Furthermore, there is a significant challenge in real world usage of omics data due to different data formats available or lacking of a standardized data formats for data storage. Firstly, this can be resolved by adopting researchers to utilize universal data formats of omics data. Then, more expert knowledge and knowledge dissemination can solve this problem to some extent by supporting the implementations of metadata standards. Further, development of data conversion tools and pipelines is also required in some instances. For instance, some bioinformatics platforms Galaxy, Bioconductor, and Nextflow provide integrated environments. They can process multiple formats and transform into compatible formats through workflows. As most of the AI models act as black boxes, lacking of interpretability is a major issue in understanding the rationale behind the clinical settings. Therefore, it should be managed with the collaborations between the experts in the fields. Further, explainable AI can be utilized with omics data sources to resolve this problem. LIME (Local Interpretable Model-agnostic Explanations) and SHAP (SHapley Additive exPlanations) are two famous model-agnostic methods that can be used to interpret predictions made by the current complex AI models such as Deep Neural Networks or transformers. In addition to this, as the omics data is highly sensitive they may limit the availability of the data for research purposes or ethical clearance should be received in order to utilize the data for AI model training and validation. To address the challenges of infrastructure and omics data accessibility in tropical and resource-poor regions, funding support can be provided by encouraging the collaborations between governments as well as deploying the low cost technology and infrastructure in resource- constrained settings. Further, leveraging cloud computing for omics data access can be facilitated for these regions to overcome this issue. Furthermore, organizing training programmes and workshops for the local universities and other educational institutions may enhance the technical expertise in the region as well.
According to the Table 2. AI-enabled advancements in omics data for tropical disease identifications are significantly improving different aspects of genome editing, personalized medicine, and healthcare. Major points include:
• Genome Editing and CRISPR: AI-assistnce enhances the accuracy of genome editing tools and speed up the design of guide RNAs (gRNAs). This increases the success rate of CRISPR interventions and makes treatments for genetic diseases more effective and success.
• Therapeutic Development: AI assists the design and implementation of new therapeutic approaches personalized to individual genetic makeups. This improves patient outcomes.
• Innovation in Healthcare: The integration of AI-assisted genome editing holds the possibility for groundbreaking innovations in healthcare/biomedicine, accelerating target identification and analysis processes from years to months. It facilitates the identification of high confidence therapeutic targets for several diseases, including ALS.
• Precision Medicine: AI enhances the biomarker identification for precision medicine and the personalized therapy development. It improves the diagnostic accuracy and efficiency via XAI models.
• Pharmacometrics and Quantitative Systems Pharmacology (QSP): AI and ML based identifications enhance pharmacometrics and workflows of QSP. It further provides insights into drug discovery and improves reliability of AI-assisted systems for healthcare tools and applications.
• Personalized Medicine: AI-assisted models accelerate the identification of novel biomarkers and drug targets. It further leads to improve the diagnostics and personalized medicine. Furthermore it helps in personalizing cancer treatment plans by improving patient treatments, and reducing their side effects.
• Medical Diagnostics: AI-assisted models enhance diagnostic accuracy as well as efficiency, providing critical analysis on predictions and identifications for disease classification and progression, and bringing up collaboration between AI systems and clinicians.
• Public Health: AI-assisted models support public health delivery through spatial modeling, disease forecasting, misinformation control, risk prediction, surveillance, and epidemic modeling. XAI further enhances healthcare effects by providing high level of transparency and interpretability, allowing clinicians to make better choices.
• Clinical Trials: AI-assisted models can speed up clinical trial tasks, such as sample size reduction and improvement of enrollment, and can identify matching candidates and predict responses to different therapies.
• Big Data and Cloud Computing: The integration of big data, cloud computing, as well as precision medicine improves the usage of AI, with the support of next-generation sequencing for personalized medicine.
In summary, AI-assisted models can revolutionize personalized medicine and targeted treatments, improving accuracy and efficiency of diagnostics and healthcare delivery, and bringing up collaboration between computer scientists and biologists to reveal novel insights from various omics datasets.
