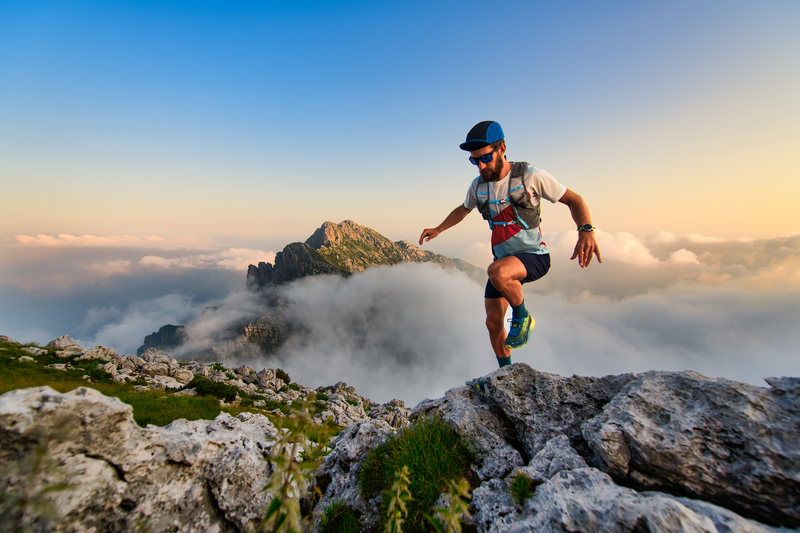
94% of researchers rate our articles as excellent or good
Learn more about the work of our research integrity team to safeguard the quality of each article we publish.
Find out more
OPINION article
Front. Digit. Health , 09 May 2024
Sec. Health Communications and Behavior Change
Volume 6 - 2024 | https://doi.org/10.3389/fdgth.2024.1374359
This article is part of the Research Topic Digital Health Past, Present, and Future View all 19 articles
Human health includes 30%–40% of clinical determinants, the rest of the determinants are genetic, environmental, social and behavioural (1). The genetic and environmental information that contributes to 10%–30% of human health determinants is recorded within an individual's system-biology (2, 3), the multiple biological information entities from the whole collections of genes/proteins/metabolites. Precision and personalized health informatics are scientific areas that utilize system-biology to improve an individual's health. These areas could reach their full potential by designing an architecture of these multiple systems to support the decision-making process in diagnosis, monitoring, and prevention. The recent publication “INTUITION: a data platform to integrate human epilepsy clinical care and support for discovery” (4) has provided an excellent frontier example of the system-biology information, integrated with clinical information. This opinion article responds to this call by suggesting a centralized system with translation and other components that could make these types of integrated systems become utilized in real practice.
The importance and potentials of the system-biology, including proteomics and other omics data in the integrated system, emerge from its final translational phases of the entire pathway, which starts from discovery, prioritization, design, and optimization (5). Discovered and optimized results translated into a health context will enrich their clinical utilization. For example, better clinical pathways for diagnosis, treatment, and prognosis. Precision medicine will take its full potential when the integrated system-biology and health informatics system is established. The instrumental role of system-biology in precision medicine relies on a robust system that can integrate the translational outputs into the daily clinical function; vice versa, the daily clinical functional data will accelerate and improve the precision of the system-biology discovery. To facilitate the complexities of data pathways, data flows, and integration, we need to design a system that will optimize patient outcomes.
After the Human Genome Project (HGP) was completed in year 2003 (6), the HUPO Council started the Human Proteome Project (HPP) (7) in 2009 (8). It aims to map the entire human proteome to understand human biology at the cellular level and establish a foundation for diagnosis, prognostic, therapeutic and preventive medical applications. Gene-centric human proteome mapping has been complemented by in-depth studies of mapping proteomes with physiologic and pathologic states. Both HGP and HPP provided enriched publicly available data for basic and clinical scientists. There were also other emerging individual projects; for example, the Human Protein Atlas project (HPA) has generated a tissue-based map of the human proteome based on transcriptome data, antibody staining and expression of RNA (9). The omics data are ready to be integrated with health informatics data.
The recent enhancement in multi-omics data integration provides relevant functions for translational medicine and new components for integrated health informatics. These tools and methods propose multimodal integration, including supervising and non-supervising approaches, using Frequentist and Bayesian methods from bulk or single cell omics. The functionalities of these tools can be streamlined into:
(1) Disease subtyping and classification, where the patients will be classified at their molecular and multi-omics levels. The discovered subtypes and classification will effectively enhance treatments for patients. Examples of these methods/tools are Patient-specific data fusion (PSDF), iclusters, and Pathway Recognition Algorithm using Data Integration on Genomic Models (PARADIGM).
