- 1Columbia University School of Nursing, New York, NY, United States
- 2Department of Biomedical Informatics, Columbia University, New York, NY, United States
- 3Department of Population Health Sciences, Weill Cornell Medicine, New York, NY, United States
- 4College of Agriculture and Life Sciences, Cornell University, Ithaca, NY, United States
- 5Department of Obstetrics and Gynecology, Weill Cornell Medicine, New York, NY, United States
- 6Department of Psychiatry, Weill Cornell Medicine, New York, NY, United States
Introduction: Artificial intelligence (AI) is being developed for mental healthcare, but patients' perspectives on its use are unknown. This study examined differences in attitudes towards AI being used in mental healthcare by history of mental illness, current mental health status, demographic characteristics, and social determinants of health.
Methods: We conducted a cross-sectional survey of an online sample of 500 adults asking about general perspectives, comfort with AI, specific concerns, explainability and transparency, responsibility and trust, and the importance of relevant bioethical constructs.
Results: Multiple vulnerable subgroups perceive potential harms related to AI being used in mental healthcare, place importance on upholding bioethical constructs, and would blame or reduce trust in multiple parties, including mental healthcare professionals, if harm or conflicting assessments resulted from AI.
Discussion: Future research examining strategies for ethical AI implementation and supporting clinician AI literacy is critical for optimal patient and clinician interactions with AI in mental healthcare.
Introduction
Artificial intelligence (AI) technologies are increasingly being developed to predict, diagnose, and treat disease. While still in the formative stages of development, AI implementation is increasing in pace across health systems and will soon be widespread. Within mental healthcare, a range of datasets has been used, from electronic health records and imaging data to social media and novel activity and mood monitoring systems, to predict outcomes (1). AI has also been used in a generative manner to summarize information, provide feedback for patient questions, or automate documentation. For example, predictive AI can evaluate the risk of postpartum depression in settings where screening is limited, and generative AI can deliver cognitive-behavioral therapy via chatbot (2, 3). Because rich information is stored in clinical encounter notes so often in mental health contexts, much of this work also leverages natural language processing, a set of computational methods to extract patterns from large sources of textual data (1). In the context of limited mental health resources and mental health professionals, AI technologies provide the opportunity to expand access for patients and offload the tasks clinicians find most burdensome so they may instead focus on the patient relationship that is so unique and essential for psychiatry (4).
Patient perspectives on the use of AI in mental healthcare are critical but missing. As patient engagement and shared decision-making continue to rise in importance, it is likely that patients will interact with AI-generated risk prediction models during the course of their care (5). Moreover, AI-informed chatbots are a potential modality for delivering therapy (3). In addition to gathering general perspectives, it will be important to examine differences in perspectives based on experiences with health and the healthcare system, such as experiences with mental healthcare, implicit bias resulting from one's identity, or social determinants of health (SDOH) that influence access to care and health outcomes.
Moreover, important ethical questions arise when using AI in mental healthcare relating to issues of bias, privacy, autonomy, and distributive justice (6). For example, the broad range of health-related data that may be used to train AI algorithms may alarm patients and undermine trust. For example, mental health researchers are already attempting to correlate keystrokes and voice data captured from smartphones to mood disorders (7), which patients may be unaware of. Moreover, these datasets may have systematic biases that perpetuate inequities in who is able to access mental healthcare or experience positive mental health outcomes (8). AI could also lead to harm when instructions on appropriate reliance are not communicated to patients; for example, researchers have raised concerns about patients developing a perceived therapeutic alliance with chatbots that could cause them to over-rely on chatbot guidance or undermine the therapeutic alliance they have with their mental health professionals (3). A synthesis of the ethics literature on responsible AI (6), consumer-generated data (9), AI in psychiatry (10), and maternal health (11, 12) reveals six important constructs to be considered in this context: autonomy, beneficence/non-maleficence, justice, trust, privacy, and transparency.
However, to date, AI research has focused almost exclusively on presenting model output and fostering trust in AI among clinicians (13). The information needs and ethical considerations among patients likely differ from those of clinicians, but may not be the same for all patients; they may differ by a patient's mental health history, demographic variables, and SDOH (14). Therefore it is important to provide insight to the psychiatry community regarding patients' nuanced perspectives on AI, so that it may be effectively integrated into clinical care in a way that does not degrade the patient relationship. Several prior studies have reported on patients' perceptions of AI in healthcare broadly, which have included a range of concerns and highlighted the importance of understanding and integrating patient views into AI development and deployment (15–17). Within mental healthcare specifically, patient perspectives on AI are comparatively understudied, but early research suggests patients are optimistic. For example, a recent scoping review of patient attitudes towards chatbots in mental healthcare reported that patients have positive perceptions but require high-quality, guideline-concordant, trustworthy and personalized interactions to feel comfortable using them in their care (18). However, differences in attitudes towards AI in mental healthcare by clinical or sociodemographic characteristics remain understudied.
The primary objective of this study was to determine whether a history of mental illness or current mental health status is associated with differences in attitudes towards AI being used in mental healthcare. Secondarily, we also aimed to investigate differences by demographic characteristics (age, gender, race, and ethnicity) and SDOH (financial resources, education, health literacy, and subjective numeracy).
Methods
Sample and study design
This study involved a cross-sectional survey administered to an online sample of U.S. adults in September 2022. Participants were recruited using Prolific (19), a web-based recruitment and survey administration platform. Inclusion criteria were: (1) age 18 or older and (2) able to complete the survey in English. Using Prolific's survey sampling strata, we recruited a sample balanced on age, gender, and race reflecting the U.S. demographic distributions (20). The Weill Cornell Medicine (WCM) Institutional Review Board approved this study.
Data collection
Survey questions addressed six domains relating to attitudes toward AI. Survey items were developed with input from experts in AI, human-centered design, and psychiatry, and some were based on a prior study of general (non-mental health) AI uses in healthcare (21). The topics included general perspectives (baseline knowledge and general attitudes towards AI in mental health), comfort with the use of AI in place of mental health professionals for various tasks and with data sharing for AI purposes, specific concerns regarding the use of AI for mental healthcare, explainability and transparency of how the model works and what data are used, impacts on trust in clinicians and responsibility for harms from AI, and the importance of the six relevant bioethical constructs in this context. Additionally, we collected sociodemographic characteristics, health literacy measured with the Chew Brief Health Literacy Screening Questions (22), subjective numeracy measured with the 3-Item Version of the Subjective Numeracy Scale (23), and mental health history from all participants. Mental health history was assessed with a single item: “Has a trained health professional ever told you that you have any mental illness?” with an explanation of what was considered a trained health professional.
Data were collected using a secure local instance of Qualtrics survey software. Eligible participants were recruited via Prolific and directed to complete the Qualtrics survey. Participants provided informed consent prior to initiating the survey and were compensated at an hourly rate of $13.60 consistent with Prolific policies. At the beginning and throughout the survey, we provided participants with definitions of AI, mental health, clinical depression, and bipolar disorder using lay terms. Additionally, per Prolific recommendations (20), two “attention check” questions were included in the survey to ensure survey respondents were fully reading each question and thoughtfully responding. All survey questions, including the specific attention check questions, are provided in Supplementary File 1.
Statistical analysis
We first assessed data missingness and the time taken to complete the survey. We planned to exclude participants who did not complete the survey, failed both attention check questions, or completed the survey in under three minutes (suggesting inattention to the questions). We computed basic descriptive statistics of mean, frequency, and central tendency to characterize the sample. We used Fisher's Exact tests to compare differences in survey responses by mental health history, current mental health rating, demographic characteristics, and SDOH. Statistical significance (alpha) was set at 0.05. After these omnibus tests of significant differences were run, visual inspections of percentages in the contingency tables were used to identify differences between groups. We used R version 4.2.1 (R Foundation for Statistical Computing, Vienna, Austria, 2022) for the analysis.