Validating AI-assisted models such as transformers and DL based models that use omics data in medical and clinical settings requires precise evaluation methods and techniques to ensure they are effective and secure for use. Though these AI models have the opportunities to revolutionize healthcare, validation in real-world clinical or field settings is crucial and a must to receive regulatory approval. Validating AI-assisted models using omics data, in clinical or field settings needs extensive testing across diverse datasets and environments. It requires both retrospective and prospective validation to verify that models are accurate, generalizable, and capable of improving patient outcomes. To this end, regulatory approval, ethical compliance, and real-world testing are some of the major entities of the validation process. As AI emerges with the time, the implementation of robust validation frameworks is important integration of these technologies into healthcare successfully.
According to the Table 2. Limitations and challenges of AI-enabled advancements in omics data for tropical disease identifications are:
Ethical Considerations: Ensure data privacy and receiving proper ethical consent are critical.
Technological Integration: Integrating AI-assisted models with genome editing technologies shows some significant challenges.
Computational Requirements: High computational nrequirements and the need of high-quality data for AI model training are essential.
Safety and Reliability: Controlling off-target problems and ensuring the safety and reliability of AI-assisted genome editing procedures are very much needed.
Multi-Omics Data: Integrating and analyzing large-scale multi-omics data provides some computational limitations and challenges.
Model Accuracy: Accuracy and reliability of AI-assisted predictions in real-world scenarios is critical.
High Costs and Infrastructure: High computational costs, infrastructure requirements, and data quality issues are important and should be addressed.
Model Interpretability: DL models limit their clinical acceptance and trust. Developing interpretable models without performance loss is complex.
Scalability and Generalizability: Problems exist in ensuring scalability and generalizability of explainable AI (XAI) models across different diseases.
Clinical Integration: Combining AI into clinical workflows involves in high data privacy, regulatory compliance, ethical considerations, and considerable investment in technology advancements and training for healthcare professionals.
Data Quality and Security: Accessing high-quality datasets, ensuring patient data security and managing computational complexity are critical.
AI in Clinical Practice: AI chatbots face difficulties to handle complex patient histories and ensuring more comprehensive information gathering.