(2) Prediction of biomarkers for diagnosis and prognosis, where identified multi-omics markers with genotypes and other patient predictors are included in statistical prediction models for risk and clinical outcomes.
(3) Disease biology insight (10) can be obtained through the multi-omics interaction networks (11) and biological pathways to reveal their regulatory processes. Understanding detailed disease mechanisms through multi-omics will help diagnose and derive innovative treatments.
(4) Drug response prediction and repurposing (12) through drug and multi-omics interaction networks (e.g., genes and proteins) (13).
These abovementioned streams use one or a combination of these typical methods: multiple data integration (14), network (15) and cluster approach (16, 17), patient fusion-based (18, 19), similarity-based (20) and other multivariate methods (e.g., Factor analysis, multi-block partial least square regression) (21).
As an example, “IntegratedLearner” (22) is a recent integrated model using a fully Bayesian Ensemble approach for classification and prediction through a multi-layer omics dataset controlling for single-layer omics bias. “IntegratedLearner” uses two-stage feature selection, allowing adjustment for confounding (e.g., environment, lifestyle) effects in both cross-sectional and longitudinal data. GLUE (23) is another recently developed tool for single cell multi-omics data integration. Utilizing prior biological knowledge guidance, it models the regulatory interactions across omics layers.
Multi-omics has many more applications in oncology via different machine learning methods for precision oncology in clinical practice (12). Its utilizations include data integration, statistical analysis, and the creation of Artificial Intelligence tools. Integrated approaches allow for an amplified view of genetic, biochemical, metabolic, proteomic, and epigenetic processes underlying cancer conditions that cannot be comprehended using single-omics approaches.
INTUITION (4) is a deidentified multimodal database platform that integrates system-biology omics data, neuroimaging, electrophysiology (EEG), neuropsychology, cellular (histology), and clinical data. Its system design and user interface include data upload/download, transformation, and data viewers with visualization. The storage units of the system comprise a file store, database, and remote storage. The purpose of the INTUITION platform is to provide an integrated understanding of information curated from biological, functional, clinical, and health data to elucidate the complex mechanism of epilepsy for better treatments. It has utilized the recent breakthrough of system-biology omics data curated from the removal of brain tissue cells. These integrations between different models include the spatial mapping between brain tissues and the electrode position, 3D imaging and omics. Its inventory management of proteins also facilitates the linkage between protein quantities, EEG electrodes, EEG quantified results, and MRI coordinates.
It is an advanced integrated informatics system but has not included human translational results and is designed for research purposes. An integrated system designed for routine clinical practice with a transition to public health will need tools and platforms of translational function, interactions between machine and human data feeds, and data flows with centralized and multiple entry points. Adding translational platforms could be the solution to make the integrated system work within the routine clinical practice.
The components of the system-biology and clinical information integrated system could include the following components described in Table 1, with the interpretation platform being the human-machine interaction portal. Figure 1 visualizes this kind of centralized system, with the potential to add on other home and personal devices, e.g., a neuropsychological assessment (24), a home environmental sensor for motion such as fall detection (sense4safety) (25), and advanced personalized medicine tests, e.g., pharmacogenetic tests (Figure 1. component G). In a centralized, integrated system-biology health informatic system, all data information is entered through different entry points from health providers of central and regional units (Figure 1. component H) and then stored in a central data portal (26). The primary function of the centralized system is to integrate the translational summary derived from system-biology analysis with routine clinical information (Figure 1. component E).
Based on the Common Data Models (CDMS) design of a centralized system for data storage, linkage, and distribution for research and health surveillance (26), an integrated system has a core data portal with different data entry points, multimodal data storage components (e.g., Figure 1. components A and B), data processing platforms for editing/filtering and viewing (Figure 1. component F), and platforms to translate results from system-biology tests (Figure 1. component C). As a function of the CDMS, it will also provide a trigger system to send alarms and notifications to health providers and end-users, including allergy reactions, abnormal drug responses, and adverse events. The user interfaces are designed for both healthcare providers and public users of health services.
An integrated system-biology health informatics system will enhance the full potential of personalized and precision medicine to deliver promising treatment as expected. The complex integration between system-biology and health information requires consideration of optimal infrastructure, security and privacy protection, linkage precision, storage capacities and inequities. The challenge from multi data modality integration in system-biology exists in missing data, inter-omics variations, and large data volume.