Results
Description of the sample
Five hundred participants ultimately completed the survey. Thirty participants opened the survey but did not complete it after reading the consent form, and were therefore excluded from the analysis. No participants failed the attention check questions or completed the survey in under three minutes.
The descriptive characteristics of the sample are described in Table 1. The median age was 46 (interquartile range 31–59). Approximately one-third of the sample was split between Millennials (31%), Generation X (26%), and Baby Boomers (30%). The sample was evenly split by gender, but the majority were White (78%) and non-Hispanic/Latino (93%). One-third reported not having enough financial resources to “make ends meet” (31%) and nearly half had obtained less than a Bachelor's degree (46%). One-quarter had inadequate health literacy (26%) and more than half had low subjective numeracy (57%). Nearly half had a history of mental illness (43%) and nearly one-third rated their current mental health as poor or fair (29%).
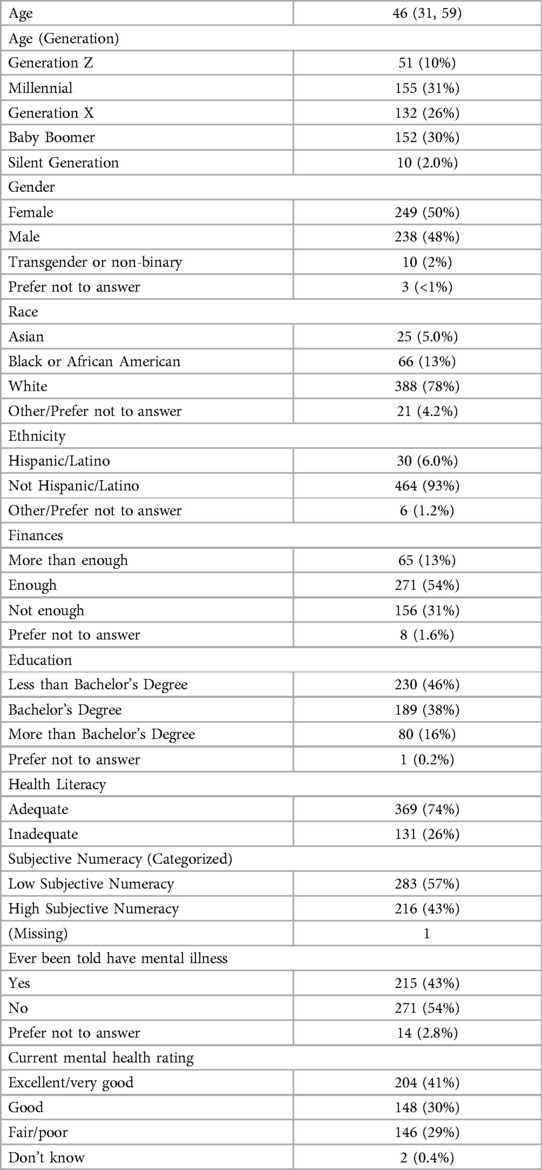
Table 1. Demographic characteristics of survey participants (n = 500); median (interquartile range) or n (%).
Primary endpoints: mental health history and current status
A summary of the differences in responses across all primary and secondary endpoints is summarized in Figure 1. All results (including those that were not statistically significant) are reported in Supplementary File 2.
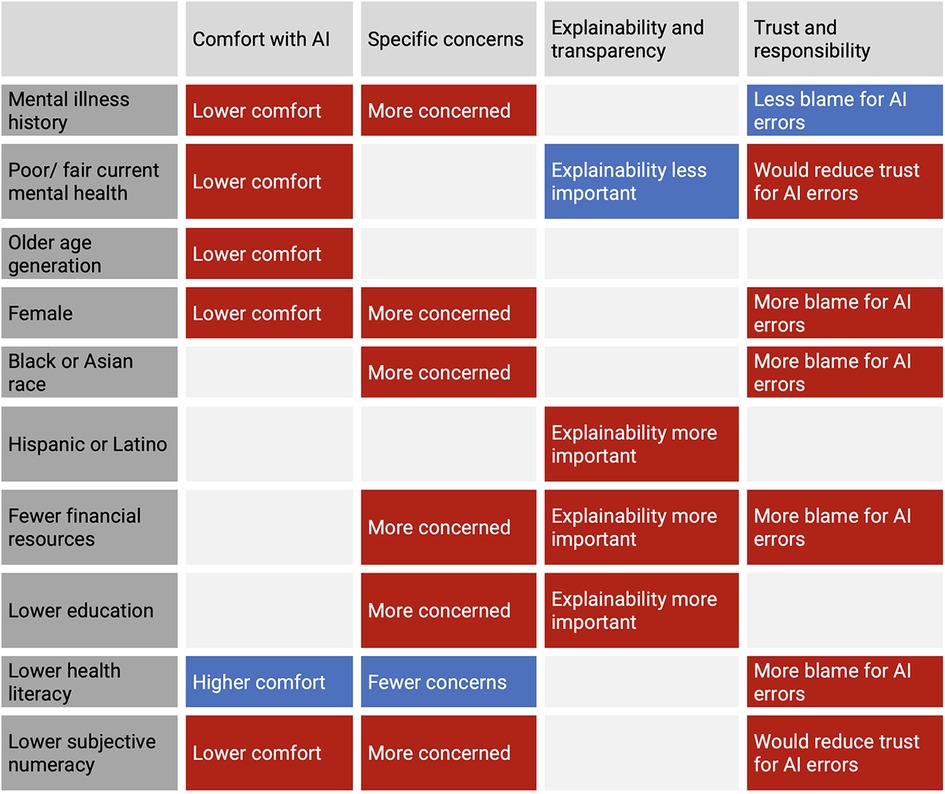
Figure 1. Summary of statistically significant differences in responses across primary (mental health) and secondary (demographic and social determinants of health) endpoints.
Significant differences in perspectives by mental health history and current mental health rating are shown in Figures 2, 3 and Table 2. Compared to those without a history of mental illness, more participants with a history of mental illness were uncomfortable with AI making a diagnosis of depression (p = 0.02) but were more comfortable sharing sensitive information with a mental health professional (p = 0.03). More participants with a history of mental illness were also concerned about AI making a wrong diagnosis (p = 0.03) and endorsed the importance of non-maleficence (p = 0.02) and transparency (p = 0.01). More participants with a history of mental illness did not assign responsibility and blame for errors from AI to various groups, including hospitals (p < 0.01), companies (p = 0.01), and government agencies (p = 0.02), and endorsed that “no one” was to blame (p = 0.03).