In summary, addressing these challenges is important to succeed in integration of AI in genome editing as well as in healthcare. According to the Table 4, we have identified significant opportunities in Vision Transformer (ViT) based Implementations for Omics Data Processing for disease predictions. It includes;
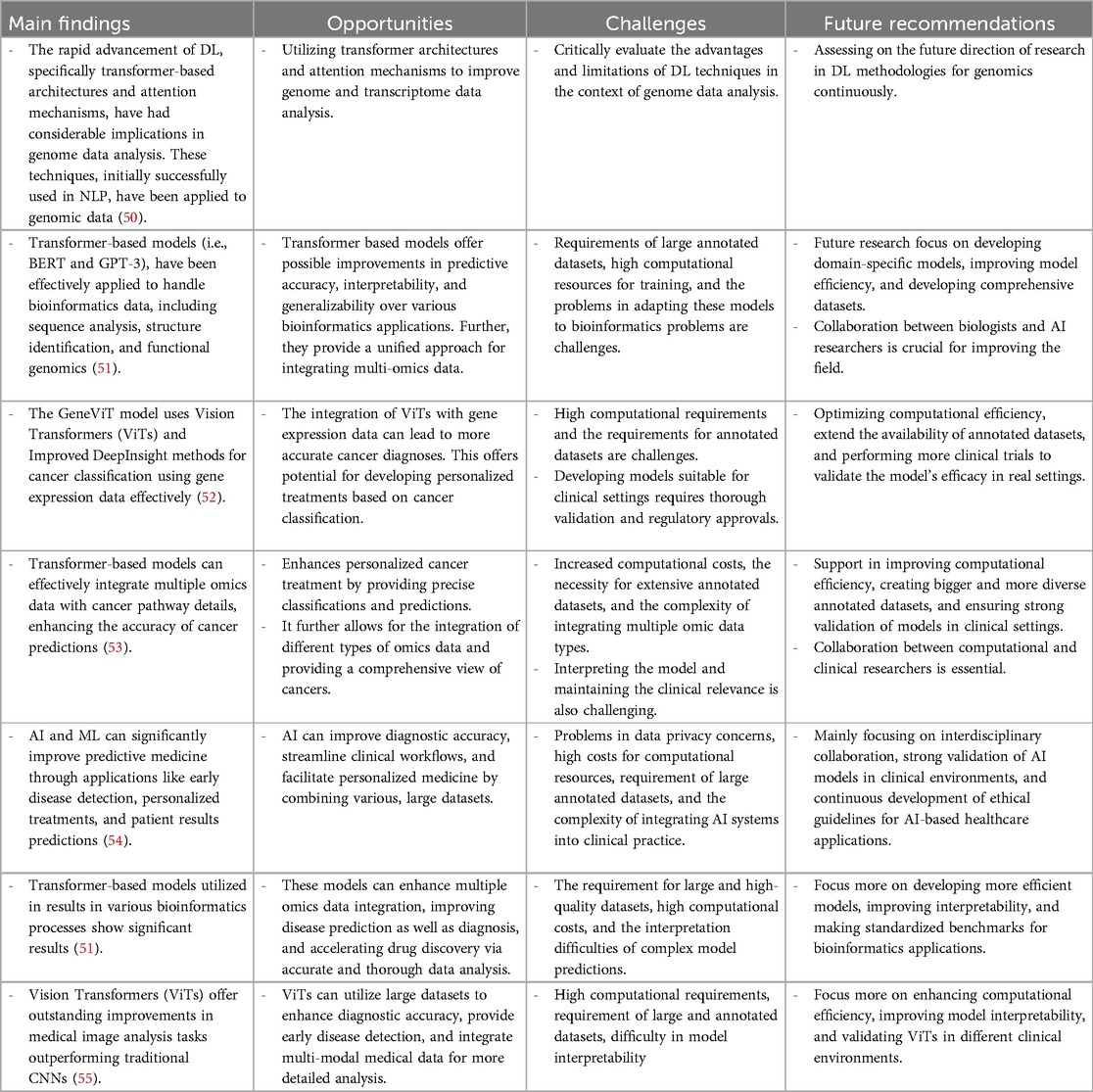
Table 4. Opportunities, challenges and future recommendations of past research on utilizing vision transformer (ViT) based implementations for omics data processing for disease predictions.
Transformer architectures and attention mechanisms have various significant opportunities in bioinformatics data analysis including genomics and transcriptomics. These models can improve interpretability, accuracy, and generalizability. Further, they offer a unified approach for integrating multi-omics data. By integrating recent Vision Transformers (ViTs) with gene expression data, cancer diagnoses can be conducted with significant accuracy, enabling personalized cancer treatments via precise classifications and predictions. In addition, transformers allow comprehensive cancer analysis with the integration o various types of omics datasets. AI further enhances diagnostic accuracy, provides clinical workflows, and facilitates personalized medicine by utilizing large volumes of datasets. These models can improve the process of multi-omics data integration, disease prediction, and diagnosis, and further accelerate drug discovery via data analysis. ViTs are utilized in improving diagnostic accuracy, enabling early disease detection, and integrate multi-modal medical data for detailed analysis.
DL techniques for genome data analysis provide several advantages with notable limitations. These techniques can improve predictive accuracy and reveal complex patterns in large datasets. However, they request large and annotated dataset and high computational resources for training, which can be challenging to obtain. Integrating DL models into bioinformatics applications and clinical environments necessitates thorough validation and regulatory approvals. The complexity of integrating multiple omic data types and high computational costs are significant bottlenecks. Interpretation of the model outputs and keeping track of clinical relevance also haven significant limitations. In addition, data privacy concerns, high costs for computational resources, and the difficulty of integrating AI systems into clinical practice further complicates the utilization of DL models.
According to the Table 3, we observed various space of improving the existing models and following observations and highlights were made for future research improvements. This is one of our main contributions in this review as well.