Some potential solutions could be considered in the integration:
1. Use standardization and data stewardship to reduce inter-omics variation and optimize data integration.
2. Work with global authorities and experts, such as Health Level Seven International (HL7) (27).
3. Consider co-designing with different end-users (live experience patients and care providers).
4. Consider universal informatics frameworks, such as the FAIR (findable, accessible, interoperable, and reusable) framework (28) to achieve an optimal infrastructure.
5. Set up translational medicine guidelines for data integration, methods, and interpretation for security, privacy protection and better linkage precision (11).
6. Encourage vertical collaborations between basic scientists, clinical scientists, and health professionals to improve interdisciplinary translations.
7. Use Multi-modality design in the multi-omics data integration, Bayesian methods/tools utilizing prior knowledge for coping with missing information and inter-omics variations.
Despite the complexity of integrating system-biology information into routine health informatics, the technologies developed today within these related disciplinary areas are well prepared to integrate them.
IZ: Writing – original draft, Writing – review & editing.
The author(s) declare that no financial support was received for the research, authorship, and/or publication of this article.
The author declares that the research was conducted in the absence of any commercial or financial relationships that could be construed as a potential conflict of interest.
All claims expressed in this article are solely those of the authors and do not necessarily represent those of their affiliated organizations, or those of the publisher, the editors and the reviewers. Any product that may be evaluated in this article, or claim that may be made by its manufacturer, is not guaranteed or endorsed by the publisher.
1. Hsueh P-YS. Ecosystem of Patient-Centered Research and Information System Design. In: Hsueh P-YS, editor. Personal Health Informatics, Cognitive Informatics in Biomedicine and Healthcare. Switzerland: Springer Nature (2022).
2. Bortz WM. Biological basis of determinants of health. Am J Public Health. (2005) 95:389–92. doi: 10.2105/AJPH.2003.033324
3. Determinants of Health. Available online at: Determinantsofhealth.org
4. Maharathi B, Mir F, Hosur K, Loeb JA. INTUITION: a data platform to integrate human epilepsy clinical care and support for discovery. Front Digit Health. (2023) 5:1091508. doi: 10.3389/fdgth.2023.1091508
5. Azer K, Leaf I. Systems biology platform for efficient development and translation of multitargeted therapeutics. Front Syst Biol. (2023) 3:1229532. doi: 10.3389/fsysb.2023.1229532
6. HGP. (2003). Available online at: https://web.ornl.gov/sci/techresources/Human_Genome/project/index.shtml
7. Hupo A. Gene-centric human proteome project: hUPO–the human proteome organization. Mol Cell Proteomics. (2010) 9:427–9. doi: 10.1074/mcp.H900001-MCP200
8. Legrain P, Aebersold R, Archakov A, Bairoch A, Bala K, Beretta L, et al. The human proteome project: current state and future direction. Mol Cell Proteomics. (2011) 10(7):1–5. doi: 10.1074/mcp.M111.009993
9. Jiang L, Wang M, Lin S, Jian R, Li X, Chan J, et al. A quantitative proteome map of the human body. Cell. (2020) 183(1):269–283.e19. doi: 10.1016/j.cell.2020.08.036
10. Subramanian I, Verma S, Kumar S, Jere A, Anamika K. Multi-omics data integration, interpretation, and its application. Bioinform Biol Insights. (2020) 14:1177932219899051. doi: 10.1177/1177932219899051
11. Athieniti E, Spyrou GM. A guide to multi-omics data collection and integration for translational medicine. Comput Struct Biotechnol J. (2023) 21:134–49. doi: 10.1016/j.csbj.2022.11.050
12. Nicora G, Vitali F, Dagliati A, Geifman N, Bellazzi R. Integrated multi-omics analyses in oncology: a review of machine learning methods and tools. Front Oncol. (2020) 10:1030. doi: 10.3389/fonc.2020.01030
13. Vitali F, Cohen LD, Demartini A, Amato A, Eterno V, Zambelli A, et al. A network-based data integration approach to support drug repurposing and multi-target therapies in triple negative breast cancer. PLoS One. (2016) 11(9):e0162407. doi: 10.1371/journal.pone.0162407
14. Kirk P, Griffin JE, Savage RS, Ghahramani Z, Wild DL. Bayesian correlated clustering to integrate multiple datasets. Bioinformatics. (2012) 28(24):3290–7. doi: 10.1093/bioinformatics/bts595