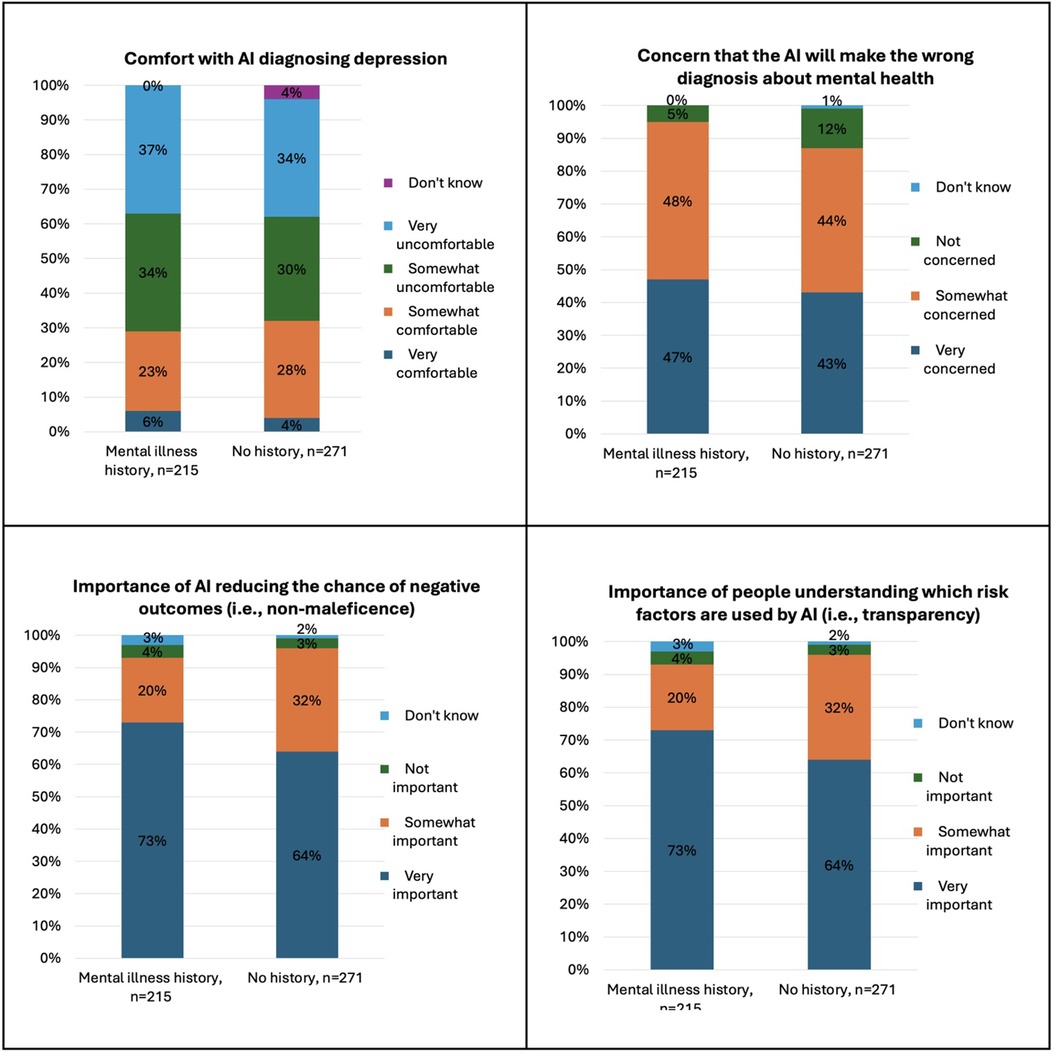
Figure 2. Significant differences in attitudes towards AI in mental healthcare by mental illness history.
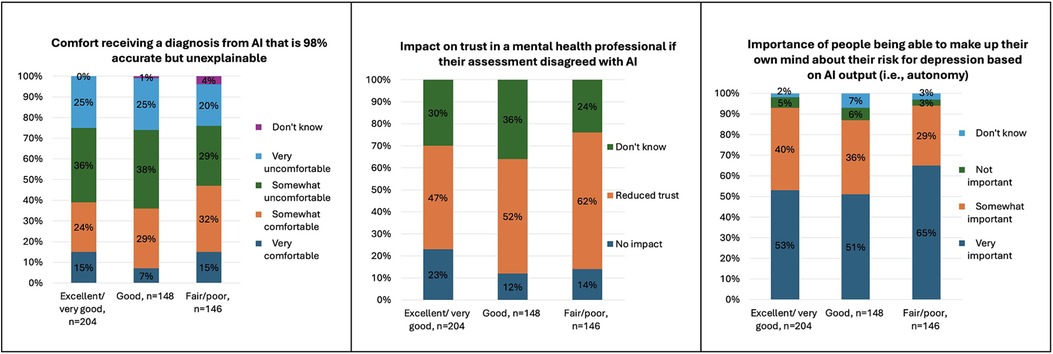
Figure 3. Significant differences in attitudes towards AI in mental healthcare by current self-rated mental health.
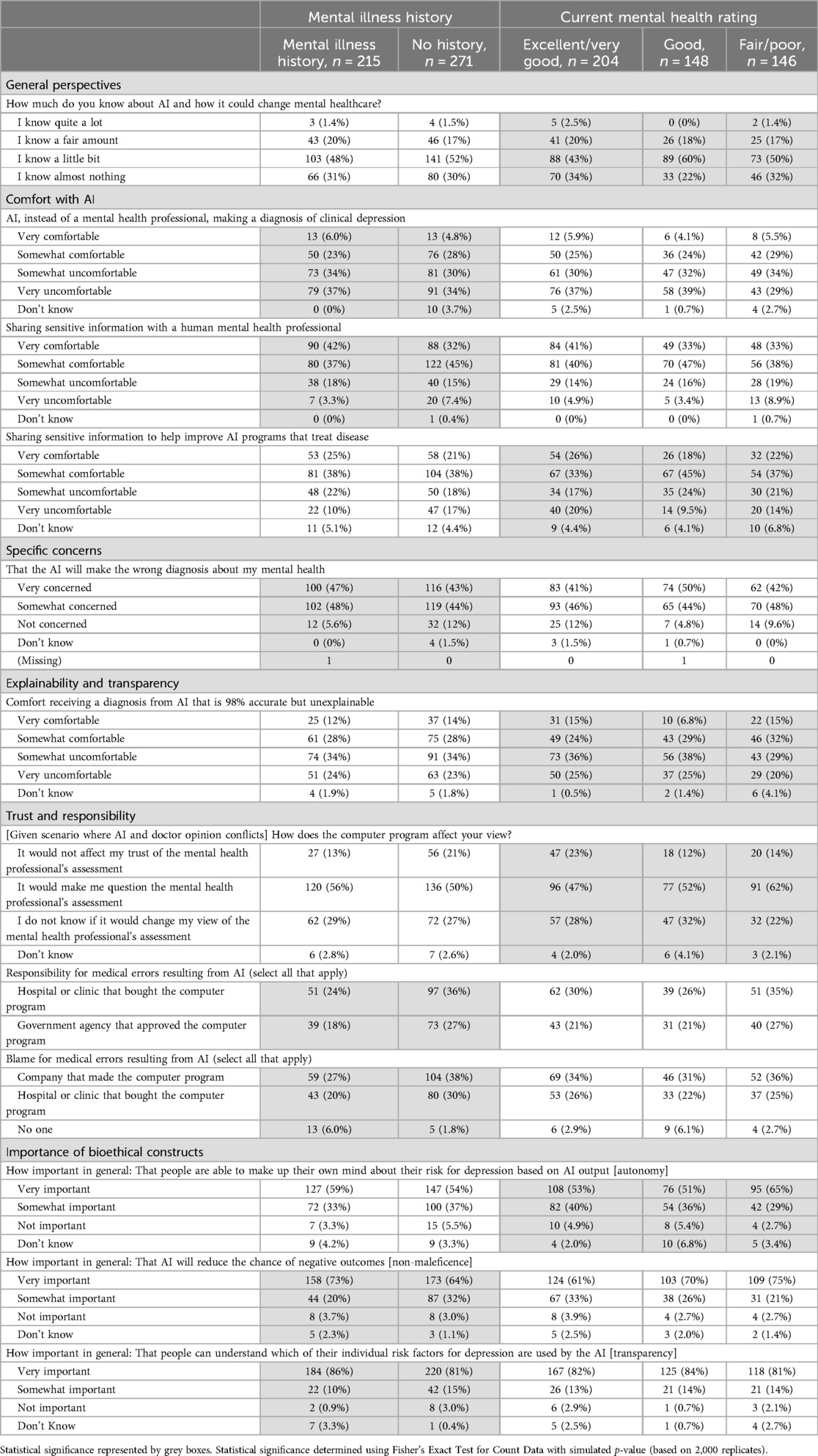
Table 2. Significant differences in perspectives on AI in mental health by mental health history and current rating among survey participants (n = 500); n (%).