- Implementation of more robust and versatile GAN meodels may allow handling multi-omics data/multiple data types improving the low quality data
- Assessing the quality of GANs in a correct way requires various augmentation performance indicators
- The applicability and the performance of Deep Deterministic Policy Gradient (an advanced Reinforcement algorithm) with omics data have to be explored
- Managing heterogeneity, privacy preservation improvements and communication optimization can be explored more with various datasets and applications using Federated Machine Learning
- Exploration of cross-validation contribution to make optimized Transfer Learning models can be observed
- Attention mechanisms can be utilized with attention maps to visualize more on how omics data can be used to do accurate predictions of tropical diseases
4 Results and conclusion
Based on our comprehensive review (under Table 1) we observed the following main findings (summary) of utilizing Bioinformatics tools for Omics Data Processing for tropical disease predictions.
1. The integration of multiple omics data improves biomarker identification, disease predictions including disease outbreak predictions, such as in the study of Hirschsprung's disease (HSCR) and vector-borne diseases. Further, it provides treatment methods from the support of ML and AI techniques.
2. Bioinformatics resources like GenBank, Uniprot, EuPathDB, and tools such as BLAST and ClustalW, Clustal Omega are commonly used in infectious disease research. They assist a lot in significant advancements.
3. Bioinformatics tools and techniques lead to considerable progress in pathogen characterization, vaccine development, monitoring progression of pathogen evolution, and host responses in infectious disease research.
4. Integration of multi-omics data assists scientific community to identify new relationships between biomolecules and disease-phenotypes. Further, it may establish biomarkers in detail, as well as investigate signaling pathways.
5. The integration of bioinformatics with genomics and proteomics data speed up the processes of antigen discovery, utilizing high-throughput techniques to identify potential vaccine candidates.
6. Molecular epidemiology tools can mainly contribute to identify disease distribution, etiological agents, and outbreak tracking, importantly in tropical-infectious diseases.
7. Recent advances in omics technologies have made them more accessible and cost-effective, with new fields like lipidomics offering insights into environmental and genetic factors.
8. Some Bioinformatics tools play a critical role in translating omics data into meaningful interpretations and conclusions. This enables the analysis of large-scale epidemiological data to support in public health decision-making.
According to our comprehensive review (under Table 2) we observed the main findings (summary) of utilizing AI assisted techniques for Omics Data Processing for tropical disease predictions. AI-assisted models can improve the precision, cost-effectiveness, and efficiency of CRISPR-based gene editing, optimizing gRNA design, and enabling advanced genetic correction techniques (i.e., base, epigenome, and prime editing). Several existing platforms like PandaOmics utilize AI-assisted models to identify and repurpose therapeutic targets and biomarkers efficiently. DL models have shown promising results in disease identification, with XAI methods like Grad-CAM++ providing human understandable insights by further improving model transparency. AI models has significantly impacted pharmacological research, supporting drug development, public health efforts, and personalized medicine by integrating large datasets for better predictions and diagnoses. However, we still have challenges in data privacy, high computational costs, and model interpretability, which XAI aims to address, improving trust and regulatory approval in several clinical applications.
According to our comprehensive review (under Table 4) we observed the main findings (summary) of utilizing vision transformers and computer vision assisted techniques for Omics Data Processing for tropical disease predictions. The novel and rapid advancements of deep learning, mainly transformer-based architectures and attention mechanisms, have significantly impacted omics data analysis including genome data analysis. Transformer-based models, i.e., BERT and GPT-3, have been mainly applied to bioinformatics data, including sequence analysis and functional genomics. The GeneViT model, utilizing Vision Transformers (ViTs), has shown effectiveness in cancer classification using gene expression data. These models enhance the accuracy of cancer predictions by integrating multiple omics data with cancer pathway details. Additionally, AI and ML applications in predictive medicine, such as early disease detection and personalized treatments, have shown significant improvements.