15. Wang B, Mezlini AM, Demir F, Fiume M, Tu Z, Brudno M, et al. Similarity network fusion for aggregating data types on a genomic scale. Nat Methods. (2014) 11(3):333–7. doi: 10.1038/nmeth.2810
16. Shen R, Olshen AB, Ladanyi M. Integrative clustering of multiple genomic data types using a joint latent variable model with application to breast and lung cancer subtype analysis. Bioinformatics. (2009) 25(22):2906–12. doi: 10.1093/bioinformatics/btp543
17. Mo Q, Wang S, Seshan VE, Olshen AB, Schultz N, Sander C, et al. Pattern discovery and cancer gene identification in integrated cancer genomic data. Proc Natl Acad Sci USA. (2013) 110(11):4245–50. doi: 10.1073/pnas.1208949110
18. Yuan Y, Savage RS, Markowetz F. Patient-specific data fusion defines prognostic cancer subtypes. PLoS Comput Biol. (2011) 7(10):e1002227. doi: 10.1371/journal.pcbi.1002227
19. Shi Q, Zhang C, Peng M, Yu X, Zeng T, Liu J, et al. Pattern fusion analysis by adaptive alignment of multiple heterogeneous omics data. Bioinformatics. (2017) 33(17):2706–14. doi: 10.1093/bioinformatics/btx176
20. Nguyen H, Shrestha S, Draghici S, Nguyen T. PINSPlus: a tool for tumor subtype discovery in integrated genomic data. Bioinformatics. (2019) 35(16):2843–6. doi: 10.1093/bioinformatics/bty1049
21. Li W, Zhang S, Liu C-C, Zhou XJ. Identifying multi-layer gene regulatory modules from multi-dimensional genomic data. Bioinformatics. (2012) 28(19):2458–66. doi: 10.1093/bioinformatics/bts476
22. Mallick H, Porwal A, Saha S, Basak P, Svetnik V, Paul E. An integrated Bayesian framework for multi-omics prediction and classification. Stat Med. (2023) 43:983–1002.38146838
23. Cao Z-J, Gao G. Multi-omics single-cell data integration and regulatory inference with graph-linked embedding. Nat Biotechnol. (2022) 40(10):1458–66. doi: 10.1038/s41587-022-01284-4
24. Jimison H, Kos M, Pavel M. Early detection of cognitive decline via mobile and home sensors. In: Hsueh P-YS, Wetter T, Zhu X, editors. Personal Health Informatics. Switherland: Springer Nature (2022). p. 47–170.
25. Demiris G, Richmond TS, Hodgson NA. Smart homes for personal health and safety. In: Hsueh P-YS, Wetter T, Zhu X, editors. Personal Health Informatics. Switherland: Springer Nature (2022). p. 49–61.
26. Podila PSB. Common data models (CDMs): the basic building blocks for fostering public health surveillance and population health research using distributed data networks (DDNs). In: Hsueh P-YS, Wetter T, Zhu X, editors. Personal Health Informatics, Switherland: Springer Nature (2022). p. 267–90.
27. Strasberg HR, Rhodes B, Del Fiol G, Jenders RA, Haug PJ, Kawamoto K. Contemporary clinical decision support standards using health level seven international fast healthcare interoperability resources. J Am Med Inform Assoc. (2021) 28(8):1796–806. doi: 10.1093/jamia/ocab070
Keywords: integrated system-biology, integrated health information system, precision medicine, personalized medicine, centralized integrated system
Citation: Zeng IS (2024) Integrating omics atlas in health informatics system design-an opinion article. Front. Digit. Health 6:1374359. doi: 10.3389/fdgth.2024.1374359
Received: 22 January 2024; Accepted: 22 April 2024;
Published: 9 May 2024.
Edited by:
Himel Mallick, Cornell University, United StatesReviewed by:
Piyali Basak, Merck, United States© 2024 Zeng. This is an open-access article distributed under the terms of the Creative Commons Attribution License (CC BY). The use, distribution or reproduction in other forums is permitted, provided the original author(s) and the copyright owner(s) are credited and that the original publication in this journal is cited, in accordance with accepted academic practice. No use, distribution or reproduction is permitted which does not comply with these terms.
*Correspondence: Irene Suilan Zeng aXJlbmUuemVuZ0BhdXQuYWMubno=
Disclaimer: All claims expressed in this article are solely those of the authors and do not necessarily represent those of their affiliated organizations, or those of the publisher, the editors and the reviewers. Any product that may be evaluated in this article or claim that may be made by its manufacturer is not guaranteed or endorsed by the publisher.
Research integrity at Frontiers
Learn more about the work of our research integrity team to safeguard the quality of each article we publish.