Compared to those who rated their mental health as excellent/very good or good, participants who rated their mental health as poor/fair reported low baseline knowledge about AI and how it could change mental healthcare (p = 0.03). More participants who rated their mental health as poor/fair were uncomfortable sharing sensitive information to help improve AI programs that treat disease (p = 0.04), would reduce trust in a mental health professional if their assessment disagreed with AI (p = 0.02) and more were somewhat comfortable receiving a diagnosis from AI that is 98% accurate but unexplainable (p = 0.03).
Secondary endpoints: demographic differences
Significant differences in perspectives by age generation and gender are shown in Table 3. Compared to all other generations, fewer members of the Baby Boomer generation were comfortable with AI making recommendations for a general wellness or stress-management strategy (p < 0.01), talk therapy (p = 0.02), or medications (p < 0.01). Compared to all other generations, more members of Generation Z and the Millennial generation felt it was “not important” that AI not reduce trust in one's mental health care provider (p < 0.01). Compared to men, more women reported low baseline knowledge about AI and how it could change mental healthcare (p < 0.01), were uncomfortable with AI diagnosing bipolar disorder (p = 0.03), and were “very” concerned that AI will lead to mental health professionals not knowing you as well (p = 0.02). Slightly more women were “very” comfortable with AI making recommendations for a general wellness or stress-management strategy (p = 0.02) and would assign responsibility and blame for errors from AI to mental health professionals (p = 0.03).
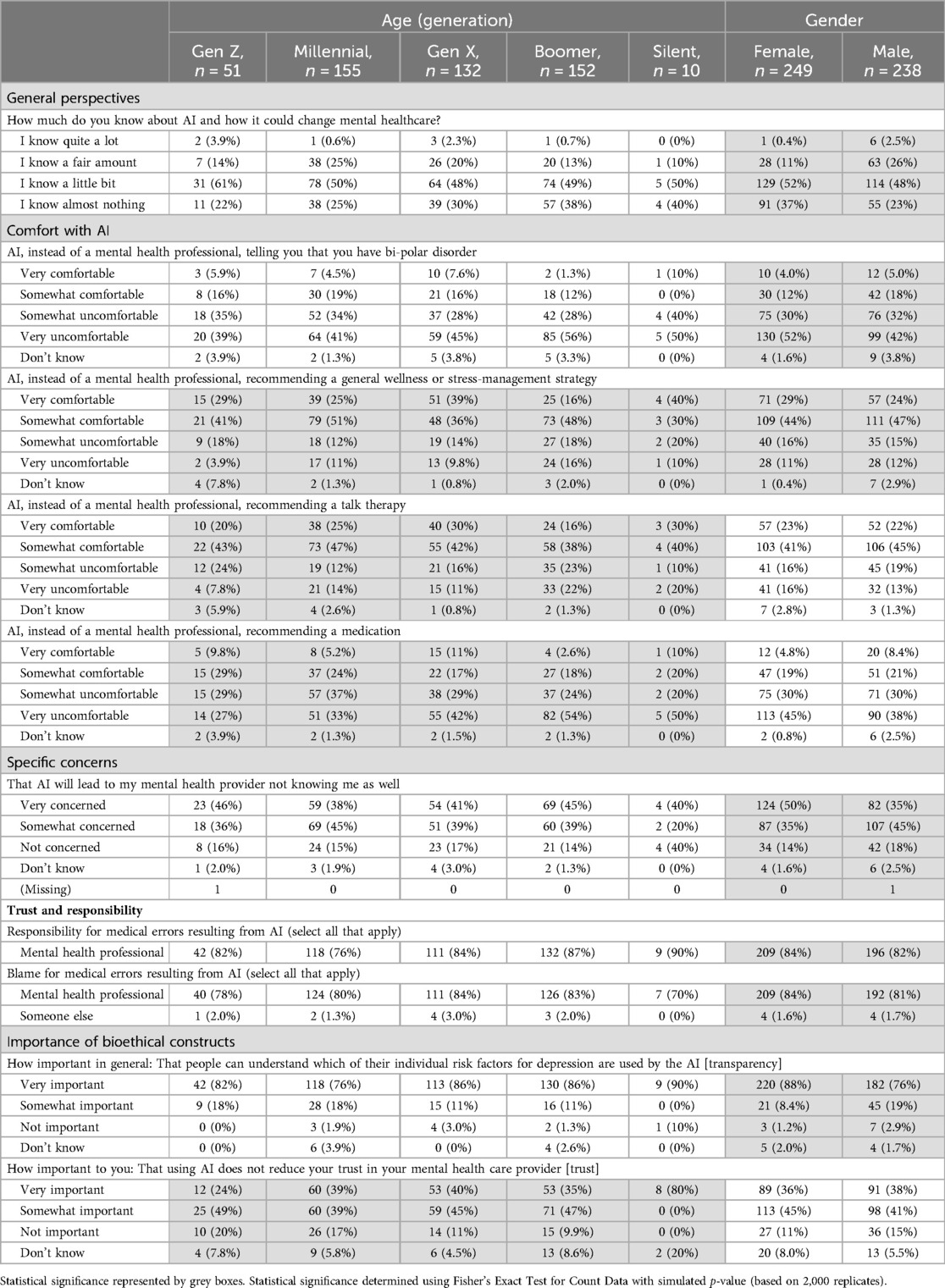
Table 3. Differences in perspectives on AI in mental health by demographic characteristics (age and gender) among survey participants (n = 500); n (%).
Significant differences in perspectives by race and ethnicity are shown in Table 4. Compared to white participants, more Asian and Black participants thought AI would make mental healthcare “much” or “somewhat” better (p = 0.03), but more were also “very” concerned that AI would make a misdiagnosis (p = 0.04) and result in less time with their mental health professional (p = 0.02), would blame hospitals or clinics (p = 0.01) and regulatory governmental agencies for AI errors (p = 0.04). Compared to other racial groups, more Asian participants expect mental health professionals to check for AI safety (p = 0.02). Compared to non-Hispanic/Latino participants, more Hispanic or Latino participants were both “very comfortable” and “very uncomfortable” (but not “somewhat”) receiving a mental health diagnosis from AI that was 98% accurate but unexplainable.