5 Future directions
Future perspectives for genome data analysis and precision medicine highlight the need for standardized protocols and open-access data sharing to ensure consistency and reproducibility. Investing more in computational settings, infrastructure and AI-assisted Bioinformatics training programs is crucial to build capacity and enhance Bioinformatics and AI applications. Motivating collaborations between Bioinformaticians, clinicians, and other stakeholders can bridge the gap between practical usage and omics data analysis. Ethical concerns, data privacy, and regulatory frameworks should be established to address concerns in AI-enabled genome editing. Developing more sophisticated and interpretable AI models, particularly hybrid models that can balance accuracy and interpretability, will facilitate better clinical integration. Further, open-access databases and collaborative research environments should be promoted to enhance multi-omics integration and applications in precision medicine. Recent advancements in algorithms and computational methods for data fusion and normalization are necessary to handle the complexity of omics data. Utilizing interdisciplinary research and ensuring robust validation of AI-assisted models in clinical settings can improve their reliability as well as generalizability. AI-driven approaches can be integrated into drug discovery workflows, highlighting experimental validation of significant candidates. Furthermore, enhancing AI's role in public health and utilizing ethical AI practices may support the broader adoption of AI-assisted models in healthcare.
Author contributions
SV: Conceptualization, Methodology, Project administration, Writing – review & editing. KW: Methodology, Writing – original draft, Writing – review & editing.
Funding
The author(s) declare that no financial support was received for the research, authorship, and/or publication of this article.
Conflict of interest
The authors declare that the research was conducted in the absence of any commercial or financial relationships that could be construed as a potential conflict of interest.
Publisher's note
All claims expressed in this article are solely those of the authors and do not necessarily represent those of their affiliated organizations, or those of the publisher, the editors and the reviewers. Any product that may be evaluated in this article, or claim that may be made by its manufacturer, is not guaranteed or endorsed by the publisher.
References
1. Karalis VD. The integration of artificial intelligence into clinical practice. Appl Biosci. (2024) 3(1):14–44. doi: 10.3390/applbiosci3010002
2. Gao F, Huang K, Xing Y. Artificial intelligence in omics. Genom Proteom Bioinform. (2022) 20(5):811–3. doi: 10.1016/j.gpb.2023.01.002
3. Saeb ATM. Current bioinformatics resources in combating infectious diseases. Bioinformation. (2018) 14(1):31–5. doi: 10.6026/97320630014031
4. Seoane A, Bou G. Bioinformatics approaches to the study of antimicrobial resistance. Rev Esp Quimioter. (2021) 34(Suppl1):15–7. doi: 10.37201/req/s01.04.2021
5. Chen C, Wang J, Pan D, Wang X, Xu Y, Yan J, et al. Applications of multi-omics analysis in human diseases. MedComm. (2023) 4(4):e315. doi: 10.1002/mco2.315
6. Paszkiewicz KH, Giezen M. Omics, bioinformatics, and infectious disease research. Genet Evol Infect Dis. (2011):523–39. doi: 10.1016/B978-0-12-384890-1.00018-2
7. Leitão JH, Rodríguez-Ortega MJ. Omics and bioinformatics approaches to identify novel antigens for vaccine investigation and development. Vaccines (Basel). (2020) 8(4):653. doi: 10.3390/vaccines8040653
8. Tigistu-Sahle F, Mekuria ZH, Sales GFC, Oliveira CJB, Satoskar AR, Gebreyes WA. Challenges and opportunities of molecular epidemiology: using omics to address Complex one health issues in tropical settings. Front Trop Dis. (2023) 4. doi: 10.3389/fitd.2023.1151336
9. Pham CA, Ngo AD, Le BN, Chu DT. Bioinformatics databases and tools for analysis of multi-omics. In: Mani I, Singh V, editors. Multi-Omics Analysis of the Human Microbiome. Singapore: Springer (2024). p. 77–88. doi: 10.1007/978-981-97-1844-3_4
10. Taijiao J. Bioinformatic approaches to infectious diseases. 3rd world summit on virology, vaccines & emerging diseases. Ann Clin Trials Vaccines Res. (2010) 2(3):70.