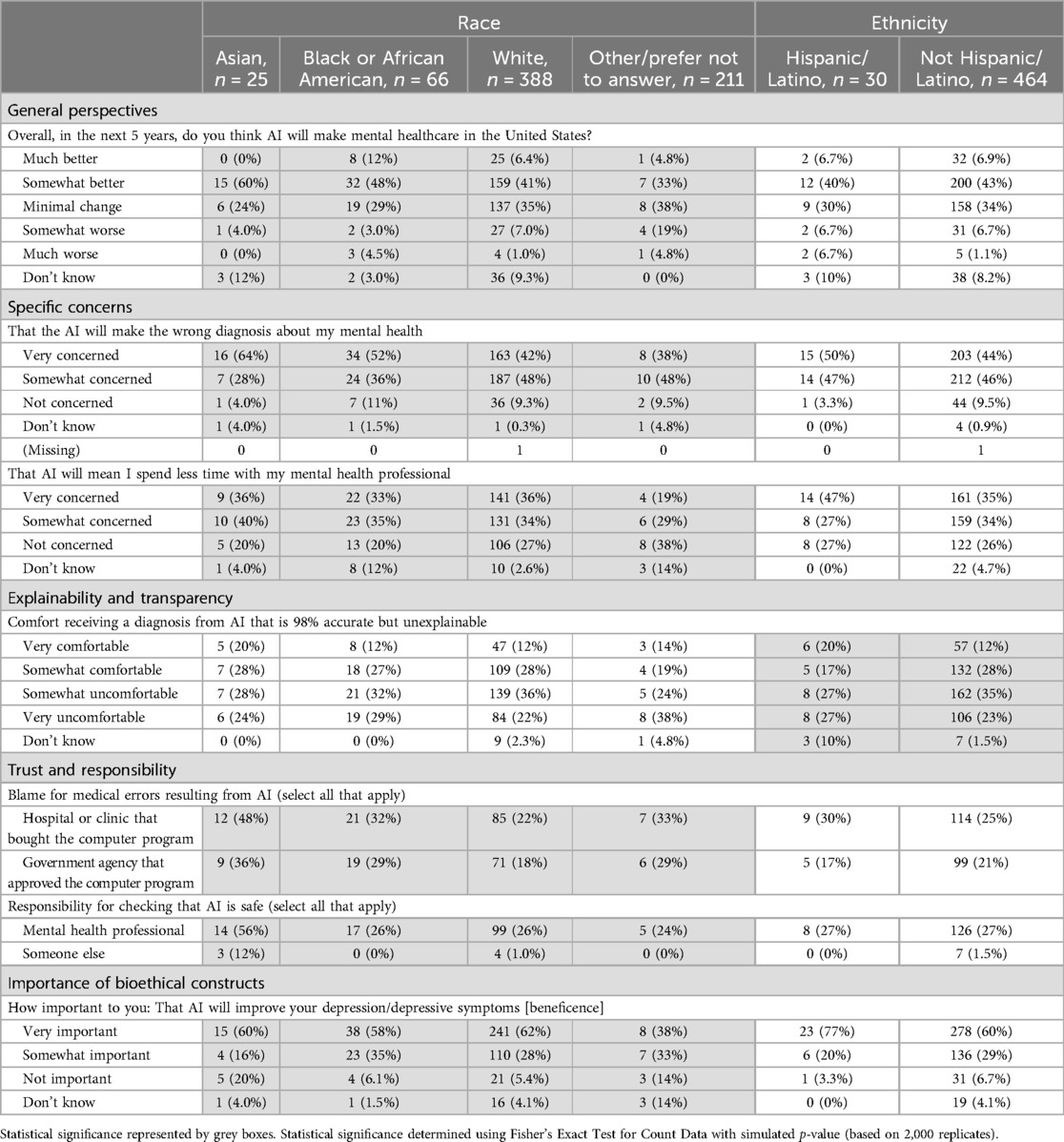
Table 4. Differences in perspectives on AI in mental health by demographic characteristics (race and ethnicity) among survey participants (n = 500); n (%).
Secondary endpoints: social determinants of health
Significant differences in perspectives by financial resources and education are shown in Table 5. Compared to those with “more than enough” financial resources, more participants with “enough” and “not enough” were “very” concerned about AI misdiagnosis (p = 0.04) and mental health costs (p < 0.01), and were “somewhat” or “very” uncomfortable receiving a mental health diagnosis from both a 90% accurate but unexplainable AI (p = 0.01) and a 98% accurate but unexplainable AI (p = 0.04). More participants with “enough” and “not enough” also placed greater importance on AI being explainable (p = 0.04) and reducing negative outcomes (p < 0.01). Compared to those with a Bachelor's or higher, more participants with less than a Bachelor's degree were “very” concerned that AI will increase mental health costs (p < 0.01), “very” uncomfortable receiving a mental health diagnosis from AI that was 98% accurate but unexplainable (p = 0.03), and “did not know” who was responsible for harm caused by AI (p = 0.02).
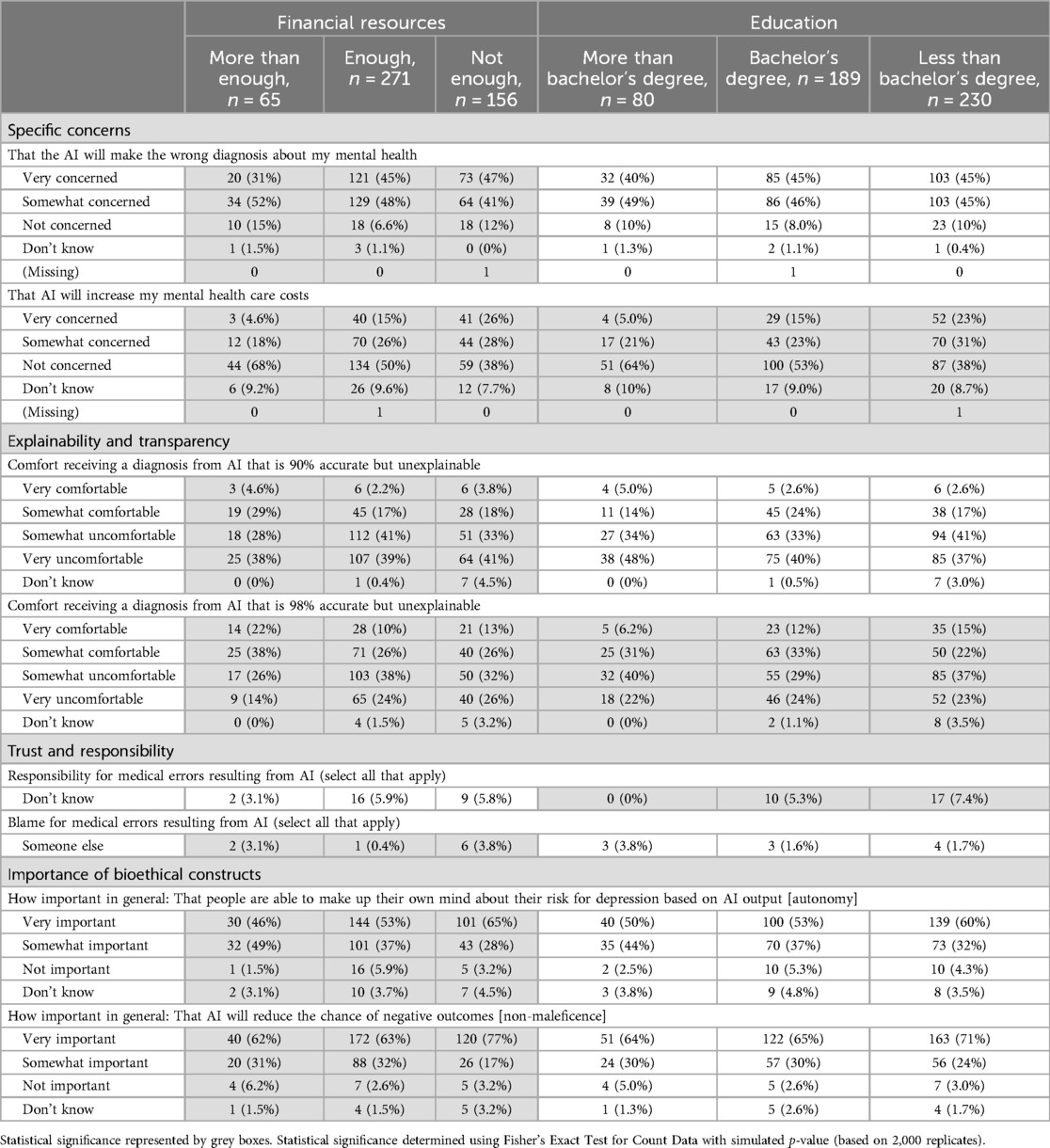
Table 5. Differences in perspectives on AI in mental health by social determinants of health (SDOH; financial resources and education) among survey participants (n = 500); n (%).
Significant differences in perspectives by health literacy and subjective numeracy are shown in Table 6. Compared to those with adequate health literacy, more participants with inadequate health literacy thought that AI would make mental healthcare “much” or “somewhat” better (p = 0.01), were not concerned about AI leading to spending less time with a mental health professional (p < 0.01), were “somewhat” comfortable sharing sensitive information with an AI chatbot (p = 0.01) and to help improve AI that treats mental health conditions (p < 0.01), and endorsed that privacy was “not important” both in general (p < 0.01) and to them personally (p = 0.02). However, more participants with inadequate health literacy also felt hospitals or clinics are to blame for harm caused by AI (p = 0.02), and had mixed responses regarding their comfort in receiving a diagnosis from AI that is 90% accurate but unexplainable (more endorsed either “somewhat” comfortable or uncomfortable vs. “very”; p = 0.03).
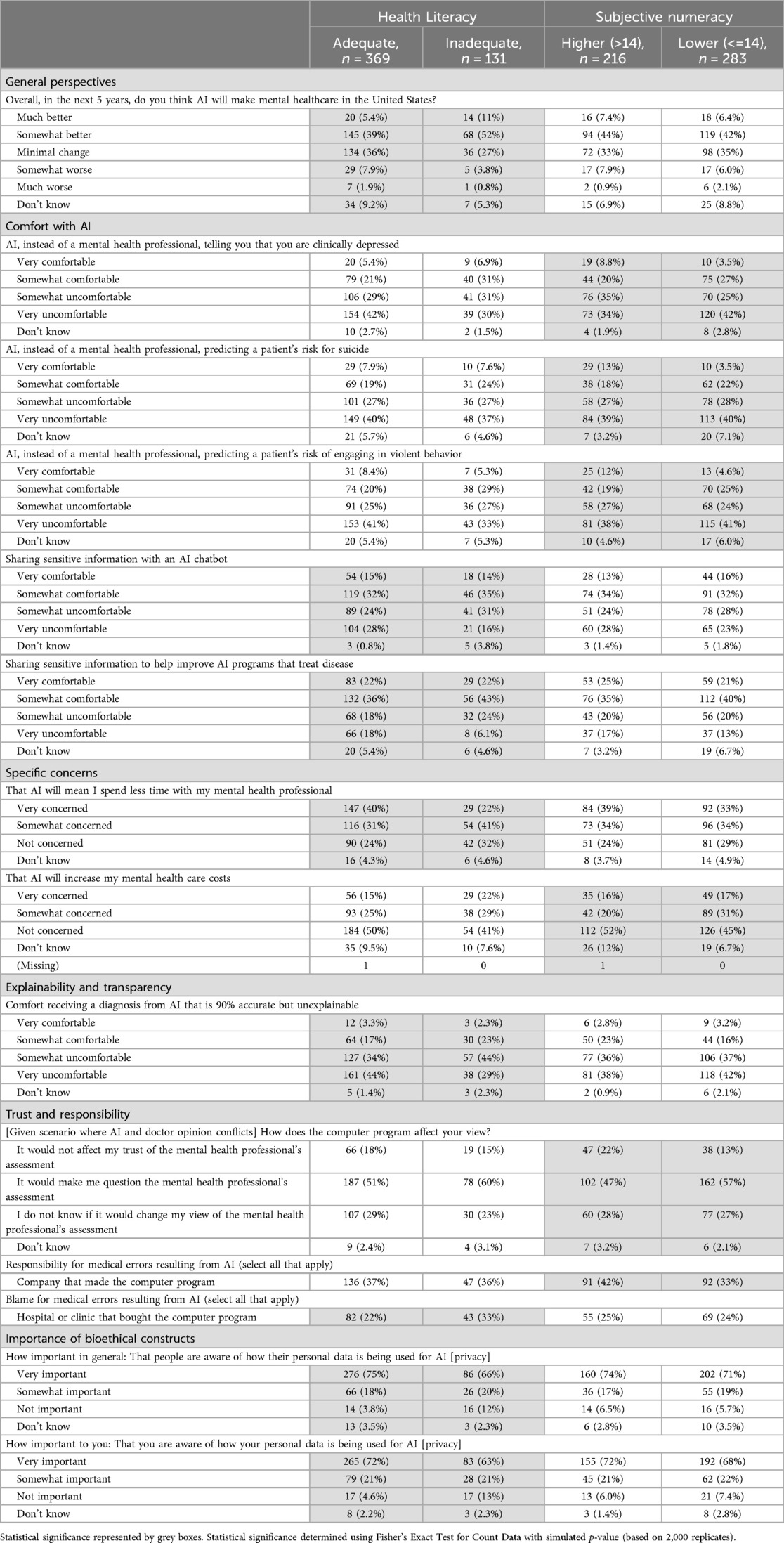
Table 6. Differences in perspectives on AI in mental health by social determinants of health (SDOH; health literacy and subjective numeracy) among survey participants (n = 500); n (%).
Compared to those with high subjective numeracy, more participants with low subjective numeracy were “very” uncomfortable with AI diagnosing depression (p < 0.01), predicting the risk of suicide (p < 0.01), and predicting the risk of engaging in violent behavior (p = 0.03). More participants with low subjective numeracy were also “very” or “somewhat” concerned that AI will increase mental health costs (p < 0.01), and would reduce trust in a mental health professional if their assessment disagreed with AI (p = 0.04), but fewer would blame the company making the AI for harm caused by AI (p = 0.03).
Discussion
In this analysis of 500 U.S. adults, significant differences in perceptions of AI in mental healthcare were found by mental health history, current mental health status, demographic characteristics, and SDOH. In general, participants with a history of mental illness, members of older generations (e.g., Baby Boomers), those identifying as women, Asian, Black, Latinx, those reporting “not enough” financial resources, those with less than a Bachelor's degree in education, and those with lower subjective numeracy expressed greater discomfort with various potential uses of AI in mental healthcare and expressed greater concerns about potential harms from AI in mental healthcare. Similarly, more participants with a history of mental illness, who rated their current mental health as poor or fair, members of older generations (e.g., Baby Boomers), those who identified as women, and those reporting “not enough” financial resources felt at least one of the bioethical constructs were “very important.” Paradoxically, participants with inadequate health literacy were more comfortable with AI, less concerned about its potential harms, and rated some of the bioethical constructs as “less important.”
This study is among the first to describe differences in attitudes towards AI by mental health history; to our knowledge, this has not been previously reported. However, our findings do align with prior research on patient perspectives on AI in healthcare more broadly, in which individuals reporting good to excellent health are less concerned and more comfortable with AI in healthcare compared to those with poorer health status (24). Our findings on differences in attitudes by other characteristics generally align with the small but growing body of literature in the area. Specifically, in prior studies, women, older and racially and ethnically minoritized adults, and those with lower education, socioeconomic status, and/or digital literacy, express greater concern about the use of AI in mental healthcare, particularly surrounding privacy, accuracy, lack of transparency, loss of human empathy and connection, and financial costs (24, 25).
These findings highlight significant concerns among multiple vulnerable populations about the use of AI in mental healthcare. At the same time, they are unsurprising in the context of historical failures to consider and integrate the needs of vulnerable populations when emerging technologies are developed and integrated into healthcare systems (26). For example, there are known and persistent disparities in the adoption and use of patient portals (27), telehealth (28), and wearable technologies (29, 30) by many of the groups of patients who expressed concerns and discomfort with AI in this study. In fact, the finding that lower health literacy participants did not perceive these threats suggests that these concerns could be realized, as the patients least able to find, understand, and use health-related information are the least aware or concerned about AI-related harms. Future research should examine the intersectionality of multiple vulnerabilities and its impact on perceptions of AI in mental healthcare, given that important differences along a matrix of personal characteristics may exist that were not uncovered in this analysis.