11. Lucena-Padros H, Bravo-Gil N, Tous C, Rojano E, Seoane-Zonjic P, Fernández RM, et al. Bioinformatics prediction for network-based integrative multi-omics expression data analysis in Hirschsprung disease. Biomolecules. (2024) 14(2):164. doi: 10.3390/biom14020164
12. Mungmunpuntipantip R, Wiwanitkit V. Bioinformatics approach for searching for natural products in vector-borne disease management. North Clin Istanb. (2024) 11(2):171–6. doi: 10.14744/nci.2023.87523
13. Sun M, Li L, Xiao H, Feng J, Wang J, Wan S. Editorial: bioinformatics analysis of omics data for biomarker identification in clinical research. Volume II. Front Genet. (2023) 14:256468. doi: 10.3389/fgene.2023.1256468
14. Yingzhou L, Yi-Tan C, Eric PH, Guoqiang Y, David MH, Robert C, et al. Integrated Identification of Disease Specific Pathways Using Multi-omics data. (2023). Volume II. doi: 10.1101/666065
15. Tran TO, Vo TH, Lam LHT, Le NQK. ALDH2 As a potential stem cell-related biomarker in lung adenocarcinoma: comprehensive multi-omics analysis. Comput Struct Biotechnol J. (2023) 21:1921–9. doi: 10.1016/j.csbj.2023.02.045
16. Doran S, Arif M, Lam S, Bayraktar A, Turkez H, Uhlen M, et al. Multi-omics approaches for revealing the complexity of cardiovascular disease. Brief Bioinform. (2023) 22(5):bbab061. doi: 10.1093/bib/bbab061
17. Dixit S, Kumar A, Srinivasan K, Vincent PMDR, Ramu KN. Advancing genome editing with artificial intelligence: opportunities, challenges, and future directions. Front Bioeng Biotechnol. (2024) 11:1335901. doi: 10.3389/fbioe.2023.1335901
18. Kamya P, Ozerov IV, Pun FW, Tretina K, Fokina T, Chen S, et al. PandaOmics: an AI-driven platform for therapeutic target and biomarker discovery. J Chem Inf Model. (2024) 64(10):3961–9. doi: 10.1021/acs.jcim.3c01619
19. Chiranjib C, Manojit B, Soumen P, Sang-Soo L. From machine learning to deep learning: advances of the recent data-driven paradigm shift in medicine and healthcare. Curr Res Biotechnol. (2024) 7:100164. doi: 10.1016/j.crbiot.2023.100164
20. Kinger S, Kulkarni V. Explainable AI for deep learning based disease detection. Proceedings of the 2021 Thirteenth International Conference on Contemporary Computing (IC3-2021). New York, NY: Association for Computing Machinery (2021). p. 209–16. doi: 10.1145/3474124.3474154
21. Shruti S, Rajesh K, Shuvasree P, Sunil KS. Artificial intelligence and machine learning in pharmacological research: bridging the gap between data and drug discovery. Cureus. (2023) 15(8):e44359. doi: 10.7759/cureus.44359
22. Olawade DB, Wada OJ, David-Olawade AC, Kunonga E, Abaire O, Ling J. Using artificial intelligence to improve public health: a narrative review. Front Public Health. (2023) 11:1196397. doi: 10.3389/fpubh.2023.1196397
23. Hulsen T. Explainable artificial intelligence (XAI): concepts and challenges in healthcare. AI. (2023) 4(3):652–66. doi: 10.3390/ai4030034
24. Toussaint PA, Leiser F, Thiebes S, Schlesner M, Brors B, Sunyaev A. Explainable artificial intelligence for omics data: a systematic mapping study. Brief Bioinform. (2023) 25(1):bbad453. doi: 10.1093/bib/bbad453
25. Terranova N, Renard D, Shahin MH, Menon S, Cao Y, Hop CECA, et al. Artificial intelligence for quantitative modeling in drug discovery and development: an innovation and quality consortium perspective on use cases and best practices. Clin Pharmacol Ther. (2024) 115(4):658–72. doi: 10.1002/cpt.3053