In this study, participants’ perceptions of who is responsible or to blame for harm resulting from AI, who is responsible for ensuring the safety of AI, and the impacts on trust with mental health professionals were inconsistent across vulnerable groups. In general, more participants who identified as Asian or Black, reported “not enough” financial resources, or had inadequate health literacy assigned responsibility or blame to hospitals or clinics, regulatory governmental agencies, mental health professionals, and/or other groups for harm resulting from AI. More participants who rated their current mental health as poor or fair, and those with lower subjective numeracy, would also reduce trust in their mental health professional if their assessment disagreed with AI. However, more participants with lower education were unsure about who was responsible, fewer participants with a history of mental illness would assign responsibility or blame to hospitals or clinics, regulatory governmental agencies, and AI companies, and fewer participants with lower subjective numeracy would blame AI companies for harms caused by AI.
Given the speed with which AI is being embraced across many disciplines within healthcare, including mental healthcare, and the perspective among some groups of patients that mental health professionals are responsible, hold blame, or are less trustworthy as a result of harm or conflicting information from AI, AI literacy among clinicians is imperative. AI literacy calls for clinicians to understand data governance, basic statistics, data visualization, and the impact of AI on clinical processes (31). Mental health professionals will need to be able to continue practicing in an effective, efficient, safe, and equitable way in the context of AI-enriched healthcare systems. Perhaps more importantly, it will be essential that AI will not be used, inadvertently or otherwise, to extend existing biases that create disparities between patients in timely and accurate mental health diagnoses and care. Their perspectives will also be critical as those developing AI systems for mental healthcare aim to create more accurate systems, for example using deep learning methods, while combating the “black box” problem of limited explainability (1, 3). Similarly, reviews have demonstrated that AI in mental healthcare is in an early, proof-of-concept stage; in future work, the engagement of mental health professionals, along with patients and caregivers, will be critical to successful clinical implementation in ways that do not overburden clinicians (1).
AI-related competencies for clinicians have been proposed; Russell et al. (32) identified six competencies including basic knowledge of AI, social and ethical implications, workflow analysis for AI-enabled systems, evidence-based evaluation of AI-based tools, conducting AI-enhanced clinical encounters, and continuing education and quality improvement initiatives related to AI. While both continuing professional development (32) and medical school education (33) have been proposed venues for implementing these competencies, strategies for training the mental health workforce for impending AI interactions and influences remain to be determined (4).
Additionally, the findings of this study suggest mental health professionals may need to be especially prepared for vulnerable patient populations to express greater reticence about AI being used in their care. These conversations will be critical as failure to engage these populations in technologies that have a demonstrated benefit with respect to mental health outcomes could actually widen disparities (26). For example, we found that older generations expressed greater concern and discomfort with specific uses of AI in mental healthcare. This is salient because of the known underdiagnosis of mental illnesses (particularly racial and ethnic minorities), distinct symptom presentation, and lower utilization of mental health services among older adults (34). While AI may be poised to address these issues through improved disease detection, caregiver support, and novel solutions to reduce loneliness, failure to engage older adults could further exacerbate these disparities (34).
Limitations of this study include the limited generalizability of the sample, especially with respect to race and ethnicity. Oversampling of racially and ethnically minoritized adults may be important in future work to better understand differences in attitudes toward AI. Moreover, we only examined overall differences and did not apply corrections to account for multiple comparisons. Further, we did not report on group post-hoc comparisons due to small sample sizes in some cells.
Conclusion
Multiple vulnerable subgroups of U.S. adults perceive potential harms related to AI being used in mental healthcare, place importance on upholding bioethical constructs related to AI use in mental healthcare, and would blame or reduce trust in multiple parties, including mental healthcare professionals, if harm or conflicting assessments resulted from AI. Future research examining strategies to uphold bioethical constructs in AI implementation and support clinician AI literacy is critical for optimal patient and clinician interactions with AI in the future of mental healthcare.
Data availability statement
The raw data supporting the conclusions of this article will be made available by the authors, without undue reservation.
Ethics statement
The studies involving humans were approved by Weill Cornell Medicine Institutional Review Board. The studies were conducted in accordance with the local legislation and institutional requirements. The participants provided their written informed consent to participate in this study.
Author contributions
MR: Conceptualization, Funding acquisition, Investigation, Methodology, Writing – original draft. PD: Investigation, Project administration, Writing – review & editing. SH: Formal Analysis, Investigation, Writing – review & editing. JK: Formal Analysis, Writing – review & editing. SK: Writing – review & editing. YZ: Writing – review & editing. RJ: Writing – review & editing. JP: Funding acquisition, Writing – review & editing. AH: Writing – review & editing. NB: Conceptualization, Funding acquisition, Methodology, Writing – review & editing.
Funding
The author(s) declare financial support was received for the research, authorship, and/or publication of this article. R41MH124581 (NIH/NIMH); R41MH124581-02S1(NIH/NIMH); R00MD015781 (NIH/NIMHD); R00NR019124 (NIH/NINR).
Conflict of interest
MRT, JP, and AH are co-founders and have equity in Iris OB Health, New York.
The remaining authors declare that the research was conducted in the absence of any commercial or financial relationships that could be construed as a potential conflict of interest.
Publisher's note
All claims expressed in this article are solely those of the authors and do not necessarily represent those of their affiliated organizations, or those of the publisher, the editors and the reviewers. Any product that may be evaluated in this article, or claim that may be made by its manufacturer, is not guaranteed or endorsed by the publisher.
Supplementary material
The Supplementary Material for this article can be found online at: https://www.frontiersin.org/articles/10.3389/fdgth.2024.1410758/full#supplementary-material
References
1. Graham S, Depp C, Lee EE, Nebeker C, Tu X, Kim HC, et al. Artificial intelligence for mental health and mental illnesses: an overview. Curr Psychiatry Rep. (2019) 21(11):116. doi: 10.1007/s11920-019-1094-0
2. Zhang Y, Wang S, Hermann A, Joly R, Pathak J. Development and validation of a machine learning algorithm for predicting the risk of postpartum depression among pregnant women. J Affect Disord. (2020) 279:1–8. doi: 10.1016/j.jad.2020.09.113
3. Kellogg KC, Sadeh-Sharvit S. Pragmatic AI-augmentation in mental healthcare: key technologies, potential benefits, and real-world challenges and solutions for frontline clinicians. Front Psychiatry. (2022) 13:990370. doi: 10.3389/fpsyt.2022.990370
4. Kim JW, Jones KL, D’Angelo E. How to prepare prospective psychiatrists in the era of artificial intelligence. Acad Psychiatry. (2019) 43(3):337–9. doi: 10.1007/s40596-019-01025-x
5. Walsh CG, McKillop MM, Lee P, Harris JW, Simpson C, Novak LL. Risky business: a scoping review for communicating results of predictive models between providers and patients. JAMIA Open. (2021) 4(4):ooab092. doi: 10.1093/jamiaopen/ooab092
6. The IEEE Global Initiative on Ethics of Autonomous and Intelligent Systems. Ethically Aligned Design: A Vision for Prioritizing Human Well-being with Autonomous and Intelligent Systems, Version 2. IEEE. Published online 2017. Available online at: http://standards.ieee.org/develop/indconn/ec/autonomous_systems.html (accessed April 01, 2024).
7. Huang H, Cao B, Yu PS, Wang CD, Leow AD. dpMood: exploiting local and periodic typing dynamics for personalized mood prediction. 2018 IEEE International Conference on Data Mining (ICDM) (2018). p. 157–66.