26. Hui WL, Chui PO, Silvia S, Prabal DB, Filippo M, Rajendra A. Application of explainable artificial intelligence for healthcare: a systematic review of the last decade (2011–2022). Comput Methods Programs Biomed. (2022) 226:107161. doi: 10.1016/j.cmpb.2022.107161
27. Baciu C, Xu C, Alim M, Prayitno K, Bhat M. Artificial intelligence applied to omics data in liver diseases: enhancing clinical predictions. Front Artif Intell. (2022) 5:1050439. doi: 10.3389/frai.2022.1050439
28. Santorsola M, Lescai F. The promise of explainable deep learning for omics data analysis: adding new discovery tools to AI. New Biotechnol. (2023) 77:1–11. doi: 10.1016/j.nbt.2023.06.002
29. Krishnan G, Singh S, Pathania M, Gosavi S, Abhishek S, Parchani A, et al. Artificial intelligence in clinical medicine: catalyzing a sustainable global healthcare paradigm. Front Artif Intell. (2023) 6:1227091. doi: 10.3389/frai.2023.1227091
30. Zhang C, Xu J, Tang R, Yang J, Wang W, Yu X, et al. Novel research and future prospects of artificial intelligence in cancer diagnosis and treatment. J Hematol Oncol. (2023) 16:114. doi: 10.1186/s13045-023-01514-5
31. Chen SF, Loguercio S, Chen KU, Lee SE, Park JB, Liu SE, et al. Artificial intelligence for risk assessment on primary prevention of coronary artery disease. Curr Cardiovasc Risk Rep. (2023) 17:215–31. doi: 10.1007/s12170-023-00731-4
32. Can Demirbaş K, Yıldız M, Saygılı S, Canpolat N, Kasapçopur O. Artificial intelligence in pediatrics: learning to walk together. Turk Arch Pediatr. (2024) 59(2):121–30. doi: 10.5152/TurkArchPediatr.2024.24002
33. Tran TO, Vo TH, Le NQK. Omics-based deep learning approaches for lung cancer decision-making and therapeutics development. Brief Funct Genomics. (2024) 15(23):3. doi: 10.1093/bfgp/elad031
34. Shenoy S, Rajan AK, Rashid M, Chandran VP, Poojari PG, Kunhikatta V, et al. Artificial intelligence in differentiating tropical infections: a step ahead. PLoS Negl Trop Dis. (2022) 16(6):e0010455. doi: 10.1371/journal.pntd.0010455
35. Kaur I, Sandhu AK, Kumar Y. Artificial intelligence techniques for predictive modeling of vector-borne diseases and its pathogens: a systematic review. Arch Comput Methods Eng. (2022) 29:1–31. doi: 10.1007/s11831-022-09724-9
36. Rayner A, Joe HO. The roles of machine learning methods in limiting the spread of deadly diseases: a systematic review. Heliyon. (2023) 7(6). doi: 10.1016/j.heliyon.2021.e07371
37. Stockdale JE, Liu P, Colijn C. The potential of genomics for infectious disease forecasting. Nat Microbiol. (2022) 7:1736–43. doi: 10.1038/s41564-022-01233-6
38. Dias R, Torkamani A. Artificial intelligence in clinical and genomic diagnostics. Genome Med. (2019) 11:70. doi: 10.1186/s13073-019-0689-8
39. Ahmed KT, Sun J, Cheng S, Yong J, Zhang W. Multi-omics data integration by generative adversarial network. Bioinformatics. (2021) 38(1):179–86. doi: 10.1093/bioinformatics/btab608
40. Alice L, Michèle S, Blaise H. GAN-based data augmentation for transcriptomics: survey and comparative assessment. Bioinformatics. (2023) 39(1):i111–20. doi: 10.1093/bioinformatics/btad239
41. Khan ZA, Feng Z, Uddin MI, Mast N, Shah SAA, Imtiaz M, et al. Optimal policy learning for disease prevention using reinforcement learning. Sci Program. (2020) 1:7627290. doi: 10.1155/2020/7627290
42. Papoutsoglou G, Karaglani M, Lagani V, Thomson N, Røe OD, Tsamardinos I, et al. Automated machine learning optimizes and accelerates predictive modeling from COVID-19 high throughput datasets. Sci Rep. (2023) 11(1):15107. doi: 10.1038/s41598-021-94501-0
43. Manduchi E, Romano JD, Moore JH. The promise of automated machine learning for the genetic analysis of complex traits. Hum Genet. (2022) 141:1529–44. doi: 10.1007/s00439-021-02393-x
44. Chen L, Wang Y, Zhao F. Exploiting deep transfer learning for the prediction of functional non-coding variants using genomic sequence. Med Image Anal. (2022) 38(12):3164–72. doi: 10.1093/bioinformatics/btac214
45. Lee M. Recent advances in generative adversarial networks for gene expression data: a comprehensive review. Mathematics. (2023) 2023(11):3055. doi: 10.3390/math11143055
46. Tsimenidis S. Omics data and data representations for deep learning-based predictive modeling. Int J Mol Sci. (2022) 23:12272. doi: 10.3390/math11143055
47. Wekesa JS, Kimwele M. A review of multi-omics data integration through deep learning approaches for disease diagnosis, prognosis, and treatment. Front Genet. (2023) 14:1199087. doi: 10.3389/fgene.2023.1199087
48. Moshawrab M, Adda M, Bouzouane A, Ibrahim H, Raad A. Reviewing federated machine learning and its use in diseases prediction. Sensors. (2023) 23:2112. doi: 10.3390/s23042112
49. Aurélien B, Milad RV, Franck A, Farida Z, Blaise H. Attomics: attention-based architecture for diagnosis and prognosis from omics data. Bioinformatics. (2023) 39:i94–i102. doi: 10.1093/bioinformatics/btad232
50. Choi SR, Lee M. Transformer architecture and attention mechanisms in genome data analysis: a comprehensive review. Biology (Basel). (2023) 12(7):1033. doi: 10.3390/biology12071033
51. Zhang S, Fan R, Liu Y, Chen S, Liu Q, Zeng W. Applications of transformer-based language models in bioinformatics: a survey. Bioinform Adv. (2023) 3(1):vbad001. doi: 10.1093/bioadv/vbad001
52. Madhuri G, Sraban KM, Aparajita O. Genevit: gene vision transformer with improved DeepInsight for cancer classification. Comput Biol Med. (2023) 155:106643. doi: 10.1016/j.compbiomed.2023.106643
53. Wang J, Liao N, Du X, Chen Q, Wei B. A semi-supervised approach for the integration of multi-omics data based on transformer multi-head self-attention mechanism and graph convolutional networks. BMC Genomics. (2024) 25:86. doi: 10.1186/s12864-024-09985-7
54. Sharma A, Lysenko A, Jia S, Boroevich KA, Tsunoda T. Advances in AI and machine learning for predictive medicine. J Hum Genet. (2024). doi: 10.1038/s10038-024-01231-y
Keywords: genomics, transcriptomics, proteomics, tropical, bioinformatics, AI, vision transformers, advanced AI
Citation: Vidanagamachchi SM and Waidyarathna KMGTR (2024) Opportunities, challenges and future perspectives of using bioinformatics and artificial intelligence techniques on tropical disease identification using omics data. Front. Digit. Health 6:1471200. doi: 10.3389/fdgth.2024.1471200
Received: 30 July 2024; Accepted: 6 November 2024;
Published: 25 November 2024.
Edited by:
Anwar Musah, University College London, United KingdomReviewed by:
Nguyen Quoc Khanh Le, Taipei Medical University, TaiwanZahra Mungloo, University of Mauritius, Mauritius
Copyright: © 2024 Vidanagamachchi and Waidyarathna. This is an open-access article distributed under the terms of the Creative Commons Attribution License (CC BY). The use, distribution or reproduction in other forums is permitted, provided the original author(s) and the copyright owner(s) are credited and that the original publication in this journal is cited, in accordance with accepted academic practice. No use, distribution or reproduction is permitted which does not comply with these terms.
*Correspondence: S. M. Vidanagamachchi, c212QGRjcy5ydWguYWMubGs=