8. Raymond N. Safeguards for human studies can’t cope with big data. Nature. (2019) 568(7752):277. doi: 10.1038/d41586-019-01164-z
9. Skopac EL, Mbawuike JS, Levin SU, Dwyer AT, Rosenthal SJ, Humphreys AS, et al. An Ethical Framework for the Use of Consumer-Generated Data in Health Care. Published online 2019. Available online at: https://www.mitre.org/sites/default/files/publications/A%20CGD%20Ethical%20Framework%20in%20Health%20Care%20final.pdf (accessed April 01, 2024).
10. Doraiswamy PM, Blease C, Bodner K. Artificial intelligence and the future of psychiatry: insights from a global physician survey. Artif Intell Med. (2020) 102:101753. doi: 10.1016/j.artmed.2019.101753
11. Brandon AR, Shivakumar G, Lee SC, Inrig SJ, Sadler JZ. Ethical issues in perinatal mental health research. Curr Opin Psychiatry. (2009) 22(6):601–6. doi: 10.1097/YCO.0b013e3283318e6f
12. Scott KA, Britton L, McLemore MR. The ethics of perinatal care for black women: dismantling the structural racism in “mother blame” narratives. J Perinat Neonatal Nurs. (2019) 33(2):108–15. doi: 10.1097/JPN.0000000000000394
13. Benda NC, Novak LL, Reale C, Ancker JS. Trust in AI: why we should be designing for APPROPRIATE reliance. J Am Med Inform Assoc. (2021) 29(1):207–12. doi: 10.1093/jamia/ocab238
14. Ledford H. Millions of Black People Affected by Racial Bias in Health-Care Algorithms. UK: Nature Publishing Group (2019). doi: 10.1038/d41586-019-03228-6
15. Moy S, Irannejad M, Manning SJ, Farahani M, Ahmed Y, Gao E, et al. Patient perspectives on the use of artificial intelligence in health care: a scoping review. J Patient Cent Res Rev. (2024) 11(1):51–62. doi: 10.17294/2330-0698.2029
16. Richardson JP, Smith C, Curtis S, Watson S, Zhu X, Barry B, et al. Patient apprehensions about the use of artificial intelligence in healthcare. npj Digit Med. (2021) 4(1):1–6. doi: 10.1038/s41746-021-00509-1
17. Adus S, Macklin J, Pinto A. Exploring patient perspectives on how they can and should be engaged in the development of artificial intelligence (AI) applications in health care. BMC Health Serv Res. (2023) 23(1):1163. doi: 10.1186/s12913-023-10098-2
18. Abd-Alrazaq AA, Alajlani M, Ali N, Denecke K, Bewick BM, Househ M. Perceptions and opinions of patients about mental health chatbots: scoping review. J Med Internet Res. (2021) 23(1):e17828. doi: 10.2196/17828
19. Palan S, Schitter C. Prolific.ac—a subject pool for online experiments. J Behav Exp Finance. (2018) 17:22–7. doi: 10.1016/j.jbef.2017.12.004
20. Prolific’s best practice guide. Available online at: https://researcher-help.prolific.co/hc/en-gb/categories/360000850653–Prolific-s-Best-Practice-Guide (Accessed February 24, 2023).
21. Khullar D, Casalino LP, Qian Y, Lu Y, Krumholz HM, Aneja S. Perspectives of patients about artificial intelligence in health care. JAMA Netw Open. (2022) 5(5):e2210309. doi: 10.1001/jamanetworkopen.2022.10309
22. Chew LD, Bradley KA, Boyko EJ. Brief questions to identify patients with inadequate health literacy. Fam Med. (2004) 36(8):588–94.15343421
23. McNaughton CD, Cavanaugh KL, Kripalani S, Rothman RL, Wallston KA. Validation of a short, 3-item version of the subjective numeracy scale. Med Decis Making. (2015) 35(8):932–6. doi: 10.1177/0272989X15581800
24. Antes AL, Burrous S, Sisk BA, Schuelke MJ, Keune JD, DuBois JM. Exploring perceptions of healthcare technologies enabled by artificial intelligence: an online, scenario-based survey. BMC Med Inform Decis Mak. (2021) 21(1):221. doi: 10.1186/s12911-021-01586-8
25. Thakkar A, Gupta A, De Sousa A. Artificial intelligence in positive mental health: a narrative review. Front Digit Health. (2024) 6:1280235. doi: 10.3389/fdgth.2024.1280235
26. Veinot TC, Mitchell H, Ancker JS. Good intentions are not enough: how informatics interventions can worsen inequality. J Am Med Inform Assoc. (2018) 25(8):1080–8. doi: 10.1093/jamia/ocy052
27. Grossman LV, Masterson Creber RM, Benda NC, Wright D, Vawdrey DK, Ancker JS. Interventions to increase patient portal use in vulnerable populations: a systematic review. J Am Med Inform Assoc. (2019) 26(8-9):855–70. doi: 10.1093/jamia/ocz023
28. Valdez RS, Rogers CC, Claypool H, Trieshmann L, Frye O, Wellbeloved-Stone C, et al. Ensuring full participation of people with disabilities in an era of telehealth. J Am Med Inform Assoc. (2020) 28(2):389–92. doi: 10.1093/jamia/ocaa297
29. Colvonen PJ, DeYoung PN, Bosompra NOA, Owens RL. Limiting racial disparities and bias for wearable devices in health science research. Sleep. (2020) 43(10). doi: 10.1093/sleep/zsaa159
30. Dhingra LS, Aminorroaya A, Oikonomou EK, Nargesi AA, Wilson FP, Krumholz HM, et al. Use of wearable devices in individuals with or at risk for cardiovascular disease in the US, 2019 to 2020. JAMA Netw Open. (2023) 6(6):e2316634. doi: 10.1001/jamanetworkopen.2023.16634
31. Wiljer D, Hakim Z. Developing an artificial intelligence-enabled health care practice: rewiring health care professions for better care. J Med Imaging Radiat Sci. (2019) 50(4 Suppl 2):S8–14. doi: 10.1016/j.jmir.2019.09.010
32. Russell RG, Lovett Novak L, Patel M, Garvey KV, Craig KJT, Jackson GP, et al. Competencies for the use of artificial intelligence-based tools by health care professionals. Acad Med. (2023) 98(3):348–56. doi: 10.1097/ACM.0000000000004963
33. Wood EA, Ange BL, Miller DD. Are we ready to integrate artificial intelligence literacy into medical school curriculum: students and faculty survey. J Med Educ Curric Dev. (2021) 8:23821205211024078. doi: 10.1177/23821205211024078
Keywords: artificial intelligence, mental health, patient engagement, bioethics aspects, machine learning
Citation: Reading Turchioe M, Desai P, Harkins S, Kim J, Kumar S, Zhang Y, Joly R, Pathak J, Hermann A and Benda N (2024) Differing perspectives on artificial intelligence in mental healthcare among patients: a cross-sectional survey study. Front. Digit. Health 6:1410758. doi: 10.3389/fdgth.2024.1410758
Received: 1 April 2024; Accepted: 14 October 2024;
Published: 29 November 2024.
Edited by:
Matt V. Ratto, University of Toronto, CanadaReviewed by:
Beenish Chaudhry, University of Louisiana at Lafayette, United StatesMarta Maslej, Centre for Addiction and Mental Health, Canada
Copyright: © 2024 Reading Turchioe, Desai, Harkins, Kim, Kumar, Zhang, Joly, Pathak, Hermann and Benda. This is an open-access article distributed under the terms of the Creative Commons Attribution License (CC BY). The use, distribution or reproduction in other forums is permitted, provided the original author(s) and the copyright owner(s) are credited and that the original publication in this journal is cited, in accordance with accepted academic practice. No use, distribution or reproduction is permitted which does not comply with these terms.
*Correspondence: Meghan Reading Turchioe, bXIzNTU0QGN1bWMuY29sdW1iaWEuZWR1